- 1Mediterranean Institute for Advanced Studies, IMEDEA, Esporles, Spain
- 2Department of Physics, University of the Balearic Islands, Palma, Spain
- 3Department of Applied Physics, Technical University of Catalonia, Barcelona, Spain
- 4UNESCO-IHE Institute for Water Education, Delft, Netherlands
In this study, we estimate the shoreline retreat, the vulnerability and the erosion rates of an open beach-dune system under projected sea level rise (SLR) and the action of wind-waves (separately and in combination). The methodology is based on the combination of two state-of-the-art numerical models (XBeach and Q2D-morfo) applied in a probabilistic framework and it is implemented in an open sandy beach in Menorca Island (Western Mediterranean). We compute the shoreline response to SLR during the 21st century and we assess the changing impacts of storm waves on the aerial beach-dune system. Results demonstrate the relevant role that the beach backshore features, such as the berm, play as coastal defense, reducing the shoreline retreat and dune vulnerability rates in the near-term (a few decades ahead) and highlighting the importance of simulating the beach morphodynamic processes in coastal impacts assessments. Our findings point at SLR as the major driver of the projected impacts over the beach-dune system, leading to an increase of ∼25% of the volume eroded due to storm waves by the end of the century with respect to present-day conditions.
Introduction
Projected mean sea level rise (SLR) in response to increased greenhouse gasses concentrations and its combination with land subsidence and other oceanic forces such as wind-waves and storm surges affect the coastal zone, increasing coastal vulnerability, erosion rates and shoreline retreat among other potential impacts (Nicholls, 2002; Idier et al., 2013; Ranasinghe, 2016). These coastal damages are expected to have numerous negative social consequences since the littoral is the most populated zone worldwide (Small and Nicholls, 2003). As a consequence, the economy of the littoral areas will be also affected because of its strong dependence on the coastal environmental resources (Gössling et al., 2012; Bujosa, 2014).
Given the threats that climate change and the associated SLR pose to the coastal regions, the assessments of future coastal vulnerability have become topic research issues in the last years (Nicholls, 2002; Revell et al., 2011; Idier et al., 2013; Le Cozannet et al., 2014; Ranasinghe, 2016; Luijendijk et al., 2018; Sayol and Marcos, 2018) as well as their socio-economic consequences (Small and Nicholls, 2003; Dasgupta et al., 2009; Gössling et al., 2012). Among these, studies focused on sandy shores, mostly beaches, are very common because this type of coasts is subject to a very high level of human utilization (Gössling et al., 2012). One major challenge in predicting the response of beaches to increased mean sea level is their highly dynamic character. This is due to the fact that their morphology responds relatively quickly to changes in the hydrodynamic forces in action (including sea level but also waves, currents and river flows) in many ways. In addition, the morphological state of the beach itself affects the hydrodynamic conditions, leading to a complex feedback between coastal morphology and these forces.
To address these challenges, different approaches have been applied in the literature to assess the beach alterations under projected climate change scenarios. Depending on the hydrodynamic forcing considered and on the tools that are used, several assumptions are necessary in order to simplify the problem. For example, available numerical models are generally able to simulate processes occurring at a single spatio-temporal scale (e.g., one storm event). Thus, their application is limited in the context of climate change (Le Cozannet et al., 2014; Ranasinghe, 2016). When these are used to investigate the continuous long-term beach response, estimations have mostly considered SLR as the unique hydrodynamic forcing, ignoring the contribution of waves (Purvis et al., 2008; Dasgupta et al., 2009). On the contrary, those estimations that consider the marine extreme events (associated to storminess) combined with sea level changes and sediment transport processes, have commonly been performed using statistical methods. In these cases, their main aim is to assess the coastal changes in specific time lapses and neglecting the cumulative effects over time, such as the continuous long-term SLR (Revell et al., 2011; Ranasinghe et al., 2012; de Winter and Ruessink, 2017). When coastal changes are concerned, the estimation of the shoreline retreat is widely used as a proxy of the long-term impact of SLR. One simplified way to assess shoreline changes is the application of the Bruun Rule (Bruun, 1962) that predicts an upward and landward movement of the beach profile, controlled by the beach slope, in response to mean SLR. The Bruun Rule has proved to be a useful tool for rough coastal retreat assessments, but it is less reliable if more accurate estimations are required (Ranasinghe et al., 2012). In addition, the Bruun Rule highly simplifies the cross-shore sediment transport and neglects some morphological processes such as dune erosion. In consequence, there have been some attempts to improve this simplified approach. In this respect, Rosati et al. (2013) proposed a modified form of the Bruun Rule that included, not only the cross-shore sediment transport, but also the aerial sand transport. Other studies have combined SLR with short-term impacts of storm waves and surges, which have been considered in several ways. For instance, Revell et al. (2011) developed a new equation adding the run-up contribution to the Bruun Rule in order to estimate the erosion rates at dunes and cliff areas along the coast of California. They calculated the dune erosion based on the recession associated with a 100-year storm event that takes place at the end of the century using different shore slopes. Another example is found in Wahl et al. (2016) who assessed the erosion and flooding risk in the Gulf of Mexico using a probabilistic model that considered the run-up of wind-waves and the long-term sea level changes. On the other hand, several approaches have focused on the use of numerical models to resolve the local wave propagation and the sediment transport. For instance, de Winter and Ruessink (2017) used XBeach numerical model combined with three climate change SLR projections and three time horizons (years 2050, 2085, and 2200) to assess the dune erosion. They investigated the beach-dune system effects caused by changes in wave direction on the Dutch coasts. Ranasinghe et al. (2012) developed a simplified process-based model to estimate the coastal recession and the volume of dune erosion due to SLR and single storm events in Narrabeen Beach, Australia. They performed a simulation of the combined sea level and storm wave effects and the sediment transport. Similarly, Idier et al. (2013) investigated the vulnerability of sandy coasts in the near term at four beaches in France in a short-term simulation using three different numerical models.
Overall, coastal impact assessments on sandy coasts usually compute erosion volume and/or shoreline retreat resulting from a single storm event and for a sea level change in a time lapse. However, they generally neglect the effect of long-term continuous processes that may significantly modify the beach morphology. In this paper, we consider long-term changes due to SLR in a beach environment and we infer the beach-dune vulnerability, the shoreline retreats and the dune erosion rates resulting from the wave action. The assessment is performed under both, mean and extreme wave conditions during the 21st century and under two climate change scenarios. To address our purposes, we apply a combination of two numerical models and, additionally, the Bruun Rule is used to compare the numerical results with this widely used formulation.
Our case study is Son Bou beach, located in Menorca Island (Balearic Islands, Western Mediterranean). This beach-dune system is a well preserved environmental area that has been extensively monitored during the last years. The beach has special relevance since it constitutes a touristic point in the island and, therefore, a regional economic source.
Data
Site Description
Son Bou beach is a micro-tidal (tidal components lower than 10 cm, Tsimplis et al., 1995) sandy beach located in Menorca Island (Balearic Islands, Western Mediterranean Sea). The beach is 2.4 km alongshore, 20–30 m cross-shore and it presents a wide dune area behind it (70 m wide, approximately) (see Figure 1 for location). Its shoreline is oriented NW-SE direction and it is bounded by two rock capes. This area is part of the beach monitoring program of the Balearic Islands Coastal Observing and Forecasting System (SOCIB, Tintoré et al., 2013) since 2011. The program includes periodic topography and bathymetry surveys and in situ measurements of nearshore waves, among others coastal features. The observed average wave conditions are characterized by waves of medium to low energy with average significant wave height (Hs) of 0.52 m, peak period (Tp) of 5.84 s and mean wave direction (Dm) of 200°. Higher waves associated to extreme events exceeding 95th and 99th quantiles, are represented by Hs of 1.37 and 2 m, which are associated with Tp of 7.9 and 8.5 s, respectively, and Dm of 209° in both cases. As expected, higher mean Hs (0.6 m), as well as larger directional spread, are found at offshore conditions. Directional distributions of Hs at offshore location and coastal buoy are shown in the right panel of Figure 1.
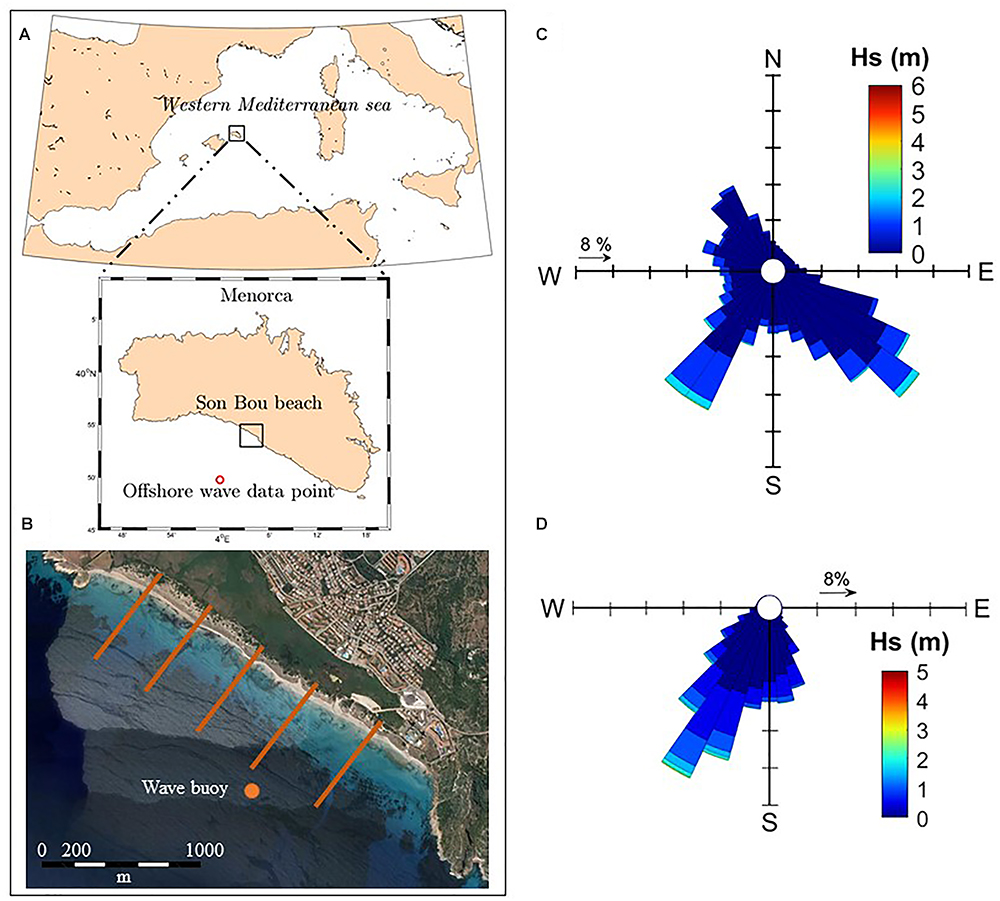
Figure 1. Left panel: (A) location map of Son Bou beach, Menorca Island, Western Mediterranean and the offshore wave data point. The buoy location and the location of the beach profile transects are shown in (B). Right panel; directional distributions of at (C) offshore conditions and at (D) the coastal wave buoy.
Son Bou topo-bathymetry survey was conducted in October 2011. The bathymetry data was recorded using a single-beam echo sounder while the aerial beach was monitored using a survey-grad RTK-GPS (Real Time Kinematic Global Position System) with a spatial resolution of 5 m in both cases. Moreover, the topography of the dune area was retrieved from the Geoportal data base, a Spanish national geographic information service1. This topo-bathymetry data was complemented with twelve beach profiles measured during three field surveys carried out in 10/May/2012, 08/May/2013, and 14/April/2014. The observed beach profiles have been used to quantify the intra-model variability depending on the imposed equilibrium profile, while the bathymetry data have been used as bottom input of the models.
Wave Data
Nearshore wave parameters in Son Bou (Hs, Tp, and Dm) have been measured using a directional Acoustic Waves and Currents (AWAC) sensor located at 22 m depth in the southern part of the beach (see Figure 1 for location). The AWAC records consist of hourly wave parameters spanning the period from October 2011 to September 2016.
Wave parameters in offshore conditions are retrieved from the SIMAR database (Pilar et al., 2008), which is available upon request2. The dataset consists of a 58-year (1958–2016) wave reanalysis over the Western Mediterranean basin generated with the WAM model (The Wamdi Group, 1988) with a spatial resolution of 12.5 km. The temporal resolution is 3-hourly from 1958 to 2011 and hourly since then. The SIMAR dataset has been evaluated against observations in several locations showing good performance when compared to in situ and remote observations (Pilar et al., 2008; Martínez-Asensio et al., 2013, 2015). For our purposes, the closest grid point to Son Bou, situated at 152 m depth, has been used as representative of the offshore wave climate in the beach (see Figure 1 for location).
Mean Sea Level Data
Yearly mean SLR projections up to 2100 for the Western Mediterranean are obtained from the last global IPCC projections (Church et al., 2013), available through the Integrated Climate Data Centre website hosted at the University of Hamburg3. We consider two climate change scenarios, namely a moderate Representative Concentration Pathway (RCP4.5) and the business-as-usual RCP8.5. These projections consist of gridded 1°×1° sea surface height fields that include the contributions of ocean dynamic changes, global ocean thermal expansion, inverted barometer effect, melting land ice from Greenland, Antarctica and glaciers, changes in land water storage and glacial isostatic adjustment. Following Sayol and Marcos (2018), local mean SLR has been computed using the values at the closest grid point to our area of study for all contributors, except for the ocean dynamics, for which we have used a value in the nearby Atlantic Ocean. We have followed this approach because the Atmosphere-Ocean General Circulation Models (AOGCMs) used to provide global projections of climate variables have too coarse resolution as to resolve properly the water exchanges and ocean dynamics through the narrow Strait of Gibraltar. They can properly simulate the sea surface height variations in the neighboring region, though. Since mean sea level in the Mediterranean Sea does not differ, in the long-term, from that observed in its vicinity we have thus used the ocean dynamical changes in the nearby Atlantic Ocean as representative of the whole basin. The outputs are provided as the ensemble average of 21AOGMs, from the Coupled Model Intercomparison Project Phase 5 plus the corresponding uncertainties given by the 5 and 95% confidence intervals (Slangen et al., 2014). We have neglected land subsidence as it has not been reported in this region.
Materials and Methods
We recall here that we aim at estimating the shoreline retreat and the potential losses of the beach-dune area and volume in Son Bou that are consequence of SLR and the action of waves during the 21st century. To do so, we perform our analyses in two stages. First, we compute the shoreline changes under SLR and low energy wave conditions, considering sediment transport and the morphological shifts of the beach; we also estimate the variability around the projected mean state (see Estimation of the Shoreline Retreat). Secondly, we use the temporal evolution of the beach profiles driven by SLR to assess the vulnerability of the aerial beach-dune system, defined here as the risk of erosion due to the number of wave events reaching the dune toe as well as the eroding capacity of these events. To achieve this, we base our approach on a probabilistic tool (see Beach and Dune Vulnerability and Erosion).
Numerical Models
The shoreline changes in response to projected SLR have been calculated using the Q2D-morfo numerical model, a non-linear morphodynamic model for large-scale shoreline dynamics (Falqués et al., 2007). It considers sediment transport in two horizontal dimensions and computes the wave field over the whole domain. However, the nearshore hydrodynamics is not considered because the sediment transport is computed directly from the wave field through parametrizations. The longshore transport is computed from the CERC formula, which assumes that the sediment transport mainly depends on the incoming waves (Fredsøe and Deigaard, 1992; Smith et al., 2003). The cross-shore transport is proportional to the difference between the bed slope and the slope of the equilibrium profile for each water depth so that it causes a relaxation of the profile to the equilibrium profile. The relaxation time at each location depends on the wave energy dissipation. Therefore, the model ignores the surf zone processes like bar dynamics or rip currents. In summary, the model is 2DH regarding depth-averaged sediment flux and bed updating, but not fully as it does not resolve the hydrodynamics and is therefore called quasi two dimensional (Q2D) model. The Q2D-morfo is computationally effective both in time and in computing resources, allowing the modeling of long-term simulations.
Although it is not an open-source model yet, Q2D-morfo model has already been successfully applied to assess shoreline changes under different wave conditions. The first version of the model was tested by Falqués et al. (2007) at La Barceloneta beach (Barcelona, North-Western Mediterranean) during the period from October to November 2003, when two consecutive storms occurred. The results were compared against beach bathymetry measurements allowing the model calibration. Van den Berg et al. (2011, 2012) used the model to investigate the long-term evolution of a rectilinear coast dominated by waves with high incidence angle, testing the model under high variability conditions. More recently, Arriaga et al. (2017) have validated Q2D-morfo with 3 year-data from the Zandmotor mega-nourishment (Dutch coast). They have applied the model to predict the nourishment evolution for the next 30 years using the corresponding wave data projection.
So far, this model has not been used under long-term changing sea levels. Thus, we assess the model capability to simulate the beach adaptation to different SLR scenarios and predict the evolution of the shoreline. In order to account for the model uncertainty, it has been run using five equilibrium profiles that were obtained from observations at the five locations indicated in Figure 1. Each equilibrium profile was calculated as the average of the twelve observations for each location. In addition, the depth closure was obtained from the wave height buoy measurements. The equilibrium profiles have been forced to not have positive slope due to Q2D-morfo model requirements. Imposing five equilibrium profiles, we can quantify the intra-model variability depending on this input parameter. Furthermore, we also compare the model results with the Bruun Rule approach.
The beach erosion caused by storm waves has been simulated with the XBeach model (Roelvink et al., 2009). XBeach is an open-source numerical model developed to simulate morphodynamic processes caused by high-energy wave conditions. The model solves phase-averaged coupled equations for cross-shore and longshore hydro and morphodynamics. XBeach has become a state-of-the-art numerical model and has therefore been widely validated. Among these works, Van Dongeren et al. (2009) compared XBeach model results with field measurements at eight European sites. They concluded that the model has skills in predicting the coastal profile, although it overestimates the erosion around the mean water line and the depositions at the lower beach face. Roelvink et al. (2009) tested the morphodynamics and hydrodynamics of the model in 1D and 2DH mode and contrasted the results with several lab and field cases. The model showed reasonably results regarding to the dune toe retreat (correlation of 0.83 and bias of -0.05) and the sediment/erosion rates (mean correlation of 0.91 and bias of -0.11). However, these authors also found a dune erosion overestimation of 10–15%. McCall et al. (2010) simulated the beach morphological response after a hurricane in Santa Rosa Island (FL, United States) in 2DH mode and compared the numerical results with the field measurements. The model resulted in a good capability to solve processes as over-swash, fore-dune erosion and deposition caused by a storm event. De Winter et al. (2015) calibrated the hydrodynamics and dune erosion modules comparing the model results with field observations at Egmond aan Zee (Netherlands) and concluded that XBeach model simulates the magnitude of erosion volumes satisfactorily (correlation coefficient up to 0.55) but overestimates the erosion volumes in regions with dune scarps. Nowadays, XBeach model is extensively applied to assess beach impacts and dune erosion (see for instance, Harley et al., 2011; Vousdoukas et al., 2012; Bugajny et al., 2013; Harley et al., 2016; Karunarathna et al., 2018).
Our approach to investigate the beach evolution combines both models’ capabilities: we use of the Q2D-morfo model to simulate the beach changes due to SLR and then apply the XBeach model to estimate the response to storm conditions under the modified beach. Both models are used in 2D mode in order to account the cross-shore and long-shore sediment transport.
Offshore waves have been propagated toward the coast using the state-of-the-art SWAN model (Booij et al., 1999), a third-generation wave model that solves the spectral action balance equation for the propagation of wave spectra4. Despite SWAN model is not computational demanding, the simulation of the whole offshore wave time series (58 years of three sea state parameters) is unfeasible. To avoid this problem, the nearshore wave data is obtained by applying a combination of numerical modeling and statistical downscaling approaches as it is explained in the following. Before using SWAN, a Maximum Dissimilarity Algorithm (MDA) is applied to analyze trivariate (Hs, Tp, Dm) hourly time series of offshore wave parameters (Camus et al., 2011a). The MDA algorithm allows selecting a representative subset of the offshore wave climate diversity suitable to be implemented in a nearshore propagation methodology. The algorithm chooses the representative sea states (defined by its Hs, Tp, and Dm) of the wave climate, basing on the maximum dissimilarity with respect to the whole wave dataset. This dissimilarity between one sea state and the others, is calculated from the Euclidean distance. Based on previous studies (Camus et al., 2011a), 200 sea states of the offshore time series have been selected as representative of the wave climate. These sea states are the ones that have been propagated up to the coast using SWAN model. From the resulting modified wave parameters, it is possible to reconstruct the entire nearshore wave time series through an interpolation technique based in radial basis functions (RBF) (Camus et al., 2011b). To do this reconstruction, the coefficients obtained from the RBF are applied to the complete offshore wave series transferring them from deep to shallow water.
Estimation of the Shoreline Retreat
Sandy beaches are adaptable to changes in short-term hydrodynamic conditions varying around a mean morphological state (Carter, 1988; Muñoz-Perez and Medina, 2010). Thus, persistent beach changes will be due to changes in long-term forces. Here, we assume that long-term changes in the beach morphology, including the position of the shoreline, are caused by SLR. Therefore, we neglect the short-term changes such as those resulting from storm events and cumulative storm effects. In other words, we consider that the beach always recovers its equilibrium profile after every storm, which we anticipate will likely underestimate the shoreline retreat.
We use the Q2D-morfo model to solve the sediment transport and the morphological shifts of the beach caused by sea level changes on a yearly basis and we impose low energy wave conditions. In order to cover the entire uncertainty range of SLR, we have considered four possible pathways, two of them corresponding to the ensemble average of RCP4.5 and RCP8.5. The other two correspond to the 5% confidence interval of the multi-model ensemble spread under the scenario RCP4.5 and to the 95% confident interval of the multi-model ensemble spread for the RCP8.5 scenarios. In this way, we account for the maximum (RCP8.5 95%) and minimum (RCP4.5 5%) limits of the model ensemble, taking into account the two scenarios jointly (Figure 2). In addition, constant low energy wave parameters, obtained from the more frequent low energy AWAC measurements, are used to force the model. Wave conditions are needed to run the model, but they are the same over the whole simulated period so that changes in shoreline retreat are not conditioned by wave effects. Thus, we obtain the yearly evolution of the shoreline position for each of the SLR scenarios.
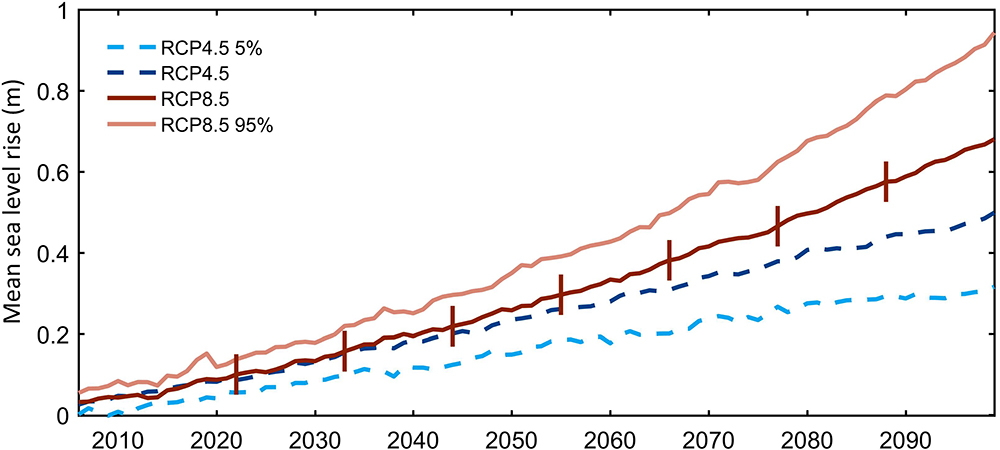
Figure 2. Projected sea level rise scenarios used in the present study. 5–95% refer to the uncertainties derived from the multi-model ensemble spread of each of the two scenarios. The vertical lines indicate the time lapses when the erosion volume is estimated (see “Beach and Dune Vulnerability and Erosion”).
In addition, we performed the same assessment using the Bruun Rule. The Bruun Rule predicts a landward and upward displacement of the cross-shore sea-bed profile in response to a SLR as follows:
where h is the depth closure, B is the elevation of dune or berm above mean sea level, S is the mean sea level change and L is the distance from the berm crest to the h location. This formulation is based on the assumption of a balance system between the sediment budget of the nearshore and bottom profile (Bruun, 1988). In this way, the shoreline retreat can be easily quantified from the landward displacement of the actual shoreline location but assuming the profile shape remains unchanged.
Changes in the position of the shoreline around its average yearly value that results from the impact of storm waves are also explored. To do so, we combine the projected yearly bathymetries obtained from the outputs of the Q2D-morfo and simulate the deviations in the shoreline after the largest annual storm event using the XBeach model. Storm conditions (wave parameters and duration) are obtained as the annual typical storm (i.e., 1-year RP) based on data generated as described in the following Section “Beach and Dune Vulnerability and Erosion.” These offshore wave time series have to be propagated toward the coast using SWAN, so the XBeach model can be forced with nearshore waves.
Beach and Dune Vulnerability and Erosion
We provide a measure of the dune vulnerability as the number of annual wave run-up (R2%, the vertical height exceeded by 2% of maxima water-level) events reaching the dune toe for each of the four SLR scenarios. Based on the field measurements, the dune toe is determined as the topographic line of 1 m height above the current mean sea level. We calculate the R2% with the empirical formulation developed by Stockdon et al. (2006):
Where βf is the foreshore beach slope, calculated from the topo-bathymetry measurements, and H0 and L0 are the wave height and wave length in deep-water conditions, respectively. Although the beach shoreline is shifted landward due to SLR (as described in Section “Estimation of the Shoreline Retreat”), the Bruun Rule assumes that the equilibrium state remains the same and, therefore, the foreshore beach slope (βf) does not change over time.
In order to increase the number of offshore wave time series, we use SIMAR data to simulate, via Monte-Carlo, a set of 10000 time series of Hs and duration of extreme events spanning 100 years each that are statistically consistent with present-day wave climate, as described below. We expect SLR to be the major driver controlling the collision of waves with the dune and therefore we will not consider changes in the wave fields during the 21st century. This assumption is supported by earlier analyses showing only slight changes of waves in the Western Mediterranean area under climate change scenarios (Enríquez et al., 2017; Mentaschi et al., 2017; Sayol and Marcos, 2018).
The steps to build this synthetic dataset are the following: first, we select the independent wave extreme events whose Hs exceeds the 90th percentile of Hs of the entire series from SIMAR, since we are interested in the largest waves that may reach the dune. We consider that these 10% highest waves are independent if they are separated by at least 72 h. We also compute the duration for each of them. Second, we fit univariate marginal probability distribution functions to the empirical Hs and to the duration distributions; we select the best fit among the Generalized Pareto Distribution (GPD), Weibull, Gamma, Log-normal and Exponential distributions, based on their minimum log-likelihood. The results indicate that the most suitable distribution is the GPD for both Hs and duration of the events. Third, we search the relationship between these marginal distributions of Hs and duration using Copula functions to fit their joint probability distribution. We fit the bivariate distribution based on the Clayton, Frank, Gumbel and Gaussian Copulas and select the one displaying smaller root mean square differences with the original values, which in our case is the Gaussian Copula. Fourth, we compute the number of events each year from our initial time series and fit a Poisson distribution, resulting in λ around 24 (λ being the parameter of the distribution and corresponding to the average number of events per year), matching with previous results that suggest a λ value between 15 and 25 (Mazas and Hamm, 2017). Finally, we use the obtained Poisson distribution to simulate the yearly distribution of wave events in 10000 time series of 100 years each; we then simulate Hs and duration of each of these events from the bivariate distribution obtained with the Copula function. Wave Tp is calculated for each simulated event using a linear relationship between Hs and Tp in the original time series. The resulting set of Hs, Tp and duration represents 10000 different realizations, each of them of 100-year long, of the highest 10% of waves, consistent with the present-day wave climate in the study region.
The synthetic wave time series have been superimposed onto the beach profiles modified by SLR resulting from Q2D-morfo model and Bruun Rule simulations. Then, they are used to assess the probability of the wave run-up (computed as R2% as indicated above, Equation 2) to reach the dune toe and induce dune erosion during the 21st century.
In addition, the erosion caused by the wave events can be calculated by the XBeach model. However, computational constraints limit our ability to provide estimates for each event in each of the 10000 synthetic time series. Instead, we have computed the Hs and duration corresponding to the 10-year and 100-year return periods from the bivariate distribution described above. The corresponding waves are propagated nearshore using the SWAN model and then, they are used to force the XBeach model at the coast for the selected years as representative of each decade (vertical lines in Figure 2). This has been carried out for a single SLR scenario, namely RCP8.5. In order to reduce the number of simulations, the eroded volume was computed for 7 years equally spaced (vertical lines in Figure 2) and for moderate (10-year return period) and high (100-year return period) extreme waves. This makes 35 simulations for each return period (resulting from 7 time steps × 5 input equilibrium profiles) with the XBeach model, which is computationally feasible.
Results
Shoreline Retreat Under SLR
Figure 3 represents the yearly shoreline retreat obtained from the outputs of the Q2D-morfo model and the Bruun Rule. For the Q2D-morfo, a 20-year spin up has been applied under low energy wave conditions and no SLR. The spin up has been needed to ensure that waves do not change the beach shoreline configuration so that we can quantified the shoreline retreat caused by SLR only. Figure 3 represents the yearly alongshore average position of the shoreline for the period ranged from 2017 to 2099 and for the two RCP4.5 and RCP8.5 scenarios (in blue and red colors, respectively). The intramodel variability of the Q2D-morfo (shadowed areas around dashed lines in Figure 3) is calculated as the spread in the model results when the set of 5 equilibrium profiles are used. For the sake of consistency, the uncertainty in the Bruun Rule arising from the set of profile slopes is also plotted as the shadowed area around solid lines. The comparison between the two approaches shows that the Bruun Rule significantly overestimates the loss of aerial beach resulting from SLR, in agreement with earlier assessments (Esteves et al., 2009; Ranasinghe et al., 2012; Deng et al., 2015). In our case, we find that, by 2100, the shoreline retreat obtained with Q2D-morfo is 12 m (9 m) on average while the Bruun Rule results in up to 28 m (19 m), under the RCP8.5 (RCP4.5) scenario. This overestimation is not constant and increases over time, since the Bruun Rule responds faster to SLR. Another notable feature is that Q2D-morfo is strongly sensitive to the assumed equilibrium profile. This is reflected in the intramodel uncertainties overlapping for the two scenarios (Figure 3).
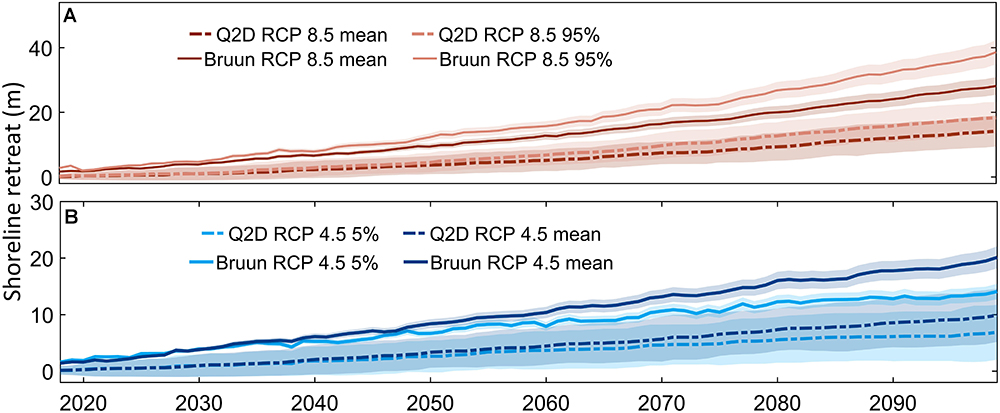
Figure 3. Shoreline retreat comparison using Q2D-morfo model (dashed lines) and Bruun Rule (solid lines) under (A) RCP8.5 (red color) and (B) RCP4.5 (blue color) scenarios. The light colors represent the uncertainties of the two scenarios and the shadow areas represent the intra-model uncertainties.
Whatever the model used, the shoreline retreat due to SLR is smaller than the beach width (∼30 m approximately); this indicates that the dune area would not be affected if only projected SLR and low energy wave conditions are taken into account. This picture may change under more energetic waves, however. In order to quantify the variability of the shoreline induced by storm waves around the time-variable mean response to SLR, the shoreline position after the annual largest storm has been obtained from the XBeach model. Due to computational constraints, only three 100-year wave time series (generated as described in Section “Beach and Dune Vulnerability and Erosion”) have been evaluated for two scenarios. Figure 4 shows the shoreline retreat, averaged along-shore, with respect to the annual mean shoreline position from 2017 to 2099, under RCP4.5 and RCP8.5. Along-shore variations of the shoreline position are represented as shadowed areas. These curves thus represent the variability of the shoreline in addition to the retreat caused by SLR. Changes around the mean shoreline position resulting from SLR are on average 12–14 m during the 2020s, and then progressively reduce until an average value of 5–8 m during the 2090s. We speculate that this decreasing trend is the result of the larger slope on the fore-dune zone with respect to the beach-face slope. As it will be shown later, it does not imply that the eroding capacity of storm waves is reducing over time.
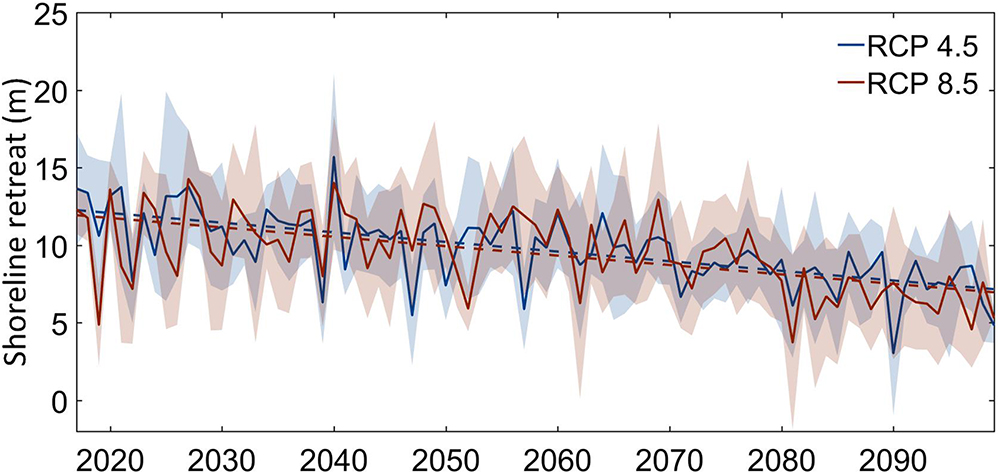
Figure 4. Shoreline retreats derived from maximum annual storms and sea level rise under two scenarios with respect to the annual mean position. Red and blue solid lines represent shoreline retreats on average, under RCP8.5 and RCP4.5 SLR scenarios, respectively. Shadow areas represent the variability of shoreline retreat along the beach and the dashed lines are the trends.
Beach and Dune Vulnerability and Erosion
The vulnerability of the beach-dune system is represented as the number of events for which the associated wave run-up reaches the dune toe for every year and for each of the 10000 synthetic 100-year time series. The beach configurations for every year are those estimated by Q2D-morfo and the Bruun Rule under SLR conditions from Section “Shoreline Retreat Under SLR.” The results for the RCP8.5 scenario are shown in Figure 5, as the annual evolution of the average number of events reaching the dune toe (bold lines). The shaded areas indicate the standard deviation of the number of run-up events reaching to the dune toe as a result of 10000 wave time series simulations performed.
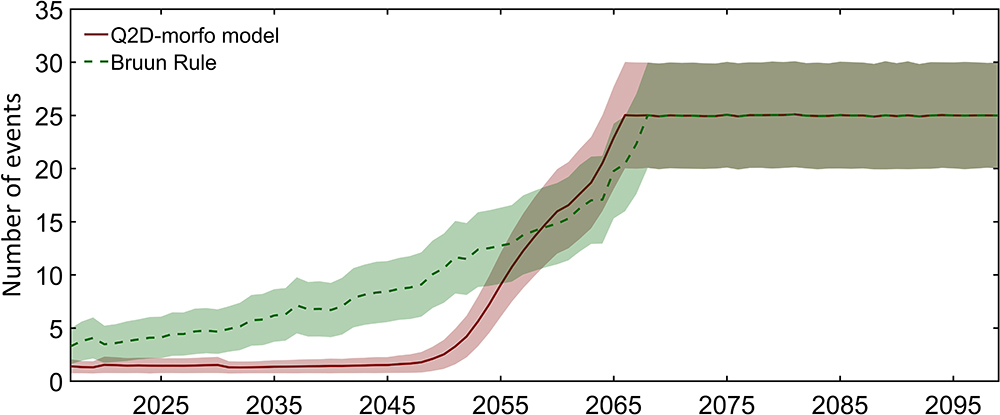
Figure 5. Number of events reaching the dune toe under RCP8.5 SLR using the beach retreat obtained by Q2D-morfo model (solid red line) and Bruun Rule (dashed green line) results. Shaded areas represent the standard deviation derived from the of 10,000 wave time series simulations performed. Horizontal lines after late 2060s indicate that all the events considered (that represent the top 10% of highest waves) reach the dune toe.
When the shoreline evolution given by the Bruun Rule is used (Figure 5, green line), the number of events that reach the dune toe increases steadily from present until 2068, ranging from 3 to 5 annual events in the near term to more than 20. Since then, all the events considered (that represent the top 10% of highest waves) reach the dune toe. We recall here that the annual average number of events that exceed the 90th percentile is 24; thus, the horizontal lines in Figure 5 indicate that this threshold has been reached and that all the modeled wave events arrive, at least, up to the dune toe. The evolution of the number of events the run-up reaches the dune toe is very different when the results of the Q2D-morfo model are used (Figure 5, red line). It shows a stable number ranging from one to two yearly events until approximately the year 2050. Since then to 2066, the average number rapidly increases until it reaches the maximum around the same year as with the Bruun Rule. The origin of these differences in the initial frequency between the two methods is twofold: first, they are consequence of the faster shoreline retreat obtained from Bruun Rule (see Figure 3). Second and most important, the Q2D-morfo model progressively reduces the berm located between the coastline and the dune simulating its continuous erosion. Until this berm does not disappear in response to SLR (around 2050), the wave run-up is unable to reach the dune because of its protective effect. When this happens, the potential for beach and dune erosion increases rapidly.
The average number of wave run-up events that can reach the dune measures the potential of erosion due to wave forcing. So, in the next step, in order to quantify this erosion, we calculate the volume that is actually eroded under the action of moderate (10-year return level) and large (100-year return level) storms. We anticipate that the impact of these events will strongly depend on the mean sea level (and by extension on the shoreline position) at their arrival; thus, we explore the induced erosion during different decades, ranging from 2020s until 2090. The years for which the erosion volume has been calculated are those indicated by vertical lines in Figure 2.
The wave parameters for 10 and 100-year return periods (RP) are 2.9 m of Hs, 8.26 s of Tp and 91 h of duration and 3.96 m, 9.5 s, and 120.5 h, respectively. The total volume eroded, per linear m, is plotted in Figure 6 for the two RP and for each time horizon. The shadowed areas correspond to the standard deviation of the erosion rates obtained with the Q2D-model outputs fed with the five equilibrium profiles (i.e., intra-model uncertainty), while the bold lines indicate the averaged erosion rates. The results show that the volume of dune eroded generally increases as SLRs, even for small values of SLR. Note that we are propagating always the same exact storm, whose eroding potential is, in most cases, larger with higher sea levels. This is not surprising, as the dunes become closer to the shoreline with higher sea levels. The increase is obtained for both the moderate and the large storms, but with different evolutions (note the different vertical scales in the figure). The eroded volume ranges from 34.1 m3/m in 2022 to 42.8 m3/m in 2088 using the 10-year RP storm (an increase of 25.5%), while it ranges from 61.2 m3/m in 2022 to 77.7 m3/m with the 100-year RP storm (increasing 27%).
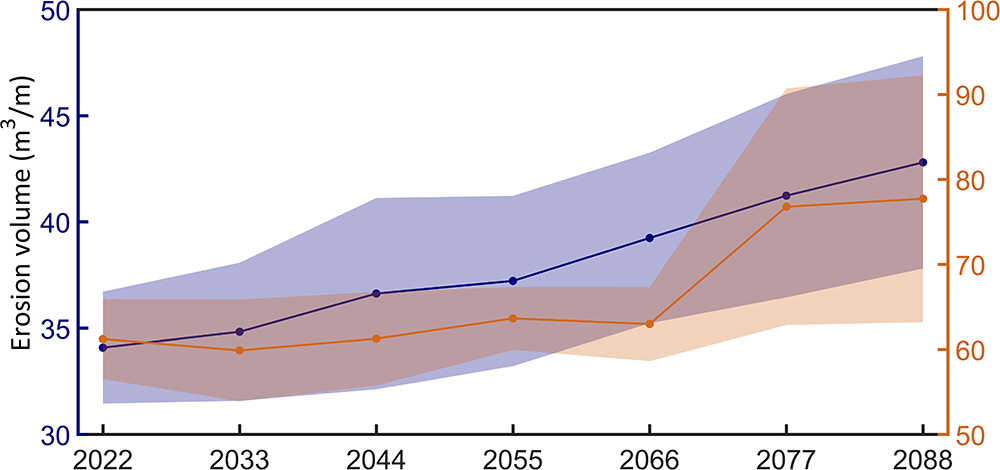
Figure 6. Dune-beach erosion volume taking into account the sea level rise and the 10-year RP (dark blue line, left axis) and 100-year RP (orange line, right axis) under RCP8.5 scenario. The shadowed areas indicate the standard deviation based on the equilibrium profile used in the Q2D-morfo model simulations.
Figure 6 shows that the erosion volume increases significantly in the 2070s under 100-year RP storm. This finding highlights the relevance of the timing when the strongest storm takes place and the importance of the combined sea level and wave effects; i.e., a storm hitting the coast without a protective berm has a stronger eroding potential. Figure 6 also reveals the same erosion in 2055 and 2066 under this 100-year RP storm. This is indicating that, under similar mean sea level conditions and similar beach configurations (with or without a berm), the effect of the largest storms is the most decisive factor in the erosion. The erosion volume of 10 years RP storms increases gradually from 2022 to 2088, which indicates that low-to-medium wave energy conditions have limited consequences for the dune area, under the assumption of neglecting the cumulative effect of several storms, and that the effect of mean sea level has a stronger weight in the erosion for moderate storms.
Conclusion
We have evaluated the response of an open sandy beach in a microtidal environment to the impacts of mean SLR and the action of wind-waves, under prescribed climate change scenarios for the 21st century. In agreement with earlier studies (e.g., Ranasinghe et al., 2012), our results indicate that the widely applied Bruun Rule overestimates the shoreline retreat in this type of coasts; therefore, impacts assessments of, for example, flood risks or vulnerability changes, must be considered with caution if they are based on this approach. On the contrary, using a numerical morphodynamic model, the shoreline retreat is slowed down due to the simulation of the berm erosion. This result highlights the relevance of the protection role of the beach and its berm, suggesting that there are tipping points after which the dune erosion may become irreversible.
Our findings indicate that, when the morphodynamic response of the beach to rising sea levels is considered, its configuration, including the shoreline position, does not change steadily even if the forcing does. The consequence is that the impacts of climate change on the beach-dune system in Son Bou may be overlooked, especially if the shoreline position is used as a proxy, as it is often the case. In addition, our results suggest that the monitoring of the aerial beach, and whether it includes a protective berm or not, could be a better alternative measurement of the response of the system to the effects of climate change.
This study concludes that SLR is the major driver of the projected changes in the shoreline position in Son Bou beach, inducing an average retreat between 12 and 23 m (2–15 m) under RCP8.5 (RCP4.5) by 2100. These numbers increase up to 25–42 m (13–22 m) under RCP8.5 (RCP4.5) when the Bruun Rule is used instead of the Q2D-morfo model. This average value is altered under storm conditions, which may temporarily displace the shoreline around ± 10 m in the near future and half of that value by the end of the century. In both cases, these values are obtained under the assumption of no cumulative storm effects. As sea level increases so does the number of waves that reach the dune toe and therefore the potential dune erosion. The estimated erosion volume for the 1 in a 100 years event is ∼60 m3/m until the 2060s, when the berm has not yet disappeared. After that period, the potential erosion increases by 27% only within a decade.
It is important to note that this study relies on some assumptions. First, we have neglected changes in wave direction that may be relevant for the beach planform evolution even under fair weather conditions. Second, we have considered that the observed equilibrium beach profile in present-day conditions remains constant under future SLR. This is a reasonable assumption since the main driver is SLR and the wave climate is not expected to change significantly. Finally, we have neglected the cumulative storm effects on the beach configuration; in other words, we have considered that the beach is able to recover its equilibrium state after every storm episode. This assumption is necessary due to the inability of present-day numerical models to simulate both the storm effects and the beach recovery in a realistic way for long-term periods. But this is also a strong limitation since previous work has demonstrated that the impact of storm groups may significantly exceed from those caused by a single event (Ferreira, 2005). Therefore, our estimates of beach-dune vulnerability and potential erosion should be taken as a lower bound.
Data Availability Statement
Publicly available datasets were analyzed in this study. This data can be found here: http://www.socib.eu/.
Author Contributions
This work was part of AE’s Ph.D. thesis. AE wrote the manuscript and performed the simulations. MM is her supervisor and contributed to conceiving the topic and the development of the argument, interpretation of the results, and writing. AF is the developer of the Q2Dmorfo model and contributed to the interpretation of its results and writing. DR contributed with his expertise in the XBeach model and revised the manuscript.
Funding
This work was supported by the CLIMPACT (CGL2014-54246-C2-1-R) funded by the Spanish Ministry of Economy.
Conflict of Interest Statement
The authors declare that the research was conducted in the absence of any commercial or financial relationships that could be construed as a potential conflict of interest.
Acknowledgments
AE acknowledges an FPI grant associated with the CLIMPACT project (CGL2014-54246-C2-1-R). We thank Puertos del Estado for providing deep water wave data from the SIMAR database. Topo-bathymetries and wave observations are part of the beach monitoring facility of SOCIB.
Footnotes
- ^https://sig.mapama.gob.es/geoportal/
- ^http://www.puertos.es/es-es/oceanografia/Paginas/portus.aspx
- ^http://icdc.cen.uni-hamburg.de/1/daten/ocean/ar5-slr.html
- ^http://swanmodel.sourceforge.net/
References
Arriaga, J., Rutten, J., Ribas, F., Falqués, A., and Ruessink, B. G. (2017). Modeling the long-term diffusion and feeding capability of a mega-nourishment. Coastal Eng. 121, 1–13. doi: 10.1016/j.coastaleng.2016.11.011
Booij, N., Ris, R. C., and Holthuijsen, L. H. (1999). A third-generation wave model for coastal regions. I- Model description and validation. J. Geophys. Res. 104, 7649–7666. doi: 10.1029/98JC02622
Bruun, P. (1962). Sea-level rise as a cause of shore erosion. Proc. Am. Soc. Civil Eng. 88, 117–132.
Bruun, P. (1988). The bruun rule of erosion by sea-level rise: a discussion on large-scale two- and three-dimensional usages. J. Coastal Res. 4, 627–648.
Bugajny, N., Furmańczyk, F., Dudzińska-Nowak, J., and Paplińska-Swerpel, B. (2013). Modelling morphological changes of beach and dune induced by storm on the Southern Baltic coast using XBeach (case study: Dziwnow Spit). J. Coastal Res. Spec. Issue 65, 672–677. doi: 10.2112/SI65-114.1
Bujosa, A. B. (2014). Substitution patterns across alternatives as a source of preference heterogeneity in recreation demand models. J. Environ. Manage. 144, 121–217.
Camus, P., Mendez, F. J., Medina, R., and Cofiño, A. S. (2011a). Analysis of clustering and selection algorithms for the study of multivariate wave climate. Coastal Eng. 58, 453–462. doi: 10.1016/j.coastaleng.2011.02.003
Camus, P., Mendez, F. J., and Medina, R. (2011b). A hybrid efficient method to downscale wave climate to coastal areas. Coastal Eng. 58, 851–862. doi: 10.1016/j.coastaleng.2011.05.007
Carter, R. W. G. (1988). Coastal Environments: An Introduction to the Physical Ecological and Cultural Systems of Coastlines. London: Academic, 99–149. doi: 10.1016/B978-0-08-050214-4.50009-7
Church, J. A., Clark, P. U., Cazenave, A., Gregory, J. M., Jevrejeva, S., Levermann, A., et al. (2013). “Sea level change, in: climate change 2013: the physical science basis,” in Contribution of Working Group I to the Fifth Assessment Report of the Intergovernmental Panel on Climate Change, eds T. F. Stocker, D. Qin, G. K. Plattner, M. Tignor, S. K. Allen, J. Boschung et al. (Cambridge: Cambridge University Press).
Dasgupta, S., Laplante, B., Meisner, C., Wheeler, D., and Yan, J. (2009). The impact of sea level rise on developing countries: a comparative analysis. Clim. Change 93, 379–388. doi: 10.1007/s10584-008-9499-5
De Winter, R. C., Gongriep, F., and Ruessink, B. G. (2015). Observations and modeling of alongshore variability in dune erosion at Egmond aan Zee, the Netherlands. Coastal Eng. 99, 167–175. doi: 10.1016/j.coastaleng.2015.02.005
de Winter, R. C., and Ruessink, B. G. (2017). Sensitivity analysis of climate change impacts on dune erosion: case study for the Dutch Holland coast. Clim. Change 141, 685–701. doi: 10.1007/s10584-017-1922-3
Deng, J., Harff, J., Schimanke, S., and Meier, M. (2015). A method for assessing the coastline recession due to the sea level rise by assuming stationary wind-wave climate. Oceanol. Hydrobiol. Stud. 44, 362–380. doi: 10.1515/ohs-2015-0035
Enríquez, A. R., Marcos, M., Álvarez-Ellalcuría, A., Orfila, A., and Gomis, D. (2017). Changes in beach shoreline due to sea level rise and waves under climate change scenarios: application to the Balearic Islands (western Mediterranean). Natur. Hazards Earth Syst. Sci. 17, 1975–1089. doi: 10.5194/nhess-17-1075-2017
Esteves, L. S., Williams, J. J., Nock, A., and Lymery, G. (2009). Quantifying shoreline changes along the Sefton Coast (UK) and the implications for research-informed coastal management. J. Coastal Res. 56, 602–606.
Falqués, A., Garnier, R., Ojeda, E., Ribas, F., and Guillen, J. (2007). Q2D-morfo: a medium to long term model for beach morphodynamics. River Coastal Estuarine Morphodynamics 2, 71–78. doi: 10.1201/NOE0415453639-c10
Ferreira, O. (2005). Storm groups versus extreme single storms: predicted erosion and management consequences. J. Coastal Res. 42, 221–227.
Fredsøe, J., and Deigaard, R. (1992). Mechanics of Coastal Sediment Transport. Advanced Series on Ocean Engineering. doi: 10.1142/1546
Gössling, S., Scott, D. C., Hall, M., Ceron, J. P., and Dubois, G. (2012). Consumer behaviour and demand response of tourists to climate change. Ann. Tour. Res. 39, 36–58. doi: 10.1016/j.annals.2011.11.002
Harley, M., Armaroli, C., and Ciavola, P. (2011). Evaluation of XBeach predictions for a real-time warning system in Emilia-Romagna, Northern Italy. J. Coastal Res. SI 64, 1861–1865.
Harley, M. D., Valentini, A., Armarolli, C., Perini, L., Calabrese, L., and Ciavola, P. (2016). Can an early-warning system help minimize the impacts of coastal storms? A case study of the 2012 Halloween storm, northern Italy. Natur. Hazards Earth Syst. Sci. 16, 209–222. doi: 10.5194/nhess-16-209-2016
Idier, D., Castelle, B., Poumadère, M., Balouin, Y., Bertoldo, R. B., Bouchette, F., et al. (2013). Vulnerability of sandy coast to climate variability. Clim. Res. 57, 19–44. doi: 10.3354/cr01153
Karunarathna, H., Brown, J., Chatzirodou, A., Dissanayake, P., and Wisse, P. (2018). Multi-timescale morphological modelling of a dune-fronted sandy beach. Coastal Eng. 136, 161–171. doi: 10.1016/j.coastaleng.2018.03.005
Le Cozannet, G., Garcin, M., Yates, M., Idier, D., and Meyssignac, B. (2014). Approaches to evaluate the recent impacts of sea-level rise on shoreline changes. Earth Sci. Rev. 138, 47–60. doi: 10.1016/j.earscirev.2014.08.005
Luijendijk, A., Hagenaars, G., Ranasinghe, R., Baart, F., Donchyts, G., and Aarninkhof, S. (2018). The stat of the World’s Beaches’. Sci. Rep. 8:6641. doi: 10.1038/s41598-018-24630-6
Martínez-Asensio, A., Marcos, M., Jordà, G., and Gomis, D. (2013). Calibration of a new wind-wave hindcast in the Western Mediterranean. J. Mar. Syst. 121–122, 1–10. doi: 10.1016/j.jmarsys.2013.04.006
Martínez-Asensio, A., Tsimplis, M. N., Marcos, M., Feng, X., Gomis, D., Jordà, G., et al. (2015). Response of the north atlantic wave climate to atmospheric modes of variability. Int. J. Climatol. 36, 1210–1225. doi: 10.1002/joc.4415
Mazas, F., and Hamm, L. (2017). An event-based approach for extreme joint probabilities of waves and sea levels. Coastal Eng. 122, 44–59. doi: 10.1016/j.coastaleng.2017.02.003
McCall, R. T., Van Thiel de Vries, J. S. M., Plant, N. G., Van Dongeren, A. R., Roelvink, J. A., Thompson, D. M., et al. (2010). Two-dimensional time dependent hurricane overwash and erosion modeling at Santa Rosa Island. Coastal Eng. 57, 668–683. doi: 10.1016/j.coastaleng.2010.02.006
Mentaschi, L., Vousdoukas, M. I., Voukouvalas, E., Dosio, A., and Feyen, L. (2017). Global changes of extreme coastal wave energy fluxes triggered by intensified teleconnection patterns. Geophys. Res. Lett. 44, 2416–2426. doi: 10.1002/2016GL072488
Muñoz-Perez, J. J., and Medina, R. (2010). Comparison of long-, medium- and –short-term variations of beach profiles with and without submerged geological control. Coastal Eng. 57, 241–251. doi: 10.1016/j.coastaleng.2009.09.011
Nicholls, R. J. (2002). Analysis of global impacts of sea-level rise: a case study of flooding. Phys. Chem. Earth 27, 1455–1466. doi: 10.1016/S1474-7065(02)00090-6
Pilar, P., Guedes, S. C., and Carretero, J. C. (2008). 44-year wave hindcast for the North East Atlantic European Coastal Coastal Eng. 55, 861–871.
Purvis, M. J., Bates, P. D., and Hayes, C. M. (2008). A probabilistic methodology to estimate future coastal flood risk due to sea level rise. Coastal Eng. 55, 1062–1073. doi: 10.1016/j.coastaleng.2008.04.008
Ranasinghe, R. (2016). Assessing climate change impacts on open sandy coasts: a review. Earth-Sci. Rev. 160, 320–332. doi: 10.1016/j.earscirev.2016.07.011
Ranasinghe, R., Callaghan, D., and Stive, M. J. F. (2012). Estimating coastal recession due to sea level rise: beyond the Bruun rule. Clim. Change 110, 561–574. doi: 10.1007/s10584-011-0107-8
Revell, D. L., Battalio, R., Spear, B., Ruggiero, P., and Vandever, J. (2011). A methodology for predicting future coastal hazards due to sea-level rise on the California Coast. Clim. Change 109, 251–276. doi: 10.1007/s10584-011-0315-2
Roelvink, D., Reniers, A., van Dongeren, A., van Thiel de Vries, J., McCall, R., Lescinski, J., et al. (2009). Modelling storm impacts on beaches, dunes and barrier islands. Coastal Eng. 56, 11–12. doi: 10.1016/j.coastaleng.2009.08.006
Rosati, J. D., Dean, R. G., and Walton, T. L. (2013). The modified Bruun Rule extended for landward transport. Mar. Geol. 340, 71–81. doi: 10.1016/j.margeo.2013.04.018
Sayol, J. M., and Marcos, M. (2018). Assessing flood risk under sea level rise and extreme sea levels scenarios: application to the ebro delta (Spain). J. Geophys. Res. Oceans 123, 794–811. doi: 10.1002/2017JC013355
Slangen, A. B. A., Carson, M., Katsman, C. A., van de Wal, R. S. W., Köhl, A., Vermeersen, L. L. A., et al. (2014). Projecting twenty-first century regional sea-level changes. Clim. Change 124, 317–332. doi: 10.1007/s10584-014-1080-9
Small, C., and Nicholls, R. J. (2003). A global analysis of human settlement in coastal zones. J. Coastal Res. 19, 584–599.
Smith, E. R., Wang, P., and Zhang J. (2003). Evaluation of the CERC Formula Using Large- Scale Laboratory Data. Tampa, FL: Geology Faculty Publications, 238.
Stockdon, H. F., Holman, R. A., Howd, P. A., and Sallenger, A. H. (2006). Empirical parameterization of setup, swash, and runup. Coast. Eng. 53, 573–588. doi: 10.1016/j.coastaleng.2005.12.005
The Wamdi Group (1988). The WAM model – A third generation ocean wave prediction model. J. Phys. Oceanogr. 18, 1775–1810. doi: 10.1175/1520-0485(1988)018<1775:TWMTGO>2.0.CO;2
Tintoré, J., Vizoso, G., Casas, B., Heslop, E., Pascual, A., Orfila, A., et al. (2013). SOCIB: the Balearic Islands coastal ocean observing and forecasting system responding to science, technology and society needs. Mar. Soc. J. 47, 101–117. doi: 10.4031/MTSJ.47.1.10
Tsimplis, M. N., Proctor, R., and Flather, A. (1995). A two-dimensional tidal model for the Mediterranean Sea. J. Geophys. Res. 100, 16223–16239. doi: 10.1029/95JC01671
Van den Berg, N., Falqués, A., and Ribas, F. (2011). Long-term evolution of nourished beaches under high angle wave conditions. J. Mar. Syst. 88, 102–112. doi: 10.1016/j.jmarsys.2011.02.018
Van den Berg, N., Falqués, A., and Ribas, F. (2012). Modeling large scale shoreline sand waves under oblique wave incidence. J. Geophys. Res. 117:F03019. doi: 10.1029/2011JF002177
Van Dongeren, A., Bolle, A., Vousdoukas, M. I., Plomaritus, T., Eftimova, P., Williams, J., et al. (2009). “MICORE: Dune erosion and overwash model validation with data from nine European field sites,” in Proceedings of Coastal Dynamics 2009, Vol. 9, Singapore: World Scientific, 1–15.
Vousdoukas, M. I., Ferreira, O., Almeida, L. P., and Pacheco, A. (2012). Toward reliable storm-hazard forecasts: XBeach calibration and its potential application in an operational early-warning system. Ocean Dynam. 62, 1001–1015. doi: 10.1007/s10236-012-0544-6
Keywords: sea level rise, shoreline changes, beach erosion, Mediterranean Sea, coastal protection
Citation: Enríquez AR, Marcos M, Falqués A and Roelvink D (2019) Assessing Beach and Dune Erosion and Vulnerability Under Sea Level Rise: A Case Study in the Mediterranean Sea. Front. Mar. Sci. 6:4. doi: 10.3389/fmars.2019.00004
Received: 15 November 2018; Accepted: 07 January 2019;
Published: 25 January 2019.
Edited by:
Juan Jose Munoz-Perez, University of Cádiz, SpainReviewed by:
Antonio Contreras De Villar, University of Cádiz, SpainPushpa Dissanayake, Christian-Albrechts-Universität zu Kiel, Germany
Copyright © 2019 Enríquez, Marcos, Falqués and Roelvink. This is an open-access article distributed under the terms of the Creative Commons Attribution License (CC BY). The use, distribution or reproduction in other forums is permitted, provided the original author(s) and the copyright owner(s) are credited and that the original publication in this journal is cited, in accordance with accepted academic practice. No use, distribution or reproduction is permitted which does not comply with these terms.
*Correspondence: Alejandra R. Enríquez, YXJvZHJpZ3VlekBpbWVkZWEudWliLWNzaWMuZXM=