- Department of Marine Chemistry, Leibniz-Institute for Baltic Sea Research, Warnemünde, Germany
Evaluating the health status of marine ecosystems becomes ever increasingly important especially against the backdrop of rising pressures from human activities. This is true especially for coastal seas such as the Baltic Sea that is surrounded by highly industrialized countries. Nutrients and pollutants such as nitrate and hydrogen sulfide, which have a major impact on ecosystem functioning, are two of several key environmental indicators for assessing the status of natural waters, and therefore of considerable interest. The frequency and the spatial coverage of the nitrate and hydrogen sulfide measurements are currently limited by the cost of the laboratory analysis and personnel. Optical in situ sensors can help to overcome this challenge by allowing reagentless and fast detection of dissolved chemical species. A chemical-free optical sensor has been used for direct and simultaneous measurements of both key parameters, and the results were compared with traditional methods. The data were collected during an observational program conducted in the Baltic Sea in February 2018. We used the OPUS UV spectral sensor, which was deployed for the first time in coastal waters, in combination with a deep-sea telemetry system to enable near-real time measurements during CTD profiling. Data processing was carried out using a multiple linear regression procedure. Measurements from both OPUS and on-board analysis were in good agreement. The results showed, that in situ UV-VIS spectrophotometry provides the capability to determine the concentration distributions of nitrate and hydrogen sulfide in the brackish waters of the Baltic Sea.
Introduction
Throughout the world, agriculture, fossil-fuel combustion, and other human activities are leading to increasing levels of reactive nitrogen in the environment (Galloway et al., 2003; Gruber and Galloway, 2008). As a result, human health and ecosystem functions increasingly suffer from the effects of eutrophication and hypoxia, both at regional and global scales (Diaz and Rosenberg, 2008; Conley et al., 2009, 2011; Villnäs et al., 2013). The Baltic Sea is a semi-enclosed sea surrounded by highly industrialized riparian states and is particularly sensitive to anthropogenic impacts and natural fluctuations (Matthäus, 1995).
High reactive nitrogen levels cause increased biomass production (Falkowski et al., 1998) and thus increased sedimentation of organic material. Subsequent remineralization leads to bottom water oxygen deficiency, increased sulfate reduction and ultimately to the production of hydrogen sulfide (H2S), which is highly toxic to most organisms (Gray et al., 2002). In the Baltic Sea, as deep water renewal is hampered by the strong pycnocline and submarine sills, total sulfide (∑S2− = H2S + HS− + S2−) accumulates in the water column and can reach concentrations of up to 200 μM. These stagnation periods can last for many years and are only interrupted by inflow events from the North Sea under certain meteorological conditions (Schinke and Matthäus, 1998).
Nitrate (), as the major component of reactive nitrogen as well as H2S, are therefore of great importance for the ecological state of the Baltic Sea and thus are monitored by the coastal countries, which have adopted the Helsinki Convention for the Protection of the Marine Environment of the Baltic Sea in order to abate eutrophication by reducing nutrient loads from point and non-point sources (Backer et al., 2010). Many methods for the detection of (Moorcroft et al., 2001) and H2S (Lawrence et al., 2000) have been developed, but in most marine laboratories the determination of both compounds is usually carried out via simple colorimetric measurements (Grasshoff et al., 2009). The frequency and the spatial coverage of these measurements are currently limited by the cost of the laboratory analysis and personnel. Furthermore, the sampling process and preparation of the mostly toxic reagents is time consuming and involves multiple sources of error. However, with the help of in situ sensors, it is possible to reduce the working time as well as contamination and altering of the sample. In addition, in situ sensors have a small size, low weight and relatively low energy consumption, and therefore are suitable for being deployed on moorings (Collins et al., 2013; Sakamoto et al., 2017), floats (Johnson et al., 2013, 2017; D'Ortenzio et al., 2014; Pasqueron de Fommervault et al., 2015), towed vehicles (Pidcock et al., 2010) or autonomous underwater vehicles (Johnson and Needoba, 2008). In situ sensors are thus helping to bridge the gap between large-scale but non-specific remote observations of satellites limited to the surface waters and highly sophisticated and specific laboratory analysis from discrete water samples (Zielinski et al., 2009).
In general, there are three different types of in situ sensors that can be used for measurements: (1) wet chemical analyzers, (2) optical UV sensors, and (3) ion selective electrodes (ISEs). Each of these methods has its own advantages and disadvantages. Wet chemical analyzers such as the WIZ probe (Vuillemin and Sanfilippo, 2010) or Lab-on-chip devices (Beaton et al., 2012) are using chemical reagents and thus produce high maintenance toxic waste. Furthermore, operating lifetime of those analyzers is strongly dependent on reagent stability. On the other side, they can be calibrated in situ and thus have small uncertainty of measurement. However, the preparation of stable standard solutions for H2S and measurements is difficult as the shelf life of these standards is limited. By contrast, optical UV sensors such as Nitratax (Hach Lange GmbH, Germany), ISUS/SUNA (Johnson and Coletti, 2002; Johnson et al., 2006; MacIntyre et al., 2009) (Satlantic, Canada), or ProPS/OPUS (Zielinski et al., 2007; Prien et al., 2009) (TriOS GmbH, Germany) do not require any chemical reagents but are influenced by optical/ionic interferences including bromide (Br−), chloride (Cl−), turbidity and water color. ISEs such as NISE (Hach Lange GmbH, Germany) and EXO (YSI Inc., USA) are not influenced by turbidity and water color, but deteriorate quite rapidly when used under environmental conditions since the sensor molecules in the membrane change or are leached out. Therefore, they are often subject to significant drift and require regular re-calibration (Moorcroft et al., 2001; Le Goff et al., 2002). UV sensor technology is therefore used most frequently and has been increasingly used for measurements in a variety of environments such as rivers (Pellerin et al., 2009; Wade et al., 2012; Feng et al., 2013; Aubert and Breuer, 2016; Chappell et al., 2017; Kunz et al., 2017), groundwaters (Huebsch et al., 2015), coastal waters (Zielinski et al., 2011; Frank et al., 2014), open ocean waters (Omand and Mahadevan, 1966; Johnson, 2010; Ascani et al., 2013) and hydrothermal vents (Le Bris et al., 2000).
The aims of this study were to validate and optimize an optical in situ method for the precise and accurate simultaneous measurement of and HS− in brackish waters of the Baltic Sea. We used the OPUS UV spectral sensor (TriOS, Oldenburg, Germany), which was deployed for the first time in coastal waters, allowing improved observations of both compounds. Near-real time measurements and online sensor control was realized by using a deep-sea telemetry system integrated into the conductivity-temperature-depth (CTD) rosette sampler. Furthermore, detailed protocols have been developed for both sensor calibration and data evaluation. Finally, results were confirmed by comparison with conventional measurements made by an independent laboratory based method.
Materials and Methods
Instrumentation
During CTD profiling, high-frequency measurements were conducted using an OPUS UV spectral sensor (TriOS GmbH, Germany) deployed on a CTD-rosette sampler. The sensor is highly portable, light weight (titanium; 2 kg) and has moderate power consumption (< 8 W). The system is approximately 470 mm long with a diameter of 48 mm, has an open optical path length of 10 mm and can be deployed to a maximum depth of 300 m. It utilizes a xenon flash lamp and a 256 channel high-end miniature spectrometer (MMS, Zeiss, Jena, Germany) that covered the spectral range 200– 360 nm. The light source intensity of the xenon lamp is monitored by a reference photodiode and changes caused by temperature and aging phenomena are taken into account by an internal function of the sensor software. Measurements were carried out at 20 s intervals using an integration time of 256 ms. The lowering speed of the rosette sampler of 0.3 m/s thus resulted in a vertical resolution of 6 m and a smearing of the signal of 0.08 m for the OPUS measurements. Time synchronization was realized via coordinated universal time (UTC), which was used by all in situ instruments and allowed for the direct comparison of OPUS data with CTD measurements. A segmented flow analyzer (FlowSys, Alliance Instruments, Austria) for on-board spectrophotometric detection of was used as a reference method. In case of ∑S2−, collected water samples were analyzed by the methylene blue method (Cline, 1969) and the resulting concentration values were used for sensor validation. Limit of detection for and ∑S2− was 0.1 μM and 1 μM, respectively.
Online control of the sensor was realized by using a deep-sea telemetry system (Sea & Sun Technology GmbH) integrated into the CTD rosette sampler. Hydrographic data were collected using a standard CTD system (Model SBE911Plus; Sea-Bird Electronics Inc., USA). Turbidity was measured at 700 nm using the ECO-FLNTU sensor (WETLabs, USA). Profiling was carried out with active heave compensation using a special hydraulic crane winch (Krüger, S., and Ruickoldt, J., inventors; Leibniz-Institute for Baltic Sea Research Warnemünde, assignee. Measurement of measured quantities and/or sampling under water. Germany patent DE 102014201815. 2014 Jan 31). All measurements were stored in the database of the Leibniz Institute for Baltic Sea Research (IOWDB). Free registration is required for open access to IOWDB data (https://odin2.io-warnemuende.de/registration).
Field Deployments
Field studies were conducted during a RV Elisabeth Mann Borgese monitoring cruise in the Baltic Sea (Cruise No.: EMB175; from 30.01.2018 to 09.02.2018), which is the largest brackish water ecosystem on earth (average depth: 52 m; maximum depth: 459 m, surface area: 4.2 × 105 km2, volume: 22 × 103 km3 (Seifert et al., 1995; HELCOM, 2002). It is a semi-enclosed, non-tidal estuary consisting of several sub-basins and sills. The deep water of the central basins can become temporary or permanently euxinic (anoxic and sulfidic) due to biodegradation processes (Piker et al., 1998). In this area, stratification and topographic features (e.g., sills, trenches) have a great impact on hydrodynamics with respect to mixing and water renewal processes (Matthäus and Schinke, 1999; Elken and Matthäus, 2008). Ventilation of the deeper layers is occurring only under specific meteorological conditions (strong and long lasting easterly winds followed by strong and long lasting westerly winds) which then cause an inflow of high saline and oxygen-rich water masses from the North Sea (Schinke and Matthäus, 1998). The last strong Major Baltic Inflow (MBI) event that led to a renewal of bottom waters in many parts of the Baltic Sea occurred in December 2014 (Mohrholz et al., 2015). During our study, however, the development of a new stagnation period could be observed (Leibniz Institute for Baltic Sea Research, 2016). The field studies included three sensor deployments at multiple sites (Figure 1A) each representing a certain area (Bornholm Basin, Eastern Gotland Basin, Western Gotland Basin). All of these stations belong to the Baltic Proper, which is most affected by eutrophication and hypoxia. In addition, changing environmental conditions (e.g., decreasing salinity and increasing H2S concentrations) from the Bornholm Basin over the Eastern Gotland Basin to the Western Gotland Basin (Figure 3) allowed us to more accurately investigate the suitability of the applied method.
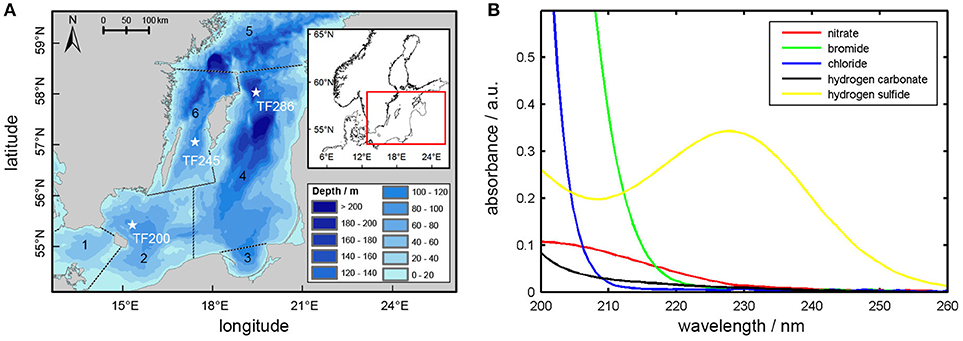
Figure 1. (A) Map of the study area including the locations of OPUS deployments indicated by white stars; 1 = Arkona Basin, 2 = Bornholm Basin, 3 = Gulf of Gdansk, 4 = Eastern Gotland Basin, 5 = Northern Baltic Proper, 6 = Western Gotland Basin. (B) UV-spectra of standard solutions ( μM; μM; mM; μM; μM) measured in the laboratory by using the OPUS sensor.
Optical Measurements
Interfering Ions
The Baltic Sea, like all natural waters, is a demanding environment for optical in situ measurements due to interfering ions which have high absorbances in the same wavelength range as and HS−. Interfering ions include Br− (Ogura and Hanya, 1966), Cl− (Finch et al., 1998), nitrite () (Johnson and Coletti, 2002), hydrogen carbonate () (Kröckel et al., 2011), and certain functional groups of the dissolved organic carbon (DOC) pool (Stedmon and Nelson, 2014). Sample absorption spectra are thus always a superposition of the absorption spectra of several different species. Hence, a mathematical approach has to be applied in order to decompose the obtained spectra. For the deconvolution and subsequent calculation of the analyte concentrations, a multiple linear regression method (henceforth referred to as MLR) was chosen (Thomas and Gallot, 1990) and implemented in the MATLAB environment (Mathworks, R2014a). A detailed description of this procedure is given in the subsection “Data Processing.”
For the regression to work, it needs the individual absorption spectra of all ions involved. For this reason, standard solutions of typical Baltic Sea concentrations were prepared for all relevant ions (, HS−, Br−, Cl−, ) and absorption spectra were measured in the laboratory by using the OPUS sensor. An exception is CDOM (colored dissolved organic matter), the optically active part of DOC, since its composition is widely unknown and highly variable. It should be noted at this point that different approaches to the treatment of CDOM interferences exist in the literature (Johnson and Coletti, 2002; Sakamoto et al., 2009; Zielinski et al., 2011). Usually, a simple linear or quadratic function is used during data processing to take into account background signals caused by CDOM. However, the influence of CDOM on OPUS measurements will be discussed later in conjunction with our findings. The resulting absorption spectra of the standard solutions are shown in Figure 1B. Br− represents the main interfering ion, followed by Cl− and . Nitrite was neglected as concentrations found during our study were below 0.2 μM. In the case of HS−, the pH of the standard solution was adjusted to a pH of 7.3 by using a KH2PO4/Na2HPO4 buffer solution to provide pH conditions similar to the in situ conditions at the study area, as the dissociation of H2S is pH-dependent (Millero et al., 1988).
Temperature Effect
Temperature dependencies must be taken into account when measuring calibration spectra, as otherwise accuracy of the regression results decreases (Zielinski et al., 2007). For this reason, temperature studies for the standard solutions of , HS−, Br−, and Cl− were made. Standard solutions were first cooled down by using an ice bath and then slowly warmed up by putting the ice bath aside. While slowly warming up, the solutions were then pumped through the OPUS sensor by using a flow cell in which the measurements were carried out every 20 s. Temperature measurements were realized by using an Arduino-controlled DS18B20 temperature sensor with a sensitivity of 0.5°C mounted in the flow cell.
The results can be seen in Figure 2A, where absorbances at a wavelength of 210 nm are plotted as a function of temperature. As can be seen from this figure the Cl− absorbance at 210 nm is rather insensitive to temperature changes, while Br− and HS− absorbances at this wavelength are strongly sensitive to temperature. For Br− and Cl− this interrelationship has already been demonstrated by other workers (Zielinski et al., 2007; Sakamoto et al., 2009) and is probably due to the interaction of the dissolved ions with water, i.e., due to a charge transfer to solvent complex (Jortner et al., 1964). Similar considerations could be made for HS−, but as far as we know there have been no studies on its photochemistry, yet. In fact, and to the best of our knowledge, our data is showing for the first time the temperature dependence of HS− absorbance in the deeper UV region. In contrast, the absorbance shows no temperature dependence at all owing to the π to π* transition, which occurs only within the molecule and without any solvent interaction (Mack and Bolton, 1999).
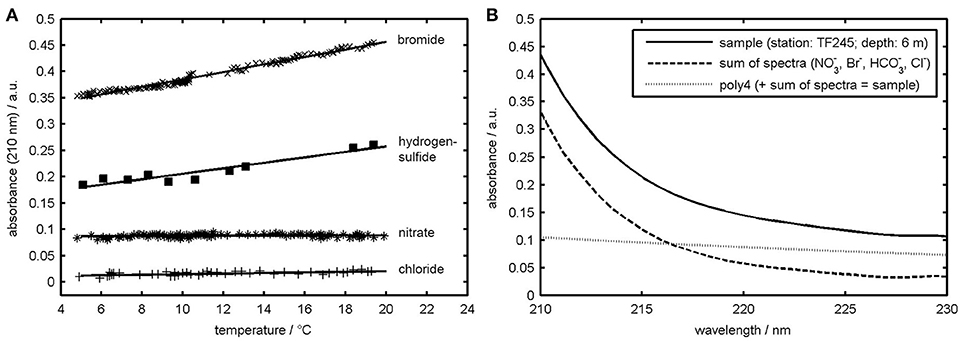
Figure 2. (A) OPUS absorbance of Br−, HS−, , and Cl− at 210 nm wavelength plotted as a function of temperature. Equations for the regression lines are: Br− = 0.007*x+0.314 (R2 = 0.98), HS− = 0.005*x+0.154 (R2 = 0.90), x+0.087 (R2 = 0.03), Cl− = 0.001*x+0.010 (R2 = 0.47). (B) Sample absorption spectrum measured at station TF245 at 4.1 °C (solid line) and UV-spectra resulting from the multiple linear regression procedure. Added up “sum of spectra” and “poly_4” give the solid line.
Data Processing
The absorbance at each pixel of the detector array was determined from following equation: A(λ) = -log((Iλ−Id)/(Iλ, 0−Id)), where Iλ is the detector current (counts) after light passing through the sample, Iλ, 0 detector current after light passing Milli-Q water (resistance ≥ 18 MΩcm−1, Merck Millipore, Bellerica, USA) as reference and Id the detector current without light source (dark current measurement). Each time a spectrum of a calibration solution was measured, a Milli-Q water spectrum was recorded to determine Iλ, 0.
The following equations, using the example of , illustrate the steps taken to obtain and HS− concentrations respectively.
First, a temperature corrected Cl− and a spectrum were subtracted from the respective sample absorption spectrum as both components are potential interferents (see Equation 1). They were not included in the MLR procedure but subtracted from the sample spectrum in order to reduce the number of unknowns in the subsequent regression. As the salinity of the sample differs from the salinity of the Cl− calibration spectrum (S = 8) a simple correction (multiplication by a factor) was carried out using salinity data from the CTD probe. The -values so were obtained by dividing CTD salinity by 8, since the Cl− calibration solution had a salinity of 8. In the case of the calibration solution ( μM) multiplication factors were set to 1 (< 60 m), 1.1 (> 60 and < 80 m), and 1.2 (> 80m), to provide total CO2 conditions similar to the in situ conditions at the study area (Beldowski et al., 2010). In other environments, such as the North Atlantic, the multiplication factors would have to be chosen differently, since the total CO2 concentrations differ from those of the Baltic Sea (Takahashi, 1981). Calibration spectra (absorbances) of , HS−, and Br− were then combined to form a matrix M (henceforth referred to as calculation group) that was used in the MLR procedure (see Equation 2). Furthermore, a poly_4 spectrum (λ−4) was added to the calculation group in order to take into account that scattered light can also contribute to the sample absorption signal.
As we will see later in the discussion part, other non-scattering components can also be added to the poly_4 spectrum during the regression, if the shape of the spectra are not very different from each other. Finally, the MLR (210– 230 nm wavelength range) was carried out depending on the dissolved oxygen concentrations (O2) measured at each depth. When O2 was present (> 1 μM) the calculation group included Br−, poly_4 and . In contrast, when O2 was absent (< 1 μM), the calculation group included Br−, poly_4, and HS−. All calibration spectra within the calculation group were selected from the temperature study to be as close as possible to the in situ temperature conditions. This is of considerable importance for Br− and HS− as can be seen from Figure 2A. Polynomial coefficients (p) obtained from MLR were then used for calculating the , HS−, and Br− concentrations as well as the poly_4 contribution (see Equation 3).
Results
During a monitoring cruise in the Baltic Sea in February 2018, in situ measurements were carried out with the OPUS. The OPUS was mounted on the CTD rosette sampler and could be controlled online via a deep-sea telemetry system. The hydrographic data of the measured stations (TF200, TF286, TF245) and the results of the MLR are shown in Figure 3. In addition, summarized results of laboratory and sensor measurements can be found in Table 1. The CTD data (Figures 3A,D,G) indicated a stratified water column with cold, low-saline surface water and warm, saline deep water. O2, temperature and salinity values were fairly uniform in the surface layer at all three stations due to the winter mixing (TF200: 357 μM, 4.6°C, 7.5; TF286: 358 μM, 4.5°C, 7.3; TF245: 359 μM, 4.1°C, 7.3). Below 50 m depth, the salinity and the temperature increased and reached nearly constant values in the bottom water (TF200: 6.8°C, 17.3; TF286: 6.8°C, 12.8; TF245: 5.6°C, 10.3). In contrast, the O2 concentrations sharply decreased from 50 m depth so that no O2 was present in the bottom water of all three stations. In addition, intrusions of O2-rich water could be located at station TF200 and TF286 at 60 m and 125 m depth, respectively. When O2 was not present, ∑S2− was determined using the methylenblue method (MBM) as well as the OPUS sensor (Figures 3B,E,H). Concentrations close to the bottom obtained by MBM were 4.3 μM (TF200), 14.6 μM (TF286) and 33.6 μM (TF245). Concentrations of ∑S2− obtained by the OPUS sensor at about the same depths were 5.6 μM (TF200), 16.9 μM (TF286), and 34.3 μM (TF245). Furthermore, was measured using a segmented flow analyzer (SFA) and the OPUS sensor (Figures 3B,E,H). At the surface, concentrations were consistently high and slowly increasing northwards. Concentrations above 50 m depth obtained by SFA were in average 2.4 μM ± 0.1 μM (TF200), 3.6 μM ± 0.04 μM (TF245), and 3.9 μM ± 0.02 μM (TF286). Concentrations of obtained by the OPUS sensor above 50 m depth were in average 2.5 μM ± 0.2 μM (TF200), 3.3 μM ± 0.2 μM (TF245), and 5.9 μM ± 0.2 μM (TF286). maxima were observed at station TF200 and station TF286 at 60 m and 125 m depth, respectively, and coincided with above-mentioned O2 intrusions.
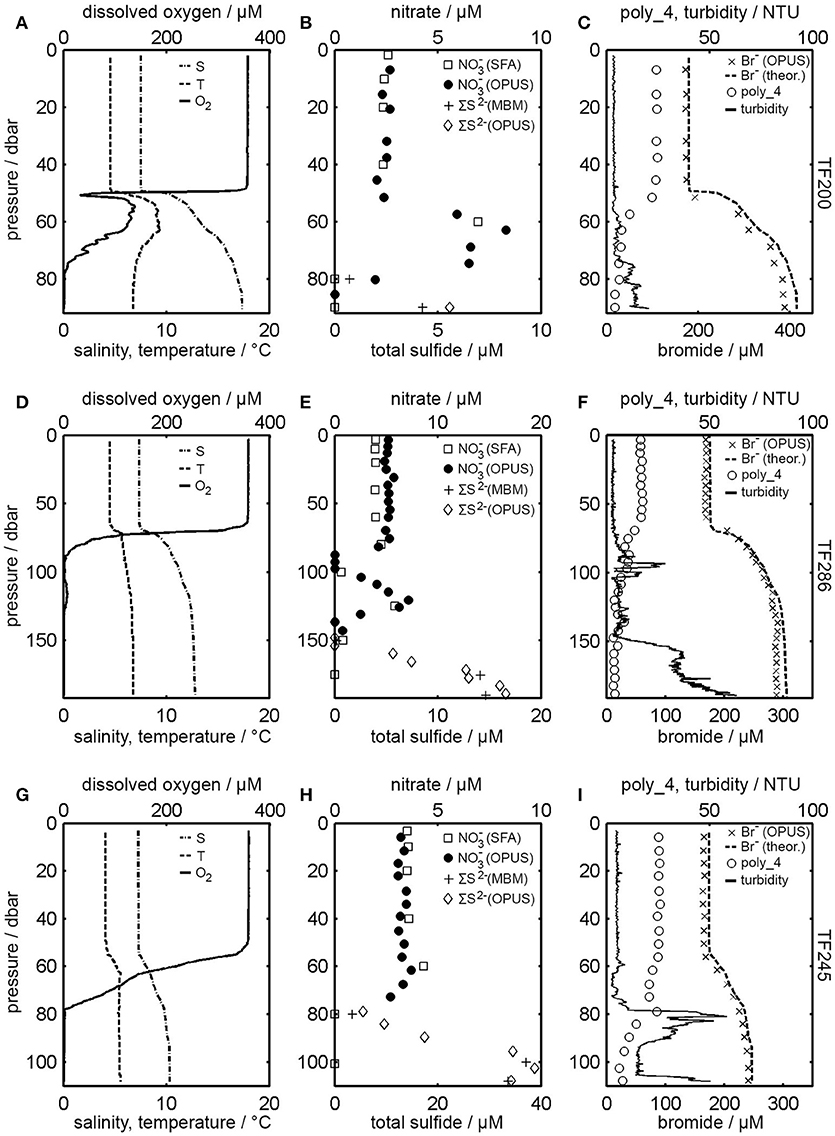
Figure 3. Data from the February 2018 cruise. (A,D,G) Vertical profiles of salinity, temperature and O2 concentration; (B,E,H) Comparison of bottle measured /∑S2− data and OPUS results.(C,F,I) Theoretical Br− concentrations (calculated using salinity data), Br− concentrations measured by OPUS, turbidity data from CTD measurements (multiplied by 50), and poly_4 results presented as “percentage of sample absorption at a wavelength of 210 nm”.
Additional parameters (Br−, turbidity, and poly_4) are shown in Figures 3C,F,I. Br− was measured with the OPUS sensor but was also calculated from salinity using CTD data whereby conservative behavior was assumed. Turbidity (reported in nephelometric turbidity units or NTU) was measured in parallel with temperature and salinity and was multiplied by 50 for plotting in the figures. In the upper water column turbidity values were rather low. High turbidity was observed especially in the euxinic deep water where O2 was absent. Furthermore, poly_4 values resulting from the MLR are also shown Figure 3 and are presented as “percentage of sample absorption at a wavelength of 210 nm” in order to show how much of the sample absorbance could not be allocated to any of the calibration spectra included in the calculation group. Poly_4 values were about 24% (TF200), 25% (TF245), and 17% (TF286) in the surface water and 4% (TF200), 8% (TF245), and 4% (TF286) in the bottom water.
Discussion
OPUS and laboratory results were measured at different depths and thus a direct correlation of the concentration data would be inappropriate. Instead, averaged concentrations above a depth of 50 m, as presented in the results section were compared for each station. Resulting deviations for were 4% (TF200), 8% (TF245), and 51% (TF286). In the case of ∑S2− the bottom water concentrations between bottle data and OPUS data were compared. Deviations found here were 30% (TF200), 2% (TF245), and 14% (TF286). As mentioned above, dissociation of H2S is pH-dependent and the calibration solution used in this study was buffered to a constant pH of 7.3. Therefore, the reported deviations could be explained by variations in the in situ pH of the study area. However, considering that the highest deviations observed for (51%) and ∑S2− (30%), correspond to only 2 and 1.3 μM, respectively, it can be stated that and ∑S2− measurements carried out at the Baltic Sea using the OPUS sensor are in satisfactory agreement with those obtained from reference methods. Although, the findings of our study are limited by the small number of measured stations, presented results show that both and ∑S2−, as important environmental parameters, can be determined in situ, simultaneously and without reagents during classical CTD work, providing near real time readings with sufficient accuracy without time and cost intensive traditional laboratory analysis. That the deconvolution of the sample spectra was successful is also shown by the MLR results of the other parameters that were included in the calculation groups. For instance, OPUS Br− concentrations and theoretical Br− concentrations correspond very well with each other (Figures 3C,F,I). Maximum deviations were found in the bottom water and were only 6% (TF200), 3% (TF245), and 6% (TF286). It should be noted here, that pressure effects on bromide absorbance were neglected in our study because we have worked in shallow waters, but should be taken into account at higher pressures (Sakamoto et al., 2017).
But although , Br−, and ∑S2− concentration data of the OPUS sensor fit very closely with the laboratory and theoretical data and all features of the concentration distributions are reproduced some questions still remain. Figure 3B, for example, shows a sample absorption spectrum from station TF245 at 6 m depth and the results of the deconvolution using the calibration spectra during the MLR. The sum of the , Br−, HCO, Cl−, and poly_4 spectrum would give the sample spectrum. The polynomial coefficients provided by the MLR with regard to the light scattering term (poly_4) seem to be remarkable high. This is especially true for the upper water column as can be seen from Figure 3, where up to 25% of the sample absorption is allocated to the poly_4 spectrum.
On the one side, we assume that light scattering actually could be increased by suspended particulate matter (SPM), as certain colloidal fractions can have scattering coefficients significantly greater than pure sea water (Stramski and Woźniak, 2005). On the other hand, we think that absorption fractions of CDOM were added to the poly_4 term during the regression, since we did not add such a calibration spectrum to the calculation groups. This assumption is underpinned by the striking inverse correlation between poly_4 and salinity (Figure 3), as it has also been found for CDOM in other coastal waters (Fichot and Benner, 2011). Although CDOM should be the most relevant parameter at this point, one aspect of future research thus could be to investigate the impact of both the particulate and the dissolved components of organic material on in situ UV measurements. Since the optical properties of CDOM have been found to be empirically related to DOC concentration (Fichot and Benner, 2011) further research could also help to get a better understanding of DOC cycling. This could be of considerable interest, since dissolved organic material (DOM) and DOC, as a major component of the DOM pool, can enhance eutrophication and pollution of natural waters, for instance, by the mobilization of phosphorous and heavy metals (Fest et al., 2008; Ros et al., 2010). Since the aim of the current work was to detect and ∑S2−, correction for this offset as performed by other workers (Sakamoto et al., 2009; Zielinski et al., 2011) was not carried out.
Although, the findings of our study are clearly limited by the small number of measured stations, the OPUS sensor in combination with deep-sea telemetry allowed in situ determination of and ∑S2− in the Baltic Sea, enhancing monitoring data set. This approach increases the simplicity of the determination of both parameters and the speed of data acquisition. Concentration gradients as well as maxima and minima can be determined more accurately as the spatial resolution of the data can be higher than using traditional bottle sampling. The OPUS is capable of resolving variability on a scale of 6 m at a CTD lowering speed of 0.3 m/s (OPUS sampling rate: 0.05 Hz). At lower profiling speed the vertical resolution could be even higher. A future cooperation with the manufacturer TriOS (Oldenburg, Germany) could help to further improve the sampling rate. Meanwhile, we have received a new firmware allowing OPUS measurements at a sampling rate of 0.1 Hz which results in 3 m vertical resolution. A relevant step to resolve e.g., steep gradients in the water column.
Finally, it can be concluded that there is a clear need of innovative and cost-effective monitoring (Zielinski et al., 2009; Borja and Elliott, 2013) and using sensors such as OPUS is a smart way of gathering relevant information quickly. Modern oceanographic tools like the instrument described here should be more widely used. This is especially important to identify anthropogenic impact (e.g., in the form of sporadic events) and their consequences for the marine ecosystems. The affected processes can have a wide range of temporal and spatial scales, requiring frequent and distributed observations. Only then can the feedbacks be unraveled and the ecosystem status adequately assessed.
Author Contributions
DM, LR, MP, and RP conceived and designed the experiments. LR, MP, and DM performed the experiments. DM and LR compiled and processed all the field and laboratory data. DS-B, RP, and JW contributed materials, reagents, analysis tools. DM wrote the paper, with edits and contributions from all co-authors. RP, JW, and DS-B directed the study and its scope.
Funding
Parts of this work have been carried out within the BONUS SEAMOUNT project (BMBF 03F0771C).
Conflict of Interest Statement
The authors declare that the research was conducted in the absence of any commercial or financial relationships that could be construed as a potential conflict of interest.
Acknowledgments
We thank the captain and the crew of RV Elisabeth Mann Borgese (EMB175). We acknowledge in particular Susanne Schöne and Lars Kreuzer for sampling and conducting on-board measurements of O2, ∑S2− and concentrations. Thanks are due to Siegfried Krüger for his help with the deep-sea telemetry system. Furthermore, the support of Birgit Sadkowiak during the lab work is greatly appreciated. We are thankful for the technical support we experienced from TriOS GmbH (Oldenburg, Germany). DM would also like to thank Anne Köhler for measuring ∑S2− concentrations during the sensor calibration experiments. The authors thank the three reviewers for their detailed and valuable comments that helped improve the manuscript.
References
Ascani, F., Richards, K. J., Firing, E., Grant, S., Johnson, K. S., Jia, Y., et al. (2013). Physical and biological controls of nitrate concentrations in the upper subtropical North Pacific Ocean. Deep Sea Res. II Top. Stud. Oceanogr. 93, 119–134. doi: 10.1016/j.dsr2.2013.01.034
Aubert, A. H., and Breuer, L. (2016). New seasonal shift in in-stream diurnal nitrate cycles identified by mining high-frequency data. PLoS ONE 11:e0153138. doi: 10.1371/journal.pone.0153138
Backer, H., Leppänen, J.-M., Brusendorff, A. C., Forsius, K., Stankiewicz, M., Mehtonen, J., et al. (2010). HELCOM Baltic Sea Action Plan–a regional programme of measures for the marine environment based on the ecosystem approach. Mar. Pollut. Bull. 60, 642–649. doi: 10.1016/j.marpolbul.2009.11.016
Beaton, A. D., Cardwell, C. L., Thomas, R. S., Sieben, V. J., Legiret, F.-E., Waugh, E. M., et al. (2012). Lab-on-chip measurement of nitrate and nitrite for in situ analysis of natural waters. Environ. Sci. Technol. 46, 9548–9556. doi: 10.1021/es300419u
Beldowski, J., Löffler, A., Schneider, B., and Joensuu, L. (2010). Distribution and biogeochemical control of total CO2 and total alkalinity in the Baltic Sea. J. Mar. Syst. 81, 252–259. doi: 10.1016/j.jmarsys.2009.12.020
Borja, A., and Elliott, M. (2013). Marine monitoring during an economic crisis: the cure is worse than the disease. Mar. Pollut. Bull. 1, 1–3. doi: 10.1016/j.marpolbul.2013.01.041
Chappell, N. A., Jones, T. D., and Tych, W. (2017). Sampling frequency for water quality variables in streams: systems analysis to quantify minimum monitoring rates. Water Res. 123, 49–57. doi: 10.1016/j.watres.2017.06.047
Cline, J. D. (1969). Spectrophotometric determination of hydrogen sulfide in natural waters. Limnol. Oceanogr. 14, 454–458. doi: 10.4319/lo.1969.14.3.0454
Collins, J. R., Raymond, P. A., Bohlen, W. F., and Howard-Strobel, M. M. (2013). Estimates of new and total productivity in central Long Island Sound from in situ measurements of nitrate and dissolved oxygen. Estuar. Coasts 36, 74–97. doi: 10.1007/s12237-012-9560-5
Conley, D. J., Björck, S., Bonsdorff, E., Carstensen, J., Destouni, G., Gustafsson, B. G., et al. (2009). Hypoxia related processes in the baltic sea. Environ. Sci. Technol. 43, 3412–3420. doi: 10.1021/es802762a
Conley, D. J., Carstensen, J., Aigars, J., Axe, P., Bonsdorff, E., Eremina, T., et al. (2011). Hypoxia is increasing in the coastal zone of the Baltic Sea. Environ. Sci. Technol. 45, 6777–6783. doi: 10.1021/es201212r
Diaz, R. J., and Rosenberg, R. (2008). Spreading dead zones and consequences for marine ecosystems. Science 321, 926–929. doi: 10.1126/science.1156401
D'Ortenzio, F., Lavigne, H., Besson, F., Claustre, H., Coppola, L., Garcia, N., et al. (2014). Observing mixed layer depth, nitrate and chlorophyll concentrations in the northwestern Mediterranean: a combined satellite and NO3 profiling floats experiment. Geophys. Res. Lett. 41, 6443–6451. doi: 10.1002/2014GL061020
Elken, J., and Matthäus, W. (2008). Baltic Sea oceanography. Regional Climate Studies, Assessment of Climate Change for the Baltic Sea Basin Annex A, 379–385. doi: 10.1007/978-3-540-72786-6
Falkowski, P. G., Barber, R. T., and Smetacek, V. (1998). Biogeochemical controls and feedbacks on ocean primary production. Science 281, 200–206. doi: 10.1126/science.281.5374.200
Feng, Z., Schilling, K. E., and Chan, K.-S. (2013). Dynamic regression modeling of daily nitrate-nitrogen concentrations in a large agricultural watershed. Environ. Monitor. Assess. 185, 4605–4617. doi: 10.1007/s10661-012-2891-7
Fest, E. P., Temminghoff, E., Comans, R., and Van Riemsdijk, W. (2008). Partitioning of organic matter and heavy metals in a sandy soil: effects of extracting solution, solid to liquid ratio and pH. Geoderma 146, 66–74. doi: 10.1016/j.geoderma.2008.05.005
Fichot, C. G. and Benner, R. (2011). A novel method to estimate DOC concentrations from CDOM absorption coefficients in coastal waters. Geophys. Res. Lett. 38, 1–5. doi: 10.1029/2010GL046152
Finch, M. S., Hydes, D. J., Clayson, C. H., Weigl, B., Dakin, J., and Gwilliam, P. (1998). A low power ultra violet spectrophotometer for measurement of nitrate in seawater: introduction, calibration and initial sea trials. Anal. Chim. Acta 377, 167–177. doi: 10.1016/S0003-2670(98)00616-3
Frank, C., Meier, D., and Voß, D. Zielinski, O. (2014). Computation of nitrate concentrations in coastal waters using an in situ ultraviolet spectrophotometer: Behavior of different computation methods in a case study a steep salinity gradient in the southern North Sea. Methods Oceanogr. 9, 34–43. doi: 10.1016/j.mio.2014.09.002
Galloway, J. N., Aber, J. D., Erisman, J. W., Seitzinger, S. P., Howarth, R. W., Cowling, E. B., et al. (2003). The nitrogen cascade. AIBS Bull. 53, 341–356. doi: 10.1641/0006-3568(2003)053[0341:TNC]2.0.CO;2
Grasshoff, K., Kremling, K., and Ehrhardt, M. (2009). Methods of Seawater Analysis. Weinheim: John Wiley & Sons.
Gray, J. S., Wu, R. S.-S., and Or, Y. Y. (2002). Effects of hypoxia and organic enrichment on the coastal marine environment. Mar. Ecol. Prog. Ser. 238, 249–279. doi: 10.3354/meps238249
Gruber, N., and Galloway, J. N. (2008). An Earth-system perspective of the global nitrogen cycle. Nature 451:293. doi: 10.1038/nature06592
HELCOM (2002). “Environment of the Baltic Sea area 1994-1998,” in Baltic Sea Environment Proceedings, No. 82B, Helsinki Commission, Baltic Marine Environment Protection Commission (Helsinki), 1–215
Huebsch, M., Grimmeisen, F., Zemann, M., Fenton, O., Richards, K. G., Jordan, P., et al. (2015). Field experiences using UV/VIS sensors for high-resolution monitoring of nitrate in groundwater. Hydrol. Earth Syst. Sci. 19:1589. doi: 10.5194/hess-19-1589-2015
Johnson, K. S. (2010). Simultaneous measurements of nitrate, oxygen, and carbon dioxide on oceanographic moorings: observing the Redfield ratio in real time. Limnol. Oceanogr. 55, 615–627. doi: 10.4319/lo.2009.55.2.0615
Johnson, K. S., and Coletti, L. J. (2002). in situ ultraviolet spectrophotometry for high resolution and long-term monitoring of nitrate, bromide and bisulfide in the ocean. Deep Sea Res. I Oceanogr. Res. Pap. 49, 1291–1305. doi: 10.1016/S0967-0637(02)00020-1
Johnson, K. S., Coletti, L. J., and Chavez, F. P. (2006). Diel nitrate cycles observed with in situ sensors predict monthly and annual new production. Deep Sea Res. I Oceanogr. Res. Pap. 53, 561–573. doi: 10.1016/j.dsr.2005.12.004
Johnson, K. S., Coletti, L. J., Jannasch, H. W., Sakamoto, C. M., Swift, D. D., and Riser, S. C. (2013). Long-term nitrate measurements in the ocean using the in situ ultraviolet spectrophotometer: sensor integration into the Apex profiling float. J. Atmos. Ocean. Technol. 30, 1854–1866. doi: 10.1175/JTECH-D-12-00221.1
Johnson, K. S., and Needoba, J. A. (2008). Mapping the spatial variability of plankton metabolism using nitrate and oxygen sensors on an autonomous underwater vehicle. Limnol. Oceanogr. 53, 2237–2250. doi: 10.4319/lo.2008.53.5_part_2.2237
Johnson, K. S., Plant, J. N., Coletti, L. J., Jannasch, H. W., Sakamoto, C. M., Riser, S. C., et al. (2017). Biogeochemical sensor performance in the SOCCOM profiling float array. J. Geophys. Res. Oceans 122, 6416–6436. doi: 10.1002/2017JC012838
Jortner, J., Ottolenghi, M., and Stein, G. (1964). On the photochemistry of aqueous solutions of chloride, bromide, and iodide ions. J. Phys. Chem. 68, 247–255. doi: 10.1021/j100784a005
Kröckel, L., Schwotzer, G., Lehmann, H., and Wieduwilt, T. (2011). Spectral optical monitoring of nitrate in inland and seawater with miniaturized optical components. Water Res. 45, 1423–1431. doi: 10.1016/j.watres.2010.10.033
Kunz, J. V., Annable, M. D., Cho, J., von Tümpling, W., Hatfield, K., Rao, S., et al. (2017). Quantifying nutrient fluxes with a new hyporheic passive flux meter (HPFM). Biogeosciences 14:631. doi: 10.5194/bg-14-631-2017
Lawrence, N. S., Davis, J., and Compton, R. G. (2000). Analytical strategies for the detection of sulfide: a review. Talanta 52, 771–784. doi: 10.1016/S0039-9140(00)00421-5
Le Bris, N., Sarradin, P.-M., Birot, D., and Alayse-Danet, A.-M. (2000). A new chemical analyzer for in situ measurement of nitrate and total sulfide over hydrothermal vent biological communities. Mar. Chem. 72, 1–15. doi: 10.1016/S0304-4203(00)00057-8
Le Goff, T., Braven, J., Ebdon, L., Chilcott, N. P., Scholefield, D., and Wood, J. W. (2002). An accurate and stable nitrate-selective electrode for the in situ determination of nitrate in agricultural drainage waters. Analyst 127, 507–511. doi: 10.1039/b201899n
Leibniz Institute for Baltic Sea Research (2016). Hypoxic and anoxic regions in the Baltic Sea, 1969–2015. Marine Science Reports. Leibniz Institute for Baltic Sea Research, Warnemnde. Available online at: http://www.io-warnemuende.de/msr-2016-0100.html
MacIntyre, G., Plache, B., Lewis, M., Andrea, J., Feener, S., McLean, S., et al. (2009). “ISUS/SUNA nitrate measurements in networked ocean observing systems,” in OCEANS 2009, MTS/IEEE Biloxi-Marine Technology for Our Future: Global and Local Challenges (Biloxi: IEEE), 1–7.
Mack, J., and Bolton, J. R. (1999). Photochemistry of nitrite and nitrate in aqueous solution: a review. J. Photochem. Photobiol. A Chem. 128, 1–13. doi: 10.1016/S1010-6030(99)00155-0
Matthäus, W. (1995). Natural variability and human impacts reflected in longterm changes in the baltic deep water conditions a brief review. Deutsche Hydrografische Zeitschrift 47, 47–65. doi: 10.1007/BF02731990
Matthäus, W., and Schinke, H. (1999). “The influence of river runoff on deep water conditions of the Baltic Sea,” in Biological, Physical and Geochemical Features of Enclosed and Semi-enclosed Marine Systems, eds E. M. Blomqvist, E. Bonsdorff and K. Essink (Dordrecht: Springer), 1–10.
Millero, F. J., and Plese, T. Fernandez, M. (1988). The dissociation of hydrogen sulfide in seawater 1. Limnol. Oceanogr. 33, 269–274. doi: 10.4319/lo.1988.33.2.0269
Mohrholz, V., Naumann, M., Nausch, G., Krüger, S., and Gräwe, U. (2015). Fresh oxygen for the Baltic Sea: an exceptional saline inflow after a decade of stagnation. J. Mar. Syst. 148, 152–166. doi: 10.1016/j.jmarsys.2015.03.005
Moorcroft, M. J., Davis, J., and Compton, R. G. (2001). Detection and determination of nitrate and nitrite: a review. Talanta 54, 785–803. doi: 10.1016/S0039-9140(01)00323-X
Ogura, N., and Hanya, T. (1966). Nature of ultra-violet absorption of sea water. Nature 212:758. doi: 10.1038/212758a0
Omand, M. M., and Mahadevan, A. (1966). The shape of the oceanic nitracline. Biogeosciences 12, 3273–3287. doi: 10.5194/bg-12-3273-2015
Pasqueron de Fommervault, O., D'Ortenzio, F., Mangin, A., Serra, R., Migon, C., Claustre, H., et al. (2015). Seasonal variability of nutrient concentrations in the Mediterranean Sea: contribution of Bio-Argo floats. J. Geophys. Res. Oceans 120, 8528–8550. doi: 10.1002/2015JC011103
Pellerin, B. A., Downing, B. D., Kendall, C., Dahlgren, R. A., Kraus, T. E., Saraceno, J., et al. (2009). Assessing the sources and magnitude of diurnal nitrate variability in the San Joaquin River (California) with an in situ optical nitrate sensor and dual nitrate isotopes. Freshw. Biol. 54, 376–387. doi: 10.1111/j.1365-2427.2008.02111.x
Pidcock, R., Srokosz, M., Allen, J., Hartman, M., Painter, S., Mowlem, M., et al. (2010). A novel integration of an ultraviolet nitrate sensor on board a towed vehicle for mapping open-ocean submesoscale nitrate variability. J. Atmos. Ocean. Technol. 27, 1410–1416. doi: 10.1175/2010JTECHO780.1
Piker, L., Schmaljohann, R., and Imhoff, J. F. (1998). Dissimilatory sulfate reduction and methane production in Gotland Deep sediments (Baltic Sea) during a transition period from oxic to anoxic bottom water (1993 - 1996). Aquat. Microb. Ecol. 14, 183–193. doi: 10.3354/ame014183
Prien, R. D., and Meyer, D. Sadkowiak, B. (2009). “Optical measurements of nitrate and H2S concentrations in Baltic waters,” in Oceans'09 IEEE Bremen, 11.-14.5.2009 (Bremen), 1–5.
Ros, G. H., Tschudy, C., Chardon, W. J., Temminghoff, E. J., van der Salm, C., and Koopmans, G. F. (2010). Speciation of water-extractable organic nutrients in grassland soils. Soil Sci. 175, 15–26. doi: 10.1097/SS.0b013e3181c8ef3c
Sakamoto, C. M., Johnson, K. S., and Coletti, L. J. (2009). Improved algorithm for the computation of nitrate concentrations in seawater using an in situ ultraviolet spectrophotometer. Limnol. Oceanogr. Methods 7, 132–143. doi: 10.4319/lom.2009.7.132
Sakamoto, C. M., Johnson, K. S., Coletti, L. J., Maurer, T. L., Massion, G., Pennington, J. T., et al. (2017). Hourly in situ nitrate on a coastal mooring: a 15-year record and insights into new production. Oceanography 30, 114–127. doi: 10.5670/oceanog.2017.428
Schinke, H., and Matthäus, W. (1998). On the causes of major Baltic inflows–an analysis of long time series. Contin. Shelf Res. 18, 67–97. doi: 10.1016/S0278-4343(97)00071-X
Seifert, T., Kayser, B., and Tauber, F. (1995). Bathymetry Data of the Baltic Sea. Baltic Sea Research Institute, Warnemünde.
Stedmon, C. A., and Nelson, N. B. (2014). “The optical properties of DOM in the ocean,” in Biogeochemistry of Marine Dissolved Organic Matter, 2nd Edn, D. A. Hansell and C. A. Carlson (San Diego, CA: Elsevier), 481–508.
Stramski, D., and Woźniak, S. B. (2005). On the role of colloidal particles in light scattering in the ocean. Limnol. Oceanogr. 50, 1581–1591. doi: 10.4319/lo.2005.50.5.1581
Takahashi, T., Broecker, W. S., and Bainbridge, A. E. (1981). The alkalinity and total carbon dioxide concentration in the world oceans. Scope 16, 271–286.
Thomas, O., and Gallot, S. (1990). Ultraviolet multiwavelength absorptiometry (UVMA) for the examination of natural waters and wastewaters. Fresenius J. Anal. Chem. 338, 234–237.
Villnäs, A., Norkko, J., Hietanen, S., Josefson, A. B., Lukkari, K., and Norkko, A. (2013). The role of recurrent disturbances for ecosystem multifunctionality. Ecology 94, 2275–2287. doi: 10.1890/12-1716.1
Vuillemin, R., and Sanfilippo, L. (2010). A compact, low-power in-situ flow analyzer for marine applications. Sea Technol. 51, 29–32.
Wade, A. J., Palmer-Felgate, E., Halliday, S. J., Skeffington, R. A., Lowenthal, M., Jarvie, H., et al. (2012). Hydrochemical processes in lowland rivers: insights from in situ, high-resolution monitoring. Hydrol. Earth Syst. Sci. 16, 4323–4342. doi: 10.5194/hess-16-4323-2012
Zielinski, O., Busch, J., Cembella, A. D., Daly, K. L., Engelbrektsson, J., Hannides, A. K., et al. (2009). Detecting marine hazardous substances and organisms: sensors for pollutants, toxins, and pathogens. Ocean Sci. 5, 329–349. doi: 10.5194/os-5-329-2009
Zielinski, O., Fiedler, B., Heuermann, R., Körtzinger, A., Kopiske, E., Meinecke, G., et al. (2007). “A new nitrate continuous observation sensor for autonomous sub-surface applications: technical design and first results,” in IEEE, Proceedings of the International Conference on Marine Challenges: From Coastline to Deep Sea, Aberdeen (Aberdeen), 18–22.
Keywords: nutrients, sulfide, monitoring, Baltic Sea, UV-VIS spectrophotometry, hypoxia
Citation: Meyer D, Prien RD, Rautmann L, Pallentin M, Waniek JJ and Schulz-Bull DE (2018) In situ Determination of Nitrate and Hydrogen Sulfide in the Baltic Sea Using an Ultraviolet Spectrophotometer. Front. Mar. Sci. 5:431. doi: 10.3389/fmars.2018.00431
Received: 28 August 2018; Accepted: 29 October 2018;
Published: 20 November 2018.
Edited by:
Hervé Claustre, Centre National de la Recherche Scientifique (CNRS), FranceReviewed by:
Oliver Zielinski, University of Oldenburg, GermanyOrens de Fommervault, Alseamar, France
Carole M. Sakamoto, Monterey Bay Aquarium Research Institute (MBARI), United States
Copyright © 2018 Meyer, Prien, Rautmann, Pallentin, Waniek and Schulz-Bull. This is an open-access article distributed under the terms of the Creative Commons Attribution License (CC BY). The use, distribution or reproduction in other forums is permitted, provided the original author(s) and the copyright owner(s) are credited and that the original publication in this journal is cited, in accordance with accepted academic practice. No use, distribution or reproduction is permitted which does not comply with these terms.
*Correspondence: David Meyer, david.meyer@io-warnemuende.de