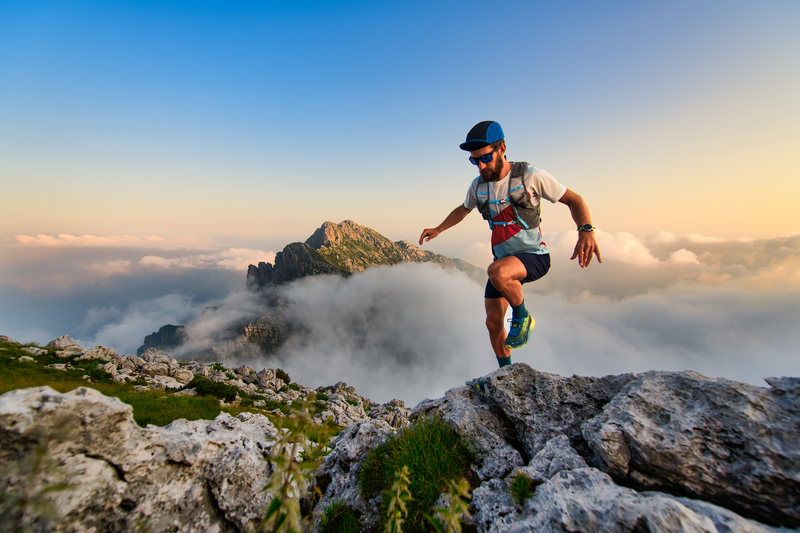
94% of researchers rate our articles as excellent or good
Learn more about the work of our research integrity team to safeguard the quality of each article we publish.
Find out more
OPINION article
Front. Mar. Sci. , 07 August 2018
Sec. Marine Evolutionary Biology, Biogeography and Species Diversity
Volume 5 - 2018 | https://doi.org/10.3389/fmars.2018.00280
For hundreds of millions of years, the biogeography of many marine species has been shaped by geological events at various times throughout Earth's history (Palumbi, 1996). The allopatric barriers that have emerged as a result of these long processes have contributed to the stunning diversity that exists in the world's oceans. Since the early works of John Avise and his proposal of using genes to assess biogeographical patterns of species i.e., “phylogeography” (Avise, 2008), marine ecologists have become heavily reliant on genetic data to investigate species dispersal on both global and regional scales. This involves the development of complex statistical models to measure everything from population structure and migration rates to divergence times. One reason for this popularity is due to the difficulty in tracking individual organisms in vast ocean basins, especially species that produce thousands to millions of offspring per female, which are then carried off by ocean currents (Levin, 2006 and references therein). Phylogeography has also been used in important applications such as the designing of marine protected areas (MPAs) (Von der Heyden et al., 2014), genetic assessment of fish stocks (Carvalho and Hauser, 1994), and tracking the spread of invasive species (Rius et al., 2015). Despite its popularity, phylogeographic assessments remain an indirect method of determining biogeographic patterns. It is limited because the statistical algorithms used, regardless of how complex they are, cannot completely incorporate the dynamic nature of the marine environment i.e., the “seascape” (Selkoe et al., 2008, 2010).
Here, I discuss one of the limitations of marine phylogeography that has not attracted much attention in the literature but is of particular importance due to the contemporary issue of biological invasions. This is the inability of molecular data to effectively detect panmixia due to the dilution and subsequent erosion of phylogeographic barriers caused by multiple introductory events. Also proposed is a solution for dealing with this issue which includes a combination of the traditional genetic approaches coupled with high resolution biophysical models.
The mass movement of alien fauna across marine biogeographic regions and the impacts associated is one of the greatest threats facing global biodiversity (Molnar et al., 2008). While the visible ecological impacts of these “introductory events” are often the major focus of invasion research, there lies a more inconspicuous threat: the potential for genetic homogenization (David and Loveday, 2018). Genetic homogeneity can reduce the genetic diversity of a species and in doing so decrease its evolutionary potential (Johnson, 2000). Phylogeographic barriers across a seascape therefore maintain a certain level of structure that allows for the diversification of a species (e.g., ecotypes) (Neigel and Avise, 1986; Irwin, 2002). So what happens if humans move individual organisms across a dispersal barrier? Minimal genetic exchange via anthropogenic transport across a strong barrier can usually be detected using variable genetic markers such as microsatellites and single nucleotide polymorphisms (SNPs) followed by the calculation of F-statistics (as a measure of population genetic differentiation) and assessing the degree of haplotype sharing(Darling et al., 2008; Reusch et al., 2010). However, as individuals are moved across barriers bi-directionally and consistently enough, the genetic patterns may begin to mimic a natural pattern of dispersal. At this point a convergence is reached where “phylogeographic signal” is lost and a genetic pattern consistent with panmixia (gene-flow without barriers) is observed (Figure 1). Yet in actuality, a barrier does exist and populations which should be genetically distinct have now begun the process of homogenization not because of natural movement but because of anthropogenic transport. Examples of anthropogenic transport mechanisms include rafting—where organisms can adhere to, and be transported with marine debris (Carlton and Fowler, 2018), aquaculture—which facilitates the movement of “hitchhikers” on farmed shellfish (Williams et al., 2016) and shipping—which includes the transportation of alien fauna in both ballast water and on the hulls of ships (Seebens et al., 2013). Based on this theoretical framework, unless natural movement can be disentangled from anthropogenic movement, a conclusion that proposes panmixia based on standard population genetic parameters is arguably an unfounded assumption. One has to wonder whether past studies that proposed panmixia as a result of natural processes, were really simply the result of human-mediated introductions over a long period of time that went undetected.
Figure 1. Simplistic hypothetical scenario showing how consistent bi-directional anthropogenic movement of individuals across a dispersal barrier alters genetic patterns to mimic a panmictic seascape. Time step 1–natural dispersal without introductory events, Time step 2–natural dispersal coupled with unidirectional introductory events, Time step 3: natural dispersal coupled with bidirectional introductory events. Thin arrows represent natural movement of individuals, within each biogeographic region, in addition to leakage across a barrier, while thick black arrows represent anthropogenic transport. Fst (LEFT) is a standard population genetic parameter for measuring genetic differentiation between populations with 0 indicating low genetic structure (high levels of gene flow) and 1 indicating completely separate populations (low levels of gene flow). Hypothetical haplotype networks (RIGHT) shows the effects of introductory events on haplotype patterning from highly structured (time step 1) to panmixia (time step 3).
For the last 20 years, researchers have slowly begun to incorporate biophysical modeling into marine phylogeographic studies (Levin, 2006). Biophysical modeling involves the use of Ocean General Circulation Models (OGCM), which express the dynamical processes that regulate ocean circulation and consist of both horizontal and vertical profiles. Examples of popular OGCMs used today include MICOM (Miami Isopycnic Coordinate Ocean Model) (Bleck and Chassignet, 1994), ROMS (Regional Ocean Modeling System) (Shchepetkin and McWilliams, 2003), and HYCOM (HYbrid Coordinate Ocean Model System) (Chassignet et al., 2007) These can then be coupled to a particle tracking model (usually Lagrangian based) where virtual drifters are seeded and released at specific sites to simulate a dispersal event (Schiavina et al., 2014; David et al., 2016). Examples of such dispersal models include ARIANE (Blanke and Raynaud, 1997), the Connectivity Modeling System (CMS) which possess novel features such as the ability to code variability within individual particles (Paris et al., 2013), and LTRANS which can also incorporate larval behavior by altering particle speed and direction in response to the particle's “age” (North et al., 2008). There is a number of comprehensive reviews in the literature that explore the mechanics of these models in detail (see (Lett et al., 2010) and references therein). Model outputs often include density maps, predicted trajectories and particle capture percentages, all of which can then be cross-referenced with connectivity patterns inferred from the genetic data. If both approaches reveal discordant patterns, then it is likely that an unforeseen variable is at play (David and Loveday, 2018). For example, previous dispersal studies on the jellyfish Aurelia aurita found that its wide geographic distribution and high level of gene-flow around the globe was driven solely by anthropogenic transport. This was inferred from the mixed haplotype network and low FST values obtained from the genetic component of the project and the discordant constrained trajectory pattern which showed that the species' maximum dispersal distance (based on currents, circulation pattern, and the species' ecological characteristics) is too short to allow such widespread connectivity (Dawson et al., 2005). Similarly, a more recent study found that the polychaete, Polydora hoplura showed high levels of gene-flow across multiple dispersal barriers on the southern African coast. This was not surprising at first considering that the species is a generalist, exhibits a broad thermo-tolerance and can produce long lived planktotrophic larvae. However, a coastal model that was developed to simulate P. hoplura's dispersal found limited to no dispersal across a major barrier on the South African coast. Later studies eventually supported the model's conclusion by showing that P. hoplura can hitchhike with oysters that are regularly transported across shellfish farms in South Africa (David et al., 2016; Williams et al., 2016). In both of these studies, the hydrodynamic models acted as a control by filtering out anthropogenic influence on the genetic pattern and only incorporating ocean circulation patterns and some of the ecological traits of the study species. Biophysical models therefore add a layer of oceanographic realism to connectivity studies, which are usually absent from traditional phylogeographic approaches.
While there are now a growing number of publications that are incorporating biophysical modeling into marine phylogeographic research and it shows promise for dealing with the “pseudo-panmictic” issue, there are few published protocols on best practices when using this integrated approach [but see (North et al., 2009)]. For example, virtually all marine phylogeographic studies that incorporate hydrodynamic models simply use whatever existing models are available (grid resolution is often a secondary concern). In addition, many of these models do not accurately capture nearshore coastal processes that play an important role in dictating trajectory of individuals (David et al., 2016). Finally, while these models are often termed “biophysical” models, it is only in the last few years, that advances have been made to truly simulate biological entities within a fluid flow and many models are still particle based. Of course, none of these problems are insurmountable since most of them stem from the differences between the research goals of the marine biologist and the oceanographer. To remediate this problem, more meaningful collaborations between the two camps will be needed to develop more realistic larval transport simulations within OGCMs and a standard protocol needs to be developed which can be used across different study systems. Familiarizing marine biologists with numerical modeling early on in their career (e.g., in graduate school) may facilitate this because it can minimize the disciplinary “gap” that exist between workers in the two fields. Indeed, the motivation to develop pedagogical approaches to do this is not that far-fetched if one considers the popularity of Big Data analytics across the STEM landscape, along with the computational demands and programming skills needed to execute these massive ocean models.
The author confirms being the sole contributor of this work and approved it for publication.
The author declares that the research was conducted in the absence of any commercial or financial relationships that could be construed as a potential conflict of interest.
Fruitful discussions at the Marine Evolution 2018 Symposium in Stromstad, Sweden was instrumental in the preparation of this manuscript along with comments and suggestions from two reviewers.
Avise, J. C. (2008). Phylogeography: retrospect and prospect. J. Biogeogr. 36, 3–5. doi: 10.1111/j.1365-2699.2008.02032.x
Blanke, B., and Raynaud, S. (1997). Kinematics of the Pacific equatorial undercurrent: a Eulerian and Lagrangian approach from GCM results. J. Phys. Oceanogr. 27, 1038–1053.
Bleck, R., and Chassignet, E. P. (1994). “Simulating the oceanic circulation with isopycnic-coordinate models,” in The Oceans: Physical-Chemical Dynamics and Human Impact, eds S. K. Majumar, E. W. Miller, G. S. Forbes, R. F. Schmalz and A. A. Panah (Easton, PA: Pennsylvania Academy of Sciences), 17–39.
Carlton, J. T., and Fowler, A. E. (2018). Ocean rafting and marine debris: a broader vector menu requires a greater appetite for invasion biology research support. Aquat. Invasions 13, 11–15. doi: 10.3391/ai.2018.13.1.02
Carvalho, G. R., and Hauser, L. (1994). Molecular genetics and the stock concept in fisheries. Rev. Fish Biol. Fish 4, 326–350. doi: 10.1007/BF00042908
Chassignet, E. P., Hurlburt, H. E., Smedstad, O. M., Halliwell, G. R., Hogan, P. J., Wallcraft, A. J., et al. (2007). The HYCOM (Coordinate Ocean Model) data assimilative system. J. Mar. Syst. 65, 60–83. doi: 10.1016/j.jmarsys.2005.09.016
Darling, J. A., Bagley, M. J., Roman, J., Tepolt, C. K., and Geller, J. B. (2008). Genetic patterns across multiple introductions of the globally invasive crab genus Carcinus. Mol. Ecol. 17, 4992–5007. doi: 10.1111/j.1365-294X.2008.03978.x
David, A. A., and Loveday, B. R. (2018). The role of cryptic dispersal in shaping connectivity patterns of marine populations in a changing world. J. Mar. Biol. Assoc. UK. 98, 647–655. doi: 10.1017/S0025315417000236
David, A. A., Matthee, C. A., Loveday, B. R., and Simon, C. A. (2016). Predicting the dispersal potential of an invasive polychaete pest along a complex coastal biome. Integr. Comp. Biol. 56, 600–610. doi: 10.1093/icb/icw011
Dawson, M. N., Sen Gupta, A., and England, M. H. (2005). Coupled biophysical global ocean model and molecular genetic analyses identify multiple introductions of cryptogenic species. Proc. Natl. Acad. Sci. U.S.A. 102, 11968–11973. doi: 10.1073/pnas.0503811102
Irwin, D. E. (2002). Phylogeographic breaks without geographic barriers to gene flow. Evolution 56, 2383–2394. doi: 10.1111/j.0014-3820.2002.tb00164.x
Johnson, M. S. (2000). Measuring and interpreting genetic structure to minimize the genetic risks of translocations. Aquat. Res. 31, 133–143. doi: 10.1046/j.1365-2109.2000.00396.x
Lett, C., Ayata, S.-D., Huret, M., and Irisson, J.-O. (2010). Biophysical modelling to investigate the effects of climate change on marine population dispersal and connectivity. Prog. Oceanogr. 87, 106–113. doi: 10.1016/j.pocean.2010.09.005
Levin, L. A. (2006). Recent progress in understanding larval dispersal: new directions and digressions. Integr. Comp. Biol. 46, 282–297. doi: 10.1093/icb/icj024
Molnar, J. L., Gamboa, R. L., Revenga, C., and Spalding, M. D. (2008). Assessing the global threat of invasive species to marine biodiversity. Front. Ecol. Environ. 6, 485–492. doi: 10.1890/070064
Neigel, J. E., and Avise, J. C. (1986). Phylogenetic relationships of mitochondrial DNA under various demographic models of speciation. Evol. Proc. Theory. 1986, 515–534. doi: 10.1016/B978-0-12-398760-0.50026-2
North, E. W., Gallego, A., and Petitgas, P. (2009). Manual of Recommended Practices for Modelling Physical-Biological Interactions During Fish Early Life. ICES Cooperative Research Report. 295, 1–111. Available online at: http://archimer.ifremer.fr/doc/00157/26792
North, E. W., Schlag, Z., Hood, R. R., Li, M., Zhong, L., Gross, T., et al. (2008). Vertical swimming behavior influences the dispersal of simulated oyster larvae in a coupled particle-tracking and hydrodynamic model of Chesapeake Bay. Mar. Ecol. Prog. Ser. 359, 99–115. doi: 10.3354/meps07317
Palumbi, S. R. (1996). What can molecular genetics contribute to marine biogeography? An urchin's tale. J. Exp. Mar. Biol. Ecol. 203, 75–92.
Paris, C. B., Helgers, J., van Sebille, E., and Srinivasan, A. (2013). Connectivity modeling system: a probabilistic modeling tool for the multi-scale tracking of biotic and abiotic variability in the ocean. Environ. Model. Softw. 42, 47–54. doi: 10.1016/j.envsoft.2012.12.006
Reusch, T. B., Bolte, S., Bolte, S., Sparwel, M., Moss, A. G., and Javidpour, J. (2010). Microsatellites reveal origin and genetic diversity of Eurasian invasions by one of the world's most notorious marine invader, Mnemiopsis leidyi (Ctenophora). Mol. Ecol. 19, 2690–2699. doi: 10.1111/j.1365-294X.2010.04701.x
Rius, M., Turon, X., Bernardi, G., Volckaert, F. A. M., and Viard, F. (2015). Marine invasion genetics: from spatio-temporal patterns to evolutionary outcomes. Biol. Invasions 17, 869–885. doi: 10.1007/s10530-014-0792-0
Schiavina, M., Marino, I. A., Zane, L., and Melia, P. (2014). Matching oceanography and genetics at the basin scale. Seascape connectivity of the Mediterranean shore crab in the Adriatic Sea. Mol. Ecol. 23, 5496–5507. doi: 10.1111/mec.12956
Seebens, H., Gastner, M. T., and Blasius, B. (2013). The risk of marine bioinvasion caused by global shipping. Ecol. Lett. 16, 782–790. doi: 10.1111/ele.12111
Selkoe, K. A., Henzler, C. M., and Gaines, S. D. (2008). Seascape genetics and the spatial ecology of marine populations. Fish Fish. 9, 363–377. doi: 10.1111/j.1467-2979.2008.00300.x
Selkoe, K. A., Watson, J. R., White, C., Horin, T. B., Iacchei, M., Mitarai, S., et al. (2010). Taking the chaos out of genetic patchiness: seascape genetics reveals ecological and oceanographic drivers of genetic patterns in three temperate reef species. Mol. Ecol. 19, 3708–3726. doi: 10.1111/j.1365-294X.2010.04658.x
Shchepetkin, A. F., and McWilliams, J. C. (2003). A method for computing horizontal pressure-gradient force in an oceanic model with non-alignmed vertical coordinate. J. Geophys. Res. 108, 1–34. doi: 10.1029/2001JC001047
Von der Heyden, S., Beger, M., Toonen, R. J., van Herwerden, L., Juinio-Menez, M. A., Ravagp-Gotanco, R., et al. (2014). The application of genetics to marine management and conservation: examples from the Indo-Pacific. Bull. Mar. Sci. 90, 123–158. doi: 10.5343/bms.2012.1079
Keywords: panmixia, biophysical modeling, marine populations, cryptic dispersal, connectivity
Citation: David AA (2018) Reconsidering Panmixia: The Erosion of Phylogeographic Barriers Due to Anthropogenic Transport and the Incorporation of Biophysical Models as a Solution. Front. Mar. Sci. 5:280. doi: 10.3389/fmars.2018.00280
Received: 22 May 2018; Accepted: 23 July 2018;
Published: 07 August 2018.
Edited by:
Juan Armando Sanchez, University of Los Andes, ColombiaReviewed by:
Mauricio Romero Torres, Corporation Center of Excellence in Marine Sciences (CEMarin), ColombiaCopyright © 2018 David. This is an open-access article distributed under the terms of the Creative Commons Attribution License (CC BY). The use, distribution or reproduction in other forums is permitted, provided the original author(s) and the copyright owner(s) are credited and that the original publication in this journal is cited, in accordance with accepted academic practice. No use, distribution or reproduction is permitted which does not comply with these terms.
*Correspondence: Andrew A. David, YWRhdmlkQGNsYXJrc29uLmVkdQ==
Disclaimer: All claims expressed in this article are solely those of the authors and do not necessarily represent those of their affiliated organizations, or those of the publisher, the editors and the reviewers. Any product that may be evaluated in this article or claim that may be made by its manufacturer is not guaranteed or endorsed by the publisher.
Research integrity at Frontiers
Learn more about the work of our research integrity team to safeguard the quality of each article we publish.