- 1Key Laboratory of Tropical Disease Control (Sun Yat-sen University), Ministry of Education, Guangzhou, China
- 2Key Laboratory of Tropical Translational Medicine of Ministry of Education, Hainan Medical University, Haikou, China
- 3Joint Program of Pathobiology, Fifth Affiliated Hospital, Zhongshan School of Medicine, Sun Yat-sen University, Guangzhou, China
- 4Instrumental Analysis and Research Center, Sun Yat-sen University, Guangzhou, China
- 5Department of Gastroenterology, The Fifth Affiliated Hospital of Sun Yat-sen University, Zhuhai, China
- 6Provincial Engineering Technology Research Center for Biological Vector Control, Zhongshan School of Medicine, Sun Yat-sen University, Guangzhou, China
Schistosoma japonicum (S. japonicum) is one of the etiological agents of schistosomiasis, a widespread zoonotic parasitic disease. However, the mechanism of the balanced co-existence between the host immune system and S. japonicum as well as their complex interaction remains unclear. In this study, 16S rRNA gene sequencing, combined with metagenomic sequencing approach as well as ultraperformance liquid chromatography–mass spectrometry metabolic profiling, was applied to demonstrate changes in the gut microbiome community structure during schistosomiasis progression, the functional interactions between the gut bacteria and S. japonicum infection in BALB/c mice, and the dynamic metabolite changes of the host. The results showed that both gut microbiome and the metabolites were significantly altered at different time points after the infection. Decrease in richness and diversity as well as differed composition of the gut microbiota was observed in the infected status when compared with the uninfected status. At the phylum level, the gut microbial communities in all samples were dominated by Firmicutes, Bacteroidetes, Proteobacteria, and Deferribacteres, while at the genus level, Lactobacillus, Lachnospiraceae NK4A136 group, Bacteroides, Staphylococcus, and Alloprevotella were the most abundant. After exposure, Roseburia, and Ruminococcaceae UCG-014 decreased, while Staphylococcus, Alistipes, and Parabacteroides increased, which could raise the risk of infections. Furthermore, LEfSe demonstrated several bacterial taxa that could discriminate between each time point of S. japonicum infection. Besides that, metagenomic analysis illuminated that the AMP-activated protein kinase (AMPK) signaling pathway and the chemokine signaling pathway were significantly perturbed after the infection. Phosphatidylcholine and colfosceril palmitate in serum as well as xanthurenic acid, naphthalenesulfonic acid, and pimelylcarnitine in urine might be metabolic biomarkers due to their promising diagnostic potential at the early stage of the infection. Alterations of glycerophospholipid and purine metabolism were also discovered in the infection. The present study might provide further understanding of the mechanisms during schistosome infection in aspects of gut microbiome and metabolites, and facilitate the discovery of new targets for early diagnosis and prognostic purposes. Further validations of potential biomarkers in human populations are necessary, and the exploration of interactions among S. japonicum, gut microbiome, and metabolites is to be deepened in the future.
Introduction
Schistosomiasis is a zoonotic parasitic disease mainly caused by the infection of Schistosoma japonicum (S. japonicum), Schistosoma mansoni (S. mansoni), and Schistosoma haematobium (S. haematobium), which seriously damages human and animal health and hinders socio-economic development. This neglected tropical disease affects ~200 million people, and ~779 million are at risk of infection worldwide (1). S. japonicum is distributed principally in East Asia, especially in China, the Philippines, and Indonesia, with more than 1 million people infected and ~46 million people at risk (2). In China, S. japonicum is endemic in mainly 12 provinces along the middle and the lower reaches of the Yangtze River and regions south of it. The life cycle of S. japonicum is complex and consists of asexual generation in the intermediate host and sexual generation in the definitive host, including the seven developmental stages of egg, miracidium, mother sporocyst, daughter sporocyst, cercaria, juvenile schistosomulum, and adult worm (3). Different stages of S. japonicum cause various damages to the host, and complex immune pathological reactions lead to diverse clinical symptoms. Larval worms induce Th1 responses with elevated levels of the inflammatory cytokines IFN-γ, IL-12, and TNF-α in the early phase of schistosomiasis and cause diarrhea, fatigue, and anemia, while adult worms become mature and lay eggs; parasite eggs that deposit in the liver and colon of infected hosts elicit Th2 responses and then upregulate the serum cytokine levels of IL-4, IL-5, IL-13, and TGF-β, leading to portal vein hypertension syndrome, ascites, and liver fibrosis (2, 4, 5). During S. japonicum infection, egg deposits in the tissues are a determining factor that shifts the Th1 response to the Th2 response (5). To protect against eggs, delayed-type hypersensitivity reactions of the host are triggered but lead to the formation of circumoval granuloma in livers and colons, followed by fibrosis, which is the main cause of death (5). Immunological downregulation occurs to both, protecting the host from inflammatory damage and preventing the parasites from being eliminated during late chronic infection (4). However, the mechanism of this balanced co-existence between the host's immune system and S. japonicum as well as their complex interaction remains unclear.
At present, schistosomiasis is diagnosed by a clinical history of contact with fresh water from endemic foci, followed by both direct methods and indirect tests in the clinical laboratory. The former includes using the Kato–Katz technique to examine the feces under light microscopy for detection of eggs, while the latter includes using immunological approaches, such as detecting soluble antigens secreted from eggs via the antigen–antibody reaction (2, 6, 7). Imaging methods, for instance, ultrasonography (US), CT scan and MR, scan, are established to inspect the presence of periportal fibrosis (7). Nevertheless, these diagnostic methods are not sensitive or specific enough and are not suitable for early diagnosis; therefore, a well-suited approach with high sensitivity and specificity is urgently required to detect acute stage infection.
Metabolomics is a quantitative measurement of multi-parametric metabolic responses of multi-cellular systems and aims to identify and quantify numerous small molecules (<1,200 Da) present in various biological samples or specific physiological states; metabolomics can provide a comprehensive systems-level study of the relationships between host genetic and environmental factors with high-density data and multivariate mathematical modeling (8–10). Nuclear magnetic resonance (NMR) spectroscopy, liquid chromatography–mass spectrometry (LC–MS), and gas chromatography–mass spectrometry are the most widely used analytical techniques for metabolomic analyses. Due to the main advantages of much better sensitivity and resolution, more coverage of metabolites, and high-throughput capacity (11), MS-based techniques have been implemented more frequently than NMR. Metabolomics has already been widely applied in parasitological studies, with comprehensive characterizations of the host metabolic responses to infections by several parasites, such as Plasmodium falciparum (12–14), Trypanosoma brucei (15–17), Toxoplasma gondii (18–20), and S. japonicum (2, 6, 21). Hence, metabolomics is a suitable diagnostic tool to provide novel insights into the mechanisms underlying the progression of schistosomiasis, clearly revealing the resistance mechanism between the host and S. japonicum and thus leading to the discovery of potential metabolic biomarkers that are useful for early diagnosis.
The gut microbiota, consisting of diverse microbial communities, has a profound impact on influencing host physiology by the composition, density, and activities of colonizing microorganisms as well as on animal evolution through the interplay between host and microbial communities (22). Once the balance in proportions among core bacterial communities breaks down, dysbiosis occurs, which may alter host interactions and lead to numerous diseases, such as inflammatory gut disorders, diabetes, and obesity (23). S. japonicum adult worms live in the mesenteric veins of the host and can cause intestinal schistosomiasis with symptoms of mucosal granulomatous inflammation, superficial bleeding, and pseudopolyposis (24). However, the interaction between the host, S. japonicum, and the microbiota and the potential effects of helminths on shaping the host–microbiota composition and structure are unclear; therefore, further investigation is warranted.
Previous studies have shown that infection with S. japonicum modified both bacterial richness and bacterial community composition (25), reduced the levels of tricarboxylic acid cycle intermediates, increased the levels of amino acids, choline, and urinary 3-ureidopropionate, and perturbed lipid metabolism, glycolysis stimulation, tricarboxylic acid cycle, and a series of microbial-related metabolites (2, 6). Nevertheless, the alterations of microbiome and metabolome in the time course of infection progression have not been described yet; therefore, the aim of the current study is to investigate the dynamic alteration of gut microbiome community structure and the metabolite profile of the hosts infected with S. japonicum, and the correlations between host metabolism and gut microbiome after the infection by omics-based and systems-level approaches involving metabolic profiling with ultraperformance liquid chromatography–mass spectrometry (UPLC–MS), 16S rRNA gene sequencing, and shotgun metagenomics sequencing. To our knowledge, employing metagenomic sequencing and untargeted metabolic profiling to investigate the effects of infection with S. japonicum in BALB/c mice would allow a better understanding of the mechanisms during schistosomiasis development and potentially reveal new targets for early diagnosis and prognosis.
Materials and Methods
Ethics Statement
All animal experiments were conducted strictly in accordance with the guidelines of the National Institutes of Health on animal care and the ethical guidelines. The protocol was approved by the Animal Care and Use Committee of Sun Yat-sen University [permit no. SYXK (Guangdong) 2017-0081]. All efforts were made to minimize the suffering of the animals.
Mice and Cercariae
Seventy specific-pathogen-free 6- to 8-week-old female BALB/c mice (18 ± 2 g body weight) were purchased from the Animal Experiment Center at Sun Yat-sen University (Guangzhou, China) and were housed in plastic cages with free access to autoclaved chow and water under controlled temperature and humidity and a 12-h light/12-h dark cycle. The animals were randomly divided into seven groups, with 10 mice in each group. After the mice acclimated to the new environment, 60 of them were infected with 30 ± 2 S. japonicum cercariae per individual via the shaved abdominal skin. The cercariae were obtained from infected Oncomelania, which were provided by the National Institute of Parasitic Diseases, Chinese Center for Disease Control and Prevention (Shanghai, China), that were placed in dechlorinated water and exposed to artificial light for more than 2 h. The other mice were left uninfected and served as controls.
Sample Collection
The mice in each group were sacrificed by chloral hydrate asphyxiation and cervical dislocation either before infection or at 3, 7, 14, 21, 28, and 42 days post-infection (dpi). Blood samples were drawn from orbital veins and centrifuged at 3,000 ×g for 10 min to collect the serum after clotting. The serum was stored at−80°C until further analysis. Urine and feces samples were collected the day before the mice were sacrificed by placing them individually in metabolic cages, which can separate feces from urine using different small plastic tubes embedded at the bottom of the cages. Dry ice was placed around the collection tubes to prevent oxidation or degradation of metabolites. At least 0.5 ml of urine and 1 g of feces were obtained; then, the samples were transferred into Eppendorf tubes and stored in a freezer at−80°C for further testing.
Genomic DNA Extraction and 16S rRNA Gene Sequencing
Nucleic acid extraction of thirty fecal samples of the 0, 7, 14, 21, 28, and 42 dpi groups (five samples per group) was carried out using a QIAamp Fast DNA Stool Mini kit (cat. no. 51604, QIAGEN, Hilden, Germany), following the manufacturer's instructions. The concentration of DNA was measured on a NanoDrop (Thermo Scientific, Waltham, MA, USA), and 1% agarose gel electrophoresis was used to assess the integrity and the purity of DNA. After that, extracted DNA was diluted to a concentration of 1 ng/μl and used as a template to amplify the V3–V4 regions of the 16S rRNA gene, utilizing the primers 343F (5′-TACGGRAGGCAGCAG-3′) and 798R (5′-AGGGTATCTAATCCT-3′) with barcodes in combination with HiFi Hot Start Ready Mix (cat. no. KK2501, Kapa Biosystems, Boston, MA, USA). Polymerase chain reaction (PCR) amplicon product quality was demonstrated through agarose gel electrophoresis, and PCR amplicons were purified with Agencourt AMPure XP beads (cat. no. A63881, Beckman Coulter, Brea, CA, USA), followed by another round of PCR amplification. The final amplicons were quantified with a Qubit® dsDNA HS Assay Kit (cat. no. Q32854, Life Technologies, Waltham, MA, USA) after a subsequent clean-up, as described above. Afterwards, the purified amplicons from each sample were pooled in equal amounts for subsequent 16S rRNA sequencing on the Illumina MiSeq platform.
Metagenome Sequencing
Two microliters of DNA (10 ng/μl) from nine fecal samples of the 0, 21, and 42 dpi groups (three samples per group) was fragmented to ~300–500 bp with a Covaris S220 (Covaris, Woburn, MA, USA) individually. Subsequently, library construction was performed using a TruSeq Nano DNA LT Sample Preparation Kit (cat. no. FC-121-4001, Illumina, San Diego, CA, USA) according to the manufacturer's instructions, and then a TruSeq PE Cluster Kit v3-cBot-HS (cat. no. PE-401-3001, Illumina, San Diego, CA, USA) was used for bridge PCR. The resulting DNA was then pooled and quantified by Kapa Library Quantification Kits (cat. no. KK4824, Kapa Biosystems, Boston, MA, USA) and sequenced using the Illumina HiSeq platform with a TruSeq SBS Kit v3-HS (cat. no. FC-401-3001, Illumina, San Diego, CA, USA).
16S rRNA Gene Analysis
Paired-end reads were reprocessed using Trimmomatic software (26) to detect and cut off ambiguous bases from the N terminal. Low-quality sequences with an average quality score lower than 20 were removed by the sliding window trimming approach. After trimming, the paired-end reads were assembled using Fast Ligation-based Automatable Solid-phase High-throughput software (version 1.2.11) (27). Only sequences with 10 base pairs (bp) of minimal overlapping, 200 bp of maximum overlapping, and 20% of maximum mismatch rate were assembled according to their overlap sequence. Reads with ambiguous, homologous sequences and a total length of <200 bp were abandoned, while reads with 75% of bases above Q20 were retained. Next, the sequences were checked by Quantitative Insights Into Microbial Ecology (QIIME) software (version 1.8.0) (28) for the following criteria: the maximum length of a homopolymer run was six, the maximum number of mismatches in the primer was two, the maximum number of errors in the barcode was zero, and reads with chimeras were detected and removed. After that, clean reads were obtained, followed by subjecting to primer sequence removal and clustering to generate operational taxonomic units (OTUs) using UPARSE software (version 6.1.351) with 97% sequence similarity cutoff (equal to bacterial species level) (29). All representative reads were chosen from each OTU by selecting the most abundant sequence with the QIIME package. High-quality representative sequences were annotated and blasted against the Silva database (version 123) on the basis of the Ribosomal Database Project classifier (the confidence threshold was set as 70%) (30).
Alpha diversity (within-sample diversity) was estimated for each group using the number of observed species, Chao1 richness estimator, Shannon–Wiener index, and phylogenetic diversity index, while beta diversity (between-sample diversity) was monitored with two-dimensional and three-dimensional principal coordinate analysis (PCoA) plots on the basis of weighted UniFrac distance metrics. Bar plots were generated to visualize the relative abundances and alterations over time in bacterial communities for fecal samples from each group. To distinguish significant differences in microbial communities at different taxonomic levels between healthy mice and S. japonicum-infected mice, one-way analysis of variance (ANOVA) was performed; statistical significance level was set at p < 0.05. Linear discriminant analysis (LDA) coupled with effect size (LEfSe) measurement1 was implemented to illuminate microbial taxa that were differentially represented between the groups, in order to discover the potential markers at different time points. Pearson correlation coefficients between the top 30 dominant gut bacteria at the genus level were calculated, and bacterial genera with high correlations and p < 0.05 were used to build an associated network with Cytoscape software (version 3.6.1) to find correlations between gut microbiota changes and explore the ecological significance related to each other. Unless otherwise stated, statistical analyses and plots were carried out using R software (version 3.5.1).
Metagenomic Analysis
Next-generation sequencing quality control (QC) Toolkit (version 2.3.2) (31) was used to discard raw metagenomic reads with 70% of bases below Q20 and remove reads from the 3′ end until reaching the first nucleotide with a minimum quality score cutoff of 20 and if either read was shorter than 70 bp or contained “N” bases or ambiguous bases. Afterwards, sequences of Mus musculus2 were filtered out by Burrows–Wheeler Alignment (version 0.7.9a) (32). After the scaffold sequences from all samples were assembled with Short Oligonucleotide Analysis Package Denovo3 (version 4.5.4) (33), open reading frames (ORFs) were predicted and translated into amino acid sequences by prodigal4 (version 2.6.3) (34). Cluster Database at High Identity with Tolerance5 (version 4.5.4) was implemented to build non-redundant gene sets for all predicted genes and to cluster ORFs with more than 95% identity and more than 90% coverage. The gene with the longest full length from each cluster was selected as the representative read of each gene set. For further analysis, annotations were performed with the gene set representative reads by using Blastp6 (Blast version 2.2.28+) alignment (E-value < 0.00001) between ORFs and the protein databases of the Kyoto Encyclopedia of Genes and Genomes7.
Metabolic Profiling
Sample Processing for Metabolomics
Each 10-μl serum sample was thawed on ice and diluted 1:10 in methanol. After that, the mixture was vortexed briefly and incubated overnight at 4°C to precipitate proteins thoroughly, followed by centrifugation at 10,000 ×g for 10 min, and ~80-μl aliquots of the supernatant were then transferred to a vial for analysis. The urine samples were prepared by diluting 50 μl of urine with 450 μl of Milli-Q water and centrifuging for 10 min at 10,000 ×g at 4°C to remove particulates. A 200-μl volume of supernatant was collected and transferred into a glass vial afterwards. Liver and colon samples were processed following a modified version of the method of Elizabeth et al. (35). Briefly, frozen tissue (50 ± 0.5 mg) was added to 1.5 ml of prechilled methanol/water (1:1, v:v) solvent, which was subsequently completely homogenized in an ice bath. The suspension was centrifuged at 16,000 ×g for 10 min, and the resulting supernatant was transferred into an Eppendorf tube and then processed through vacuum freeze-drying to obtain an aqueous extract. The dried residue was redissolved in 120 μl of methanol/water (1:1, v:v) and centrifuged at 13,000 ×g for 10 min to remove particulates. Thereafter, the clear supernatant was transferred to a sampling vial. For each type of specimen, QC samples were prepared by mixing an equal volume of all individuals into the vial to ensure the repeatability and the stability of the analysis.
Ultraperformance Liquid Chromatography Conditions
An ACQUITY UPLC-I Class System (Waters Ltd., Milford, MA, USA) was used to perform chromatographic separations. Each sample was injected onto an ACQUITY UPLC C18 BEH column (2.1 × 100 mm, 1.7 μm; Waters, Milford, MA, USA) in a random order at 38°C, while QC samples were detected every 10 specimens throughout the injection.
For the serum samples, mobile phase A was water mixed with 0.1% formic acid, while mobile phase B was 70% isopropanol and 30% acetonitrile containing 0.1% formic acid. The serum samples were eluted under gradient conditions at a flow rate of 400 μl/min with 1% B, which was held for 1 min and then was ramped up from 1 to 40% B for 2 min, from 40 to 75% B for 5 min, from 75 to 85% B for 4 min, and from 85 to 99% B for 6 min, held at 99% B over 4 min, and then returned to 1% B for 3 min. The volume of the sample injected onto the column was 1.000 μl.
For the urine samples, the gradient solvent system included water (A) and acetonitrile (B), each containing 0.1% formic acid. The injection volume of the urine samples was set to 0.300 μl; the separation gradient was held at 3% B for 1.2 min, ramped up from 3 to 45% B over 8.8 min and from 45 to 98% B for 4 min, held at 98% B over 2 min, and then returned to 3% B for 3 min, with a flow rate of 400 μl/min.
For the liver supernatant, the injection volume was 0.400 μl; the mobile phase was held at 2% B for 2 min, increased from 2 to 45% B over the next 8 min, followed by 45 to 98% B over 3 min, held at 98% B for 2 min, and decreased to 2% B, which was held for 2 min, at a flow rate of 400 μl/min. However, the injection volume of the colon supernatant was set to 1.500 μl; the separation gradient was held at 25% B for 0.5 min, ramped from 25 to 50% B over 4.5 min, from 50 to 65% B for 7 min, and from 65 to 95% B for 4 min, held 95% B for 2 min, and then returned to 25% B over 3 min, with a flow rate of 400 μl/min. The gradient solvent systems used for the liver and the colon supernatants were the same as that used for the urine samples.
Quadrupole-Time-of-Flight Mass Spectrometry Conditions
Mass spectrometry data were collected by a SYNAPT G2-Si High-Definition Mass Spectrometer with an electrospray ionization (ESI) source (Waters Ltd., Milford, MA, USA) in both positive and negative ion modes for the serum and the urine samples, whereas only negative ion mode was used for the liver and colon aqueous extracts due to the limited valuable compounds detected in the positive ion mode. Nitrogen gas was set as desolvation and cone gas. The capillary voltage was set at 2.5 kV, nebulizer gas at 6 bar, cone voltage at 35 kV, cone gas flow at 30 L/h, source temperature at 110°C, desolvation gas temperature at 350°C, and desolvation gas flow at 700 L/h. The eluted compounds were scanned from mass/charge (m/z) 50 to m/z 1,200 at a rate of 0.3 s per scan for both MS mode and MSE mode. The collision energy was set from 20 to 50 eV for MSE mode. To ensure mass accuracy and reproducibility, leucine enkephalin was used to correct data (m/z 556.2720 in positive mode and m/z 554.2615 in negative mode) at a concentration of 1 ng/μl and a flow rate of 5 μl/min continuously.
Data Analysis
The raw data were acquired by Masslynx (version 4.1, Waters, Manchester, UK) and imported into Progenesis QI (version 2.1, Nonlinear Dynamics, Waters, Manchester, UK) for data preprocessing, including peak alignment, picking, and normalization as well as compound identification. Normalized and scaled datasets were imported into SIMCA-P (version 13.0, Umetrics, Umea, Sweden) and MetaboAnalyst 4.08 (36, 37) to carry out statistical analyses. The statistical significance between experimental groups was determined by one-way ANOVA, with p < 0.05. Principal component analysis (PCA) and partial least squares-discriminatory analysis (PLS-DA) were used to visualize natural separation and trends among the groups by score plots, while orthogonal partial least squares-discriminatory analysis (OPLS-DA) was conducted to find the maximum separation between healthy mice and S. japonicum-infected mice. Advanced statistical and visualization tools, such as variable importance in projection (VIP) and S-plots, were performed to reveal underlying trends in data. The discriminated metabolites were selected based on significant changes, including VIP scores that were taken from comparisons in OPLS-DA models >2, p < 0.05, and QC samples' coefficient of variation (CV) <30 and were putatively identified by searching databases such as the Human Metabolome Database9 (38) and METLIN10 (39) with accurate mass spectral data and MS/MS spectra. Furthermore, receiver operating characteristic (ROC) curve analysis was carried out to evaluate the early diagnostic capability of identified potential biomarkers. Metabolite set enrichment analysis (MSEA) and pathway analysis were performed by MetaboAnalyst 4.0 to investigate the most significant metabolic pathways involved in S. japonicum infection. Finally, correlations between the dominant gut bacteria changes and shifted metabolites were calculated in R software (version 3.5.1) by a hierarchical clustering algorithm to determine the relationships between them.
Results
Gut Microbiome Community Structure Changes in Mice Infected by S. japonicum
High-throughput sequencing of the 16S rRNA gene was implemented to illustrate the alterations in the gut bacterial compositions of BALB/c mice associated with S. japonicum infection. A total of 1,210,516 valid reads were retained from 30 fecal samples, with an average of 40,350 sequences per sample, for further processing after filtering, which generated 19,868 OTUs at 97% similarity level (Supplementary Table 1) and 56 OTUs shared among all samples (Supplementary Figure 1). Most of the shared OTUs are members of the families Lachnospiraceae, Ruminococcaceae, and Bacteroidales S24-7 group. The four different alpha diversity estimators mentioned above increased over time among all groups but to a lesser extent in the infected mice than in the uninfected mice, whereas the least diversity presented at 21 dpi, indicating that the infection of S. japonicum reduced the alpha diversity of host gut bacteria, especially at 21 dpi (Supplementary Figure 2). However, the differences were not statistically significant (p > 0.05). A three-dimensional PCoA plot based on weighted UniFrac distances (Supplementary Figure 3) showed the variations of the gut bacterial communities of BALB/c mice, with 49.84, 18.29, and 7.12% variation explained by principal component (PC) 1, PC2, and PC3, respectively. The difference was observed between the 0-dpi group and the 42-dpi group, which demonstrated that the effect of S. japonicum infection in the late stage on the gut microbiome composition of the host was relatively stronger.
Perturbed Gut Bacteria in Mice With S. japonicum Infection
To observe S. japonicum infection effects on the gut microbiome of BALB/c mice, bar plots were generated according to the relative abundance of the 15 most abundant gut bacterial phyla (Figure 1A) and genera in different groups (Figure 1B). Subsequently, one-way ANOVA was applied to identify significant alterations in the composition of the host gut microbiota at the phylum and the genus levels during infection. It is obvious that Firmicutes and Bacteroidetes were the most abundant gut bacterial phyla, with total average relative abundances over 90% in all groups, and the abundances of Proteobacteria and Deferribacteres were altered according to the time of infection but with no statistical significance (p > 0.05, Supplementary Table 2). At the genus level, Lactobacillus, Lachnospiraceae NK4A136 group, Bacteroides, Staphylococcus, and Alloprevotella were the most prevalent gut microbiome in BALB/c mice, but only alterations of the relative abundances of Staphylococcus, Parabacteroides, Alistipes, Roseburia, and Ruminococcaceae UCG-014 showed statistical significance in the top 15 most important gut genera (p < 0.05, Supplementary Table 3). Notably, Staphylococcus, with a relative abundance of 14.46% in the 21-dpi group, was almost undetectable in other groups (Figure 2A). Furthermore, the average relative abundance of Parabacteroides was decreased before 7 dpi, but it was increased after that (Figure 2B); Alistipes was significantly more abundant in the infected groups than in the uninfected group (Figure 2C). In contrast, Roseburia and Ruminococcaceae UCG-014 (Figures 2D,E) were reduced after infection with S. japonicum, while the average relative abundance of the latter increased approximately twofold at 7 dpi.
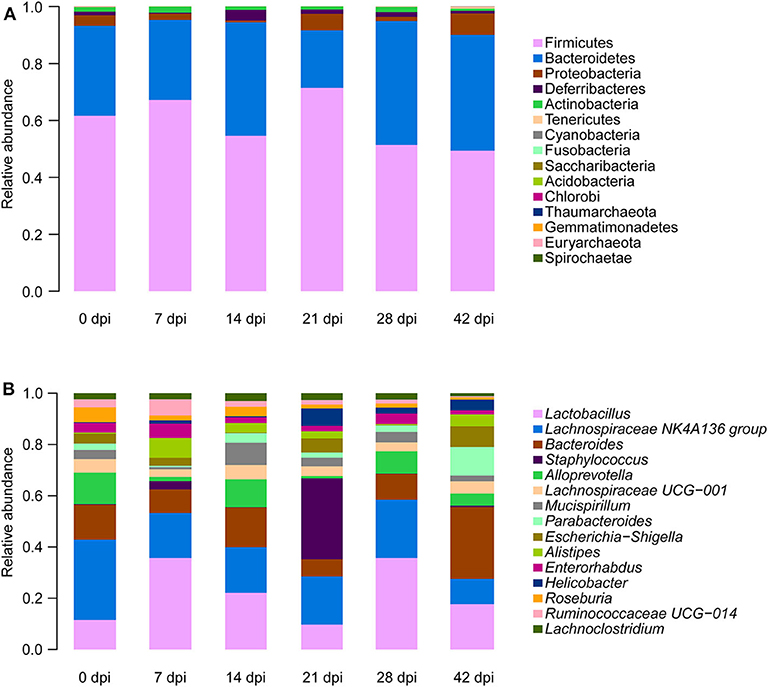
Figure 1. Relative abundances of the top 15 most important gut microbiota constituents at the phylum level (A) and genus level (B) across different time points as assessed by 16S rRNA sequencing. Each column represents the composition of the microbial taxa in one group.
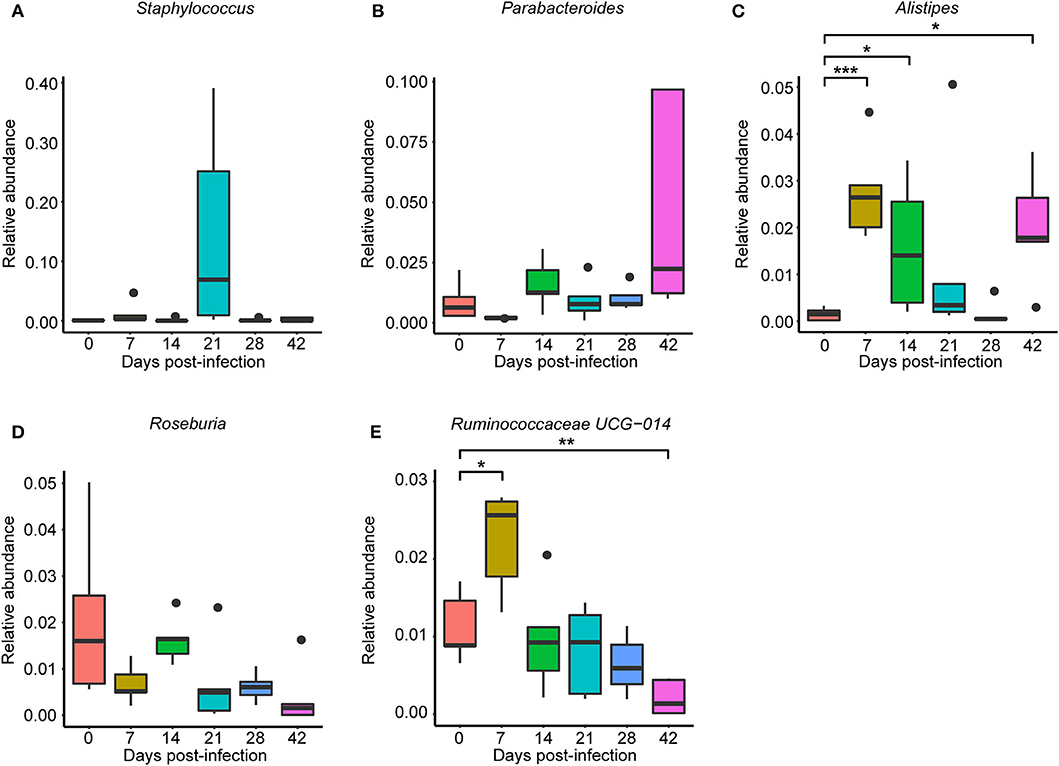
Figure 2. Relative abundances of significantly altered top 15 most important gut genera associated with Schistosoma japonicum infection. Each column represents one group. (A) Staphylococcus. (B) Parabacteroides. (C) Alistipes. (D) Roseburia. (E) Ruminococcaceae UCG-014. The top and the bottom whiskers indicate the maximum and the minimum values, respectively, and the hyphen represents the median value (*p < 0.05, **p < 0.01, ***p < 0.001).
To determine the taxa ranging from the phylum to the genus level that discriminated between each time point of S. japonicum infection, LEfSe, with an adjusted p < 0.05 and LDA score threshold >6.0, was performed. Bacteria from the phyla Firmicutes and Saccharibacteria, in addition to Alistipes and other taxa, were significantly associated with the 7-dpi group, while only Parabacteroides and Lachnospiraceae UCG-005 were significantly related to the 14-dpi group. Some members of the phyla Firmicutes and Proteobacteria were significantly enriched in the 21-dpi group, but the most significant was Staphylococcus. The Gammaproteobacteria class significantly distinguished the 28-dpi group from the other groups. Over-abundances of other members from the phyla Firmicutes, Bacteroidetes, and Proteobacteria were significantly linked to the 42-dpi group. However, no discriminative gut flora was found in the 0-dpi group. It is worth noting that Lachnospiraceae UCG-005 was related to the 14-dpi group, whereas Lachnospiraceae UCG-010 was associated with the 42-dpi group (Figure 3). The cladogram in Figure 4 showed the most relevant clades among each group, which was in accordance with the above mentioned results.
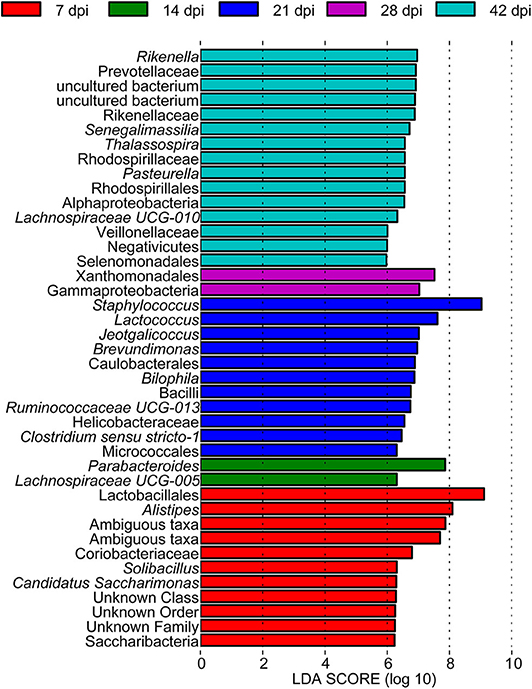
Figure 3. A histogram with linear discriminant analysis scores showing differentially abundant gut bacteria during the course of Schistosoma japonicum infection. Taxa highlighted in different colors indicate over-representation in the corresponding groups.
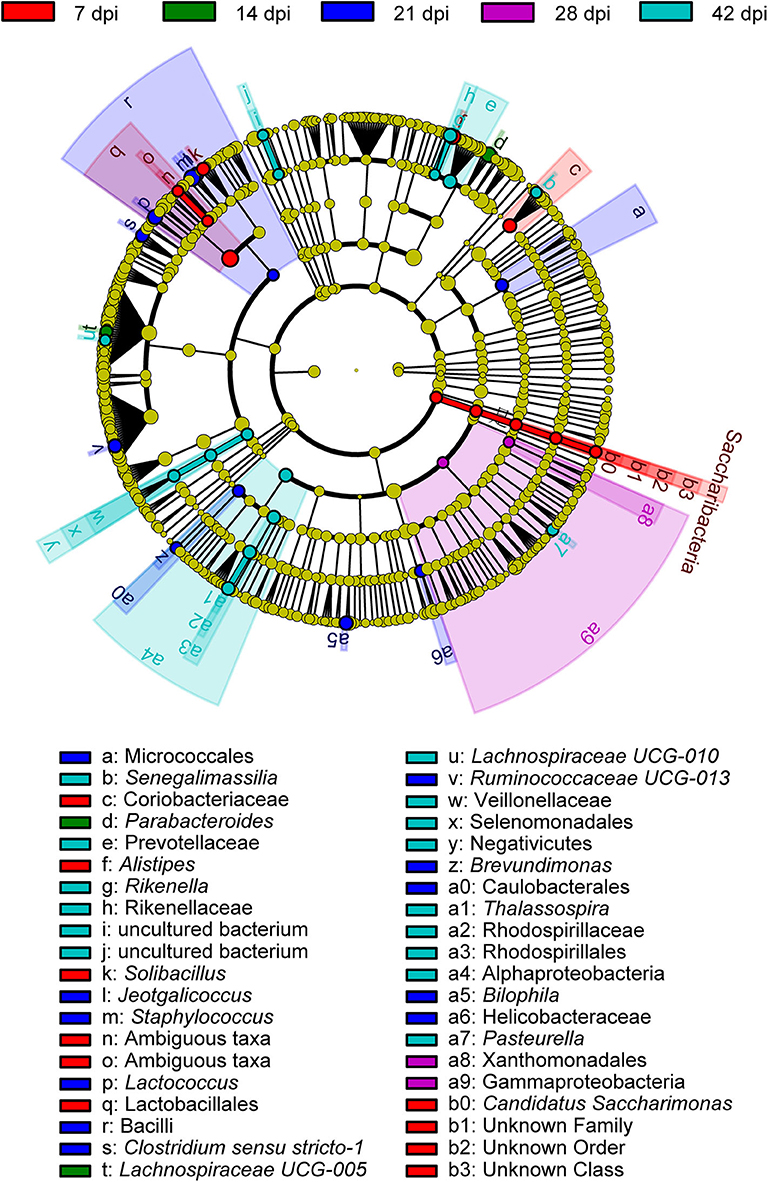
Figure 4. A cladogram showing the discriminated taxa in different groups. Regions with different colors represent different groups. Differently colored nodes in the branches represent the microbial groups that play an important role in the corresponding groups, whereas yellow nodes indicate bacterial groups that are insignificant in all groups.
Correlations between the top 30 dominant bacterial genera are demonstrated in Figure 5, with either a positive or a negative Pearson correlation coefficient (p < 0.05). The gut bacteria were divided into four clusters, and the most connected bacteria were Anaerotruncus, Coprococcus 1, Parabacteroides, Bacteroides, Erysipelatoclostridium, and Odoribacter, while Odoribacter had the maximum connections with other genera. It is interesting that significant negative correlations were only found in Odoribacter and Lachnoclostridium, as well as Enterorhabdus and Lachnospiraceae UCG-001, whereas the remaining bacteria were significantly positively related to each other.
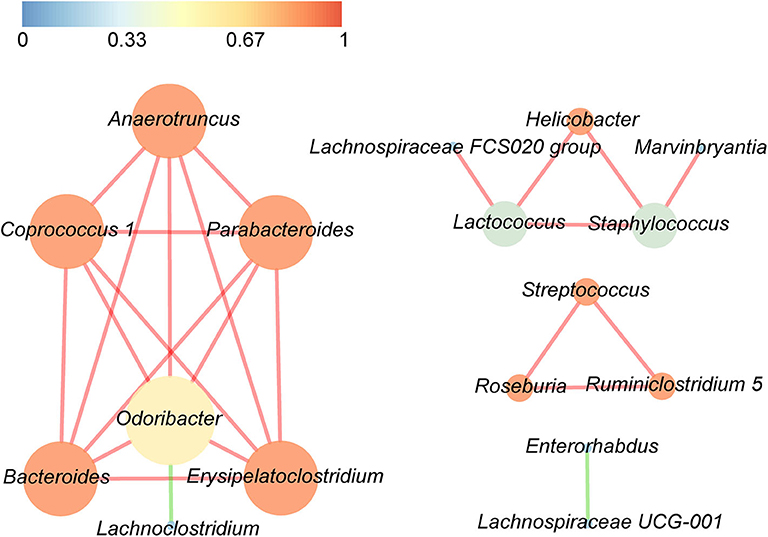
Figure 5. Significant relationships among the top 30 abundant bacterial genera were detected by Pearson correlation coefficient (p < 0.05). The nodes in the interaction network represent the dominant genus, the connection between them shows an association between the two genera, and the red line indicates a positive correlation, whereas the green line indicates a negative correlation. The size of a node is proportionally positive to the degree of the node, and the color of the node is related to the clustering coefficient, that is, the color gradient from dark colors to bright colors corresponds to the value of the aggregation coefficient from low to high.
S. japonicum Infection-Induced Metagenome Changes
Metagenomic analysis was used in this study to identify genes of host gut microbiota involved in specific pathways or functions during S. japonicum infection. An average of 56,686,511 valid reads per sample was obtained after removing sequences of the host, and then a non-redundant gene catalog that contained 1,048,575 clusters assembled into bacterial genes was constructed for further annotation. Afterwards, annotated metagenomic data were obtained by mapping to KEGG orthologs (kos), and the ko number was implemented to correspond to the KEGG pathway to indicate which genes were associated with specific metabolic pathways or functions (Supplementary Table 4). A hierarchical clustering heat map constructed based on the results from analysis of KEGG at level 3 demonstrated a remarkable ability to discriminate between healthy mice and infected mice in 14 different KEGG pathways (Figure 6). The 0-dpi group showed seven enriched pathways including transport and catabolism (regulation of mitophagy—yeast ko04139, regulation of autophagy ko04140), signal transduction (mTOR signaling pathway ko04150), immune system (RIG-I-like receptor signaling pathway ko04622), metabolism of terpenoids and polyketides (sesquiterpenoid and triterpenoid biosynthesis ko00909), xenobiotic biodegradation and metabolism (steroid degradation ko00984), and biosynthesis of other secondary metabolites (aflatoxin biosynthesis ko00254) (p < 0.05). Additionally, signal transduction (AMPK signaling pathway ko04152), immune system (chemokine signaling pathway ko04062), metabolism of terpenoids and polyketides (biosynthesis of vancomycin group antibiotics ko01055), metabolism of other amino acids (beta-alanine metabolism ko00410), biosynthesis of other secondary metabolites (penicillin and cephalosporin biosynthesis ko00311), and substance dependence (cocaine addiction ko05030) were significantly perturbed in the 42-dpi group (p < 0.05), whereas biosynthesis of other secondary metabolites (betalain biosynthesis ko00965) was altered significantly in the 21-dpi group, with p < 0.05.
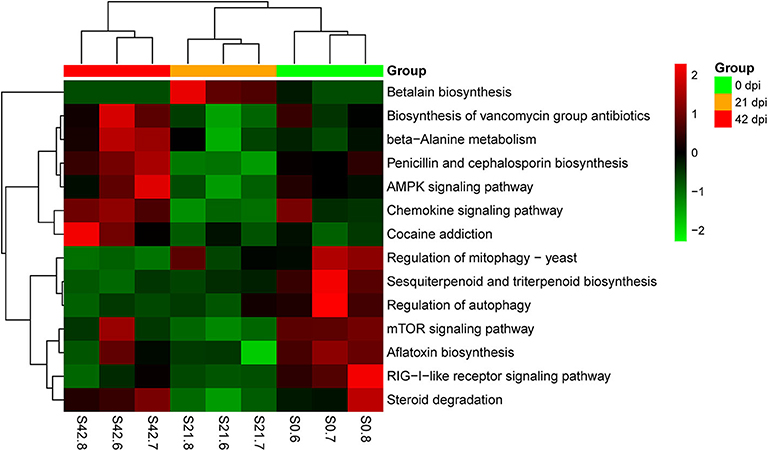
Figure 6. Hierarchical clustering heat map constructed using annotated metagenomic data on the basis of the Kyoto Encyclopedia of Genes and Genomes (KEGG). Samples are shown in columns, and KEGG pathways are shown in rows.
Multivariate Statistical Analysis of Metabolite Profiling
In PCA score plots, QC samples that applied to test analytical repeatability and instrument performance and stability were tightly clustered, which demonstrated high reproducibility of the instrument (Supplementary Figure 4). Representative base peak intensity chromatograms of all types of specimens at seven time points are shown in Supplementary Figures 5–8. Differences in peaks and peak heights were observed among all groups, which indicated that the composition of metabolite profiles of BALB/c mice was changed during S. japonicum infection.
Initially, PCA, which is an unsupervised method of pattern recognition aiming to identify the overall clustering patterns and trends in a data set without considering groups, was implemented to obtain a global view and determine whether the metabolites from these seven groups of mice differed. Based on the top three principal components, the PCA results showed distinguished classifications between the control mice and the mice with different statuses of S. japonicum infection from serum and urine samples separately in both ESI modes, indicating that S. japonicum infection had significant effects on mouse metabolism (Figure 7). However, the 0-dpi group and early infection groups appeared to be partially overlapping, and the latter were closer than the late infection groups to the controls in the PCA score plots, whereas the most profound differences were found between the 0-dpi group and the late infection groups, which illustrate that the changes in metabolite profiles were miniscule at the early stage of infection and then became significant in the later stage. However, samples derived from liver and colon aqueous extracts demonstrated unclear classifications between healthy mice and infected mice of different time periods of S. japonicum infection with PCA in negative ion mode. This finding is evident in the PCA score plots in Figure 7F, which revealed that the effects of S. japonicum infection on mouse metabolism were not as evident in tissue as they were in body fluids.
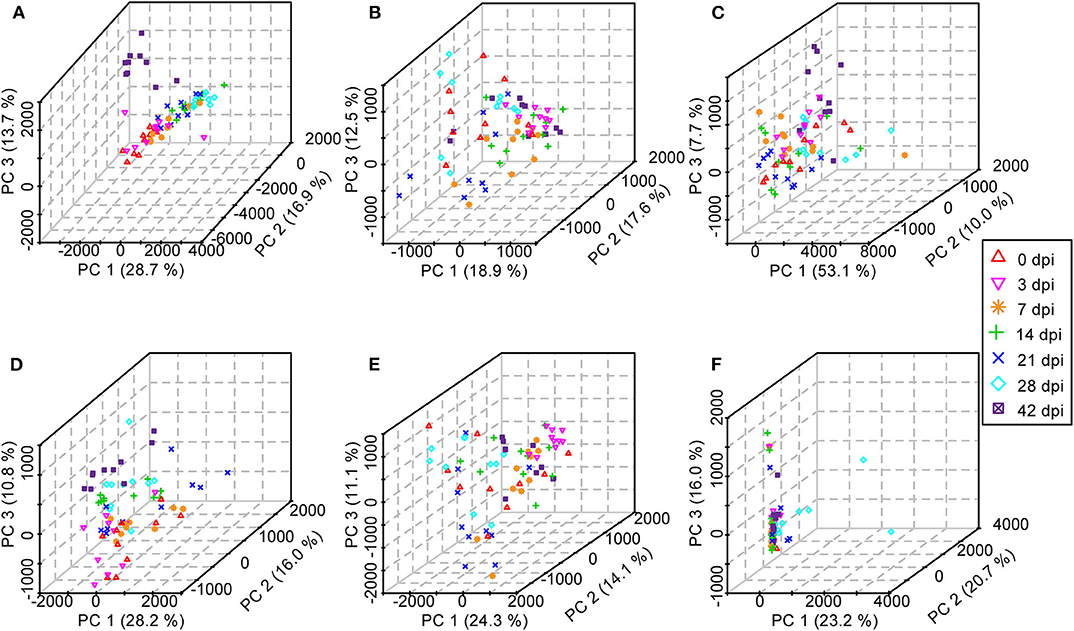
Figure 7. Three-dimensional score plots of principal component analysis from healthy mice and infected mice at different time points. Each point represents an individual. (A) Serum samples in positive electrospray ionization (ESI+) mode. (B) Urine samples in ESI+ mode. (C) Liver aqueous extracts in negative electrospray ionization (ESI-) mode. (D) Serum samples in ESI- mode. (E) Urine samples in ESI- mode. (F) Colon aqueous extracts in ESI- mode.
Subsequently, in order to enhance the separation among the different classes of samples, a supervised method, PLS-DA was performed. For serum, urine, and liver aqueous extracts, PLS-DA models were constructed for all time points of S. japonicum infection separately, and all groups could be readily clearly differentiated from each other by PLS1, PLS2, and PLS3 in both ESI modes; high values of R2 and Q2 of each model reflected the data stability and the good fit of the model parameters (Figure 8, Supplementary Table 5). These results revealed that the biochemical perturbations and the metabolic profiles of the infected groups were distinct from those of the uninfected group. Furthermore, notable separation was found between control mice and infected mice during the process of schistosomiasis. Nevertheless, colon aqueous extract samples from all groups still clustered together in the PLS-DA score plot.
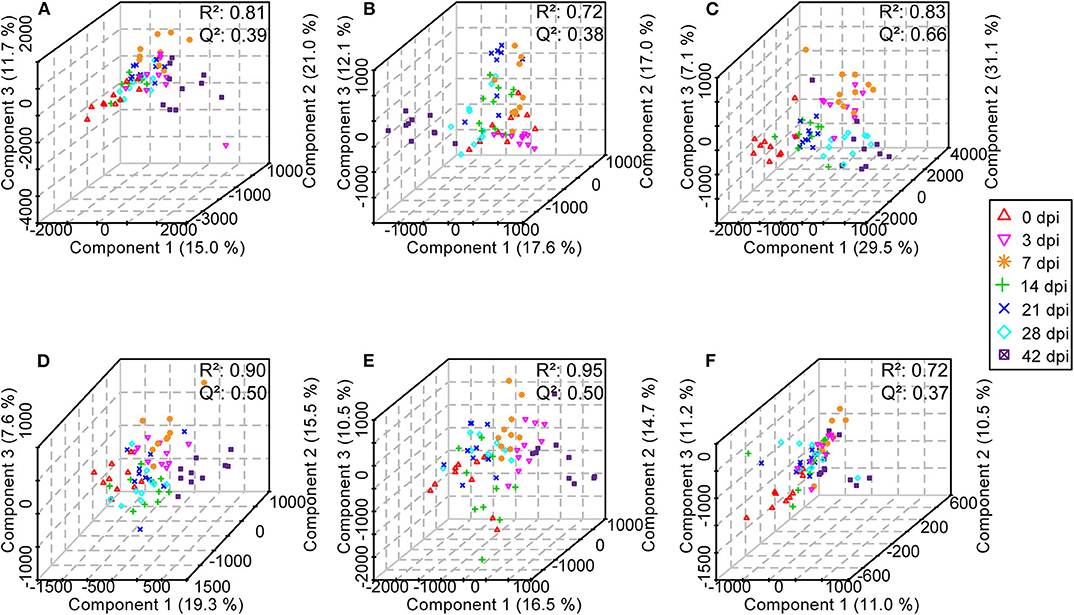
Figure 8. Three-dimensional score plots of partial least squares-discriminatory analysis from healthy mice and infected mice at different time points. Each point represents an individual. (A) Serum samples in positive electrospray ionization (ESI+) mode. (B) Urine samples in ESI+ mode. (C) Liver aqueous extracts in negative electrospray ionization (ESI-) mode. (D) Serum samples in ESI- mode. (E) Urine samples in ESI- mode. (F) Colon aqueous extracts in ESI- mode.
Finally, OPLS-DA was applied to reduce the dimension and produce the clearest separation between two groups as well as identifying metabolites that drive group distinction with one predictive and one orthogonal component (Supplementary Figures 9–14). The S-plots obtained from OPLS-DA were used to find out the meaningful and reliable variables that were attributable to the separation between two groups; the ions farther from the origin in the plot represent higher VIP values and were selected as potential metabolite biomarkers (Supplementary Figures 9–14).
Biomarker Identification and Analysis
A total of 42 unique compounds were identified in serum extracts, 53 unique compounds were identified in urine samples, and 24 unique compounds were identified from liver and colon aqueous extracts on the basis of the S-plot, VIP scores >2, p < 0.05, and QC samples' CV <30 (Supplementary Table 6). The majority of these metabolites were lipids, glucose, organic acids, nucleic acids, and amino acids. As shown in Supplementary Figure 15, hierarchical cluster analysis demonstrated the trends of significantly identified compounds for seven time points from all individuals. Most of the lipids were downregulated persistently during S. japonicum infection, while some phospholipids were upregulated in medium-term infection or late infection. However, several phospholipids were altered in only early- and medium-term infection, with downregulation. In addition, glucose and some organic acids were increased at 3 dpi but dropped to the uninfected levels after that. We picked out five potential biomarkers related to the early diagnosis of schistosomiasis (at 3 dpi) based on VIP value >5 and the value of the area under the ROC curve (AUC) >0.9. Phosphatidylcholine (PC) (22:6/18:0) and colfosceril palmitate in serum as well as xanthurenic acid, naphthalenesulfonic acid, and pimelylcarnitine in urine demonstrated promising diagnostic potential between the uninfected group and the 3-dpi group, with an AUC value of 0.9–1, while xanthurenic acid and naphthalenesulfonic acid exhibited the most discriminatory power since the sensitivity and the specificity were both 100% (Figure 9B). Therefore, xanthurenic acid and naphthalenesulfonic acid may be the most powerful targets for early diagnosis of schistosomiasis. The abundance of xanthurenic acid, PC (22:6/18:0), and colfosceril palmitate were significantly reduced at 3 dpi, whereas naphthalenesulfonic acid and pimelylcarnitine levels rose extremely at 3 dpi (Figure 9A).
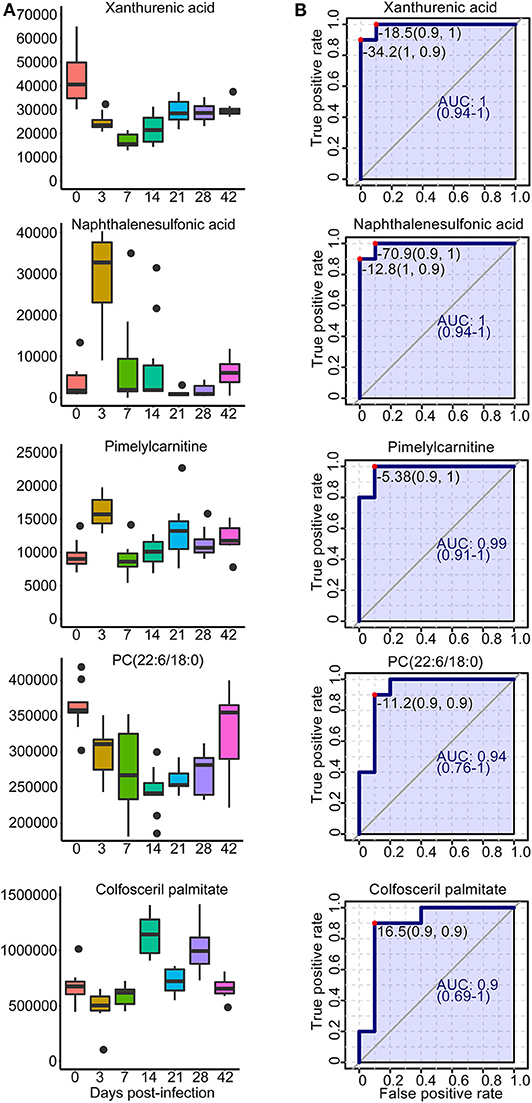
Figure 9. Overview of selected representative metabolites from serum and urine associated with schistosomiasis. (A) Box plots showing the abundance of selected biomarkers. Each column represents a time point. (B) The receiver operating characteristic curve for selected biomarkers. The x-axis represents the specificity, while the y-axis represents the sensitivity.
MSEA and Pathway Analysis
The MSEA results indicated that two sets of identified metabolites extracted from serum (Figure 10A) and six sets of identified metabolites in liver aqueous extracts (Figure 10C) were different between the uninfected group and the infected groups; among these, purine metabolism, caffeine metabolism, pyrimidine metabolism, and galactose metabolism were significantly enriched (p < 0.05). Moreover, the metabolites were grouped based on the KEGG database by pathway analysis, while purine metabolism (ko00230), sphingolipid metabolism (ko00600), and glycerophospholipid metabolism (ko00564) were significantly related to the process of schistosomiasis (p < 0.05) (Figures 10B,D). Among pathways of serum metabolites, glycerophospholipid metabolism and purine metabolism showed the impact factors of 0.18 and 0.04, respectively (Figure 10B), while purine metabolism demonstrated the impact factor of 0.03 in pathways of liver aqueous metabolites (Figure 10D), which indicated that these two pathways were disturbed mostly during S. japonicum infection.
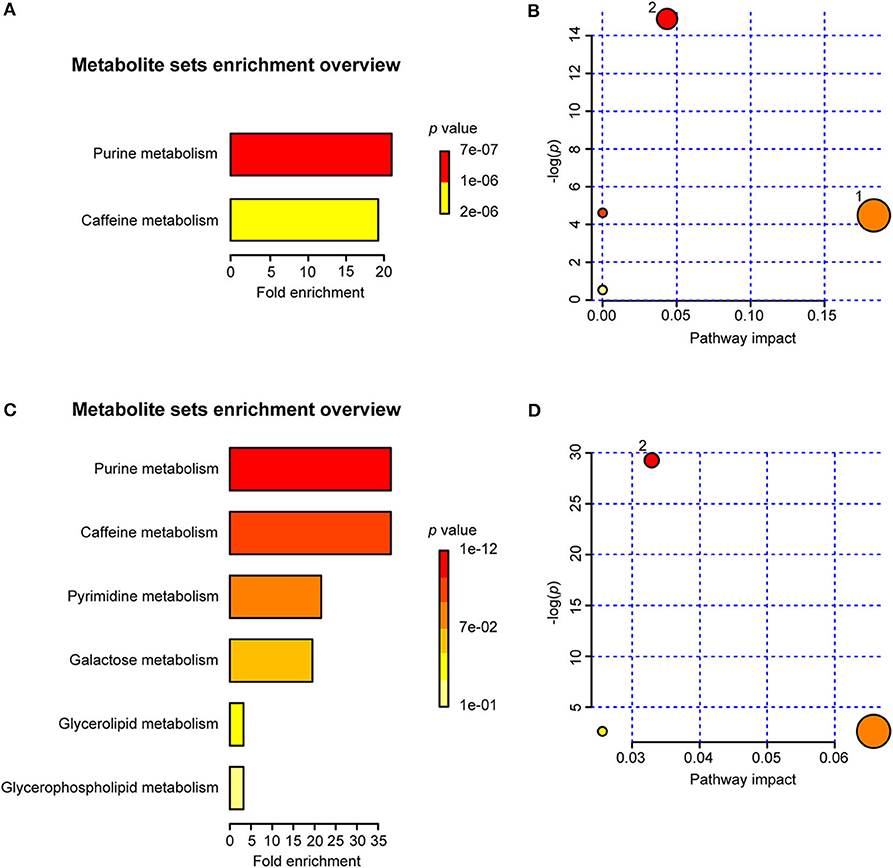
Figure 10. Summary of metabolite set enrichment analysis (MSEA) and pathway analysis. (A) Summary plot for MSEA in serum is ranked according to Holm p-value. (B) Pathway analysis of serum metabolites shows key nodes in metabolic pathways that have been significantly changed during an infection. The x-axis represents increasing metabolic pathway impact from pathway topology analysis, whereas the y-axis represents unadjusted p-value by pathway enrichment analysis. Greater pathway enrichment and higher pathway impact values are exhibited in larger sizes and darker colors, respectively. (C) Summary plot for MSEA in liver aqueous extracts is ranked according to Holm p-value. (D) Pathway analysis of liver aqueous metabolite shows key nodes in metabolic pathways that have been significantly changed during an infection. 1, glycerophospholipid metabolism; 2, purine metabolism.
Relationships Between Host Metabolome and Gut Microbiome
To investigate the functional correlation between the altered metabolites from the colon and fecal flora alterations, correlation analysis was conducted by calculating the Pearson's correlation coefficient. As shown in Figure 11, clear correlations between altered metabolic profiles and gut microbiome were observed; however, more metabolites were negatively correlated with fecal flora, whereas two compounds exhibited significant positive correlations with some bacterial groups (p < 0.05). Of particular note is that LysoPC (20:1), which decreased in the colon of S. japonicum-infected BALB/c mice, was positively correlated with the reduction of Lachnospiraceae NK4A136 group and Roseburia. Additionally, cortolone, which increased in the colon of S. japonicum-infected BALB/c mice, was positively correlated with the elevation of Bacteroides as well as Parabacteroides. In summary, S. japonicum infection induced significant perturbation in both the gut microbiome and the metabolomic profile of the host, which were interactive during the process of schistosomiasis.
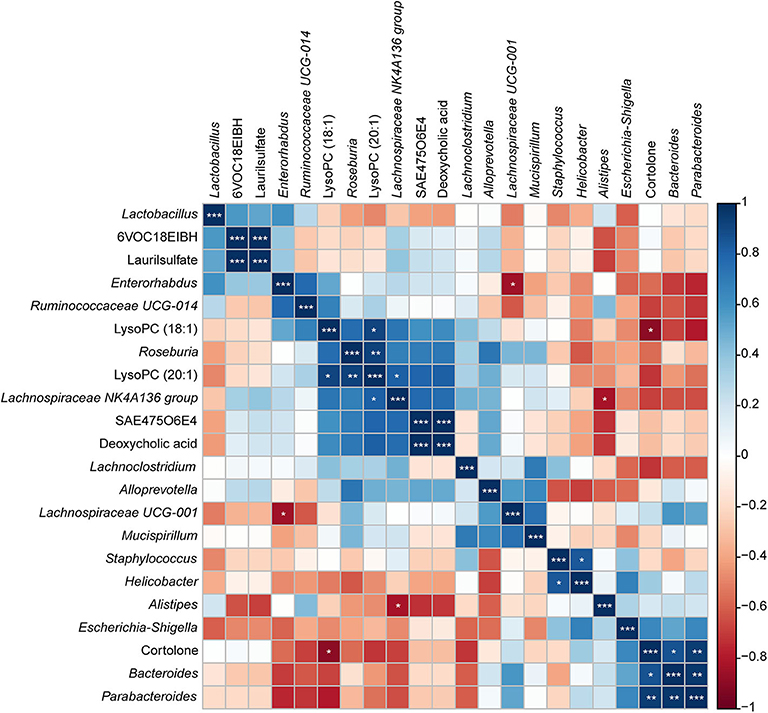
Figure 11. Correlation plot showing the relationship between the altered metabolites from the colon and the top 15 abundant gut bacteria. The correlations between them are exhibited by colors; blue indicates a positive correlation, red indicates a negative correlation, and a darker color illustrates a stronger correlation (*p < 0.05, **p < 0.01, ***p < 0.001).
Discussion
Parasitic infection can impact the gut microbiota composition of the host, and the underlying mechanism is its effect on the host immune system, which could break the balance between the gut microbiome and the host that has already been established (40). In this study, 16S rRNA sequencing was applied to demonstrate the dynamic alteration in the gut microbiome of BALB/c mice in response to S. japonicum infection and to identify bacteria that are crucial in the complex host-parasite interplay. The result of diversity analysis showed an overall reduction in alpha diversity and a relative increase in beta diversity of host gut bacteria after S. japonicum infection; beta diversity analysis also demonstrated strong associations between the gut microbiota composition and stage of infection, especially in the late stage, which was in conformity with previous studies of S. japonicum and S. mansoni (25, 41). As we have known, the schistosomula enter the venous blood vessels and are transported to the lungs where they become lung schistosomula at 3 dpi. At 7 dpi, the schistosomula enter the arterial circulation and then migrate to the mesenteric veins of the liver and become mature a week later. At 21 dpi, the worms migrate to the mesenteric veins of the bowel, and gametes of both the female and the male worms occur. Then, the female worm produces immature eggs at 28 dpi. At 42 dpi, mature eggs deposit in the liver of the host through the bloodstream or pass through the intestinal wall and are excreted in the feces. Thus, the gut microbiome community structures of the host are altered variously by S. japonicum infection due to the varying parasitic sites of the worm at different time points, and the gut microbiota of each individual respond differently according to the degree of homeostasis disruption. While the decrease in richness of the gut microbiota is harmful to the healthy individual who is infected by the parasite because the gut microbiome is crucial in providing a protective environment (42), for the individual with autoimmune diseases, such as Crohn's disease, ulcerative colitis (UC), and coeliac disease, parasites may actively contribute to reinstating gut homeostasis with quantitative and qualitative modifications of the gut microbiota, which can profoundly influence immune cell development and function in the intestine (43–46).
Firmicutes, Bacteroidetes, Proteobacteria, and Deferribacteres, which are common in the mouse (47), were also the dominant gut bacterial phyla in both infected and uninfected BALB/c mice, but no statistically significant differences were observed during the infection. Similarly, previous research has shown that Firmicutes decreased relatively and Bacteroidetes and Proteobacteria increased relatively in response to S. japonicum infection in C57BL/6 mice, whereas the same variations were not found in BALB/c mice (25). These results illustrated that the alteration in gut microbiome composition after S. japonicum infection was closely related to the host itself. The abundance of some prevalent genera, including Staphylococcus, Parabacteroides, Alistipes, Roseburia, and Ruminococcaceae UCG-014, was changed significantly during the infection. Both Parabacteroides and Alistipes are members of Bacteroidetes and were relatively abundant in response to infection, while members of Firmicutes, Roseburia, and Ruminococcaceae UCG-014 were decreased after the infection, which indicated that although no significant differences were found at the phylum level, significant differences were observed at the genus level, and the change trend of different genera from the same phylum was consistent in the course of the infection. Earlier studies have illustrated that Alistipes can produce anti-inflammatory metabolites, which would promote the differentiation of anti-inflammatory Treg/Tr1 cells in the gut of mice, and Alistipes can also protect mice suffering from the effects of dextran sulfate sodium (48, 49). Hence, depletion of Alistipes was found in Salmonella-infected mice, porcine epidemic diarrhea virus-infected piglets, and chronic hepatitis B patients (50–52). Nevertheless, Alistipes was reported to result in the increase of trimethylamine N-oxide and the decrease of short-chain fatty acids (SCFA) production, which led to the destruction of the intestinal barrier (51, 53). The enhanced abundance of Alistipes during S. japonicum infection may be caused by the stress reaction of the hosts. Furthermore, as probiotic-type bacteria, which are able to prevent pathogen infection, a reduction in the diversity and the abundance of Parabacteroides was observed in mice infected with intestinal helminth parasites in an earlier study (54), which was found at only 7 dpi of S. japonicum; however, the increased abundance of Parabacteroides after 7 dpi might be a consequence of stress response because some bacteria belonging to Parabacteroides were involved in the metabolism of amino acid. Consistently, previous studies have suggested that Roseburia, one kind of SCFA-producing bacteria that is essential for maintaining the gut function of humans and animals (55), was significantly decreased both in chronic kidney disease and in end-stage renal disease patients (56, 57), so the intestinal homeostasis was broken. Similarly, some members of Ruminococcaceae were butyrate producers, and the abundance of Ruminococcaceae UCG-014 reduced in a UC carcinogenesis model and even in a hypertriglyceridemia-related acute necrotizing pancreatitis model (58, 59). Interestingly, Staphylococcus, a classical non-enteric pathogen, was also found in the gut microbiome and increased in cystic fibrosis patients (60, 61), which was evident at 21 dpi of S. japonicum.
Using LEfSe, we noted that Alistipes (phylum Bacteroidetes) showed great abundance at 7 dpi and that another member from Bacteroidetes, Parabacteroides, was significantly associated with 14 dpi of S. japonicum. Furthermore, LEfSe identified a greater differential abundance of Staphylococcus (phylum Firmicutes) at 21 dpi, before the female adults laid eggs, while the greater differential abundance of Gammaproteobacteria was highlighted by LEfSe at 28 dpi, after the female adults laid eggs. Conversely, a significant over-representation of the class Gammaproteobacteria (phylum Proteobacteria) was identified in Clostridium difficile infection patients and children with acute diarrhea (62, 63). However, since we did not study bacteria with low abundance, we cannot draw a more precise conclusion based on the results of LEfSe. Correlation analysis of the top 30 dominant genera in the course of S. japonicum infection illustrated that most genera were positively related to each other, which showed that the co-occurrence relation was general in the gut microbiome for those who would work together even though they may belong to different phyla. Regardless, co-exclusion relationships were observed in Odoribacter (phylum Bacteroidetes) and Lachnoclostridium (phylum Firmicutes) as well as in Lachnospiraceae UCG-001 (phylum Firmicutes) and Enterorhabdus (phylum Actinobacteria). Odoribacter and Lachnospiraceae UCG-001 became more abundant, while the others became relatively less abundant during S. japonicum infection.
The results of the 16S rRNA gene-based analysis clarified the dynamic changes in the host gut microbiome in response to S. japonicum infection and the interactions between dominant genera. Subsequently, metagenomics sequencing was performed to search for altered pathways or functions at 0, 21, and 42 dpi of S. japonicum, which might enable us to generate hypotheses about the mechanisms underlying the infection. Consistent with the alterations in the gut microbiota, the functional metagenome was also changed significantly. In particular, the only ko category significantly enriched among the microbiome of BALB/c mice at 21 dpi was the biosynthesis of betalain, and the involved gene was tyrosinase (K00505), a kind of oxidoreductase. Betalain biosynthesis plays a crucial part in response to osmotic adjustment and salt resistance, and betaine is vital to enhance stress resistance (64), so the activation of betalain biosynthesis was helpful to the gut microbiome to resist stress while S. japonicum migrates to the mesenteric veins. Other particularly notable signaling pathways associated with S. japonicum infection at 42 dpi were the AMPK and the chemokine signaling pathways. The former was activated by calcium/calmodulin-dependent protein kinase exclusion β (CaMKK β, K07359), one of the upstream kinases, which reflected the elevated AMP/ATP ratio in response to the infection. Once activated, AMPK would lead to a concomitant inhibition of energy-consuming biosynthetic pathways, including the synthesis of glycogen and cholesterol. Chemokines play an important role in protective host response, and the upregulation of the chemokine signaling pathway at 42 dpi showed that the gut microbiota was sensitive to the infection and chemokine receptors were activated to produce an inflammatory immune response. In summary, we hypothesize that the gut microbiota in the host provide signals that resist the parasites and enhance the immune response of the host. These findings from the functional metagenome analysis offered a more detailed view of specific microbiome-encoded functions that are associated with S. japonicum infection and may facilitate further studies on the interaction between specific genes.
To describe the metabolic profile of BALB/c mice after S. japonicum infection, an untargeted UPLC–MS/MS-based high-resolution metabolomics analysis was performed in the present study. Both PCA and PLS-DA revealed differences between uninfected and infected mice, and the differences increased over time; however, more obvious variances were observed in body fluids than in tissues, which indicated that body fluids were affected more severely than cells during S. japonicum infection and that is why more differential metabolites were obtained in the former than in the latter. This result is expected because S. japonicum does not parasitize the host cell.
In response to S. japonicum infection, the significantly different metabolites were lipids, and the result is consistent with the prior study on S. japonicum and T. brucei rhodesiense (T. b. rhodesiense) (2, 16). Lipids are the major constituents of membranes and are important in reserving energy; they are also highly biologically active metabolites that are involved or even play a part in signaling and a range of inflammatory processes (65). As the major structural lipids that form cellular membranes, phospholipids participate in the regulation of nutrient transport as well as toxic host-cell effector molecules; they are synthesized to support the growth of cells in the course of infection, and phospholipid biosynthetic pathways are the targets of drugs (66–68). Our results demonstrated that most of the altered lipids belonged to glycerophospholipids, which are structural components of biological membranes (69), followed by sphingolipids and diacylglycerol. The majority of glycerophospholipids were significantly reduced in response to the infection, such as PC, phosphatidylethanolamine (PE), phosphatidylserine, phosphatidylinositol, and LysoPC. However, the concentration of some PCs was elevated in S. japonicum-infected mice, which was different from the results in S. haematobium-infected patients, where high levels of PC and PE were found (70). It is believed that the abundance of PC and PE in bladder endothelial cells may be one of the mechanisms for inducing cancer in chronic urogenital schistosomiasis (70); nevertheless, the different variations presented in PC after S. japonicum infection remain unclear, and future studies should address this question.
An endogenous phospholipid, LysoPC, which is derived from PC, has various stimulating or modulating effects on immune cells and possesses pro-inflammatory activities as well as anti-inflammatory properties; a heightened level of LysoPC in the plasma was considered as a marker for cell membrane injury (71, 72). Besides that, LysoPC participates in the elimination of dead eukaryotic and prokaryotic cells and controlling bacterial growth during infection (72, 73). Earlier studies have proven that LysoPC has direct antibacterial activities against methicillin-resistant Staphylococcus aureus as well as the ability to enhance neutrophil antimicrobial ability to remove ingested bacteria (74–76); however, our results illustrated that LysoPC does not inhibit all bacteria because LysoPC (20:1) was positively correlated with Lachnospiraceae NK4A136 group and Roseburia, the genera that are beneficial to the host during S. japonicum infection.
It cannot be ignored that some sphingomyelin (SM) species were reduced after infection, while the concentration of ceramide (Cer) was elevated, especially at the late stage of infection; the variation trend of SM and Cer was also observed in T. b. rhodesiense human African trypanosomiasis patients (16). Sphingolipids not only are components of the membrane that are able to protect the cell from harmful environmental factors but also are involved in cellular signaling as a second messenger (77, 78); the significant observations in sphingolipids (SM and Cer) may offer the basis and foundation for revealing the resistance mechanism between hosts and S. japonicum since infection can trigger the generation of Cer, which is generated in part by sphingomyelinase enzymes, leading to cell autophagy and apoptosis, additional pro-inflammatory cytokines, and chemokine synthesis, as well as other metabolic disorders (16, 79, 80). It is more interesting to note that the metagenomic analysis revealed that the chemokine signaling pathway was upregulated at 42 dpi, which suggests that inflammatory effects existed in gut bacteria also; the host and the gut microbiota collaborate with each other to eliminate the parasites.
Surprisingly, the levels of two kinds of carnitine species (acetylcarnitine and oleoylcarnitine) were significantly lower in infected mice than in uninfected mice, while another two kinds of carnitine species (hydroxyisovaleroyl carnitine and pimelylcarnitine) were increased at 3 dpi. Carnitine plays a crucial role in fat metabolism and energy production in mammals, and it can support the production of CD4+ and CD8+ T cells during infection (81, 82); a prior study has shown that the levels of carnitine and several acylcarnitines were elevated in S. japonicum-infected Microtus fortis (M. fortis) and C57BL/6 mice, but the phenomenon was more obvious in M. fortis (21). Hence, we suggest that host responses to S. japonicum infection vary and that the protective reaction against S. japonicum is relatively less strong in BALB/c mice.
Pathway analysis illuminated that both sphingolipid metabolism and glycerophospholipid metabolism were altered in the process of schistosomiasis, which is consistent with an earlier study of S. haematobium (70). In fact, sphingolipids and their derivatives have recently presented as promising drug targets for controlling infectious and inflammatory disease; however, how sphingolipid-mediated pathologies and how the host modifies sphingolipid metabolism to benefit itself remain unclear, and a better understanding of these mechanisms may provide new insights into new therapeutic strategies (83).
Nevertheless, because of the absence of age-matched uninfected BALB/c mice in this study, the alterations of gut microbiome and metabolites could be partially related to the age effect rather than the progression of S. japonicum infection alone.
Conclusions
In the present study, we applied 16S rRNA gene sequencing combined with a metagenomic sequencing approach and UPLC–MS metabolic profiling to highlight three aspects of the interrelationships between S. japonicum, the gut microbiome, and metabolites. We demonstrated that both the gut microbiome and the metabolites were significantly altered in S. japonicum-infected BALB/c mice; moreover, they were also associated with the time course of S. japonicum infection. In response to S. japonicum infection, not only the richness and diversity of gut microbiota were decreased but also the composition of the microbiota which differed obviously from that present during the uninfected status. In summary, the abundance of some bacteria that could produce SCFA was decreased, while those of some opportunistic pathogens that could raise the risk of infections were increased. In addition, metagenomic analysis revealed that the AMPK and chemokine signaling pathways were significantly perturbed after infection. The metabolic biomarkers that we identified in this study were found in serum or urine, with little or no invasiveness, and could distinguish S. japonicum infection from non-infection at 3 dpi with high sensitivity and specificity. Additionally, alterations in glycerophospholipid and purine metabolism were discovered in S. japonicum infection. As a result, these findings may provide a novel understanding of the mechanisms during schistosomiasis development regarding aspects of the gut microbiome and metabolites and facilitate the discovery of new targets for early diagnosis and prognosis. Nevertheless, further validations of potential biomarkers in human populations are essential, and the exact mechanisms of interactions between S. japonicum, the gut microbiome, and metabolites also await future research.
Data Availability Statement
The sequencing data of 16S rRNA gene and metagenome have been deposited in the NCBI Sequence Read Archive under the project number PRJNA602960 and PRJNA602878.
Ethics Statement
The animal study was reviewed and approved by the Animal Care and Use Committee of Sun Yat-sen University.
Author Contributions
ZL conceived and designed the study. ZL, YH, and JC drafted the manuscript. YH and JC carried out the experiments. YX, HoZ, PH, YM, MG, SC, and HaZ participated in data analysis. HaZ participated in study design, technological guidance, and coordination. All authors contributed to the article and approved the submitted version.
Funding
This work was supported by grants from the National Natural Science Foundation of China (grant nos. 81572023 and 81371836), the Science and Technology Planning Project of Guangdong Province (grant no. 2019B030316025), the Natural Science Foundation of Guangdong Province (grant no. 2019A1515011541), the National Key Research and Development Program of China (grant nos. 2016YFC1202000 and 2016YFC1200500), the Project of Basic Platform of National Science and Technology Resources of the Ministry of Sciences and Technology of China (grant no. TDRC-2017-22), the 111 Project (grant no. B12003), the Undergraduates Innovation Training Program of Guangdong Province (grant no. 201601084), and the Teaching Reform Project of Guangdong Province (grant no. 2017001).
Conflict of Interest
The authors declare that the research was conducted in the absence of any commercial or financial relationships that could be construed as a potential conflict of interest.
Acknowledgments
We would like to thank Mrs. Tingchan Liang for polishing the manuscript.
Supplementary Material
The Supplementary Material for this article can be found online at: https://www.frontiersin.org/articles/10.3389/fimmu.2020.569727/full#supplementary-material
Supplementary Figure 1. A flower plot showing unique and common operational taxonomic units among all samples.
Supplementary Figure 2. Rarefaction plots of 16S rRNA gene sequences obtained from fecal samples across multiple time points based on alpha diversity analyses. Lines represent the means, and the standard deviations are shown by error bars. (A) Observed species. (B) Chao1. (C) Shannon–Wiener index. (D) Phylogenetic diversity index.
Supplementary Figure 3. Principal coordinate analysis (PCoA) plots showing beta diversity differences on the basis of 16S rRNA gene sequencing during S. japonicum infection. Figures were calculated using weighted UniFrac distance, and each point represents an individual. (A) Two-dimensional PCoA plot. (B) Three-dimensional PCoA plot.
Supplementary Figure 4. Three-dimensional score plots of principal component analysis from quality control and samples. (A) Serum samples in positive electrospray ionization (ESI+) mode. (B) Urine samples in ESI+ mode. (C) Liver aqueous extracts in negative electrospray ionization (ESI-) mode. (D) Serum samples in ESI- mode. (E) Urine samples in ESI- mode. (F) Colon aqueous extracts in ESI- model.
Supplementary Figure 5. Base peak intensity chromatograms of serum samples from different time points in ESI+ mode (A) and ESI- mode (B) as analyzed by quadrupole-time-of-flight mass spectrometry with a full scan.
Supplementary Figure 6. Base peak intensity chromatograms of urine samples from different time points in ESI+ mode (A) and ESI- mode (B) as analyzed by quadrupole-time-of-flight mass spectrometry with a full scan.
Supplementary Figure 7. Base peak intensity chromatograms of liver aqueous extracts from different time points in ESI- mode as analyzed by quadrupole-time-of-flight mass spectrometry with a full scan.
Supplementary Figure 8. Base peak intensity chromatograms of colon aqueous extracts from different time points in ESI- mode as analyzed by quadrupole-time-of-flight mass spectrometry with a full scan.
Supplementary Figure 9. Orthogonal partial least squares-discriminatory analysis (OPLS-DA) of serum extracts in ESI+ mode at different stages of Schistosoma japonicum infection. Each point represents one sample in score plots. OPLS-DA loadings S-plot combined with the covariance and the correlation loading profile indicate the variable importance. Each point represents a variable.
Supplementary Figure 10. Orthogonal partial least squares-discriminatory analysis (OPLS-DA) from serum extracts in ESI- mode at different stages of Schistosoma japonicum infection. Each point represents one sample in score plots. OPLS-DA loadings S-plot combined with the covariance and the correlation loading profile indicate the variable importance. Each point represents a variable.
Supplementary Figure 11. Orthogonal partial least squares-discriminatory analysis (OPLS-DA) from urine extracts in ESI+ mode at different stages of Schistosoma japonicum infection. Each point represents one sample in score plots. OPLS-DA loadings S-plot combined with the covariance and the correlation loading profile indicate the variable importance. Each point represents a variable.
Supplementary Figure 12. Orthogonal partial least squares-discriminatory analysis (OPLS-DA) from urine extracts in ESI- mode at different stages of Schistosoma japonicum infection. Each point represents one sample in score plots. OPLS-DA loadings S-plot combined with the covariance and the correlation loading profile indicate the variable importance. Each point represents a variable.
Supplementary Figure 13. Orthogonal partial least squares-discriminatory analysis (OPLS-DA) from liver aqueous extracts in ESI- mode at different stages of Schistosoma japonicum infection. Each point represents one sample in score plots. OPLS-DA loadings S-plot combined with the covariance and the correlation loading profile indicate the variable importance. Each point represents a variable.
Supplementary Figure 14. Orthogonal partial least squares-discriminatory analysis (OPLS-DA) from colon aqueous extracts in ESI- mode at different stages of Schistosoma japonicum infection. Each point represents one sample in score plots. OPLS-DA loadings S-plot combined with the covariance and the correlation loading profile indicate the variable importance. Each point represents a variable.
Supplementary Figure 15. Heat map visualization for the significantly different identified metabolites at different time points. Each column represents a sample, and each row represents a metabolite. The scaled intensity of the metabolites is reflected by the color key; higher intensity levels, when compared to the mean metabolite intensity value, are colored red, while lower intensity levels are colored green. Branch height represents the similarity between two metabolites, and metabolites with the shortest vertical difference indicate the closest connection.
Supplementary Table 1. Operational taxonomic unit tables and taxonomic classifications of the 16S rRNA gene.
Supplementary Table 2. One-way ANOVA of relative abundances of gut microbiome constituents at the phylum level.
Supplementary Table 3. One-way ANOVA of relative abundances of gut microbiome constituents at the genus level.
Supplementary Table 4. KEGG annotation of metagenomic data.
Supplementary Table 5. Q2 and R2 values are provided to show partial least squares-discriminatory analysis (PLS-DA) classification performance with different number of components.
Supplementary Table 6. Statistically significantly changed metabolites identified in serum samples, urine samples, liver aqueous extracts and colon aqueous extracts (p < 0.05).
Footnotes
1. ^http://huttenhower.org/galaxy
2. ^http://www.ncbi.nlm.nih.gov/assembly/GCF_000001635.23
3. ^http://soap.genomics.org.cn/
4. ^https://github.com/hyattpd/Prodigal
5. ^http://www.bioinformatics.org/cd-hit/
6. ^http://blast.ncbi.nlm.nih.gov/Blast.cgi
7. ^http://www.genome.jp/kegg/pathway.html (KEGG)
References
1. Li Q, Zhao N, Liu M, Shen H, Huang L, Mo X, et al. Comparative analysis of proteome-wide lysine acetylation in juvenile and adult Schistosoma japonicum. Front Microbiol. (2017) 8:2248. doi: 10.3389/fmicb.2017.02248
2. Wu J, Xu W, Ming Z, Dong H, Tang H, Wang Y. Metabolic changes reveal the development of schistosomiasis in mice. PLoS Negl Trop Dis. (2010) 4:e807. doi: 10.1371/journal.pntd.0000807
3. Liu S, Zhou X, Piao X, Wu C, Hou N, Chen Q. Comparative analysis of transcriptional profiles of adult Schistosoma japonicum from different laboratory animals and the natural host, water buffalo. PLoS Negl Trop Dis. (2015) 9:e0003993. doi: 10.1371/journal.pntd.0003993
4. Ke XD, Shen S, Song LJ, Yu CX, Kikuchi M, Hirayama K, et al. Characterization of Schistosoma japonicum CP1412 protein as a novel member of the ribonuclease T2 molecule family with immune regulatory function. Parasit Vectors. (2017) 10:89. doi: 10.1186/s13071-016-1962-y
5. Qiu S, Fan X, Yang Y, Dong P, Zhou W, Xu Y, et al. Schistosoma japonicum infection downregulates house dust mite-induced allergic airway inflammation in mice. PLoS ONE. (2017) 12:e0179565. doi: 10.1371/journal.pone.0179565
6. Wang Y, Utzinger J, Xiao SH, Xue J, Nicholson JK, Tanner M, et al. System level metabolic effects of a Schistosoma japonicum infection in the Syrian hamster. Mol Biochem Parasitol. (2006) 146:1–9. doi: 10.1016/j.molbiopara.2005.10.010
7. Gouveia LR, Santos JC, Silva RD, Batista AD, Domingues ALC, Lopes EPA, et al. Diagnosis of coinfection by schistosomiasis and viral hepatitis B or C using 1H NMR-based metabonomics. PLoS ONE. (2017) 12:e0182196. doi: 10.1371/journal.pone.0182196
8. Martin FP, Wang Y, Sprenger N, Yap IK, Lundstedt T, Lek P, et al. Probiotic modulation of symbiotic gut microbial-host metabolic interactions in a humanized microbiome mouse model. Mol Syst Biol. (2008) 4:157. doi: 10.1038/msb4100190
9. Feng Q, Liu Z, Zhong S, Li R, Xia H, Jie Z, et al. Integrated metabolomics and metagenomics analysis of plasma and urine identified microbial metabolites associated with coronary heart disease. Sci Rep. (2016) 6:22525. doi: 10.1038/srep22525
10. Li N, Liu Y, Li W, Zhou L, Li Q, Wang X, et al. A UPLC/MS-based metabolomics investigation of the protective effect of ginsenosides Rg1 and Rg2 in mice with Alzheimer's disease. J Ginseng Res. (2016) 40:9–17. doi: 10.1016/j.jgr.2015.04.006
11. Nassar AF, Wu T, Nassar SF, Wisnewski AV. UPLC-MS for metabolomics: a giant step forward in support of pharmaceutical research. Drug Discov Today. (2017) 22:463–70. doi: 10.1016/j.drudis.2016.11.020
12. Olszewski KL, Morrisey JM, Wilinski D, Burns JM, Vaidya AB, Rabinowitz JD, et al. Host-parasite interactions revealed by Plasmodium falciparum metabolomics. Cell Host Microbe. (2009) 5:191–9. doi: 10.1016/j.chom.2009.01.004
13. MacRae JI, Dixon MW, Dearnley MK, Chua HH, Chambers JM, Kenny S, et al. Mitochondrial metabolism of sexual and asexual blood stages of the malaria parasite Plasmodium falciparum. BMC Biol. (2013) 11:67. doi: 10.1186/1741-7007-11-67
14. Orikiiriza J, Surowiec I, Lindquist E, Bonde M, Magambo J, Muhinda C, et al. Lipid response patterns in acute phase paediatric Plasmodium falciparum malaria. Metabolomics. (2017) 13:41. doi: 10.1007/s11306-017-1174-2
15. Creek DJ, Mazet M, Achcar F, Anderson J, Kim DH, Kamour R, et al. Probing the metabolic network in bloodstream-form Trypanosoma brucei using untargeted metabolomics with stable isotope labelled glucose. PLoS Pathog. (2015) 11:e1004689. doi: 10.1371/journal.ppat.1004689
16. Lamour SD, Gomez-Romero M, Vorkas PA, Alibu VP, Saric J, Holmes E, et al. Discovery of infection associated metabolic markers in human african trypanosomiasis. PLoS Negl Trop Dis. (2015) 9:e0004200. doi: 10.1371/journal.pntd.0004200
17. Vincent IM, Daly R, Courtioux B, Cattanach AM, Bieler S, Ndung'u JM, et al. Metabolomics identifies multiple candidate biomarkers to diagnose and stage human african trypanosomiasis. PLoS Negl Trop Dis. (2016) 10:e0005140. doi: 10.1371/journal.pntd.0005140
18. Ramakrishnan S, Docampo MD, MacRae JI, Ralton JE, Rupasinghe T, McConville MJ, et al. The intracellular parasite Toxoplasma gondii depends on the synthesis of long-chain and very long-chain unsaturated fatty acids not supplied by the host cell. Mol Microbiol. (2015) 97:64–76. doi: 10.1111/mmi.13010
19. Chen XQ, Zhou CX, Elsheikha HM, He S, Hu GX, Zhu XQ. Profiling of the perturbed metabolomic state of mouse spleen during acute and chronic toxoplasmosis. Parasit Vectors. (2017) 10:339. doi: 10.1186/s13071-017-2282-6
20. Zhou CX, Cong W, Chen XQ, He SY, Elsheikha HM, Zhu XQ. Serum metabolic profiling of oocyst-induced Toxoplasma gondii acute and chronic infections in mice using mass-spectrometry. Front Microbiol. (2017) 8:2612. doi: 10.3389/fmicb.2017.02612
21. Hu Y, Sun L, Yuan Z, Xu Y, Cao J. High throughput data analyses of the immune characteristics of Microtus fortis infected with Schistosoma japonicum. Sci Rep. (2017) 7:11311. doi: 10.1038/s41598-017-11532-2
22. Douglas AE. Lessons from studying insect symbioses. Cell Host Microbe. (2011) 10:359–67. doi: 10.1016/j.chom.2011.09.001
23. Schwarz RS, Moran NA, Evans JD. Early gut colonizers shape parasite susceptibility and microbiota composition in honey bee workers. Proc Natl Acad Sci USA. (2016) 113:9345–50. doi: 10.1073/pnas.1606631113
24. McManus DP, Dunne DW, Sacko M, Utzinger J, Vennervald BJ, Zhou XN. Schistosomiasis. Nat Rev Dis Primers. (2018) 4:13. doi: 10.1038/s41572-018-0013-8
25. Zhao Y, Yang S, Li B, Li W, Wang J, Chen Z, et al. Alterations of the Mice Gut Microbiome via Schistosoma japonicum Ova-Induced Granuloma. Front Microbiol. (2019) 10:352. doi: 10.3389/fmicb.2019.00352
26. Bolger AM, Lohse M, Usadel B. Trimmomatic: a flexible trimmer for Illumina sequence data. Bioinformatics. (2014) 30:2114–20. doi: 10.1093/bioinformatics/btu170
27. Reyon D, Tsai SQ, Khayter C, Foden JA, Sander JD, Joung JK. FLASH assembly of TALENs for high-throughput genome editing. Nat Biotechnol. (2012) 30:460–5. doi: 10.1038/nbt.2170
28. Caporaso JG, Kuczynski J, Stombaugh J, Bittinger K, Bushman FD, Costello EK, et al. QIIME allows analysis of high-throughput community sequencing data. Nat Methods. (2010) 7:335–6. doi: 10.1038/nmeth.f.303
29. Edgar RC. UPARSE: highly accurate OTU sequences from microbial amplicon reads. Nat Methods. (2013) 10:996–8. doi: 10.1038/nmeth.2604
30. Wang Q, Garrity GM, Tiedje JM, Cole JR. Naive Bayesian classifier for rapid assignment of rRNA sequences into the new bacterial taxonomy. Appl Environ Microbiol. (2007) 73:5261–7. doi: 10.1128/AEM.00062-07
31. Patel RK, Jain M. NGS QC Toolkit: a toolkit for quality control of next generation sequencing data. PLoS ONE. (2012) 7:e30619. doi: 10.1371/journal.pone.0030619
32. Li H, Durbin R. Fast and accurate long-read alignment with Burrows-Wheeler transform. Bioinformatics. (2010) 26:589–95. doi: 10.1093/bioinformatics/btp698
33. Luo R, Liu B, Xie Y, Li Z, Huang W, Yuan J, et al. SOAPdenovo2: an empirically improved memory-efficient short-read de novo assembler. Gigascience. (2012) 1:18. doi: 10.1186/2047-217X-1-18
34. Hyatt D, Chen GL, Locascio PF, Land ML, Larimer FW, Hauser LJ. Prodigal: prokaryotic gene recognition and translation initiation site identification. BMC Bioinform. (2010) 11:119. doi: 10.1186/1471-2105-11-119
35. Want EJ, Masson P, Michopoulos F, Wilson ID, Theodoridis G, Plumb RS, et al. Global metabolic profiling of animal and human tissues via UPLC-MS. Nat Protoc. (2013) 8:17–32. doi: 10.1038/nprot.2012.135
36. Xia J, Wishart DS. Web-based inference of biological patterns, functions and pathways from metabolomic data using MetaboAnalyst. Nature Protocols. (2011) 6:743–60. doi: 10.1038/nprot.2011.319
37. Chong J, Soufan O, Li C, Caraus I, Li S, Bourque G, et al. MetaboAnalyst 4.0: towards more transparent and integrative metabolomics analysis. Nucleic Acids Res. (2018) 46:W486–94. doi: 10.1093/nar/gky310
38. Wishart DS, Jewison T, Guo AC, Wilson M, Knox C, Liu Y, et al. HMDB 3.0–the human metabolome database in 2013. Nucleic Acids Res. (2013) 41(Database issue):D801–7. doi: 10.1093/nar/gks1065
39. Tautenhahn R, Cho K, Uritboonthai W, Zhu Z, Patti GJ, Siuzdak G. An accelerated workflow for untargeted metabolomics using the METLIN database. Nat Biotechnol. (2012) 30:826–8. doi: 10.1038/nbt.2348
40. Guernier V, Brennan B, Yakob L, Milinovich G, Clements AC, Soares Magalhaes RJ. Gut microbiota disturbance during helminth infection: can it affect cognition and behaviour of children? BMC Infect Dis. (2017) 17:58. doi: 10.1186/s12879-016-2146-2
41. Jenkins TP, Peachey LE, Ajami NJ, MacDonald AS, Hsieh MH, Brindley PJ, et al. Schistosoma mansoni infection is associated with quantitative and qualitative modifications of the mammalian intestinal microbiota. Sci Rep. (2018) 8:12072. doi: 10.1038/s41598-018-30412-x
42. Van den Abbeele P, Van de Wiele T, Verstraete W, Possemiers S. The host selects mucosal and luminal associations of coevolved gut microorganisms: a novel concept. FEMS Microbiol Rev. (2011) 35:681–704. doi: 10.1111/j.1574-6976.2011.00270.x
43. Broadhurst MJ, Ardeshir A, Kanwar B, Mirpuri J, Gundra UM, Leung JM, et al. Therapeutic helminth infection of macaques with idiopathic chronic diarrhea alters the inflammatory signature and mucosal microbiota of the colon. PLoS Pathog. (2012) 8:e1003000. doi: 10.1371/journal.ppat.1003000
44. Boyett D, Hsieh MH. Wormholes in host defense: how helminths manipulate host tissues to survive and reproduce. PLoS Pathog. (2014) 10:e1004014. doi: 10.1371/journal.ppat.1004014
45. Lee SC, Tang MS, Lim YA, Choy SH, Kurtz ZD, Cox LM, et al. Helminth colonization is associated with increased diversity of the gut microbiota. PLoS Negl Trop Dis. (2014) 8:e2880. doi: 10.1371/journal.pntd.0002880
46. Giacomin P, Croese J, Krause L, Loukas A, Cantacessi C. Suppression of inflammation by helminths: a role for the gut microbiota? Philos Trans R Soc Lond B Biol Sci. (2015) 370:1675. doi: 10.1098/rstb.2014.0296
47. Deng H, Yang S, Zhang Y, Qian K, Zhang Z, Liu Y, et al. Bacteroides fragilis prevents Clostridium difficile infection in a mouse model by restoring gut barrier and microbiome regulation. Front Microbiol. (2018) 9:2976. doi: 10.3389/fmicb.2018.02976
48. Dziarski R, Park SY, Kashyap DR, Dowd SE, Gupta D. Pglyrp-regulated gut microflora Prevotella falsenii, Parabacteroides distasonis and Bacteroides eggerthii enhance and Alistipes finegoldii attenuates colitis in mice. PLoS ONE. (2016) 11:e0146162. doi: 10.1371/journal.pone.0146162
49. Li J, Sung CY, Lee N, Ni Y, Pihlajamaki J, Panagiotou G, et al. Probiotics modulated gut microbiota suppresses hepatocellular carcinoma growth in mice. Proc Natl Acad Sci USA. (2016) 113:E1306–15. doi: 10.1073/pnas.1518189113
50. Borton MA, Sabag-Daigle A, Wu J, Solden LM, O'Banion BS, Daly RA, et al. Chemical and pathogen-induced inflammation disrupt the murine intestinal microbiome. Microbiome. (2017) 5:47. doi: 10.1186/s40168-017-0264-8
51. Wang J, Wang Y, Zhang X, Liu J, Zhang Q, Zhao Y, et al. Gut Microbial dysbiosis is associated with altered hepatic functions and serum metabolites in chronic hepatitis B patients. Front Microbiol. (2017) 8:2222. doi: 10.3389/fmicb.2017.02222
52. Huang A, Cai R, Wang Q, Shi L, Li C, Yan H. Dynamic change of gut microbiota during porcine epidemic diarrhea virus infection in suckling piglets. Front Microbiol. (2019) 10:322. doi: 10.3389/fmicb.2019.00322
53. Chiu CY, Cheng ML, Chiang MH, Kuo YL, Tsai MH, Chiu CC, et al. Gut microbial-derived butyrate is inversely associated with IgE responses to allergens in childhood asthma. Pediatr Allergy Immunol. (2019) 30:689–97. doi: 10.1111/pai.13096
54. Houlden A, Hayes KS, Bancroft AJ, Worthington JJ, Wang P, Grencis RK, et al. Chronic trichuris muris infection in C57BL/6 mice causes significant changes in host microbiota and metabolome: effects reversed by pathogen clearance. PLoS ONE. (2015) 10:e0125945. doi: 10.1371/journal.pone.0125945
55. Liang H, Dai Z, Liu N, Ji Y, Chen J, Zhang Y, et al. Dietary L-tryptophan modulates the structural and functional composition of the intestinal microbiome in weaned piglets. Front Microbiol. (2018) 9:1736. doi: 10.3389/fmicb.2018.01736
56. Jiang S, Xie S, Lv D, Zhang Y, Deng J, Zeng L, et al. A reduction in the butyrate producing species Roseburia spp. and Faecalibacterium prausnitzii is associated with chronic kidney disease progression. Antonie Van Leeuwenhoek. (2016) 109:1389–96. doi: 10.1007/s10482-016-0737-y
57. Jiang S, Xie S, Lv D, Wang P, He H, Zhang T, et al. Alteration of the gut microbiota in Chinese population with chronic kidney disease. Sci Rep. (2017) 7:2870. doi: 10.1038/s41598-017-02989-2
58. Huang C, Chen J, Wang J, Zhou H, Lu Y, Lou L, et al. Dysbiosis of intestinal microbiota and decreased antimicrobial peptide level in paneth cells during hypertriglyceridemia-related acute necrotizing pancreatitis in rats. Front Microbiol. (2017) 8:776. doi: 10.3389/fmicb.2017.00776
59. Wang CS, Li WB, Wang HY, Ma YM, Zhao XH, Yang H, et al. VSL#3 can prevent ulcerative colitis-associated carcinogenesis in mice. World J Gastroenterol. (2018) 24:4254–62. doi: 10.3748/wjg.v24.i37.4254
60. Tamburini FB, Andermann TM, Tkachenko E, Senchyna F, Banaei N, Bhatt AS. Precision identification of diverse bloodstream pathogens in the gut microbiome. Nat Med. (2018) 24:1809–14. doi: 10.1038/s41591-018-0202-8
61. Vernocchi P, Del Chierico F, Russo A, Majo F, Rossitto M, Valerio M, et al. Gut microbiota signatures in cystic fibrosis: Loss of host CFTR function drives the microbiota enterophenotype. PLoS ONE. (2018) 13:e0208171. doi: 10.1371/journal.pone.0208171
62. Milani C, Ticinesi A, Gerritsen J, Nouvenne A, Lugli GA, Mancabelli L, et al. Gut microbiota composition and Clostridium difficile infection in hospitalized elderly individuals: a metagenomic study. Sci Rep. (2016) 6:25945. doi: 10.1038/srep25945
63. Dinleyici EC, Martinez-Martinez D, Kara A, Karbuz A, Dalgic N, Metin O, et al. Time series analysis of the microbiota of children suffering from acute infectious diarrhea and their recovery after treatment. Front Microbiol. (2018) 9:1230. doi: 10.3389/fmicb.2018.01230
64. Zhang X, Yuan J, Zhang X, Liu C, Xiang J, Li F. Genome-wide analysis of alternative splicing provides insights into stress response of the pacific white shrimp Litopenaeus vanname. Front Genet. (2019) 10:845. doi: 10.3389/fgene.2019.00845
65. Calder PC. Polyunsaturated fatty acids, inflammation, and immunity. Lipids. (2001) 36:1007–24. doi: 10.1007/s11745-001-0812-7
66. de Kroon AI, Rijken PJ, De Smet CH. Checks and balances in membrane phospholipid class and acyl chain homeostasis, the yeast perspective. Prog Lipid Res. (2013) 52:374–94. doi: 10.1016/j.plipres.2013.04.006
67. Takenami I, de Oliveira CC, Petrilli JD, Machado A, Riley LW, Arruda S. Serum antiphospholipid antibody levels as biomarkers for diagnosis of pulmonary tuberculosis patients. Int J Tuberc Lung Dis. (2018) 22:1063–70. doi: 10.5588/ijtld.17.0874
68. Tams RN, Cassilly CD, Anaokar S, Brewer WT, Dinsmore JT, Chen YL, et al. Overproduction of phospholipids by the kennedy pathway leads to hypervirulence in Candida albicans. Front Microbiol. (2019) 10:86. doi: 10.3389/fmicb.2019.00086
69. Zufferey R, Pirani K, Cheung-See-Kit M, Lee S, Williams TA, Chen DG, et al. The Trypanosoma brucei dihydroxyacetonephosphate acyltransferase TbDAT is dispensable for normal growth but important for synthesis of ether glycerophospholipids. PLoS ONE. (2017) 12:e0181432. doi: 10.1371/journal.pone.018143
70. Adebayo AS, Mundhe SD, Awobode HO, Onile OS, Agunloye AM, Isokpehi RD, et al. Metabolite profiling for biomarkers in Schistosoma haematobium infection and associated bladder pathologies. PLoS Negl Trop Dis. (2018) 12:e0006452. doi: 10.1371/journal.pntd.0006452
71. Gao X, Guo M, Li Q, Peng L, Liu H, Zhang L, et al. Plasma metabolomic profiling to reveal antipyretic mechanism of Shuang-huang-lian injection on yeast-induced pyrexia rats. PLoS ONE. (2014) 9:e100017. doi: 10.1371/journal.pone.0100017
72. Lee HJ, Ko HJ, Song DK, Jung YJ. Lysophosphatidylcholine promotes phagosome maturation and regulates inflammatory mediator production through the protein kinase a-phosphatidylinositol 3 kinase-p38 mitogen-activated protein kinase signaling pathway during Mycobacterium tuberculosis infection in mouse macrophages. Front Immunol. (2018) 9:920. doi: 10.3389/fimmu.2018.00920
73. Parra Millan R, Jimenez Mejias ME, Sanchez Encinales V, Ayerbe Algaba R, Gutierrez Valencia A, Pachon Ibanez ME, et al. Efficacy of lysophosphatidylcholine in combination with antimicrobial agents against Acinetobacter baumannii in experimental murine peritoneal sepsis and pneumonia models. Antimicrob Agents Chemother. (2016) 60:4464–70. doi: 10.1128/AAC.02708-15
74. Yan JJ, Jung JS, Lee JE, Lee J, Huh SO, Kim HS, et al. Therapeutic effects of lysophosphatidylcholine in experimental sepsis. Nat Med. (2004) 10:161–7. doi: 10.1038/nm989
75. Hong CW, Kim TK, Ham HY, Nam JS, Kim YH, Zheng H, et al. Lysophosphatidylcholine increases neutrophil bactericidal activity by enhancement of azurophil granule-phagosome fusion via glycine.GlyR alpha 2/TRPM2/p38 MAPK signaling. J Immunol. (2010) 184:4401–13. doi: 10.4049/jimmunol.0902814
76. Miyazaki H, Midorikawa N, Fujimoto S, Miyoshi N, Yoshida H, Matsumoto T. Antimicrobial effects of lysophosphatidylcholine on methicillin-resistant Staphylococcus aureus. Ther Adv Infect Dis. (2017) 4:89–94. doi: 10.1177/2049936117714920
77. Stewart CJ, Mansbach JM, Wong MC, Ajami NJ, Petrosino JF, Camargo CAJr, et al. Associations of nasopharyngeal metabolome and microbiome with severity among infants with bronchiolitis. A multiomic analysis. Am J Respir Crit Care Med. (2017) 196:882–91. doi: 10.1164/rccm.201701-0071OC
78. Liu R, Cheng WJ, Tang HB, Zhong QP, Ming ZP, Dong HF. Comparative metabonomic investigations of Schistosoma japonicum from SCID mice and BALB/c mice: clues to developmental abnormality of schistosome in the immunodeficient host. Front Microbiol. (2019) 10:440. doi: 10.3389/fmicb.2019.00440
79. Maceyka M, Spiegel S. Sphingolipid metabolites in inflammatory disease. Nature. (2014) 510:58–67. doi: 10.1038/nature13475
80. Hoehn RS, Seitz AP, Jernigan PL, Gulbins E, Edwards MJ. Ischemia/reperfusion injury alters sphingolipid metabolism in the gut. Cell Physiol Biochem. (2016) 39:1262–70. doi: 10.1159/000447831
81. Schutsky K, Portocarrero C, Hooper DC, Dietzschold B, Faber M. Limited brain metabolism changes differentiate between the progression and clearance of rabies virus. PLoS ONE. (2014) 9:e87180. doi: 10.1371/journal.pone.0087180
82. Meadows JA, Wargo MJ. Carnitine in bacterial physiology and metabolism. Microbiology. (2015) 161:1161–74. doi: 10.1099/mic.0.000080
Keywords: Schistosoma japonicum, gut microbiome, metagenomics, metabolomics, 16S rRNA, UPLC-MS 3
Citation: Hu Y, Chen J, Xu Y, Zhou H, Huang P, Ma Y, Gao M, Cheng S, Zhou H and Lv Z (2020) Alterations of Gut Microbiome and Metabolite Profiling in Mice Infected by Schistosoma japonicum. Front. Immunol. 11:569727. doi: 10.3389/fimmu.2020.569727
Received: 04 June 2020; Accepted: 24 August 2020;
Published: 08 October 2020.
Edited by:
Jun-Hu Chen, National Institute of Parasitic Diseases (China), ChinaReviewed by:
Wanchai Maleewong, Khon Kaen University, ThailandGuillaume Sarrabayrouse, Vall d'Hebron Research Institute (VHIR), Spain
Jilong Shen, Anhui Medical University, China
Copyright © 2020 Hu, Chen, Xu, Zhou, Huang, Ma, Gao, Cheng, Zhou and Lv. This is an open-access article distributed under the terms of the Creative Commons Attribution License (CC BY). The use, distribution or reproduction in other forums is permitted, provided the original author(s) and the copyright owner(s) are credited and that the original publication in this journal is cited, in accordance with accepted academic practice. No use, distribution or reproduction is permitted which does not comply with these terms.
*Correspondence: Zhiyue Lv, lvzhiyue@mail.sysu.edu.cn
†These authors have contributed equally to this work