- 1School of Medicine, Duke University, Durham, NC, United States
- 2Division of Pediatric Rheumatology, Department of Pediatrics, Duke University, Durham, NC, United States
- 3Mayo Clinic, Rochester, MN, United States
Humans have an innate desire to observe and subsequently dissect an event into component pieces in an effort to better characterize the event. We then examine these pieces individually and in combinations using this information to determine the outcome of future similar events and the likelihood of their recurrence. Practically, this attempt to foretell an occurrence and predict its outcomes is evident in multiple disciplines ranging from meteorology to sociologic studies. In this manuscript we share the historical and present-day tools to predict course and outcome in juvenile idiopathic inflammatory myopathy including clinical features, testing, and biomarkers. Further we discuss considerations for building more complex predictive models of outcome especially in diseases such as juvenile idiopathic inflammatory myopathy where patients numbers are low. Many of the barriers to developing risk prediction models for juvenile idiopathic inflammatory myopathy outcomes have improved with many remaining challenges being addressed.
Humans have an innate desire to observe and subsequently dissect an event into component pieces in an effort to better characterize the event. We then examine these pieces individually and in combinations using this information to determine the outcome of future similar events and the likelihood of their recurrence. Practically, this attempt to foretell an occurrence and predict its outcomes is evident in multiple disciplines ranging from meteorology to sociologic studies. In medicine, the ancient Greek physicians, most notably Hippocrates and Asclepius, relied on examination and observation of patients to develop the art and science of diagnostic and prognostic medicine. The word prognosis (Greek: πρóγνωσις) translates into “knowledge beforehand,” how an event is likely to conclude (1). The ability to make a prognosis is a tenet and legacy of Hippocratic medicine. The study of biological systems evolved from defining the essential character of an observation or occurrence, natural philosophy, to using empiric methods for descriptions of how they occurred, the scientific method. The evolution of scientific capabilities led to the ability to look at these systems on a microscopic and molecular level, to attempt to understand the larger entity by breaking it into the smallest component pieces, known as reductionism. Advances in medical research have paralleled the advances in biologic research. Currently, we are in an era of system level observation of cellular networks where high-throughput technologies allow further resolution of these systems and generate complex data. Multivariate statistical methods are integral to the analysis of these biologic networks and are vital tools in the effort to discover biomarkers that are predictive of disease activity, severity, and response to therapeutic interventions.
Predictive and prognostic models are statistical tools that predict a clinical outcome determined by at least 2 points of patient data and ideally more with novel statistical models which take into account change over time (2, 3). Adequate prediction of prognostic endpoints generally requires multiple prognostic factors (variables, predictors, or markers) (2). Therefore, the original dataset may contain numerous covariates including clinical and biological markers identified as potential predictors of disease characteristics (phenotype/severity, or outcomes). Development is a process that includes identification of a relevant pool of predictors, formulation of a statistical model that may employ techniques such as linear regression, logistic regression, or Bayesian models, among others. Once a model is developed, it undergoes internal and external validation (2, 3). In the context of patient care, the goal is to develop a model that predicts an accurate diagnosis based on entered data. Treatment tailored to that disease has the potential to limit morbidity associated with inappropriate therapy (4). This is important in a disease with numerous clinical phenotypes such as the Juvenile Idiopathic Inflammatory Myopathies (JIIMs). A second combination of data utilizing a different statistical model may predict disease course or prognosis. Prognostic models have advanced from basic decision rules (prediction rules) used at the bedside to aid diagnostic and clinical decision making into complex mathematical formulas developed based on large population databases (3, 5).
The JIIMs represent a rare heterogeneous group of systemic autoimmune vasculopathies characterized by variable involvement of the skin and muscle primarily, but with the potential to affect multiple organs. The most common JIIM is juvenile dermatomyositis (JDM). Other JIIMs include juvenile polymyositis (JPM), immune-mediated necrotizing myositis, and myositis associated with connective tissue disease (6). Prediction of course and prognosis in JIIM has been difficult. In 1983, Bowyer et al. stated, “It has been impossible to predict at the onset of juvenile dermatomyositis whether a child will have complete recovery…” (7) More recently, van Dijkhuizen reporting for the Juvenile Dermatomyositis Research Group (JDRG) stated, “It is currently impossible to predict the prognosis of patients with JDM” (4). Bowyer's group sought to identify factors present early in the course of disease that might determine significant morbidity looking at clinical and treatment variables. Van Dijkhuizen's group, 35 years later, reports employing a Bayesian model of disease activity utilizing four continuous outcome variables to stratify patients by disease activity and allow for more sign/symptom-specific treatment based on these variables. Logically, predictors of disease course and prognosis have historically paralleled development of capabilities to characterize and examine disease manifestations, first by description of signs and symptoms, and later by immunohistochemistry and molecular techniques (8–16).
The years just prior to and since the new millennium have seen development, validation, and revision of measures of disease activity, severity, and outcome in the JIIMs, particularly JDM. Organizations instrumental in development of these measures include the International Myositis Assessment and Clinical Studies Group (IMACS) and the pediatric Rheumatology International Trials Organization (PRINTO). These measures assess various domains of the JIIMs including global disease activity, muscle strength, physical function, and quality of life (Table 1) (14). Core set measures (CSMs) have been developed and validated for assessment of disease and treatment variables in JDM (16, 18, 19). CSMs are the minimum set of measures, in aggregate, that allow for adequate assessment of the disease within the various domains studied, and are required for implementation in all clinical and therapeutic trials (20, 21). In order for measures to be useful in clinical care and research, definitions of disease improvement, severity, and response to therapy need to be available. For example, response to therapy being defined as at least a 20% improvement in three of six CSMs with no more than one or two worsening (which cannot be muscle strength) had been established as preliminary response criteria employed by both PRINTO and IMACS (20).
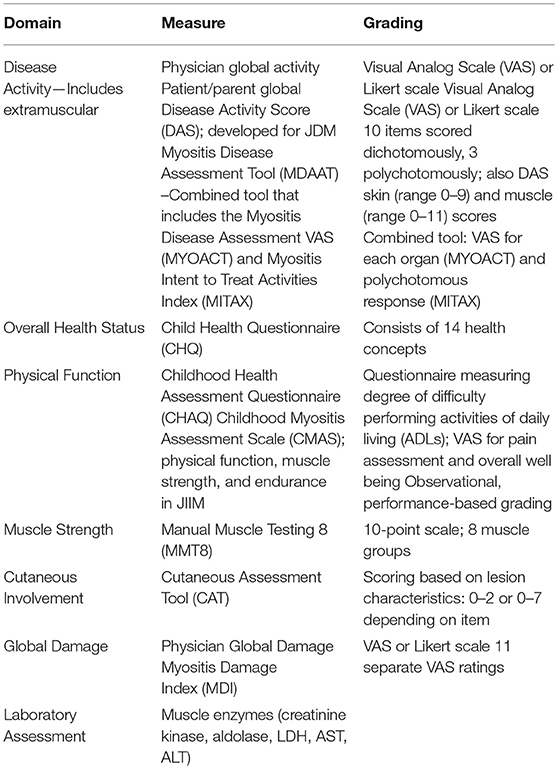
Table 1. Disease related measures used in predicting disease severity and outcome (17).
Predictive modeling utilizing clinical and laboratory data has been employed in JDM. Van Dijkhuizen's et al. utilized data from the UK Juvenile dermatomyositis cohort and biomarker study (JDCBS) in which data were analyzed using a Bayesian model to develop a model of disease activity (21). They identified signs and symptoms that associated with four outcome parameters. These parameters measured longitudinally included creatinine kinase (CK), childhood myositis assessment scale (CMAS), manual muscle testing 8 (MMT8), and physician global assessment (PGA). Among other associations, they discuss the association of periorbital rash with lower CMAS and higher CK values concluding this may support the opinion that ongoing skin disease reflects ongoing systemic disease activity (21). Deakin reporting for the Juvenile Dermatomyositis Research Group (JDRG) describes the use of marginal structural modeling (MSM) in determining the efficacy and safety of cyclophosphamide (CYC) treatment in severe JDM (22).
Marginal systems modeling (MSM), a statistical strategy utilizing multi-step estimation was employed by Lam et al. to adjust for baseline confounding bias to establish causal relationships using observational data between control and treatment groups in a cohort of JDM patients receiving IVIG (23). This study was significant in its application of bias-reduction methods to demonstrate the efficacy of IVIG in controlling JDM, most notably in corticosteroid-resistant patients. Deakin et al. also used MSM to determine the efficacy and safety of cyclophosphamide (CYC) in the treatment of severe JDM. The retrospective study consisted of 200 cases, 56 patients receiving, and 144 not receiving CYC (22). Descriptive analysis as well as MSM revealed improvements in three domains of disease activity (skin, muscle, and overall) in CYC-treated patients vs. those not treated. In the MSM analysis, the improvement was greatest at 12 months after the start of CYC in skin disease and global disease activity. Only minor adverse events were noted in three patients within 1 year of stopping CYC. In addition to efficacy and safety, the relative cost was lower and course of treatment was shorter using CYC as compared to biologic therapies.
Key factors for validation across universal cohorts is the ability to have comparable measures and outcomes. Work by CARRA, IMACS, PRINTO, cure JM, and JDRG all have worked to identify an international set of evaluations, measures for disease change and treatment protocols and place them into routine care of patients and not only in clinical trials (14, 16, 24–29).
In addition to clinical and laboratory measures, additional biomarkers are now being assessed as variables utilizing statistical methods to develop predictive and prognostic models.
Historical Biomarkers
Our ability to predict outcomes in JIIM continues to be limited by our subjective clinical assessments and our minimal and insensitive laboratory data. Exploration into biomarkers has been ongoing and continues to include cytokines, dysregulated inflammatory markers, autoantibodies, and muscle tissue markers. Individual biomarkers hold promise to be informative. However, it is more likely that these measures in concert hold a stronger association than they do individually, as demonstrated with disease modeling.
Over the past few decades, the development of new technologies, specifically high-throughput systems, has allowed identification of markers such as individual proteins, RNA immune related elements, and autoantibodies as markers of disease. Many of these same markers have been studied longitudinally and related to disease activity markers.
Transcriptional Analysis
RNA profiling of peripheral blood, muscle and skin biopsies in JIIM demonstrated similar patterns of the activation of the innate immune system with type 1 interferon (IFN1) induced, and the adaptive immune system with IL-17 and IL-6 pathway involvement, along with Th1 and Th2 related transcripts dysregulation (Figure 1) (30–33). The earliest differentially expressed transcripts reported in JIIM muscle tissue included an increase in IFN1 and Human Leucocyte Antigen (HLA) class I and II. The upregulation of HLA class I is now used in the disease diagnosis (31, 33–37). Chemokines related to both monocyte and lymphocyte immune function, such as CXCL9 [Monokine induced by gamma interferon (MIG)], CXCL10 (also known as IP-10, interferon gamma-induced proteins), and CXCL11 [Interferon-inducible T-cell alpha chemoattractant (I-TAC)], along with Calcium Binding Proteins such as S100A10, TNFSF13B (BAFF), ISG15 an ubiquitin-like modifier, are all reported dysregulated in JIIM (30, 38–40). An early study demonstrated that a cluster of IFN-regulated transcripts are upregulated in most JIIM patients and consist of classical IFN-induced genes including STAT1, SOCS1, the myxovirus resistance genes MX1 and MX2, the oligoadenylate synthetase transcripts OAS1, OAS2, and OAS3, Fcγ receptor FCGR1A, the interleukin 1 receptor antagonist (IL1RN), the pro-apoptotic TNFSF1 0/TRAIL, and another inflammatory chemokine CXCL10/IP-10 (30, 37).
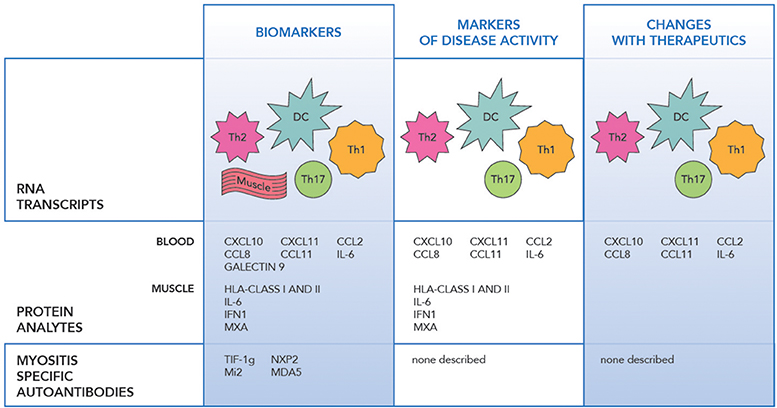
Figure 1. The figure illustrates the transcript, protein and antibody biomarkers in juvenile idiopathic myopathy. RNA Transcripts include DC dendritic cells (IFNα/β, OAS1, 2), Th1 (IL-1, CXCR3, FCGR1A), Th2 (IL-4, IL-13, and GATA3), Th17 (IL-6, IL17D, IL-17F, IL-21, IL-23A, IL-27, RORC/RORγt, and IRF4), Monocytes (CXCL9, CXCL10, CXCL11), Muscle (HLA class I and II, MX1 and MX2, MxA, SGF-15, RORc, STAT3, cytochrome C oxidase, and NADH dehydrogenase), IFNβ, IRF7 (24–34).
Non-immune genes are also differentially expressed and include transcripts related to the oxidative pathways and mitochondrial function such as cytochrome C oxidase and NADH dehydrogenase (30, 33).
Not only have these transcripts been reported as dysregulated in JIIM, but they have also been reported to correlate with disease activity measures. Many individual transcripts were statistically significant. However, as with other disorders such as Systemic Lupus and JIIM, Baechler et al found combinations of interferon related transcripts specifically built as interferon scores appeared to be more reliable and withstand longitudinal disease variability including treatment (30, 41, 42). Further refinement of the IFN gene signature in JIIM was determined using the expression levels of 3 IFN-regulated genes (IFIT1, G1P2, and IRF7) using quantitative real-time reverse transcription-polymerase chain reaction and normalization to obtain an IFN score (41). This was later verified and used in adult myositis (40). This also led to the development of an IFN protein score with proteins found to be associated with disease activity. (MCP-2, CXCL10, and CXCL11) (25, 36).
Protein Markers
Just as microarray technologies have allowed identification of new biomarkers, advances in proteomics have allowed identification of protein changes with disease states and interventions. A variety of proteins, including levels of IFN regulated proteins included anti-viral proteins, humoral and adaptive immune proteins, and chemokines are seen in the peripheral blood, in specific cell subsets and in the muscle tissue in JIIM. Specific analytes include IFN alpha and beta, IL-6, IL-17, chemokines including MCP-1/CCL2, MCP-2/CCL8, IP-10/CXCL10, I-TAC/CXCL11, IFNγ, Galectin 9, IL-1Ra, GM-CSF, and Eotaxin (Figure 1) (41, 43–46). Markers including those related to IFN1 upregulation along with IL-6, CCL11, MCP-1, CXCL11, and CXCL10 appear to hold the strongest correlation with disease activity in JIIM (37, 41, 43, 45, 47, 48).
Many of these proinflammatory cytokines induce or enhance the metabolic effects on muscle tissue, especially during the regeneration process (49). Cytokines such as type-1 interferon, IL-1, and TNFa are upregulated in myocytes along with the increased expression of HLA-class I. In addition, with myocyte regeneration, seen in IIM, there also is upregulation of HLA-class I along with type-1 IFN and IL-6, which could lead to further inflammation (44, 50–53).
Tissue-specific markers, such as those related to atrophic myofibers, include the ISG15-conjugation pathway proteins such as MxA, which are upregulated in active disease. Several IFN-regulated chemokines showed significant positive correlations with muscle enzymes, including MCP-1, MCP-2, and CXCL10. Baechler (30) and Bilgic (41) demonstrated that JIIM with the highest degree of disease activity had elevation of IFN- regulated proteins CXCL10, MCP-1, and MCP-2. Similar to RNA transcripts, scores using multiple transcripts and multiple protein analytes combined together created a stronger association with disease activity measures (41). The set of JIIM markers indicate the intricate matrix and interconnectedness of the innate, humoral and adaptive immune systems in autoimmunity.
Autoantibodies
Identification of a myositis specific autoantibody (MSAs) is highly suggestive of an inflammatory myositis and more recently recognized in JIIM. This has led to autoantibody relationships with clinical disease phenotypes, and possibly antibody levels themselves fluctuate with disease activity. Those more commonly seen in JIIM include anti-TIF 1(Transcriptional intermediary factor 1), NXP2 (Nuclear Matric protein 2), MDA5 (Melanoma differentiation-associated gene 5), Mi2 (Nucleosome-remodeling deacetylase complex), and less commonly anti-SAE, ASA (synthetase), SRP (Signal recognition particle and HMGCR (3-hydroy-3-methlglutaryl coenzyme A reductase (54–56).
Myositis-specific antibodies have an increasing utility as both diagnostic and prognostic biomarkers with multiple publications suggesting clinical features related to antibody specificity (54–56) (Figure 1). Not only are MSAs markers of the clinical phenotype, but they have also been investigated as disease activity markers. Myositis-associated antibody levels for anti-Jo-1 TIF1-γ, SRP, and -Mi-2 were investigated after B cell depletion in adult and pediatric DM and adult PM and correlated with disease activity. Anti-Jo-1 serum levels correlated with clinical improvement specifically MMT and muscle enzymes (p = 0.007) (17). In DM patients who had anti-CADM-140/MDA5 autoantibodies and rapidly progressive interstitial lung disease, the mean titer of anti-CADM-140/MDA5 of anti-CADM-140/MDA5 significantly decreased in the responder group compared to non-responders (P = 0.033) (57).
In the future, predicting disease response to treatment as well as disease- and treatment-related outcomes will require classification of myositis patients in more homologous groups than the traditional PM and DM subtypes.
Predicting Disease Outcomes in JIIM
While risk prediction models have recently become commonplace in the medical literature, there have been few attempts to develop risk prediction models in patients with JIIM. This is likely due to the rarity of the disease, as a minimum sample size of 200 patients is preferred for risk prediction models. In addition, the heterogeneity of disease activity and therapeutic strategies in patients with JIIM has made risk prediction difficult in this patient population. For example, a small observational study of 39 patients with JIIM, among whom six achieved clinical remission, found that female sex, negative Gower's sign and photosensitivity were associated with achievement of complete remission, but the small sample size precluded development of a multivariable model that could be used for prediction purposes (58).
Cooperative efforts including registries and biobanks are now making this task more feasible (59). Challa et al. recently published a model to predict changes in disease activity among children with JIIM using the Childhood Arthritis and Rheumatology Research Alliance (CARRA) Legacy Registry (60). They found that anti-nuclear antibody (ANA) positivity and use of hydroxychloroquine predicted improvement in patient/parent global health score over 6 months, and ANA positivity along with V/shawl sign predicted improvement in patient pain.
The Rituximab in Myositis (RIM) trial also provided opportunities to develop risk prediction models in patients with refractory disease. Aggarwal et al. (45) found that the presence of anti-synthetase and anti-Mi-2 autoantibodies and lower disease damage strongly predicted clinical improvement in these patients, and that the juvenile patients had better prognosis than the adults (61). Reed et al. found that biomarker signatures involving type-1 interferon regulated and other proinflammatory chemokines and cytokines in conjunction with autoantibodies predicted response to rituximab in patients with refractory myositis (45). Furthermore, Olazagasti et al. found that adding gene expression, cytokine and chemokine data to clinical and standard laboratory assessments improved prediction of response to rituximab in patients with JIIM (62).
Practical Considerations for Building Predictive Models in JIIM
In addition to the importance of a sufficient sample size, there are several other important considerations for building risk prediction models. First, the study design and population of interest should be considered. As mentioned above, registries are making risk prediction in JIIM more feasible. While registries are observational studies, which may suffer from confounding, they can be used to predict outcomes from baseline characteristics. However, confounding by indication is common, as patients with more severe disease often require more therapies and interventions. This confounding can make it difficult to assess the impact of treatment on the outcome of interest. Alternatives to address confounding my indication are causal inference analysis methods (e.g., MSM) or randomized clinical trials. By randomizing patients to receive a treatment, confounding by indication is eliminated. However, clinical trials also have limitations because they often have strict inclusion criteria. Thus, patients enrolled in clinical trials may not represent the population of interest, as some of the patients for whom the risk prediction model could be useful were excluded from the trial.
Risk factor selection is another important consideration when developing a risk prediction model. The existence and assessment of relevant disease activity measures and biomarkers, such as those mentioned previously in this review, is critical. Without reliable disease activity measures, it is not possible to build models that will predict disease-related outcomes. In addition, causality is important, because when factors included in a risk prediction model improve, it is assumed that the risk of the outcome will be reduced. However, proving causality is difficult and requires causal inference analysis methods or a randomized clinical trial. Finally, the practicality and cost of each potential risk factor needs to be considered. Measurements that are financially costly or those that require a great deal of effort to assess, such as cumulative measures, provide challenges for implementing a risk prediction model in clinical practice.
The choice of model is also an important consideration. Historically, logistic regression models for cross-sectional data and Cox regression models for longitudinal time-to-event data were commonly used for risk prediction. These models had the advantage of providing an easily understandable equation for predicting the risk of an outcome. However, they were often overly simplistic and only considered linear effects of the continuous risk factors. More recent advances in statistical methods, which evolved from computer science methodology, including machine-learning techniques, have demonstrated improved performance for risk prediction models resulting from complex algorithms that do not provide simple risk calculation formulas. Computing advances have made it much easier to implement these complex algorithms into clinical practice using web-based tools, so simple formulas are no longer required and has opened the possibility of novel algorithms to apply to complex biomedical datasets.
Finally, once the risk prediction model has been developed, it is important to objectively assess its performance. Model performance assessment should include both discrimination and calibration. Discrimination is the ability to correctly rank patients from low to high risk. Calibration is the ability to accurately predict the absolute risk level. There is a wealth of literature on how to assess model performance for different types of models, which is beyond the scope of this review.
Risk prediction models should be validated prior to use in clinical decision-making. External validation in a separate dataset is preferred. However, internal validation based on subdividing the study patients into training and test dataset is also common. Szodoray et al. randomly subdivided their patients into 50 cases and 50 controls for training and 29 cases and 20 controls for testing (44). Analyzing multiplex cytokine assays using principal components, hierarchical clustering and discriminant function analyses, they identified unique immune profiles that seem to perpetuate autoimmune processed in patients with JIIM and may be able to identify disease subsets. Many of the modern modeling techniques use cross-validation methods to avoid over-optimism or over-fitting of a risk prediction model. None of the previously mentioned risk prediction models for JIIM have been validated due to study sample sizes that were too small to subdivide and a lack of available external data sources for validation.
In conclusion, while many of the barriers to developing risk prediction models for JIIM outcomes have improved, there are still many remaining challenges. The availability of registries to provide larger sample sizes and newer biomarkers that can predict outcomes has reduced the challenges, but careful planning will still be required to navigate these challenges and ultimately develop useful risk prediction models for patients with JIIM. However, there continues to be a major need to compare across data sets and cohorts that require continued standardization and validation across cohorts and centers.
Author Contributions
All authors listed have made a substantial, direct and intellectual contribution to the work, and approved it for publication.
Conflict of Interest Statement
The authors declare that the research was conducted in the absence of any commercial or financial relationships that could be construed as a potential conflict of interest.
References
1. Kleisiaris CF, Sfakianakis C, Papathanasiou IV. Health care practices in ancient Greece: the hippocratic ideal. J Med Ethics Hist Med. (2014) 7:6.
2. Steyerberg EW, Moons KG, van der Windt DA, Hayden JA, Perel P, Schroter S, et al. Prognosis Research Strategy (PROGRESS) 3: prognostic model research. PLoS Med. (2009) 10:e1001381. doi: 10.1371/journal.pmed.1001381
3. Vogenberg FR. Predictive and prognostic models: implications for healthcare decision-making in a modern recession. Am Health Drug Benefits. (2009) 2:218–22.
4. van Dijkhuizen EP, Deakin CT, Wedderburn LR, De Iorio M. Modelling disease activity in juvenile dermatomyositis: a Bayesian approach. Stat Methods Med Res. (2019) 28:35–49. doi: 10.1177/0962280217713233
5. Stiell IG, Wells GA. Methodologic standards for the development of clinical decision rules in emergency medicine. Ann Emerg Med. (1998) 5:437–47.
6. Huber AM. Juvenile idiopathic inflammatory myopathies. Pediatr Clin North Am. (2018) 65:739–56. doi: 10.1016/j.pcl.2018.04.006
7. Bowyer SL, Blane CE, Sullivan DB, Cassidy JT. Childhood dermatomyositis: factors predicting functional outcome and development of dystrophic calcification. J Pediatrics. (1983) 103:882–8. doi: 10.1016/S0022-3476(83)80706-9
8. Rider LG, Gurley RC, Pandey JP, Garcia de la Torre I, Kalovidouris AE, O'Hanlon TP, et al. Clinical, serologic, and immunogenetic features of familial idiopathic inflammatory myopathy. Arthritis Rheum. (1998) 41:710–9. doi: 10.1002/1529-0131(199804)41:4<710::AID-ART19>3.0.CO;2-K
9. Arahata K, Engel AG. Monoclonal antibody analysis of mononuclear cells in myopathies. I: quantitation of subsets according to diagnosis and sites of accumulation and demonstration and counts of muscle fibers invaded by T cells. Ann Neurol. (1984) 16:193–208. doi: 10.1002/ana.410160206
10. Lovell DJ, Lindsley CB, Rennebohm RM, Ballinger SH, Bowyer SL, Giannini EH, et al. Development of validated disease activity and damage indices for the juvenile idiopathic inflammatory myopathies. II. The Childhood Myositis Assessment Scale (CMAS): a quantitative tool for the evaluation of muscle function. The Juvenile Dermatomyositis Disease Activity Collaborative Study Group. Arthritis Rheum. (1999) 42:2213–9. doi: 10.1002/1529-0131(199910)42:10<2213::AID-ANR25>3.0.CO;2-8
11. Huber AM, Hicks JE, Lachenbruch PA, Perez MD, Zemel LS, Rennebohm RM, et al. Validation of the Childhood Health Assessment Questionnaire in the juvenile idiopathic myopathies. Juvenile Dermatomyositis Disease Activity Collaborative Study Group. J Rheumatol. (2001) 28:1106–11.
12. Rider LG, Feldman BM, Perez MD, Rennebohm RM, Lindsley CB, Zemel LS, et al. Development of validated disease activity and damage indices for the juvenile idiopathic inflammatory myopathies: I. Physician, parent, and patient global assessments. Juvenile Dermatomyositis Disease Activity Collaborative Study Group. Arthritis Rheum. (1997) 40:1976–83. doi: 10.1002/art.1780401109
13. Rider LG, Koziol D, Giannini EH, Jain MS, Smith MR, Whitney-Mahoney K, et al. Validation of manual muscle testing and a subset of eight muscles for adult and juvenile idiopathic inflammatory myopathies. Arthritis Care Res. (2010) 62:465–72. doi: 10.1002/acr.20035
14. Rider LG, Werth VP, Huber AM, Alexanderson H, Rao AP, Ruperto N, et al. Measures of adult and juvenile dermatomyositis, polymyositis, and inclusion body myositis. Arthritis Care Res. (2011) 63(S11):S118–57. doi: 10.1002/acr.20532
15. Varnier GC, Rosina S, Ferrari C, Pistorio A, Consolaro A, Bovis F, et al. Development and testing of a hybrid measure of muscle strength in juvenile dermatomyositis in routine care. Arthritis Care Res. (2018) 70:1312–9. doi: 10.1002/acr.23491
16. McCann LJ, Pilkington CA, Huber AM, Ravelli A, Appelbe D, Kirkham JJ, et al. Development of a consensus core dataset in juvenile dermatomyositis for clinical use to inform research. Ann Rheum Dis. (2018) 77:241–50. doi: 10.1136/annrheumdis-2017-212141
17. Aggarwal R, Oddis CV, Goudeau D, Koontz D, Qi Z, Reed AM, et al. Autoantibody levels in myositis patients correlate with clinical response during B cell depletion with rituximab. Rheumatology. (2016) 55:1710. doi: 10.1093/rheumatology/kew275
18. McCann LJ, Kirkham JJ, Wedderburn LR, Pilkington C, Huber AM, Ravelli A, et al. Development of an internationally agreed minimal dataset for juvenile dermatomyositis (JDM) for clinical and research use. Trials. (2015) 16:268. doi: 10.1186/s13063-015-0784-0
19. Ruperto N, Ravelli A, Murray KJ, Lovell DJ, Andersson-Gare B, Feldman BM, et al. Preliminary core sets of measures for disease activity and damage assessment in juvenile systemic lupus erythematosus and juvenile dermatomyositis. Rheumatology. (2003) 42:1452–9. doi: 10.1093/rheumatology/keg403
20. Rider LG, Aggarwal R, Pistorio A, Bayat N, Erman B, Feldman BM, et al. 2016 American College of Rheumatology/European League against rheumatism criteria for minimal, moderate, and major clinical response in juvenile dermatomyositis: an International Myositis Assessment and Clinical Studies Group/Paediatric Rheumatology International Trials Organisation Collaborative Initiative. Arthritis Rheumatol. (2017) 69:911–23. doi: 10.1002/art.40060
21. van Dijkhuizen EHP, De Iorio M, Wedderburn LR, Deakin CT, JDRG. Clinical signs and symptoms in a joint model of four disease activity parameters in juvenile dermatomyositis: a prospective, longitudinal, multicenter cohort study. Arthritis Res Ther. (2018) 20:180. doi: 10.1186/s13075-018-1687-8
22. Deakin CT, Campanilho-Marques R, Simou S, Moraitis E, Wedderburn LR, Pullenayegum E, et al. Efficacy and safety of cyclophosphamide treatment in severe juvenile dermatomyositis shown by marginal structural modeling. Arthritis Rheumatol. (2018) 70:785–93. doi: 10.1002/art.40418
23. Lam CG, Manlhiot C, Pullenayegum EM, Feldman BM. Eficacy of Ig therapy in juvenile dermatomyositis. Ann Rheum Dis. (2011) 70:2089–94. doi: 10.1136/ard.2011.153718
24. Stringer E, Bohnsack J, Bowyer SL, Griffin TA, Huber AM, Lang B, et al. Treatment approaches to juvenile dermatomyositis (JDM) across North America: the Childhood Arthritis and Rheumatology Research Alliance (CARRA) JDM Treatment Survey. J Rheumatol. (2010) 37:1953–61. doi: 10.3899/jrheum.090953
25. Huber AM, Lang B, LeBlanc CM, Birdi N, Bolaria RK, Malleson P, et al. Medium- and long-term functional outcomes in a multicenter cohort of children with juvenile dermatomyositis. Arthritis Rheum. (2000) 43:541–9. doi: 10.1002/1529-0131(200003)43:3<541::AID-ANR9>3.0.CO;2-T
26. Huber AM, Robinson AB, Reed AM, Abramson L, Bout-Tabaku S, Carrasco R, et al. Consensus treatments for moderate juvenile dermatomyositis: beyond the first two months. Results of the second Childhood Arthritis and Rheumatology Research Alliance consensus conference. Arthritis Care Res. (2012) 64:546–53. doi: 10.1002/acr.20695
27. Huber AM, Kim S, Reed AM, Carrasco R, Feldman BM, Hong SD, et al. Childhood Arthritis and Rheumatology Research Alliance Consensus Clinical Treatment Plans for Juvenile Dermatomyositis with Persistent Skin Rash. J Rheumatol. (2017) 44:110–6. doi: 10.3899/jrheum.160688
28. Kim S, Kahn P, Robinson AB, Lang B, Shulman A, Oberle EJ, et al. Childhood Arthritis and Rheumatology Research Alliance consensus clinical treatment plans for juvenile dermatomyositis with skin predominant disease. Pediatr Rheumatol Online J. (2017) 15:1. doi: 10.1186/s12969-016-0134-0
29. Isenberg DA, Allen E, Farewell V, Ehrenstein MR, Hanna MG, Lundberg IE, et al. International consensus outcome measures for patients with idiopathic inflammatory myopathies. Development and initial validation of myositis activity and damage indices in patients with adult onset disease. Rheumatology. (2004) 43:49–54. doi: 10.1093/rheumatology/keg427
30. Baechler EC, Bauer JW, Slattery CA, Ortmann WA, Espe KJ, Novitzke J, et al. An interferon signature in the peripheral blood of dermatomyositis patients is associated with disease activity. Mol Med. (2007) 13:59–68. doi: 10.2119/2006-00085.Baechler
31. Liao AP, Salajegheh M, Nazareno R, Kagan JC, Jubin RG, Greenberg SA. Interferon beta is associated with type 1 interferon-inducible gene expression in dermatomyositis. Ann Rheum Dis. (2011) 70:831–6. doi: 10.1136/ard.2010.139949
32. López De Padilla CM, Crowson CS, Hein MS, Pendegraft RS, Strausbauch MA, Niewold TB, et al. Gene expression profiling in blood and affected muscle tissues reveals differential activation pathways in patients with new-onset juvenile and adult dermatomyositis. J Rheumatol. (2017) 44:117–24. doi: 10.3899/jrheum.160293
33. Tezak Z, Hoffman EP, Lutz JL, Fedczyna TO, Stephan D, Bremer EG, et al. Gene expression profiling in DQA1*0501+ children with untreated dermatomyositis: a novel model of pathogenesis. J Immunol. (2002) 168:4154–63. doi: 10.4049/jimmunol.168.8.4154
34. Walsh RJ, Kong SW, Yao Y, Jallal B, Kiener PA, Pinkus JL, et al. Type I interferon-inducible gene expression in blood is present and reflects disease activity in dermatomyositis and polymyositis. Arthritis Rheum. (2007) 56:3784–92. doi: 10.1002/art.22928
35. Chen YW, Shi R, Geraci N, Shrestha S, Gordish-Dressman H, Pachman LM. Duration of chronic inflammation alters gene expression in muscle from untreated girls with juvenile dermatomyositis. BMC Immunol. (2008) 9:43. doi: 10.1186/1471-2172-9-43
36. Greenberg SA, Bradshaw EM, Pinkus JL, Pinkus GS, Burleson T, Due B, et al. Plasma cells in muscle in inclusion body myositis and polymyositis. Neurology. (2005) 65:1782–7. doi: 10.1212/01.wnl.0000187124.92826.20
37. Greenberg SA, Higgs BW, Morehouse C, Walsh RJ, Kong SW, Brohawn P, et al. Relationship between disease activity and type 1 interferon- and other cytokine-inducible gene expression in blood in dermatomyositis and polymyositis. Genes Immun. (2012) 13:207–13. doi: 10.1038/gene.2011.61
38. Suárez-Calvet X, Gallardo E, Nogales-Gadea G, Querol L, Navas M, Díaz-Manera J, et al. Altered RIG-I/DDX58-mediated innate immunity in dermatomyositis. J Pathol. (2014) 233:258–68. doi: 10.1002/path.4346
39. Zhou X, Dimachkie MM, Xiong M, Tan FK, Arnett FC. cDNA microarrays reveal distinct gene expression clusters in idiopathic inflammatory myopathies. Med Sci Monit. (2004) 10:BR191–7.
40. Salajegheh M, Kong SW, Pinkus JL, Walsh RJ, Liao A, Nazareno R, et al. Interferon-stimulated gene 15 (ISG15) conjugates proteins in dermatomyositis muscle with perifascicular atrophy. Ann Neurol. (2010) 67:53–63. doi: 10.1002/ana.21805
41. Bilgic H, Ytterberg SR, Amin S, McNallan KT, Wilson JC, Koeuth T, et al. Interleukin-6 and type I interferon-regulated genes and chemokines mark disease activity in dermatomyositis. Arthritis Rheum. (2009) 60:3436–46. doi: 10.1002/art.24936
42. Petri M, Singh S, Tesfasyone H, Dedrick R, Fry K, Lal P, et al. Longitudinal expression of type I interferon responsive genes in systemic lupus erythematosus. Lupus. (2009) 18:980–9. doi: 10.1177/0961203309105529
43. Sanner H, Schwartz T, Flatø B, Vistnes M, Christensen G, Sjaastad I. Increased levels of eotaxin and MCP-1 in juvenile dermatomyositis median 16.8 years after disease onset; associations with disease activity, duration and organ damage. PLoS ONE. (2014) 9:e92171. doi: 10.1371/journal.pone.0092171
44. Szodoray P, Alex P, Knowlton N, Centola M, Dozmorov I, Csipo I, et al. Idiopathic inflammatory myopathies, signified by distinctive peripheral cytokines, chemokines and the TNF family members B-cell activating factor and a proliferation inducing ligand. Rheumatology. (2010) 49:1867–77. doi: 10.1093/rheumatology/keq151
45. Reed AM, Crowson CS, Hein M, de Padilla CL, Olazagasti JM, Aggarwal R, et al. Biologic predictors of clinical improvement in rituximab-treated refractory myositis. BMC Musculoskelet Disord. (2015) 16:257. doi: 10.1186/s12891-015-0710-3
46. Bellutti Enders F, van Wijk F, Scholman R, Hofer M, Prakken BJ, van Royen-Kerkhof A, et al. Correlation of CXCL10, tumor necrosis factor receptor type II, and galectin 9 with disease activity in juvenile dermatomyositis. Arthritis Rheumatol. (2014) 66:2281–9. doi: 10.1002/art.38676
47. Gao S, Luo H, Zhang H, Zuo X, Wang L, Zhu H. Using multi-omics methods to understand dermatomyositis/polymyositis. Autoimmun Rev. (2017) 16:1044–8. doi: 10.1016/j.autrev.2017.07.021
48. López De Padilla CM, Crowson CS, Hein MS, Strausbauch MA, Aggarwal R, Levesque MC, et al. Interferon-regulated chemokine score associated with improvement in disease activity in refractory myositis patients treated with rituximab. Clin Exp Rheumatol. (2015) 33:655–63.
49. Nagaraju K. Role of major histocompatibility complex class I molecules in autoimmune myositis. Curr Opin Rheumatol. (2005) 17:725–30. doi: 10.1097/01.bor.0000179947.58271.9a
50. Malm C, Nyberg P, Engstrom M, Sjodin B, Lenkei R, Ekblom B, et al. Immunological changes in human skeletal muscle and blood after eccentric exercise and multiple biopsies. J Physiol. (2000) 529(Pt 1):243–62. doi: 10.1111/j.1469-7793.2000.00243.x
51. Tournadre A, Miossec P. A critical role for immature muscle precursors in myositis. Nat Rev Rheumatol. (2013) 9:438–42. doi: 10.1038/nrrheum.2013.26
52. Kuru S, Inukai A, Kato T, Liang Y, Kimura S, Sobue G. Expression of tumor necrosis factor-alpha in regenerating muscle fibers in inflammatory and non-inflammatory myopathies. Acta Neuropathol. (2003) 105:217–24. doi: 10.1007/s00401-002-0635-4
53. Reed A, Hein MS, Colbert RA. UPR activation and IFNb production in myocytes in adult and juvenile myositis. Arthritis Rheum. (2010) 62(S10):1376.
54. Betteridge ZE, Gunawardena H, McHugh NJ. Novel autoantibodies and clinical phenotypes in adult and juvenile myositis. Arthritis Res Ther. (2011) 13:209. doi: 10.1186/ar3275
55. Betteridge Z, McHugh N. Myositis-specific autoantibodies: an important tool to support diagnosis of myositis. J Intern Med. (2016) 280:8–23. doi: 10.1111/joim.12451
56. Tansley SL, Simou S, Shaddick G, Betteridge ZE, Almeida B, Gunawardena H, et al. Autoantibodies in juvenile-onset myositis: their diagnostic value and associated clinical phenotype in a large UK cohort. J Autoimmun. (2017) 84:55–64. doi: 10.1016/j.jaut.2017.06.007
57. Sato S, Kuwana M, Fujita T, Suzuki Y. Anti-CADM-140/MDA5 autoantibody titer correlates with disease activity and predicts disease outcome in patients with dermatomyositis and rapidly progressive interstitial lung disease. Mod Rheumatol. (2013) 23:496–502. doi: 10.3109/s10165-012-0663-4
58. Sun C, Lee JH, Yang YH, Yu HH, Wang LC, Lin YT, et al. Juvenile dermatomyositis: a 20-year retrospective analysis of treatment and clinical outcomes. Pediatr Neonatol. (2015) 56:31–9. doi: 10.1016/j.pedneo.2014.02.006
59. Wu Q, Wedderburn LR, McCann LJ. Juvenile dermatomyositis: latest advances. Best Pract Res Clin Rheumatol. (2017) 31:535–57. doi: 10.1016/j.berh.2017.12.003
60. Challa D, Crowson CS, Niewold TB, Reed AM, CARRA Legacy Registry Investigators. Predictors of changes in disease activity among children with juvenile dermatomyositis enrolled in the Childhood Arthritis and Rheumatology Research Alliance (CARRA) Legacy Registry. Clin Rheumatol. (2018) 37:1011–5. doi: 10.1007/s10067-017-3901-5
61. Aggarwal R, Bandos A, Reed AM, Ascherman DP, Barohn RJ, Feldman BM, et al. Predictors of clinical improvement in rituximab-treated refractory adult and juvenile dermatomyositis and adult polymyositis. Arthritis Rheumatol. (2014) 66:740–9. doi: 10.1002/art.38270
62. Olazagasti J, Crowson C, Hein M, de Padilla C, Aggarwal R, Oddis C, et al. Predictive models of clinical improvement in rituximab-treated myositis patients using clinical features, autoantibodies, and biomarkers. predictive models of clinical improvement in rituximab-treated myositis patients using clinical features, autoantibodies, and biomarkers. Open J Rheumatol Autoimmune Dis. (2015) 5:68–80. doi: 10.4236/ojra.2015.53012
Keywords: juvenile myositis/deratomyositis, outcomes, myositis, predictive model, biomarkers
Citation: Reed AM, Crowson CS and Dvergsten JA (2019) A Path to Prediction of Outcomes in Juvenile Idiopathic Inflammatory Myopathy. Front. Immunol. 10:638. doi: 10.3389/fimmu.2019.00638
Received: 06 November 2018; Accepted: 08 March 2019;
Published: 02 April 2019.
Edited by:
Massimo Gadina, National Institute of Arthritis and Musculoskeletal and Skin Diseases (NIAMS), United StatesReviewed by:
Iago Pinal-Fernandez, National Institutes of Health (NIH), United StatesLucy R. Wedderburn, Great Ormond Street Institute of Child Health, University College London, United Kingdom
Copyright © 2019 Reed, Crowson and Dvergsten.This is an open-access article distributed under the terms of the Creative Commons Attribution License (CC BY). The use, distribution or reproduction in other forums is permitted, provided the original author(s) and the copyright owner(s) are credited and that the original publication in this journal is cited, in accordance with accepted academic practice. No use, distribution or reproduction is permitted which does not comply with these terms.
*Correspondence: Ann Marie Reed, YW5uLnJlZWRAZHVrZS5lZHU=