- 1Department of Microbiology, Kindai University Faculty of Medicine, Osakasayama, Japan
- 2Department of Microbiology and Immunology, Louisiana State University Health Sciences Center-Shreveport, Shreveport, LA, United States
- 3Department of Pathology, University of Utah, Salt Lake City, UT, United States
- 4Department of Computer Science, Louisiana State University Shreveport, Shreveport, LA, United States
- 5Department of Neurology, Louisiana State University Health Sciences Center-Shreveport, Shreveport, LA, United States
- 6Department of Molecular and Cellular Physiology, Louisiana State University Health Sciences Center-Shreveport, Shreveport, LA, United States
Previously, we have established two distinct progressive multiple sclerosis (MS) models by induction of experimental autoimmune encephalomyelitis (EAE) with myelin oligodendrocyte glycoprotein (MOG) in two mouse strains. A.SW mice develop ataxia with antibody deposition, but no T cell infiltration, in the central nervous system (CNS), while SJL/J mice develop paralysis with CNS T cell infiltration. In this study, we determined biomarkers contributing to the homogeneity and heterogeneity of two models. Using the CNS and spleen microarray transcriptome and cytokine data, we conducted computational analyses. We identified up-regulation of immune-related genes, including immunoglobulins, in the CNS of both models. Pro-inflammatory cytokines, interferon (IFN)-γ and interleukin (IL)-17, were associated with the disease progression in SJL/J mice, while the expression of both cytokines was detected only at the EAE onset in A.SW mice. Principal component analysis (PCA) of CNS transcriptome data demonstrated that down-regulation of prolactin may reflect disease progression. Pattern matching analysis of spleen transcriptome with CNS PCA identified 333 splenic surrogate markers, including Stfa2l1, which reflected the changes in the CNS. Among them, we found that two genes (PER1/MIR6883 and FKBP5) and one gene (SLC16A1/MCT1) were also significantly up-regulated and down-regulated, respectively, in human MS peripheral blood, using data mining.
Introduction
Multiple sclerosis (MS) is an inflammatory demyelinating disease of the central nervous system (CNS) (1). World-wide, MS affects about 2.5 million people (2). Although the precise etiology of MS remains unclear, MS has been proposed to be a disease caused by interactions between autoimmunity, microbial infections, and/or genetic factors (3). The clinical courses of MS are classified into four types: (1) clinically isolated syndrome (CIS), (2) relapsing-remitting (RR), (3) primary progressive (PP), (4) secondary progressive (SP) (4). CIS is a first clinical episode with CNS inflammation and demyelination (5). RR-MS is defined by “relapses” (disease attacks) with periods of “remission” (recovery) and is the most frequent occurring. SP-MS is defined by an initial RR disease course followed by continuous disease progression. Approximately 95% of RR-MS patients develop SP-MS (6). PP-MS progresses continuously from the onset without recovery. There is no biomarker that can be used to classify or predict clinical courses of the four subtypes of MS (7).
Neuroimaging studies suggest that MS lesions shifted from inflammatory demyelination to neurodegeneration during the disease progression (8, 9). In contrast, neuropathology studies suggest that the pathogenesis of MS remained the same throughout the course (10, 11). There was neither a definite mechanistic explanation of how the pathogenesis shifts from inflammatory demyelination to neurodegeneration in all MS cases, nor an explanation of whether the two conflicting views based on neuroimaging or neuropathology observations can be reconciled. The different views on disease pathogenesis in MS could be attributed to the fact that each view is based on one aspect of the disease: neuroimaging or histological changes. Alternatively, MS pathogenesis might differ among individual patients (12). The neuropathological view might be based on MS patients whose effector mechanism remains the same during the disease course, while the neuroimaging view could be based on the patient subgroup whose effector mechanism changes during the disease course. We hypothesized that inconsistencies of effectiveness of treatment, neuroimaging and neuropathology among progressive MS patients could be heterogeneities of the pathogenesis of MS.
Clinical courses of animal models for MS are also variable. Experimental autoimmune encephalomyelitis (EAE) can be induced by sensitization with CNS antigens, including myelin basic protein (MBP), myelin proteolipid protein (PLP), and myelin oligodendrocyte glycoprotein (MOG) (3, 13). The clinical course of EAE can be RR, PP, and SP, which are similar to the various forms of MS: RR-MS, PP-MS, and SP-MS, respectively. Several EAE models with different clinical courses have been established: RR-course in SJL/J mice with PLP139−151, PLP178−191, or MOG92−106, PP-, and SP-course in A.SW mice with MOG92−106 and SJL/J mice with MOG92−106 and additional treatment (ultraviolet irradiation, injection of Bordetella Pertussis, apoptotic cell, or curdlan) (14–17). Monophasic EAE can also be induced in PL/J mice with MBP1−11 and C57BL/6 mice with MOG35−55 (18, 19). In this study, we used two PP-EAE models, A.SW mice sensitized with MOG92−106 and SJL/J mice sensitized with MOG92−106 and curdlan. Previously, we reported that A.SW mice sensitized with MOG92−106 developed PP-EAE with large areas of demyelination, immunoglobulin deposition, neutrophil infiltration, and spleen atrophy (14, 16).
Systemic and multivariate analyses of data from animal models for MS are powerful methods to characterize each model. In MS, microarray analyses have been performed mainly using peripheral blood lymphocytes. Several reports showed that various genes related to the immune response, apoptosis, and cell cycle progression were up- or down-regulated in disease (20, 21), while microarray analyses using human CNS tissues have been limited by its nature (22). In most microarray analyses in EAE, genes related to the immune response, such as cytokines, chemokines, and complement components, are known to be up-regulated in the CNS (23–26).
We aimed to determine CNS biomarkers and peripheral surrogate markers that could characterize the two PP-EAE models induced in A.SW and SJL/J mice. We have conducted microarray and bioinformatics analyses, using the brains and spleens which reflect the changes in the CNS and peripheral lymphoid organs, respectively. There were differences in numbers of genes that were up- and down-regulated in the brains and spleens between A.SW and SJL/J mice with PP-EAE, while immune response-related genes were highly up-regulated in the brains and erythrocyte-related genes highly down-regulated in the spleens from both mouse strains. Pathway analysis showed that Fc receptor and complement-related genes were up-regulated in both mouse strains' brains, but pro-inflammatory cytokine-related genes were up-regulated only in SJL/J mouse brains. Genes irrelevant to immune responses were down-regulated in the spleens of PP-EAE mice, and the expression of T helper (Th)1/Th2-related genes differed between A.SW and SJL/J mouse brains. Principal component analysis (PCA) of transcriptome data of brains and spleens separated between control and EAE groups. Pattern matching analysis between brain PCA data and spleen transcriptome data identified the spleen surrogate marker candidates that reflect the gene expression patterns in the brain. Translational application of our bioinformatics approach would be useful to identify the brain biomarkers and peripheral surrogate markers for MS.
Materials and Methods
Animal Experiments
To induce PP-EAE, 5-week-old female nine SJL/J mice and 13 A.SW mice (The Jackson Laboratory, Bar Harbor, ME) were sensitized in the base of the tail with 100 nmol of MOG92−106 peptide (DEGGYTCFFRDHSYQ, Core Facility, University of Utah Huntsman Cancer Institute, Salt Lake City, UT) in complete Freund's adjuvant (CFA) (14–16). On day −1 prior to MOG injection, 5 mg curdlan (a Th17 inducer produced by Alcaligenes faecalis var. myxogenes, Wako Pure Chemical Industries, Osaka, Japan) in PBS was injected for SJL/J mice intraperitoneally (27). To induce RR-EAE, six SJL/J mice were sensitized PLP139−151 (HSLGKWLGHPDKF) in CFA (28). Mice were given standard laboratory rodent chow and water ad libitum. All experimental procedures were reviewed and approved by the Institutional Animal Care and Use Committee of Louisiana State University Health Sciences Center (LSUHSC)-Shreveport, and performed according to the criteria outlined by the National Institutes of Health (NIH) (29).
Clinical signs of EAE and body weights were monitored daily (14, 30). Mice were euthanized at disease peak and remission of RR-EAE and at latent period, onset and peak of PP-EAE (Figure 1). At each time point, brains and spleens were harvested from three to six mice per group and frozen immediately in liquid nitrogen.
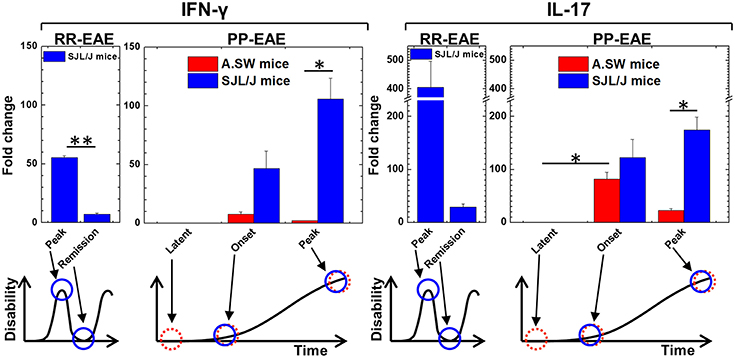
Figure 1. Kinetics of interferon (IFN)-γ and interleukin (IL)-17 expression of relapsing-remitting (RR)-experimental autoimmune encephalomyelitis (EAE) and primary progressive (PP)-EAE in SJL/J mice (blue column) and A.SW mice (red column). Expression of IFN-γ and IL-17 in brains were determined by real-time PCR. Expression levels were shown as fold changes compared with three control mice of each strain. In SJL/J mice with RR-EAE, both IFN-γ and IL-17 levels were high at the disease peak and low during remission (n = 3, at each time point). On the other hand, in PP-EAE, expression levels of both IFN-γ and IL-17 were associated with disease activity in SJL/J mice with EAE (n = 3, at each time point), while they increased at the onset (no increase at latent period) but decreased at the disease peak in A.SW mice with EAE (n = 3–6, at each time point). Data are presented as means ± standard error of the mean (SEM). *P < 0.05, **P < 0.001, ANOVA.
RNA Preparation
Brains and spleens from three to six mice per group were homogenized individually in TRI-Reagent® (Molecular Research Center, Cincinnati, OH), using the Kinematica Polytron™ homogenizer (Kinematica, Bohemia, NY). Total RNA was extracted with an RNeasy Mini Kit (Qiagen, Germantown, MD) according to the manufacturer's instructions from brain and spleen homogenate. DNase treatment was performed during RNA isolation with an RNase-Free DNase Set (Qiagen). All samples were purified to an absorbance ratio (A260/A280) between 1.9 and 2.1 (31).
Real-Time PCR
We reverse-transcribed 1 μg of total RNA into cDNA, using ImProm-II™ Reverse Transcription System (Promega Corporation, Madison, WI) (n = 3–7). We mixed 50 ng of cDNA with RT2 Fast SYBER® Green qPCR Master Mixes (Qiagen) and primer set. The mixture was amplified and monitored using iCycler iQ System (Bio-Rad Laboratories, Hercules, CA). The following primer sets were purchased from Real Time Primers (Elkins Park, PA): interferon (IFN)-γ, interleukin (IL)-17A, chemokine (C-X-C motif) ligand 13 (CXCL13), lipocalin 2 (LCN2), CD3 antigen γ subunit (CD3G), Kell blood group (KEL), and stefin A2 like 1 (STFA2L1). The results were normalized using housekeeping genes, glyceraldehyde-3-phosphate dehydrogenase (Gapd) or phosphoglycerate kinase 1(Pgk1) (32, 33).
Microarray Analysis
We used total RNA samples of brains and spleens from three mice with PP-EAE at the disease peak and three age-matched control mice for each mouse strain. We conducted microarray analyses, using Affymetrix GeneChip® Mouse Gene 1.0 ST Array (Affymetrix, Santa Clara, CA), according to the manufacturer's instruction. The data were visualized and quantified by Affymetrix GeneChip Command Console (AGCC), and normalized by Robust Multi-array Average (RMA) using Expression Console. Data were analyzed using the Ingenuity Pathway Analysis® (Qiagen), NetAffx database (Affymetrix; http://www.affymetrix.com/index.affx), and Mouse Genome Informatics (The Jackson Laboratory, Bar Harbor, ME; http://www.informatics.jax.org/). The datasets generated and/or analyzed during the current study are available in the Gene Expression Omnibus (GEO; http://www.ncbi.nlm.nih.gov/geo/) repository in National Center for Biotechnology Information (NCBI) (Accession number: GSE99300).
Bioinformatics and Statistics Analyses
Volcano Plot
We drew a volcano plot, using the OriginPro 8.1 (OriginLab Corporation, Northampton, MA), to visualize significance and fold changes of transcriptome data (34–36). In the volcano plot, log ratios (logarithms of fold changes to base 2) of gene expression in the brains and spleens from EAE mice compared with age-matched control mice were used as an x-axis and the logarithms of P values to base 10 were used as a y-axis.
Heat Map
We drew heat maps to determine the expression patterns of top 20 up- or down-regulated genes of brain and spleen samples from EAE mice, and compared the expression levels between EAE vs. control groups, using R version 3.2.2 and the programs “gplots” and “genefilter” (37). A list of abbreviations of genes is shown in Supplemental Table 1.
K-means Clustering
To find the differences of gene expression patterns between organs or mouse strains, we conducted k-means clustering using an R package “cclust” (37). We used Davies-Bouldin index (38) to determine the optimum number of clusters and obtained the lowest score (0.78), when microarray data were separated into 35 clusters (Supplemental Figure 2). Graphs were drawn using 240 genes (top 80, middle 80, and bottom 80 genes) in each cluster. A radar chart was drawn using the expression patterns of cluster center genes.
Ingenuity Pathway Analysis (IPA)
To classify the genes functionally, we used IPA (Qiagen) where we entered the genes whose genes were over- or under-transcribed more than 2-fold compared with control samples (P values <0.05). IPA shows possible networks involved in microarray profiles by the IPA Network Generation Algorithm (39). The algorithm clustered and classified the entered genes, which generated the networks, each of which was composed of three canonical pathways. The networks were ranked by the network score. The network score was calculated based on the right-tailed Fisher's Exact Test that uses several parameters, including the numbers of network eligible molecules in the network, the given dataset, and the IPA database. We focused the networks whose network score was higher than 35, since the only networks with high network scores have interpretable connections.
Principal Component Analysis (PCA)
Using PCA, we reduced the dimensionality of a microarray data set consisting of 28,853 mRNA expression signals into two components, principal component (PC) 1 and PC2 (37, 40, 41). PCA was conducted as an “unsupervised” analysis to clarify the variance among microarray data from brain and spleen samples using an R program “prcomp,” as we described previously (37, 42). The proportion of variance was also calculated to determine the percentage of variance explained by each PC, while factor loading for PC1 or PC2 was used to rank a set of genes contributing to PC1 or PC2 values.
Pattern Matching Analysis
To find the splenic genes whose expression patterns correlated with PC1 values in PCA of the brains, we conducted a pattern matching analysis based on correlation (43), using the R. We focused the genes whose expression levels, compared with control samples, were up- or down-regulated more than 2-fold, and whose correlation coefficients (r) were more than 0.8 or <−0.8.
Data Mining on Human MS Transcriptome Database
We obtained the gene expression profile datasets relevant to MS patients from GEO profile database (https://www.ncbi.nlm.nih.gov/geoprofiles/), using search keywords with the gene symbols identified in the current study as follows:
“multiple sclerosis”[All Fields] AND “Homo sapiens”[Organism] AND “peripheral blood”[All Fields] AND “disease state”[Flag Information] AND (gene symbols connected by OR). The data were processed according to the instructions of GEO profile database (44), and the differentially expressed genes (P < 0.05) between MS patients and controls were extracted.
Statistics
The data were shown as mean ± standard error of the mean (SEM). Statistical comparisons were conducted using the Student t test or analysis of variance (ANOVA), using the OriginPro 8.1. P < 0.05 was considered as significant difference.
Results
Levels of IFN-γ and IL-17 Were Associated With Disease Activity of PP-EAE in SJL/J Mice, but Not in A.SW Mice
The precise effector mechanism of PP-MS is currently unknown. Since the pro-inflammatory cytokines, IFN-γ, and IL-17 have been shown to be key effector molecules in many, but not all EAE models (15, 16, 45–48), we first examined the kinetics of IFN-γ and IL-17 in animal models for PP-MS, using two mouse strains. We induced PP-EAE with MOG92−106 in A.SW mice as we described previously (14–16). We also induced PP-EAE in SJL/J mice with MOG92−106 sensitization, 1 day after injection of curdlan. In SJL/J mice, disease continuously progressed until mice became moribund without remission within 20 days after initial clinical signs (Supplemental Figure 1). A.SW mice developed ataxic EAE and weight loss 1 month post induction (p.i.) of EAE (14, 16), while SJL/J mice developed classical EAE with tail and limb paralysis and weight loss in both PP- and RR-EAE.
Using real-time PCR, we conducted a time course analysis of IFN-γ and IL-17 mRNA levels in the brains of A.SW and SJL/J mice with PP-EAE (Figure 1). For comparison, we also used brain samples from SJL/J mice with RR-EAE. In both RR-EAE and PP-EAE in SJL/J mice, clinical signs were associated with increased levels of both IFN-γ and IL-17 in the brain. However, in PP-EAE in A.SW mice, the levels of IFN-γ and IL-17 increased at the disease onset, but declined during the disease progression. These results suggested that the effector mechanisms in disease progression of SJL/J mice and A.SW mice differed at the disease peak. Thus, we decided to analyze potentially distinct pathomechanisms of disease progression between A.SW and SJL/J mice with PP-EAE.
Volcano Plots of Brain and Spleen Transcriptome Data Showed Overall Greater Changes in SJL/J Mice, While A.SW Mice Had More Down-Regulated Spleen Genes
To compare the potentially distinct effector mechanisms during the disease progression between A.SW and SJL/J mice, we conducted conventional “supervised” two-way comparison analyses of the brain and spleen transcriptome data at the disease peak from the two mouse strains with PP-EAE. First, using volcano plots, we visualized the numbers of genes whose expression levels were significantly (P < 0.05) up- or down-regulated more than 2-fold compared with control samples (Figures 2A,B). In the brain, higher numbers of genes were up- or down-regulated in SJL/J mice than in A.SW mice, suggesting that molecular changes in SJL/J mice could be more complex than in A.SW mice (Figure 2A). On the other hand, in the spleen, the numbers of down-regulated genes were higher in A.SW mice, while those of up-regulated genes were higher in SJL/J mice (Figure 2B). An increased number of down-regulated genes in A.SW mouse spleen seemed to be associated with spleen weight changes at disease peak in PP-EAE, where the spleen of A.SW mice, but not SJL/J mice, showed significant atrophy (16).
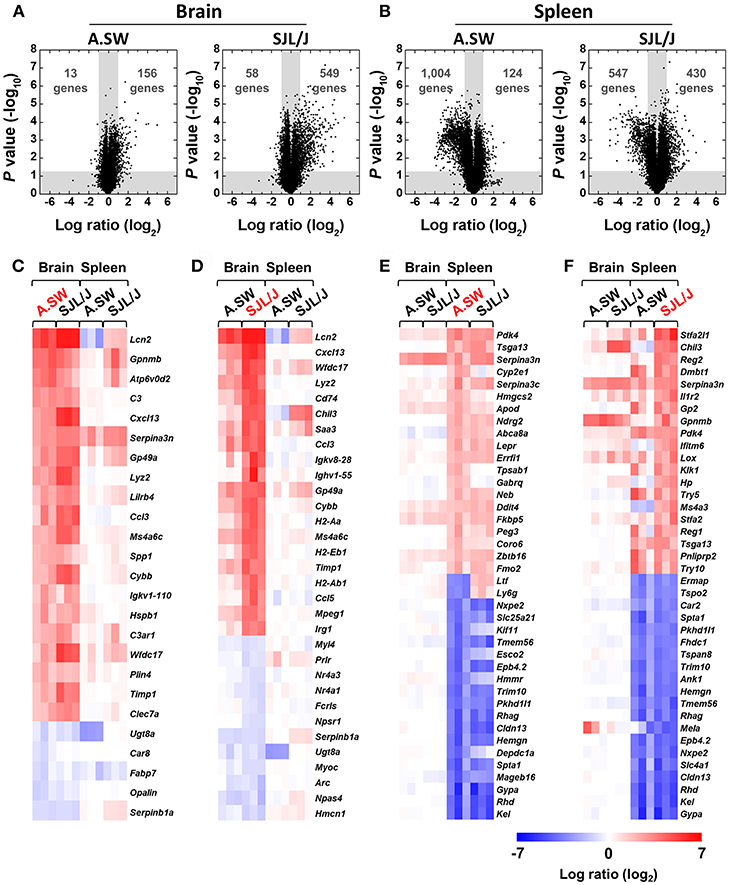
Figure 2. (A,B) Volcano plots of up- or down-regulated genes in the brains (A) and spleens (B) of PP-EAE mice. Gene expression profiles in the brains and spleens of three mice with PP-EAE at the disease peak and three age-matched control mice for each mouse strain were determined by microarray. Fold changes were calculated by division of signal values of EAE samples by those of controls. P values were calculated using the Student t test. Dots in the white areas were genes whose expressions were up- or down-regulated more than 2-fold (log2 ratio = 1, compared with controls), significantly (P < 0.05). In the brain, SJL/J mice had substantially higher numbers of both up- and down-regulated genes, compared with A.SW mice. On the other hand, in the spleen, SJL/J mice had a higher number of up-regulated genes, but a lower number of down-regulated genes, compared with A.SW mice. (C–F) Heat maps of identified genes among most highly up- or down-regulated 20 genes in the brains and spleens at disease peak of PP-EAE. Red, blue, and white indicate up-regulation, down-regulation, and no change, respectively. (C) In the brains of A.SW mice with PP-EAE, significantly up-regulated genes included: lipocalin 2 (Lcn2), complement-related genes, and chemokines. (D) In the brains of SJL/J mice with PP-EAE, Lcn2, chemokine-, MHC molecule-, and immunoglobulin-related genes were highly up-regulated. (E,F) In the spleens of both A.SW (E) and SJL/J (F) mice with PP-EAE, erythrocyte-related genes were down-regulated significantly. Overall, heat maps between A.SW and SJL/J mice were similar in the brains or spleens. A list of abbreviations of genes is shown in Supplemental Table 1.
Heat Maps Revealed the Up-Regulation of Immune Response-Related Genes in the Brains and Down-Regulation of Erythrocyte-Related Genes in the Spleens From Both Mouse Strains
Next, to visualize the differences in most highly up- or down-regulated genes in the brains and spleens, we drew heat maps, using microarray data at the disease peak (Figures 2C–F). Overall, heat maps of each organ (brain or spleen) were similar among samples from A.SW and SJL/J mice. Most of the highly up- or down-regulated genes in each organ in A.SW mice were also up- or down-regulated in SJL/J mice. On the other hand, heat maps between brains and spleens were different regardless of the mouse strains. Only serine (or cysteine) peptidase inhibitor, clade A, member 3N (Serpina3n) was highly up-regulated in both brains and spleens in both mouse strains. In the heat maps based on brain gene expression levels from A.SW (Figure 2C) and SJL/J mice (Figure 2D), commonly up-regulated genes included: lipocalin 2 (Lcn2); chemokines, such as chemokine (C-X-C motif) ligand 13 (Cxcl13); and chemokine (C-C motif) ligand 3 (Ccl3); complement-related genes, C3 and complement component 3a receptor (C3ar1); immunoglobulin (Igkv1-110); MHC class II-related genes, H2-Aa and Cd74 (CLIP). Serine (or cysteine) peptidase inhibitor, clade B, member 1a (Serpinb1a), and UDP galactosyltransferase 8A (Ugt8a) were down-regulated in common.
In the spleen heat maps based on gene expression levels from A.SW (Figure 2E) and SJL/J mice (Figure 2F), several genes, including pyruvate dehydrogenase kinase, isoenzyme 4 (Pdk4), testis specific gene A13 (Tsga13), and Serpina3n, were up-regulated in common, while erythrocyte-related genes, such as glycophorin A (Gypa), Kell blood group (Kel), Rh blood group, D antigen (Rhd), and claudin 13 (Cldn13) (49), were down-regulated significantly. On the other hand, lactotransferrin (Ltf) and lymphocyte antigen 6 complex, locus G (Ly6g/Gr1, a granulocyte marker) (50) showed different expression patterns between the spleens of two mouse strains with PP-EAE, down-regulation in A.SW mice and up-regulation in SJL/J mice. In addition, tryptase α/β 1 (Tpsab1) and γ-aminobutyric acid (GABA) A receptor, subunit θ (Gabrq) were up-regulated only in the spleen of A.SW mice, while immune response-related genes, such as chitinase-like 3 (Chil3), interferon induced transmembrane protein 6 (Ifitm6), and haptoglobin (Hp), were up-regulated only in the spleen of SJL/J mice.
K-means Clustering Revealed the Different Expression Patterns of Genes Between the Brains and Spleens of A.SW and SJL/J Mice With PP-EAE
To further identify the genes that had distinct expression patterns among the transcriptome data from brains and spleens of A.SW and SJL/J mice with PP-EAE at the disease peak, we divided all genes into 35 clusters, using k-means clustering, based on Davies-Bouldin Index (Supplemental Figures 2, 3). The centroid genes of the 14 of 35 clusters showed substantial changes (>2- or <1/2-fold compared with controls), at least, in one organ or in one mouse strain (Supplemental Figures 3, 4, lists of genes in each cluster were shown in Supplemental Tables 2–15). A radar chart for centroid genes of each cluster showed that, in most clusters, gene expression levels in one organ between the two mouse strains were similar, while those between brains vs. spleens were different (a radar chart using the 14 clusters in Figure 3A; radar chart using all the 35 clusters in Supplemental Figure 4). The radar chart showed that, in most clusters, the gene expression patterns in one organ between the two mouse strains were similar, while those between brain and spleen were different.
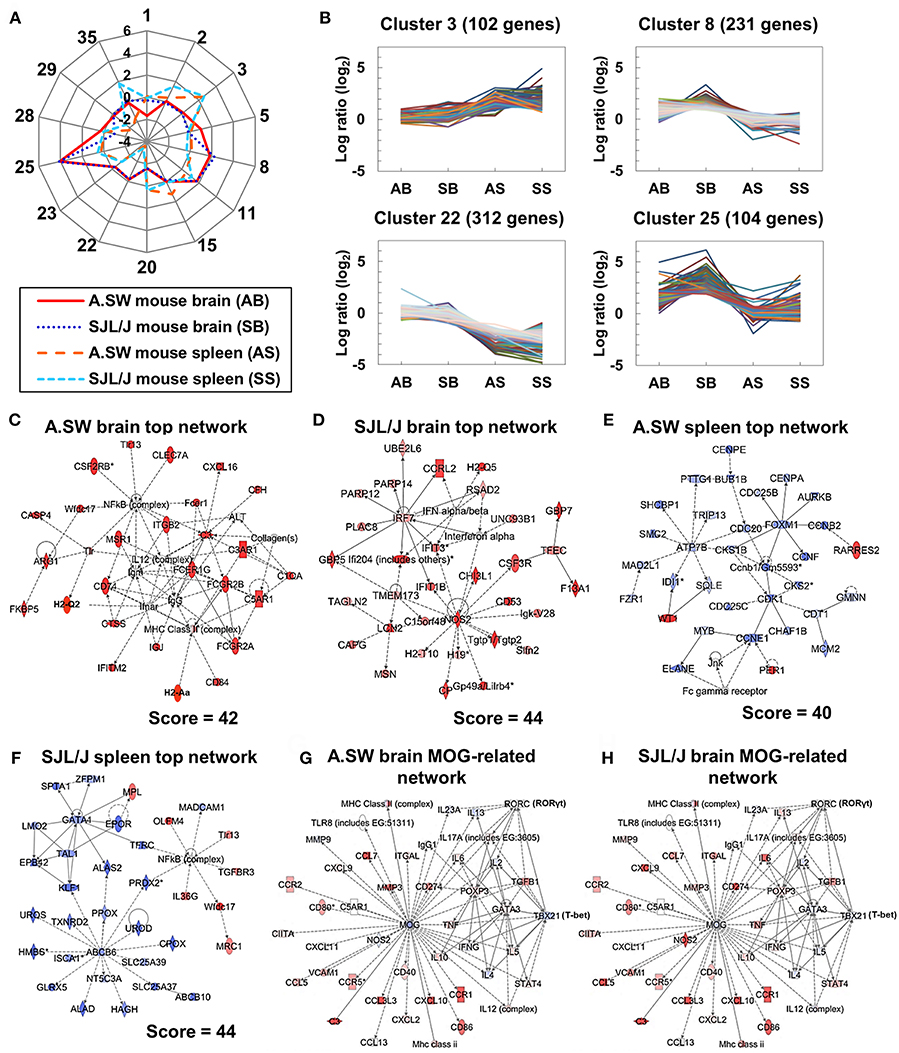
Figure 3. (A,B) K-means clustering for brain and spleen microarray data from two models. (A) A radar chart of 14 cluster center genes whose log ratios were higher than 1 or lower than −1, at least in one organ or in one mouse strain, compared with controls, among the total 35 clusters. (B) Dynamic expression patterns of four clusters. AB, SB, AS, and SS indicate A.SW mouse brain, SJL/J mouse brain, A.SW mouse spleen, and SJL/J mouse spleen, respectively. We used microarray data from three PP-EAE and three naïve mice of each strain (the total number of mice = 12). Genes in cluster 3 were up-regulated in the spleens of both models. Genes in cluster 8 were up-regulated in the brains of SJL/J mice. Genes in cluster 22 were down-regulated in the spleens of both models. Genes in cluster 25 were up-regulated in the brains of both models. (C–H) Gene networks up-regulated in the brains or down-regulated in the spleens from two models. Transcriptome data at disease peak were clustered and categorized by the Ingenuity Pathway Analysis (IPA) Network Generation. (C) The top network in the brains of A.SW mice was categorized as “Cell-To-Cell Signaling and Interaction,” “Hematological System Development and Function,” and “Immune Cell Trafficking,” which were composed of Fc receptor and complement-related genes. (D) The top network in SJL/J mouse brain was categorized as “Immunological Disease,” “Endocrine System Disorders,” and “Gastrointestinal Disease,” which were composed of IFN-α/β-induced genes and nitric oxide synthase 2 (Nos2). (E) The top network in A.SW mouse spleen was categorized as “Cell Cycle,” “Reproductive System Development and Function,” and “Cancer” which were composed of cell cycle-related genes. (F) The top network in SJL/J mouse spleen was categorized as “Small Molecule Biochemistry,” “Hematological Disease,” and “Metabolic Disease” which were composed of GATA1- and transporter-related genes. (G,H) Up- or down-regulated genes associated with MOG in the brains of A.SW mice (G) and SJL/J mice (H). Pro-inflammatory genes were down-regulated in A.SW mice and up-regulated in SJL/J mice, while Th2-associated genes were up-regulated in A.SW mice and down-regulated in SJL/J mice. Red and blue nodes indicate up- and down-regulated genes, respectively. Solid and dashed lines indicated direct and indirect connections, respectively. Score = network score.
The genes in the spleen were up-regulated in cluster 3 and down-regulated in cluster 22 in both mouse strains, while there were no substantial changes (log ratios ≈0, compared with controls) in cluster 3 or 22 in the two mouse brain samples (Figure 3B). Cluster 3 included stefins (Stfa1 and Stfa2l1) (Supplemental Table 4), while cluster 22 included erythrocyte-related genes, such as Kel, Rhd, and Gypa (Supplemental Table 10). On the other hand, the genes in clusters 8 and 25 were up-regulated only in the brains, but not in the spleens, in both mouse strains. Immune response-related genes were included in clusters 8 and 25: Cxcl9, Cxcl10, and Cd3g in cluster 8; Lcn2, Cd74, and H2-Aa in cluster 25 (Supplemental Tables 6, 12). Some genes in cluster 8 were up-regulated only in the brains of SJL/J mice (e.g., Cd3g: 1.3-fold in A.SW mice, 4.9-fold in SJL/J mice), while several genes in cluster 25 were only down-regulated in A.SW mouse spleens (e.g., Lcn2: 0.3-fold). Up-regulation of Cd3g in SJL/J mouse brain, but not A.SW mouse brain, was consistent with our previous histological finding that CNS CD3+ T cell infiltration was seen only in SJL/J mice (14, 16). Thus, k-means clustering clearly showed that groups of immune response-related genes were induced in each organ commonly in two mouse strains, but differentially between brains vs. spleens at the peak of disease progression. However, k-means clustering alone was insufficient to identify individual genes that were expressed differentially between the two strains, requiring further analyses.
Brain Pathway Analysis Revealed That Fc Receptor and Complement-Related Genes Were Up-Regulated in Both Mouse Strains Brains, but Pro-inflammatory Cytokine-Related Genes Were Up-Regulated Only in SJL/J Mice
Using the IPA, we clustered and categorized the genes up- or down-regulated in the brains and spleens of mouse models for PP-EAE (Figures 3C–F). The IPA identified one network in A.SW mice and five networks in SJL/J mice with a high network score (>35). The network identified in the brains of A.SW mice was categorized as “Cell-To-Cell Signaling and Interaction,” “Hematological System Development and Function” and “Immune Cell Trafficking” (Figure 3C). This network contained up-regulation of immune response-related genes: particularly MHC molecules [H2-Aa (MHC class II), H2-Q2 (MHC class I), and Cd74 (invariant polypeptide of MHC class II molecule)]; immunoglobulin-related genes, including immunoglobulin J chain (Igj) (51) and Fc receptors (Fcgr2a, Fcgr2b, Fcer1a, and Fcer1g); and complement-related genes, including complement component 3 (C3), C3a receptor 1 (C3ar1), C5a receptor 1 (C5ar1), complement component factor h (Cfh), and C1q α chain (C1qa) (52, 53).
In the brains of SJL/J mice, all five networks with a high network score were associated with immune responses (Figure 3D and Supplemental Figure 5). The top 1 network was categorized as “Immunological Disease,” “Endocrine System Disorders,” and “Gastrointestinal Disease” (Figure 3D). The network was composed of up-regulated genes related to IFN-α/β and nitric oxide synthase 2 (Nos2). The top 2 network contained similar genes to the top 1 network of A.SW mouse brain, such as Fc receptor and complement-related genes, while substantial up-regulation of the genes related to IL-6-related genes and costimulatory molecules Cd80/Cd86 (B7-1/B7-2) was seen only in SJL/J mice (Supplemental Figure 5). All the top 3, 4, and 5 networks were associated with pro-inflammatory cytokines, IL-1β, tumor necrosis factor (TNF)-α, and IFN-γ, respectively.
Spleen Pathway Analysis Revealed That Genes Irrelevant to Immune Responses Down-Regulated in the Spleens of PP-EAE Mice
In the spleen of A.SW mice, we identified three networks, which were composed of mainly down-regulated genes that are irrelevant to immune responses (Figure 3E and Supplemental Figure 6). The top 1 network in the spleens of A.SW mice was categorized as “Cell Cycle,” “Reproductive System Development and Function,” and “Cancer,” including down-regulated genes: cyclin family (Ccne1, Ccnb1, and Ccnb2) and cell division cycle family (Cdc20, Cdc25b, and Cdc25c) (Figure 3E). The top 2 and 3 networks were mainly composed of down-regulated GATA binding protein 1 (Gata1)-related genes and transporter genes (Abcb6, Slc25a39, and Slc25a37), respectively (Supplemental Figure 6). On the other hand, in the spleens of SJL/J mice, we identified only one network with a high network score (Figure 3F). The network was categorized as “Small Molecule Biochemistry,” “Hematological Disease,” and “Metabolic Disease,” which were composed of down-regulated genes that were listed in the top 2 and 3 networks of A.SW mouse spleen: Gata1-related genes and transporter genes (Abcb6, Slc25a39, and Slc25a37). Gata1-related genes are essential for normal hematopoiesis, particularly erythropoiesis (54), while transporter genes are cell membrane proteins that control the uptake and efflux of various compounds (55, 56). The network also included up-regulated genes, such as Toll-like receptor 13 (Tlr13) and transforming growth factor (TGF) β receptor III (Tgfbr3).
MOG-related Pathway Analysis Revealed That Expression of Th1/Th2-Related Genes Differed Between Two Mouse Brains
In both mouse strains, we also determined the gene expression changes in a MOG-related network in the brains, using IPA (Figures 3G,H). Mog itself was down-regulated in both mouse strains (A.SW, 0.81-fold, P < 0.05; SJL/J, 0.85-fold, P < 0.05). The gene expression of several cytokines and chemokines was up-regulated similarly in both mouse strains, including Cxcl10, Ccr1, and Il6. However, some pro-inflammatory genes, such as Ifng, Cxcl11, Mmp9, and Nos2, were down-regulated in A.SW mice (Figure 3G), while they were up-regulated in SJL/J mice (Figure 3H). On the other hand, Th2-related genes (Gata3 and Il5, but not Il4) were up-regulated in A.SW mice, while they were down-regulated in SJL/J mice.
PCA of Transcriptome Data of Brains and Spleens Separated Two EAE Groups
To identify the molecules (biomarkers) that distinguish the samples between PP-EAE and control mice, we analyzed microarray data, using an unsupervised approach, PCA (Figure 4). PCA clearly separated brain samples into four groups, each of which was composed of samples from A.SW and SJL/J mice with PP-EAE, and their control mice (Figure 4A), showing that distinct gene expression patterns were present between the four groups. PCA showed that PC1 likely reflected the presence or absence of EAE, while PC2 reflected strain differences. Proportion of variance indicated that PC1 explained 33% of variance among samples, while PC2 explained 20% of variance (Figure 4B). By using factor loading for PC1, we ranked the genes that contributed to the PC1 values (Figure 4C and Supplemental Table 16). Up-regulation of immune response-related genes, including Lcn2, Cxcl13, Chil3, immunoglobulins (Igkv8-28 and Ighv1-55), and MHC class II molecule (H2-Aa), as well as down-regulation of prolactin (Prl), contributed to the PC1 values. Among factor loadings for PC2, although most genes were unidentified, cytochrome P450, family two, subfamily g, polypeptide 1 (Cyp2g1), and BPI fold containing family B, member 9B (Bpifb9b) were listed (Supplemental Figure 7 and Supplemental Table 17).
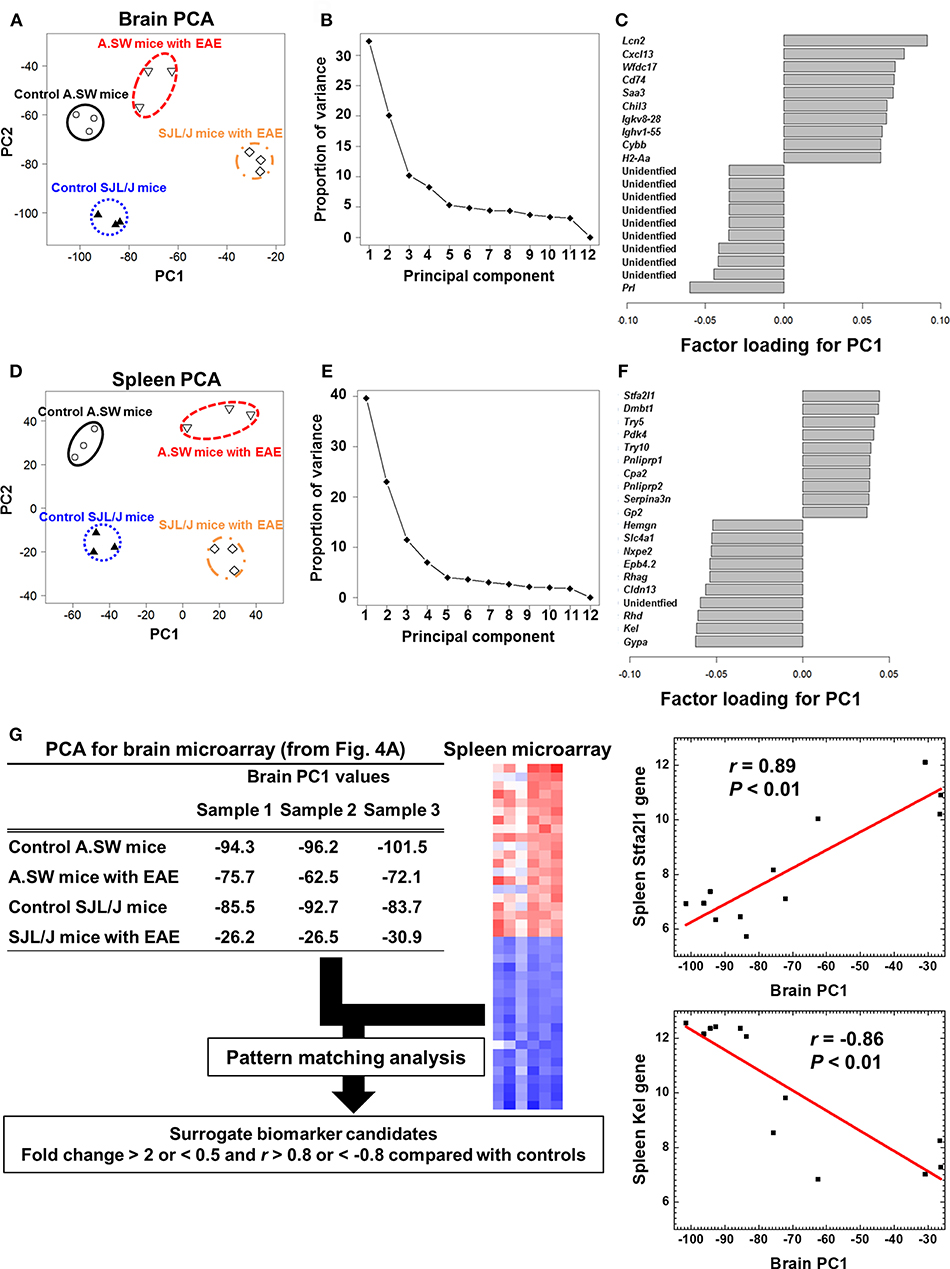
Figure 4. (A–F) Principal component analysis (PCA) of transcriptome data of brains and spleens from A.SW and SJL/J mice with PP-EAE and control mice. PCA separated the samples into four groups in both brains (A) and spleens (D), where principal component (PC) 1 reflected the presence of EAE, while PC2 reflected strain difference. The proportion of variance showed that PC1 explained variance among samples 33% in the brains (B) and 40% in the spleens (E). In the brain (C), factor loading for PC1 showed that up-regulation of immune response-related genes, including lipocalin 2 (Lcn2), CXCL13, and immunoglobulins, and down-regulation of prolactin (Prl) contributed to PC1 values. In the factor loading for PC1 of spleen PCA (F), stefin A2 like 1 (Stfa2l1) and erythrocyte-related genes (Gypa, Kel, and Rhd) contributed to PC1 distribution positively and negatively, respectively. Transcriptome data from three PP-EAE and three naïve mice of each strain were used. (G) A flow chart of pattern matching analysis using brain PC1 values and spleen microarray data from A.SW and SJL/J mice. To find peripheral surrogate biomarkers that reflect the changes in the brain, we conducted pattern matching analysis. Genes whose fold changes were >2 or <0.5 with correlation coefficients of >0.8 or <-0.8 were identified as surrogate marker candidates (Supplemental Table 15). Stfa2l1 and Kel genes, which were up- and down-regulated significantly in the spleens of PP-EAE mice, respectively, were strongly correlated with brain PC1 values (Stfa2l1: r = 0.89, P < 0.01; Kel: r = −0.86, P < 0.01). We used microarray data of brains and spleens of three mice with PP-EAE and three age-matched control mice for each mouse strain (the total number of mice = 12).
PCA of spleen microarray data also separated samples clearly into four groups (Figure 4D). PC1 explained 40% of variance among samples, while PC2 explained 23% of variance (Figure 4E). PC1 reflected the presence or absence of EAE, while PC2 reflected the strain difference. Factor loading showed that stefin A2 like 1 (Stfa2l1), deleted in malignant brain tumors 1 (Dmbt1), and trypsin genes (Try5 and Try10) contributed to the PC1 values positively, while erythrocyte-related genes (Gypa, Kel, Rhd, and Cldn13) contributed to the PC1 value negatively (Figure 4F and Supplemental Tables 18, 19). Among top or bottom 100 genes that were listed in factor loading for PC1 values, only three genes, Chil3, Serpina3n, and leucine-rich α-2-glycoprotein 1 (Lrg1), were in common in the brains and spleens: Chil3, which is also known as Ym1, is rodent-specific chitinase-like protein and associated with Th2 inflammation (57), Serpina3n is an inhibitor of granzyme b (58), and Lrg1 is related to TGF-β signaling pathway (59). Thus, most CNS gene expression changes seemed to occur independently from those in the peripheral lymphoid organs, during the disease progression of EAE.
Pattern Matching Analysis Showed Spleen Surrogate Marker Candidates That Reflect the Gene Expression Patterns in the Brain
In the above PCA, we attempted to find peripheral surrogate markers that reflect the changes in the brain. However, we were not able to identify the common genes using factor loading for PC1 among the brain and spleen transcriptome data. Thus, we conducted a pattern matching analysis using brain PC1 values and spleen microarray data from two PP-EAE models and controls; pattern matching analysis allowed us to find splenic genes whose expression patterns matched the PC1 values of brain samples (Figure 4G). When the results were sorted by correlation coefficients (r >0.8 or <-0.8) and expression ratios (>2- or <1/2-fold, compared with controls), 333 genes showed strong correlation (Supplemental Table 20). Among the 333 genes, we found 29 splenic genes positively correlated with the brain PC1 values, including adhesion G protein-coupled receptor G2 (Adgrg2), Lrg1, and phosphoinositide-3-kinase interacting protein 1 (Pik3ip1). On the other hand, we found 304 splenic genes negatively correlated with the brain PC1 values, including progestin and adipoQ receptor family member IX (Paqr9), RAB3A interacting protein (rabin3)-like 1 (Rab3il1), and Josephin domain containing 2 (Josd2). Among the positively and negatively correlated genes, Stfa2l1 (r = 0.89) and erythrocyte-related genes, including Kel (r = −0.86), were listed in the top 10 of factor loading for PC1 in spleen PCA (Figure 4F). Thus, using pattern matching analysis, we were able to find the peripheral surrogate marker candidates among non-immune-related molecules that could reflect the gene expression changes in the brain.
Next, we determined whether the genes listed as peripheral surrogate marker candidates in the mouse spleens (Supplemental Table 20) were also up- or down-regulated in blood transcriptome of human MS patients obtained from the GEO profile database, using a data mining approach with following search keywords: “multiple sclerosis,” “Homo sapiens,” “peripheral blood,” “disease state,” and the 29 up-regulated gene symbols or the 304 downregulated gene symbols (Supplemental Table 21). Among the 29 positively correlated genes listed in Supplemental Table 20, we found that two genes, period circadian clock 1 [PER1, also known as microRNA 6883 (MIR6883)] and FK506 binding protein 5 (FKBP5) were up-regulated in MS peripheral blood, significantly (P < 0.01, Supplemental Table 21). Among the 304 negatively correlated genes, we found that only one gene, solute carrier family 16 member 1 [SLC16A1, also known as monocarboxylate transporter (MCT) 1] was down-regulated in MS peripheral blood, significantly (P < 0.05, Supplemental Table 21). Up-regulation of Per1 and down-regulation SLC16A1 were found in the data set (60) from 12 MS patients compared with 15 unaffected controls, whose other clinical data were not available. Upregulation of FKBP5 was found in the data set of peripheral blood cells from three MS patients with high serum levels of transmembrane-type semaphorin (Sema4A) (but not from MS patients with low Sema4A levels), compared with four healthy controls with low serum levels of Sema4A (61).
Validation of Transcriptome Data of Biomarker Candidates in the Brains and Spleens
To validate transcriptome data of brain and spleen samples, we conducted real-time PCR for the representative genes listed in the clustering, PCA factor loading and pattern matching data (Supplemental Figure 8). The expression patterns of Cxcl13, Lcn2, and Cd3g in the brain samples and those of Kel and Stfa2l1 in the spleen samples between microarray and real-time PCR data were consistent. The levels of Cxcl13, Lcn2, and Cd3g in the brains with PP-EAE were higher in SJL/J mice than in A.SW mice. Similarly, in the spleen, the expression of Stfa2l1 was also up-regulated. On the other hand, Kel was down-regulated in the spleens of both PP-EAE mice. Expression of Lcn2 in the spleens was significantly down-regulated in A.SW mice and up-regulated in SJL/J mice.
Discussion
There have been controversies on whether MS is a heterogeneous or homogenous disease (12, 47, 62). The heterogeneity of MS can be further discussed in three aspects; whether MS is heterogeneous or homogenous (1) “in time (during the time course),” (2) “in space” in individual patients with MS, and (3) in the pathology type among MS patients. These theories are based on mainly clinical neuroimaging and neuropathological studies of human MS cases, which have limitations; for example, longitudinal biopsies of CNS tissues are not possible in one individual. While such human studies have often supported one theory, and tended to deny the other theories, this can be due to the limitation of the methodology employed in each study. Our current computational studies of two EAE models for progressive MS can be a proof of concept that autoimmune demyelinating diseases can be either homogenous or heterogeneous in all three aspects, to some extent.
First, “Is MS a heterogeneous in time?” in other word, “Is MS a 1-stage or 2-stage disease (47)?” The “1-stage” disease theory is that the pathophysiology (effector mechanism) of MS is the same during the entire course of MS in individual patients. The “2-stage” disease theory is that CNS tissue damage is caused by inflammation in Stage 1, while neurodegeneration in Stage 2 is independent of inflammation, leading to disease progression. While some neuropathology studies in MS supported the 1-stage disease theory, neuroimaging and clinical studies, such as drug responses and epidemiological data, supported the 2-stage disease theory (63, 64).
In our current study, when we assessed kinetics of IFN-γ and IL-17 levels, these pro-inflammatory cytokines were associated with disease activities in RR-EAE and PP-EAE in SJL/J mice (common effector mechanism in initiation, acute attack, and disease progression), while IFN-γ and IL-17 levels in PP-EAE in A.SW mice were up-regulated only in disease initiation, but declined at disease peak. Since these results suggested that another effector mechanism independent of the pro-inflammatory responses could contribute to disease progression in A.SW mice, we further conducted transcriptome analyses of the CNS at disease peak of PP-EAE in both mouse strains. Volcano plots of transcriptome data showed the different number of up- or down-regulated genes between brains and spleens or between A.SW and SJL/J mice. While many genes were up-regulated in the brains and down-regulated in the spleens, down-regulation of genes in the spleen may be associated with splenic atrophy (16). Heat maps showed highly up-regulated genes in each brain and spleen of two mouse strains as a result of “supervised” two-way comparison. In the brains of both models, several genes were up-regulated in common. Among the genes, Lcn2 was the most highly up-regulated gene, which has been reported as an immune mediator of EAE and MS (65, 66). Glycoprotein nonmetastatic melanoma B (Gpnmb) is a type I transmembrane protein which works in various biological processes, such as inflammation (67, 68). Activation of complement components, including C3, plays a pivotal role by recruiting inflammatory cells, increasing myelin phagocytosis by macrophages, and exerting direct cytotoxic effects on oligodendrocytes (69). Cxcl13 attracts B lymphocytes and Th cells via chemokine receptor CXCR5 (70) and can be used as a biomarker of inflammation in MS (71). Since Cybb/Nox2 was also up-regulated, oxidative stress may be related to damage in the brain (72).
In the spleens of both models, we found significant down-regulation of Kel, Rhd, Gypa, and Cldn13, which have been reported as erythrocyte-related genes (Figures 2E,F) (49). This is consistent with splenic pathway analysis data (Figure 3F and Supplemental Figure 6A), in which we found down-regulation of Gata1-related genes that are essential for erythropoiesis (54). Stfa2l1, which acts as a cathepsin inhibitor, was up-regulated in common and could regulate antigen presentation processes involved in immune response and autoimmune diseases (73). Interestingly, two neutrophil-associated proteins, Ltf, and Ly6g were down-regulated in A.SW spleens, but up-regulated in SJL/J spleens. Ltf is a protein contained in secondary granules of neutrophils and can ameliorate the signs of EAE (74), while Ly6g is expressed in neutrophils and can regulate leukocyte activation and adhesion (75). Distinct expression of these genes suggested the different role of neutrophils in two PP-EAE models.
Interestingly, our bioinformatics analyses, including pathway analyses and PCA, demonstrated that antibody-mediated pathophysiology (composed of immunoglobulin-, complement-, and FcR-related molecules) seemed to be active in both mouse strains. We confirmed the presence of immunoglobulin deposition and complement receptor positive cells by immunohistochemistry (data not shown). In PP-EAE in SJL/J mice, bioinformatics analyses also showed that the top network present in the CNS was associated with pro-inflammatory responses composed of most major inflammatory pathways, including those of IFN-α/β, IFN-γ, IL-17, IL-6, TNF-α, and IL-1β. Thus, in SJL/J mice, the pro-inflammatory effector mechanism could play a pathogenic role during the entire course (here, the disease might look a homogeneous disease, if one focuses only on these pro-inflammatory responses), while the antibody-mediated effector mechanism also seemed to be active at disease peak in both mouse strains (Figure 5). On the other hand, downregulation of prolactin also contributed to the separation between EAE and control groups in PCA. Prolactin is secreted not only by the anterior pituitary but also extra-pituitary tissues including immune cells, while prolactin receptor is found on lymphocytes and other immune cells (76). Prolactin has several roles including immunomodulation and remyelination. Although our current PCA demonstrated downregulation of prolactin could be associated with EAE progression, prolactin has been suggested to exacerbate other EAE models (77). Similarly, in human MS, an association between prolactin levels and disease activities remains controversial (76). Ysrraelit and Correale proposed that prolactin may exert dual and opposing effects in MS and that caution must be taken when prolactin levels are manipulated in MS.
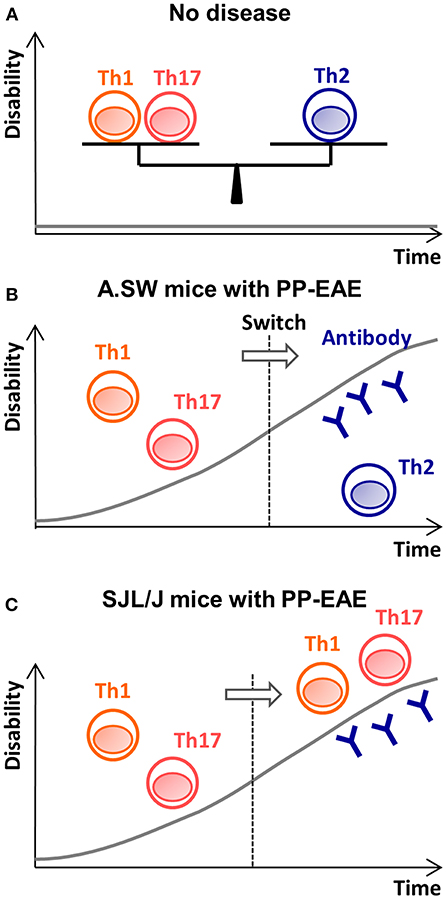
Figure 5. The disease theories in A.SW and SJL/J mice with PP-EAE. (A) When Th1/Th17 cells vs. Th2 cells is well-balanced, no disease is induced. (B) In A.SW mice, increased Th1/Th17 immune responses initiate disease. Later, the Th1/Th17 responses decline, and increased Th2 immune responses help auto-antibody production. Here, effector mechanism switches from proinflammatory Th1/Th17 responses (first stage) to antibody-mediated pathology (second stage). (C) In SJL/J mice, while Th1/Th17 cells play an effector role during the entire disease course, anti-myelin antibody also contributes to disease progression at the “second” stage.
However, these results do not deny the possibility of disease progression based on the 1-stage disease theory, since uncontrolled pro-inflammatory cellular responses alone can lead to disease progression regardless of the presence of involvement of antibody and complement, in theory. Indeed, many experimentally proven encephalitogenic antigens, including MBP, PLP, and neurofilament light chain (NF-L), can induce only pathogenic T cell responses that cause neurological deficits (antibodies to MBP, PLP, and NF-L do not cause tissue or cell injury because their epitopes are not expressed on cell surface) (78).
Our bioinformatics transcriptome analyses also addressed the second question, “Is MS pathology homogeneous or heterogeneous in space, in one patient, and at one time point?” or “Is there only one pathology (one effector mechanism) present in the CNS or are multiple different pathologies simultaneously present in the CNS in one patient?” At the disease peak of A.SW mice with PP-EAE, we identified only one major effector mechanism (= antibody and complement-mediated tissue damage), while two effector mechanisms may be involved in SJL/J mice: (1) antibody and complement-mediated tissue damage and (2) pro-inflammatory CD3+ T cell-mediated tissue damage. Histologically, in SJL/J mice, we found that some areas showed antibody deposition without T cell infiltration and other areas contained T cell infiltration with or without antibody deposition (data not shown). These results suggested that CNS demyelinating pathology can be homogenous (contain one pathology type) or can be heterogeneous (contain more than two pathologies) in a single individual. In most CNS peptide-induced EAE models, pathology has been shown to be homogeneous since most peptides are either major T cell epitopes or B cell epitopes, but not both. In contrast, multiple effector mechanisms as well as heterogeneous neuropathology can be present in one single EAE model, when EAE is induced with encephalitogens that have both T-cell and B-cell epitopes [for example, MOG92−106 (current experiment) and brain homogenates].
Our results also addressed the third question, “Is MS pathophysiology homogeneous (common) in all MS patients, or are there heterogeneities in pathophysiology among MS patients?” Our current experiments showed that a single encephalitogen (MOG92−106) can cause two different pathophysiologies (pro-inflammatory and antibody-mediated). This supports a concept and clinical pathology findings that MS neuropathology is heterogeneous. However, this does not deny the presence of possible common (homogeneous) pathologic component of demyelinating diseases. For example, in our current studies, the antibody-mediated tissue damage seemed to be a common effector mechanism in two PP-EAE models; we also found that some genes, such as Lcn2 and Chil3, were commonly up-regulated in two models. In addition, common neuropathology and effector components have been demonstrated among several different EAE models that were induced with different encephalitogenic antigens. For example, EAE can be induced in SJL/J mice or C57BL/6 mice, using different encephalitogens, such as PLP139−151, PLP178−192, MOG92−106, and MOG35−55 (13, 79). Here, neuropathology and pro-inflammatory immune responses in EAE induced with these different peptides were overall indistinguishable (14, 28, 80). In this context, it should also be noted that virus-induced demyelinating models share a common pathology and effector mechanism (3). Therefore, in theory, the cause of MS (several different autoantigens or even viruses) can be homogeneous or heterogeneous. Here, one autoantigen can cause different (heterogeneous) pathology depending on the genetic background or the presence of adjuvant (which mimics polymicrobial infection). On the other hand, several different autoantigens (different causes) can induce the same (homogeneous) pathology in the CNS of MS patients.
In this study, we have also conducted splenic transcriptome analyses to find peripheral surrogate markers that reflect the change in the CNS. In clinical studies in MS, while some reports showed that immune profiles in the blood reflected disease activity, others showed that peripheral profiles did not reflect the change in the CNS (81, 82). Using heat map and network/pathway analyses, we found that highly up-regulated and down-regulated genes and pathways were different between the spleens and brains in both mouse strains. Interestingly, in splenic pathway analysis, both mouse strains had down-regulation of GATA1-related genes and transporter genes (Figure 3F and Supplemental Figures 6A,B), while only A.SW mice had down-regulation of a network related to the cell cycle (Figure 3E). Cell cycle arrest could occur in the atrophic spleen with apoptosis in A.SW mice with progressive EAE, as we reported previously (16). Thus, A.SW mice had an additional major change in the network, comparing with SJL/J mice; this is in contrast to the CNS network profiles where SJL/J mice had an additional effector mechanism, comparing with A.SW mice.
We also conducted PCA using splenic transcriptome data from A.SW and SJL/J mice. Although PC1 values reflected the presence or absence of EAE in both the CNS and spleens, we did not find commonly up- or down-regulated genes contributing to PC1 between the brain and spleen factor loading for PC1. Thus, both supervised two-way comparison and unsupervised PCA showed that there were only three genes in common between the brains and spleens: Chil3, Serpina3n, and Lrg1. This could be consistent with a hypothesis that immune responses in progressive MS are sequestered from the systemic immune responses; the pathophysiology in the CNS at this stage may occur within the intact blood-brain barrier, and be independent of systemic immune responses (83).
On the other hand, our pattern matching analyses between the brain PC1 (that may reflect brain disease) and spleen transcriptome data showed that the pattern changes in a set of peripheral genes were significantly correlated with the brain PC1 values. Interestingly, the splenic genes showed the significant correlation with brain PC1 values were not immune-mediated genes. Although the causal relationship between the brain pathophysiology and splenic transcriptome changes is unclear, these set of splenic genes could be used as surrogate markers, or may be the contributing factor and/or outcomes of the pathology in the CNS. Among the genes listed as peripheral surrogate marker candidates (Supplemental Table 20), three genes, PER1, FKBP5, and SLC16A1 were up- or down-regulated significantly in the peripheral blood data sets from MS patients, although these data sets were from small numbers of patients with unknown clinical histories (Supplemental Table 21). PER1 encodes microRNA6883, which is associated with circadian rhythm (84). FKBP5 is a member of immunophilin protein family which works in immunoregulation and interacts with the progesterone receptor and GATA-2 (85). SLC16A1 encodes the MCT1, whose inhibition has been found to modulate T cell responses (86, 87). This is the first report showing the association between these three genes and MS. Peripheral surrogate marker candidates identified in this study might be worth monitoring in MS blood samples.
Data Availability
The datasets generated for this study can be found in Gene Expression Omnibus, GSE99300.
Author Contributions
AM, JA, and IT conceived and supervised the project. SO and NK designed the experiments. SO, FS, and NM conducted the experiments. SO, A-MP, MF, and UC conducted bioinformatics analyses. SO and IT wrote the manuscript. All authors read and approved the final manuscript.
Funding
This work was supported by an Institutional Development Award (IDeA) from the National Institute of General Medical Science of the NIH (5P30GM110703, IT), the fellowships from the Malcolm Feist Cardiovascular Research Endowment, LSUHSC-Shreveport (SO and FS), Science Research Promotion Fund from the Promotion and Mutual Aid Corporation for Private Schools of Japan (FS), Faculty Assistance and Development Research Grant from the Kindai University Research Enhancement Grant (SO and FS), and KAKENHI from the Japan Society for the Promotion of Science [JSPS, Grant-in-Aid for Scientific Research (B)-MEXT KAKENHI Grant #15K08975 (A-MP), Grant-in-Aid for Young Scientists (B) KAKENHI, JP17K15628 (FS), and Grants-in-Aid for Scientific Research-KAKENHI, 16H07356 (IT)].
Conflict of Interest Statement
The authors declare that the research was conducted in the absence of any commercial or financial relationships that could be construed as a potential conflict of interest.
Acknowledgments
We thank Elaine Cliburn Stewart, Sadie Faith Pearson, Lesya Ekshyyan, and Paula Polk for excellent technical assistance. We also thank Drs. Tsutomu Mori and Takashi Kawamura, Department of Human Life Sciences, Fukushima Medical University School of Nursing, for helpful comments.
Supplementary Material
The Supplementary Material for this article can be found online at: https://www.frontiersin.org/articles/10.3389/fimmu.2019.00516/full#supplementary-material
References
1. Willenborg DO, Staykova MA. Cytokines in the pathogenesis and therapy of autoimmune encephalomyelitis and multiple sclerosis. Adv Exp Med Biol. (2003) 520:96–119. doi: 10.1007/978-1-4615-0171-8_7
2. Browne P, Chandraratna D, Angood C, Tremlett H, Baker C, Taylor BV, et al. Atlas of multiple sclerosis 2013: a growing global problem with widespread inequity. Neurology. (2014) 83:1022–4. doi: 10.1212/WNL.0000000000000768
3. Sato F, Omura S, Martinez NE, Tsunoda I. Animal models of multiple sclerosis. In: Minagar A, editor. Neuroinflammation. 2nd ed. Burlington, MA: Academic Press (2018). p. 37–72.
4. Katz Sand I. Classification, diagnosis, and differential diagnosis of multiple sclerosis. Curr Opin Neurol. (2015) 28:193–205. doi: 10.1097/WCO.0000000000000206
5. Miller DH, Chard DT, Ciccarelli O. Clinically isolated syndromes. Lancet Neurol. (2012) 11:157–69. doi: 10.1016/S1474-4422(11)70274-5
6. Ebers GC. Prognostic factors for multiple sclerosis: the importance of natural history studies. J Neurol. (2005) 252 (Suppl. 3) iii15–iii20. doi: 10.1007/s00415-005-2012-4
7. Rudick RA. The elusive biomarker for personalized medicine in multiple sclerosis: the search continues. Neurology. (2012) 79:498–9. doi: 10.1212/WNL.0b013e318259e13a
8. Kalinski P, Moser M. Consensual immunity: success-driven development of T-helper-1 and T-helper-2 responses. Nat Rev Immunol. (2005) 5:251–60. doi: 10.1038/nri1569
9. Yanagawa K, Kawachi I, Toyoshima Y, Yokoseki A, Arakawa M, Hasegawa A, et al. Pathologic and immunologic profiles of a limited form of neuromyelitis optica with myelitis. Neurology. (2009) 73:1628–37. doi: 10.1212/WNL.0b013e3181c1deb9
10. Lucchinetti C, Brück W, Parisi J, Scheithauer B, Rodriguez M, Lassmann H. Heterogeneity of multiple sclerosis lesions: implications for the pathogenesis of demyelination. Ann Neurol. (2000) 47:707–17. doi: 10.1002/1531-8249(200006)47:6%3C707::AID-ANA3%3E3.0.CO;2-Q
11. Breij ECW, Brink BP, Veerhuis R, van den Berg C, Vloet R, Yan R, et al. Homogeneity of active demyelinating lesions in established multiple sclerosis. Ann Neurol. (2008) 63:16–25. doi: 10.1002/ana.21311
12. Sato F, Martinez NE, Omura S, Tsunoda I. Heterogeneity versus homogeneity of multiple sclerosis. Expert Rev Clin Immunol. (2011) 7:165–7. doi: 10.1586/eci.11.3
13. Didonna A. Preclinical models of multiple sclerosis: advantages and limitations towards better therapies. Curr Med Chem. (2016) 23:1442–59. doi: 10.2174/0929867323666160406121218
14. Tsunoda I, Kuang L-Q, Theil DJ, Fujinami RS. Antibody association with a novel model for primary progressive multiple sclerosis: induction of relapsing-remitting and progressive forms of EAE in H2s mouse strains. Brain Pathol. (2000) 10:402–18. doi: 10.1111/j.1750-3639.2000.tb00272.x
15. Tsunoda I, Kuang L-Q, Igenge IZ, Fujinami RS. Converting relapsing remitting to secondary progressive experimental allergic encephalomyelitis (EAE) by ultraviolet B irradiation. J Neuroimmunol. (2005) 160:122–34. doi: 10.1016/j.jneuroim.2004.11.007
16. Tsunoda I, Libbey JE, Kuang L-Q, Terry EJ, Fujinami RS. Massive apoptosis in lymphoid organs in animal models for primary and secondary progressive multiple sclerosis. Am J Pathol. (2005) 167:1631–46. doi: 10.1016/S0002-9440(10)61247-3
17. Libbey JE, Cusick MF, Tsunoda I, Fujinami RS. Antiviral CD8+ T cells cause an experimental autoimmune encephalomyelitis-like disease in naive mice. J Neurovirol. (2012) 18:45–54. doi: 10.1007/s13365-012-0077-2
18. Mendel I, Kerlero de Rosbo N, Ben-Nun A. A myelin oligodendrocyte glycoprotein peptide induces typical chronic experimental autoimmune encephalomyelitis in H-2b mice: fine specificity and T cell receptor Vβ expression of encephalitogenic T cells. Eur J Immunol. (1995) 25:1951–9. doi: 10.1002/eji.1830250723
19. Deng C, Minguela A, Hussain RZ, Lovett-Racke AE, Radu C, Ward ES, et al. Expression of the tyrosine phosphatase Src homology 2 domain-containing protein tyrosine phosphatase 1 determines T cell activation threshold and severity of experimental autoimmune encephalomyelitis. J Immunol. (2002) 168:4511–8. doi: 10.4049/jimmunol.168.9.4511
20. Satoh J, Nakanishi M, Koike F, Miyake S, Yamamoto T, Kawai M, et al. Microarray analysis identifies an aberrant expression of apoptosis and DNA damage-regulatory genes in multiple sclerosis. Neurobiol Dis. (2005) 18:537–50. doi: 10.1016/j.nbd.2004.10.007
21. Singh MK, Scott TF, LaFramboise WA, Hu FZ, Post JC, Ehrlich GD. Gene expression changes in peripheral blood mononuclear cells from multiple sclerosis patients undergoing beta-interferon therapy. J Neurol Sci. (2007) 258:52–9. doi: 10.1016/j.jns.2007.02.034
22. Kinter J, Zeis T, Schaeren-Wiemers N. RNA profiling of MS brain tissues. Int MS J. (2008) 15:51–8.
23. Baranzini SE, Bernard CCA, Oksenberg JR. Modular transcriptional activity characterizes the initiation and progression of autoimmune encephalomyelitis. J Immunol. (2005) 174:7412–22. doi: 10.4049/jimmunol.174.11.7412
24. Brand-Schieber E, Werner P, Iacobas DA, Iacobas S, Beelitz M, Lowery SL, et al. Connexin43, the major gap junction protein of astrocytes, is down-regulated in inflamed white matter in an animal model of multiple sclerosis. J Neurosci Res. (2005) 80:798–808. doi: 10.1002/jnr.20474
25. Gilgun-Sherki Y, Barhum Y, Atlas D, Melamed E, Offen D. Analysis of gene expression in MOG-induced experimental autoimmune encephalomyelitis after treatment with a novel brain-penetrating antioxidant. J Mol Neurosci. (2005) 27:125–35. doi: 10.1385/JMN:27:1:125
26. Roscoe WA, Welsh ME, Carter DE, Karlik SJ. VEGF and angiogenesis in acute and chronic MOG(35−55) peptide induced EAE. J Neuroimmunol. (2009) 209:6–15. doi: 10.1016/j.jneuroim.2009.01.009
27. Libbey JE, Tsunoda I, Fujinami RS. Studies in the modulation of experimental autoimmune encephalomyelitis. J Neuroimmune Pharmacol. (2010) 5:168–75. doi: 10.1007/s11481-010-9215-x
28. Tsunoda I, Kuang L-Q, Tolley ND, Whitton JL, Fujinami RS. Enhancement of experimental allergic encephalomyelitis (EAE) by DNA immunization with myelin proteolipid protein (PLP) plasmid DNA. J Neuropathol Exp Neurol. (1998) 57:758–67. doi: 10.1097/00005072-199808000-00005
29. Sato F, Kawai E, Martinez NE, Omura S, Park AM, Takahashi S, et al. T-bet, but not Gata3, overexpression is detrimental in a neurotropic viral infection. Sci Rep. (2017) 7:10496. doi: 10.1038/s41598-017-10980-0
30. McRae BL, Vanderlugt CL, Dal Canto MC, Miller SD. Functional evidence for epitope spreading in the relapsing pathology of experimental autoimmune encephalomyelitis. J Exp Med. (1995) 182:75–85. doi: 10.1084/jem.182.1.75
31. Martinez NE, Sato F, Kawai E, Omura S, Takahashi S, Yoh K, et al. Th17-biased RORγt transgenic mice become susceptible to a viral model for multiple sclerosis. Brain Behav Immun. (2014) 43:86–97. doi: 10.1016/j.bbi.2014.07.008
32. Boda E, Pini A, Hoxha E, Parolisi R, Tempia F. Selection of reference genes for quantitative real-time RT-PCR studies in mouse brain. J Mol Neurosci. (2009) 37:238–53. doi: 10.1007/s12031-008-9128-9
33. Martinez NE, Karlsson F, Sato F, Kawai E, Omura S, Minagar A, et al. Protective and detrimental roles for regulatory T cells in a viral model for multiple sclerosis. Brain Pathol. (2014) 24:436–51. doi: 10.1111/bpa.12119
34. Chen JJ, Wang S-J, Tsai C-A, Lin C-J. Selection of differentially expressed genes in microarray data analysis. Pharmacogenomics J. (2007) 7:212–20. doi: 10.1038/sj.tpj.6500412
35. Oberg AL, Mahoney DW, Eckel-Passow JE, Malone CJ, Wolfinger RD, Hill EG, et al. Statistical analysis of relative labeled mass spectrometry data from complex samples using ANOVA. J Proteome Res. (2008) 7:225–33. doi: 10.1021/pr700734f
36. Li W. Volcano plots in analyzing differential expressions with mRNA microarrays. J Bioinform Comput Biol. (2012) 10:1231003. doi: 10.1142/S0219720012310038
37. Omura S, Kawai E, Sato F, Martinez NE, Chaitanya GV, Rollyson PA, et al. Bioinformatics multivariate analysis determined a set of phase-specific biomarker candidates in a novel mouse model for viral myocarditis. Circ Cardiovasc Genet. (2014) 7:444–54. doi: 10.1161/CIRCGENETICS.114.000505
38. Davies DL, Bouldin DW. A cluster separation measure. IEEE Trans Pattern Anal Mach Intell. (1979) 1:224–7. doi: 10.1109/TPAMI.1979.4766909
39. Savli H, Szendröi A, Romics I, Nagy B. Gene network and canonical pathway analysis in prostate cancer: a microarray study. Exp Mol Med. (2008) 40:176–85. doi: 10.3858/emm.2008.40.2.176
40. Ringnér M. What is principal component analysis? Nat Biotechnol. (2008) 26:303–4. doi: 10.1038/nbt0308-303
41. Loke P, Favre D, Hunt PW, Leung JM, Kanwar B, Martin JN, et al. Correlating cellular and molecular signatures of mucosal immunity that distinguish HIV controllers from noncontrollers. Blood. (2010) 115:e20–32. doi: 10.1182/blood-2009-12-257451
42. Chaitanya GV, Omura S, Sato F, Martinez NE, Minagar A, Ramanathan M, et al. Inflammation induces neuro-lymphatic protein expression in multiple sclerosis brain neurovasculature. J Neuroinflamm. (2013) 10:125. doi: 10.1186/1742-2094-10-125
43. Pavlidis P, Noble WS. Analysis of strain and regional variation in gene expression in mouse brain. Genome Biol. (2001) 2:research0042. doi: 10.1186/gb-2001-2-10-research0042
44. Barrett T, Edgar R. Mining microarray data at NCBI's gene expression omnibus (GEO)*. Methods Mol Biol. (2006) 338:175–90. doi: 10.1385/1-59745-097-9:175
45. Lafaille JJ, Van de Keere F, Hsu AL, Baron JL, Haas W, Raine CS, et al. Myelin basic protein-specific T helper 2 (Th2) cells cause experimental autoimmune encephalomyelitis in immunodeficient hosts rather than protect them from the disease. J Exp Med. (1997) 186:307–12. doi: 10.1084/jem.186.2.307
46. Komiyama Y, Nakae S, Matsuki T, Nambu A, Ishigame H, Kakuta S, et al. IL-17 plays an important role in the development of experimental autoimmune encephalomyelitis. J Immunol. (2006) 177:566–73. doi: 10.4049/jimmunol.177.1.566
47. Martinez NE, Sato F, Omura S, Minagar A, Alexander JS, Tsunoda I. Immunopathological patterns from EAE and Theiler's virus infection: is multiple sclerosis a homogenous 1-stage or heterogenous 2-stage disease? Pathophysiology. (2013) 20:71–84. doi: 10.1016/j.pathophys.2012.03.003
48. Martinez NE, Sato F, Omura S, Kawai E, Takahashi S, Yoh K, et al. RORγ, but not T-bet, overexpression exacerbates an autoimmune model for multiple sclerosis. J Neuroimmunol. (2014) 276:142–9. doi: 10.1016/j.jneuroim.2014.09.006
49. Thompson PD, Tipney H, Brass A, Noyes H, Kemp S, Naessens J, et al. Claudin 13, a member of the claudin family regulated in mouse stress induced erythropoiesis. PLoS ONE. (2010) 5:e12667. doi: 10.1371/journal.pone.0012667
50. Bamezai A. Mouse Ly-6 proteins and their extended family: markers of cell differentiation and regulators of cell signaling. Arch Immunol Ther Exp. (2004) 52:255–66.
51. Koshland ME. Structure and function of the J chain. Adv Immunol. (1975) 20:41–69. doi: 10.1016/S0065-2776(08)60206-0
52. Ingram G, Loveless S, Howell OW, Hakobyan S, Dancey B, Harris CL, et al. Complement activation in multiple sclerosis plaques: an immunohistochemical analysis. Acta Neuropathol Commun. (2014) 2:53. doi: 10.1186/2051-5960-2-53
53. Kouser L, Madhukaran SP, Shastri A, Saraon A, Ferluga J, Al-Mozaini M, et al. Emerging and novel functions of complement protein C1q. Front Immunol. (2015) 6:317. doi: 10.3389/fimmu.2015.00317
54. Crispino JD, Horwitz MS. GATA factor mutations in hematologic disease. Blood. (2017) 129:2103–10. doi: 10.1182/blood-2016-09-687889
55. Löscher W, Potschka H. Blood-brain barrier active efflux transporters: ATP-binding cassette gene family. NeuroRx. (2005) 2:86–98. doi: 10.1602/neurorx.2.1.86
56. Schlessinger A, Matsson P, Shima JE, Pieper U, Yee SW, Kelly L, et al. Comparison of human solute carriers. Protein Sci. (2010) 19:412–28. doi: 10.1002/pro.320
57. Lee CG, Da Silva CA, Dela Cruz CS, Ahangari F, Ma B, Kang M-J, et al. Role of chitin and chitinase/chitinase-like proteins in inflammation, tissue remodeling, and injury. Annu Rev Physiol. (2011) 73:479–501. doi: 10.1146/annurev-physiol-012110-142250
58. Ang LS, Boivin WA, Williams SJ, Zhao H, Abraham T, Carmine-Simmen K, et al. Serpina3n attenuates granzyme B-mediated decorin cleavage and rupture in a murine model of aortic aneurysm. Cell Death Dis. (2011) 2:e209. doi: 10.1038/cddis.2011.88
59. Zhong D, He G, Zhao S, Li J, Lang Y, Ye W, et al. LRG1 modulates invasion and migration of glioma cell lines through TGF-β signaling pathway. Acta Histochem. (2015). doi: 10.1016/j.acthis.2015.05.001
60. Kamppinen AK, Saarela J. Expression Data From Peripheral Blood Mononuclear Cells in Multiple Sclerosis Patients and Controls. Gene Expression Omnibus. (2011). Available online at: https://www.ncbi.nlm.nih.gov/geo/query/acc.cgi?acc=GSE21942
61. Nakatsuji Y, Okuno T, Moriya M, Sugimoto T, Kinoshita M, Takamatsu H, et al. Sema4A, a Novel Serum Marker of Multiple Sclerosis, Implicates Th17 Pathology and Efficacy of Interferon-β. Gene Expression Omnibus (2011). Available online at: https://www.ncbi.nlm.nih.gov/geo/query/acc.cgi?acc=GSE26484
62. Weiner HL. The challenge of multiple sclerosis: how do we cure a chronic heterogeneous disease? Ann Neurol. (2009) 65:239–48. doi: 10.1002/ana.21640
63. Leray E, Yaouanq J, Le Page E, Coustans M, Laplaud D, Oger J, et al. Evidence for a two-stage disability progression in multiple sclerosis. Brain. (2010) 133:1900–13. doi: 10.1093/brain/awq076
64. Edan G, Leray E. A new treatment era in multiple sclerosis: clinical applications of new concepts. J Neurol Sci. (2011) 306:170–2. doi: 10.1016/j.jns.2010.09.018
65. Berard JL, Zarruk JG, Arbour N, Prat A, Yong VW, Jacques FH, et al. Lipocalin 2 is a novel immune mediator of experimental autoimmune encephalomyelitis pathogenesis and is modulated in multiple sclerosis. Glia. (2012) 60:1145–59. doi: 10.1002/glia.22342
66. Marques F, Mesquita SD, Sousa JC, Coppola G, Gao F, Geschwind DH, et al. Lipocalin 2 is present in the EAE brain and is modulated by natalizumab. Front Cell Neurosci. (2012) 6:33. doi: 10.3389/fncel.2012.00033
67. Huang J-J, Ma W-J, Yokoyama S. Expression and immunolocalization of Gpnmb, a glioma-associated glycoprotein, in normal and inflamed central nervous systems of adult rats. Brain Behav. (2012) 2:85–96. doi: 10.1002/brb3.39
68. Järve A, Mühlstedt S, Qadri F, Nickl B, Schulz H, Hübner N, et al. Adverse left ventricular remodeling by glycoprotein nonmetastatic melanoma protein B in myocardial infarction. FASEB J. (2017) 31:556–68. doi: 10.1096/fj.201600613R
69. Chen Y, Li R, Wu AM, Shu YQ, Lu ZQ, Hu XQ. The complement and immunoglobulin levels in NMO patients. Neurol Sci. (2014) 35:215–20. doi: 10.1007/s10072-013-1481-y
70. Lalor SJ, Segal BM. Lymphoid chemokines in the CNS. J Neuroimmunol. (2010) 224:56–61. doi: 10.1016/j.jneuroim.2010.05.017
71. Alvarez E, Piccio L, Mikesell RJ, Klawiter EC, Parks BJ, Naismith RT, et al. CXCL13 is a biomarker of inflammation in multiple sclerosis, neuromyelitis optica, and other neurological conditions. Mult Scler. (2013) 19:1204–8. doi: 10.1177/1352458512473362
72. Fischer MT, Sharma R, Lim JL, Haider L, Frischer JM, Drexhage J, et al. NADPH oxidase expression in active multiple sclerosis lesions in relation to oxidative tissue damage and mitochondrial injury. Brain. (2012) 135:886–899. doi: 10.1093/brain/aws012
73. Bilodeau M, MacRae T, Gaboury L, Laverdure J-P, Hardy M-P, Mayotte N, et al. Analysis of blood stem cell activity and cystatin gene expression in a mouse model presenting a chromosomal deletion encompassing Csta and Stfa2l1. PLoS ONE. (2009) 4:e7500. doi: 10.1371/journal.pone.0007500
74. Zimecki M, Kocięba M, Chodaczek G, Houszka M, Kruzel ML. Lactoferrin ameliorates symptoms of experimental encephalomyelitis in Lewis rats. J Neuroimmunol. (2007) 182:160–6. doi: 10.1016/j.jneuroim.2006.10.008
75. Abbitt KB, Cotter MJ, Ridger VC, Crossman DC, Hellewell PG, Norman KE. Antibody ligation of murine Ly-6G induces neutropenia, blood flow cessation, and death via complement-dependent and independent mechanisms. J Leukoc Biol. (2009) 85:55–63. doi: 10.1189/jlb.0507305
76. Ysrraelit MC, Correale J. Impact of sex hormones on immune function and multiple sclerosis development. Immunology. (2019) 156:9–22. doi: 10.1111/imm.13004
77. Oki S. Novel mechanism and biomarker of chronic progressive multiple sclerosis. Clin Exp Neuroimmunol. (2018) 9:25–34. doi: 10.1111/cen3.12449
78. Puentes F, van der Star BJ, Victor M, Kipp M, Beyer C, Peferoen-Baert R, et al. Characterization of immune response to neurofilament light in experimental autoimmune encephalomyelitis. J Neuroinflamm. (2013) 10:118. doi: 10.1186/1742-2094-10-118
79. Tsunoda I, Fujinami RS. Two models for multiple sclerosis: experimental allergic encephalomyelitis and Theiler's murine encephalomyelitis virus. J Neuropathol Exp Neurol. (1996) 55:673–86. doi: 10.1097/00005072-199606000-00001
80. Tsunoda I, Tanaka T, Terry EJ, Fujinami RS. Contrasting roles for axonal degeneration in an autoimmune versus viral model of multiple sclerosis: when can axonal injury be beneficial? Am J Pathol. (2007) 170:214–26. doi: 10.2353/ajpath.2007.060683
81. Frisullo G, Plantone D, Marti A, Iorio R, Damato V, Nociti V, et al. Type 1 immune response in progressive multiple sclerosis. J Neuroimmunol. (2012) 249:112–6. doi: 10.1016/j.jneuroim.2012.04.019
82. Gurevich M, Achiron A. The switch between relapse and remission in multiple sclerosis: Continuous inflammatory response balanced by Th1 suppression and neurotrophic factors. J Neuroimmunol. (2012) 252:83–8. doi: 10.1016/j.jneuroim.2012.07.014
83. Lassmann H, van Horssen J, Mahad D. Progressive multiple sclerosis: pathology and pathogenesis. Nat Rev Neurol. (2012) 8:647–56. doi: 10.1038/nrneurol.2012.168
84. Burioka N, Fukuoka Y, Takata M, Endo M, Miyata M, Chikumi H, et al. Circadian rhythms in the CNS and peripheral clock disorders: function of clock genes: influence of medication for bronchial asthma on circadian gene. J Pharmacol Sci. (2007) 103:144–9. doi: 10.1254/jphs.FMJ06003X4
85. Magklara A, Smith CL. A composite intronic element directs dynamic binding of the progesterone receptor and GATA-2. Mol Endocrinol. (2009) 23:61–73. doi: 10.1210/me.2008-0028
86. Murray CM, Hutchinson R, Bantick JR, Belfield GP, Benjamin AD, Brazma D, et al. Monocarboxylate transporter MCT1 is a target for immunosuppression. Nat Chem Biol. (2005) 1:371–6. doi: 10.1038/nchembio744
Keywords: multi-variate analysis, primary progressive EAE, principal component analysis (PCA), pattern matching, data mining
Citation: Omura S, Sato F, Martinez NE, Park A-M, Fujita M, Kennett NJ, Cvek U, Minagar A, Alexander JS and Tsunoda I (2019) Bioinformatics Analyses Determined the Distinct CNS and Peripheral Surrogate Biomarker Candidates Between Two Mouse Models for Progressive Multiple Sclerosis. Front. Immunol. 10:516. doi: 10.3389/fimmu.2019.00516
Received: 27 December 2018; Accepted: 26 February 2019;
Published: 19 March 2019.
Edited by:
Zsolt Illes, University of Southern Denmark, DenmarkReviewed by:
Michael Joseph Olek, Touro University Nevada, United StatesJorge Tolivia, Universidad de Oviedo, Spain
Copyright © 2019 Omura, Sato, Martinez, Park, Fujita, Kennett, Cvek, Minagar, Alexander and Tsunoda. This is an open-access article distributed under the terms of the Creative Commons Attribution License (CC BY). The use, distribution or reproduction in other forums is permitted, provided the original author(s) and the copyright owner(s) are credited and that the original publication in this journal is cited, in accordance with accepted academic practice. No use, distribution or reproduction is permitted which does not comply with these terms.
*Correspondence: Ikuo Tsunoda, itsunoda@med.kindai.ac.jp