- 1Department of Immunology, Erasmus MC, University Medical Centre Rotterdam, Rotterdam, Netherlands
- 2Department of Bioinformatics, Erasmus MC, University Medical Centre Rotterdam, Rotterdam, Netherlands
- 3Department of Pathology, Erasmus MC, University Medical Centre Rotterdam, Rotterdam, Netherlands
- 4Department of Internal Medicine, Erasmus MC, University Medical Centre Rotterdam, Rotterdam, Netherlands
Background: Primary Sjögren's syndrome (pSS) is a systemic autoimmune disease, where patients often suffer from fatigue. Biological pathways underlying fatigue are unknown. In this study aptamer-based SOMAscan technology is used to identify potential biomarkers and treatment targets for fatigue in pSS.
Methods: SOMAscan® Assay 1.3k was performed on serum samples of healthy controls (HCs) and pSS patients characterized for interferon upregulation and fatigue. Differentially expressed proteins (DEPs) between pSS patients and HC or fatigued and non-fatigued pSS patients were validated and discriminatory capacity of markers was tested using independent technology.
Results: Serum concentrations of over 1,300 proteins were compared between 63 pSS patients and 20 HCs resulting in 58 upregulated and 46 downregulated proteins. Additionally, serum concentrations of 30 interferon positive (IFNpos) and 30 interferon negative (IFNneg) pSS patients were compared resulting in 25 upregulated and 13 downregulated proteins. ELISAs were performed for several DEPs between pSS patients and HCs or IFNpos and IFNneg all showing a good correlation between protein levels measured by ELISA and relative fluorescence units (RFU) measured by the SOMAscan. Comparing 22 fatigued and 23 non-fatigued pSS patients, 16 serum proteins were differentially expressed, of which 14 were upregulated and 2 were downregulated. Top upregulated DEPs included neuroactive synaptosomal-associated protein 25 (SNAP-25), alpha-enolase (ENO1) and ubiquitin carboxyl-terminal hydrolase isozyme L1 (UCHL1). Furthermore, the proinflammatory mediator IL36a and several complement factors were upregulated in fatigued compared to non-fatigued pSS patients. ROC analysis indicated that DEPs showed good capacity to discriminate fatigued and non-fatigued pSS patients.
Conclusion: In this study we validated the use of aptamer-based proteomics and identified a novel set of proteins which were able to distinguish fatigued from non-fatigued pSS patients and identified a so-called “fatigue signature.”
Introduction
Primary Sjögren's syndrome (pSS) is a common systemic autoimmune disease, characterized by lymphocytic infiltrations in salivary and lachrymal glands. This is accompanied by sicca symptoms of the eyes and mouth and frequently also extraglandular manifestations (1–3). Fatigue is one of the most common extraglandular manifestation in pSS and is associated with a poor quality of life (4–9). Fatigue affects up to 70% of pSS patients, while ~20% of healthy adults are affected (10–13).
The biological basis of fatigue is largely unknown, however proinflammatory mechanisms are thought to play a role. Interferons (IFNs) are proinflammatory cytokines, which play a pivotal role in the pathogenesis of pSS and are systemically upregulated in 57% of the pSS patients (14). Elevated levels of IFNs induce increased expression of IFN-stimulated genes in the salivary glands, peripheral blood mononuclear cells (PBMCs), isolated monocytes and B cells of pSS patients (15–19). This so-called “IFN type I signature” is associated with higher disease activity and higher levels of autoantibodies. In addition, mutations affecting IFN signaling are observed in TREX, IRF5, STAT4, and PTPN22W and are associated with pSS (20–26). There is evidence for a link between IFNs and fatigue. Patients receiving IFNα treatment for viral hepatitis or melanoma can develop severe fatigue (27, 28). However, we and others have previously shown that there was no correlation between systemic upregulation of IFNs and fatigue in pSS patients (13, 29).
Because fatigue is a common problem in pSS, it is important to identify pathways underlying this fatigue. Here we use a proteomics approach to identify pathways related to fatigue. We used the aptamer-based SOMAscan technology, a highly multiplexed proteomic assay that queries 1,300 proteins in serum for protein biomarker discovery and identification of serum proteomic signatures and possible treatment targets for fatigue in pSS.
Methods
Patients and Methods
PSS patients and healthy controls (HC) were recruited at the Erasmus Medical Centre, Rotterdam, the Netherlands. All pSS patients fulfilled the 2002 American-European Consensus Group classification criteria (30) and were free of symptoms of viral infection at inclusion. HC did not suffer from autoimmune disease and did not use corticosteroids. Written informed consents were obtained from all participants in the study, in compliance with the Helsinki Declaration. Medical Ethical Review Committee of the Erasmus MC approved this study.
Blood Collection
Blood was collected in clotting tubes for serum preparation and in PAXgene RNA tubes (PreAnalytix, Hombrechtikon, Switzerland) for whole blood RNA analysis.
Real-Time Quantitative PCR and Calculation of IFN Score
Total RNA was isolated from PAXgene tubes and reverse-transcribed to cDNA. For calculation of relative expression, samples were normalized to expression of the household gene Abl [31]. Relative expression values were determined from normalized CT values using 2∧-ΔΔCT method (User Bulletin, Applied Biosystems). The IFN score was defined by the relative expression of 5 genes, IFI44, IFI44L, IFIT1, IFIT3, MXA. MeanHC and SDHC of each gene in the HC-group were used to standardize expression levels. IFN scores per subject represent the sum of these standardized scores, calculated as previously described (31, 32). Patients were divided in groups being positive or negative for the IFN score using a threshold of MeanHC + 2 x SDHC.
Proteomic Analysis of Serum Protein Concentrations
Serum protein concentrations were measured using the SOMAscan platform. SOMAscan utilizes single stranded DNA-based protein affinity reagents called SOMAmers (Slow Off-rate Modified Aptamers) (33, 34). The SOMAscan 1.3k kit was used following manufacturer's protocol, measuring over 1,300 proteins in 65 μl of serum. Intra-run normalization and inter-run calibration were performed according to SOMAscan assay data quality-control procedures as defined in the SomaLogic good laboratory practice quality system. Data from all samples passed quality-control criteria.
Measurement of Complement, Immunoglobulin Levels, and Autoantibodies
C3, C4, and IgG were measured as described previously (14). Anti-SSA and anti-SSB were determined by EliA (Thermo Fisher Scientific), confirmed with ANA profile immunoblot (EuroImmun) and re-confirmed where needed by QUANTA Lite ELISA-kit (INOVA).
Assessment of Fatigue and Depressive Symptoms
Fatigue was assessed using the Dutch version of the multidimensional fatigue inventory (MFI) (35). As a cut-off the 25 percentile highest (fatigued group) and lowest scores (non-fatigued group) were used resulting in the inclusion of 45 patients. The Dutch-validated Center for Epidemiologic Studies Depression (CES-D) was used to study depression and anxiety (36, 37).
Statistics
SOMAscan was performed to identify differences in quantitative binding of proteins to aptamers. Data were analyzed using empirical Bayes moderate t-test by the limma Bioconductor package in the R environment (38–40). P-values were corrected for multiple hypothesis testing using Benjamini-Hochberg method (FDR < 0.05). Differential expression was calculated on normalized log10 intensities. Visualization of differentially expressed proteins (DEPs) between pSS and HC and fatigued and non-fatigued pSS patients was based on 2Log transformed binding intensities and geometric means were calculated for HC and pSS patients.
Independent T-test was used to compare means and the Mann-Whitney U test was used to compare medians. Categorical data were compared using Fisher's exact test and correlations were assessed using Spearman's rho (rs). In order to determine the discriminatory capacity of markers receiver operating characteristics (ROC) curves and areas under the curves (AUCs) were calculated. Statistical analysis and visualization of DEP was performed using Instem/Omniviz, R version 3.4.3 bioconductor package limma version 3.34., IBM SPSS 24.0 (SPSS, Chicago, IL, USA) and Graphpad Prism 5.0 (Graphpad Software, La Jolla, CA, USA), 9.
Results
Differential Protein Expression in Serum of pSS and Interferon Positive Patients
Characteristics of pSS patients and HC are summarized in Supplementary Table 1. Using the SOMAscan multiplex proteomic assay, in total 104 serum proteins were differentially expressed between pSS patients and HCs after correction for multiple testing. Of these proteins 58 were upregulated and 46 were downregulated. A heatmap representing the most significant DEPs (2LogFC>0.5) is shown in Figure 1A and indicates a clear distinction between pSS and HCs (Figure 1A). Figure 1B shows in a volcano plot for the same DEPs. Top upregulated DEPs include Fcγ receptor 3B, a receptor binding immune complexes which are often observed in pSS, the interferon-inducible protein ISG15 and several female hormones including follicle-stimulating hormone (FSH) and human chorionic gonadotropin (HCG). A complete list of all significantly up- and downregulated proteins is shown in Supplementary Table 2.
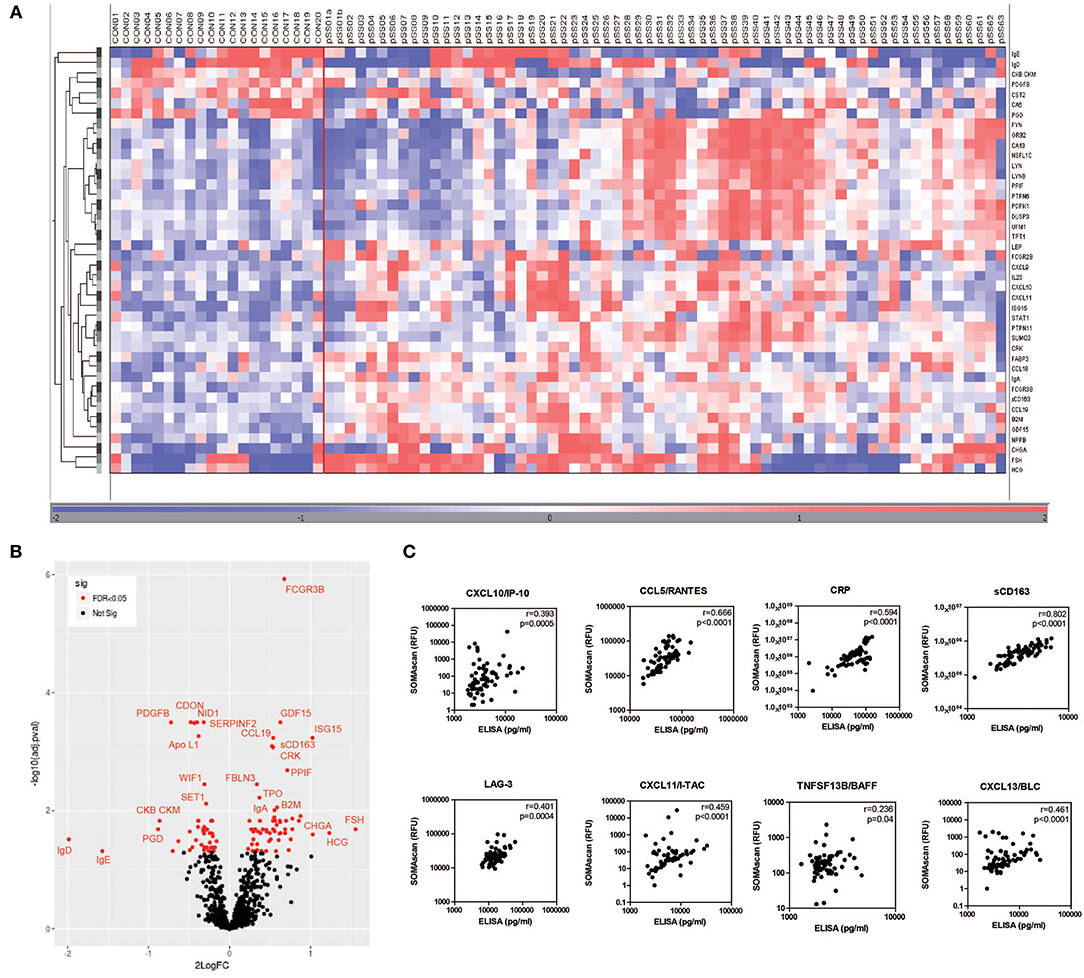
Figure 1. Differential protein expression in serum of pSS and healthy controls. Heatmap of differentially expressed proteins measured by SOMAscan technology in serum samples of pSS patients (pSS) (n = 63) and healthy controls (CON) (n = 20) clustered unsupervised within the groups (A) and volcano plot (B) visualizing the same DEPs. The correlation between RFU determined by SOMAscan and protein levels determined by ELISA is shown in (C).
Additionally, IFN positive (IFNpos) and IFN negative (IFNneg) pSS patients were compared. Characteristics of IFNpos and IFNneg pSS are summarized in Supplementary Table 3. IFNpos patients showed significantly higher IgG levels, higher frequency of anti-SSA and anti-SSB autoantibodies and lower C3 complement levels compared to IFNneg patients. Comparing protein expression between IFNpos and IFNneg patients 38 proteins were DEPs of which 25 were upregulated and 13 were downregulated. As expected many interferon-inducible proteins were found upregulated including ISG15, CXCL11/I-TAC, CXCL10/IP-10, OAS1, and TNFSF13B/BAFF. Volcano plots and a full list of all significantly up- and downregulated proteins between IFNpos and IFNneg pSS patients is shown in Supplementary Figure 1 and Supplementary Table 4.
To validate the SOMAscan data, ELISAs were performed for several DEPs between pSS patients and HCs or IFNpos and IFNneg pSS patients. Proteins for validation were selected based upon availability of reliable ELISAs. The selected proteins included CXCL10/IP-10, CCL5/RANTES, CRP, sCD163, LAG-3, CXCL11/I-TAC, TNFSF13B/BAFF, and CXCL13/BLC. All protein levels measured by ELISA significantly correlated with relative fluorescence units (RFUs) determined by the SOMAscan (Figure 1C).
Fatigued pSS Patients Are Characterized by a Differential Serum Protein Expression Pattern
Characteristics of fatigued and non-fatigued pSS patients are summarized in Table 1. In total 16 serum proteins were differentially expressed between fatigued and non-fatigued pSS patients, of which 14 were upregulated and 2 were downregulated in fatigued patients. Top upregulated DEPs included neuroactive synaptosomal-associated protein 25 (SNAP-25), alpha-enolase (ENO1) and ubiquitin carboxyl-terminal hydrolase isozyme L1 (UCHL1). Furthermore, the proinflammatory mediator IL36a and several complement factors were upregulated in fatigued compared to non-fatigued pSS patients. A heatmap representing the DEPs is shown in Figure 2A. When unsupervised clustering of patients was performed 15 of the 22 fatigued patients (~68%) clustered together and only one non-fatigued patients clustered with this group. This grouping of fatigued pSS patients indicated a signature for fatigue in pSS. A volcano plot of the DEPs is shown in Figure 2B and a full list of all DEPs, Log Fold changes and (adjusted) p-values is depicted in Table 2 and Supplementary Table 5.
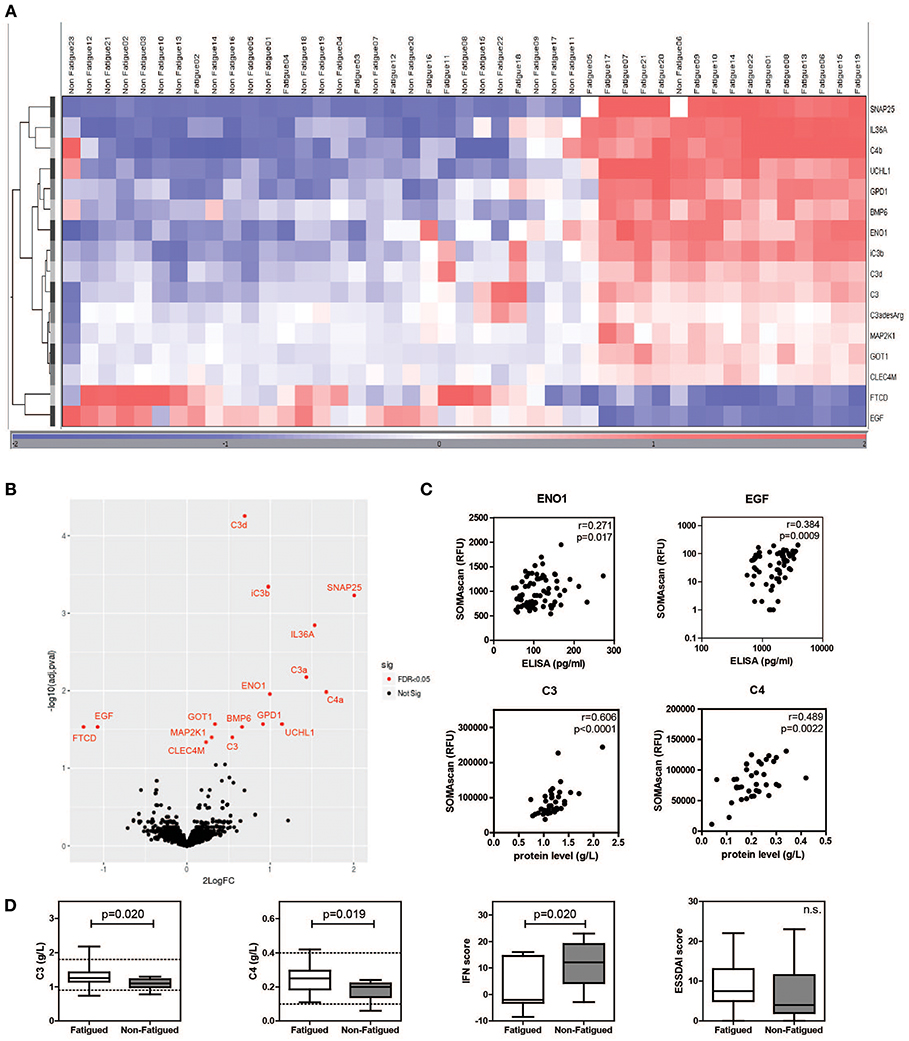
Figure 2. Differential protein expression in serum of fatigued pSS patients. Heatmap showing the unsupervised clustering of differentially expressed proteins between fatigued (n = 22) and non-fatigued (n = 23) pSS patients (A) and volcano plot (B) visualizing the same DEPs. (C) Correlation between RFU determined by SOMAscan and protein levels determined by ELISA (for ENO1 and EGF) and Immage nephelometer (for C3 and C4). (D) Comparison of complement levels, IFN and ESSDAI score between fatigued and non-fatigued pSS patients.
As hydroxychloroquine (HCQ) is sometimes used to treat fatigue, differential protein expression was additionally determined after exclusion of patients who used HCQ. No differences were found compared to the analyses including HCQ users (data not shown). Additionally, dimensions of fatigue were compared between HCQ users and non-users and no differences were observed (Supplementary Figure 2).
The SOMAscan data of DEPs were validated by ELISAs when these were available. ELISAs for ENO1 and epidermal growth factor (EGF) showed significant correlations with RFUs determined by SOMAscan (Figure 2C). In addition, C3 and C4 RFUs were compared to C3 and C4 complement levels determined through routine diagnostics at the Erasmus MC, clinical chemistry lab by Immage nephelometer. Proteins selected for validation showed good correlation with protein levels determined by ELISA and Immage nephelometer. Fatigued pSS patients showed higher complement levels and lower IFN scores than non-fatigued patients (Figure 2D). There was no difference in European League Against Rheumatism Sjögren's Syndrome Disease Activity Index (ESSDAI) score, although there was a trend toward higher ESSDAI scores in the fatigued patients, which had higher scores in the articular and pulmonary domain (data not shown).
Predictive Value of Markers for Fatigue in pSS
The predictive potential of the proteins found to be differentially expressed between fatigued and non-fatigued pSS patients was studied. In order to do this ROC curves were calculated for the most DEPs (2LogFC>1) including SNAP25, complement factors C4a/C4b and C3a, IL36a, UCHL1, ENO1, EGF and formimidoyltransferase-cyclodeaminase (FTCD) (Figure 3A). Additionally, the corresponding boxplots are shown (Figure 3B). ROC analysis yielded AUC values between 0.752 and 0.845 confirming a robust discriminatory capacity between fatigued from non-fatigued patients pSS patients using these proteins (Table 3).
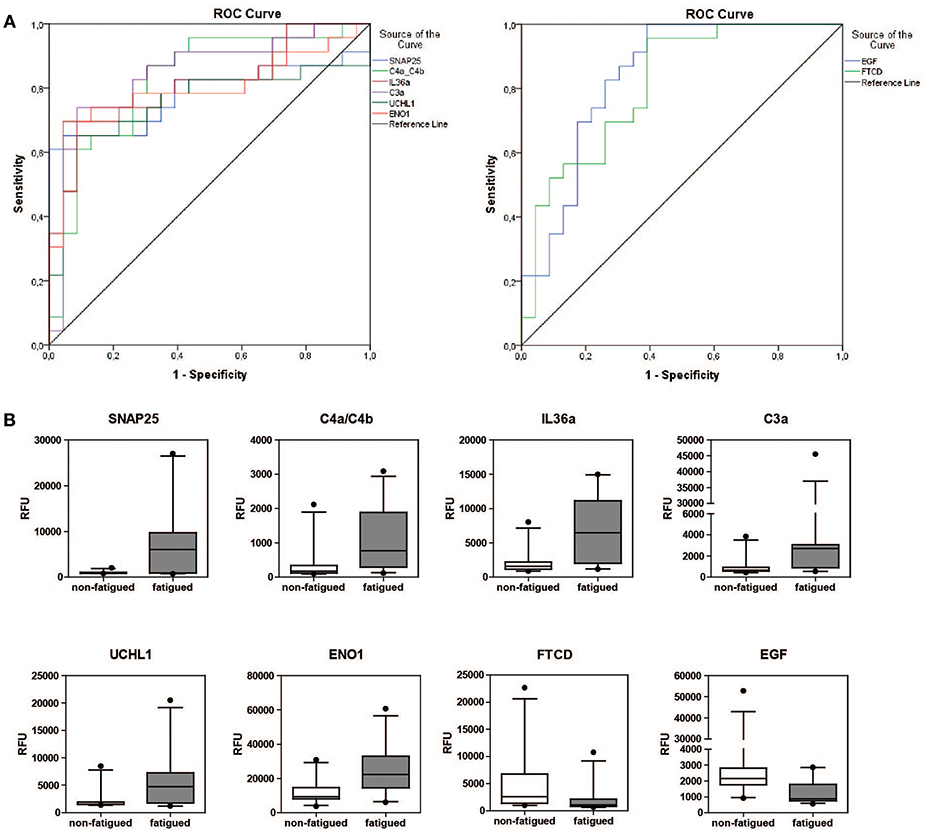
Figure 3. Discriminatory capacity of markers for fatigue in pSS. (A) ROC curves of positively and negatively predictive proteins (2LogFC>1) for fatigue in pSS. (B) Boxplots of differentially expressed proteins between fatigued (n = 22) and non-fatigued (n = 23) pSS patients.
Discussion
PSS is a heterogeneous disease with complex pathogenesis. Traditional proteomic approaches of lachrymal or salivary fluids have shown increased expression of inflammatory and immune response-related proteins (41). Furthermore, gene expression profiling of pSS blood also revealed systemic upregulation of immune related pathways, like the IFN pathway and B cell receptor signaling pathway (18, 42, 43). Using SOMAscan technology we were able to identify upregulation of similar pathways as described using other proteomic techniques. To our knowledge one other study used SOMAscan technology to study pSS (44), although a more limited number of proteins were measured. Nishikawa et al. identified several DEPs in serum of pSS patients compared to serum of HCs and DEPs were linked to disease activity measured by ESSDAI score. When we compared pSS patients with HC we identified sets of upregulated proteins such as CD163, CXCL10, TNFSF15, FSH, CXCL11, and β2-microglobulin, that were in agreement with previously published data (44). In summary, we identified similar upregulated pathways as identified with other microarray platforms (15–19) and found similar upregulated proteins with the same technique in a different cohort of pSS patients (44) indicating the SOMAscan technology as a reliable method for the discovery of biomarkers for fatigue in pSS.
Fatigue is the most prevalent extraglandular symptom in pSS of which we do not know the biological basis. Since fatigue is often seen in conditions where the immune system is dysregulated, proinflammatory mechanisms have been thought to play a role. Previous attempts, however, to find a link between proinflammatory signatures in serum or tissue and fatigue have failed (13, 29, 45). Previous studies even showed decreasing levels of several proinflammatory cytokines like IP-10/CXCL10, TNFα, LTα, and IFNγ in fatigued pSS patients (13). Furthermore, we previously described a negative trend between IFNs and fatigue (29). In our current multiplexed proteomic analysis we show the coordinated upregulation of a set of proteins of which some are involved in inflammation including IL36a and complement factors. IL36a is a pro-inflammatory cytokine belonging to the IL-1 family and induces maturation of dendritic cells and drives Th1 and Th17 responses in CD4+ T cells (46). This cytokine was previously shown to be overexpressed in the salivary glands and serum of pSS patients (47). Upregulation of this cytokine is also seen in other diseases like psoriasis, rheumatoid arthritis, systemic lupus erythematosus, inflammatory bowel disease and fibromyalgia (46, 48, 49). In addition to IL36a, several complement factors were upregulated in fatigued pSS patients compared to non-fatigued patients. Quantification of the complement levels, however, showed that all values were in the normal range, but the non-fatigued patients lean toward reduced complement levels. Reduced complement levels are often associated with more severe disease manifestations, vasculitis, and lymphoma in pSS (50).
Interestingly, among the “fatigue-signature“ proteins were several proteins which have functions in the brain like SNAP-25, UCHL1, and ENO1. SNAP-25 protein is a SNARE protein, critical in neurotransmitter release (51). Aberrancies in this protein are described in several neurological, cognitive and psychological disorders like Alzheimer's disease and fibromyalgia (52–55). Also UCHL1 is particularly abundant in the brain, where it is critical for proper function of the ubiquitin-proteasome system in neurons (56). Reduced levels of this gene have also been linked to among others Parkinson and Alzheimer's disease (56–59). ENO1 is a glycolytic enzyme which can be expressed in the brain, but other tissues can also express this protein and it has a wide variety of functions [reviewed in (60)]. This protein has also been implicated in Alzheimer's disease. Interestingly, data indicate that ENO1 acts as an autoantigen in several autoimmune diseases. Antibodies against ENO1 have been described in Hashimoto's encephalopathy, Behçet's disease, Crohn's disease, rheumatoid arthritis (61–65) Recently, antibodies against citrullinated ENO1 (Anti-CEP-1) peptides have also been observed in pSS (66) and this raises the question if such autoantibodies associate with fatigue. Although aberrancies in all these proteins have been linked to a variety of conditions they have never been described in the context of fatigue.
EGF and FTCD were both significantly reduced in fatigued patients compared to non-fatigued patients. EGF is found in many secretions including saliva. After binding to the EGF receptor it regulates epithelial cell proliferation and survival and therefore is thought to have protective effects. EGF has previously been shown to be reduced in tears (67), salivary glands (68, 69) and saliva of pSS patients and correlates with progression of intraoral manifestations (70, 71). FTCD is a metabolic enzyme, which is primary active in the liver and kidneys. However, recently a study described additional neurological effects (72). So far none of these proteins have been linked to fatigue.
Glycerol-3-phosphate dehydrogenase [NAD(+)] (GPD1), bone morphogenetic protein 6 (BMP6), aspartate aminotransferase (GOT1), dual specificity mitogen-activated protein kinase kinase 1 (MAP2K1) and C-type lectin domain family 4 member M (CLEC4M) were additionally found slightly elevated in fatigued pSS patients compared to non-fatigued patients. These proteins have a variety of metabolic and immunological functions and GPD1, BMP6 and GOT1 also have functions in the brain. However, it is unclear how these proteins could contribute to fatigue.
Recently, proteomics performed on CSF revealed a signature for fatigue in pSS patients (73). In this abstract they describe similar as in our study upregulation of molecules in the complement system. Overall most discriminatory proteins between fatigued and non-fatigued pSS patients were involved in innate immunity, cellular stress defense and/or function in the central nervous system. It would be interesting to compare the proteins found differentially expressed in the CSF of fatigued pSS patients with the proteins we found in the serum.
A limitation of this study is that we were not able to validate all DEPs between fatigued and non-fatigued patients because there were no sensitive ELISAs available for these proteins. However, in this study we showed that when ELISAs were available, DEPs identified by SOMAscan showed good correlation with protein levels measured using different techniques indicating the reliability of the technology. Another limitation of this study is the cross-sectional design and limited number of patients in the fatigue vs. non-fatigue comparison. Furthermore, there could be underlying confounding comorbidities leading to fatigue in some patients.
Conclusion
In this study we validated the use of aptamer-based multiplex proteomics and identified a novel set of proteins which were able to distinguish fatigued from non-fatigued pSS patients and identified a so-called “fatigue-signature.” Overall these proteins were involved in inflammatory mechanisms and have neurological and metabolic functions. More studies are necessary to validate these proteins as markers for fatigue in pSS.
Data Availability
The raw data supporting the conclusions of this manuscript will be made available by the authors, without undue reservation, to any qualified researcher. For this the corresponding author can be contacted.
Author Contributions
IB, MS, PK, and MV conceived and designed the study. IB, AW, and CvH-M performed the experiments. IB, LL, MS, PK, and PvdS contributed to the data analyses and interpretation. LT and PvD were involved in clinical data acquisition. IB, PvdS, and MV wrote the manuscript.
Funding
This work was supported by a grant from the Dutch Arthritis Foundation (Reumafonds) [14-3-404], and funding from ImmunAID project of the European Union's Horizon 2020 research and innovation programme (grant agreement No 779295) and the Department of Immunology, Erasmus MC, University Medical Center.
Conflict of Interest Statement
The authors declare that the research was conducted in the absence of any commercial or financial relationships that could be construed as a potential conflict of interest.
Acknowledgments
The research for this manuscript was (partly) performed within the framework of the Erasmus Postgraduate School Molecular Medicine. The authors thank patients and healthy volunteers for taking part in this study.
Supplementary Material
The Supplementary Material for this article can be found online at: https://www.frontiersin.org/articles/10.3389/fimmu.2019.00312/full#supplementary-material
Supplementary Figure 1. Volcano plot of differentially expressed proteins between interferon positive (n = 30) and interferon negative (n = 30) pSS patients.
Supplementary Figure 2. Comparison of dimensions of fatigue measured by the multiple fatigue inventory questionnaire between HCQ (n = 40) and non-HCQ (n = 22) treated pSS patients.
Supplementary Table 1. Characteristics of patients and healthy controls.
Supplementary Table 2. Differentially expressed serum proteins between pSS patients and HC.
Supplementary Table 3. Characteristics of IFNpos and IFNneg pSS patients.
Supplementary Table 4. Differentially expressed serum proteins between IFNpos and IFNneg pSS patients.
Supplementary Table 5. Differentially expressed serum proteins between fatigued and non-fatigued pSS patients.
References
1. Gabriel SE, Michaud K. Epidemiological studies in incidence, prevalence, mortality, and comorbidity of the rheumatic diseases. Arthritis Res Ther. (2009) 11:1–16. doi: 10.1186/ar2669
2. Fox RI, Howell FV, Bone RC, Michelson PE. Primary sjogren syndrome: clinical and immunopathologic features. Semin Arthritis Rheum. (1984) 14:77–105. doi: 10.1016/0049-0172(84)90001-5
3. Asmussen K, Andersen V, Bendixen G, Schiødt M, Oxholm P. A new model for classification of disease manifestations in primary Sjögren's syndrome: evaluation in a retrospective long-term study. J Intern Med. (1996) 239:475–82. doi: 10.1046/j.1365-2796.1996.418817000.x
4. Champey J, Corruble E, Gottenberg J-E, Buhl C, Meyer T, Caudmont C, et al. Quality of life and psychological status in patients with primary Sjögren's syndrome and sicca symptoms without autoimmune features. Arthritis Rheum. (2006) 55:451–7. doi: 10.1002/art.21990
5. Segal B, Thomas W, Rogers T, Leon JM, Hughes P, Patel D, et al. Prevalence, severity and predictors of fatigue in primary sjogren's syndrome. Arthritis Rheum. (2008) 59:1780–7. doi: 10.1002/art.24311
6. Haldorsen K, Bjelland I, Bolstad AI, Jonsson R, Brun JG. A five-year prospective study of fatigue in primary Sjögren's syndrome. Arthritis Res Ther. (2011) 13:1–8. doi: 10.1186/ar3487
7. Theander L, Strömbeck B, Mandl T, Theander E. Sleepiness or fatigue? can we detect treatable causes of tiredness in primary Sjögren's syndrome? Rheumatology (2010) 49:1177–83. doi: 10.1093/rheumatology/keq023
8. Karageorgas T, Fragioudaki S, Nezos A, Karaiskos D, Moutsopoulos HM, Mavragani CP. Fatigue in primary Sjögren's syndrome: clinical, laboratory, psychometric, and biologic associations. Arthritis Care Res. (2016) 68:123–31. doi: 10.1002/acr.22720
9. Overman CL, Kool MB, Da Silva JAP, Geenen R. The prevalence of severe fatigue in rheumatic diseases: an international study. Clin Rheumatol. (2016) 35:409–15. doi: 10.1007/s10067-015-3035-6
10. Chen MK. The epidemiology of self-perceived fatigue among adults. Prev Med. (1986) 15:74–81. doi: 10.1016/0091-7435(86)90037-X
11. Loge JH, Ekeberg Ø, Kaasa S. Fatigue in the general norwegian population: normative data and associations. J Psychosom Res. (1998) 45:53–65. doi: 10.1016/S0022-3999(97)00291-2
12. Lerdal A, Wahl AK, Rustoen T, Hanestad BR, Moum T. Fatigue in the general population: a translation and test of the psychometric properties of the Norwegian version of the fatigue severity scale. Scand J Public Health (2005) 33:123–30. doi: 10.1080/14034940410028406
13. Howard Tripp N, Tarn J, Natasari A, Gillespie C, Mitchell S, Hackett KL, et al. Fatigue in primary Sjögren's syndrome is associated with lower levels of proinflammatory cytokines. RMD Open (2016) 2:e000282. doi: 10.1136/rmdopen-2016-000282
14. Brkic Z, Maria NI, Van Helden-Meeuwsen CG, Van De Merwe JP, Van Daele PL, Dalm VA, et al. Prevalence of interferon type I signature in CD14 monocytes of patients with Sjogren's syndrome and association with disease activity and BAFF gene expression. Ann Rheum Dis. (2013) 72:728–35. doi: 10.1136/annrheumdis-2012-201381
15. Wildenberg ME, Van Helden-Meeuwsen CG, Van De Merwe JP, Drexhage HA, Versnel MA. Systemic increase in type I interferon activity in Sjögren's syndrome: a putative role for plasmacytoid dendritic cells. Eur J Immunol. (2008) 38:2024–33. doi: 10.1002/eji.200738008
16. Gottenberg JE, Cagnard N, Lucchesi C, Letourneur F, Mistou S, Lazure T, et al. Activation of IFN pathways and plasmacytoid dendritic cell recruitment in target organs of primary Sjögren's syndrome. Proc Natl Acad Sci USA. (2006) 103:2770–5. doi: 10.1073/pnas.0510837103
17. Hjelmervik TOR, Petersen K, Jonassen I, Jonsson R, Bolstad AI. Gene expression profiling of minor salivary glands clearly distinguishes primary Sjögren's syndrome patients from healthy control subjects. Arthritis Rheum. (2005) 52:1534–44. doi: 10.1002/art.21006
18. Emamian ES, Leon JM, Lessard CJ, Grandits M, Baechler EC, Gaffney PM, et al. Peripheral blood gene expression profiling in Sjogren's syndrome. Genes Immun. (2009) 10:285–96. doi: 10.1038/gene.2009.20
19. Imgenberg-Kreuz J, Sandling JK, Almlöf JC, Nordlund J, Signér L, Norheim KB, et al. Genome-wide DNA methylation analysis in multiple tissues in primary Sjögren's syndrome reveals regulatory effects at interferon-induced genes. Ann Rheum Dis. (2016) 75:2029–36. doi: 10.1136/annrheumdis-2015-208659
20. Barizzone N, Monti S, Mellone S, Godi M, Marchini M, Scorza R, et al. Rare Variants in the TREX1 Gene and susceptibility to autoimmune diseases. Biomed Res Int. (2013) 2013:6. doi: 10.1155/2013/471703
21. Miceli-Richard C, Comets E, Loiseau P, Puechal X, Hachulla E, Mariette X. Association of an IRF5 gene functional polymorphism with Sjögren's syndrome. Arthritis Rheum. (2007) 56:3989–94. doi: 10.1002/art.23142
22. Nordmark G, Kristjansdottir G, Theander E, Eriksson P, Brun JG, Wang C, et al. Additive effects of the major risk alleles of IRF5 and STAT4 in primary Sjögren's syndrome. Genes Immun. (2008) 10:68–76. doi: 10.1038/gene.2008.94
23. Miceli-Richard C, Gestermann N, Ittah M, Comets E, Loiseau P, Puechal X, et al. The CGGGG insertion/deletion polymorphism of the IRF5 promoter is a strong risk factor for primary Sjögren's syndrome. Arthritis Rheum. (2009) 60:1991–7. doi: 10.1002/art.24662
24. Korman BD, Alba MI, Le JM, Alevizos I, Smith JA, Nikolov NP, et al. Variant form of STAT4 is associated with primary Sjögren's syndrome. Genes Immun. (2008) 9:267. doi: 10.1038/gene.2008.1
25. Gestermann N, Mekinian A, Comets E, Loiseau P, Puechal X, Hachulla E, et al. STAT4 is a confirmed genetic risk factor for Sjögren's syndrome and could be involved in type 1 interferon pathway signaling. Genes Immun. (2010) 11:432–8. doi: 10.1038/gene.2010.29.
26. Vlachogiannis NI, Nezos A, Tzioufas AG, Koutsilieris M, Moutsopoulos HM, Mavragani CP. Increased frequency of the PTPN22W* variant in primary Sjogren's syndrome: association with low type I IFN scores. Clin Immunol. (2016) 173:157–60. doi: 10.1016/j.clim.2016.10.015
27. Udina M, Castellvi P, Moreno-Espana J, Navines R, Valdes M, Forns X, et al. Interferon-induced depression in chronic hepatitis C: a systematic review and meta-analysis. J Clin Psychiatry (2012) 73:1128–38. doi: 10.4088/JCP.12r07694
28. Andrew B, Corneel C, Stefan S, Mario S, Willem K, Alessandro T, et al. Adjuvant therapy with pegylated interferon alfa-2b versus observation in resected stage III melanoma: a phase III randomized controlled trial of health-related quality of life and symptoms by the European organisation for research and treatment of cancer melanoma group. J Clin Oncol. (2009) 27:2916–23. doi: 10.1200/JCO.2008.20.2069
29. Bodewes ILA, Al-Ali S, Van Helden-Meeuwsen CG, Maria NI, Tarn J, Lendrem DW, et al. Systemic interferon type I and type II signatures in primary Sjögren's syndrome reveal differences in biological disease activity. Rheumatology (2018) 57:921–30. doi: 10.1093/rheumatology/kex490
30. Vitali C, Bombardieri S, Jonsson R, Moutsopoulos HM, Alexander EL, Carsons SE, et al. Classification criteria for Sjögren's syndrome: a revised version of the European criteria proposed by the American-European Consensus Group. Ann Rheum Dis. (2002) 61:554–8. doi: 10.1136/ard.61.6.554
31. Kirou KA, Lee C, George S, Louca K, Papagiannis IG, Peterson MGE, et al. Coordinate overexpression of interferon-α-induced genes in systemic lupus erythematosus. Arthritis Rheum. (2004) 50:3958–67. doi: 10.1002/art.20798
32. Feng X, Wu H, Grossman JM, Hanvivadhanakul P, Fitzgerald JD, Park GS, et al. Association of increased interferon-inducible gene expression with disease activity and lupus nephritis in patients with systemic lupus erythematosus. Arthritis Rheum. (2006) 54:2951–62. doi: 10.1002/art.22044
33. Davies DR, Gelinas AD, Zhang C, Rohloff JC, Carter JD, O'connell D, et al. Unique motifs and hydrophobic interactions shape the binding of modified DNA ligands to protein targets. Proc Natl Acad Sci USA. (2012) 109:19971–6. doi: 10.1073/pnas.1213933109
34. Rohloff JC, Gelinas AD, Jarvis TC, Ochsner UA, Schneider DJ, Gold L, et al. Nucleic acid ligands with protein-like side chains: modified aptamers and their use as diagnostic and therapeutic agents. Mol Ther Nucleic Acids (2014) 3:e201. doi: 10.1038/mtna.2014.49
35. Smets EM, Garssen B, Bonke B, De Haes JC. The Multidimensional Fatigue Inventory (MFI) psychometric qualities of an instrument to assess fatigue. J Psychosom Res. (1995) 39:315–25. doi: 10.1016/0022-3999(94)00125-O
36. Zigmond AS, Snaith RP. The hospital anxiety and depression scale. Acta Psychiatr Scand. (1983) 67:361–70. doi: 10.1111/j.1600-0447.1983.tb09716.x
37. Schroevers MJ, Sanderman R, Van Sonderen E, Ranchor AV. The evaluation of the Center for Epidemiologic Studies Depression (CES-D) scale: depressed and positive affect in cancer patients and healthy reference subjects. Qual Life Res. (2000) 9:1015–29. doi: 10.1023/A:1016673003237
38. Billing AM, Ben Hamidane H, Bhagwat AM, Cotton RJ, Dib SS, Kumar P, et al. Complementarity of SOMAscan to LC-MS/MS and RNA-seq for quantitative profiling of human embryonic and mesenchymal stem cells. J Proteomics (2017) 150:86–97. doi: 10.1016/j.jprot.2016.08.023
39. Sattlecker M, Kiddle SJ, Newhouse S, Proitsi P, Nelson S, Williams S, et al. Alzheimer's disease biomarker discovery using SOMAscan multiplexed protein technology. Alzheimer's Dement. (2014) 10:724–34. doi: 10.1016/j.jalz.2013.09.016
40. Candia J, Cheung F, Kotliarov Y, Fantoni G, Sellers B, Griesman T, et al. Assessment of variability in the SOMAscan assay. Sci Rep. (2017) 7:14248. doi: 10.1038/s41598-017-14755-5
41. Baldini C, Cecchettini A, Gallo A, Bombardieri S. Updates on Sjögren's syndrome: from proteomics to protein biomarkers. Expert Rev Proteomics (2017) 14:491–8. doi: 10.1080/14789450.2017.1333904
42. Imgenberg-Kreuz J, Sandling JK, Björk A, Nordlund J, Kvarnström M, Eloranta ML, et al. Transcription profiling of peripheral B cells in antibody-positive primary Sjögren's syndrome reveals upregulated expression of CX3CR1 and a type I and type II interferon signature. Scand J Immunol. (2018) 87:e12662. doi: 10.1111/sji.12662
43. Song GG, Kim J-H, Seo YH, Choi SJ, Ji JD, Lee YH. Meta-analysis of differentially expressed genes in primary Sjogren's syndrome by using microarray. Hum Immunol. (2014) 75:98–104. doi: 10.1016/j.humimm.2013.09.012
44. Nishikawa A, Suzuki K, Kassai Y, Gotou Y, Takiguchi M, Miyazaki T, et al. Identification of definitive serum biomarkers associated with disease activity in primary Sjögren's syndrome. Arthritis Res Ther. (2016) 18:106. doi: 10.1186/s13075-016-1006-1
45. James K, Al-Ali S, Tarn J, Cockell SJ, Gillespie CS, Hindmarsh V, et al. A transcriptional signature of fatigue derived from patients with primary Sjögren's syndrome. PLoS ONE (2015) 10:e0143970. doi: 10.1371/journal.pone.0143970
46. Ding L, Wang X, Hong X, Lu L, Liu D. IL-36 cytokines in autoimmunity and inflammatory disease. Oncotarget (2018) 9:2895–901. doi: 10.18632/oncotarget.22814
47. Ciccia F, Accardo-Palumbo A, Alessandro R, Alessandri C, Priori R, Guggino G, et al. Interleukin-36α axis is modulated in patients with primary Sjögren's syndrome. Clin Exp Immunol. (2015) 181:230–8. doi: 10.1111/cei.12644
48. Frey S, Derer A, Messbacher M-E, Baeten DLP, Bugatti S, Montecucco C, et al. The novel cytokine interleukin-36α is expressed in psoriatic and rheumatoid arthritis synovium. Ann Rheum Dis. (2013) 72:1569–74. doi: 10.1136/annrheumdis-2012-202264
49. Jones KD, Gelbart T, Whisenant TC, Waalen J, Mondala TS, Iklé DN, et al. Genome-wide expression profiling in the peripheral blood of patients with fibromyalgia. Clin Exp Rheumatol. (2016) 34:89–98.
50. Solans-Laqu,é R, López-Hernandez A, Angel Bosch-Gil J, Palacios A, Campillo M, Vilardell-Tarres M. Risk, predictors, and clinical characteristics of lymphoma development in primary Sjögren's syndrome. Semin Arthritis Rheum. (2011) 41:415–423. doi: 10.1016/j.semarthrit.2011.04.006
51. Hu C, Ahmed M, Melia TJ, Söllner TH, Mayer T, Rothman JE. Fusion of cells by flipped SNAREs. Science (2003) 300:1745–9. doi: 10.1126/science.1084909
52. Balkarli A, Sengül C, Tepeli E, Balkarli H, Cobankara V. Synaptosomal-associated protein 25 (Snap-25) gene polymorphism frequency in fibromyalgia syndrome and relationship with clinical symptoms. BMC Musculoskelet Disord. (2014) 15:191. doi: 10.1186/1471-2474-15-191
53. Faraone SV, Perlis RH, Doyle AE, Smoller JW, Goralnick JJ, Holmgren MA, et al. Molecular genetics of attention-deficit/hyperactivity disorder. Biol Psychiatry (2005) 57:1313–23. doi: 10.1016/j.biopsych.2004.11.024
54. Corradini I, Verderio C, Sala M, Wilson MC, Matteoli M. SNAP-25 in neuropsychiatric disorders. Ann N Y Acad Sci. (2009) 1152:93–9. doi: 10.1111/j.1749-6632.2008.03995.x
55. Brinkmalm A, Brinkmalm G, Honer WG, Frölich L, Hausner L, Minthon L, et al. SNAP-25 is a promising novel cerebrospinal fluid biomarker for synapse degeneration in Alzheimer's disease. Mol Neurodegener. (2014) 9:53. doi: 10.1186/1750-1326-9-53
56. Jara JH, Genç B, Cox GA, Bohn MC, Roos RP, Macklis JD, et al. Corticospinal motor neurons are susceptible to increased ER stress and display profound degeneration in the absence of UCHL1 function. Cereb Cortex (2015) 25:4259–72. doi: 10.1093/cercor/bhu318
57. Cartier AE, Ubhi K, Spencer B, Vazquez-Roque RA, Kosberg KA, Fourgeaud L, et al. Differential effects of UCHL1 modulation on alpha-synuclein in PD-like models of alpha-synucleinopathy. PLoS ONE (2012) 7:e34713. doi: 10.1371/journal.pone.0034713
58. Alves-Rodrigues A, Gregori L, Figueiredo-Pereira ME. Ubiquitin, cellular inclusions and their role in neurodegeneration. Trends Neurosci. (1998) 21:516–20. doi: 10.1016/S0166-2236(98)01276-4
59. Mcnaught KSP, Olanow CW, Halliwell B, Isacson O, Jenner P. Failure of the ubiquitin–proteasome system in Parkinson's disease. Nat Rev Neurosci. (2001) 2:589–94. doi: 10.1038/35086067
60. Ji H, Wang J, Guo J, Li Y, Lian S, Guo W, et al. Progress in the biological function of alpha-enolase. Anim Nutr. (2016) 2:12–7. doi: 10.1016/j.aninu.2016.02.005
61. Lundberg K, Kinloch A, Fisher BA, Wegner N, Wait R, Charles P, et al. Antibodies to citrullinated α-enolase peptide 1 are specific for rheumatoid arthritis and cross-react with bacterial enolase. Arthritis Rheum. (2008) 58:3009–19. doi: 10.1002/art.23936
62. Pontillo A, Di Toro N, Edomi P, Shadlow A, Ammadeo A, Gattorno M, et al. Anti–enolase antibodies in serum from pediatric patients affected by inflammatory diseases: diagnostic and pathogenetic insights. Int J Rheumatol. (2011) 2011:6. doi: 10.1155/2011/870214
63. Yoneda M, Fujii A, Ito A, Yokoyama H, Nakagawa H, Kuriyama M. High prevalence of serum autoantibodies against the amino terminal of α-enolase in Hashimoto's encephalopathy. J Neuroimmunol. (2007) 185:195–200. doi: 10.1016/j.jneuroim.2007.01.018
64. Lee KH, Chung H-S, Kim HS, Oh S-H, Ha M-K, Baik J-H, et al. Human α-enolase from endothelial cells as a target antigen of anti–endothelial cell antibody in Behçet's disease. Arthritis Rheum. (2003) 48:2025–35. doi: 10.1002/art.11074
65. Gilliam EB, Chauhan A, Moore T. Evaluation of anti-citrullinated type II collagen and anti-citrullinated vimentin antibodies in patients with juvenile idiopathic arthritis. Pediatr Rheumatol Online J. (2013) 11:31. doi: 10.1186/1546-0096-11-31
66. Nezos A, Cinoku I, Mavragani CP, Moutsopoulos HM. Antibodies against citrullinated alpha enolase peptides in primary Sjogren's syndrome. Clin Immunol. (2017) 183:300–3. doi: 10.1016/j.clim.2017.09.012
67. Ohashi Y, Ishida R, Kojima T, Goto E, Matsumoto Y, Watanabe K, et al. Abnormal protein profiles in tears with dry eye syndrome. Am J Ophthalmol. (2003) 136:291–9. doi: 10.1016/S0002-9394(03)00203-4
68. Koskenpato K, Ainola M, Przybyla B, Kouri VP, Virkki L, Koskenpato J, et al. Diminished salivary epidermal growth factor secretion: a link between Sjögren's syndrome and autoimmune gastritis? Scand J Rheumatol (2016) 45:118–21. doi: 10.3109/03009742.2015.1072243
69. Koski H, Konttinen YT, Hietanen J, Tervo T, Malmström M. Epidermal growth factor, transforming growth factor-alpha, and epidermal growth factor receptor in labial salivary glands in Sjögren's syndrome. J Rheumatol. (1997) 24:1930–5.
70. Azuma N, Katada Y, Kitano S, Sekiguchi M, Kitano M, Nishioka A, et al. Correlation between salivary epidermal growth factor levels and refractory intraoral manifestations in patients with Sjögren's syndrome. Mod Rheumatol. (2014) 24:626–32. doi: 10.3109/14397595.2013.850766
71. Azuma N, Katada Y, Kitano S, Sekiguchi M, Kitano M, Nishioka A, et al. Rapid decrease in salivary epidermal growth factor levels in patients with Sjögren's syndrome: a 3-year follow-up study. Mod Rheumatol. (2015) 25:876–82. doi: 10.3109/14397595.2015.1034941
72. Greenwood P, Schmidt K, Lipsky R, Parasuraman R, Jankord R. A Functional Promoter Variant of the Human Formimidoyltransferase Cyclodeaminase (FTCD) gene is associated with working memory performance in young but not older adults. Neuropsychology (2018). 32:973–84. doi: 10.1037/neu0000470
Keywords: Sjögren's syndrome, fatigue, interferon, proteomics, SOMAscan
Citation: Bodewes ILA, van der Spek PJ, Leon LG, Wijkhuijs AJM, van Helden-Meeuwsen CG, Tas L, Schreurs MWJ, van Daele PLA, Katsikis PD and Versnel MA (2019) Fatigue in Sjögren's Syndrome: A Search for Biomarkers and Treatment Targets. Front. Immunol. 10:312. doi: 10.3389/fimmu.2019.00312
Received: 05 September 2018; Accepted: 06 February 2019;
Published: 26 February 2019.
Edited by:
Clio Mavragani, National and Kapodistrian University of Athens, GreeceReviewed by:
Yasser Mohamed El-Sherbiny, Nottingham Trent University, United KingdomJuerg Hamacher, Lindenhofspital, Switzerland
Copyright © 2019 Bodewes, van der Spek, Leon, Wijkhuijs, van Helden-Meeuwsen, Tas, Schreurs, van Daele, Katsikis and Versnel. This is an open-access article distributed under the terms of the Creative Commons Attribution License (CC BY). The use, distribution or reproduction in other forums is permitted, provided the original author(s) and the copyright owner(s) are credited and that the original publication in this journal is cited, in accordance with accepted academic practice. No use, distribution or reproduction is permitted which does not comply with these terms.
*Correspondence: Marjan A. Versnel, m.versnel@erasmusmc.nl
†These author share first authorship