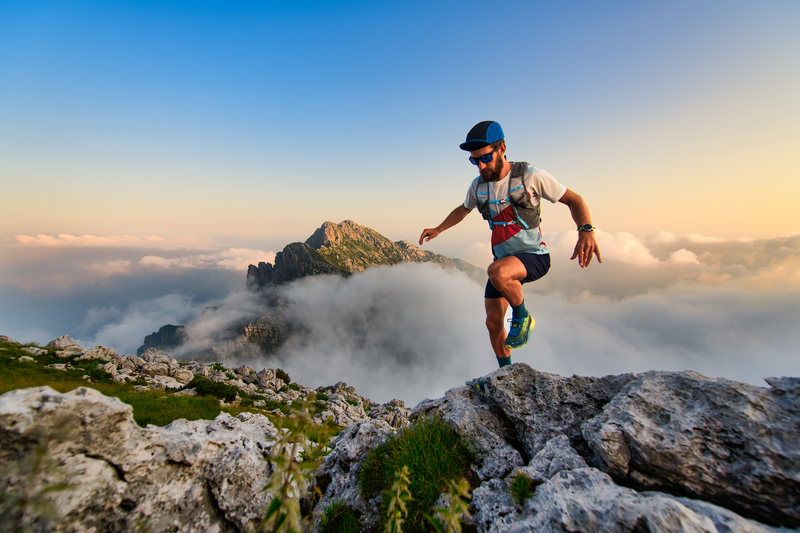
94% of researchers rate our articles as excellent or good
Learn more about the work of our research integrity team to safeguard the quality of each article we publish.
Find out more
REVIEW article
Front. Immunol. , 14 December 2018
Sec. Inflammation
Volume 9 - 2018 | https://doi.org/10.3389/fimmu.2018.02964
This article is part of the Research Topic Immunophysiology of Pediatric Rheumatic Diseases View all 18 articles
The presence of abnormal gene expression signatures is a well-described feature of the oligoarticular and polyarticular forms of juvenile idiopathic arthritis. In this review, we discuss how new insights into genetic risk for JIA and the three dimensional architecture of the genome may be used to develop a better understanding of the mechanisms driving these gene expression patterns.
The completion of the first assembly of the human genome was accompanied by considerable surprise with the discovery that only ~2% of the genome contained protein-encoding genes (1). The lay press seized on these findings and disseminated the idea that 98% of human genomes contained so-called “junk DNA,” on the assumption that the only things of interest in genomes were the protein-coding sequences. Subsequent genome annotation efforts, such as the Encyclopedia of DNA Elements (ENCODE) and Roadmap Epigenomics in the United States and Blueprint Epigenomics in Europe, demonstrated that much of this so-called “junk DNA” actually encoded RNA molecules (2, 3) or contained regulatory regions that played critical roles in fine-tuning transcription during development, cell differentiation, and in response to external stimuli (4).
The era of genome-wide association studies (GWAS), which were undertaken to better understand the genetic contribution to a broad range of complex traits, brought additional surprises. Investigators were perplexed to find that the strongest genetic associations occurred with single nucleotide polymorphisms (SNPs) that were located within non-coding regions of the genome. Indeed, taken together, GWAS SNPs are far more likely to occur in intronic or intergenic regions than in either exons or gene promoters (4).
These findings provide the basis for our inquiry into the causes of the well-described transcriptional abnormalities in JIA. In this paper, we will review the wealth of literature demonstrating abnormal patterns of gene expression in the peripheral blood cells of children with juvenile idiopathic arthritis (JIA). We will then discuss the role of non-coding genomic elements (especially enhancers) in regulating transcription and how an understanding of these mechanisms may allow a deeper understanding of the JIA-associated transcriptional patterns and/or the genetics of JIA.
In this review, we will focus on the oligoarticular and polyarticular, RF-negative subtypes of JIA, as these subtypes appear to represent a continuous spectrum of shared genetic risk (5). In contrast, systemic-onset (6) and RF+ polyarticular disease (7) show unique clinical and genetic features. We should note, however, that many of the broad ideas that we will present in this review are relevant to these other two JIA subtypes, as they are to most complex genetic traits.
The emergence of technologies to assess transcription on a genome-wide basis was initially met with considerable optimism that these tools could be used to develop clinical biomarkers (8), to classify heterogeneous patient populations more accurately (9, 10), to develop a better understanding of the mechanisms of therapeutic response (11–13) and to better understand disease pathogenesis (14). Different groups have reported a broad range of transcriptional abnormalities in patients with polyarticular and oligoarticular JIA. These transcriptional abnormalities can be observed in whole blood (12), unsorted white blood cells (buffy coats) (15), peripheral blood mononuclear cells (PBMC) (9, 16), neutrophils (17), and CD4+ T cells (18). These studies have generally identified clusters of interferon gamma (IFNg) and tumor necrosis factor alpha (TNFa) regulated genes that display differential expression in children with active polyarticular JIA when they are compared to healthy control children. An IL8 “signature” can also be seen in both neutrophil (19) and whole blood (14) gene expression studies. In general, whole blood expression studies have suggested complex interactions between innate and adaptive immunity in JIA (14, 20). It is curious to note that, even though the oligoarticular and polyarticular rheumatoid factor (RF)-negative subtypes share common genetic risk loci, the largest published study comparing these two phenotypes found that each was distinguishable from the other at the gene expression level (21). Similarly, RF-negative and RF-positive subtypes, which are genetically distinct (7), have remarkably similar gene expression profiles on whole blood microarray analysis. Thus, it is clear that genetic factors are not the sole drivers of the gene expression abnormalities observed in oligoarticular and polyarticular JIA.
The question arises as to whether these transcriptional abnormalities reflect intrinsic defects in gene regulation in the cells of interest, or merely reflect the inflammatory milieu to which these cells are exposed. After all, elevated levels of a broad range of inflammatory mediators can be observed in the serum (22) or plasma (23) of children with JIA. On the other hand, our group has shown that the transcriptional abnormalities observed in JIA neutrophils are accompanied by aberrations in the metabolism of glucose via the hexose monophosphate shunt (19). Similarly, Throm and colleagues have shown distinct aberrations in interferon gamma-mediated (IFNg) signaling pathways in JIA T cells (24) studied in vitro. Finally, neither PBMC nor neutrophil signatures “normalize” after children have achieved clinical remission on medication (CRM), although it's curious to note that the neutrophil aberrations are more prominent (13). We should also note that the intrinsic defect vs. externally-driven hypotheses to explain the distinct transcriptional profiles of JIA peripheral blood cells are not mutually exclusive. Although we are coming to understand the strong effect that the environment (broadly considered) has on peripheral blood gene expression (25), underlying genetics and the immediate external milieu to which the cells are exposed may both play a role in the transcriptional patterns observed in peripheral blood leukocytes of children with JIA.
We were naturally led us to ask whether the emerging knowledge of the genetics of JIA might provide a useful framework from which to understand the mechanisms driving the transcriptional abnormalities in JIA peripheral blood cells. This necessarily leads to a brief discussion of the genetics on JIA. This discussion will not be comprehensive, and the reader wishing to have a deeper understanding is invited to read the recent reviews available on this subject (26, 27).
Using candidate gene approaches, GWAS, and genetic fine mapping studies, investigators have identified >30 genetic loci associated with JIA (26–28). Each of these 3 approaches queries a single or small groups of SNPs (candidate gene approaches) or large numbers of SNPs (GWAS and genetic fine mapping studies) and asks whether specific alleles occur more frequently in individuals with a specific disease or phenotype than they do in controls (29) to identify alleles that have a strong association with the disease or trait of interest. It is important to note, however, that SNPs identified by such studies may not be the actual genetic variants that exert the biological effects that confer risk. This is because the so-called tag SNPs (or candidate SNPs used in candidate gene approaches) are in linkage disequilibrium (LD) with hundreds or thousands of other SNPs in the same region, any one of which may exert risk-enhancing biological effects. To use an analogy, GWAS can be understood as something like a crude global positioning satellite (GPS) that can tell you, say, that you are on the M1, somewhere between London and Sheffield, assuring you that you are not in Cornwall, but not providing any information as to whether you're actually closer to Leicester or Nottingham. Thus, GWAS have merely identified regions of the genome where genetic risk may be exerted. These regions can be referred to as “LD blocks” or, more commonly, risk haplotypes. It is common to refer to the risk haplotypes in JIA (and other complex traits) by the gene nearest to the tag SNP, and this has led to the common misunderstanding that the GWAS SNP: (1) is the one that actually exerts the relevant biological effects and (2) exerts those effects on the nearest gene. Neither is necessarily the case.
Figure 1 illustrates this point. IL6R is a haplotype that was identified on the genetic fine mapping study published by Hinks et al. (5). The haplotype spans the region marked by the genomic coordinates, chr1:154291718-154392674, a length of >100,000 bp. The reader will also note that there are functional elements other than the coding genes (ATP8B2 and a portion of IL6R); this region is also characterized by prominent, overlapping H3K4me1/H3K27ac histone marks (for simplicity, only the H3K27 marks are shown in the figure), and dense transcription factor binding even in non-coding regions (i.e., within introns and the intergenic area). These chromatin features are commonly associated with enhancers, about which it is useful to say more as we query the possible role of genetics in driving the transcriptional abnormalities observed in JIA.
Figure 1. Genome browser screen shot showing the JIA-associated IL6R locus, identified by the tag SNP, rs11265608, and encompassing the region chr1:154291718–154379369. Transcription is from left to right. This locus consists largely of an intergenic region between the IL6R and ATP8B2 genes. The haplotype block also encompasses the first exon and intron of the IL6R gene and most of the coding sequence of the ATP8B2 gene. The position of the tag SNP, rs11265608, is indicated by the red arrow. ChIPseq peaks for the histone mark H3K27ac from ENCODE and Roadmap Epigenomics data, are shown with the blue/magenta peaks. Transcription factor (TF) binding data and DNAse hypersensitivity data (also from ENCODE and Roadmap Epigenomics) are is represented black and gray boxes at the bottom of the figure. The regions where TF and H3K27ac marks overlap are putative enhancers. Almost identical chromatin architecture is seen in both CD4+ T cells and neutrophils within this haplotype. Note that neutrophils also express an intergenic RNA molecule at chr1:154350688-154350783 (not shown).
H3K4me1 and H3K27ac refer to covalent modifications to the tails of histones, epigenetic features that typically accompany enhancers, as noted above. One of the striking features that emerged from multiple GWAS studies was the high frequency with which the genetic “hits” occurred within H3K4me1/H3K27ac-marked regions of pathologically-relevant cells for the traits of interest (4). The reverse is also true: if one maps enhancer elements in specific cell types, those mapped regions are highly enriched in GWAS-identified SNPs (30) for diseases that affect those cells/tissues. We have reported that the risk loci for both JIA (31, 32) and systemic lupus (33), are highly enriched (compared to randomly-selected regions of functional chromatin) for H3K4me1/H3K27ac histone marks. These findings have led us to suggest that much of the genetic risk for JIA is exerted through altered function of these enhancers.
Enhancers are non-coding DNA elements that play an important role in regulating gene expression, serving as rheostats that adjust gene expression to fit finely-tuned physiologic contexts (34). Enhancers have a characteristic structure that includes the presence of open chromatin bound by multiple transcription factors (TFs) flanked by H3K4me1/H3K27ac-marked boundaries (Figure 2). These TFs form a complex that typically includes p300, mediator, and cohesin, which together facilitate looping and physical contact with the promoters of target genes. While many enhancers function constitutively, others remain latent, activated only by specific cellular or environmental triggers (35). It is important, from the standpoint of our understanding the genetics and pathobiology of JIA, to note that enhancers do not always regulate the nearest gene. Furthermore, a given enhancer may regulate more than one gene, and a given gene may be regulated by more than one enhancer. Although enhancers may not regulate the nearest gene, they typically regulate genes within the same chromatin loop or topologically associated domain (TAD). Our understanding of the three-dimensional structure of chromatin within the nucleus of eukaryotic cells has expanded significantly in the past 10 years, and readers interested in learning more on this fascinating topic and its relation to human disease may wish to consult some of the recent reviews (36–39). For the purposes of this review, TADs can be considered the basic chromatin loop structure that regulates enhancer-promoter interactions, as shown in Figure 3. TADs can be identified using non-targeted chromatin conformation techniques such as HiC (40), and visualized using publically available software such as JuiceBox (41). Figure 4 shows how the TAD for the IL6R locus can be visualized. The larger loop contains multiple genes, including IL6R, IL6R-AS1, ATP8B2, SHE, TDRD10, UBE2Q1, and UBE2Q1-AS1. Any or all of these genes may be regulated by enhancer(s) within the IL6R locus, and thus dysregulated by genetic variants that disrupt or alter enhancer function within this locus.
Figure 2. Structure of a typical enhancer. H3K4me1/H3K27ac-marked histones flank a region densely bound by transcription factors (TFs-represented by the pentagon, hexagon, and trapezoid). The enhancer complex physically interacts with gene promoters, stabilizing TFs within the promoter as well as RNA polymerase (RNAP) binding, facilitating transcription.
Figure 3. Regulation of gene expression by enhancers within topologically associated domains (TADs). The chromatin loop structure facilitates interactions between the enhancer and some (or all) of the genes within the same TAD, while constricting the interactions between the enhancer and genes outside the TAD.
Figure 4. Juicebox software visualization of the topologically associated domain (TAD) containing the JIA-associated IL6R haplotype. Data are from HiC analysis of K562 cells. The cursor (crossed blue lines) is set at the center of a putative enhancer in the region spanning chr1:154, 353, 745–154, 361, 534. Three distinct chromatin loops are identified, as indicted by the gray, green, and yellow triangles, suggesting complex chromatin architecture in this region.
Now we return to the question of the role of genes vs. environment (broadly considered) in driving the gene expression abnormalities in JIA. We recently reported on results of whole blood expression studies from children enrolled in the NIH-funded Trial of Early Aggressive Therapy in JIA (TREAT trial) (14). In that study, we identified 158 genes that showed differential expression when we compared children with new-onset polyarticular JIA with healthy controls. We then used conventional computational techniques to intersect the differentially expressed genes with the known JIA haplotypes. We were unable to identify a single differentially expressed gene within the known JIA haplotypes. We have subsequently repeated this technique using all publically available gene expression data from children with oligoarticular and polyarticular JIA and gotten the same result. This has led us to the conclusion that if genetic variants associated with JIA influence the observed transcriptional patterns, they must either do so within specific leukocyte subsets that are not detectable using whole blood, or they must act on longer-range chromatin interactions. Once again these are not mutually exclusive considerations. We anticipate that the emergence of single-cell technologies and perhaps the development of disease-specific three-dimensional chromatin maps will allow us to understand the genetic contribution (if any) to the observed peripheral blood expression abnormalities.
Let us return to Figure 1, which shows the multiple features within the IL6R haplotype, which occupies the genomic coordinates chr1:154291718-154392674 and spans >100,000 bp. This risk locus is representative of the other JIA risk loci, which almost invariably show the same of similar chromatin features (31, 32).
The reader will note immediately that the haplotype contains only a portion of the IL6R gene as well as an additional gene, ATP8B2. The protein product of ATP8B2 is an ATPase, and the gene is expressed in both lymphoid cells as well as in myeloid cells such as macrophages (42); its exact role in these cells has not been investigated in any detail, although it is reasonable to speculate that it is involved in cellular energy production and utilization. Using RNA sequencing in human neutrophils, we have also shown that the haplotype also contains at least one non-coding intergenic RNA (ncRNA), a species of RNAs that are important in regulating both three dimensional chromatin architecture (43) and gene expression (44). We have previously shown that the presence of ncRNA molecules expressed in neutrophils is a common feature within the JIA risk loci (31, 32) and the presence of such RNA species in pathologically relevant cells is a characteristic that the JIA risk loci share with those of most other complex traits.
Finally, we note once again the rich in H3K4me1/H3K27ac histone marks, which overlap with abundant transcription factor binding sites, features that can be observed particularly in CD4+ T cells (ENCODE and Roadmap Epigenomics data) as well as our own neutrophil ChIP-seq data (31). Indeed the chromatin architecture at this locus suggests the presence of multiple intronic and intergenic enhancers in both cell types.
These observations raise multiple questions regarding the mechanism(s) through which genetic variants within this locus impinge on immune function. Do genetic variants alter the structure of the ncRNA or its regulation? Do they alter the expression of ATP8B2 or IL6R through alterations in their promoters? Do they alter the function of one or more of the enhancers? If so, what genes are dysregulated by altered enhancer function? If genetically-mediated dysregulation isn't exerted on peripheral blood cells, then where is it exerted?
These aren't either/or questions. That is, different genetic variants in different individuals might alter one or more of the genomic functions within the JIA haplotypes to different degrees. This might explain, for example, the considerable differences we see between individuals with JIA at both the phenotypic and gene expression levels. Indeed, we believe that one of the reasons why there is so much overlap in the genetic associations seen for a broad range of autoimmune/inflammatory diseases in the fact that these loci contain multiple important genomic elements which, if perturbed, could lead to an immune phenotype.
We are still rather in the dark as to the origin of the abnormal transcriptional signatures in JIA. It's clearly not as straightforward as we initially thought it might be: that polymorphisms in gene promoters, for example, would lead to alterations in gene expression that might be easily observed in cells or in serum protein levels. It should also be sufficiently clear to the reader that there is limited, if any, utility in trying to understand genetic mechanisms in JIA by focusing solely on the coding functions of the genes in close proximity to the SNPs identified on GWAS and genetic fine mapping studies. This “nearest gene” focus ignores the broader chromatin architecture in which the biologically relevant variants are likely to operate. We propose that future studies of individual JIA-associated loci consider not only the entire risk haplotype and the multiple genomic elements contained within it, but also broaden the inquiry to include three dimensional chromatin architecture and the genes included within the TADs that incorporate the risk haplotypes. This means that there is going to be a lot of work to do at each risk locus.
Enhancers seem to be the logical place to start, given their demonstrated importance in JIA (31, 32) and rheumatic diseases in general (45, 46). While the chromatin signatures within the JIA risk loci are strong indicators that these regions have enhancer function, the specific functional regions will need to be identified and verified empirically using reporter assays. Once the specific functional regions are identified, it will be a straightforward task to clarify the effects of genetic variants within these functional regions. Publically available data like the 1000 Genomes Project will give investigators a large but finite number of common genetic variants (allele frequencies >1%) to test within the defined regions. Our recent whole genome sequencing data from children with polyarticular, RF-negative JIA (47) have been made available to investigators through the National Center for Biotechnology Information (https://www.ncbi.nlm.nih.gov/bioproject/?term=PRJNA343545) and will provide the scientific community with a rich list of rare variants to interrogate.
Once enhancer function is confirmed and the biologically-relevant genetic variants identified, we still have before us the task of identifying the genes (dys)regulated by the enhancers on which genetic variance operates. To accomplish this aim, our laboratory is taking advantage of the fact that most enhancers regulate genes within the same TAD. We are therefore using an epigenome editing approach (48) to attenuate enhancer function and identify genes whose expression is altered when specific enhancers are attenuated.
The identification of aberrant transcriptional patterns in the peripheral blood cells of children with JIA has opened the door to intriguing inquiries into the epigenetics and genetics of this family of diseases. With regard to the latter, our growing understanding of the structure and function of mammalian genomes makes it imperative that we broaden our investigations beyond the “nearest gene” to GWAS tag SNPs. Rather, developing a mechanistic understanding of how and where genetic variants alter transcription and immune function will require a complete understanding of the range of genomic functions altered by variants within the risk haplotypes. Furthermore, the field will require a detailed understanding of the larger chromatin “neighborhoods” within which each of the haplotypes resides. These are reachable goals that are most likely to be achieved by a focused and coordinated effort among the different pediatric rheumatology genetics research consortia to prioritize loci, share expertise and reagents, and develop plans for using genetic information to inform clinical care.
JJ developed the concept for the paper and assisted in the writing. HK assisted in the 3D chromatin analysis. KJ assisted in developing the concepts and generated data cited in this paper.
This work was supported by NIH R21- AR071878 (JJ) from the National Institutes of Health and research grants from the Arthritis Foundation (#6490) and the Rheumatology Research Foundation (JJ), as well as by the National Center for Advancing Translational Sciences of the National Institutes of Health under award number UL1TR001412 to the University at Buffalo. The content is solely the responsibility of the authors and does not necessarily represent the official views of the NIH. This work was also supported by a medical student summer research fellowship (HK) from the Rheumatology Research Foundation.
The authors declare that the research was conducted in the absence of any commercial or financial relationships that could be construed as a potential conflict of interest.
1. Stamatoyannopoulos J. What does our genome encode? Genome Res. (2012) 22:1602–11. doi: 10.1101/gr.146506.112
2. Jacquier A. The complex eukaryotic transcriptome: unexpected pervasive transcription and novel small RNAs. Nat Rev Genet. (2009) 10:833–44. doi: 10.1038/nrg2683
3. Karpanov P, Willingham AT, Gingeras TR. Genome-wide transcription and implications for genomic organization. Nat Rev Genet. (2007) 8:413–23. doi: 10.1038/nrg2083
4. Maurano MT. Humbert R, Rynes E, Thurman RE, Haugen E, Wang H, et al. Systematic localization of common disease association in regulatory DNA. Science (2013) 337:1190–5. doi: 10.1126/science.1222794
5. Hinks A, Cobb J, Marion MC, Prahalad S, Sudman M, Bowes JG, et al. Dense genotyping of immune-related disease regions identifies 14 new susceptibility loci for juvenile idiopathic arthritis. Nat Genet. (2013) 45:644–69. doi: 10.1038/ng.2614
6. Ombrello MJ. Arthur VL, Remmers EF, Hinks A, Tachmazidou I, Grom AA, et al. Genetic architecture distinguishes systemic juvenile idiopathic arthritis from other forms of juvenile idiopathic arthritis: clinical and therapeutic implications. Ann Rheum Dis. (2016) 76:906–13. doi: 10.1136/annrheumdis-2016-210324
7. Hinks A, Marion MC, Cobb J, Comeau ME, Sudman M, Ainsworth HC, et al. Brief report: the genetic profile of rheumatoid factor-positive polyarticular juvenile idiopathic arthritis resembles that of adult rheumatoid arthritis. Arthritis Rheumatol. (2018) 70:957–62. doi: 10.1002/art.40443
8. Jiang K, Sawle AD, Frank MB, Chen Y, Wallace CA, Jarvis JN. (2014). Whole blood gene expression profiling predicts therapeutic response in polyarticular juvenile idiopathic arthritis at 6 months. Arthritis Rheumatol. (2014) 66:1363–71. doi: 10.1002/art.38341
9. Griffin TA. Barnes MG, Ilowite NT, Olson JC, Sherry DD, Gottlieb BS, et al. Gene expression signatures in polyarticular juvenile idiopathic arthritis demonstrate disease heterogeneity and offer molecular classification of disease subsets. Arthritis Rheumatol. (2009) 60:2113–23. doi: 10.1002/art.24534
10. Eng SWM. Duong TT, Rosenberg AM, Morris Q, Yeung RSM. The biological basis of clinical heterogeneity in juvenile idiopathic arthritis. Arthritis Rheumatol. (2014) 66:3463–75. doi: 10.1002/art.38875
11. Du N, Jiang K, Sawle AD, Frank MB, Wallace CA, Zhang A, et al. Dynamic tracking of functional gene modules in treated juvenile idiopathic arthritis. Genome Med. (2015) 7:109. doi: 10.1186/s13073-015-0227-2
12. Hu Z, Jiang K, Frank MB, Chen Y, Jarvis JN. Transcriptional rewiring in neutrophils through the course of treated juvenile idiopathic arthritis. Sci. Rep. (2018) 8:7805. doi: 10.1038/s41598-018-26163-4
13. Jiang K, Frank MB, Chen Y, Osban J, Jarvis JN. Genomic characterization of remission in juvenile idiopathic arthritis. Arthritis Res Ther. (2013) 15:R100. doi: 10.1186/ar4280
14. Jiang K, Wong L, Sawle AD, Frank MB, Chen Y, Wallace CA, et al. Whole blood expression profiling from the TREAT trial: insights for the pathogenesis of polyarticular juvenile idiopathic arthritis. Arthritis Res Ther. (2016) 8:157. doi: 10.1186/s13075-016-1059-1
15. Jarvis JN, Dozmorov I, Jiang K, Frank MB, Szodoray P, Alex P, et al. Novel approaches to gene expression analysis of active polyarticular juvenile rheumatoid arthritis. Arthritis Res Ther. (2004) R15–32. doi: 10.1186/ar1018
16. Knowlton N, Jiang K, Frank MB, Aggarwal A, Wallace C, McKee R, et al. The meaning of clinical remission in polyarticular juvenile idiopathic arthritis: gene expression profiling in peripheral blood mononuclear cells identifies distinct disease states. Arthritis Rheumatol. (2009) 60:892–900. doi: 10.1002/art.24298
17. Jarvis JN. Jiang K, Frank MB, Knowlton N, Aggarwal A, Wallace CA, et al. Gene expression profiling in neutrophils of children with polyarticular juvenile idiopathic arthritis. Arthritis Rheumatol. (2009) 60:1488–95. doi: 10.1002/art.24450
18. Wong L, Jiang K, Chen Y, Hennon T, Holmes L, Wallace CA, et al. Limits of peripheral blood mononuclear cells for gene expression-based biomarkers in juvenile idiopathic arthritis. Sci Rep. (2016) 6:29477 doi: 10.1038/srep29477
19. Jarvis JN, Petty HR, Tang Y, Frank MB, Tessier PA, Dozmorov I, et al. Evidence for chronic, peripheral activation of neutrophils in polyarticular juvenile rheumatoid arthritis. Arthritis Res Ther. (2006) 8:R154. doi: 10.1186/ar2048
20. Jarvis JN, Jiang K, Petty HR, Centola M. Neutrophils: the forgotten cell in JIA disease pathogenesis. Pediatr Rheumatol. (2007) 5:13. doi: 10.1186/1546-0096-5-13
21. Barnes MG, Grom AA, Thompson SD, Griffin TA, Pavlidis P, Itert L, et al. Subtype-specific gene expression profiles in recent-onset juvenile idiopathic arthritis. Arthritis Rheum. (2009) 60:2102–12. doi: 10.1002/art.24601
22. De Jaeger W, Hoppenreijs EP, Wulffrat NM. Wedderburn LR, Kuis W, Prakken BJ. Blood and synovial fluid cytokine signatures in patients with juvenile idiopathic arthritis: a cross-sectional study. Ann Rheum Dis. (2007) 66:589–98. doi: 10.1136/ard.2006.061853
23. Jarvis JN, Pousak T, Krenz M, Iodidze MV, Taylor H. Complement activation and immune complexes in juvenile rheumatoid arthritis. J Rheumatol. (1993) 20:114–7.
24. Thom AA, Moncrieffe H, Orandi AB, Pingel JT, Geurs TL, Miller HL, et al. Identification of enhanced IFN-γ signaling in polyarticular juvenile idiopathic arthritis with mass cytometry. JCI Insight (2018) 3:e121544. doi: 10.1172/jci.insight.121544
25. Favé MJ, Lamaze FC, Soave D, Hodgkinson A, Gauvin H, Bruat V, et al. Gene-by-environment interactions in urban populations modulate risk phenotypes. Nat Comm. (2018) 9:827. doi: 10.1038/s41467-018-03202-2
26. Hersh AO, Prahalad S. Immunogenetics of juvenile idiopathic arthritis: a comprehensive review. J Autoimmun. (2015) 64:113–24. doi: 10.1016/j.jaut.2015.08.002
27. Hersh AO, Prahalad S. Genetics of juvenile idiopathic arthritis. Rheum Dis Clin N Am. (2017) 43:435–48. doi: 10.1016/j.rdc.2017.04.007
28. McIntosh LA. Marion MC, Sudman M, Comeau ME, Becker ML, Bohnsack JF, et al. Genome-wide association meta-analysis reveals novel juvenile idiopathic arthritis susceptibility loci. Arthritis Rheumatol. (2017) 69:2222–32. doi: 10.1002/art.40216
29. McCarthy MI. Abecasis GR, Cardon LR, Goldstein DB, Little J, Ioannidis JPA, et al. Genome-wide association studies for complex traits: consensus, uncertainty, challenges. Nat Rev Genet. (2008) 9:356–69. doi: 10.1038/nrg2344
30. Ernst J, Kheradpour P, Mikkelsen TS, Shoresh N, Ward LD, Epstein CB, et al. Mapping and analysis of chromatin state dynamics in nine human cell lines. Nature (2011) 337:1190–5. doi: 10.1038/nature09906
31. Jiang K, Zhu L, Buck MJ, Chen Y, Carrier B, Liu T, et al. Disease-associated SNPs from non-coding regions in juvenile idiopathic arthritis are located within or adjacent to functional genomic elements of human neutrophils and CD4+ T cells. Arthritis Rheumatol. (2015) 67:1966–77. doi: 10.1002/art.39135
32. Zhu L, Jiang K, Webber K, Wong L, Liu T, Chen Y, et al. Chromatin landscapes and risk for juvenile idiopathic arthritis. Arthritis Res Ther. (2017) 19:57 doi: 10.1186/s13075-017-1260-x
33. Hui-Yuen JS, Zhu L, Wong L, Jiang K, Chen Y, Liu T, et al. Chromatin landscapes and genetic risk in systemic lupus. Arthritis Res Ther. (2016) 18:281. doi: 10.1186/s13075-016-1169-9
34. Shlyueva D, Stampfel G, Stark A. Transcriptional enhancers: from properties to genome-wide predictions. Nat Rev Genet. (2014) 15:272–86. doi: 10.1038/nrg3682
35. Ostuni R, Piccolo V, Barozzi I, Polletti S, Termanini A, Bonifacio S, et al. Latent enhancers activated by stimulation in differentiated cells. Cell (2013). 152:157–71. doi: 10.1016/j.cell.2012.12.018
36. Lupiáñez DG. Spielmann M, Mundlos S. Breaking TADs: how alterations of chromatin domains result in disease. Trends Genet. (2016) 32:225–37. doi: 10.1016/j.tig.2016.01.003
37. Hnisz D, Day DS, Young RA. Insulated neighborhoods: structural and functional units of mammalian gene control. Cell (2016) 167:1188–200. doi: 10.1016/j.cell.2016.10.024
38. Mishra A, Hawkins RD. Three-dimensional genome architecture and emerging technologies: looping in disease. Genome Med. (2017) 9:87. doi: 10.1186/s13073-017-0477-2
39. Roy SS, Mukherjee AK, Chowdhury S. Inisights about genome function from spatial organization of the genome. Hum Genomics (2018) 12:8. doi: 10.1186/s40246-018-0140-z
40. Belton JM, McCord RP, Gibcus JH, Naumova N, Zhan Y, Dekker J. HiC: a comprehensive technique to capture the conformation of genomes. Methods (2012) 58:268–76. doi: 10.1016/j.ymeth.2012.05.001
41. Durand NC, Robinson JT, Shamim MS, Machol I, Mesirov JP, Lander ES, et al. Juicebox provides a visualization system for HiC contact maps with unlimited zoom. Cell Systems (2016) 3:99–101. doi: 10.1016/j.cels.2015.07.012
42. Poczobutt JM, De S, Yadav VK, Nguyen TT, Li H, Weiser-Evans MCM, et al. Expression profiling of macrophages reveals multiple populations with distinct biological roles in an immunocompetent orthotopic model of lung cancer. J Immunol. (2016) 196:2847–59. doi: 10.4049/jimmunol.1502364
43. Isoda T, Moore AJ, He Z, Chandra V, Aida M, Denholtz M, et al. Non-coding transcription instructs chromatin folding and compartmentalization to dictate enhancer-promoter communication and T cell fate. Cell (2017) 171:103–19.e18. doi: 10.1016/j.cell.2017.09.001
44. Chen YC, Satpathy AT, Chang HY. Gene regulation in the immune system by long non-coding RNAs. Nat Immunol. (2017) 18:962–72. doi: 10.1038/ni.3771
45. Fahr KK, Marson A, Kleinewietfeld M, Housely WJ, Beik S, Shoresh N, et al. Genetic and epigenetic fine mapping of causal autoimmune disease variants. Nature (2015) 518:337–43. doi: 10.1038/nature13835
46. Freudenberg J, Gregersen P, Li W. Enrichment of genetic variants for rheumatoid arthritis within T cell and NK cell enhancer regions. Mol Med. (2015) 21:180–4. doi: 10.2119/molmed.2014.00252
47. Wong L, Jiang K, Chen Y, Jarvis JN. Genetic insights into juvenile idiopathic arthritis derived from deep whole genome sequencing. Sci Rep. (2017) 7:2657. doi: 10.1038/s41598-017-02966-9
Keywords: juvenile idiopathic arthritis (JIA), genetics, enhancer, non-coding genome, topically associated domains
Citation: Kessler H, Jiang K and Jarvis JN (2018) Using Chromatin Architecture to Understand the Genetics and Transcriptomics of Juvenile Idiopathic Arthritis. Front. Immunol. 9:2964. doi: 10.3389/fimmu.2018.02964
Received: 01 November 2018; Accepted: 03 December 2018;
Published: 14 December 2018.
Edited by:
Lucy R. Wedderburn, Great Ormond Street Institute of Child Health, University College London, United KingdomReviewed by:
Georg Wilhelm Otto, University College London, United KingdomCopyright © 2018 Kessler, Jiang and Jarvis. This is an open-access article distributed under the terms of the Creative Commons Attribution License (CC BY). The use, distribution or reproduction in other forums is permitted, provided the original author(s) and the copyright owner(s) are credited and that the original publication in this journal is cited, in accordance with accepted academic practice. No use, distribution or reproduction is permitted which does not comply with these terms.
*Correspondence: James N. Jarvis, amFtZXNqYXJAYnVmZmFsby5lZHU=
Disclaimer: All claims expressed in this article are solely those of the authors and do not necessarily represent those of their affiliated organizations, or those of the publisher, the editors and the reviewers. Any product that may be evaluated in this article or claim that may be made by its manufacturer is not guaranteed or endorsed by the publisher.
Research integrity at Frontiers
Learn more about the work of our research integrity team to safeguard the quality of each article we publish.