- 1Division of Immunology, Allergy & Rheumatology, Department of Internal Medicine, University of Cincinnati, Cincinnati, OH, United States
- 2Division of Asthma Research, Department of Pediatrics, Cincinnati Children's Hospital Medical Center, University of Cincinnati, Cincinnati, OH, United States
- 3Division of Allergy and Immunology, Department of Pediatrics, Cincinnati Children's Hospital Medical Center, University of Cincinnati, Cincinnati, OH, United States
Atopic dermatitis (AD) is a complex multifactorial inflammatory skin disease that affects ~280 million people worldwide. About 85% of AD cases begin in childhood, a significant portion of which can persist into adulthood. Moreover, a typical progression of children with AD to food allergy, asthma or allergic rhinitis has been reported (“allergic march” or “atopic march”). AD comprises highly heterogeneous sub-phenotypes/endotypes resulting from complex interplay between intrinsic and extrinsic factors, such as environmental stimuli, and genetic factors regulating cutaneous functions (impaired barrier function, epidermal lipid, and protease abnormalities), immune functions and the microbiome. Though the roles of high-throughput “omics” integrations in defining endotypes are recognized, current analyses are primarily based on individual omics data and using binary clinical outcomes. Although individual omics analysis, such as genome-wide association studies (GWAS), can effectively map variants correlated with AD, the majority of the heritability and the functional relevance of discovered variants are not explained or known by the identified variants. The limited success of singular approaches underscores the need for holistic and integrated approaches to investigate complex phenotypes using trans-omics data integration strategies. Integrating omics layers (e.g., genome, epigenome, transcriptome, proteome, metabolome, lipidome, exposome, microbiome), which often have complementary and synergistic effects, might provide the opportunity to capture the flow of information underlying AD disease manifestation. Overlapping genes/candidates derived from multiple omics types include FLG, SPINK5, S100A8, and SERPINB3 in AD pathogenesis. Overlapping pathways include macrophage, endothelial cell and fibroblast activation pathways, in addition to well-known Th1/Th2 and NFkB activation pathways. Interestingly, there was more multi-omics overlap at the pathway level than gene level. Further analysis of multi-omics overlap at the tissue level showed that among 30 tissue types from the GTEx database, skin and esophagus were significantly enriched, indicating the biological interconnection between AD and food allergy. The present work explores multi-omics integration and provides new biological insights to better define the biological basis of AD etiology and confirm previously reported AD genes/pathways. In this context, we also discuss opportunities and challenges introduced by “big omics data” and their integration.
Background
Atopic dermatitis (AD) is an inflammatory potentially debilitating skin disease associated with itch and eczematous lesions. It is primarily characterized by epidermal barrier dysfunction and immune alterations. Nearly 80% of children with AD progress to develop food allergy, asthma or rhinitis, underscoring its public health impact (1–3). Clinically, AD shows great patient-to-patient variability (probably representing multiple endophenotypes) and is associated with a wide range of abnormalities such as epidermal lipid and protease abnormalities, compromised cutaneous barrier function and inflammation. AD is diagnosed using patient history and visual assessment of the skin (as no specific laboratory test is available) and frequently managed with topical moisturizers, corticosteroids or calcineurin inhibitors—none of which are specific to AD (4, 5). A major gap that has hindered management strategies is the lack of treatment modalities tailored to the well-defined AD phenotypes that are still under investigation. It is widely accepted that integrative approaches linking multiple omics with clinical and epidemiological data are needed in order to develop better treatment and prediction models. Emerging biologic therapies, including cytokine-targeted therapies (e.g., anti–IL-4Rα mAb Dupilimab and anti–IL-31R mAb Nemolizumab), are costly but show promising results in patients sub-groups (6–8). Due to wide clinical variability, both topical and systemic therapies require better molecular tools/biomarkers for (a) identifying target patients to choose suitable treatment options and (b) assessing therapeutic outcomes.
The promise of the multi-omics approach to decipher the endotypes of complex diseases has been well-described (9), and several investigators have proposed potential biomarkers and endotypes for AD using omics resources (10–12). However, although “omics-level” studies have been very useful in understanding the mechanism of AD manifestation (Figure 1), there have not been sufficient data nor attempts to integrate different omics data. This integration seems essential to interpret clinical variability and endotyping of AD. The present work outlines the roles of omics resources (such as genome, epigenome, transcriptome, proteome, metabolome, lipidome, exposome, microbiome) as parts of a puzzle and the approaches to integrate these layers and obtain an integrated systems-level overview of AD. Integrating multiple layers of omics information with clinical outcome data will be helpful in capturing the etiology of AD and its endotypes that can be useful in managing of this condition.
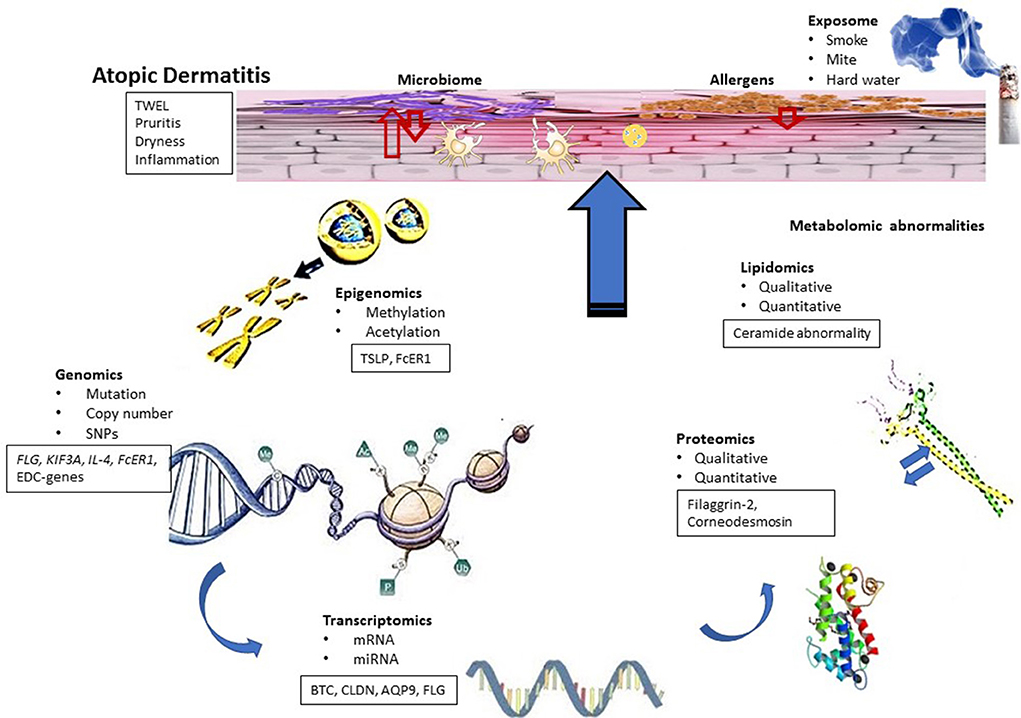
Figure 1. Conceptual biologic model for AD. Functional dysregulation in AD investigated using different omics techniques has been shown. AD skin is characterized by barrier dysfunction, inflammation and bacterial infection and associated with symptoms such as dry skin, itch, and inflammation. Genomic studies identified candidate genes that can be linked to transcriptomic studies. Some results also indicated the role of epigenetic modifications in AD, whereas transcriptomic studies identified functional clusters of genes related to AD pathogenesis. Differentially regulated genes of these functional clusters can be correlated with the results obtained from proteomic and lipidomic studies. On the other hand, differential regulation of innate immune genes and proteins can influence microbial diversity of the skin, which can also contribute to AD pathogenesis.
The Pathobiology of AD is Incompletely Understood
AD has been renamed more than twenty times within the last 125 years, reflecting its wide clinical, genetic, and immunologic variability (11, 13). It is a disease of multifactorial etiology involving complex immunologic and inflammatory pathways (13, 14). The etiology of AD has been described using two opposing yet overlapping hypotheses. The “inside-out hypothesis” suggests that type 2 immune activation precedes cutaneous barrier impairment. Experimental data showing down-regulation of skin barrier genes by immune cytokines and mediators supports this hypothesis (15, 16). However, this hypothesis does not adequately explain the root cause of systemic inflammation, only highlighting the relevance of classical adaptive TH2 cells, in causing barrier dysfunction. In contrast, the “outside-in hypothesis” suggests that a broad skin barrier dysfunction, which precedes inflammation, is required for the manifestation of AD (16). This model is largely supported by data showing that the loss-of-function mutations in the filaggrin gene are linked to a subpopulation of AD patients (17). This outside-in hypothesis explains the causative role of epithelial barrier function in a subset of patients, as only about 20% of Northern European and Asian patients with AD bear known underlying defects in the barrier function, such as filaggrin mutations (compared to ~10% in non-AD population) (18). Moreover, in a study conducted by our group, ~39% of children with at least one parent with atopy developed AD by 3 years of age (19). This cannot be explained by FLG mutation alone, as only about 10% of AD cases could be projected from this mutation in general population (20). Thus, the “outside-in hypothesis” does not sufficiently explain the etiology of AD, showing the need to interrogate AD using novel approaches such as omics integration.
Biological Variability and Endotypes—Challenges in the Management of AD
AD is clinically heterogeneous and lacks standardized laboratory assessment approaches. In the absence of a specific laboratory test to diagnose AD, evaluating the severity and treatment outcomes in AD patients are currently dependent on visually assessing the skin using clinical scoring such as EASI or SCORAD. However, these scoring systems are time-consuming and subject to human error (21). Recent efforts are also being made to incorporate cutaneous barrier function (determined by trans-epidermal water loss [TEWL]) and atopic status (cutaneous reactions to allergens, as measured by serum allergen-specific IgE with or without total IgE). The assessment is further complicated by factors such as (a) clinical variations, (b) age and ancestry/genetic variations of the subjects, and (c) experimental and omics-level variations. Heterogeneity in patients with AD concerning clinical features, age of onset, and genetic background has been studied by several groups (described below). Differences in sample collection (full thickness biopsy vs. skin stripping) and processing and tissue (blood vs. skin) used in the assays are known sources of data variability. Finally, investigators have used different “omics” tools (e.g., genomics, epigenomics, transcriptomics, proteomics) to study complex disease conditions like AD and generate potential omics candidates (e.g., candidate genes, transcripts, proteins, lipid mediators). However, thus far, there is little effort to either integrate the omics datasets or explore the overlap between omics candidates, which could potentially reduce variability.
The clinical variability in AD has been extensively reviewed by Bieber et al. (22). Interestingly, though both lesional and non-lesional AD demonstrate epidermal barrier defects as demonstrated by higher TEWL, significantly elevated allergen-specific serum IgE may (extrinsic AD; most frequent) or may not (intrinsic AD; also called non-atopic eczema) be present in symptomatic patients, indicative of two distinct subtypes, with higher IL-17 levels in intrinsic AD (23–25). Clinical phenotypic variability in AD may include time of disease onset (infancy, adolescence, adulthood), likelihood of persistence into adulthood, severity (mild-moderate, severe), co-existence of other allergic disease, co-existence of mendelian disorders associated with AD (e.g., Netherton's syndrome), IgE-mediated food or aeroallergen sensitization, presence of Staphylococcus aureus colonization and presence or absence of viral infections (e.g., eczema herpeticum) (22, 26).
In addition to clinical heterogeneity, the roles of age, gender, and race on manifestation/variability of AD symptoms have also been recognized. Esaki et al. demonstrated that young patients with AD (0–3 years old) may have a distinct immune activation pattern compared to that of older children with AD (27). Additionally, recent data indicated a relationship between a patient's ancestry and the underlying immune activation in AD. Noda et al. pointed out that Asian patients with extrinsic AD showed a phenotype with increased elongated epidermal rete ridges and parakeratosis similar to psoriasis (28). Skin biopsy specimens from Asian patients showed TH17/TH22-dominance compared to European American patients. The immune activation pattern in other ethnicities, such as African American, remains to be elucidated. Similar to clinical, age and race variability, treatment response variability is observed for AD. For example, promising yet variable response to treatments has been demonstrated in Dupilumab trials (reduction in Global Assessment score to 0 or 1 in 38% of patients) (7, 26, 29–31). Collectively, clinical, age, and race variability, along with differential response to treatment, in patients with AD demonstrated the need for patient-tailored treatment strategies (29).
Several endotypes of AD have been proposed on the basis of omics data (22). For example, Thijs et al. (12) analyzed 147 serum analytes (representing biomarkers for apoptosis, chemokines, growth factors, complement activation, epithelial cytokines, galectins, glucose regulation, immunomodulatory cytokines, inflammatory biomarkers, innate immunity biomarkers, leukocyte migration biomarkers, neutrophil/granulocyte biomarkers, proteases, and protease inhibitors, remodeling biomarkers, TH2 cytokines, vascular regulation, viral response and serum total and allergen-specific IgE) obtained from patients with moderate to severe AD (n = 200). A group of healthy, non-atopic subjects (n = 30) were used as controls. Principal component analysis revealed four clusters, each potentially representing a distinct endotype (12). However, neither candidate-focused nor global/omics-level information have been provided from other layers (e.g., epidermal differentiation complex gene variants, methylation, epidermal lipid composition, cutaneous Staphylococcus infection). Though serum-based studies provided useful hints about probable endotypes, interrogating more than one omic layers would be useful to better understand the root causes of clinical variability in AD.
Although a number of potential endotypes were suggested for AD on the basis of clinical phenotypes, the biomarkers for AD and AD endotypes are still under investigation. Biomarkers derived from an individual “omics” layer may not be sufficient to define all endotypes, underscoring the need to better connect candidate biomarkers to achieve an integrated systems-level view of AD pathogenesis and endotypes. Thus far, there are no specific treatments, nor biomarkers, particularly indicated for specific AD endotypes. Research is needed to connect AD endotypes suggested from studies involving individual “omics” layers.
Unraveling the Pieces of the AD Puzzle: Role of Omics
Omics approaches have been applied to interrogate patient samples to identify endotypes and associated biomarker(s). Most studies, however, are restricted to only one omics layer, such as genomics or serum proteomics (12). Results interrogating multi-omics data, such as microbiome, proteome, lipidome, transcriptome, epigenome, exposome, and genome, are largely lacking. Publicly accessible databases can serve as powerful resources of omics-level data to unravel new biological insights into the etiology of AD and to confirm previously reported AD genes and pathways (32).
Genomics Data
International collaborative efforts have generated genome informatic resources and databases, such as HapMap, 1000 genome and dbSNP, that are accessible to researchers searching for gene variants, mutations and other population genetics information. The genome-wide association study (GWAS) catalog (https://www.ebi.ac.uk/gwas/) represents a database of published GWAS. There are at least 12 GWAS for AD. Mining the GWAS catalog using “AD” as the query term showed genes (FLG, KIF3A) that are also known as AD-relevant genes from global expression studies (33–35). Interestingly, all GWAS indicated that AD is associated with immune regulation genes and cutaneous barrier function genes as indicated in Table 1 (43–47). In addition, a recent GWAS has indicated that asthma, hay fever and eczema partly coexist owing to their shared genetic risk variants leading to dysregulated expression of immune-related genes (48). AD-associated genes can be retrieved from the GWAS catalog and used for an enrichment analysis to identify significant disease-associated pathways. Molecular and cellular biologists may also query specific genetic variants for levels of gene expression in different tissues by searching GTEx and pathways from KEGG and Reactome (49–52).
Apart from the well-known null mutation, a general down-regulation of filaggrin (FLG) expression has been reported in AD (53, 54). FLG is the gene most replicated in AD GWAS among multiple ethnic groups. It is located in the chromosome 1q21 region, which represents a gene cluster encoding proteins involved in the epidermal differentiation. Clinical data indicate that FLG loss-of-function mutations may represent a patient sub-population with severe, early onset, extrinsic AD that may persist into adulthood (55). On the other hand, KIF3A is a member of the kinesin superfamily of microtubule-associated motors and is important for transporting protein complexes within cilia (56). Down-regulation of this protein may be related to insufficient aeroallergen clearance (56). Recent association studies have linked KIF3A with AD (57, 58) and childhood asthma (59). Although single-nucleotide polymorphisms (SNPs) have been identified for AD from GWAS, the functional implications and mechanisms of the associated loci are largely unknown. Genomic variants alone are not able to explain the changes in disease risk along an individual's entire life span. DNA, RNA, protein, and their metabolites often have complementary roles to jointly perform a certain biological function.
Epigenomics Data
Epigenetic data can lead to information regarding heritable changes (methylation, histone modification and non-coding RNA-mediated gene silencing etc.) expression without involving modifications in the DNA sequence. Potential roles of epigenetic modifications in allergic diseases has recently been highlighted (60). A limited number of studies at the epigenome scale have been performed on samples from patients with AD to identify epigenetic signatures related to this condition. Hypomethylation of the promoters of TSLP and FCERG (encodes FcεRγ, a high-affinity IgE receptor chain) are responsible for over-expression of these genes in AD (61). Rodriguez et al. described significant methylation differences for various CpG sites between lesional AD skin samples vs. healthy control skin. These methylation differences partially correlated with differences in transcript levels of epidermal differentiation and innate immune function genes (62). Transcriptome data from this study can be obtained from NCBI GEO (GSE 60709) and used to identify pathway-level changes between lesional and non-lesional AD associated with detected epigenomic alterations.
Overexpression of the high-affinity IgE receptor on monocytes and dendritic cells can contribute to AD manifestation (63). Monocytes from patients with AD showed global-, as well as locus-specific hypomethylation at the FCERG promoter, compared to monocytes from healthy control subjects. Interestingly, this hypermethylation is inversely correlated with FCERG expression. Ziyab et al. also reported the synergistic roles of FLG genetic variants and differential DNA methylation on the development of AD (64). In another study, no significant difference was observed in genome-wide DNA methylation levels of whole blood, T cell, and B cell samples obtained from AD cases and controls (62). However, DNA methylation differences were not correlated in blood and skin samples, indicating that blood may not be an ideal surrogate for skin, but show the potential involvement of epigenetic factors in AD (62). In addition, two preliminary studies regarding the role of FLG methylation on allergy and AD were performed using buccal cell DNA or whole blood DNA and yielded conflicting results, potentially indicating the roles of tissue-specific epigenomic changes (64, 65).
Epigenetic databases, tools and resources are growing rapidly due to active research in this area, including the NIH Roadmap Epigenomics Mapping Consortium and the preparation of the human epigenome atlas (http://www.genboree.org/site/). The NCBI Epigenomics database was built as a repository for whole epigenetic data sets and was later merged to Gene Expression Omnibus (GEO). DiseaseMeth (http://202.97.205.78/diseasemeth/index.html) is a web resource hosting human disease–related, curated methylome data of more than 175 datasets extracted from methylation arrays and sequencing results, as well as scattered, aberrant methylation information. However, there are limited data available on the AD-related epigenome.
Transcriptomic Data
AD transcriptome has been studied in patient samples cross-sectionally using microarray-based or RNAseq-based methods (66–68). Publicly accessible databases, such as NCBI GEO and EBI (European Bioinformatics Institute) Array Express, can serve as important resources of transcriptome data (32). Multi-origin transcriptome data obtained from public databases have been previously analyzed to obtain valuable insight into the AD disease profile (69, 70). Using this approach, our group recently developed a panel of 89 genes that can be used as a transcriptomic signature for AD (69). These differentially regulated genes were categorized under the following functional classes: (a) barrier function–related genes, e.g., LCE2B, LOR; (b) dysregulated lipid genes, e.g., FADS1, FABP7; (c) chemokine/cytokine genes, e.g., CCL17, CCL18; (d) protease and protease-inhibitor genes, e.g., KLK5, SERPINB3; (e) genes related to anti-microbial function, e.g., MSMB, LTF; and (f) genes of diverse metabolic functions, e.g., ARGAP18. Interestingly, the transcriptional signature from this unique combination of 89 genes could discriminate AD from control skin biopsy samples with a very high degree of predictive accuracy. Another meta-analysis–based study of the AD transcriptome data identified the relevance of the atherosclerosis signaling (IL-37, selectin E) pathways combined with the wide-range lipid and Th2-gene abnormalities in AD (70).
Proteomic Data
With the availability of quantitative proteomic data resources, peptide abundance, modifications, and interactions are increasingly used to analyze molecules secreted by a variety of immune cells. High-resolution protein separation and mass spectrometry–based applications have generated a large volume of proteomic data that are deposited in proteomic databases for reuse (71, 72). Quantitative proteomic diversity in human tissues and organs in disease and health has been extensively investigated resulting in comprehensive human proteome databases (73, 74). The draft map of the human tissue proteome has been detected from > 80 % of the annotated protein-coding genes (75). Though the Human Proteome Project (HPP) was initiated by the Human Proteome Organization (HUPO) to understand the entire human proteome at the cellular level, its biology and disease-oriented branch (named B/D-HPP) supports state-of-the-art assessments of human proteome in health and disease (76). The Plasma Proteome Database (PPD) was developed by the HUPO as a part of the HPP, which shows great promise in understanding the plasma proteome function in health and disease (e.g., grouping plasma proteins with cardiovascular functions and their roles in heart disease) (77–79). However, thus far, there is no similar initiative to explore the AD proteome. Searching other proteomic databases, such as PRIDE (proteomics identifications database) (80–82) and GPM (global proteome machine) (83, 84), which represent very rich resources of raw proteomic data, using the search term “atopic dermatitis,” did not retrieve relevant results (search performed on 1st September, 2017).
Proteomic data related to AD can also be obtained by literature search from Uniprot, which is a well-known database of protein sequences and their functional information, some of which is derived from DNA sequences. Querying the Uniprot database using the search terms “atopic dermatitis” AND “organism:Homo sapiens (Human) [9606]” retrieved 27 AD-related proteins (retrieved on 1st September 2017). Selected studies reporting proteomic data are listed in Table 2 (85–87). In contrast to transcriptomic studies, skin proteomic studies thus far used skin taping to obtain samples. However, this method only collects samples from the stratum corneum, the upper skin layer. Thus, results using skin taping samples may not be directly comparable to those using full-thickness punch biopsy skin samples. However, GWA studies strongly indicate the roles of barrier- and protease functions of the stratum corneum in the development of AD. Particularly, filaggrin (FLG) mutations, low profilaggrin/filaggrin monomer expression and skin protease dysregulation have been found in AD. Comparing three independent, stratum corneum proteomic datasets, we identified 20 proteins involved in AD pathogenesis. The proteins in this set of 20 differentially regulated proteins were unique to certain datasets or represented in multiple datasets.
Metabolomic Data
Metabolomic approaches use mass spectrometry and high-resolution proton nuclear magnetic resonance (NMR) to assesses cellular metabolites of multiple small molecule types (e.g., <1,200 Da and biochemical intermediates; i.e., metabolites), including amino acids, fatty acids, carbohydrates or other products of cellular metabolic functions, in order to explore disease metabolome related to allergic diseases (92–94). Information obtained from metabolomics provides useful insights about the biochemical phenotype (i.e., the metabotype). A limited number of metabolomic studies has been performed to analyze the AD metabolome using urine, serum and sweat analyzed by NMR and liquid chromatography–coupled mass spectrometry. In an exploratory study using NMR-based spectral analysis followed by statistical and chemometric approaches, Assfalg et al. noted significant spectral shifts indicative of broad changes in the AD urine metabolome compared to that of age-matched, non-atopic controls (95). Subsequently, Huang et al. used high-performance liquid chromatography–coupled mass spectrometry to investigate metabolic abnormalities in AD using fasting sera obtained from children with AD and healthy control subjects. Subjects with AD with high IgE showed significant differences in multiple metabolic intermediates, including carnitines, free fatty acids and lactic acids, indicating metabolic abnormalities. In contrast, higher levels of cytochrome P450 and epoxygenase metabolites were reported in the AD group with normal IgE. Statistical modeling discriminated high IgE AD from low/normal IgE AD and control samples in scattered plots. In a targeted metabolome analysis, the investigators analyzed thirty eicosanoids, which are products of the arachidonic acid pathway and mediate inflammation.
There are several recently released metabolomic databases in use. Examples include HMDB (The Human Metabolome Database), BiGG, and SetupX, among others (96–98) The HMDB is a publicly accessible database that contains detailed information regarding small molecule metabolites related to the human body (96, 99, 100). This database is designed for applications in metabolomics, clinical chemistry, and biomarker discovery. However, none of these databases presently contain AD-focused or atopy-related metabolome data.
Lipidomic Data
Lipidomics involves system-wide identification and quantitation of lipids with lipid-associated pathways and networks. Lipids play distinct roles in mediating inflammation, as well as maintaining the skin moisture content. Genomic, transcriptomic and proteomic data strongly indicated the involvement of cutaneous lipid dysregulation in AD. However, only a few studies have directly addressed AD lipidomics thus far. A recent study aimed to characterize the lipid mediator profile in sweat samples obtained from patients with non-lesional AD and non-atopic controls (n = 23/group) using liquid chromatography–mass spectroscopy and mass spectroscopy. Increases in the C30–C40 ceramide and C18:1 sphingosine concentrations were found in AD patients despite no differences in TEWL between study groups, and this effect was strongest in men. No differences in oxylipins and endocannabinoids were observed between study groups. The increases in short-chain lipids do not support the current report indicating a general ceramide deficiency in AD (101). However, an imbalance between different ceramide groups was observed, indicating that ceramide species involved in barrier function and keratinocyte signaling are dysregulated in AD. Lipid abnormalities in AD have also been shown using skin and sweat samples (102–104).
Searchable interactive databases for lipids and lipid-associated proteins, in combination with mass-spectrometric and other experimental approaches, offers an opportunity to construct lipid metabolic networks and to devise therapeutic strategies (105–108). LMSD (lipid maps structural database) contains structures and annotations of biologically relevant lipids. Lipidomic pathways and networks can be constructed from experimental data. Interrogating the epidermal lipidome has been described as a tool to assess and predict the progression of inflammatory skin diseases (109). In spite of the recent reports showing the roles of epidermal and sweat lipidome in AD, relevant lipidomic data generated by independent investigators are not usually made available through publicly accessible databases (104, 110–112).
Microbiome Data
The human skin is colonized by microorganisms, including bacteria, viruses and fungi, collectively known as the microbiota, and their genes constitute the microbiome. Skin microbiomes in AD have been studied in the recent years using samples obtained during in-flare and out-of-flare conditions (113, 114). AD treatments can also lead to increased epidermal bacterial diversity preceding improvements in symptoms. Staphylococcus sequences (mostly S. aureus) were more abundant during disease flares (lower microbial diversity), compared to baseline or post-treatment conditions (higher microbial diversity), and correlated with disease severity (113, 115). Modern high throughput studies also indicated a higher representation of skin commensal S. epidermidis during flares which was not previously demonstrated in culture-based studies (113). Increases in Streptococcus, Propionibacterium, and Corynebacterium species were observed following therapy. These findings reveal connections between microbial communities and inflammatory diseases such as AD and demonstrate that high resolution examination of microbiota associated with human disease can provide novel insights into global shifts of bacteria relevant to disease progression and treatment.
Interestingly, altered epidermal lipid composition has been found to correlate with the status of S. aureus colonization on the AD skin (116). In general, a number of studies indicated a clear difference between AD- and healthy skin microbiome, which can be associated with disease state, i.e., appearance of flares (113, 117, 118). AD skin often shows S. aureus infection, which exacerbates the disease through inflammatory mechanisms. Microbiome studies indicated that coagulase-negative Staphylococcus strains having antimicrobial activity are common in the normal population but rare in subjects with AD. The antimicrobial activity has been related to previously unknown antimicrobial peptides produced by coagulase-negative species such as Staphylococcus epidermidis and Staphylococcus hominis. Our present knowledge clearly indicates that some commensal bacteria, that are deficient in AD skin, can protect against harmful S. aureus infection and can have a great translational value in reducing infection and inflammation (119). Another study showed that topical corticosteroids might influence the lesional AD microbiome, with the post-treatment AD skin microbiome resembling the non-lesional AD skin microbiome but both exhibiting distinct characteristics from the normal skin microbiome (120). Additional studies on gut microbiome have also suggested that alterations in the gut microbiome may be associated with an altered host immune function related to AD.
Over the last few years, identifying the composition of microbiome has progressed enormously with the application of high-throughput DNA sequencing technologies based on two principal methods. The first method is based on sequencing the 16S rRNA gene of bacteria (121). Identifying variations in specific regions of this gene allows decent classification of bacterial taxa. 16S is relatively inexpensive, but has limited resolution (not all bacteria can be classified, species-level identification may not be possible), nor can the 16S method detect viruses and eukaryotic communities. The second method, metagenomics sequencing, is based on sequencing of all DNA fragments and aligning them to reference databases from all life forms (122). This approach is capable of identifying bacterial, viral, fungal, and protozoan DNA. It produces a much better resolution of bacteria at the species level. However, this method is associated with high sequencing costs, significant bioinformatic load (due to the large number of sequence reads produced), and the inability to analyze genomes not present in the reference databases or genes with unknown function. The statistical methods widely used in microbiome analysis mostly derived from ecological studies, including alpha diversity (diversity of species or other taxa within a sample) and beta diversity (difference in taxonomic composition between samples). However, unlike ecological studies, microbiome researchers are dealing with relatively large numbers and employ additional multidimensional statistics and complex statistical approaches, including pathway and network analysis. The NIH human microbiome project (HMP) has generated data to interpret the role of the microbiome in human health and disease. This project has generated a large volume of publicly accessible metagenomic sequence data from five major body locations (including skin and gut) of healthy and disease (preterm birth, inflammatory bowel disease, and type-2 diabetes) cohorts. More AD-focused data is required.
Exposome Data
The exposome is defined as the totality of environmental exposures over the life course, with exposure timings ranging from prenatal to post-natal periods. It is now well-recognized that human omics alone cannot explain the rise in allergic diseases in the industrialized world, clearly indicating the role of the exposome in allergic diseases including AD (123). Birth cohort studies indicated that prenatal exposure to antibiotics through expecting mothers' antibiotic consumption might be associated with an increased risk for AD, particularly within the children of mothers with atopy. Antibiotic exposure also might occur through breast feeding. Both prenatal and post-natal exposure to antibiotics may influence intestinal microbial diversity of the neonates, leading to subsequent manifestation of atopic diseases (124–126). Other prenatal exposures include oxidative stress induced by maternal distress (127). maternal exposure to air pollutants (128, 129), smoke (130, 131), phthalate (132), cadmium (133), house dust mite (134), house pets, and farm animal (protective effects) (135).
Numerous studies have been designed to assess the role of post-natal exposures on AD. There is growing evidence that the use of hard water might be a risk factor for developing AD (136–138). According to a recent study, fall/winter birth season and exposure to hard water were associated with increased relative prevalence of AD in the first 18 months of life (136). Other potential factors included meteorological factors (139) and pollutants (139–141), allergenic grass pollen (142) mold (143, 144), probiotics (145–147), and vitamin D exposure (148–150). Although, high-throughput techniques have been developed and have been widely used for omics such as transcriptomics, proteomics, metabolomics; exposure to environmental stressors affects each of these components (genes, transcript, proteins, metabolites). Thus, there is a need to combine omics with environmental exposure measures to better understand the complex network of cellular responses to the exposome.
Exposome-Explorer (http://exposome-explorer.iarc.fr) is a database supported by the World Health Organization. It is dedicated to biomarkers of exposure to environmental risk factors (151). It contains detailed information on the nature of biomarkers (e.g., dietary and pollutant) and their concentrations in various human samples (e.g., blood, urine). Recently there is a great interest in exposome studies to identify disease risks. However, thus far, there are limited data pertinent to AD in exposome databases.
Omics-Wide Integration—Putting the Pieces Together
Each omics data type typically provides a list of differential factors potentially associated with the disease. These data can be useful as disease markers while providing insight as to which biological pathways or processes are different between the disease and control groups. However, analysis of only one omic(s) data type is limited to correlations and provides a partial view of the biological system. Integrating different omics data types is often used to elucidate the potential causative changes that lead to disease or can be used to identify potential therapeutic targets for further molecular studies. For example, a GWA study can reveal the statistical associations between genetic loci and disease status. While it can suggest potential causal effects, pinpointing particular causal variant(s) and associated molecular mechanisms can be challenging. On the other hand, gene expression or epigenomic profiling can detect associations between disease and genes or epigenomic markers, but these associations are correlative in nature. By integrating different types of data, it becomes possible to circumvent the limitations of individual studies and better identify disease-causing DNA variants and their downstream molecular targets. For instance, when DNA and RNA are measured simultaneously, it is possible to determine whether a particular genetic variant affects the downstream expression of a gene in a “genetics of gene expression” (expression quantitative trait loci; eQTL) analysis. Furthermore, if a genetic variant resides in a functional site associated with transcription factor binding, epigenetic modification, or protein regulation, as revealed by the ENCODE project, it becomes possible to narrow the basis of potential targets. Co-analyses of genomic data with expression profiles from either the transcriptome, proteome or methylome help to identify the quantitative trait locus and eQTL, proteome QTL, or methylome QTL. Integrating transcriptome and proteome data has also led to the discovery of post-translational activities and correlation between two omics types under identical conditions. A network-based method, along with omics data integration approaches, will be discussed in the data integration section.
Omics Integration to Address AD Etiology and Clinical Variability/Endotypes
Clinical management of AD is often challenging due to its heterogeneity with multiple endotypes (e.g., intrinsic vs. extrinsic, with or without other allergic or immune diseases, mild or moderate vs. severe forms, Staphylococcus-infected vs. uninfected forms). Therefore, much of current research has been focused on gaining a better understanding of the endotypes with omics data. However, these studies are often underpowered. In an attempt to connect endotypes with transcriptome data, Martel et al. (152) described that in mild, extrinsic AD (total IgE >200 kU/L; n = 5), the expression of most skin barrier genes, including filaggrin and loricrin, remained unchanged (or moderately upregulated) compared to controls (n = 9), despite the presence of heterozygous mutations in the filaggrin (FLG) gene in majority of patients. On the other hand, mild, intrinsic AD (n = 9) resembled mild psoriasis (n = 9), rather than AD, when expression profiles of genes involved in inflammatory responses were compared between these conditions (152).
In AD, the skin is infected with viral and bacterial strains in at least 30% of patients, while about 90% of active skin lesions are associated with Staphylococcus infection (153). However, studies so far have not been performed to directly connect the skin microbiome with transcriptome or any other omics layer in AD. Transcriptome studies have linked infection with down-regulation of innate immune genes (such as genes coding antimicrobial peptides) in the epidermis (69). Down-regulation of a wide array of innate immune genes, like LTF, MSMB, RNASE7, and SCGB2A1, has also been shown from AD transcriptomic studies, which might be linked to increased susceptibility to microbial infection in AD (69).
Filaggrin is important for the formation of stratum corneum layer and also for its hydration of this barrier properties. Subjects carrying mutations in the FLG gene produce dry, flaky skin and potentially get sensitized to allergens due to enhanced exposure (154). To assess the effect FLG loss-of-function mutations on skin transcriptome, uninvolved skin of pediatric AD patients (n = 26) has been compared with site-matched samples from controls (n = 10 non-atopic) (155). Cases and controls were screened for FLG genotype to stratify the transcriptome data. Interestingly, FLG wild-type cases showed dysregulation of genes involved with lipid metabolism, while FLG haplo-insufficiency affected global gene expression and was characterized by a type 1 interferon–mediated stress response. This study, along with clinical stratification based on FLG status, suggested that subjects with the FLG loss-of-function mutation might represent a specific endotype characterized by early onset disease with more severe and generalized manifestation of symptoms (156) However, there is an insufficient number of genomics studies to further define AD endotypes.
Omics Integration: Biological Relevance and Approaches
Genes identified from genomic (GWAS), epigenomic and proteomic studies can be used to build AD disease- associated networks from each omics level and a network-level overlap can be calculated. Previous studies identified centrally located network genes or “hub genes” from individual omics layers (69). For a more integrated systems level overview, AD-relevant candidates (genes/transcripts/proteins) from each omics level could be combined to build a disease network. Significant AD-relevant genes can be prioritized from omics databases, or from experimentally derived multi-omics data available from existing literature using standard literature interrogation tools (157). This comprehensive approach (inclusive method) uses advanced analytics to explore causal relationships between omics candidates and disease conditions/ pathways giving insights not achievable from any single-layer omics data.
Figure 2A represents network of 52 AD-relevant candidates from multi-omics approach (Ingenuity Pathway Analysis, Qiagen, USA). These candidates contain members from different functional groups such as keratinocyte development, cutaneous barrier function, inflammation, fatty acid metabolism and cytokine function. Their functional interaction can be visualized by STRING protein network (Figure 2B). STRING v10.0 (http://string-db.org/) is a database of known and predicted protein interaction, including physical and function association (158). Furthermore, these candidates can be used to develop a tissue-based expression heatmaps to directly explore their functional relevance in disease-relevant target tissue(s). Figure 2C represents a tissue-resolved expression heatmap of 52 AD-relevant candidates using FUMA (a functional genomic annotation tool) (159). The heatmap, when clustered by AD genes/ candidates and by target tissues, shows the relatedness of skin and esophagus in the context of AD-relevant candidates, which potentially explains the shared etiology between AD and food allergy. Our findings suggest that the pathways shared between AD and other allergic diseases might have significant functions in skin, esophagus, vagina—areas characterized by shared structural protein components. Particularly, the functional significance of structural proteins co-expressed by oral and skin tissues have been described (160). By applying gene ontology (GO) enrichment analysis, we found that these candidate genes are associated with epidermal development, keratinocyte differentiation, and epithelial cell differentiation pathways were most differentially enriched in asthmatics. Interestingly, many of these pathways derived from these candidate genes have also been related to Th2 pathway, immune cells, and response to cytokine.
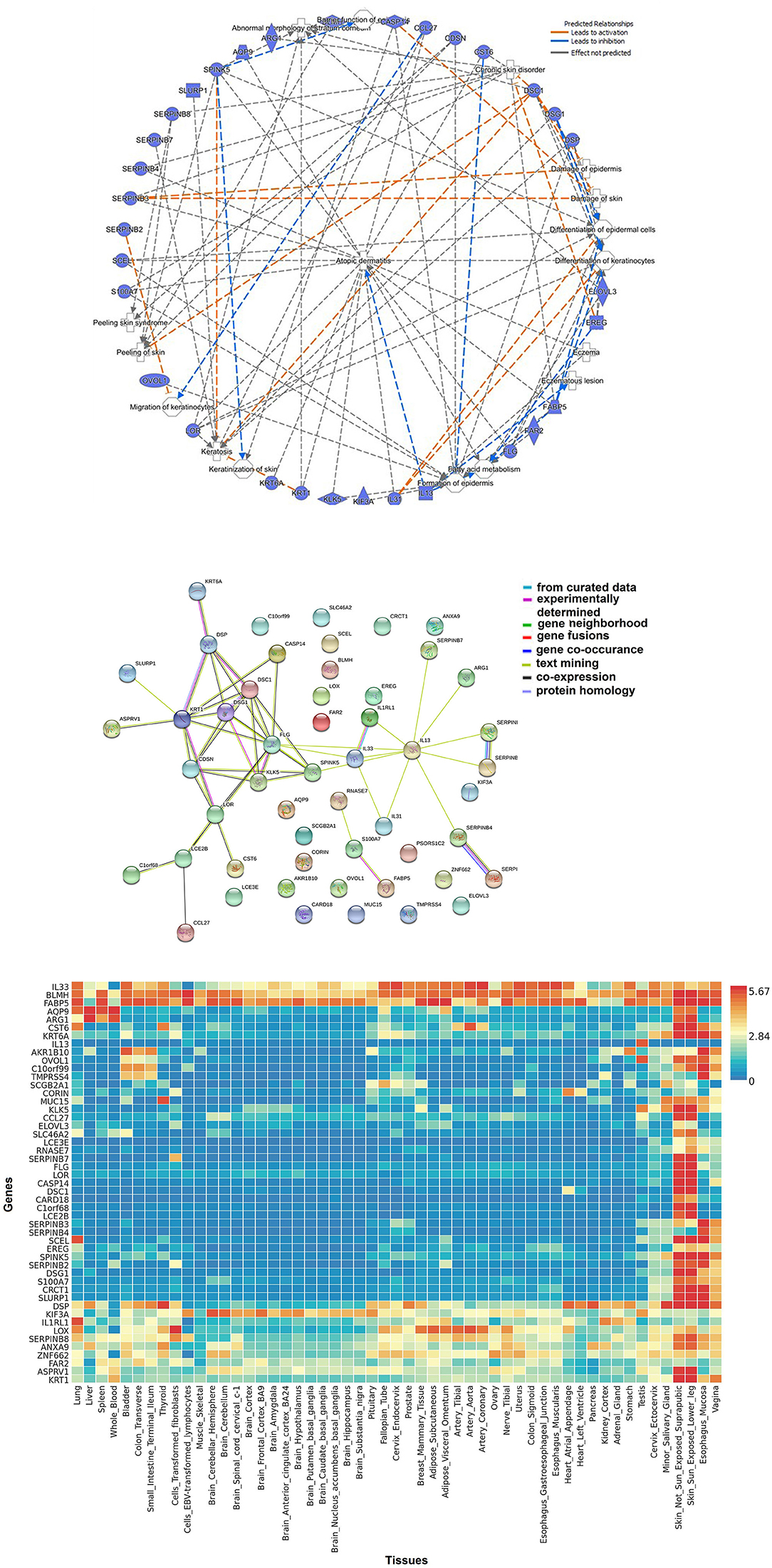
Figure 2. Biological approaches of omics integration: (A) Network of significant AD-relevant candidates from multi-omics data. These candidates contain members from different functional groups such as keratinocyte development, cutaneous barrier function, inflammation, fatty acid metabolism, and cytokine function. (B) Protein-protein interaction networks: functional interaction was visualized using STRING protein network. STRING (http://string-db.org/) is a database of known and predicted protein interaction, including physical and function association. STRING quantitatively integrates interaction data derived from genomic context, high-throughput experiments, co-expression and previous knowledge [Szklarczyk et al. (158)]. Connections, based on co-expression and experimental evidence have been shown. Filled nodes indicate genes; edges between nodes show protein-protein interactions between protein products of the corresponding genes. Different edge colors represent the types of evidence for the connection. (C) Tissue-based expression heatmap to directly explore the functional relevance of AD-relevant candidates/genes clustered by genes and by tissue type. The heatmap indicates the relatedness of skin and esophagus in the context of AD-relevant candidates.
We further ran an overlapping analysis of candidate genes identified from multiple omics levels. Figure 3 represents an omics data integration by multi-omics overlap. The genomic, epigenomic, transcriptomic and proteomic candidates that are associated with AD have been obtained from GWAS catalog and from published epigenomic, transcriptomics, and proteomic experiments. Individual omics datasets were analyzed, and Venn diagrams were produced to visualize the overlap between genes and pathways. The result shows that filaggrin was associated with AD from four omics (genomic, epigenomic, transcriptomic, and proteomic) level experiments, followed by SERPINB3 (reported from most transcriptomic, epigenomic, and proteomic studies). The number of candidates from each omic level might increase, as more data will be available from different levels. Interestingly although we observed a limited gene level overlap between different omics-level data in AD (candidate included SPINK5, KLK5, FLG, CRCT1, ARG1, S100A8, and CCL27 reported ≥2 omics level overlap) there was a remarkable overlap at the pathway level. The pathway-level overlap included macrophage, endothelial cell and fibroblast activation pathways, Th1/Th2 lymphocytes and NFkB activation pathways.
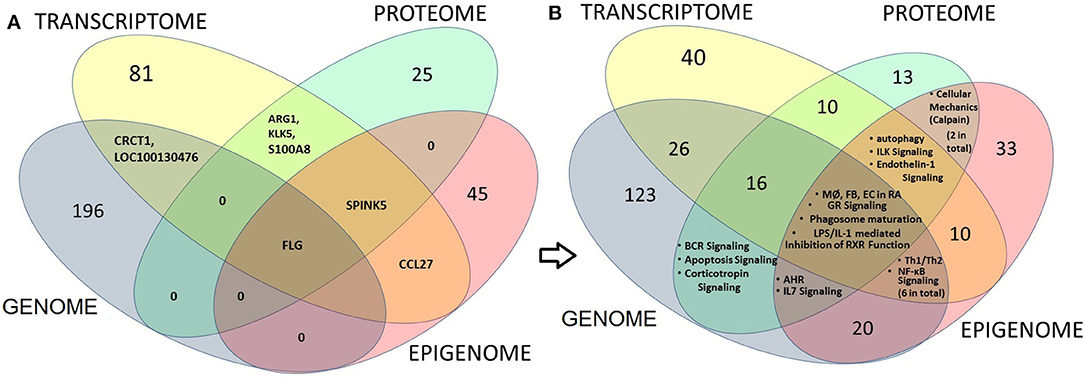
Figure 3. Multi-omics overlap analysis at gene and pathway level to integrate and identify AD candidate loci (at gene, transcript or protein) level (left panel) or at the pathway level (right panel). The genomic, epigenomic, transcriptomic and proteomic candidates that are associated with AD have been obtained from GWAS, from published epigenomic, Transcriptomics, and proteomic data respectively. Venn diagrams show the overlap between candidate gene, transcript or proteins (left panel; A) or between pathways (right panel; B) derived from each level. Numbers indicate the numbers of shared candidates or pathways. The result shows that only one candidate i.e., filaggrin (FLG) could be associated with AD from four omics (Genomic, epigenomic, Transcriptomic and proteomic) level experiments, followed by SERPINB3 (reported from most transcriptomic, epigenomic and proteomic studies; A). Interestingly we noticed that although there is a limited overlap between candidates obtained from individual omics-level data, while the candidates demonstrated considerable overlap at the canonical pathway levels showing the significance of macrophage (MØ), endothelial cell (EC) and fibroblast (FB) activation pathways (from GWAS, transcriptome and Epigenome—four omics levels) as found in rheumatoid arthritis (RA), as well as Th1/Th2 activation and NFkB activation pathways (GWAS, transcriptome and Epigenome) and autophagy, ILK and Endothelin-1 signaling pathways (transcriptome, proteome, and epigenome) in AD (B).
The potential role of NFkB in the manifestation of inflammatory responses in AD is well-described (161). In fact, NFkB -targeted therapies, although not very specific to AD, have shown promising results in a mouse model of AD (162–164). The role of Th1/Th2 lymphocytes in AD has been the subject of many studies, but the significance of other cells and tissues, particularly macrophage, endothelial cell and fibroblasts, is relatively unexplored. However, their involvement in AD is strongly indicated by our multi-omics approach. Experimental data indicated that fibroblasts from the skin biopsy samples of patients with atopy modulate the proliferation and terminal differentiation of keratinocytes obtained from control subjects; this modulation leads to alteration of the keratinocytes' morphologic features, which could be restored by healthy fibroblasts (165). These effects were linked to low expression of the differentiation-associated cytokine leukemia inhibitory factor by fibroblasts from subjects with AD (165). On the other hand, experimental data also demonstrated that granulocyte macrophage colony-stimulating factor (GM-CSF) was overproduced by keratinocytes in AD, which was linked to sustained dendritic cell activation in AD skin (166). A significant role of macrophages in the manifestation of AD has been reviewed by Kasraie et al. (167). Experimental data further demonstrated an increased macrophage infiltration of the skin and interactions through surface-bound IgE and by secreting chemokine mediators. Human macrophages are also activated through its surface-expressed IL-31RA, particularly when exposed to staphylococcal enterotoxin in AD (168). Finally, the central role of epithelium has long been recognized in the pathogenesis of asthma and AD (169). Skin and airway epithelium produce TSLP, which directs the cross-talk of epithelium and dendritic cells to augment allergic inflammation. It can activate neurons to induce itch in AD (170). Epithelium-derived TSLP can also directly initiate Th2 type cytokine production by mast cells, providing a T cell–independent route to pro-allergic responses (169). Interestingly, since TSLP induction occurs through epithelial Toll-like receptors, TSLP-mediated allergic manifestations represent an important link between innate immunity and allergic disease (169). In summary, published experimental data strongly support our multi-omics results, which demonstrate the role of other cells (i.e., macrophage, endothelial cell, fibroblasts) beyond the lymphocytes and of barrier function proteins (e.g., filaggrin, occludin, claudin) in AD.
Our multi-omics approach also suggests the roles of autophagy, integrin-linked kinase (ILK) signaling and endothelin 1 (ET-1) signaling in AD. Though ILK signaling has not been well-explored in the context of AD, there are reports showing that autophagy and ET-1 might play very significant roles. ILK activation might be a result of S. aureus infection, which is frequently associated with AD skin (171). The role of autophagy in regulating keratinocyte inflammation in case of skin diseases, including AD, has been previously discussed (172–175). Interestingly, experimental data also suggest that Staphylococcus strains might persist within keratinocytes by stimulating autophagy (176). This promotes the degradation of inflammasome components and facilitates Staphylococcal survival. Thus, autophagy is relevant in explaining the persistent, methysilate-resistant Staphylococcal infection in AD (176). However, the roles of ET-1 in AD have been better explored. ET-1 functions as a pruritogenic mediator in the manifestation of AD symptoms (177). Its multifaceted roles in AD have been investigated by several groups, and its plasma concentration has been correlated with AD disease severity (178–180).
Biological data from multi-dimensional information levels are highly inter-connected and when combined, can provide meaningful insights. Genetic variants are subject to epigenetic regulation, which helps determine the level of gene expression producing “intermediate phenotypes” and ultimately alter an individual's risk of disease development or disease severity. For example, a combined epigenetic-transcriptomic analyses of epidermal samples obtained from AD lesions compared to healthy epidermis, indicated differences in methylation status, which partially correlated with differentially regulated transcript levels of epidermal differentiation and immune response genes (61, 62). Although there is a limited number of reports in the literature that use a multi-omics approach to interrogate AD samples, additional results from multi-omics studies are expected in the future due to the increasing availability of cost-effective omics tools that can generate big data utilizing a minute amount of a patient sample. However, this requires collecting samples for each subject for multiple omics applications. Ongoing research is directed toward utilizing minimally invasive methods, such as tape striping, which could help obtain samples (microbiome, DNA, RNA) from the same affected site for multi-omics experiments.
Approaches to Integrate Omics-Wide Data
Omics resources and omics integration tools exist (represented in Table 3). The rationale to develop systems biology technologies that integrate multiple omics data types for molecular taxonomy of complex diseases has been emphasized by several authors (9). However, this approach has not been used in AD so far. Computational biology tools have emerged in the recent years for integrating high-throughput data using multistage and meta-dimensional approaches to meet the demands for within and between omic-level integrations. An integrative approach to interpret multi-omics data can significantly alleviate the bottlenecks by providing valuable insights may not be achievable by any single-layer omic approach. Gene network and pathway modeling approaches to integrate resources from different omics domains are being developed to address this knowledge gap (9, 181–187). Network analysis investigates the functional and physical interaction among genes (188). A widely used approach to translate diverse analytic data into biological understanding is by projecting and visualizing the data onto curated biological pathways (e.g., KEGG). Bioinformatics and visualization tools allow the investigators to identify co-regulated genes, metabolites, and proteins in an intuitive and easy-to-use manner. For example, pathway-based analysis tools that integrate GWAS with curated pathways and accept eQTL information have been developed (189, 190). In addition to knowledge-based pathway analysis, data-driven approaches that utilize gene regulation and protein-protein interaction networks could be applied to identify the most likely pathologic perturbations and target genes for disease-associated loci.
Three data integration techniques are widely used: (a) biochemical pathway- and ontology-based, (b) network-based and (c) empirical correlation-based methods (191). (a) Biochemical pathway- and ontology-based methods are based on enrichment analysis in individual omics levels and thus are highly sensitive to the expert definitions of the constituent pathway members. In the most complex disease types, candidate genes and proteins can be integrated at the pathway level rather than at the candidate gene and protein levels. (b) Network-based approaches utilize biological networks involving individual genes, proteins and metabolites that can be used to generate an integrated map of multiple omics-level with results independent of any predefined biochemical pathways. (c) Empirical correlation-based approaches are useful for biological and other meta-data (e.g., clinical outcomes) integration, particularly in the absence of other domain knowledge.
A large number of publicly available tools have been developed for omics data integration based on the above-mentioned approaches (191). Integrated molecular pathway level analysis (IMPALA) is a widely used online tool that accepts gene or protein expression and metabolomics data as inputs and identifies novel pathways from the combined datasets on the basis of over-representation and enrichment analysis from a large number of pre-annotated pathways linked to multiple databases (192, 193). A similar approach has been used by other programs such as iPEAP and MetaboAnalyst (194, 195). iPEAP accepts transcriptomics, proteomics, metabolomics, and GWAS data, whereas MetaboAnalyst accepts data from either targeted profiling (concentration tables) or metabolic fingerprinting approaches (spectral bins, peak lists) from NMR, LC-MS, or GC-MS and performs a huge number of statistical analyses for pathway and biomarker analyses that can be correlated with other omics layers (195, 196). Additional tools are continuously developed to integrate metabolomic data with genomic and pathway data (e.g., RaMP) and transcriptomic data (197, 198). Network-based program suites are available for either online use or installing locally as “R” packages/cytoscape plug-ins (199–201). For example, SAMNetWeb is a web tool capable of accepting two distinct data types (e.g., transcriptome and proteome data) across multiple experiments to identify and visualize activated pathways (199). On the other hand, programs such as pwOmics and metamapper are available as “R” packages (191, 202).
Several empirical correlation-based methods are developed to integrate multi-omics data. For example, weighted correlation network analysis (WGCNA) is an “R” package that can be used for finding clusters of highly correlated genes (gene clusters) (203). It can also be used to connect gene clusters to additional information, such as single-nucleotide polymorphisms (SNPs) and proteomic and clinical data, and thus can be used to identify candidate biomarkers or therapeutic targets (191, 203). These methods have been widely applied in various biological/ biomedical contexts (e.g., cancer, mouse genetics, yeast genetics, brain imaging data) (191). Several powerful omics integration packages have been developed for additional data integration including physiological conditions, biochemical reactions, molecular and mass-spectral similarity. Interestingly, the Grinn package provides a dynamic interface to rapidly integrate gene, protein and metabolite data using both biological (network–based) and empirical (correlation–based) approaches (http://kwanjeeraw.github.io/grinn/).
In addition to omics data, there are novel health data resources that are expected to revolutionize personalized medicine. For example, big data analytics in personalized and predictive medicine of complex diseases, including allergic disorders, has been previously emphasized by several authors (204–206). Leveraging big data (using emerging, data-science tools such as deep-learning) can be very efficient in obtaining insight into complex disease outcomes and suggesting the best use of healthcare resources. Beyond traditional data sources (e.g., electronic health record), novel tools capable of recording personal health profiles captured by individuals themselves are going to contribute a high volume of data to the systems biology of complex diseases. Though traditional omics data represent snapshots from individuals at research and clinic visits, big data can capture intrinsic, and extrinsic variables in real time through recording using novel technologies such as wearable devices and mobile health apps (205). Patients and control subjects can record parameters related to skin care, food, exercise, adherence to medication, and others. In addition, modern sensor technologies allow recording of several parameters, such as physical activity, blood pressure, glucose level and heart rate, but can be extended to include measures of TEWL, lung function, symptom scores, humidity and environmental exposures (205, 207).
Approaches to Connect Omics to Endotypes
Steps involved in a successful identification of disease subtypes and integration of multi-omics data and associated clinical outcomes measures have been described by Roger et al. (9). In such studies, patients were clustered using different omics-level data (exome sequences, transcriptome, and proteome data), and the results were integrated with clinical data to effectively characterize complex disease subtypes. The challenges with this “molecular-data-first” approach, however, need to be carefully addressed. In the case of AD, this model is largely impeded by limited or lack of information at multiple omics levels of particular clinical phenotypes included in the studies conducted thus far. We created a schema for omics integration in AD (Figure 4). Omics datasets can be obtained from publicly accessible databases that are continuously updated with curated data primarily derived from omics-level experiments. SNP set association scores for each gene can be computed on the basis of the SNPs assigned to the respective gene. Differential gene expression, DNA methylation and metabolite (including fatty acids for lipidome) scores can be computed for each gene from gene expression, DNA methylation and metabolite profiles. The gene association scores integrate evidences from expression, methylation, metabolite signature, and the genotype signature for each gene. Finally, the pathway/ network association test can identify gene sets associated with samples of a single endotype by integrating evidence across genes in the gene sets. This can identify molecular subtypes, which after associating with clinical phenotypes, can be used to designate endotypes. Endotype-specific genes and pathways can be useful for rational designing of treatment strategies.
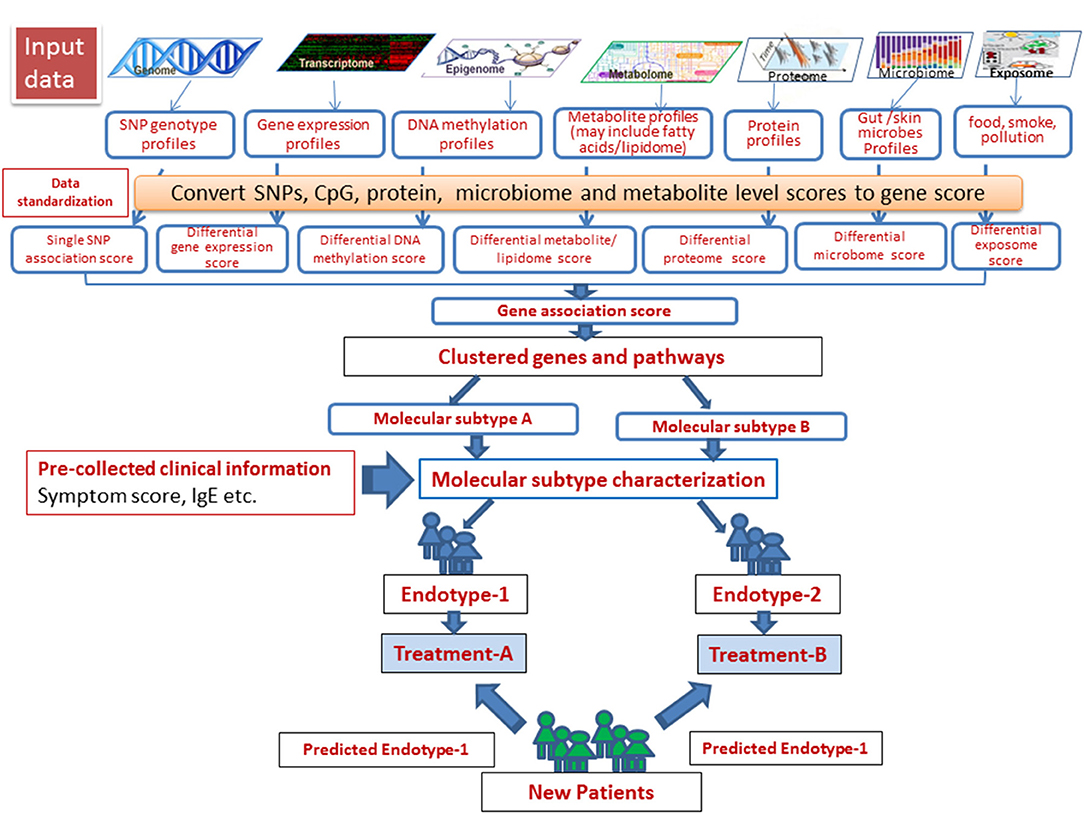
Figure 4. A schema for omics data integration to generate molecular subtypes for personalized medicine. With recent high throughput technologies, a wide range of omics level data has been generated. Omics datasets can be stored in publicly accessible databases that are continuously fed with curated data derived from omics-level experiments and scored to integrate using appropriate statistical tools. Next, the samples can be resolved into groups using integrated scores to identify molecular subtypes, which after associating with clinical phenotypes, can be used to designate endotypes. Endotype-specific genes/pathways can be useful for rational designing of treatment strategies.
Omics-Wide Integration: Recent Advances and Remaining Challenges
The pathogenesis of AD has been attributed to adaptive immune abnormalities, with dysregulated Th1/Th2/Th22 response, IgE production, chemokine signaling, and dendritic cell recruitment, resulting in the itch and inflammatory dermatosis (208). It is increasingly evident that inflammation in AD results initially from inherited and acquired insults that converge to drive structural and functional alterations, followed by immune system activation and compromised skin-barrier homeostasis. This cycle has been designated as the “outside-inside-outside” model of AD (208). Th2 cytokines can down-regulate epidermal barrier protein, which can enhance antigen/allergen exposure and thereby increase inflammation. In spite of evolving models to explain etiology being proposed, the management of AD is primarily aimed at controlling inflammation and itch. The underlying mechanism of early emollient use in preventing AD is still not completely understood (209). In addition to Th2 activation, AD is probably much more heterogeneous, with additional activation of Th1, Th22, and Th17/IL-23 cytokine pathways depending on AD subtype and endotype (210). FLG mutation represents an early onset AD subtype with more severity. Among two other relatively clear phenotypes, intrinsic AD (normal IgE level) shows significantly elevated expression of IL-17, IL-23, IL-22 cytokines associated with S100As, elafin/PI3, CCL20 expression by keratinocytes (thus resembling psoriasis), whereas extrinsic AD (high IgE level) shows similar expression levels of Th2 cytokines (29). Although cytokine-targeted dupilimab therapy shows efficacy in both intrinsic and extrinsic AD, the exact mechanism is not completely clear in the case of intrinsic AD. Contrasting clinical, immunologic and histochemical features of AD and psoriasis have been elaborated elsewhere (211). Both lesional and non-lesional AD can be associated with epidermal barrier defects (as demonstrated by higher TEWL), with or without specific IgE. In molecular terms, psoriasis has been considered as a TH17/IL23–skewed disease, whereas AD has been considered a TH2-centered disease, both sharing TH22 components. However, Martel et al. demonstrated that the transcriptomic signature of intrinsic AD is more similar to psoriasis than to extrinsic AD (152). However, the TH17 and TH22 pathways along with expression of S100A and innate immune genes by keratinocytes are less activated in patients with AD compared to psoriasis (212). In addition to intrinsic AD, results involving Asian AD subjects (mostly extrinsic), showed an induction of the TH17 and TH22 axes (28). Taken together, these studies demonstrate there are molecular subtypes of AD that demonstrate features common with psoriasis, but does not conclusively indicate that they are the subtypes of psoriasis. Nevertheless, molecular subtyping of AD is very important in personalized treatment and results from ongoing clinical trials targeting TH1, TH2, TH17, and TH22 pathways will continue to dissect the patho-mechanisms associated to this complex condition.
The methods to analyze patient omics data into molecular subtypes can be adapted to fit the type and volume of data, leading to characterization of unique and shared pathways for molecular subtyping. Next, standard statistical models can be implemented for connecting molecular subtypes with clinical phenotypes. This approach previously led to successful identification of complex disease endotypes, understanding prognosis and appropriately targeting treatments (9). However, it has also been mentioned that since new patients may often have limited data compared to the patient group used to generate the molecular subtypes, endotyping needs to be based on simpler, easily obtainable data and preferably connected to stable biomarkers. Finally, a significant challenge for connecting multi-omics to complex disease endotypes is the availability of high quality phenotypic data. In case of AD, not all clinically relevant parameters (such as TEWL), are available for omics datasets. It is therefore important to collect as much phenotypic data as possible to correlate with different omics layers.
Omics-wide Integration: Analytical Challenges and Opportunities
Big data has been defined as “large volumes of high velocity, complex, and variable data that require advanced techniques and technologies to enable the capture, storage, distribution, management and analysis of the information” (204, 213). Big data has led to new data warehouses and technologies including cloud-based computing with user-friendly input and output interfaces. Big data from real-time physiologic and environmental data connected to patients omics data can uncover novel pathways and processes related to complex disease etiology in the future (204). However, there are several challenges to integrate “big omics data.” Some of these challenges include problems with the data being scattered in several annotation databases and thereby a lack of a unified portal for data annotation and analytics. In addition, integrating all types of omics data (such the datasets generated from genomic, epigenomic, transcriptomic, proteomic, interactomic, metabolomic studies) are difficult due to non-linearity of the data. Seamless downstream analysis and prediction of actionable insight require multiple, disparate tools and manual interventions. In addition, emerging clinical data sources are typically less structured, since they were designed to serve a different purpose (e.g., clinical care and billing). Current integrative analyses are primarily focused on the combination of omics data from the same source material, such as tumor, human brain or blood samples representing one level of regulation. However, the integration of data from different levels of regulation (i.e., blood and tumor) or different patient samples may also reveal important knowledge about the hierarchy of the human genetic architecture.
High throughput technologies are becoming increasingly efficient and affordable. Moreover, efficient use of publicly available datasets and novel bioinformatic-based tools it can be possible to identify interactive networks of genes, transcripts, proteins and metabolites providing a framework for data integration useful to detect, rank and predict functional variants linked with AD. The rationale is that by integrating different omics data, we can learn more about genotype-phenotype relationships than would not be possible otherwise. Our ability to measure millions of data points for every single omics experiment makes hypothesis generation dependent on advanced computational approaches. Machine learning (ML) techniques are among the most widely used approaches to address this problem (214). ML represents computer algorithms that can learn from existing data and use that knowledge to make predictions from new data such as distinguishing between disease states on the basis of gene expression or methylation profiles of samples. ML algorithms can improve the accuracy of prediction over conventional regression models by capturing complex, non-linear relationships in multi-omics and clinical data. The algorithms used in ML include data mining and cluster approaches of supervised or unsupervised nature (215).
Conclusion and Future Direction
The power of the multi-omics approach in personalized and predictive medicine for complex diseases has recently been emphasized by several authors (205, 216). Despite increased research efforts in recent years, most omics-level data concerns only genomics and transcriptomics in AD. There are some novel proteomic (in serum) and lipidomic (in sweat samples) studies showing great promise toward identifying AD endotypes. Using multi-omics analysis, we showed that previously suspected genes, such as FLG and SERPINB3, were associated with AD among multiple omics types. At the pathway level, macrophage, endothelial cell/ ET-1, Th1/Th2, NFκB, and fibroblast activation pathways were overrepresented in AD from multiple omics level results. Thus, integrated multi-omics data provide new biological insights, as well as confirm previously reported AD-associated genes and pathways. The relative importance of one or more pathways in individual patients might be helpful in establishing endotypes. It should be noted that not all biomarkers for identifying endotypes can be visible by interrogating one single omics layer; thus, more studies are required to unravel multi-layered omics data in AD. Because most of the advanced data integration tools mentioned above are to be used to analyze multidimensional omics data obtained from the same set of samples, future studies should be directed to consistently obtaining multilevel (e.g., genomic, transcriptomic, proteomic) data from AD samples to obtain an integrated omics level picture of the disease and its endotypes. Despite the growing availability of genome-wide data in multiple omics types, so far, publicly available omics databases contain limited data related to AD. To address this limitation, data obtained from all omics-level experiments (e.g., epigenome, lipidome exposome, microbiome) should be deposited in appropriate public data repositories. Improved computational tools are needed to handle big data generated by high-throughput technology along with a patient's personalized exposure profile to achieve the promise of precision medicine in AD.
Author Contributions
DG and TM wrote the manuscript and JB, GK, and MR contributed to write the manuscript.
Funding
This work was supported by the National Institutes of Health (NIH) grant R01HL132344 and Center for Pediatrics Genomics (CpG) Award of the Cincinnati Children's Research Foundation.
Conflict of Interest Statement
The authors declare that the research was conducted in the absence of any commercial or financial relationships that could be construed as a potential conflict of interest.
The reviewer AA and handling Editor declared their shared affiliation at the time of review.
Acknowledgments
We thank Shawna Hottinger for editorial assistance.
References
1. Bos JD, Brenninkmeijer EEA, Schram ME, Middelkamp-Hup MA, Spuls PI, Smitt JHS. Atopic eczema or atopiform dermatitis. Exp Dermatol. (2010) 19:325–31. doi: 10.1111/j.1600-0625.2009.01024.x
2. Gupta J, Johansson E, Bernstein JA, Chakraborty R, Khurana Hershey GK, Rothenberg ME, et al. Resolving the etiology of atopic disorders by using genetic analysis of racial ancestry. J Allergy Clin Immunol. (2016) 138:676–99. doi: 10.1016/j.jaci.2016.02.045
3. Bantz SK, Zhu Z, Zheng T. The Atopic March: progression from atopic dermatitis to allergic rhinitis and asthma. J Clin Cell Immunol. (2014) 5:202. doi: 10.4172/2155-9899.1000202
4. Jensen JM, Scherer A, Wanke C, Brautigam M, Bongiovanni S, Letzkus M, et al. Gene expression is differently affected by pimecrolimus and betamethasone in lesional skin of atopic dermatitis. Allergy (2012) 67:413–23. doi: 10.1111/j.1398-9995.2011.02747.x
5. Jensen JM, Pfeiffer S, Witt M, Brautigam M, Neumann C, Weichenthal M, et al. Different effects of pimecrolimus and betamethasone on the skin barrier in patients with atopic dermatitis. J Allergy Clin Immunol. (2009) 124:R19–28. doi: 10.1016/j.jaci.2009.07.015
6. Beck LA, Thaci D, Hamilton JD, Graham NM, Bieber T, Rocklin R, et al. Dupilumab treatment in adults with moderate-to-severe atopic dermatitis. N Engl J Med. (2014) 371:130–9. doi: 10.1056/NEJMoa1314768
7. Mennini M, Dahdah L, Fiocchi A. Two phase 3 trials of dupilumab versus placebo in atopic dermatitis. N Engl J Med. (2017) 376:1090. doi: 10.1056/NEJMc1700366
8. Ruzicka T, Hanifin JM, Furue M, Pulka G, Mlynarczyk I, Wollenberg A, et al. Anti-interleukin-31 receptor A antibody for atopic dermatitis. N Engl J Med. (2017) 376:826–35. doi: 10.1056/NEJMoa1606490
9. Higdon R, Earl RK, Stanberry L, Hudac CM, Montague E, Stewart E, et al. The promise of multi-omics and clinical data integration to identify and target personalized healthcare approaches in autism spectrum disorders. OMICS (2015) 19:197–208. doi: 10.1089/omi.2015.0020
10. Thijs J, Krastev T, Weidinger S, Buckens CF, de Bruin-Weller M, Bruijnzeel-Koomen C, et al. Biomarkers for atopic dermatitis: a systematic review and meta-analysis. Curr Opin Allergy Clin Immunol. (2015) 15:453–60. doi: 10.1097/ACI.0000000000000198
11. Ardern-Jones MR. Characterisation of atopic dermatitis (AD) endotypes and novel treatment targets: towards a molecular classification. Exp Dermatol. (2016) 27:433–4. doi: 10.1111/exd.13152
12. Thijs JL, Strickland I, Bruijnzeel-Koomen C, Nierkens S, Giovannone B, Csomor E, et al. Moving toward endotypes in atopic dermatitis: identification of patient clusters based on serum biomarker analysis. J Allergy Clin Immunol. (2017) 140:730–7. doi: 10.1016/j.jaci.2017.03.023
13. Novak N, Bieber T, Leung DY. Immune mechanisms leading to atopic dermatitis. J Allergy Clin Immunol. (2003) 112:S128–39. doi: 10.1016/j.jaci.2003.09.032
14. Leung DY, Boguniewicz M, Howell MD, Nomura I, Hamid QA. New insights into atopic dermatitis. J Clin Invest. (2004) 113:651–7. doi: 10.1172/JCI21060
15. Czarnowicki T, Krueger JG, Guttman-Yassky E. Novel concepts of prevention and treatment of atopic dermatitis through barrier and immune manipulations with implications for the atopic march. J Allergy Clin Immunol. (2017) 139:1723–34. doi: 10.1016/j.jaci.2017.04.004
16. Sullivan M, Silverberg NB. Current and emerging concepts in atopic dermatitis pathogenesis. Clin Dermatol. (2017) 35:349–53. doi: 10.1016/j.clindermatol.2017.03.006
17. Irvine AD, McLean WH, Leung DY. Filaggrin mutations associated with skin and allergic diseases. N Engl J Med. (2011) 365:1315–27. doi: 10.1056/NEJMra1011040
18. Brown SJ, Irvine AD. Atopic eczema and the filaggrin story. Semin Cutan Med Surg. (2008) 27:128–37. doi: 10.1016/j.sder.2008.04.001
19. Biagini Myers JM, Wang N, LeMasters GK, Bernstein DI, Epstein TG, Lindsey MA, et al. Genetic and environmental risk factors for childhood eczema development and allergic sensitization in the CCAAPS cohort. J Invest Dermatol. (2010) 130:430–7. doi: 10.1038/jid.2009.300
20. Silverberg NB, Silverberg JI. Inside out or outside in: does atopic dermatitis disrupt barrier function or does disruption of barrier function trigger atopic dermatitis? Cutis (2015) 96:359–61.
21. Chopra R, Vakharia PP, Sacotte R, Patel N, Immaneni S, White T, et al. Relationship between EASI and SCORAD severity assessments for atopic dermatitis. J Allergy Clin Immunol. (2017) 140:1708–10. doi: 10.1016/j.jaci.2017.04.052
22. Bieber T, D'Erme AM, Akdis CA, Traidl-Hoffmann C, Lauener R, Schappi G, et al. Clinical phenotypes and endophenotypes of atopic dermatitis: where are we, and where should we go? J Allergy Clin Immunol. (2017) 139:S58–64. doi: 10.1016/j.jaci.2017.01.008
23. Gupta J, Grube E, Ericksen MB, Stevenson MD, Lucky AW, Sheth AP, et al. Intrinsically defective skin barrier function in children with atopic dermatitis correlates with disease severity. J Allergy Clin Immunol. (2008) 121:725–30.e2. doi: 10.1016/j.jaci.2007.12.1161
24. Suarez-Farinas M, Dhingra N, Gittler J, Shemer A, Cardinale I, de Guzman Strong C, et al. Intrinsic atopic dermatitis shows similar TH2 and higher TH17 immune activation compared with extrinsic atopic dermatitis. J Allergy Clin Immunol. (2013) 132:361–70. doi: 10.1016/j.jaci.2013.04.046
25. Karimkhani C, Silverberg JI, Dellavalle RP. Defining intrinsic vs. extrinsic atopic dermatitis. Dermatol Online J. (2015) 21.
26. Bieber T. Atopic dermatitis 2.0: from the clinical phenotype to the molecular taxonomy and stratified medicine. Allergy (2012) 67:1475–82. doi: 10.1111/all.12049
27. Esaki H, Czarnowicki T, Gonzalez J, Oliva M, Talasila S, Haugh I, et al. Accelerated T-cell activation and differentiation of polar subsets characterizes early atopic dermatitis development. J Allergy Clin Immunol. (2016) 138:1473–7 e5. doi: 10.1016/j.jaci.2016.04.052
28. Noda S, Suarez-Farinas M, Ungar B, Kim SJ, de Guzman Strong C, Xu H, et al. The Asian atopic dermatitis phenotype combines features of atopic dermatitis and psoriasis with increased TH17 polarization. J Allergy Clin Immunol. (2015) 136:1254–64. doi: 10.1016/j.jaci.2015.08.015
29. Leung DY, Guttman-Yassky E. Deciphering the complexities of atopic dermatitis: shifting paradigms in treatment approaches. J Allergy Clin Immunol. (2014) 134:769–79. doi: 10.1016/j.jaci.2014.08.008
30. Simpson EL, Akinlade B, Ardeleanu M. Two phase 3 trials of dupilumab versus placebo in atopic dermatitis. N Engl J Med. (2017) 376:1090–1. doi: 10.1056/NEJMc1700366
31. Simpson EL, Bieber T, Guttman-Yassky E, Beck LA, Blauvelt A, Cork MJ, et al. Two phase 3 trials of dupilumab versus placebo in atopic dermatitis. N Engl J Med. (2016) 375:2335–48. doi: 10.1056/NEJMoa1610020
32. Rung J, Brazma A. Reuse of public genome-wide gene expression data. Nat Rev Genet. (2013) 14:89–99. doi: 10.1038/nrg3394
33. Hindorff LA, Sethupathy P, Junkins HA, Ramos EM, Mehta JP, Collins FS, et al. Potential etiologic and functional implications of genome-wide association loci for human diseases and traits. Proc Natl Acad Sci USA. (2009) 106:9362–7. doi: 10.1073/pnas.0903103106
34. Paternoster L, Standl M, Chen CM, Ramasamy A, Bonnelykke K, Duijts L, et al. Meta-analysis of genome-wide association studies identifies three new risk loci for atopic dermatitis. Nat Genet. (2012) 44:187–92. doi: 10.1038/ng.1017
35. Marenholz I, Zirra M, Fischer DF, Backendorf C, Ziegler A, Mischke D. Identification of human epidermal differentiation complex (EDC)-encoded genes by subtractive hybridization of entire YACs to a gridded keratinocyte cDNA library. Genome Res. (2001) 11:341–55. doi: 10.1101/gr.114801
36. Esparza-Gordillo J, Weidinger S, Fölster-Holst R, Bauerfeind A, Ruschendorf F, Patone G, et al. A common variant on chromosome 11q13 is associated with atopic dermatitis. Nat Genet. (2009) 41:596–601. doi: 10.1038/ng.347
37. Sun LD, Xiao FL, Li Y, Zhou WM, Tang HY, Tang XF, et al. Genome-wide association study identifies two new susceptibility loci for atopic dermatitis in the Chinese Han population. Nat Genet. (2011) 43:690–4. doi: 10.1038/ng.851
38. Hirota T, Takahashi A, Kubo M, Tsunoda T, Tomita K, Sakashita M, et al. Genome-wide association study identifies eight new susceptibility loci for atopic dermatitis in the Japanese population. Nat Genet. (2012) 44:1222–6. doi: 10.1038/ng.2438
39. Weidinger S, Willis-Owen SA, Kamatani Y, Baurecht H, Morar N, Liang L, et al. A genome-wide association study of atopic dermatitis identifies loci with overlapping effects on asthma. Hum Mol Genet. (2013) 22:4841–56. doi: 10.1093/hmg/ddt317
40. Paternoster L, Standl M, Waage J, Baurecht H, Hotze M, Strachan DP, et al. Multi-ancestry genome-wide association study of 21,000 cases and 95,000 controls identifies new risk loci for atopic dermatitis. Nat Genet. (2015) 47:1449–56. doi: 10.1038/ng.3424
41. Kim KW, Myers RA, Lee JH, Igartua C, Lee KE, Kim YH, et al. Genome-wide association study of recalcitrant atopic dermatitis in Korean children. J Allergy Clin Immunol. (2015) 136:678.e4-84.e4. doi: 10.1016/j.jaci.2015.03.030
42. Schaarschmidt H, Ellinghaus D, Rodríguez E, Kretschmer A, Baurecht H, Lipinski S, et al. A genome-wide association study reveals 2 new susceptibility loci for atopic dermatitis. J Allergy Clin Immunol. (2015) 136:802–6. doi: 10.1016/j.jaci.2015.01.047
43. Paternoster L, Standl M, Chen CM, Ramasamy A, Bonnelykke K, Duijts L, et al. Meta-analysis of genome-wide association studies identifies three new risk loci for atopic dermatitis. Nat Genet. (2011) 44:187–92. doi: 10.1038/ng.1017
44. Kang Z, Li Q, Fu P, Yan S, Guan M, Xu J, et al. Correlation of KIF3A and OVOL1, but not ACTL9, with atopic dermatitis in Chinese pediatric patients. Gene (2015) 571:249–51. doi: 10.1016/j.gene.2015.06.068
45. Paternoster L, Standl M, Waage J, Baurecht H, Hotze M, Strachan DP, et al. Multi-ancestry genome-wide association study of 21,000 cases and 95,000 controls identifies new risk loci for atopic dermatitis. Nat Genet. (2015) 47:1449–56. doi: 10.1038/ng.3424
46. Manousaki D, Paternoster L, Standl M, Moffatt MF, Farrall M, Bouzigon E, et al. Vitamin D levels and susceptibility to asthma, elevated immunoglobulin E levels, and atopic dermatitis: a Mendelian randomization study. PLoS Med. (2017) 14:e1002294. doi: 10.1371/journal.pmed.1002294
47. Esparza-Gordillo J, Schaarschmidt H, Liang L, Cookson W, Bauerfeind A, Lee-Kirsch MA, et al. A functional IL-6 receptor (IL6R) variant is a risk factor for persistent atopic dermatitis. J Allergy Clin Immunol. (2013) 132:371–7. doi: 10.1016/j.jaci.2013.01.057
48. Ferreira MA, Vonk JM, Baurecht H, Marenholz I, Tian C, Hoffman JD, et al. Shared genetic origin of asthma, hay fever and eczema elucidates allergic disease biology. Nat Genet. (2017) 49:1752–7. doi: 10.1038/ng.3985
49. Carithers LJ, Moore HM. The Genotype-Tissue Expression (GTEx) Project. Biopreserv Biobank (2015) 13:307–8. doi: 10.1089/bio.2015.29031.hmm
50. Du J, Yuan Z, Ma Z, Song J, Xie X, Chen Y. KEGG-PATH: Kyoto encyclopedia of genes and genomes-based pathway analysis using a path analysis model. Mol Biosyst. (2014) 10:2441–7. doi: 10.1039/C4MB00287C
51. Kanehisa M, Goto S. KEGG: kyoto encyclopedia of genes and genomes. Nucleic Acids Res. (2000) 28:27–30. doi: 10.1093/nar/28.1.27
52. Croft D, O'Kelly G, Wu G, Haw R, Gillespie M, Matthews L, et al. Reactome: a database of reactions, pathways and biological processes. Nucleic Acids Res. (2011) 39:D691–7. doi: 10.1093/nar/gkq1018
53. Palmer CN, Irvine AD, Terron-Kwiatkowski A, Zhao Y, Liao H, Lee SP, et al. Common loss-of-function variants of the epidermal barrier protein filaggrin are a major predisposing factor for atopic dermatitis. Nat Genet. (2006) 38:441–6. doi: 10.1038/ng1767
54. Howell MD, Kim BE, Gao P, Grant AV, Boguniewicz M, DeBenedetto A, et al. Cytokine modulation of atopic dermatitis filaggrin skin expression. J Allergy Clin Immunol. (2009) 124:R7–12. doi: 10.1016/j.jaci.2009.07.012
55. Irvine AD. Fleshing out filaggrin phenotypes. J Invest Dermatol. (2007) 127:504–7. doi: 10.1038/sj.jid.5700695
56. Fliegauf M, Benzing T, Omran H. When cilia go bad: cilia defects and ciliopathies. Nat Rev Mol Cell Biol. (2007) 8:880–93. doi: 10.1038/nrm2278
57. Lepre T, Cascella R, Ragazzo M, Galli E, Novelli G, Giardina E. Association of KIF3A, but not OVOL1 and ACTL9, with atopic eczema in Italian patients. Br J Dermatol. (2013) 168:1106–8. doi: 10.1111/bjd.12178
58. Johansson E, Biagini Myers JM, Martin LJ, He H, Pilipenko V, Mersha T, et al. KIF3A genetic variation is associated with pediatric asthma in the presence of eczema independent of allergic rhinitis. J Allergy Clin Immunol. (2017) 140:595–8.e5. doi: 10.1016/j.jaci.2017.02.003
59. Kovacic MB, Myers JM, Wang N, Martin LJ, Lindsey M, Ericksen MB, et al. Identification of KIF3A as a novel candidate gene for childhood asthma using RNA expression and population allelic frequencies differences. PLoS ONE (2011) 6:e23714. doi: 10.1371/journal.pone.0023714
60. Potaczek DP, Harb H, Michel S, Alhamwe BA, Renz H, Tost J. Epigenetics and allergy: from basic mechanisms to clinical applications. Epigenomics (2017) 9:539–71. doi: 10.2217/epi-2016-0162
61. Bin L, Leung DY. Genetic and epigenetic studies of atopic dermatitis. Allergy Asthma Clin Immunol. (2016) 12:52. doi: 10.1186/s13223-016-0158-5
62. Rodriguez E, Baurecht H, Wahn AF, Kretschmer A, Hotze M, Zeilinger S, et al. An integrated epigenetic and transcriptomic analysis reveals distinct tissue-specific patterns of DNA methylation associated with atopic dermatitis. J Invest Dermatol. (2014) 134:1873–83. doi: 10.1038/jid.2014.87
63. Liang Y, Wang P, Zhao M, Liang G, Yin H, Zhang G, et al. Demethylation of the FCER1G promoter leads to FcepsilonRI overexpression on monocytes of patients with atopic dermatitis. Allergy (2012) 67:424–30. doi: 10.1111/j.1398-9995.2011.02760.x
64. Ziyab AH, Karmaus W, Holloway JW, Zhang H, Ewart S, Arshad SH. DNA methylation of the filaggrin gene adds to the risk of eczema associated with loss-of-function variants. J Eur Acad Dermatol Venereol. (2013) 27:e420–3. doi: 10.1111/jdv.12000
65. Tan HT, Ellis JA, Koplin JJ, Martino D, Dang TD, Suaini N, et al. Methylation of the filaggrin gene promoter does not affect gene expression and allergy. Pediatr Allergy Immunol. (2014) 25:608–10. doi: 10.1111/pai.12245
66. Barrett T, Troup DB, Wilhite SE, Ledoux P, Evangelista C, Kim IF, et al. NCBI GEO: archive for functional genomics data sets−10 years on. Nucleic Acids Res. (2011) 39:D1005–10. doi: 10.1093/nar/gkq1184
67. Kolesnikov N, Hastings E, Keays M, Melnichuk O, Tang YA, Williams E, et al. ArrayExpress update–simplifying data submissions. Nucleic Acids Res. (2015) 43:D1113–6. doi: 10.1093/nar/gku1057
68. Rustici G, Kapushesky M, Kolesnikov N, Parkinson H, Sarkans U, Brazma A. Data storage and analysis in ArrayExpress and Expression Profiler. Curr Protoc Bioinformatics (2008) Chapter 7:Unit 7 13. doi: 10.1002/0471250953.bi0713s23
69. Ghosh D, Ding L, Sivaprasad U, Geh E, Biagini Myers J, Bernstein JA, et al. Multiple transcriptome data analysis reveals biologically relevant atopic dermatitis signature genes and pathways. PLoS ONE (2015) 10:e0144316. doi: 10.1371/journal.pone.0144316
70. Ewald DA, Malajian D, Krueger JG, Workman CT, Wang T, Tian S, et al. Meta-analysis derived atopic dermatitis (MADAD) transcriptome defines a robust AD signature highlighting the involvement of atherosclerosis and lipid metabolism pathways. BMC Med Genomics (2015) 8:60. doi: 10.1186/s12920-015-0133-x
71. Riffle M, Eng JK. Proteomics data repositories. Proteomics (2009) 9:4653–63. doi: 10.1002/pmic.200900216
72. Perez-Riverol Y, Alpi E, Wang R, Hermjakob H, Vizcaino JA. Making proteomics data accessible and reusable: current state of proteomics databases and repositories. Proteomics (2015) 15:930–49. doi: 10.1002/pmic.201400302
73. Lindskog C. The potential clinical impact of the tissue-based map of the human proteome. Expert Rev Proteomics (2015) 12:213–5. doi: 10.1586/14789450.2015.1040771
74. Uhlen M, Fagerberg L, Hallstrom BM, Lindskog C, Oksvold P, Mardinoglu A, et al. Proteomics. Tissue-based map of the human proteome. Science (2015) 347:1260419. doi: 10.1126/science.1260419
75. Kim MS, Pinto SM, Getnet D, Nirujogi RS, Manda SS, Chaerkady R, et al. A draft map of the human proteome. Nature (2014) 509:575–81. doi: 10.1038/nature13302
76. Aebersold R, Bader GD, Edwards AM, van Eyk JE, Kussmann M, Qin J, et al. The biology/disease-driven human proteome project (B/D-HPP): enabling protein research for the life sciences community. J Proteome Res. (2013) 12:23–7. doi: 10.1021/pr301151m
77. Omenn GS. THE HUPO Human Plasma Proteome Project. Proteomics Clin Appl. (2007) 1:769–79. doi: 10.1002/prca.200700369
78. Omenn GS, Baker MS, Aebersold R. Recent Workshops of the HUPO Human Plasma Proteome Project (HPPP): a bridge with the HUPO CardioVascular Initiative and the emergence of SRM targeted proteomics. Proteomics (2011) 11:3439–43. doi: 10.1002/pmic.201100382
79. Berhane BT, Zong C, Liem DA, Huang A, Le S, Edmondson RD, et al. Cardiovascular-related proteins identified in human plasma by the HUPO Plasma Proteome Project pilot phase. Proteomics (2005) 5:3520–30. doi: 10.1002/pmic.200401308
80. Vizcaino JA, Cote RG, Csordas A, Dianes JA, Fabregat A, Foster JM, et al. The PRoteomics IDEntifications (PRIDE) database and associated tools: status in 2013. Nucleic Acids Res. (2013) 41:D1063–9. doi: 10.1093/nar/gks1262
81. Jones P, Martens L. Using the PRIDE proteomics identifications database for knowledge discovery and data analysis. Methods Mol Biol. (2010) 604:297–307. doi: 10.1007/978-1-60761-444-9_20
82. Martens L, Hermjakob H, Jones P, Adamski M, Taylor C, States D, et al. PRIDE: the proteomics identifications database. Proteomics (2005) 5:3537–45. doi: 10.1002/pmic.200401303
83. Fenyo D, Eriksson J, Beavis R. Mass spectrometric protein identification using the global proteome machine. Methods Mol Biol. (2010) 673:189–202. doi: 10.1007/978-1-60761-842-3_11
84. Beavis RC. Using the global proteome machine for protein identification. Methods Mol Biol. (2006) 328:217–28. doi: 10.1385/1-59745-026-X:217
85. Broccardo CJ, Mahaffey SB, Strand M, Reisdorph NA, Leung DY. Peeling off the layers: skin taping and a novel proteomics approach to study atopic dermatitis. J Allergy Clin Immunol. (2009) 124:1113–5.e1–11. doi: 10.1016/j.jaci.2009.07.057
86. Broccardo CJ, Mahaffey S, Schwarz J, Wruck L, David G, Schlievert PM, et al. Comparative proteomic profiling of patients with atopic dermatitis based on history of eczema herpeticum infection and Staphylococcus aureus colonization. J Allergy Clin Immunol (2011) 127:186–93, 93 e1–11. doi: 10.1016/j.jaci.2010.10.033
87. Sakabe J, Kamiya K, Yamaguchi H, Ikeya S, Suzuki T, Aoshima M, et al. Proteome analysis of stratum corneum from atopic dermatitis patients by hybrid quadrupole-orbitrap mass spectrometer. J Allergy Clin Immunol. (2014) 134:957–60.e8. doi: 10.1016/j.jaci.2014.07.054
88. Sääf AM, Tengvall-Linder M, Chang HY, Adler AS, Wahlgren CF, Scheynius A, et al. Global expression profiling in atopic eczema reveals reciprocal expression of inflammatory and lipid genes. PLoS ONE (2008) 3:e4017. doi: 10.1371/journal.pone.0004017
89. Zeeuwen PLJM, de Jongh GJ, Rodijk-Olthuis D, Kamsteeg M, Verhoosel RM, van Rossum MM, et al. Genetically programmed differences in epidermal host defense between psoriasis and atopic dermatitis patients. PLoS ONE (2008) 3:e2301. doi: 10.1371/journal.pone.0002301
90. Gittler JK, Shemer A, Suárez-Fariñas M, Fuentes-Duculan J, Gulewicz KJ, Wang CQ, et al. Progressive activation of T(H)2/T(H)22 cytokines and selective epidermal proteins characterizes acute and chronic atopic dermatitis. J Allergy Clin Immunol. (2012) 130:1344–54. doi: 10.1016/j.jaci.2012.07.012
91. Suárez-Fariñas M, Tintle SJ, Shemer A, Chiricozzi A, Nograles K, Cardinale I, et al. Nonlesional atopic dermatitis skin is characterized by broad terminal differentiation defects and variable immune abnormalities. J Allergy Clin Immunol. (2011) 127:954.e1-4-64.e1-4. doi: 10.1016/j.jaci.2010.12.1124
92. Goodacre R, Vaidyanathan S, Dunn WB, Harrigan GG, Kell DB. Metabolomics by numbers: acquiring and understanding global metabolite data. Trends Biotechnol. (2004) 22:245–52. doi: 10.1016/j.tibtech.2004.03.007
93. Assfalg M, Bertini I, Colangiuli D, Luchinat C, Schafer H, Schutz B, et al. Evidence of different metabolic phenotypes in humans. Proc Natl Acad Sci USA. (2008) 105:1420–4. doi: 10.1073/pnas.0705685105
94. Carraro S, Rezzi S, Reniero F, Heberger K, Giordano G, Zanconato S, et al. Metabolomics applied to exhaled breath condensate in childhood asthma. Am J Respir Crit Care Med. (2007) 175:986–90. doi: 10.1164/rccm.200606-769OC
95. Assfalg M, Bortoletti E, D'Onofrio M, Pigozzi R, Molinari H, Boner AL, et al. An exploratory (1) H-nuclear magnetic resonance metabolomics study reveals altered urine spectral profiles in infants with atopic dermatitis. Br J Dermatol. (2012) 166:1123–5. doi: 10.1111/j.1365-2133.2011.10711.x
96. Wishart DS, Jewison T, Guo AC, Wilson M, Knox C, Liu Y, et al. HMDB 3.0–The Human Metabolome Database in 2013. Nucleic Acids Res. (2013) 41:D801–7. doi: 10.1093/nar/gks1065
97. Schellenberger J, Park JO, Conrad TM, Palsson BO. BiGG: a Biochemical Genetic and Genomic knowledgebase of large scale metabolic reconstructions. BMC Bioinformatics (2010) 11:213. doi: 10.1186/1471-2105-11-213
98. Scholz M, Fiehn O. SetupX–a public study design database for metabolomic projects. Pac Symp Biocomput. (2007) 169–80. doi: 10.1142/9789812772435_0017
99. Wishart DS, Knox C, Guo AC, Eisner R, Young N, Gautam B, et al. HMDB: a knowledgebase for the human metabolome. Nucleic Acids Res. (2009) 37:D603–10. doi: 10.1093/nar/gkn810
100. Wishart DS, Tzur D, Knox C, Eisner R, Guo AC, Young N, et al. HMDB: the Human Metabolome Database. Nucleic Acids Res. (2007) 35:D521–6. doi: 10.1093/nar/gkl923
101. Ishikawa J, Narita H, Kondo N, Hotta M, Takagi Y, Masukawa Y, et al. Changes in the ceramide profile of atopic dermatitis patients. J Invest Dermatol. (2010) 130:2511–4. doi: 10.1038/jid.2010.161
102. Masukawa Y. Atopic dermatitis (AD) and lipids. In: Pappas A, editor. Lipids and Skin Health. Cham: Springer (2014). p. 343–56.
103. Janssens M, van Smeden J, Gooris GS, Bras W, Portale G, Caspers PJ, et al. Increase in short-chain ceramides correlates with an altered lipid organization and decreased barrier function in atopic eczema patients. J Lipid Res. (2012) 53:2755–66. doi: 10.1194/jlr.P030338
104. Agrawal K, Hassoun LA, Foolad N, Pedersen TL, Sivamani RK, Newman JW. Sweat lipid mediator profiling: a noninvasive approach for cutaneous research. J Lipid Res. (2017) 58:188–95. doi: 10.1194/jlr.M071738
105. Fahy E, Sud M, Cotter D, Subramaniam S. LIPID MAPS online tools for lipid research. Nucleic Acids Res. (2007) 35:W606–12. doi: 10.1093/nar/gkm324
106. Cotter D, Maer A, Guda C, Saunders B, Subramaniam S. LMPD: LIPID MAPS proteome database. Nucleic Acids Res. (2006) 34:D507–10. doi: 10.1093/nar/gkj122
107. Sud M, Fahy E, Cotter D, Dennis EA, Subramaniam S. LIPID MAPS-Nature lipidomics gateway: an online resource for students and educators interested in lipids. J Chem Educ. (2012) 89:291–2. doi: 10.1021/ed200088u
108. Martin-Lorenzo M, Balluff B, Maroto AS, Carreira RJ, van Zeijl RJ, Gonzalez-Calero L, et al. Lipid and protein maps defining arterial layers in atherosclerotic aorta. Data Brief (2015) 4:328–31. doi: 10.1016/j.dib.2015.06.005
109. Li S, Ganguli-Indra G, Indra AK. Lipidomic analysis of epidermal lipids: a tool to predict progression of inflammatory skin disease in humans. Expert Rev Proteomics (2016) 13:451–6. doi: 10.1080/14789450.2016.1177462
110. van Smeden J, Bouwstra JA. Stratum corneum lipids: their role for the skin barrier function in healthy subjects and atopic dermatitis patients. Curr Probl Dermatol. (2016) 49:8–26. doi: 10.1159/000441540
111. Indra AK, Li S, Villarreal M, Babineau DC, Philpot C, David G, et al. Skin lipid composition varies based on clinical subphenotypes in adult european american atopic dermatitis subjects. J Allergy Clin Immunol. (2016) 137:AB142. doi: 10.1016/j.jaci.2015.12.598
112. Berdyshev E, Goleva E, Bronova I, Rios C, Dyjack N, Wesolowska-Andersen A, et al. Lipid abnormalities associated with skin lesions in atopic dermatitis. J Allergy Clin Immunol. (2017) 139:AB87. doi: 10.1016/j.jaci.2016.12.236
113. Kong HH, Oh J, Deming C, Conlan S, Grice EA, Beatson MA, et al. Temporal shifts in the skin microbiome associated with disease flares and treatment in children with atopic dermatitis. Genome Res. (2012) 22:850–9. doi: 10.1101/gr.131029.111
114. Wollina U. Microbiome in atopic dermatitis. Clin Cosmet Investig Dermatol. (2017) 10:51–6. doi: 10.2147/CCID.S130013
115. Chng KR, Tay AS, Li C, Ng AH, Wang J, Suri BK, et al. Whole metagenome profiling reveals skin microbiome-dependent susceptibility to atopic dermatitis flare. Nat Microbiol. (2016) 1:16106. doi: 10.1038/nmicrobiol.2016.106
116. Li S, Villarreal M, Stewart S, Choi J, Indra G, Babineau DC, et al. Altered composition of epidermal lipids correlates with Staphylococcus aureus colonization status in Atopic Dermatitis. Br J Dermatol. (2017) 177:e125–7. doi: 10.1111/bjd.15409
117. Williams MR, Gallo RL. The role of the skin microbiome in atopic dermatitis. Curr Allergy Asthma Rep. (2015) 15:65. doi: 10.1007/s11882-015-0567-4
118. Dybboe R, Bandier J, Skov L, Engstrand L, Johansen JD. The role of the skin microbiome in atopic dermatitis: a systematic review. Br J Dermatol. (2017). 177:1272–8. doi: 10.1111/bjd.15390
119. Nakatsuji T, Chen TH, Narala S, Chun KA, Two AM, Yun T, et al. Antimicrobials from human skin commensal bacteria protect against Staphylococcus aureus and are deficient in atopic dermatitis. Sci Transl Med. (2017) 9:eaah4680. doi: 10.1126/scitranslmed.aah4680
120. Gonzalez ME, Schaffer JV, Orlow SJ, Gao Z, Li H, Alekseyenko AV, et al. Cutaneous microbiome effects of fluticasone propionate cream and adjunctive bleach baths in childhood atopic dermatitis. J Am Acad Dermatol. (2016) 75:481–93.e8. doi: 10.1016/j.jaad.2016.04.066
121. Jovel J, Patterson J, Wang W, Hotte N, O'Keefe S, Mitchel T, et al. Characterization of the gut microbiome using 16S or shotgun metagenomics. Front Microbiol. (2016) 7:459. doi: 10.3389/fmicb.2016.00459
122. Truong DT, Franzosa EA, Tickle TL, Scholz M, Weingart G, Pasolli E, et al. MetaPhlAn2 for enhanced metagenomic taxonomic profiling. Nat Methods (2015) 12:902–3. doi: 10.1038/nmeth.3589
123. Kantor R, Silverberg JI. Environmental risk factors and their role in the management of atopic dermatitis. Expert Rev Clin Immunol. (2017) 13:15–26. doi: 10.1080/1744666X.2016.1212660
124. Timm S, Schlunssen V, Olsen J, Ramlau-Hansen CH. Prenatal antibiotics and atopic dermatitis among 18-month-old children in the Danish National Birth Cohort. Clin Exp Allergy (2017) 47:929–36. doi: 10.1111/cea.12916
125. McKeever TM, Lewis SA, Smith C, Hubbard R. The importance of prenatal exposures on the development of allergic disease: a birth cohort study using the West Midlands General Practice Database. Am J Respir Crit Care Med. (2002) 166:827–32. doi: 10.1164/rccm.200202-158OC
126. Sariachvili M, Droste J, Dom S, Wieringa M, Vellinga A, Hagendorens M, et al. Is breast feeding a risk factor for eczema during the first year of life? Pediatr Allergy Immunol. (2007) 18:410–7. doi: 10.1111/j.1399-3038.2007.00543.x
127. Chang HY, Suh DI, Yang SI, Kang MJ, Lee SY, Lee E, et al. Prenatal maternal distress affects atopic dermatitis in offspring mediated by oxidative stress. J Allergy Clin Immunol. (2016) 138:468–75.e5. doi: 10.1016/j.jaci.2016.01.020
128. Huang CC, Wen HJ, Chen PC, Chiang TL, Lin SJ, Guo YL. Prenatal air pollutant exposure and occurrence of atopic dermatitis. Br J Dermatol. (2015) 173:981–8. doi: 10.1111/bjd.14039
129. Ochiai S, Shimojo N, Yuka I, Watanabe M, Matsuno Y, Suzuki S, et al. A pilot study for foetal exposure to multiple persistent organic pollutants and the development of infant atopic dermatitis in modern Japanese society. Chemosphere (2014) 94:48–52. doi: 10.1016/j.chemosphere.2013.09.009
130. Wang IJ, Chen SL, Lu TP, Chuang EY, Chen PC. Prenatal smoke exposure, DNA methylation, and childhood atopic dermatitis. Clin Exp Allergy (2013) 43:535–43. doi: 10.1111/cea.12108
131. Wang IJ, Guo YL, Lin TJ, Chen PC, Wu YN. GSTM1, GSTP1, prenatal smoke exposure, and atopic dermatitis. Ann Allergy Asthma Immunol. (2010) 105:124–9. doi: 10.1016/j.anai.2010.04.017
132. Herberth G, Pierzchalski A, Feltens R, Bauer M, Roder S, Olek S, et al. Prenatal phthalate exposure associates with low regulatory T-cell numbers and atopic dermatitis in early childhood: results from the LINA mother-child study. J Allergy Clin Immunol. (2017) 139:1376–9.e8. doi: 10.1016/j.jaci.2016.09.034
133. Kim JH, Jeong KS, Ha EH, Park H, Ha M, Hong YC, et al. Association between prenatal exposure to cadmium and atopic dermatitis in infancy. J Korean Med Sci. (2013) 28:516–21. doi: 10.3346/jkms.2013.28.4.516
134. Hagendorens MM, Ebo DG, Bridts CH, Van de Water L, De Clerck LS, Stevens WJ. Prenatal exposure to house dust mite allergen (Der p 1), cord blood T cell phenotype and cytokine production and atopic dermatitis during the first year of life. Pediatr Allergy Immunol. (2004) 15:308–15. doi: 10.1111/j.1399-3038.2004.00169.x
135. Roduit C, Wohlgensinger J, Frei R, Bitter S, Bieli C, Loeliger S, et al. Prenatal animal contact and gene expression of innate immunity receptors at birth are associated with atopic dermatitis. J Allergy Clin Immunol. (2011) 127:179–85, 185.e1. doi: 10.1016/j.jaci.2010.10.010
136. Engebretsen KA, Bager P, Wohlfahrt J, Skov L, Zachariae C, Nybo Andersen AM, et al. Prevalence of atopic dermatitis in infants by domestic water hardness and season of birth: Cohort study. J Allergy Clin Immunol. (2017) 139:1568–74.e1. doi: 10.1016/j.jaci.2016.11.021
137. Perkin MR, Craven J, Logan K, Strachan D, Marrs T, Radulovic S, et al. Association between domestic water hardness, chlorine, and atopic dermatitis risk in early life: A population-based cross-sectional study. J Allergy Clin Immunol. (2016) 138:509–16. doi: 10.1016/j.jaci.2016.03.031
138. Miyake Y, Yokoyama T, Yura A, Iki M, Shimizu T. Ecological association of water hardness with prevalence of childhood atopic dermatitis in a Japanese urban area. Environ Res. (2004) 94:33–7. doi: 10.1016/S0013-9351(03)00068-9
139. Kim YM, Kim J, Han Y, Jeon BH, Cheong HK, Ahn K. Short-term effects of weather and air pollution on atopic dermatitis symptoms in children: A panel study in Korea. PLoS ONE (2017) 12:e0175229. doi: 10.1371/journal.pone.0175229
140. Tang KT, Ku KC, Chen DY, Lin CH, Tsuang BJ, Chen YH. Adult atopic dermatitis and exposure to air pollutants-a nationwide population-based study. Ann Allergy Asthma Immunol. (2017) 118:351–5. doi: 10.1016/j.anai.2016.12.005
141. Ahn K. The role of air pollutants in atopic dermatitis. J Allergy Clin Immunol. (2014) 134:993–9; discussion: 1000. doi: 10.1016/j.jaci.2014.09.023
142. Werfel T, Heratizadeh A, Niebuhr M, Kapp A, Roesner LM, Karch A, et al. Exacerbation of atopic dermatitis on grass pollen exposure in an environmental challenge chamber. J Allergy Clin Immunol. (2015) 136:96–103.e9. doi: 10.1016/j.jaci.2015.04.015
143. Yu HS, Kang MJ, Kwon JW, Lee SY, Lee E, Yang SI, et al. Claudin-1 polymorphism modifies the effect of mold exposure on the development of atopic dermatitis and production of IgE. J Allergy Clin Immunol. (2015) 135:827–30.e5. doi: 10.1016/j.jaci.2014.10.040
144. Kim HJ, Lee E, Lee SH, Kang MJ, Hong SJ. Mold elicits atopic dermatitis by reactive oxygen species: Epidemiology and mechanism studies. Clin Immunol. (2015) 161:384–90. doi: 10.1016/j.clim.2015.07.007
145. Rather IA, Bajpai VK, Kumar S, Lim J, Paek WK, Park YH. Probiotics and atopic dermatitis: an overview. Front Microbiol. (2016) 7:507. doi: 10.3389/fmicb.2016.00507
146. Simpson MR, Brede G, Johansen J, Johnsen R, Storro O, Saetrom P, et al. Human breast milk miRNA, maternal probiotic supplementation and atopic dermatitis in offspring. PLoS ONE (2015) 10:e0143496. doi: 10.1371/journal.pone.0143496
147. Weston S, Halbert A, Richmond P, Prescott SL. Effects of probiotics on atopic dermatitis: a randomised controlled trial. Arch Dis Child (2005) 90:892–7. doi: 10.1136/adc.2004.060673
148. Pacheco-Gonzalez RM, Garcia-Marcos PW, Garcia-Marcos L. Vitamin D and atopic dermatitis. Mini Rev Med Chem. (2015) 15:927–34. doi: 10.2174/1389557515666150519110209
149. Borzutzky A, Grob F, Camargo CA Jr, Martinez-Aguayo A. Vitamin D deficiency rickets in an adolescent with severe atopic dermatitis. Pediatrics (2014) 133:e451–4. doi: 10.1542/peds.2013-1114
150. Vahavihu K, Ylianttila L, Salmelin R, Lamberg-Allardt C, Viljakainen H, Tuohimaa P, et al. Heliotherapy improves vitamin D balance and atopic dermatitis. Br J Dermatol. (2008) 158:1323–8. doi: 10.1111/j.1365-2133.2008.08518.x
151. Neveu V, Moussy A, Rouaix H, Wedekind R, Pon A, Knox C, et al. Exposome-Explorer: a manually-curated database on biomarkers of exposure to dietary and environmental factors. Nucleic Acids Res. (2017) 45:D979–84. doi: 10.1093/nar/gkw980
152. Martel BC, Litman T, Hald A, Norsgaard H, Lovato P, Dyring-Andersen B, et al. Distinct molecular signatures of mild extrinsic and intrinsic atopic dermatitis. Exp Dermatol. (2016) 25:453–9. doi: 10.1111/exd.12967
153. Christophers E, Henseler T. Contrasting disease patterns in psoriasis and atopic dermatitis. Arch Dermatol Res. (1987) 279(Suppl):S48–51. doi: 10.1007/BF00585919
154. McLean WH. The allergy gene: how a mutation in a skin protein revealed a link between eczema and asthma. F1000 Med Rep. (2011) 3:2. doi: 10.3410/M3-2
155. Cole C, Kroboth K, Schurch NJ, Sandilands A, Sherstnev A, O'Regan GM, et al. Filaggrin-stratified transcriptomic analysis of pediatric skin identifies mechanistic pathways in patients with atopic dermatitis. J Allergy Clin Immunol. (2014) 134:82–91. doi: 10.1016/j.jaci.2014.04.021
156. Carson CG, Rasmussen MA, Thyssen JP, Menne T, Bisgaard H. Clinical presentation of atopic dermatitis by filaggrin gene mutation status during the first 7 years of life in a prospective cohort study. PLoS ONE (2012) 7:e48678. doi: 10.1371/journal.pone.0048678
157. Febbo PG, Mulligan MG, Slonina DA, Stegmaier K, Di Vizio D, Martinez PR, et al. Literature Lab: a method of automated literature interrogation to infer biology from microarray analysis. BMC Genomics (2007) 8:461. doi: 10.1186/1471-2164-8-461
158. Szklarczyk D, Franceschini A, Kuhn M, Simonovic M, Roth A, Minguez P, et al. The STRING database in 2011: functional interaction networks of proteins, globally integrated and scored. Nucleic Acids Res. (2011) 39:D561–8. doi: 10.1093/nar/gkq973
159. Watanabe K, Taskesen E, van Bochoven A, Posthuma D. Functional mapping and annotation of genetic associations with FUMA. Nat Commun. (2017) 8:1826. doi: 10.1038/s41467-017-01261-5
160. Presland RB, Dale BA. Epithelial structural proteins of the skin and oral cavity: function in health and disease. Crit Rev Oral Biol Med. (2000) 11:383–408. doi: 10.1177/10454411000110040101
161. Seibold MA, Goleva E, Dyjack N, Eui Kim B, Richers BN, Hall CF, et al. Minimally invasive skin tape strip RNA-Seq identifies atopic dermatitis disease endotype. J Allergy Clin Immunol. (2017) 139:AB273. doi: 10.1016/j.jaci.2016.12.878
162. Dajee M, Muchamuel T, Schryver B, Oo A, Alleman-Sposeto J, De Vry CG, et al. Blockade of experimental atopic dermatitis via topical NF-kappaB decoy oligonucleotide. J Invest Dermatol. (2006) 126:1792–803. doi: 10.1038/sj.jid.5700307
163. Nakamura H, Aoki M, Tamai K, Oishi M, Ogihara T, Kaneda Y, et al. Prevention and regression of atopic dermatitis by ointment containing NF-kB decoy oligodeoxynucleotides in NC/Nga atopic mouse model. Gene Ther. (2002) 9:1221–9. doi: 10.1038/sj.gt.3301724
164. Tanaka A, Muto S, Jung K, Itai A, Matsuda H. Topical application with a new NF-kappaB inhibitor improves atopic dermatitis in NC/NgaTnd mice. J Invest Dermatol. (2007) 127:855–63. doi: 10.1038/sj.jid.5700603
165. Berroth A, Kuhnl J, Kurschat N, Schwarz A, Stab F, Schwarz T, et al. Role of fibroblasts in the pathogenesis of atopic dermatitis. J Allergy Clin Immunol. (2013) 131:1547–54. doi: 10.1016/j.jaci.2013.02.029
166. Pastore S, Fanales-Belasio E, Albanesi C, Chinni LM, Giannetti A, Girolomoni G. Granulocyte macrophage colony-stimulating factor is overproduced by keratinocytes in atopic dermatitis. Implications for sustained dendritic cell activation in the skin. J Clin Invest. (1997) 99:3009–17. doi: 10.1172/JCI119496
167. Kasraie S, Werfel T. Role of macrophages in the pathogenesis of atopic dermatitis. Mediators Inflamm. (2013) 2013:942375. doi: 10.1155/2013/942375
168. Furue M, Yamamura K, Kido-Nakahara M, Nakahara T, Fukui Y. Emerging role of interleukin-31 and interleukin-31 receptor in pruritus in atopic dermatitis. Allergy (2018) 73:29–36. doi: 10.1111/all.13239
169. Holgate ST. The epithelium takes centre stage in asthma and atopic dermatitis. Trends Immunol. (2007) 28:248–51. doi: 10.1016/j.it.2007.04.007
170. Moran TP, Vickery BP. The epithelial cell-derived atopic dermatitis cytokine TSLP activates neurons to induce itch. Pediatrics (2014) 134(Suppl. 3):S160–1. doi: 10.1542/peds.2014-1817VV
171. Sayedyahossein S, Xu SX, Rudkouskaya A, McGavin MJ, McCormick JK, Dagnino L. Staphylococcus aureus keratinocyte invasion is mediated by integrin-linked kinase and Rac1. FASEB J. (2015) 29:711–23. doi: 10.1096/fj.14-262774
172. Yoshihara N, Ueno T, Takagi A, Oliva Trejo JA, Haruna K, Suga Y, et al. The significant role of autophagy in the granular layer in normal skin differentiation and hair growth. Arch Dermatol Res. (2015) 307:159–69. doi: 10.1007/s00403-014-1508-0
173. Chen RJ, Lee YH, Yeh YL, Wang YJ, Wang BJ. The Roles of Autophagy and the inflammasome during environmental stress-triggered skin inflammation. Int J Mol Sci (2016) 17:2063. doi: 10.3390/ijms17122063
174. Li L, Chen X, Gu H. The signaling involved in autophagy machinery in keratinocytes and therapeutic approaches for skin diseases. Oncotarget (2016) 7:50682–97. doi: 10.18632/oncotarget.9330
175. Lee HM, Shin DM, Yuk JM, Shi G, Choi DK, Lee SH, et al. Autophagy negatively regulates keratinocyte inflammatory responses via scaffolding protein p62/SQSTM1. J Immunol. (2011) 186:1248–58. doi: 10.4049/jimmunol.1001954
176. Soong G, Paulino F, Wachtel S, Parker D, Wickersham M, Zhang D, et al. Methicillin-resistant Staphylococcus aureus adaptation to human keratinocytes. MBio (2015) 6:e00289-15. doi: 10.1128/mBio.00289-15
177. Nakahara T, Kido-Nakahara M, Ohno F, Ulzii D, Chiba T, Tsuji G, et al. The pruritogenic mediator endothelin-1 shifts the dendritic cell-T-cell response toward Th17/Th1 polarization. Allergy (2017) 73:511–515. doi: 10.1111/all.13322
178. Aktar MK, Kido-Nakahara M, Furue M, Nakahara T. Mutual upregulation of endothelin-1 and IL-25 in atopic dermatitis. Allergy (2015) 70:846–54. doi: 10.1111/all.12633
179. Yamada Y, Matsumoto T. House dust mites induce production of endothelin-1 and matrix metalloproteinase-9 in keratinocytes via proteinase-activated receptor-2 activation. Int Arch Allergy Immunol. (2017) 173:84–92. doi: 10.1159/000473700
180. Tsybikov NN, Petrisheva IV, Kuznik BI, Magen E. Plasma endothelin-1 levels during exacerbation of atopic dermatitis. Allergy Asthma Proc. (2015) 36:320–4. doi: 10.2500/aap.2015.36.3846
181. Bersanelli M, Mosca E, Remondini D, Giampieri E, Sala C, Castellani G, et al. Methods for the integration of multi-omics data: mathematical aspects. BMC Bioinformatics (2016) 17(Suppl. 2):15. doi: 10.1186/s12859-015-0857-9
182. Jang Y, Yu N, Seo J, Kim S, Lee S. MONGKIE: an integrated tool for network analysis and visualization for multi-omics data. Biol Direct. (2016) 11:10. doi: 10.1186/s13062-016-0112-y
183. Zhu J, Shi Z, Wang J, Zhang B. Empowering biologists with multi-omics data: colorectal cancer as a paradigm. Bioinformatics (2015) 31:1436–43. doi: 10.1093/bioinformatics/btu834
184. Huang H, Vangay P, McKinlay CE, Knights D. Multi-omics analysis of inflammatory bowel disease. Immunol Lett. (2014) 162:62–8. doi: 10.1016/j.imlet.2014.07.014
185. Kopczynski D, Coman C, Zahedi RP, Lorenz K, Sickmann A, Ahrends R. Multi-OMICS: a critical technical perspective on integrative lipidomics approaches. Biochim Biophys Acta (2017). 1862:808–11. doi: 10.1016/j.bbalip.2017.02.003
186. Yugi K, Kuroda S. Metabolism-centric trans-omics. Cell Syst. (2017) 4:19–20. doi: 10.1016/j.cels.2017.01.007
187. Lin E, Lane HY. Machine learning and systems genomics approaches for multi-omics data. Biomark Res. (2017) 5:2. doi: 10.1186/s40364-017-0082-y
188. Szappanos B, Kovacs K, Szamecz B, Honti F, Costanzo M, Baryshnikova A, et al. An integrated approach to characterize genetic interaction networks in yeast metabolism. Nat Genet. (2011) 43:656–62. doi: 10.1038/ng.846
189. Zhu Z, Zhang F, Hu H, Bakshi A, Robinson MR, Powell JE, et al. Integration of summary data from GWAS and eQTL studies predicts complex trait gene targets. Nat Genet. (2016) 48:481–7. doi: 10.1038/ng.3538
190. Hormozdiari F, van de Bunt M, Segre AV, Li X, Joo JW, Bilow M, et al. Colocalization of GWAS and eQTL signals detects target genes. Am J Hum Genet. (2016) 99:1245–60. doi: 10.1016/j.ajhg.2016.10.003
191. Wanichthanarak K, Fahrmann JF, Grapov D. Genomic, proteomic, and metabolomic data integration strategies. Biomark Insights (2015) 10:1–6. doi: 10.4137/BMI.S29511
192. Kamburov A, Cavill R, Ebbels TM, Herwig R, Keun HC. Integrated pathway-level analysis of transcriptomics and metabolomics data with IMPaLA. Bioinformatics (2011) 27:2917–8. doi: 10.1093/bioinformatics/btr499
193. Cavill R, Kamburov A, Ellis JK, Athersuch TJ, Blagrove MS, Herwig R, et al. Consensus-phenotype integration of transcriptomic and metabolomic data implies a role for metabolism in the chemosensitivity of tumour cells. PLoS Comput Biol. (2011) 7:e1001113. doi: 10.1371/journal.pcbi.1001113
194. Sun H, Wang H, Zhu R, Tang K, Gong Q, Cui J, et al. iPEAP: integrating multiple omics and genetic data for pathway enrichment analysis. Bioinformatics (2014) 30:737–9. doi: 10.1093/bioinformatics/btt576
195. Xia J, Wishart DS. Using MetaboAnalyst 3.0 for comprehensive metabolomics data analysis. Curr Protoc Bioinformatics (2016) 55:14.10.1–14. doi: 10.1002/cpbi.11
196. Xia J, Wishart DS. Web-based inference of biological patterns, functions and pathways from metabolomic data using MetaboAnalyst. Nat Protoc. (2011) 6:743–60. doi: 10.1038/nprot.2011.319
197. Zhang B, Hu S, Baskin E, Patt A, Siddiqui JK, Mathe EA. RaMP: a comprehensive relational database of metabolomics pathways for pathway enrichment analysis of genes and metabolites. Metabolites (2018) 8:E16. doi: 10.3390/metabo8010016
198. Siddiqui JK, Baskin E, Liu M, Cantemir-Stone CZ, Zhang B, Bonneville R, et al. IntLIM: integration using linear models of metabolomics and gene expression data. BMC Bioinformatics (2018) 19:81. doi: 10.1186/s12859-018-2085-6
199. Gosline SJ, Oh C, Fraenkel E. SAMNetWeb: identifying condition-specific networks linking signaling and transcription. Bioinformatics (2015) 31:1124–6. doi: 10.1093/bioinformatics/btu748
200. Karnovsky A, Weymouth T, Hull T, Tarcea VG, Scardoni G, Laudanna C, et al. Metscape 2 bioinformatics tool for the analysis and visualization of metabolomics and gene expression data. Bioinformatics (2012) 28:373–80. doi: 10.1093/bioinformatics/btr661
201. Basu S, Duren W, Evans CR, Burant CF, Michailidis G, Karnovsky A. Sparse network modeling and metscape-based visualization methods for the analysis of large-scale metabolomics data. Bioinformatics (2017) 33:1545–53. doi: 10.1093/bioinformatics/btx012
202. Wachter A, Beissbarth T. pwOmics: an R package for pathway-based integration of time-series omics data using public database knowledge. Bioinformatics (2015) 31:3072–4. doi: 10.1093/bioinformatics/btv323
203. Langfelder P, Horvath S. WGCNA: an R package for weighted correlation network analysis. BMC Bioinformatics (2008) 9:559. doi: 10.1186/1471-2105-9-559
204. Raghupathi W, Raghupathi V. Big data analytics in healthcare: promise and potential. Health Inf Sci Syst. (2014) 2:3. doi: 10.1186/2047-2501-2-3
205. Bunyavanich S, Schadt EE. Systems biology of asthma and allergic diseases: a multiscale approach. J Allergy Clin Immunol (2015) 135:31–42. doi: 10.1016/j.jaci.2014.10.015
206. Kottyan LC, Weirauch MT, Rothenberg ME. Making it big in allergy. J Allergy Clin Immunol. (2015) 135:43–5. doi: 10.1016/j.jaci.2014.10.041
208. Elias PM, Steinhoff M. “Outside-to-inside” (and now back to “outside”) pathogenic mechanisms in atopic dermatitis. J Invest Dermatol. (2008) 128:1067–70. doi: 10.1038/jid.2008.88
209. Valdman-Grinshpoun Y, Ben-Amitai D, Zvulunov A. Barrier-restoring therapies in atopic dermatitis: current approaches and future perspectives. Dermatol Res Pract. (2012) 2012:923134. doi: 10.1155/2012/923134
210. Brunner PM, Guttman-Yassky E, Leung DY. The immunology of atopic dermatitis and its reversibility with broad-spectrum and targeted therapies. J Allergy Clin Immunol. (2017) 139:S65–76. doi: 10.1016/j.jaci.2017.01.011
211. Guttman-Yassky E, Nograles KE, Krueger JG. Contrasting pathogenesis of atopic dermatitis and psoriasis–part I: clinical and pathologic concepts. J Allergy Clin Immunol. (2011) 127:1110–8. doi: 10.1016/j.jaci.2011.01.053
212. Guttman-Yassky E, Lowes MA, Fuentes-Duculan J, Zaba LC, Cardinale I, Nograles KE, et al. Low expression of the IL-23/Th17 pathway in atopic dermatitis compared to psoriasis. J Immunol. (2008) 181:7420–7. doi: 10.4049/jimmunol.181.10.7420
213. ArobddttUSCiA. Transforming Health Care through Big Data Strategies for Leveraging Big Data in the Health Care Industry. IHTT (2013).
214. Libbrecht MW, Noble WS. Machine learning applications in genetics and genomics. Nat Rev Genet. (2015) 16:321–32. doi: 10.1038/nrg3920
215. Crouser ED, Fingerlin TE, Yang IV, Maier LA, Nana-Sinkam P, Collman RG, et al. Application of “Omics” and systems biology to sarcoidosis research. Ann Am Thorac Soc. (2017) 14:S445–51. doi: 10.1513/AnnalsATS.201707-567OT
Keywords: atopic dermatitis, omics, multi-omics integration, endotypes, biomarkers, bioinformatics, machine learning, network analysis
Citation: Ghosh D, Bernstein JA, Khurana Hershey GK, Rothenberg ME and Mersha TB (2018) Leveraging Multilayered “Omics” Data for Atopic Dermatitis: A Road Map to Precision Medicine. Front. Immunol. 9:2727. doi: 10.3389/fimmu.2018.02727
Received: 26 June 2018; Accepted: 05 November 2018;
Published: 12 December 2018.
Edited by:
Miriam Wittmann, University of Leeds, United KingdomReviewed by:
Kiyoshi Hirahara, Chiba University, JapanAdewonuola Alase, University of Leeds, United Kingdom
Copyright © 2018 Ghosh, Bernstein, Khurana Hershey, Rothenberg and Mersha. This is an open-access article distributed under the terms of the Creative Commons Attribution License (CC BY). The use, distribution or reproduction in other forums is permitted, provided the original author(s) and the copyright owner(s) are credited and that the original publication in this journal is cited, in accordance with accepted academic practice. No use, distribution or reproduction is permitted which does not comply with these terms.
*Correspondence: Tesfaye B. Mersha, tesfaye.mersha@cchmc.org