- 1Department of Human Functional Genomics, Advanced Science Research Promotion Center, Organization for the Promotion of Regional Innovation, Mie University, Tsu, Japan
- 2Graduate School of Design, Kyushu University, Fukuoka, Japan
- 3Faculty of Engineering, Hokkaido University, Hokkaido, Japan
- 4Department of Human Science, Faculty of Design, Kyushu University, Fukuoka, Japan
- 5Physiological Anthropology Research Center, Faculty of Design, Kyushu University, Fukuoka, Japan
After the genomic era, the development of high-throughput sequencing technologies has allowed us to advance our understanding of genetic variants responsible for adaptation to high altitude in humans. However, transcriptomic characteristics associated with phenotypic plasticity conferring tolerance to acute hypobaric hypoxic stress remain unclear. To elucidate the effects of hypobaric hypoxic stress on transcriptional variability, we aimed to describe transcriptomic profiles in response to acute hypobaric hypoxia in humans. In a hypobaric hypoxic chamber, young Japanese males were exposed to a barometric pressure of 493 mmHg (hypobaric hypoxia) for 75 min after resting for 30 min at the pressure of 760 mmHg (normobaric normoxia) at 28°C. Saliva samples of the subjects were collected before and after hypobaric hypoxia exposure, to be used for RNA sequencing. Differential gene expression analysis identified 30 significantly upregulated genes and some of these genes may be involved in biological processes influencing hematological or immunological responses to hypobaric hypoxic stress. We also confirmed the absence of any significant transcriptional fluctuations in the analysis of basal transcriptomic profiles under no-stimulus conditions, suggesting that the 30 genes were actually upregulated by hypobaric hypoxia exposure. In conclusion, our findings showed that the transcriptional profiles of Japanese individuals can be rapidly changed as a result of acute hypobaric hypoxia, and this change may influence the phenotypic plasticity of lowland individuals for acclimatization to a hypobaric hypoxic environment. Therefore, the results obtained in this study shed light on the transcriptional mechanisms underlying high-altitude acclimatization in humans.
Introduction
High altitude is a hostile environment for humans owing to low oxygen availability through decreased barometric pressure (PB), and high-altitude illness such as acute mountain sickness in lowlanders can occur at altitudes above 2100 m (World Health Organization, 2012). While the conventional genome-wide association study is difficult to detect genes related to phenotypic plasticity in response to acute hypobaric hypoxic stress, the transcriptome analysis can provide a better understanding of the genetic mechanisms in response to the acute environmental stress. Therefore, the identification of the responsible genes using the transcriptome analysis is clinically important for the prevention of acute hypoxic insult because it could be helpful in identifying the therapeutic targets. The Andean, Ethiopian, and Tibetan highlanders have resided in the Andean Altiplano (South America), Semien Plateau (Africa), and Tibetan Plateau (East Asia), respectively. While all three populations have settled at high altitudes for millennia, these populations are considered to have adapted to high altitude environments via different genetic variants (Bigham, 2016). In 1990, ∼2.4 million Tibetans were estimated to reside at altitudes above 3500 m (Wu, 2001). In addition, it was estimated that ∼140 million people lived at altitudes above 2500 m across the world (Moore et al., 1998). Of these, 13.7, 1.7, and 20.7 million people were estimated to live in Ethiopia, Tibet, and South American Andes, respectively. Recent high-throughput genome sequencing studies have contributed to finding signals of positive natural selection acting on genes associated with hematological or pulmonary traits in people residing at high altitudes and revealed that genes in the hypoxia-inducible factor (HIF) pathway are responsible for high-altitude adaptation in highland populations (Bigham, 2016).
In particular, genetic polymorphisms of endothelial PAS domain protein 1 (EPAS1) and egl-9 family hypoxia inducible factor 1 (EGLN1), which play a pivotal role in the upstream of the HIF pathway, in Tibetan highlanders have been extensively investigated; for instance, the study subjects lived in the Qinghai province (∼4350 m) or the Tibet Autonomous Region (∼4300–4600 m) in China and the Solu-Khumbu region in Nepal (∼3440 m), or were the descendants living in Virginia and Utah in the United States (Simonson et al., 2010; Yi et al., 2010; Hanaoka et al., 2012; Huerta-Sánchez et al., 2014; Lorenzo et al., 2014; Xu et al., 2015). The previous studies have reported that EPAS1 and EGLN1 are responsible for a relatively low hemoglobin concentration, which may be diluted by increased plasma volume (Stembridge et al., 2019), in Tibetan highlanders because it protects against the development of high-altitude polycythemia due to elevated red blood cell volume, while positive natural selection may have acted independently on EGLN1 in Tibetan and Andean highlanders (Bigham et al., 2010). Moreover, a candidate-gene approach study exhibited that genetic polymorphisms of EGLN1 in Japanese lowlanders were associated with blood oxygen saturation variation during acute hypobaric hypoxia exposure, whereas putative adaptive alleles in highland populations may be susceptible to acute hypobaric hypoxia in the lowland individuals because of lower blood oxygen saturation levels (Yasukochi et al., 2018). The discrepancy of adaptability to hypobaric hypoxia is likely due to the differences between the genetic backgrounds of highland and lowland populations.
In addition to the effects of genetic polymorphisms on the physiological response to hypobaric hypoxia, transcriptional variation from a genome in an individual can provide phenotypic plasticity in response to the extreme environmental conditions, conferring acclimatization to rapid environmental changes. In recent years, transcriptomic profiles in response to hypoxic stress have been investigated in free-ranging or wild endothermic (Pan et al., 2017; Lim et al., 2019; Park et al., 2019; Qi et al., 2019) and model animals (Sharma et al., 2015; Xu et al., 2019). It is well known that hypoxic microenvironments profoundly affect cancer progression in humans (Jing et al., 2019). Therefore, transcriptome analyses of various human cell types (Ricciardi et al., 2008; Salman et al., 2017; Parodi et al., 2018; Klomp et al., 2020) including tumor cells (Abu-Jamous et al., 2017; Berglund et al., 2018; Lichawska-Cieslar et al., 2018) under hypoxic microenvironments have been conducted, yet there is little knowledge about the effects of acute hypobaric hypoxic stress on the transcriptional profiles of humans in vivo. This might be due to the limitation of experimental facility and it makes difficult to collect a fresh sample that is essential to obtain non-degraded RNA from a study subject in vivo, while we could address the issue by using a large-size hypobaric hypoxic chamber (∼67.3 m3). Here, to elucidate the effects of hypobaric hypoxic stress on transcriptional variability, we described the transcriptomic profiles of young Japanese males, who are not athlete and mountain-climber, in response to acute hypobaric hypoxic stress conditions. The results obtained from this study may help in understanding the molecular mechanisms of acute hypobaric hypoxic tolerance in modern humans, and the findings may be applied to treat acute hypoxic insults that occur at sea level.
Materials and Methods
Study Subjects
Three Japanese male students of Kyushu University (Fukuoka, Japan) participated in the present study (mean age ± standard deviation, 22 ± 1 years; height, 175.0 ± 9.3 cm; weight, 58.4 ± 6.9 kg; body mass index, 19.1 ± 2.7 kg/m2). The participants had no clinical problems and no medium- or long-term stay at high altitudes preceding the hypobaric hypoxic experiment. They had abstained from drinking alcohol, doing strenuous exercise, and smoking for 24 h before the experiment, and had been prohibited from drinking caffeine and from eating 2 h prior to entering the hypobaric hypoxic chamber (Research Center for Human Environmental Adaptation, Kyushu University, Fukuoka, Japan). The dimension of the column-shaped chamber is as follow: the diameter and depth are 3.5 m and 7 m, respectively (cylindrical volume, ∼67.3 m3).
The study protocol complied with the Declaration of Helsinki and was approved by the Clinical Research Ethics Review Committee of Mie University Hospital (Approval number U2019-016) and the Ethics Committee of the Faculty of Design, Kyushu University (Approval number 269).
Hypobaric Hypoxic Experiment
The hypobaric hypoxic experiment was carried out with the subjects wearing undershorts and short-sleeve T-shirts (∼0.12 clo) at 28°C. The experiment was conducted in the following order of conditions: the PB of 760 mmHg (equivalent to sea level) was maintained for 30 min; the PB was gradually decreased to the PB of 493 mmHg (equivalent to the altitude of 3500 m) for 30 min; the decreased PB was maintained for 75 min, and then the PB was recovered to the ambient atmospheric pressure at sea level. The air temperature was maintained at 28°C during the experiment and the total duration of the experiment was 135 min. The experimental protocol was designed on experience, with safety as the priority. Saliva samples of study subjects were collected using the Oragene® RNA kit (DNA Genotek, Ottawa, Canada) before and after exposure to hypobaric hypoxia. To assess the basal transcriptional fluctuations under no-stimulus conditions, two saliva samples were continuously collected from the experimenter (YY), who is a non-smoker, under normobaric normoxia conditions on the same day, in the similar manner of sample collection for the hypobaric hypoxic experiment (abstinence from drinking alcohol and doing strenuous exercise for 1 day, and from eating and drinking caffeine 2 h before the experiment). To minimize the risk of RNA contamination, total RNA of these samples was extracted on the different days.
RNA Sequencing and Data Processing
Total RNA was extracted from the subjects’ saliva samples with a volume ≥ 2 mL using either the RNeasy micro kit (Qiagen, Hilden, Germany) or the NucleoSpin® RNA kit (TaKaRa Bio, Shiga, Japan) according to the manufacturers’ protocol. NucleoSpin® RNA Clean-up XS (TaKaRa Bio) was used to purify and concentrate the diluted RNA if the amount of total RNA was insufficient for RNA sequencing (RNA-seq). The sequence library was prepared using the TruSeq® Stranded Total RNA Library Prep kit (Illumina, San Diego, CA, United States) and was sequenced as 100-bp paired-end (PE) reads on an Illumina NovaSeq 6000 platform (Illumina). The library preparation and RNA-seq were performed by Macrogen (Seoul, South Korea).
We used the fastp ver. 0.19.7 (Chen et al., 2018) to remove the Illumina PE adapters, polyG tail (and polyX tail), and low quality reads with the following parameters: −q 15 −n 10 −t 1 −T 1 −l 20. The filtered PE reads were mapped to the human reference genome (GRCh38) with annotation information included in the gtf (gene transfer format) file from Ensembl GRCh38.96, using the STAR ver. 2.5.3 (Dobin et al., 2013) after the reference genome was indexed by the RSEM ver. 1.3.0 (Li and Dewey, 2011). The RSEM program was also used to estimate gene expression levels from the mapped BAM (binary alignment map) files.
Differential Gene Expression Analysis
From the estimated expression abundance (read-count) data at the gene level, we filtered out genes with < 7 read counts across all samples for further analysis. We normalized the filtered count data and estimated the log2 fold changes (LFC) using the generalized linear models with a negative binomial distribution implemented in Bioconductor R packages DESeq2 ver. 1.22.2 (Love et al., 2014) or edgeR ver. 3.24.3 (Robinson et al., 2009; McCarthy et al., 2012). The significance of gene expression differences between the normobaric normoxia and hypobaric hypoxia conditions was examined by the Wald or exact tests, and the p-value was adjusted to the false discovery rate (FDR) using the Benjamin and Hochberg method (Benjamini and Hochberg, 1995) for multiple testing. We identified genes with an FDR of < 0.05 and a |LFC| of ≥ 1 as differentially expressed genes (DEGs). After the count read data were transformed to the log2 scale by the rlog function in DESeq2, we performed principal component (PCA) and Euclidean distance analysis to compare the transcriptome similarity among samples. The differential gene expression analysis described above was performed using R software ver. 3.5.3 (R Core Team, 2019) via RStudio ver. 1.2.5019 (RStudio Team, 2019).
Gene-Set Enrichment and Pathway Analysis
We performed Gene Ontology (GO) enrichment analysis in the biological process category for the DEGs identified in the present study, using the Bioconductor R package topGO ver. 2.34.0 (Alexa et al., 2006; Alexa and Rahnenfuhrer, 2018) via the RStudio software and ClueGO ver. 2.5.4 (Bindea et al., 2009) and BiNGO ver. 3.0.3 (Maere et al., 2005) via Cytoscape ver. 3.7.1 (Shannon et al., 2003). The statistical significance of the enrichment was tested using Fisher’s exact and hypergeometric tests in the topGO and ClueGO/BiNGO, respectively (FDR < 0.05). After the gene symbol of DEGs was converted into the NCBI Entrez Gene ID using the Bioconductor R package biomaRt ver. 2.38.0 (Durinck et al., 2005, 2009), the converted IDs were mapped to the Kyoto Encyclopedia of Genes and Genomes (KEGG1) pathway. A functional network of GO terms was generated using the topGO R package. The functional interactions between the DEGs were predicted using GeneMANIA ver. 3.5.1 Cytoscape plugin (Montojo et al., 2010, 2014; Warde-Farley et al., 2010) via the Cytoscape software.
Results
Identification of Differentially Expressed Genes in the Hypobaric Hypoxic Experiments
A total of 307 million reads (51 ± 7 million reads) were obtained using RNA-seq from the six saliva samples belonging to the three Japanese individuals, to compare their transcriptomic profiles before and after exposure to acute hypobaric hypoxia. After quality control, 23 ± 3 million reads (18–26 million reads) were uniquely mapped to the human reference genome assembly. According to PCA (Figure 1A) and Euclidean distance analysis (Figure 1B) using the RNA-seq data, the expression vectors of the samples from the same individuals were clustered and formed three distinct groups of individuals, irrespective of the experimental conditions.
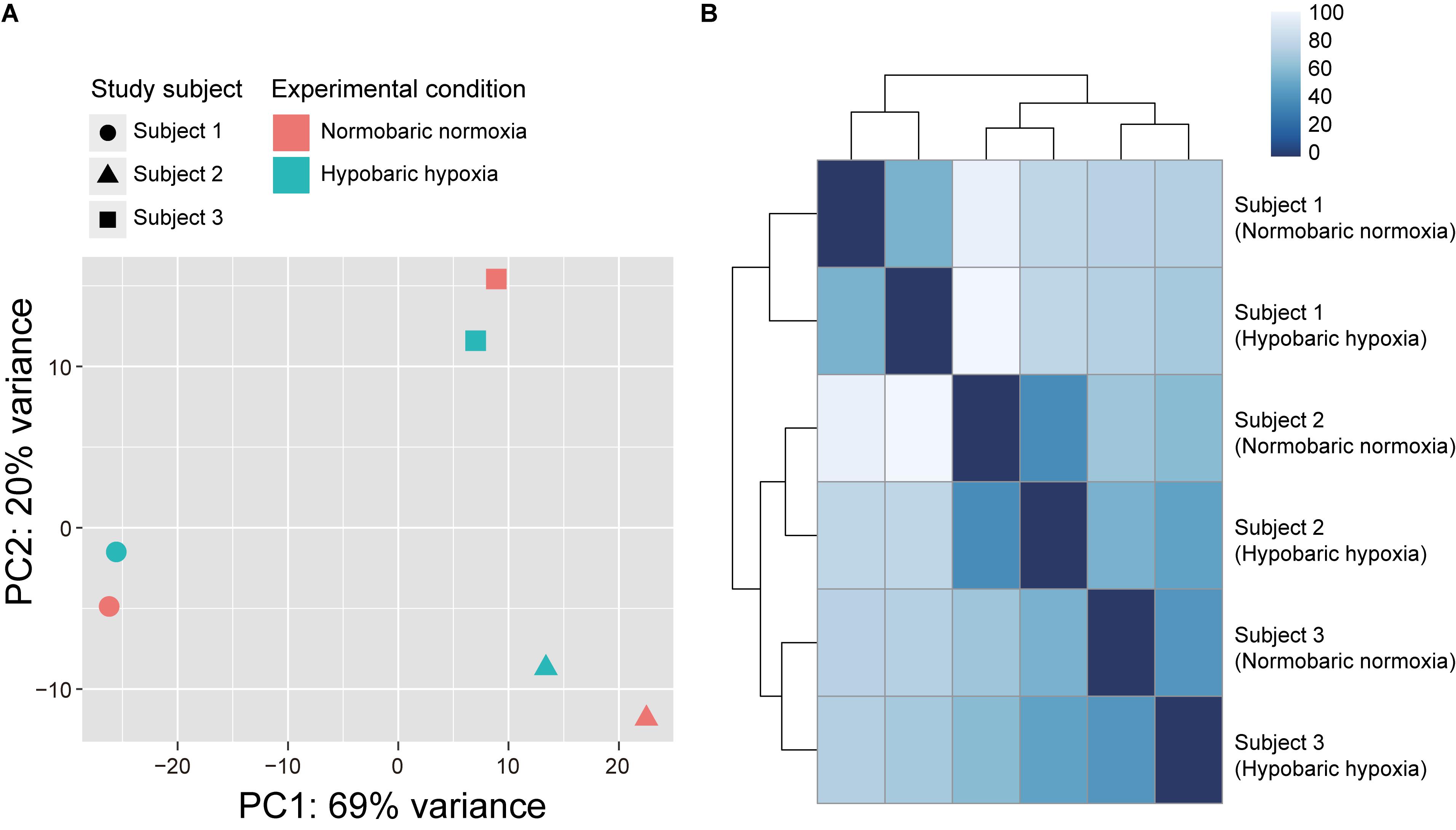
Figure 1. Transcriptome similarity among samples from three Japanese subjects participating in the acute hypobaric hypoxic experiments. (A) Principal component analysis of RNA-seq data plotted according to the first (horizontal axis) and second (vertical axis) principal components. (B) Heatmap of Euclidean distance between the expression vectors. The Euclidean distance was calculated using the R dist function.
Using the DESeq2 program, we conducted the differential gene expression analysis and identified 30 DEGs being upregulated after exposure to acute hypobaric hypoxia (FDR < 0.05, LFC ≥ 1), while none of the significantly downregulated genes were detected (Table 1). The full gene name of these DEGs is listed in Supplementary Table S1. Of the upregulated 30 DEGs (mean LFC, 1.23 ± 0.18), 28 were protein-coding genes and the expression levels of at least 17 DEGs were possibly affected by hypoxic stress including tumor hypoxia or HIF-pathway activity in human cells or animal tissues (Supplementary Table S1). The remaining two DEGs (LINC02487 and AC005083.1) were long noncoding RNA or processed transcript. We examined the differences in the expression levels among individuals in the top 10 ranked DEGs and found that the degree of transcriptional changes in each DEG varied within each individual (Figure 2).
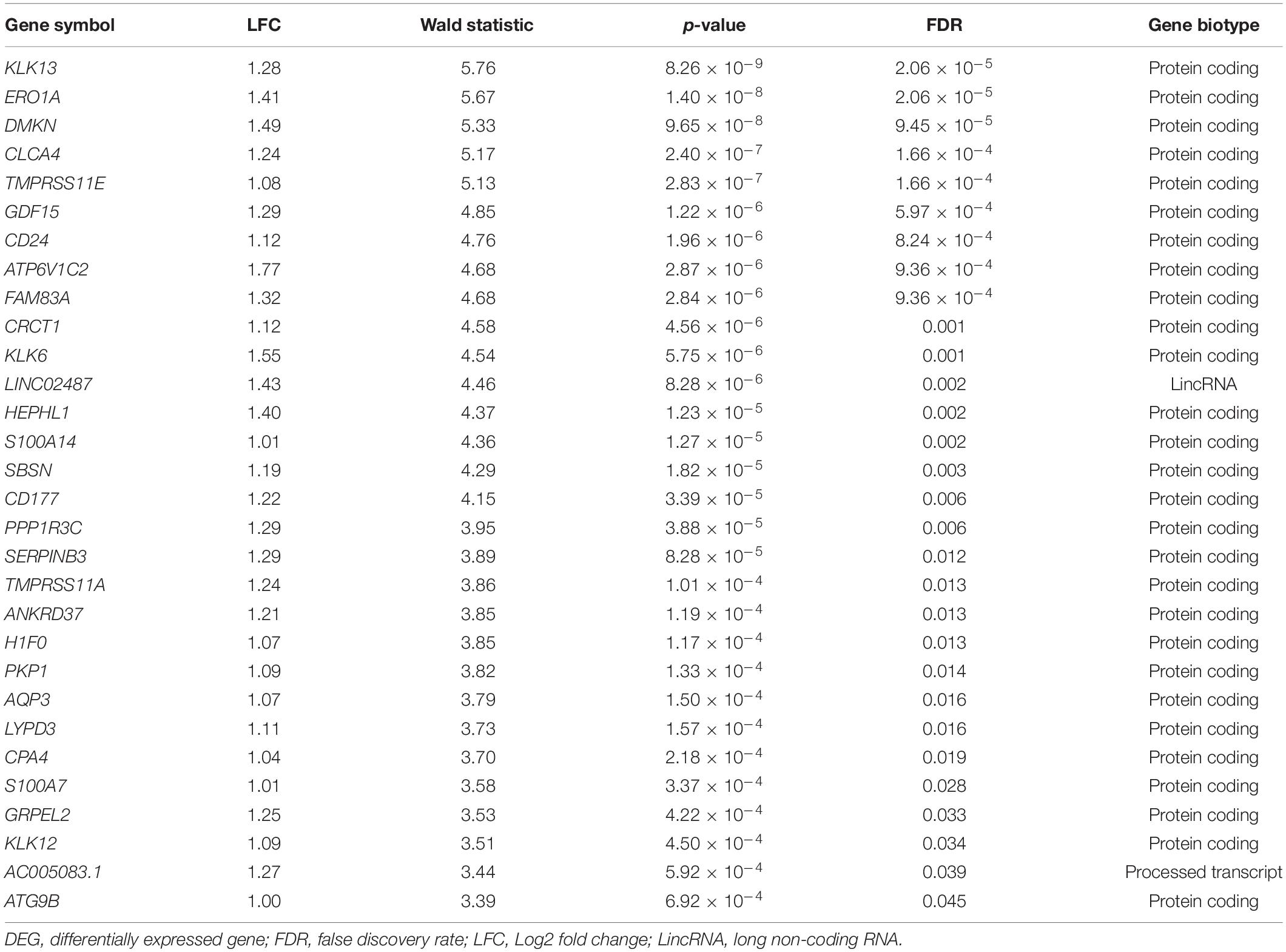
Table 1. Thirty DEGs (FDR < 0.05, LFC ≥ 1) between normobaric-normoxic and hypobaric-hypoxic conditions in male Japanese subjects.
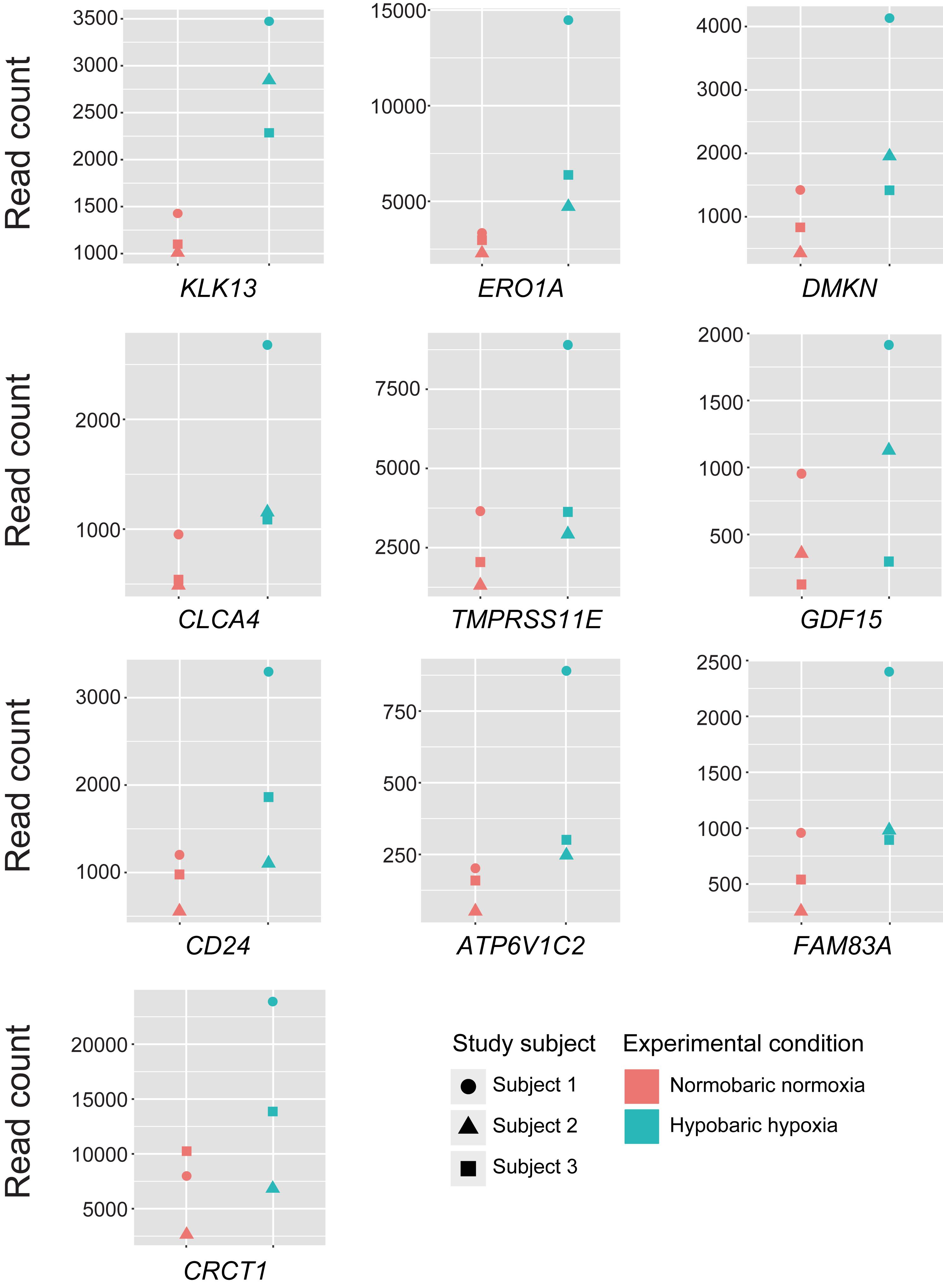
Figure 2. Expression levels of the top 10 differentially expressed genes in each of the study subjects. Read counts represent the estimated expression abundance at the gene level.
The differential gene expression analysis with the edgeR program detected 48 DEGs (36 upregulated and 12 downregulated genes) and 19 of the DEGs were also identified in the analysis with DESeq2 (Supplementary Table S2). However, |LFC| values of several DEGs were exceptionally high (> 7) whereas the read counts were low (Supplementary Figure S1). We thus used the 30 DEGs identified by DESeq2 for further analyses because the results obtained from this program were more conservative.
Assessment of Baseline Variability in Gene Expression Levels During Normobaric Normoxia
To confirm basal transcriptomic profiles under no-stimulus conditions, we carried out the RNA-seq analysis using two saliva samples collected from the experimenter (YY) under normobaric normoxic environments on the same day, in the similar manner of the hypobaric hypoxic experiment. We obtained a total of 120 million reads (60 ± 4 million reads), and after quality control 27 million reads (26–28 million reads) were uniquely mapped to the human reference genome. To detect the DEG, the differential gene expression analysis was implemented in the edgeR program. The differential gene expression analysis indicated no significant difference (FDR > 0.05) in the gene expression levels during normobaric normoxic conditions, even though edgeR detected more DEGs than DESeq2 in the expression analysis for the hypobaric hypoxic experiment. Therefore, we used the 30 DEGs identified by DESeq2 for further analyses.
Gene Function Enrichment Analysis
Using the 30 DEGs identified, we carried out GO enrichment analysis with topGO, BiNGO, and ClueGO programs, identifying a total of 145 significantly overrepresented GO terms (FDR < 0.05) based on the biological process category (Supplementary Tables S3–S5). Of these 145 GO terms, three related to the regulation of monocyte or granulocyte chemotaxis were consistently identified by all the enrichment analyses (Supplementary Figure S2). In addition, two and one GO terms were shared between BiNGO and topGO, and between ClueGO and topGO, respectively. These terms were also mainly related to the function of white blood cell subtypes. In our enrichment analyses, the GO terms associated with immunological or inflammatory processes accounted for a relatively high proportion of the enrichment analysis.
We also conducted the KEGG pathway analysis to predict the molecular function of the DEGs identified. The DEGs were mapped to 23 KEGG pathways although the number of DEGs included in each pathway was 1–2 only (Supplementary Table S6), and some of the KEGG pathways appeared to be related to functions in the immune system. Moreover, the hierarchical tree of GO terms overrepresented in the topGO analysis exhibited a significant relation of DEGs to migration and to the activation of several white blood cell types that are responsible for immune functions (Supplementary Figure S3). To further investigate the functional interactions among the DEGs, with the exception of long noncoding RNA or processed transcript, we performed a GeneMANIA network analysis (Figure 3). The network displayed potential direct or indirect interactions between the DEGs: many DEGs were coexpressed or colocalized and several pairs of DEGs shared a protein domain or directly interacted with each other.
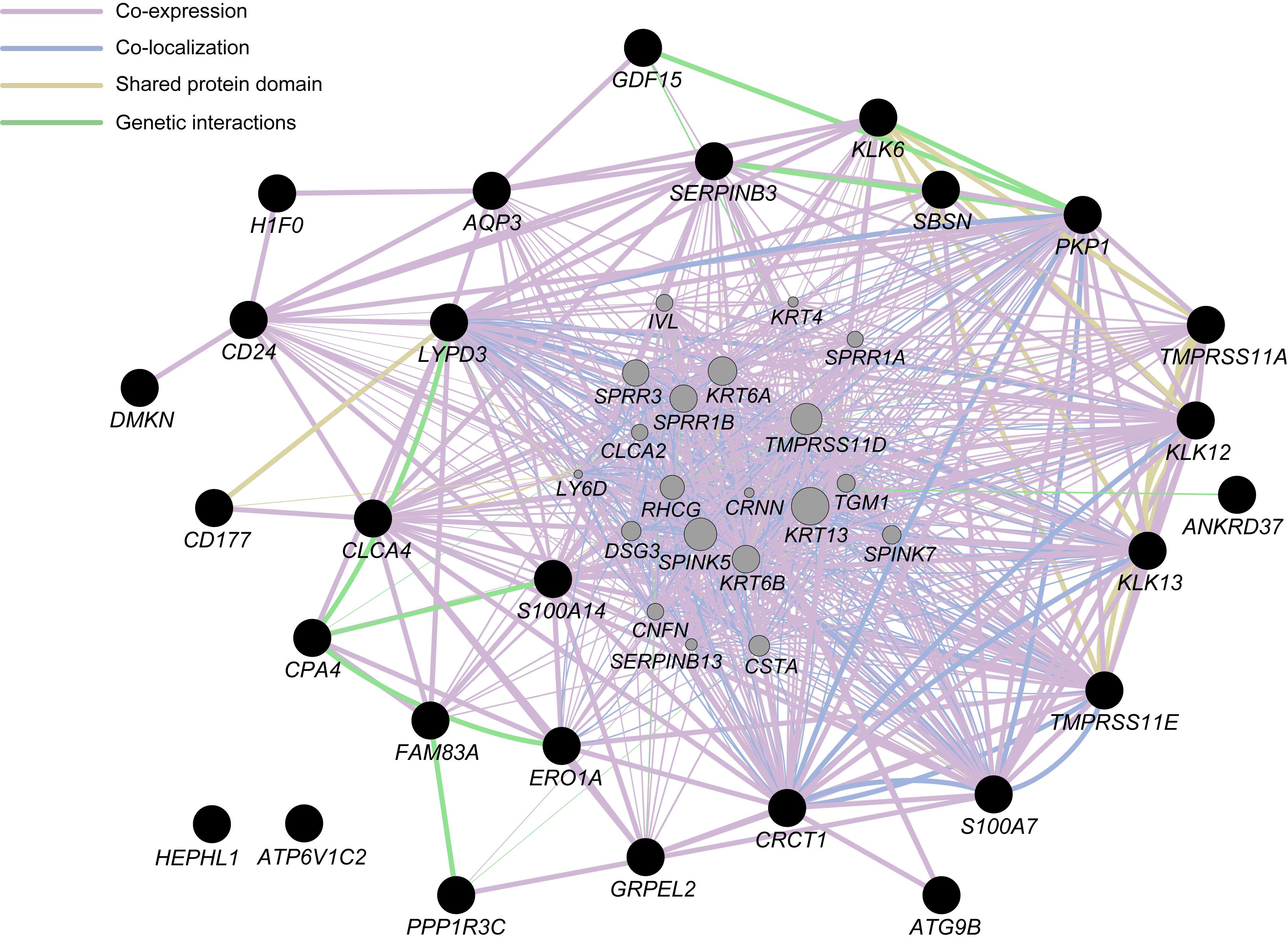
Figure 3. Network analysis for differentially expressed genes (DEGs), with the exception of long non-coding RNA or processed transcript, identified in the present study (closed black circles) with the use of GeneMANIA ver. 3.5.1 Cytoscape plugin via Cytoscape ver. 3.7.1. Interactions between the DEGs are indicated by bold lines. Genes represented by gray circles are putative mediators of the interactions between DEGs.
Discussion
The 30 DEGs were significantly upregulated in the three Japanese male subjects after exposure to acute hypobaric hypoxia. Under no-stimulus conditions, no significant transcriptional changes were observed, suggesting that the identified 30 DEGs in the Japanese individuals were actually upregulated owing to hypobaric hypoxic stress. Of these DEGs, several genes have been reported to be involved in response to hypoxic environments in previous studies using human cells and animal tissues.
The ANKRD37 gene has been identified as a HIF target gene (Benita et al., 2009) and has a relatively early response of gene expression to hypoxic exposure (within 120 min) in human endothelial cells (Klomp et al., 2020), although the protein function encoded by ANKRD37 remains to be elucidated. Our study demonstrated that the ANKRD37 expression was significantly upregulated in the Japanese individuals after acute hypobaric hypoxia exposure for 75 min, and thus this gene may play an important role in response to hypobaric hypoxic stress at an early stage. We also found a significant upregulation of S100A7 involved in various cellular processes such as proliferation and differentiation. Psoriasin, which is encoded by this gene, in keratinocytes was previously upregulated by hypoxic stress, and the expression patterns may affect several regulators of angiogenesis (Vegfors et al., 2016), suggesting that S100A7 contributes to the hematological response to hypobaric hypoxia exposure in humans. In our study, ATG9B was also significantly upregulated in the Japanese individuals. It has been reported that the ATG9B transcript downregulates the nitric oxide synthase 3 (NOS3) transcript, and these gene expression levels in human endothelial cells can be changed by hypoxic environments (Kalinowski et al., 2016). Given that NOS3 is responsible for the regulation of vascular wall homeostasis through production of nitric oxide with anti-inflammatory properties in the vascular endothelium (Fish and Marsden, 2006), ATG9B may be important for the regulation of hemodynamics in response to hypobaric hypoxic exposure. The expression levels of several other DEGs also appeared to be influenced by hypoxic stress in human cells (see Supplementary Table S1). In addition to the DEGs related to the hypoxic transcriptional changes in human cells, the gene expression of HEPHL1 and SBSN was previously upregulated under hypoxic environments in zebrafish (Long et al., 2015) and sheep (Goyal and Longo, 2014), respectively. It has been reported that physiological responses such as ventilation were different between normobaric hypoxia and hypobaric hypoxia exposure (Coppel et al., 2015). This might be due to more severe oxidative stress under hypobaric hypoxia environments (Ribon et al., 2016); thus, the decrease of PB may enhance the upregulation of genes involved in the production of reactive oxygen species (ROS) and antioxidant enzyme activities due to cellular oxidative damage. Indeed, the gene expression level of ERO1A, which is related to the ROS production (Nguyen et al., 2011), was significantly upregulated in the present study. Taken together, these results suggest that several DEGs identified in the present study are present in biological pathways relevant to physiological responses such as the alteration of hemodynamics and immune dynamics during hypoxic exposure, although the functional relevance of the DEGs to the physiological responses in humans remains unclear.
The GO enrichment and pathway analysis implemented in this study suggested that several DEGs were involved in immunological or inflammatory reactions during hypobaric hypoxia exposure. Given that the HIF pathway regulates the immune and inflammatory functions (Taylor and Colgan, 2017), the biological processes estimated from the DEGs may contribute to the altered immune cell activity through HIF-related pathways affected by exposure to acute hypobaric hypoxia. Although the KEGG pathway analysis indicated that few DEGs were involved in the same biological pathway, the GeneMANIA network analysis exhibited possible functional interactions among several DEGs. One may hypothesize that these DEGs participate in novel biological processes in response to hypobaric hypoxia exposure; alternatively, several biological processes including the DEGs might be activated simultaneously under hypobaric hypoxia.
However, transcriptional fluctuations observed in this study were limited within the same individual although we confirmed that the transcriptional patterns were altered by the hypobaric hypoxic experiments. This suggests that acute hypobaric hypoxic stress did not greatly affect the transcriptome profile of the study subjects, and it may result in no detection of significantly downregulated DEGs. It is possible that peaks of gene expression in response to hypoxia are varied and depend on the duration of hypoxic exposure (Klomp et al., 2020), while human DNA methylation levels, which can regulate gene expression, may be influenced by the severity or the duration of hypoxic exposure (Childebayeva et al., 2019). In addition, we focused on transcriptional changes after acute hypobaric hypoxia exposure but did not assess them during the exposure. This might decrease the statistical power to detect DEGs. Thus, further comprehensive analyses of RNA-seq data obtained from samples at different time points during the hypobaric hypoxic experiments are required. Also, it is necessary to collect the RNA sample for basal transcriptomic profiles under the no-stimulus condition from the hypobaric hypoxic experiment subjects.
In the present study, the magnitudes of transcriptional changes in each of the top 10 ranked DEGs under hypobaric hypoxia were relatively greater in the subject 1 than in the subjects 2 and 3. Given that the transcriptomic profile of the subject 1 appeared to be different from the other subjects in the PCA analysis, one may hypothesize that the transcriptional fluctuation of the subject 1 is different from the other subjects and may be susceptible to hypobaric hypoxic exposure. However, we cannot rule out the possibility to overestimate the transcriptional changes in the subject 1. It will be intriguing to examine interindividual variability of transcriptional changes in response to hypobaric hypoxic exposure, with a larger sample size.
The comparison of transcriptomic profiles before and after the alteration of environmental conditions is useful to evaluate phenotypic plasticity in response to environmental changes because the alteration of gene expression levels can be quantified. However, attention should be paid to the fact that gene expression levels are not necessarily correlated with protein levels, and that phenotypic plasticity can be affected by various factors including intrinsic characteristics of individuals and lifestyles. In addition, epigenetic modifications can affect gene expression levels. Further analyses for proteomic and epigenetic profiles are required to elucidate the molecular mechanisms underlying high-altitude acclimatization in humans.
In conclusion, we identified 30 DEGs being upregulated after acute hypobaric hypoxia exposure in Japanese lowlanders, and the transcriptional patterns of several genes can be altered owing to the exposure. This alteration may confer phenotypic plasticity in response to short-term hypoxic environmental changes; thus, it will be intriguing to examine differences in the transcriptomic profiles of groups of individuals showing similar physiological responses to acute hypobaric hypoxia. The results obtained in this study contribute to a better understanding of the physiological acclimatization of present-day lowlander humans to hypobaric hypoxia environments.
Data Availability Statement
The datasets presented in this article are not readily available because of ethical and privacy issues. However, scientifically motivated requests for data sharing will be considered by reviewing the ethical committee of our institute.
Ethics Statement
The studies involving human participants were reviewed and approved by the Clinical Research Ethics Review Committee of Mie University Hospital (Approval No. U2019-016) and the Ethics Committee of the Faculty of Design, Kyushu University (Approval No. 269). The participants provided their written informed consent to participate in this study.
Author Contributions
TM conceived and designed the research. YY contributed to the research design, carried out the molecular lab work, participated in the analysis and interpretation of the data, and drafted the manuscript. TM and SS carried out the hypobaric hypoxic experiments and participated in the saliva sampling and data acquisition. TM, HW, and SS contributed to the interpretation of the data and the revision of the manuscript. All authors have read and approved the final manuscript.
Funding
This work was supported by the JSPS KAKENHI Grant-in-Aid for Scientific Research (B) (Grant No. 18H02518 to TM, HW, and YY) and Grant-in-Aid for Scientific Research (C) (Grant No. 18K06441 to YY).
Conflict of Interest
The authors declare that the research was conducted in the absence of any commercial or financial relationships that could be construed as a potential conflict of interest.
Acknowledgments
We deeply thank all the subjects for their participation in the study. We owe special thanks to Dr. Midori Motoi for sample management. Computations were partially performed on the NIG supercomputer at ROIS National Institute of Genetics.
Supplementary Material
The Supplementary Material for this article can be found online at: https://www.frontiersin.org/articles/10.3389/fgene.2020.559074/full#supplementary-material
Footnotes
References
Abu-Jamous, B., Buffa, F. M., Harris, A. L., and Nandi, A. K. (2017). In vitro downregulated hypoxia transcriptome is associated with poor prognosis in breast cancer. Mol. Cancer 16:105. doi: 10.1186/s12943-017-0673-0
Alexa, A., and Rahnenfuhrer, J. (2018). topGO: Enrichment Analysis for Gene Ontology. R package version 2.34.0.
Alexa, A., Rahnenführer, J., and Lengauer, T. (2006). Improved scoring of functional groups from gene expression data by decorrelating GO graph structure. Bioinformatics 22, 1600–1607. doi: 10.1093/bioinformatics/btl140
Benita, Y., Kikuchi, H., Smith, A. D., Zhang, M. Q., Chung, D. C., and Xavier, R. J. (2009). An integrative genomics approach identifies Hypoxia Inducible Factor-1 (HIF-1)-target genes that form the core response to hypoxia. Nucleic Acids Res. 37, 4587–4602. doi: 10.1093/nar/gkp425
Benjamini, Y., and Hochberg, Y. (1995). Controlling the false discovery rate: a practical and powerful approach to multiple testing. J. R. Stat. Soc. Ser. B 57, 289–300. doi: 10.1111/j.2517-6161.1995.tb02031.x
Berglund, E., Maaskola, J., Schultz, N., Friedrich, S., Marklund, M., Bergenstråhle, J., et al. (2018). Spatial maps of prostate cancer transcriptomes reveal an unexplored landscape of heterogeneity. Nat. Commun. 9, 1–13. doi: 10.1038/s41467-018-04724-4725
Bigham, A., Bauchet, M., Pinto, D., Mao, X., Akey, J. M., Mei, R., et al. (2010). Identifying signatures of natural selection in tibetan and andean populations using dense genome scan data. PLoS Genet. 6:e1001116. doi: 10.1371/journal.pgen.1001116
Bigham, A. W. (2016). Genetics of human origin and evolution: high-altitude adaptations. Curr. Opin. Genet. Dev. 41, 8–13. doi: 10.1016/j.gde.2016.06.018
Bindea, G., Mlecnik, B., Hackl, H., Charoentong, P., Tosolini, M., Kirilovsky, A., et al. (2009). ClueGO: a cytoscape plug-in to decipher functionally grouped gene ontology and pathway annotation networks. Bioinformatics 25, 1091–1093. doi: 10.1093/bioinformatics/btp101
Chen, S., Zhou, Y., Chen, Y., and Gu, J. (2018). fastp: an ultra-fast all-in-one FASTQ preprocessor. Bioinformatics 34, i884–i890. doi: 10.1093/bioinformatics/bty560
Childebayeva, A., Harman, T., Weinstein, J., Goodrich, J. M., Dolinoy, D. C., Day, T. A., et al. (2019). DNA methylation changes are associated with an incremental ascent to high altitude. Front. Genet. 10:1062. doi: 10.3389/fgene.2019.01062
Coppel, J., Hennis, P., Gilbert-Kawai, E., and Grocott, M. P. W. (2015). The physiological effects of hypobaric hypoxia versus normobaric hypoxia: a systematic review of crossover trials. Extrem. Physiol. Med. 4:2. doi: 10.1186/s13728-014-0021-6
Dobin, A., Davis, C. A., Schlesinger, F., Drenkow, J., Zaleski, C., Jha, S., et al. (2013). STAR: ultrafast universal RNA-seq aligner. Bioinformatics 29, 15–21. doi: 10.1093/bioinformatics/bts635
Durinck, S., Moreau, Y., Kasprzyk, A., Davis, S., De Moor, B., Brazma, A., et al. (2005). BioMart and bioconductor: a powerful link between biological databases and microarray data analysis. Bioinformatics 21, 3439–3440. doi: 10.1093/bioinformatics/bti525
Durinck, S., Spellman, P. T., Birney, E., and Huber, W. (2009). Mapping identifiers for the integration of genomic datasets with the R/Bioconductor package biomaRt. Nat. Protoc. 4, 1184–1191. doi: 10.1038/nprot.2009.97
Fish, J. E., and Marsden, P. A. (2006). Endothelial nitric oxide synthase: insight into cell-specific gene regulation in the vascular endothelium. Cell. Mol. Life Sci. 63, 144–162. doi: 10.1007/s00018-005-5421-5428
Goyal, R., and Longo, L. D. (2014). Acclimatization to long-term hypoxia: gene expression in ovine carotid arteries. Physiol. Genomics 46, 725–734. doi: 10.1152/physiolgenomics.00073.2014
Hanaoka, M., Droma, Y., Basnyat, B., Ito, M., Kobayashi, N., Katsuyama, Y., et al. (2012). Genetic variants in EPAS1 contribute to adaptation to high-altitude hypoxia in Sherpas. PLoS One 7:e50566. doi: 10.1371/journal.pone.0050566
Huerta-Sánchez, E., Jin, X., Asan, Bianba, Z., Peter, B. M., Vinckenbosch, N., et al. (2014). Altitude adaptation in tibetans caused by introgression of denisovan-like DNA. Nature 512, 194–197. doi: 10.1038/nature13408
Jing, X., Yang, F., Shao, C., Wei, K., Xie, M., Shen, H., et al. (2019). Role of hypoxia in cancer therapy by regulating the tumor microenvironment. Mol. Cancer 18:157. doi: 10.1186/s12943-019-1089-1089
Kalinowski, L., Janaszak-Jasiecka, A., Siekierzycka, A., Bartoszewska, S., Woźniak, M., Lejnowski, D., et al. (2016). Posttranscriptional and transcriptional regulation of endothelial nitric-oxide synthase during hypoxia: the role of microRNAs. Cell. Mol. Biol. Lett. 21:16. doi: 10.1186/s11658-016-0017-x
Klomp, J., Hyun, J., Klomp, J., Pajcini, K., Rehman, J., and Malik, A. B. (2020). Comprehensive transcriptomic profiling reveals SOX7 as an early regulator of angiogenesis in hypoxic human endothelial cells. J. Biol. Chem. 295, 4796–4808. doi: 10.1074/jbc.RA119.011822
Li, B., and Dewey, C. N. (2011). RSEM: accurate transcript quantification from RNA-Seq data with or without a reference genome. BMC Bioinform. 12:323. doi: 10.1186/1471-2105-12-323
Lichawska-Cieslar, A., Pietrzycka, R., Ligeza, J., Kulecka, M., Paziewska, A., Kalita, A., et al. (2018). RNA sequencing reveals widespread transcriptome changes in a renal carcinoma cell line. Oncotarget 9, 8597–8613. doi: 10.18632/oncotarget.24269
Lim, M. C. W., Witt, C. C., Graham, C. H., and Dávalos, L. M. (2019). Parallel molecular evolution in pathways, genes, and sites in high-elevation hummingbirds revealed by comparative transcriptomics. Genome Biol. Evol. 11, 1573–1585. doi: 10.1093/gbe/evz101
Long, Y., Yan, J., Song, G., Li, X., Li, X., Li, Q., et al. (2015). Transcriptional events co-regulated by hypoxia and cold stresses in Zebrafish larvae. BMC Genomics 16:385. doi: 10.1186/s12864-015-1560-y
Lorenzo, F. R., Huff, C., Myllymäki, M., Olenchock, B., Swierczek, S., Tashi, T., et al. (2014). A genetic mechanism for Tibetan high-altitude adaptation. Nat. Genet. 46, 951–956. doi: 10.1038/ng.3067
Love, M. I., Huber, W., and Anders, S. (2014). Moderated estimation of fold change and dispersion for RNA-seq data with DESeq2. Genome Biol. 15:550. doi: 10.1186/s13059-014-0550-558
Maere, S., Heymans, K., and Kuiper, M. (2005). BiNGO: a Cytoscape plugin to assess overrepresentation of gene ontology categories in Biological Networks. Bioinformatics 21, 3448–3449. doi: 10.1093/bioinformatics/bti551
McCarthy, D. J., Chen, Y., and Smyth, G. K. (2012). Differential expression analysis of multifactor RNA-Seq experiments with respect to biological variation. Nucleic Acids Res. 40, 4288–4297. doi: 10.1093/nar/gks042
Montojo, J., Zuberi, K., Rodriguez, H., Bader, G. D., and Morris, Q. (2014). GeneMANIA: fast gene network construction and function prediction for cytoscape. F1000Research 3:153. doi: 10.12688/f1000research.4572.1
Montojo, J., Zuberi, K., Rodriguez, H., Kazi, F., Wright, G., Donaldson, S. L., et al. (2010). GeneMANIA cytoscape plugin: fast gene function predictions on the desktop. Bioinformatics 26, 2927–2928. doi: 10.1093/bioinformatics/btq562
Moore, L. G., Niermeyer, S., and Zamudio, S. (1998). Human adaptation to high altitude: regional and life-cycle perspectives. Am. J. Phys. Anthropol. 107, 25–64. doi: 10.1002/(sici)1096-8644(1998)107:27%2B<25::aid-ajpa3>3.0.co;2-l
Nguyen, V. D., Saaranen, M. J., Karala, A. R., Lappi, A. K., Wang, L., Raykhel, I. B., et al. (2011). Two endoplasmic reticulum PDI peroxidases increase the efficiency of the use of peroxide during disulfide bond formation. J. Mol. Biol. 406, 503–515. doi: 10.1016/j.jmb.2010.12.039
Pan, S., Zhang, T., Rong, Z., Hu, L., Gu, Z., Wu, Q., et al. (2017). Population transcriptomes reveal synergistic responses of DNA polymorphism and RNA expression to extreme environments on the Qinghai–Tibetan Plateau in a predatory bird. Mol. Ecol. 26, 2993–3010. doi: 10.1111/mec.14090
Park, W., Srikanth, K., Lim, D., Park, M., Hur, T., Kemp, S., et al. (2019). Comparative transcriptome analysis of Ethiopian indigenous chickens from low and high altitudes under heat stress condition reveals differential immune response. Anim. Genet. 50, 42–53. doi: 10.1111/age.12740
Parodi, M., Raggi, F., Cangelosi, D., Manzini, C., Balsamo, M., Blengio, F., et al. (2018). Hypoxia modifies the transcriptome of human NK cells, modulates their immunoregulatory profile, and influences NK cell subset migration. Front. Immunol. 9:2358. doi: 10.3389/fimmu.2018.02358
Qi, X., Zhang, Q., He, Y., Yang, L., Zhang, X., Shi, P., et al. (2019). The transcriptomic landscape of Yaks reveals molecular pathways for high altitude adaptation. Genome Biol. Evol. 11, 72–85. doi: 10.1093/gbe/evy264
Ribon, A., Pialoux, V., Saugy, J. J., Rupp, T., Faiss, R., Debevec, T., et al. (2016). Exposure to hypobaric hypoxia results in higher oxidative stress compared to normobaric hypoxia. Respir. Physiol. Neurobiol. 223, 23–27. doi: 10.1016/j.resp.2015.12.008
Ricciardi, A., Elia, A. R., Cappello, P., Puppo, M., Vanni, C., Fardin, P., et al. (2008). Transcriptome of hypoxic immature dendritic cells: modulation of chemokine/receptor expression. Mol. Cancer Res. 6, 175–185. doi: 10.1158/1541-7786.mcr-07-0391
Robinson, M. D., McCarthy, D. J., and Smyth, G. K. (2009). edgeR: a Bioconductor package for differential expression analysis of digital gene expression data. Bioinformatics 26, 139–140. doi: 10.1093/bioinformatics/btp616
Salman, M. M., Kitchen, P., Woodroofe, M. N., Bill, R. M., Conner, A. C., Heath, P. R., et al. (2017). Transcriptome analysis of gene expression provides new insights into the effect of mild therapeutic hypothermia on primary human cortical astrocytes cultured under hypoxia. Front. Cell. Neurosci. 11:386. doi: 10.3389/fncel.2017.00386
Shannon, P., Markiel, A., Ozier, O., Baliga, N. S., Wang, J. T., Ramage, D., et al. (2003). Cytoscape: a software environment for integrated models of biomolecular interaction networks. Genome Res. 13, 2498–2504. doi: 10.1101/gr.1239303
Sharma, P., Bansal, A., and Sharma, P. C. (2015). RNA-seq-based transcriptome profiling reveals differential gene expression in the lungs of Sprague–Dawley rats during early-phase acute hypobaric hypoxia. Mol. Genet. Genomics 290, 2225–2240. doi: 10.1007/s00438-015-1064-1060
Simonson, T. S., Yang, Y., Huff, C. D., Yun, H., Qin, G., Witherspoon, D. J., et al. (2010). Genetic evidence for high-altitude adaptation in Tibet. Science 329, 72–75. doi: 10.1126/science.1189406
Stembridge, M., Williams, A. M., Gasho, C., Dawkins, T. G., Drane, A., Villafuerte, F. C., et al. (2019). The overlooked significance of plasma volume for successful adaptation to high altitude in Sherpa and Andean natives. Proc. Natl. Acad. Sci. U S A. 116, 16177–16179. doi: 10.1073/pnas.1909002116
Taylor, C. T., and Colgan, S. P. (2017). Regulation of immunity and inflammation by hypoxia in immunological niches. Nat. Rev. Immunol. 17, 774–785. doi: 10.1038/nri.2017.103
Vegfors, J., Ekman, A.-K., Stoll, S. W., Bivik Eding, C., and Enerbäck, C. (2016). Psoriasin (S100A7) promotes stress-induced angiogenesis. Br. J. Dermatol. 175, 1263–1273. doi: 10.1111/bjd.14718
Warde-Farley, D., Donaldson, S. L., Comes, O., Zuberi, K., Badrawi, R., Chao, P., et al. (2010). The GeneMANIA prediction server: biological network integration for gene prioritization and predicting gene function. Nucleic Acids Res. 38, W214–W220. doi: 10.1093/nar/gkq537
World Health Organization (2012). International Travel and Health 2012: Situation as on 1 January 2012. Geneva: World Health Organization.
Wu, T. (2001). The Qinghai–Tibetan Plateau: how high do Tibetans live? High Alt. Med. Biol. 2, 489–499. doi: 10.1089/152702901753397054
Xu, J., Yang, Y. Z., Tang, F., Ga, Q., Tana, W., and Ge, R. L. (2015). EPAS1 gene polymorphisms are associated with high altitude polycythemia in Tibetans at the Qinghai-Tibetan Plateau. Wilderness Environ. Med. 26, 288–294. doi: 10.1016/j.wem.2015.01.002
Xu, Z., Jia, Z., Shi, J., Zhang, Z., Gao, X., Jia, Q., et al. (2019). Transcriptional profiling in the livers of rats after hypobaric hypoxia exposure. PeerJ 2019:e6499. doi: 10.7717/peerj.6499
Yasukochi, Y., Nishimura, T., Motoi, M., and Watanuki, S. (2018). Association of EGLN1 genetic polymorphisms with SpO2 responses to acute hypobaric hypoxia in a Japanese cohort. J. Physiol. Anthropol. 37:9. doi: 10.1186/s40101-018-0169-167
Keywords: acclimatization, differentially expressed gene, hypobaric hypoxia, RNA-seq, saliva, transcriptome
Citation: Yasukochi Y, Shin S, Wakabayashi H and Maeda T (2020) Transcriptomic Changes in Young Japanese Males After Exposure to Acute Hypobaric Hypoxia. Front. Genet. 11:559074. doi: 10.3389/fgene.2020.559074
Received: 05 May 2020; Accepted: 14 August 2020;
Published: 08 September 2020.
Edited by:
Raphael Faiss, University of Lausanne, SwitzerlandReviewed by:
Martin Burtscher, University of Innsbruck, AustriaNobukazu Kasai, Japan Institute of Sports Sciences (JISS), Japan
Copyright © 2020 Yasukochi, Shin, Wakabayashi and Maeda. This is an open-access article distributed under the terms of the Creative Commons Attribution License (CC BY). The use, distribution or reproduction in other forums is permitted, provided the original author(s) and the copyright owner(s) are credited and that the original publication in this journal is cited, in accordance with accepted academic practice. No use, distribution or reproduction is permitted which does not comply with these terms.
*Correspondence: Yoshiki Yasukochi, hyasukou@proof.ocn.ne.jp