- Department of Neurology, Tianjin First Central Hospital, Tianjin, China
Although dramatic progress has been achieved in the understanding and treatment of multiple sclerosis (MS) and ischemic stroke (IS), more precise and instructive support is required for further research. Recent large-scale genome-wide association studies (GWASs) have already revealed risk variants for IS and MS, but the common genetic etiology between MS and IS remains an unresolved issue. This research was designed to overlapping genes between MS and IS and unmask their transcriptional features. We designed a three-section analysis process. Firstly, we computed gene-based analyses of MS GWAS and IS GWAS data sets by VGEAS2. Secondly, overlapping genes of significance were identified in a meta-analysis using the Fisher’s procedure. Finally, we performed gene expression analyses to confirm transcriptional changes. We identified 24 shared genes with Bonferroni correction (Pcombined < 2.31E-04), and five (FOXP1, CAMK2G, CLEC2D, LBH, and SLC2A4RG) had significant expression differences in MS and IS gene expression omnibus data sets. These meaningful shared genes between IS and MS shed light on the underlying genetic etiologies shared by the diseases. Our results provide a basis for in-depth genomic studies of associations between MS and IS.
Introduction
Multiple sclerosis and IS are two major neurological diseases with serious sequelae such as motor and/or sensory disabilities, cognitive impairment, and mental disorders (Compston and Coles, 2008; Johnson et al., 2016). Researchers are increasingly interested in the genetics of complex human diseases associated with high personal, family, and social burden, including MS and IS. Clarifying the genetics of these conditions can create a “strategic hub” for further studies to prioritize targeted treatments.
Multiple sclerosis is a chronic autoimmune disease in the CNS mainly characterized by demyelination in brain and spinal cord (Compston and Coles, 2008). The etiology of MS is heterogeneous, and both the environment and genetics are influential components (Dendrou et al., 2015). As environmental variants are complicated, the genetic variants are regarded as a possible direction to make progress in immune-related mechanisms and therapies (Belbasis et al., 2015; Dendrou et al., 2015). Numerous large-scale GWASs revealed more than 200 susceptible loci of MS, such as HLA, forkhead box protein P1 (FOXP1), IQCB1, SLC9A9, and CD226 (Liu et al., 2017a; International Multiple Sclerosis Genetics Consortium, 2019). The pathway-based analysis results of MS risk variants were mostly enriched in immune- and/or inflammation-related processes (International Multiple Sclerosis Genetics Consortium, 2013; Liu et al., 2017b, c). Decades of progress in genetics have shed light on MS mechanisms and therapies. Several US Food and Drug Administration (FDA)-approved drugs have been used in patients with MS, such as ocrelizumab, daclizumab, and mitoxantrone (Baecher-Allan et al., 2018).
Ischemic stroke accounts for 70% to 85% of stroke cases, which is the most common cause of death and long-term disability worldwide (Khan et al., 2013; Johnson et al., 2016). Many pharmacologic therapies have been used to reduce damage and improve the prognosis of IS in preclinical and clinical trials, including thrombolytic, antithrombotic, antioxidation, and neuroprotective agents (Gladstone et al., 2002; Yu et al., 2005; Chamorro et al., 2016; Wechsler et al., 2018). To date, intravenous alteplase is the primary FDA-approved drug for IS. However, thrombolysis benefits a limited number of patients with IS because of the limited “therapeutic time window” and the unpredictable outcomes of recanalization. More attention should be paid to develop adjuvant treatments for IS that target inflammation and oxidative stress (Chamorro et al., 2016). Recent GWASs have uncovered ∼35 genetic risk regions for IS and hemorrhagic stroke (Dichgans et al., 2019). Early GWASs of IS identified and replicated the significant relationship for IS at ABO, cardioembolic stroke near PITX2 and ZFHX3, and for large-vessel stroke at HDAC9 and the 9p21 locus (Bellenguez et al., 2012; Traylor et al., 2012; Malik et al., 2016). Malik et al. (2018) implemented a comprehensive multiancestry GWAS using ancestral meta-analyses of 67,162 IS cases and 454,450 healthy controls. Twenty-two new loci were discovered and used for further pathway and drug–target analyses (Malik et al., 2018). Torres-Aguila et al. (2019) found that 14q24.3 locus was associated with leukocyte counts during the first 24 h after IS. Genetic and related functional studies are considered one of the breakthrough points for IS therapy (Dichgans et al., 2019). So far, because of the limitation of known GWAS data, the common gene variant that had been proved both MS and IS risk gene was SLC44A2 (P-MS = 3.5E-09, P-IS = 4.72E-08) (International Multiple Sclerosis Genetics Consortium et al., 2013; Malik et al., 2018).
Clinical studies revealed an increased prevalence of IS in MS patients compared with controls (Marrie et al., 2015; Hong et al., 2019). Tseng et al. (2015) enrolled 1,174 Chinese MS patients and 4,696 matched healthy Chinese controls. After following up for 5 years, they found that the MS group got a higher risk of stroke [hazard ratio (HR) = 12.1 for the first year; HR = 4.69 for the rest years] than the control cohort. As more immunological characteristics of human complex diseases such as MS have been uncovered, the neuroinflammatory mechanisms of IS should have deeper exploration (Fu et al., 2015). For example, the natural killer (NK) cells aggravated the infarct area after IS, but their influence on MS was proven to be dependent on the course of disease (Chanvillard et al., 2013; Gan et al., 2014). Based on preclinical studies, more immunomodulatory drugs have been administered in IS clinical translation experiments including natalizumab (Elkins et al., 2017) and fingolimod (Zhu et al., 2015; Tian et al., 2018), which are the FDA-approved agents for MS.
Here, we focus on the commonality genetic etiologies between MS and IS. First, we conducted gene-based testing of MS and IS GWAS data sets. Next, a meta-analysis was conducted to sort the shared significant genes out. Finally, we implemented differential expression analyses for shared significant genes via four gene expression omnibus (GEO) data sets.
Materials and Methods
GWAS Data Set
The large-scale MS GWAS data set consisted of 9,772 MS cases from the IMSGC and 17,376 controls from the WTCCC2. And cases were gathered by 23 teams operating in 15 countries. All individuals were self-reported as being of European descent. The original research conducted sample and single-nucleotide polymorphism (SNP) QC to create SNP metric strainers. In sample QC, Bayesian clustering and principal components analysis were mainly used to identify qualified samples. Researchers developed and utilized two novel methods (automated cluster checking and beta-binomial model) in SNP QC to exclude SNPs identified because of incorrect genotype calling. Finally, 464,357 autosomal SNPs from the entire data set were obtained for further analysis (International Multiple Sclerosis Genetics Consortium et al., 2011).
The IS data set was summarized from the METASTROKE collaboration’s discovery stage. In this stage, genotyped data from 12 case–control GWASs correspond to 10,307 IS cases and 19,326 controls (ASGC: 1,162 IS cases and 1,244 controls; BRAINS: 371 IS cases and 2,640 controls; GASROS_affy: 485 IS cases and 3,030 controls; GASROS_illumina: 296 IS cases and 377 controls; GEOS: 448 IS cases and 498 controls; HPS: 588 IS cases and 571 controls; ISGS-SWISS: 1,014 IS cases and 1,370 controls; MILANO: 366 IS cases and 407 controls; VISP: 1,723 IS cases and 1,047 controls; WHI: 306 IS cases and 2,170 controls; WTCCC2-D: 1,174 IS cases and 797 controls; WTCCC2-UK: 2,374 IS cases and 5,175 controls; all individuals in the discovery stage were Caucasian). After QC by logistic regression analysis and meta-analysis, 9,541,572 SNPs were available for gene-based testing (Malik et al., 2016).
Gene-Based Test of MS and IS GWAS Using VEGAS
We utilized the VEGAS2 to execute more elastic gene-based testing for MS and IS GWAS. The chief distinguishing feature of VEGAS2 is that this approach is computationally feasible and can be applied to any GWAS experimental design by using the summary GWAS data (Mishra and Macgregor, 2015). After uploading the SNP information (rs-number and P value of both GWASs) to VEGAS2, we selected 1000G EUROPEAN as the population reference group. For considering both the physical position and LD profiles of SNPs with others in gene-based testing, we chose “0kbldbin outside gene and SNPs in LD r2 > 0.8” (Christoforou et al., 2012). For each gene definition, P values of SNPs were first changed to upper tail χ2 statistics with one degree of freedom (df) in the gene definition. And then, if SNPs are in linkage equilibrium, the statistics of gene-based tests would have a χ2 distribution with n df under the null hypothesis. We used the reference population group of 1000 Genomes European and the model of Σ (n × n matrix of LD [r] values) for SNP correlation, because LD for the n SNPs occurs frequently. Significance was tested with reference to the aggregate χ2 statistics for each gene to simulated repetition from a multivariate normal distribution in which mean = 0 and variance = Σ. The empirical P value of every gene was calculated via the formula, P = r + 1/m + 1, where r was the number of cases where the simulation statistics surpassed the observed statistics, and m was the number of simulations (Mishra and Macgregor, 2015). In this gene-based testing, we screened the shared genes with nominal significance respectively (P-MS < 0.05, P-IS < 0.05).
Meta-Analysis of MS and IS GWAS
To combine P values calculated by VEGAS2 for MS and IS GWAS, we used Fisher’s method to perform meta-analysis for every overlapped gene. For a given gene, the formula for the statistic is as follows:
For the ith study, Pi is the P value of the genes, and k is the entire count of studies. x2 abides by a c2 distribution with 2k degrees of freedom (Begum et al., 2012). The gene-based meta-analysis was performed with R software.1
Following the meta-analysis, we performed Bonferroni correction to control type I error. The combined P value was less than 0.05/2n, where n was the number of shared genes with previous nominal P value (P < 0.05).
MS and IS Case–Control Gene Expression Analysis
We further investigated the differential expression of shared genes in the MS and IS patients and healthy control subjects using gene expression data sets from the NCBI GEO database.2
Immune cells from whole blood are the typical samples for gene expression analysis in patients with MS. PBMCs from 12 MS female patients under Poser’s criteria and 15 unrelated female controls were isolated from whole blood. The extracted samples were tested on the Affymetrix Gene Chip Human Genome U133 Plus 2.0 Array (Kemppinen et al., 2011). The expression data were obtained from GEO series GSE21942. In GEO series GSE43591, samples were extracted from 10 relapsing–remitting MS patients diagnosed in accordance with the criteria of McDonald et al. (2001) and 10 age- and sex-matched controls. T cells were purified through CD14+ removal sorting and CD3+-positive selection from PBMCs. Transcriptional data were examined with Human Genome HG-U133 plus 2.0 arrays (Jernas et al., 2013).
Ischemic stroke case–control expression analyses were performed to identify differentially expressed genes in the GEO series. GEO series GSE16561 provided the raw mRNA expression data from peripheral whole blood of a study of 39 IS patients and 24 healthy controls (O’Connell et al., 2017). More information about the inclusion and exclusion criteria was provided in the original study (O’Connell et al., 2017). RNA data from the blood of 23 control samples and 69 cardioembolic stroke samples were analyzed in GEO series GSE58294. Cardioembolic stroke is a subtype of IS and has unique genetic traits (Stamova et al., 2014). In the original research, 69 cardioembolic stroke samples were collected from 23 patients at three time points to monitor transcriptional changes in the first 24 h. Twenty-three subjects without symptomatic vascular disease history were recruited as VRFCs (Stamova et al., 2014). And we used all the 69 cardioembolic stroke samples as a whole (including the samples of three time points) to compare with the samples from VRFCs.
To calculate differential expression between case–control samples, we utilized GEO2R and performed GEO query and limma R packages (Barrett et al., 2013). Among four differential expression data sets, those with the smallest P value for each shared gene in MS and IS were selected for further analysis.
Results
Gene-Based Test for MS and IS With VEGAS2
We uploaded the 464,357 MS and 9,541,572 IS SNP rs IDs and associated P values for gene-based analysis. We sorted 1,353 genes with P-MS < 0.05 from a total of 14,811 MS gene sets obtained by VEGAS2. After Bonferroni correction (P < 0.05/14,811 = 3.38E-06), 47 non-major histocompatibility complex (MHC) and MHC risk variants were sorted including known risk genes such as AHI1, RGS1, SP140, IQCB, IL2RA, TNFRSF1A, CLEC16A, GALC, TNFSF14, and CYP24A1. The good applicability of VEGAS2 for gene-based test was proven by the well-replicated genes above. Information of the gene-wide significant genes of MS is shown in Supplementary Table S1. In IS data sets, 1,290 genes with P-IS < 0.05 were screened out from 21,913 IS genes via VEGAS2. However, no IS variant achieved the strict threshold (P-IS < 0.05/21,913 = 2.28E-06). And we utilized a looser significance threshold (P < 1.00E-04) and identified two genes that satisfied this threshold set: ZYX (P < 2.60E-05) and NCR3LG1 (P < 8.10E-05).
After making an intersection between 1,353 MS genes and 1,290 IS genes (P-MS < 0.05, P-IS < 0.05), we obtained 108 nominal shared genes. We performed meta-analysis for the 108 shared genes and obtained the P–combined for each gene. And then 24 overlapped significant genes were identified with P–combined < 2.31E-04 (0.05/2∗108), including LBH at 2p23.1, STAT4 at 2q32.2-q32.3, FOXP1 at 3p13, TEC at 4p12-p11, MICB/NFKBIL1 at 6p21.33, IL6 at 7p15.3, CAMK2G at 10q22.2, B4GALNT1 at 12q13.3, BRAP at 12q24.12, CLEC2D/CLECL1 at 12p13.31, HECTD4/NAA25 at 12q24.13, OS9 at 12q13.3-q14.1, NUDT14 at 14q32.33, PDE4C at 19p13.11, SLC44A2 at 19p13.2, and LIME1/RTEL1/RTEL1-TNFRSF6B/SLC2A4RG/ZBTB46/ZGPAT at 20q13.33. The detailed results are listed in Table 1.
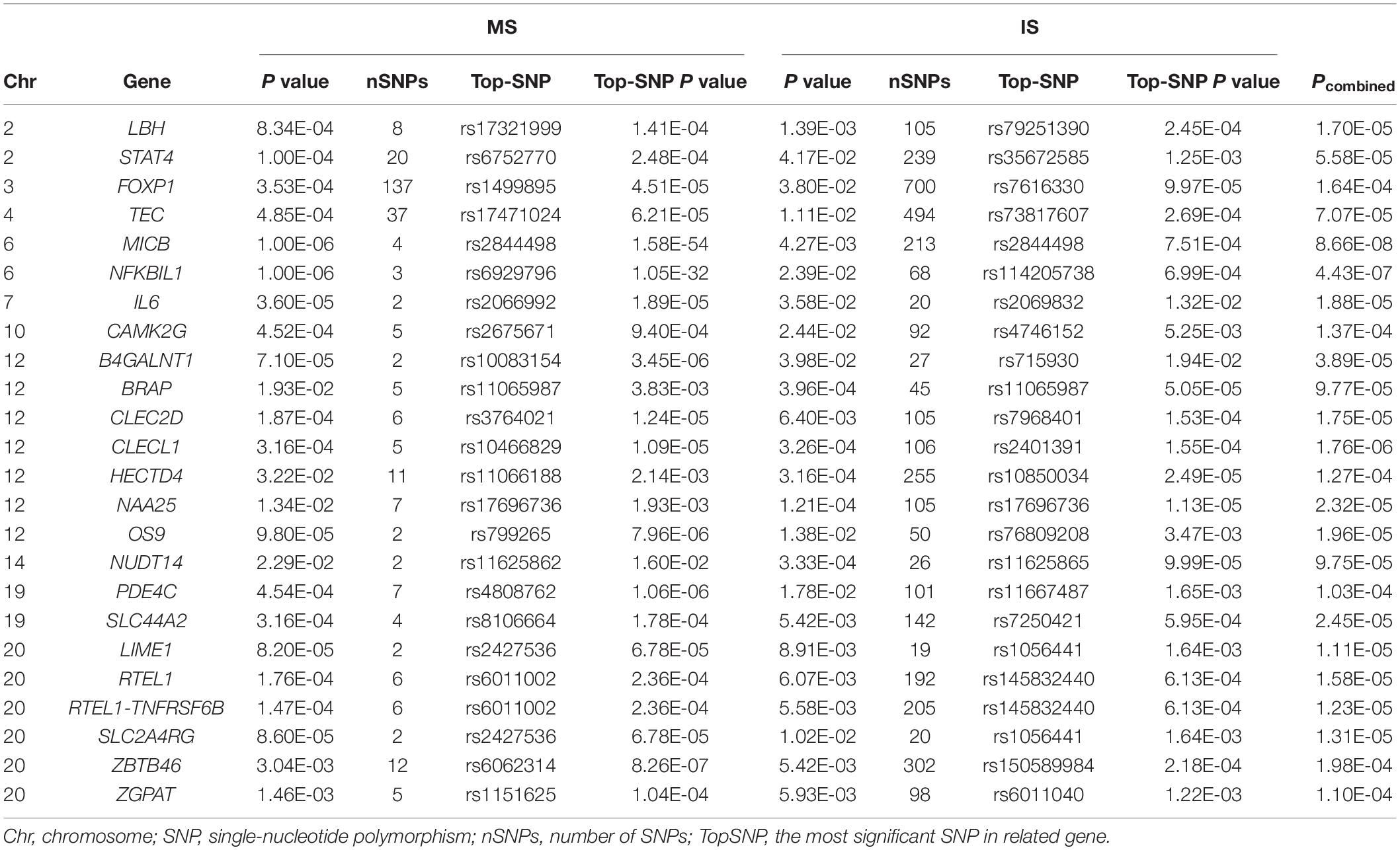
Table 1. The overlapped significant gene sets screened by VEGAS of multiple sclerosis (MS) and ischemic stroke (IS).
Differential Expression Analyses of Overlapped Genes
We analyzed and integrated GEO profiles of MS and IS to investigate the differential expression levels of shared genes. Notably, 16 of 24 common genes were determined to have significant changes in at least one of the four GEO data sets with P < 2.08E-03 (0.05/24) (Table 2).
In MS expression profiles, the transcriptional levels of CAMK2G (P = 4.15E-04), CLEC2D (P = 9.11E-04), FOXP1 (P = 2.35E-09), and NUDT14 (P = 1.51E-04) in the GEO data set GSE21942 (Supplementary Table S2) and CAMK2G (P = 3.09E-06), LBH (P = 5.60E-04), SLC2A4RG (P = 1.29E-03), and ZGPAT (P = 6.93E-04) in MS patients were markedly changed compared to controls (Supplementary Table S3). In samples from peripheral whole blood, CLEC2D (P = 2.68E-07), LBH (P = 5.45E-06), LIME1 (P = 2.33E-07), OS9 (P = 1.41E-04), SLC2A4RG (P = 5.07E-05), and SLC44A2 (P = 4.73E-04) in the acute IS case–control set (GSE16561) (Supplementary Table S4) and BRAP (P = 1.71E-20), CAMK2G (P = 5.14E-07), CLECL1 (P = 6.30E-06), FOXP1 (P = 1.55E-05), HECTD4 (P = 8.83E-05), LIME1 (P = 1.16E-09), MICB (P = 2.45E-04), NAA25 (P = 3.92E-08), NFKBIL1 (P = 3.33E-06), OS9 (P = 1.24E-12), and SLC44A2 (P = 1.27E-03) in the cardioembolic stroke case–control set (GSE58294) (Supplementary Table S5) showed significantly different expression.
It was remarkable that there were five overlapped genes with a significant expressed difference in at least one data set of each disease GEO data sets (P < 2.08E-03) (Table 3). The expression difference of every individual gene presented the same tendency between two GEO data sets in MS, and CAMK2G was also upregulated in IS GEO data sets, whereas three of five genes (CLEC2D, LBH, and SLC2A4RG) had opposite alterations in GSE16561 and GSE58294, which might be due to the heterogeneity of IS and its subtypes. FOXP1 expression in patients was remarkably increased in every data set compared to controls [GSE21942: log fold change (FC) = 1.21, GSE43591: logFC = 0.528, GSE16561: logFC = 0.15, and GSE58294: logFC = 0.34].
Discussion
With the development of high-throughput technology, more risk loci of MS and IS have been gradually identified (International Multiple Sclerosis Genetics Consortium et al., 2013; Moutsianas et al., 2015; Malik et al., 2018). However, genes shared by the two diseases remain elusive. In this study, we implemented a three-part process to assess GWAS data sets. In Part 1, we conducted independent gene-based association analyses using two GWAS data sets and VEGAS2. In Part 2, we performed a Fisher’s meta-analysis of nominally significant common genes (P-MS < 0.05, P-IS < 0.05), and 24 shared genes satisfied the strict threshold P value (P < 2.31E-04). In Part 3, we listed expression information of shared genes in four GEO data sets and found that FOXP1, CAMK2G, CLEC2D, LBH, and SLC2A4RG presented significant differential expression in both MS and IS case–control profiles.
FOXP1 Located at 3p13
Forkhead box protein P1 protein is a member of the forkhead box (FOX) family. The forkhead TF plays vital roles in diverse cell and tissue processes including development, aging, metabolism, and cancer (Coffer and Burgering, 2004; Wijchers et al., 2006). FOXP1 is also critical in the development and function of immune cells. In peritonitis models, Shi et al. (2008) illustrated that FOXP1 upregulation blunted monocyte development and macrophage biological activities by inhibiting the production of c-Fms/macrophage colony-stimulating factor receptor. Via Smad2/Smad3 and transforming growth factor β (TGF-β) signaling, increased FOXP1 expression restrained CD8+ T cells from proliferation and activation in cancer (Stephen et al., 2014). Combined with FOXP3, FOXP1 was demonstrated to play an essential role in maintaining expression of CD25, SATB1, and CTLA-4 and responsiveness to interleukin 2 in regulatory T cells (Konopacki et al., 2019). FOXP1 was also reported as a crucial negative transcriptional modulator in the differentiation of CD4+ follicular helper T cells (Wang et al., 2014). Similarly, FOXP1 is a fundamental TF in the early development of B cells (Hu et al., 2006), and abnormal FOXP1 upregulation leads to a reduction and irregular distribution of B cells in germinal centers (Sagardoy et al., 2013). FOXP1 is associated with both CNS development and abnormalities. Braccioli et al. (2017) reported that FOXP1 can adjust neural stem cells (NSCs) neurogenesis via Notch signaling and foster the differentiation of embryonic NSCs to neurons and astrocytes in vitro. Li et al. (2015) demonstrated that FOXP1 has vital influences on modulating neuronal migration and morphogenesis in cortical regions. FOXP1 mutations reportedly contribute to nervous system disorders including Huntington disease (Tang et al., 2012), autism (O’Roak et al., 2011; Chien et al., 2013), and epilepsy (Jay et al., 2019).
In this study, FOXP1 exhibited an elevated tendency of expression in both MS and IS patients compared to controls (GSE21942: logFC = 1.21; GSE43591: logFC = 0.528; GSE16561: logFC = 0.15, and GSE58294: logFC = 0.34). FOXP1 was significantly more than twofold upregulated in the PBMC of MS (P = 2.35E-09, logFC = 1.21) and significantly 1.3-fold upregulated in the blood cells of cardioembolic stroke (p = 1.55E-05, logFC = 0.34). The IMSGC assessed two sets of GWAS data and identified rs9828629/FOXP1 (P = 1.9E-10, odds ratio [OR] = 1.08) as an immune-related risk variant linked to MS (International Multiple Sclerosis Genetics Consortium et al., 2013). In research on atherosclerosis, Bot et al. (2011) illustrated that FOXP1 was expressed in diverse cell types and was related with stable plaques through the TGF-β pathway. As atherosclerosis is one cause of large vessel disease, a major subtype of IS, there may be more associations between FOXP1 and IS. In summary, FOXP1 could be a potential target in terms of its multiple functions in the immune and nervous systems.
CAMK2G Located at 10q22.2
CAMK2G encodes CaMKIIγ, an isoform of calcium (Ca2+)/calmodulin-dependent protein kinase II (CaMKII) that participates in Ca2+-related biological activities (Hudmon et al., 2001; Rokita and Anderson, 2012). In a model of acute ischemia/reperfusion, CAMK2G/CaMKIIγ improved neuronal survival by activating nuclear factor κB signaling (Ye et al., 2019). CAMK2G was identified as an enhancer gene for coronary artery disease in a GWAS meta-analysis (Gong et al., 2018). CAMKIIγ was also a key modulator to repress macrophage phagocytosis and enhance the necrosis of atherosclerotic plaques (Doran et al., 2017). We found that CAMK2G (P = 5.14E-07) was 1.3-fold upregulated in cardioembolic stroke compared to controls. In addition, CAMK2G could act through classical inflammatory pathways such as mTORC1 and STAT3, both of which play vital roles in MS (Ma et al., 2017; Meng et al., 2017).
CLEC2D Located at 12p13.31
CLEC2D encodes lectin-like transcript 1 (LLT1) and is located next to the NK gene complex (Yokoyama and Plougastel, 2003; Germain et al., 2010). LLT1 was identified as a negative ligand for NKR-P1A (CD161) receptor in humans (Rosen et al., 2005). The interaction of CD161 on NK cells with matched LLT1 can reportedly repress NK cell-mediated cytotoxicity (Aldemir et al., 2005). Llibre et al. (2016) illustrated that LLT1/CD161 interaction influences B-cell development in human germinal centers. Toll-like receptor (TLR)-activated dendritic cells and TLR or B-cell receptor-activated B cells express LLT1 (Rosen et al., 2008). LLT1 is also expressed by monocyte/macrophage in RA joints, and soluble LLT1 is elevated in patient serum (Chalan et al., 2015). Here, we detected that the expression of CLEC2D (P = 9.11E-04, logFC = 0.49) was significantly altered in PBMCs from MS patients. However, the expression of CLEC2D in IS cases was downregulated by 0.62-fold compared to controls. The distinct alterations of CLEC2D expression in MS and IS need further study.
SLC2A4RG Located at 20q13.33
SLC2A4 regulator encoded by SLC2A4RG was identified as TF binding to the SLC2A4 promoter district where it regulates expression (Oshel et al., 2000; Knight et al., 2003). In a study of Huntington disease, SLC2A4RG was found to cooperate with a key cis-element and shuttle to and from the nucleus (Tanaka et al., 2004). A recent large GWAS demonstrated that rs2256814/SLC2A4RG (P = 3.5E-9, OR = 1.08) was a novel MS susceptibility gene with immune function (International Multiple Sclerosis Genetics Consortium et al., 2013). Furthermore, Dhaouadi et al. (2014) stated that SLC2A4RG could slightly induce TGFB1 expression in atherosclerosis. Notably, SLC2A4RG showed differential expression in MS and IS GEO data sets (GSE43591, P = 1.29E-03; GSE16561, P = 5.07E-05).
LBH Located at 2p23.1
Limb-bud and heart (LBH) encodes a transcription modulator that regulates cell development in multiple tissues (Ai et al., 2008; Al-Ali et al., 2010; Ekwall et al., 2015). LBH and related variants are reportedly associated with autoimmune diseases such as SLE, RA, and celiac disease (Zhernakova et al., 2011; Yu et al., 2013; Chang et al., 2016). Wnt signaling regulates the expression of LBH in tumors and the epithelium (Rieger et al., 2010). In the CNS, the Wnt pathway is related with male-specific genes of IS and positively impacts the regenerative process after injury (Tian et al., 2012; Lambert et al., 2016). Researchers integrated Alzheimer’s disease-GWAS data and identified LBH as a pathogenic gene of amyloid β accumulation that is tightly linked to immune system (Mhatre et al., 2015; Yamaguchi-Kabata et al., 2018). In our study, LBH was significantly downregulated both in T cells from PBMCs of patients with MS (P = 5.60E-04, logFC = −0.55) and blood cells from patients with IS (P = 5.45E-06, logFC = −0.55). The potential immunomodulatory function of LBH can assist us in uncovering common mechanisms between MS and IS.
Although we conducted powerful gene-based tests that are effective extensions of traditional GWASs to discover shared genes, our study has several limitations. First, subthreshold variants will undermine the significance of the causal SNPs in the same gene region. Next, IS consists of several subtypes with genetic heterogeneity. More refined data of IS subtypes should be excavated for further analyses. Furthermore, the single sample type and limited case numbers are two major disadvantages of our GEO data. All GEO sample sources are from peripheral blood cells because they are safe and accessible in clinical settings. If possible, we will integrate brain-derived data that can directly reflect the differential expression of genes in neurological diseases.
Conclusion
In summary, we performed flexible gene-based analysis to discover significant shared genes between MS and IS and analyzed their differential expression. These genes mainly participate in cell development and immune response, and both are associated with MS and IS. Our research reveals shared genetic etiologies between MS and IS and indicates new directions for future studies examining mechanisms and new therapeutic options.
Data Availability Statement
All datasets analyzed for this study are included in the article and the Supplementary Files.
Author Contributions
ZT and ZW conceived and designed the study for MS and IS. ZT administered the analyses and wrote the manuscript. YS and ZG was responsible for manuscript revision. YY and JG provided analyses support. All authors contributed to the article and approved the submitted version.
Funding
This work was supported by the fund from the Tianjin Health Bureau (2015KZ035), the National Natural Science Foundation Youth Fund of China (31300923), and In-hospital Science and Technology Fund of Tianjin First Central Hospital.
Conflict of Interest
The authors declare that the research was conducted in the absence of any commercial or financial relationships that could be construed as a potential conflict of interest.
Supplementary Material
The Supplementary Material for this article can be found online at: https://www.frontiersin.org/articles/10.3389/fgene.2020.00646/full#supplementary-material
TABLE S1 | MS-associated genes with gene-wide significance.
TABLE S2 | The expression data of shares gene sets from peripheral blood mononuclear cells in multiple sclerosis patients and controls (Series GSE21942).
TABLE S3 | The expression data of shares gene sets from peripheral blood T-cells in multiple sclerosis patients and controls (Series GSE43591).
TABLE S4 | The expression data of shares gene sets from whole blood RNA in ischemic stroke patients and controls (Series GSE16561).
TABLE S5 | The expression data of shares gene sets from whole blood RNA in cardioembolic stroke patients and controls (Series GSE58294).
Abbreviations
CNS, central nervous system; GEO, gene expression omnibus; GWASs, large-scale genome-wide association studies; IMSGC, International MS Genetics Consortium; IS, ischemic stroke; LD, linkage disequilibrium; MS, multiple sclerosis; PBMCs, peripheral blood mononuclear cells; QC, quality control; RA, rheumatoid arthritis; SLE, systemic lupus erythematosus; SNP, single nucleotide polymorphism; TF, transcription factor; VEGAS2, Versatile Gene-based Association Study-2 version 2; VRFCs, vascular risk factor controls; WTCCC2, Wellcome Trust Case Control Consortium 2.
Footnotes
References
Ai, J., Wang, Y., Tan, K., Deng, Y., Luo, N., Yuan, W., et al. (2008). A human homolog of mouse Lbh gene, hLBH, expresses in heart and activates SRE and AP-1 mediated MAPK signaling pathway. Mol. Biol. Rep. 35, 179–187. doi: 10.1007/s11033-007-9068-4
Al-Ali, H., Rieger, M. E., Seldeen, K. L., Harris, T. K., Farooq, A., and Briegel, K. J. (2010). Biophysical characterization reveals structural disorder in the developmental transcriptional regulator LBH. Biochem. Biophys. Res. Commun. 391, 1104–1109. doi: 10.1016/j.bbrc.2009.12.032
Aldemir, H., Prod’homme, V., Dumaurier, M. J., Retiere, C., Poupon, G., Cazareth, J., et al. (2005). Cutting edge: lectin-like transcript 1 is a ligand for the CD161 receptor. J. Immunol. 175, 7791–7795. doi: 10.4049/jimmunol.175.12.7791
Baecher-Allan, C., Kaskow, B. J., and Weiner, H. L. (2018). Multiple sclerosis: mechanisms and immunotherapy. Neuron 97, 742–768. doi: 10.1016/j.neuron.2018.01.021
Barrett, T., Wilhite, S. E., Ledoux, P., Evangelista, C., Kim, I. F., Tomashevsky, M., et al. (2013). NCBI GEO: archive for functional genomics data sets–update. Nucleic Acids Res. 41, D991–D995. doi: 10.1093/nar/gks1193
Begum, F., Ghosh, D., Tseng, G. C., and Feingold, E. (2012). Comprehensive literature review and statistical considerations for GWAS meta-analysis. Nucleic Acids Res. 40, 3777–3784. doi: 10.1093/nar/gkr1255
Belbasis, L., Bellou, V., Evangelou, E., Ioannidis, J. P., and Tzoulaki, I. (2015). Environmental risk factors and multiple sclerosis: an umbrella review of systematic reviews and meta-analyses. Lancet Neurol. 14, 263–273. doi: 10.1016/s1474-4422(14)70267-4
Bellenguez, C., Bevan, S., Gschwendtner, A., Spencer, C. C., Burgess, A. I., Pirinen, M., et al. (2012). Genome-wide association study identifies a variant in HDAC9 associated with large vessel ischemic stroke. Nat. Genet. 44, 328–333. doi: 10.1038/ng.1081
Bot, P. T., Grundmann, S., Goumans, M. J., de Kleijn, D., Moll, F., de Boer, O., et al. (2011). Forkhead box protein P1 as a downstream target of transforming growth factor-beta induces collagen synthesis and correlates with a more stable plaque phenotype. Atherosclerosis 218, 33–43. doi: 10.1016/j.atherosclerosis.2011.05.017
Braccioli, L., Vervoort, S. J., Adolfs, Y., Heijnen, C. J., Basak, O., Pasterkamp, R. J., et al. (2017). FOXP1 promotes embryonic neural stem cell differentiation by repressing Jagged1 expression. Stem Cell Rep. 9, 1530–1545. doi: 10.1016/j.stemcr.2017.10.012
Chalan, P., Bijzet, J., Huitema, M. G., Kroesen, B. J., Brouwer, E., and Boots, A. M. (2015). Expression of lectin-like transcript 1, the ligand for CD161, in rheumatoid arthritis. PLoS One 10:e0132436. doi: 10.1371/journal.pone.0132436
Chamorro, A., Dirnagl, U., Urra, X., and Planas, A. M. (2016). Neuroprotection in acute stroke: targeting excitotoxicity, oxidative and nitrosative stress, and inflammation. Lancet Neurol. 15, 869–881. doi: 10.1016/s1474-4422(16)00114-9
Chang, Y., Sheng, Y., Cheng, Y., Lin, Y., Zhu, Z., Wen, L., et al. (2016). Downregulated expression of LBH mRNA in peripheral blood mononuclear cells from patients with systemic lupus erythematosus. J. Dermatol. 43, 99–102. doi: 10.1111/1346-8138.13006
Chanvillard, C., Jacolik, R. F., Infante-Duarte, C., and Nayak, R. C. (2013). The role of natural killer cells in multiple sclerosis and their therapeutic implications. Front. Immunol. 4:63. doi: 10.3389/fimmu.2013.00063
Chien, W. H., Gau, S. S., Chen, C. H., Tsai, W. C., Wu, Y. Y., Chen, P. H., et al. (2013). Increased gene expression of FOXP1 in patients with autism spectrum disorders. Mol. Autism 4:23. doi: 10.1186/2040-2392-4-23
Christoforou, A., Dondrup, M., Mattingsdal, M., Mattheisen, M., Giddaluru, S., Nothen, M. M., et al. (2012). Linkage-disequilibrium-based binning affects the interpretation of GWASs. Am. J. Hum. Genet. 90, 727–733. doi: 10.1016/j.ajhg.2012.02.025
Coffer, P. J., and Burgering, B. M. (2004). Forkhead-box transcription factors and their role in the immune system. Nat. Rev. Immunol. 4, 889–899. doi: 10.1038/nri1488
Dendrou, C. A., Fugger, L., and Friese, M. A. (2015). Immunopathology of multiple sclerosis. Nat. Rev. Immunol. 15, 545–558. doi: 10.1038/nri3871
Dhaouadi, N., Li, J. Y., Feugier, P., Gustin, M. P., Dab, H., Kacem, K., et al. (2014). Computational identification of potential transcriptional regulators of TGF-ss1 in human atherosclerotic arteries. Genomics 103, 357–370. doi: 10.1016/j.ygeno.2014.05.001
Dichgans, M., Pulit, S. L., and Rosand, J. (2019). Stroke genetics: discovery, biology, and clinical applications. Lancet Neurol. 18, 587–599. doi: 10.1016/s1474-4422(19)30043-2
Doran, A. C., Ozcan, L., Cai, B., Zheng, Z., Fredman, G., Rymond, C. C., et al. (2017). CAMKIIgamma suppresses an efferocytosis pathway in macrophages and promotes atherosclerotic plaque necrosis. J. Clin. Invest. 127, 4075–4089. doi: 10.1172/jci94735
Ekwall, A. K., Whitaker, J. W., Hammaker, D., Bugbee, W. D., Wang, W., and Firestein, G. S. (2015). The rheumatoid arthritis risk gene LBH regulates growth in fibroblast-like synoviocytes. Arthritis Rheumatol. 67, 1193–1202. doi: 10.1002/art.39060
Elkins, J., Veltkamp, R., Montaner, J., Johnston, S. C., Singhal, A. B., Becker, K., et al. (2017). Safety and efficacy of natalizumab in patients with acute ischaemic stroke (ACTION): a randomised, placebo-controlled, double-blind phase 2 trial. Lancet Neurol. 16, 217–226. doi: 10.1016/s1474-4422(16)30357-x
Fu, Y., Liu, Q., Anrather, J., and Shi, F. D. (2015). Immune interventions in stroke. Nat. Rev. Neurol. 11, 524–535. doi: 10.1038/nrneurol.2015.144
Gan, Y., Liu, Q., Wu, W., Yin, J. X., Bai, X. F., Shen, R., et al. (2014). Ischemic neurons recruit natural killer cells that accelerate brain infarction. Proc. Natl. Acad. Sci. U.S.A. 111, 2704–2709. doi: 10.1073/pnas.1315943111
Germain, C., Bihl, F., Zahn, S., Poupon, G., Dumaurier, M. J., Rampanarivo, H. H., et al. (2010). Characterization of alternatively spliced transcript variants of CLEC2D gene. J. Biol. Chem. 285, 36207–36215. doi: 10.1074/jbc.M110.179622
Gladstone, D. J., Black, S. E., and Hakim, A. M. (2002). Toward wisdom from failure: lessons from neuroprotective stroke trials and new therapeutic directions. Stroke 33, 2123–2136. doi: 10.1161/01.str.0000025518.34157.51
Gong, J., Qiu, C., Huang, D., Zhang, Y., Yu, S., and Zeng, C. (2018). Integrative functional analysis of super enhancer SNPs for coronary artery disease. J. Hum. Genet. 63, 627–638. doi: 10.1038/s10038-018-0422-2
Hong, Y., Tang, H. R., Ma, M., Chen, N., Xie, X., and He, L. (2019). Multiple sclerosis and stroke: a systematic review and meta-analysis. BMC Neurol. 19:139. doi: 10.1186/s12883-019-1366-7
Hu, H., Wang, B., Borde, M., Nardone, J., Maika, S., Allred, L., et al. (2006). Foxp1 is an essential transcriptional regulator of B cell development. Nat. Immunol. 7, 819–826. doi: 10.1038/ni1358
Hudmon, A., Kim, S. A., Kolb, S. J., Stoops, J. K., and Waxham, M. N. (2001). Light scattering and transmission electron microscopy studies reveal a mechanism for calcium/calmodulin-dependent protein kinase II self-association. J. Neurochem. 76, 1364–1375. doi: 10.1046/j.1471-4159.2001.00119.x
International Multiple Sclerosis Genetics Consortium (2013). Network-based multiple sclerosis pathway analysis with GWAS data from 15,000 cases and 30,000 controls. Am. J. Hum. Genet. 92, 854–865. doi: 10.1016/j.ajhg.2013.04.019
International Multiple Sclerosis Genetics Consortium (2019). Multiple sclerosis genomic map implicates peripheral immune cells and microglia in susceptibility. Science 365:eaav7188. doi: 10.1126/science.aav7188
International Multiple Sclerosis Genetics Consortium, Beecham, A. H., Patsopoulos, N. A., Xifara, D. K., Davis, M. F., Kemppinen, A., et al. (2013). Analysis of immune-related loci identifies 48 new susceptibility variants for multiple sclerosis. Nat. Genet. 45, 1353–1360. doi: 10.1038/ng.2770
International Multiple Sclerosis Genetics Consortium, Wellcome Trust Case Control Consortium, Sawcer, S., Hellenthal, G., Pirinen, M., Spencer, C. C., et al. (2011). Genetic risk and a primary role for cell-mediated immune mechanisms in multiple sclerosis. Nature 476, 214–219. doi: 10.1038/nature10251
Jay, K., Mitra, A., Harding, T., Matthes, D., and Van Ness, B. (2019). Identification of a de novo FOXP1 mutation and incidental discovery of inherited genetic variants contributing to a case of autism spectrum disorder and epilepsy. Mol. Genet. Genomic Med. 7:e00751. doi: 10.1002/mgg3.751
Jernas, M., Malmestrom, C., Axelsson, M., Nookaew, I., Wadenvik, H., Lycke, J., et al. (2013). microRNA regulate immune pathways in T-cells in multiple sclerosis (MS). BMC Immunol. 14:32. doi: 10.1186/1471-2172-14-32
Johnson, W., Onuma, O., Owolabi, M., and Sachdev, S. (2016). Stroke: a global response is needed. Bull. World Health Organ. 94, 634–634A. doi: 10.2471/blt.16.181636
Kemppinen, A. K., Kaprio, J., Palotie, A., and Saarela, J. (2011). Systematic review of genome-wide expression studies in multiple sclerosis. BMJ Open 1:e000053. doi: 10.1136/bmjopen-2011-000053
Khan, N. A., Quan, H., Hill, M. D., Pilote, L., McAlister, F. A., Palepu, A., et al. (2013). Risk factors, quality of care and prognosis in South Asian, East Asian and White patients with stroke. BMC Neurol. 13:74. doi: 10.1186/1471-2377-13-74
Knight, J. B., Eyster, C. A., Griesel, B. A., and Olson, A. L. (2003). Regulation of the human GLUT4 gene promoter: interaction between a transcriptional activator and myocyte enhancer factor 2A. Proc. Natl. Acad. Sci. U.S.A. 100, 14725–14730. doi: 10.1073/pnas.2432756100
Konopacki, C., Pritykin, Y., Rubtsov, Y., Leslie, C. S., and Rudensky, A. Y. (2019). Transcription factor Foxp1 regulates Foxp3 chromatin binding and coordinates regulatory T cell function. Nat. Immunol. 20, 232–242. doi: 10.1038/s41590-018-0291-z
Lambert, C., Cisternas, P., and Inestrosa, N. C. (2016). Role of wnt signaling in central nervous system injury. Mol. Neurobiol. 53, 2297–2311. doi: 10.1007/s12035-015-9138-x
Li, X., Xiao, J., Frohlich, H., Tu, X., Li, L., Xu, Y., et al. (2015). Foxp1 regulates cortical radial migration and neuronal morphogenesis in developing cerebral cortex. PLoS One 10:e0127671. doi: 10.1371/journal.pone.0127671
Liu, G., Hu, Y., Jin, S., and Jiang, Q. (2017a). Genetic variant rs763361 regulates multiple sclerosis CD226 gene expression. Proc. Natl. Acad. Sci. U.S.A. 114, E906–E907. doi: 10.1073/pnas.1618520114
Liu, G., Zhang, F., Hu, Y., Jiang, Y., Gong, Z., Liu, S., et al. (2017b). Multiple sclerosis risk pathways differ in Caucasian and Chinese populations. J. Neuroimmunol. 307, 63–68. doi: 10.1016/j.jneuroim.2017.03.012
Liu, G., Zhang, F., Jiang, Y., Hu, Y., Gong, Z., Liu, S., et al. (2017c). Integrating genome-wide association studies and gene expression data highlights dysregulated multiple sclerosis risk pathways. Mult. Scler. 23, 205–212. doi: 10.1177/1352458516649038
Llibre, A., Lopez-Macias, C., Marafioti, T., Mehta, H., Partridge, A., Kanzig, C., et al. (2016). LLT1 and CD161 expression in human germinal centers promotes B cell activation and CXCR4 downregulation. J. Immunol. 196, 2085–2094. doi: 10.4049/jimmunol.1502462
Ma, X., Meng, Z., Jin, L., Xiao, Z., Wang, X., Tsark, W. M., et al. (2017). CAMK2gamma in intestinal epithelial cells modulates colitis-associated colorectal carcinogenesis via enhancing STAT3 activation. Oncogene 36, 4060–4071. doi: 10.1038/onc.2017.16
Malik, R., Chauhan, G., Traylor, M., Sargurupremraj, M., Okada, Y., Mishra, A., et al. (2018). Multiancestry genome-wide association study of 520,000 subjects identifies 32 loci associated with stroke and stroke subtypes. Nat. Genet. 50, 524–537.
Malik, R., Traylor, M., Pulit, S. L., Bevan, S., Hopewell, J. C., Holliday, E. G., et al. (2016). Low-frequency and common genetic variation in ischemic stroke: the METASTROKE collaboration. Neurology 86, 1217–1226. doi: 10.1212/wnl.0000000000002528
Marrie, R. A., Reider, N., Cohen, J., Stuve, O., Trojano, M., Cutter, G., et al. (2015). A systematic review of the incidence and prevalence of cardiac, cerebrovascular, and peripheral vascular disease in multiple sclerosis. Mult. Scler. 21, 318–331. doi: 10.1177/1352458514564485
McDonald, W. I., Compston, A., Edan, G., Goodkin, D., Hartung, H. P., Lublin, F. D., et al. (2001). Recommended diagnostic criteria for multiple sclerosis: guidelines from the International Panel on the diagnosis of multiple sclerosis. Ann. Neurol. 50, 121–127. doi: 10.1002/ana.1032
Meng, Z., Ma, X., Du, J., Wang, X., He, M., Gu, Y., et al. (2017). CAMK2gamma antagonizes mTORC1 activation during hepatocarcinogenesis. Oncogene 36, 2446–2456. doi: 10.1038/onc.2016.400
Mhatre, S. D., Tsai, C. A., Rubin, A. J., James, M. L., and Andreasson, K. I. (2015). Microglial malfunction: the third rail in the development of Alzheimer’s disease. Trends Neurosci. 38, 621–636. doi: 10.1016/j.tins.2015.08.006
Mishra, A., and Macgregor, S. (2015). VEGAS2: software for more flexible gene-based testing. Twin Res. Hum. Genet. 18, 86–91. doi: 10.1017/thg.2014.79
Moutsianas, L., Jostins, L., Beecham, A. H., Dilthey, A. T., Xifara, D. K., Ban, M., et al. (2015). Class II HLA interactions modulate genetic risk for multiple sclerosis. Nat. Genet. 47, 1107–1113. doi: 10.1038/ng.3395
O’Connell, G. C., Treadway, M. B., Petrone, A. B., Tennant, C. S., Lucke-Wold, N., Chantler, P. D., et al. (2017). Peripheral blood AKAP7 expression as an early marker for lymphocyte-mediated post-stroke blood brain barrier disruption. Sci. Rep. 7:1172.
O’Roak, B. J., Deriziotis, P., Lee, C., Vives, L., Schwartz, J. J., Girirajan, S., et al. (2011). Exome sequencing in sporadic autism spectrum disorders identifies severe de novo mutations. Nat. Genet. 43, 585–589. doi: 10.1038/ng.835
Oshel, K. M., Knight, J. B., Cao, K. T., Thai, M. V., and Olson, A. L. (2000). Identification of a 30-base pair regulatory element and novel DNA binding protein that regulates the human GLUT4 promoter in transgenic mice. J. Biol. Chem. 275, 23666–23673. doi: 10.1074/jbc.M001452200
Rieger, M. E., Sims, A. H., Coats, E. R., Clarke, R. B., and Briegel, K. J. (2010). The embryonic transcription cofactor LBH is a direct target of the Wnt signaling pathway in epithelial development and in aggressive basal subtype breast cancers. Mol. Cell Biol. 30, 4267–4279. doi: 10.1128/mcb.01418-09
Rokita, A. G., and Anderson, M. E. (2012). New therapeutic targets in cardiology: arrhythmias and Ca2+/calmodulin-dependent kinase II (CaMKII). Circulation 126, 2125–2139. doi: 10.1161/circulationaha.112.124990
Rosen, D. B., Bettadapura, J., Alsharifi, M., Mathew, P. A., Warren, H. S., and Lanier, L. L. (2005). Cutting edge: lectin-like transcript-1 is a ligand for the inhibitory human NKR-P1A receptor. J. Immunol. 175, 7796–7799. doi: 10.4049/jimmunol.175.12.7796
Rosen, D. B., Cao, W., Avery, D. T., Tangye, S. G., Liu, Y. J., Houchins, J. P., et al. (2008). Functional consequences of interactions between human NKR-P1A and its ligand LLT1 expressed on activated dendritic cells and B cells. J. Immunol. 180, 6508–6517. doi: 10.4049/jimmunol.180.10.6508
Sagardoy, A., Martinez-Ferrandis, J. I., Roa, S., Bunting, K. L., Aznar, M. A., Elemento, O., et al. (2013). Downregulation of FOXP1 is required during germinal center B-cell function. Blood 121, 4311–4320. doi: 10.1182/blood-2012-10-462846
Shi, C., Sakuma, M., Mooroka, T., Liscoe, A., Gao, H., Croce, K. J., et al. (2008). Down-regulation of the forkhead transcription factor Foxp1 is required for monocyte differentiation and macrophage function. Blood 112, 4699–4711. doi: 10.1182/blood-2008-01-137018
Stamova, B., Jickling, G. C., Ander, B. P., Zhan, X., Liu, D., Turner, R., et al. (2014). Gene expression in peripheral immune cells following cardioembolic stroke is sexually dimorphic. PLoS One 9:e102550. doi: 10.1371/journal.pone.0102550
Stephen, T. L., Rutkowski, M. R., Allegrezza, M. J., Perales-Puchalt, A., Tesone, A. J., Svoronos, N., et al. (2014). Transforming growth factor beta-mediated suppression of antitumor T cells requires FoxP1 transcription factor expression. Immunity 41, 427–439. doi: 10.1016/j.immuni.2014.08.012
Tanaka, K., Shouguchi-Miyata, J., Miyamoto, N., and Ikeda, J. E. (2004). Novel nuclear shuttle proteins, HDBP1 and HDBP2, bind to neuronal cell-specific cis-regulatory element in the promoter for the human Huntington’s disease gene. J. Biol. Chem. 279, 7275–7286. doi: 10.1074/jbc.M310726200
Tang, B., Becanovic, K., Desplats, P. A., Spencer, B., Hill, A. M., Connolly, C., et al. (2012). Forkhead box protein p1 is a transcriptional repressor of immune signaling in the CNS: implications for transcriptional dysregulation in Huntington disease. Hum. Mol. Genet. 21, 3097–3111. doi: 10.1093/hmg/dds132
Tian, D. C., Shi, K., Zhu, Z., Yao, J., Yang, X., Su, L., et al. (2018). Fingolimod enhances the efficacy of delayed alteplase administration in acute ischemic stroke by promoting anterograde reperfusion and retrograde collateral flow. Ann. Neurol. 84, 717–728. doi: 10.1002/ana.25352
Tian, Y., Stamova, B., Jickling, G. C., Liu, D., Ander, B. P., Bushnell, C., et al. (2012). Effects of gender on gene expression in the blood of ischemic stroke patients. J. Cereb. Blood Flow Metab. 32, 780–791. doi: 10.1038/jcbfm.2011.179
Torres-Aguila, N. P., Carrera, C., Giese, A. K., Cullell, N., Muiño, E., Cárcel-Márquez, J., et al. (2019). Genome-wide association study of white blood cell counts in patients with ischemic stroke. Stroke 50, 3618–3621. doi: 10.1161/strokeaha.119.026593
Traylor, M., Farrall, M., Holliday, E. G., Sudlow, C., Hopewell, J. C., Cheng, Y. C., et al. (2012). Genetic risk factors for ischaemic stroke and its subtypes (the METASTROKE collaboration): a meta-analysis of genome-wide association studies. Lancet Neurol. 11, 951–962. doi: 10.1016/s1474-4422(12)70234-x
Tseng, C. H., Huang, W. S., Lin, C. L., and Chang, Y. J. (2015). Increased risk of ischaemic stroke among patients with multiple sclerosis. Eur. J. Neurol. 22, 500–506. doi: 10.1111/ene.12598
Wang, H., Geng, J., Wen, X., Bi, E., Kossenkov, A. V., Wolf, A. I., et al. (2014). The transcription factor Foxp1 is a critical negative regulator of the differentiation of follicular helper T cells. Nat. Immunol. 15, 667–675. doi: 10.1038/ni.2890
Wechsler, L. R., Bates, D., Stroemer, P., Andrews-Zwilling, Y. S., and Aizman, I. (2018). Cell therapy for chronic stroke. Stroke 49, 1066–1074. doi: 10.1161/strokeaha.117.018290
Wijchers, P. J., Burbach, J. P., and Smidt, M. P. (2006). In control of biology: of mice, men and Foxes. Biochem. J. 397, 233–246. doi: 10.1042/bj20060387
Yamaguchi-Kabata, Y., Morihara, T., Ohara, T., Ninomiya, T., Takahashi, A., Akatsu, H., et al. (2018). Integrated analysis of human genetic association study and mouse transcriptome suggests LBH and SHF genes as novel susceptible genes for amyloid-beta accumulation in Alzheimer’s disease. Hum. Genet. 137, 521–533.
Ye, J., Das, S., Roy, A., Wei, W., Huang, H., Lorenz-Guertin, J. M., et al. (2019). Ischemic injury-induced CaMKIIdelta and CaMKIIgamma confer neuroprotection through the NF-kappaB signaling pathway. Mol. Neurobiol. 56, 2123–2136. doi: 10.1007/s12035-018-1198-2
Yokoyama, W. M., and Plougastel, B. F. (2003). Immune functions encoded by the natural killer gene complex. Nat. Rev. Immunol. 3, 304–316. doi: 10.1038/nri1055
Yu, G. L., Wei, E. Q., Zhang, S. H., Xu, H. M., Chu, L. S., Zhang, W. P., et al. (2005). Montelukast, a cysteinyl leukotriene receptor-1 antagonist, dose- and time-dependently protects against focal cerebral ischemia in mice. Pharmacology 73, 31–40. doi: 10.1159/000081072
Yu, Z. Y., Lu, W. S., Zuo, X. B., Hu, J., Yao, S., Li, Y., et al. (2013). One novel susceptibility locus associate with systemic lupus erythematosus in Chinese Han population. Rheumatol. Int. 33, 2079–2083. doi: 10.1007/s00296-013-2697-0
Zhernakova, A., Stahl, E. A., Trynka, G., Raychaudhuri, S., Festen, E. A., Franke, L., et al. (2011). Meta-analysis of genome-wide association studies in celiac disease and rheumatoid arthritis identifies fourteen non-HLA shared loci. PLoS Genet. 7:e1002004. doi: 10.1371/journal.pgen.1002004
Keywords: multiple sclerosis, ischemic stroke, genome-wide association studies, gene-based test, gene expression analyses
Citation: Tian Z, Song Y, Yao Y, Guo J, Gong Z and Wang Z (2020) Genetic Etiology Shared by Multiple Sclerosis and Ischemic Stroke. Front. Genet. 11:646. doi: 10.3389/fgene.2020.00646
Received: 24 October 2019; Accepted: 27 May 2020;
Published: 03 July 2020.
Edited by:
Qinghua Jiang, Harbin Institute of Technology, ChinaReviewed by:
Hui Shen, Tianjin Medical University, ChinaLimin Wu, Massachusetts General Hospital and Harvard Medical School, United States
Copyright © 2020 Tian, Song, Yao, Guo, Gong and Wang. This is an open-access article distributed under the terms of the Creative Commons Attribution License (CC BY). The use, distribution or reproduction in other forums is permitted, provided the original author(s) and the copyright owner(s) are credited and that the original publication in this journal is cited, in accordance with accepted academic practice. No use, distribution or reproduction is permitted which does not comply with these terms.
*Correspondence: Zhongying Gong, MTMyMTIxMjEyNTBAMTYzLmNvbQ==; Zhiyun Wang, MTM4MjA1ODU2MjVAMTYzLmNvbQ==