- 1Department of Epidemiology, Erasmus MC University Medical Center Rotterdam, Rotterdam, Netherlands
- 2SkylineDx B.V., Rotterdam, Netherlands
- 3Department of Cardiovascular Sciences, University of Leicester, Leicester, United Kingdom
- 4Department of Internal Medicine, Erasmus MC University Medical Center Rotterdam, Rotterdam, Netherlands
- 5NIHR Leicester Biomedical Research Centre, Glenfield Hospital, Leicester, United Kingdom
- 6Center for Biomics, Erasmus MC University Medical Center Rotterdam, Rotterdam, Netherlands
- 7Department of Neurology, Erasmus MC University Medical Center Rotterdam, Rotterdam, Netherlands
- 8Nuffield Department of Population Health, University of Oxford, Oxford, United Kingdom
Telomeres are important for maintaining genomic stability. Telomere length has been associated with aging, disease, and mortality and is highly heritable (∼82%). In this study, we aimed to identify rare genetic variants associated with telomere length using whole-exome sequence data. We studied 1,303 participants of the Erasmus Rucphen Family (ERF) study, 1,259 of the Rotterdam Study (RS), and 674 of the British Heart Foundation Family Heart Study (BHF-FHS). We conducted two analyses, first we analyzed the family-based ERF study and used the RS and BHF-FHS for replication. Second, we combined the summary data of the three studies in a meta-analysis. Telomere length was measured by quantitative polymerase chain reaction in blood. We identified nine rare variants significantly associated with telomere length (p-value < 1.42 × 10–7, minor allele frequency of 0.2–0.5%) in the ERF study. Eight of these variants (in C11orf65, ACAT1, NPAT, ATM, KDELC2, and EXPH5) were located on chromosome 11q22.3 that contains ATM, a gene involved in telomere maintenance. Although we were unable to replicate the variants in the RS and BHF-FHS (p-value ≥ 0.21), segregation analysis showed that all variants segregate with shorter telomere length in a family. In the meta-analysis of all studies, a nominally significant association with LTL was observed with a rare variant in RPL8 (p-value = 1.48 × 10−6), which has previously been associated with age. Additionally, a novel rare variant in the known RTEL1 locus showed suggestive evidence for association (p-value = 1.18 × 10–4) with LTL. To conclude, we identified novel rare variants associated with telomere length. Larger samples size are needed to confirm these findings and to identify additional variants.
Introduction
Telomeres are DNA structures located at the ends of chromosomes and consist of tandem hexanucleotide sequence repeats (TTAGGG) (Blackburn and Gall, 1978). They are important for maintaining genomic stability by preventing DNA degradation and chromosomal fusions (Blackburn, 2000). Telomeres are shortened with each cell division due to the inability of DNA polymerase to fully extend the 3′ end of the DNA strand during replication. When the telomeres reach a critical length, this leads to cellular senescence and ultimately to cell death, making them regulators of the replicative capacity of a cell and markers of biological age (Lindsey et al., 1991; Allsopp et al., 1992).
Shorter leukocyte telomere length (LTL) has been associated with several age-related diseases including cardiovascular diseases (Brouilette et al., 2003, 2007; Benetos et al., 2004; Fitzpatrick et al., 2007; Willeit et al., 2010a; Haycock et al., 2014), cancer (Hastie et al., 1990; Willeit et al., 2010b, 2011; Bojesen et al., 2013) and dementia (Martin-Ruiz et al., 2006; Grodstein et al., 2008; Honig et al., 2012). LTL has also been associated with mortality (Cawthon et al., 2003; Kimura et al., 2008; Fitzpatrick et al., 2011; Honig et al., 2012; Deelen et al., 2014; Rode et al., 2015; Marioni et al., 2016). However, this association has been inconsistent (Martin-Ruiz et al., 2005; Harris et al., 2006; Njajou et al., 2009; Houben et al., 2011; Strandberg et al., 2011; Needham et al., 2015). LTL is highly heritable with heritability estimates ranging from 34 to 82% (Slagboom et al., 1994; Bischoff et al., 2005; Vasa-Nicotera et al., 2005; Andrew et al., 2006; Broer et al., 2013). Previously, genome-wide association studies (GWASs) in European ancestry studies have identified common variants associated with LTL located in multiple genes, including: TERC (Codd et al., 2010, 2013; Mangino et al., 2012; Pooley et al., 2013), TERT (Codd et al., 2013; Pooley et al., 2013), NAF1 (Codd et al., 2013), OBFC1 (Levy et al., 2010; Mangino et al., 2012; Codd et al., 2013; Pooley et al., 2013), RTEL1 (Codd et al., 2013), CTC1 (Mangino et al., 2012), ZNF676 (Mangino et al., 2012), ZNF208 (Codd et al., 2013), ACYP2 (Codd et al., 2013), DCAF4 (Mangino et al., 2015), and PXK (Pooley et al., 2013). However, these variants explain < 5% of the heritability.
Up until now, no systemic whole exome or genome screen for rare variants has been published, despite the fact that these may explain part of the heritability (Manolio et al., 2009). Rare variants are not well captured by microarrays used for GWAS and remain difficult to impute, despite the recent improvements in imputation panels (McCarthy et al., 2016). Next generation sequencing technologies, such as whole-exome sequencing (WES), are better suited to study rare variants. In this study, we present a dual analysis. First, we conducted a genome-wide WES analysis of LTL in 1,303 individuals of the Dutch Erasmus Rucphen Family (ERF) study to search for rare genetic variants associated with LTL. The advantage of a family-based study is that the segregation of rare variants can be studied. We performed a replication analysis in the Rotterdam Study (RS) and the British Heart Foundation Family Heart Study (BHF-FHS). Next, we pooled the data together and conducted a meta-analysis of the association results of all three cohorts.
Results
Descriptive statistics of the family-based and population-based studies are provided in Table 1. Mean age at LTL measurement was 49 years (SD = 15.0) in the ERF study and 61% of the study participants were female. The RS participants were older (age = 75 years, SD = 7.7) and 57% of the participants were female, while mean age in the BHF-FHS was 58 years (SD = 8.2) and most study participants were male (26% female). Mean LTL values, measured in each participant using a quantitative polymerase chain reaction (qPCR) based technique as the ratio of telomere repeat length to copy number of the single copy gene 36B4, were higher in the ERF study (LTL = 1.85, SD = 0.35) than in the RS (LTL = 0.94, SD = 0.18) and BHF-FHS (LTL = 1.37, SD = 0.22). After adjusting LTL values for age and sex, mean LTL was comparable between studies (Table 1).
The Manhattan plot and the distribution of the test statistic [quantile-quantile (QQ) plot, λ = 1.04] of the WES analysis in the ERF study are presented in Figures 1, 2, respectively. We observed significant association of nine rare variants [Minor Allele Frequency (MAF) between 0.2% and 0.5%] with LTL as shown in Table 2. The significance threshold (p-value < 1.42 × 10–7) was adjusted for multiple testing using Bonferroni correction based on the number of variants in the analysis (0.05/353,075 variants). Each variant was negatively associated with LTL and the estimated effects of the minor allele of these variants were large (−2.18 < standardized β < −1.34), suggesting a significant decrease in LTL for each minor allele.
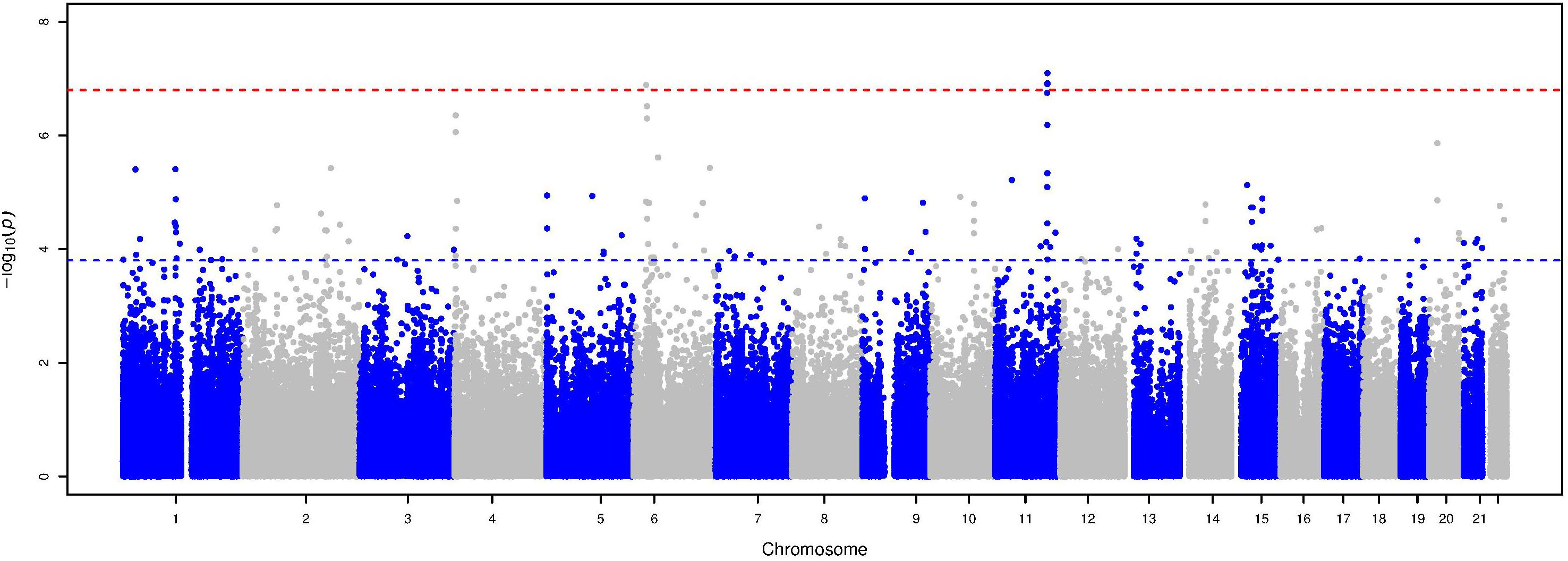
Figure 1. Manhattan plot of the association analysis with LTL in the ERF study. This plot shows –log10 transformed p-values (y-axis) for all variants present in the association analysis according to their position on each chromosome (x-axis). The red dashed line represents the Bonferroni corrected p-value threshold for significance (p-value < 1.42 × 10–7), while the blue dashed line represents the p-value threshold for suggestive significance (p-value ≤ 1.42 × 10–4).
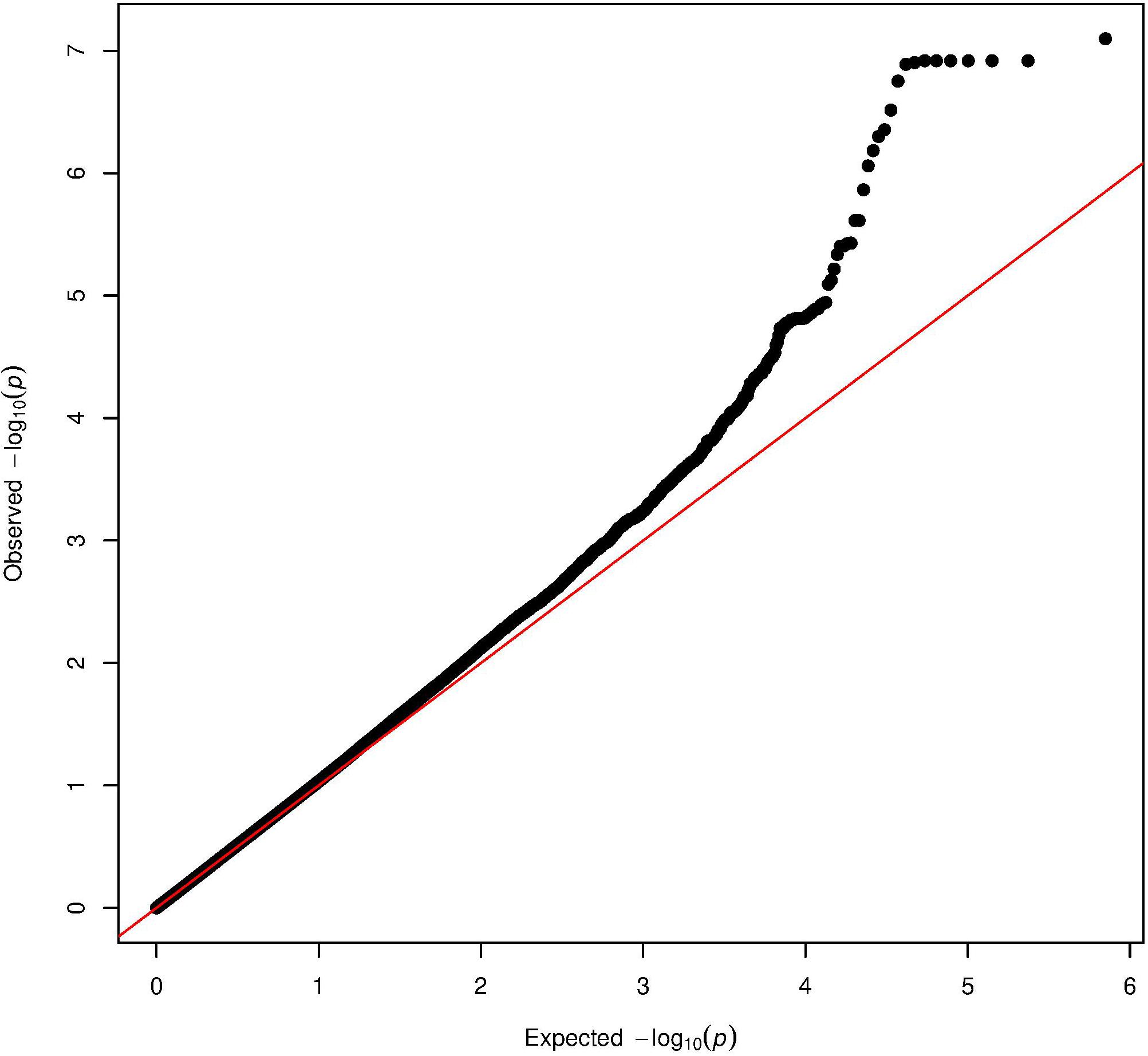
Figure 2. Quantile-quantile plot of the association analysis with LTL in the ERF study. The QQ-plot shows the observed test statistics (y-axis) plotted against the expected values of the test statistics (x-axis) (X2-distribution). The red line shows the distribution under the null hypothesis.
The top eight variants are located in a dense region on chromosome 11q22.3 (position range: 108004687 – 108384666, Figure 3) and appear to be a part of a haplotype that spans the C11orf65, ACAT1, NPAT, ATM, KDELC2, and EXPH5 genes. A haplotype can describe a pair of genes inherited together from one parent on one chromosome, or it can describe all of the genes on a chromosome that were inherited together from a single parent. This haplotype segregates with shorter LTL in a family (Supplementary Figure 1), where it is carried by 14 individuals, 11 of whom were related within 4 generations according to the pedigree data (Figure 4). Further, the genetic kinship estimates show that the other three individuals are also related within 3–4 generations. These 8 variants are in strong linkage disequilibrium (pairwise LD: r2 between 0.93 and 1.00, D′ = 1) and show very similar p-values. The top variant rs185270276 is located in an intron of the C11orf65 gene (MAF = 0.5%, β = −1.34, SE = 0.25, p-value = 7.99 × 10–8). The next six variants significantly associated with LTL (MAF = 0.5%, β = −1.38, SE = 0.26, p-value = 1.21 × 10–7) are located in the ACAT1, NPAT, ATM/C11orf65, KDELC2, and EXPH5 genes. Two of these six variants are missense variants: rs79119325 (NPAT, PolyPhen = 1, CADD score = 18.1) and rs12146512 (EXPH5, PolyPhen = 0.624, CADD score = 4.5). The eighth significant variant, rs2234993, is located within an intron of ATM (MAF = 0.5%, β = −1.37, SE = 0.26, p-value = 1.25 × 10–7). The ninth significant variant, rs144114619 (MAF = 0.2%, p-value = 1.29 × 10–7), is a missense variant located on chromosome six in the BTN3A1 gene, which is predicted to be damaging (PolyPhen = 1, CADD score = 12.2) and has the largest effect size (β = −2.18, SE = 0.41). There were six carriers of this variant in the ERF population. Interestingly, four of these carriers are also carriers of the rare variants in the chromosome 11q22.3 region (Supplementary Figure 2).
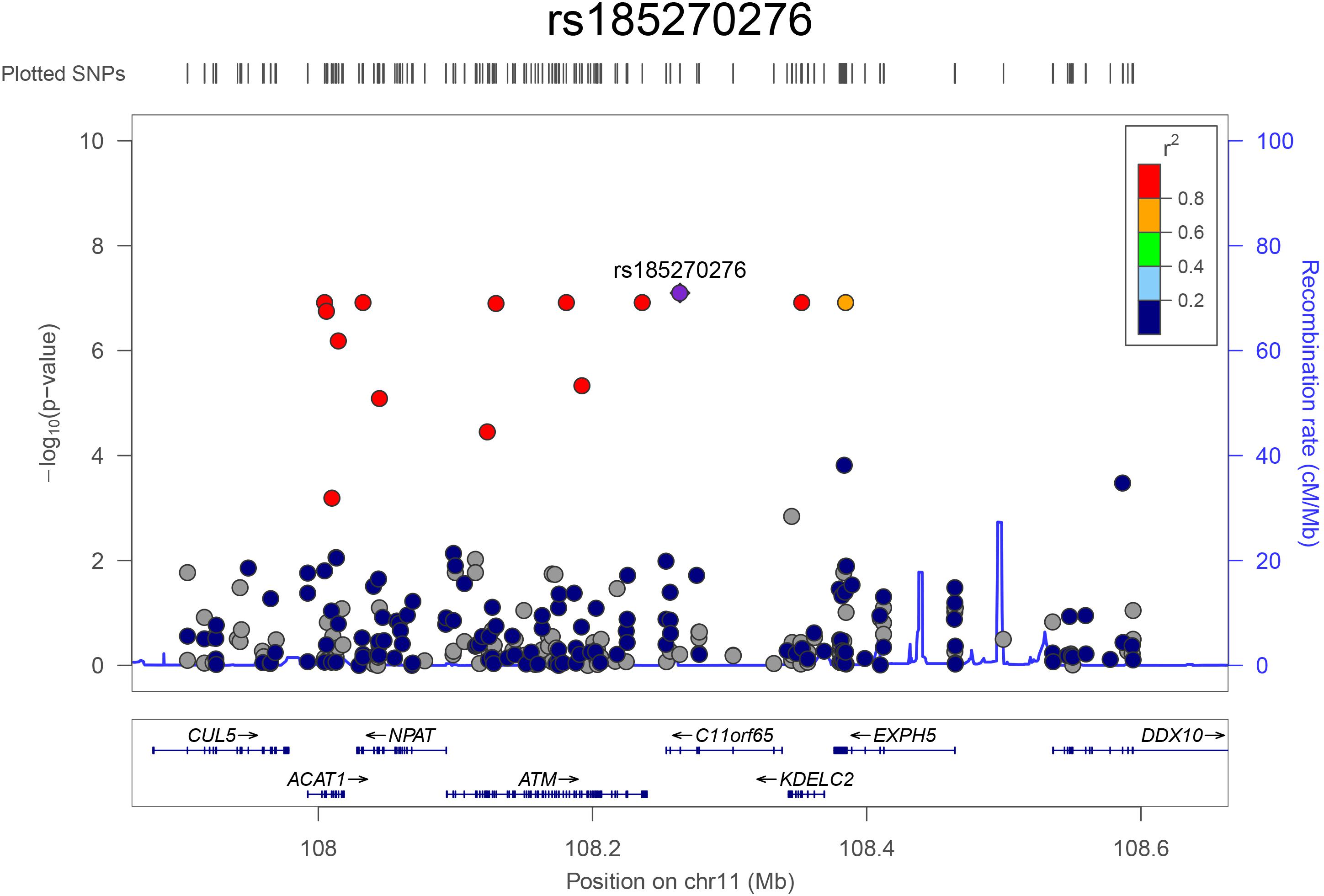
Figure 3. Regional association plot for the top hits on chromosome 11. The plot was constructed using LocusZoom (http://locuszoom.org/). The –log10 transformed p-values are plotted on the y-axis. The x-axis shows the position of the variants (dots) on chromosome 11 and the genes in this region. The most significant variant (rs185270276) is shown in purple and the color of the dots indicates the extend of linkage disequilibrium between the variant and the top variant.
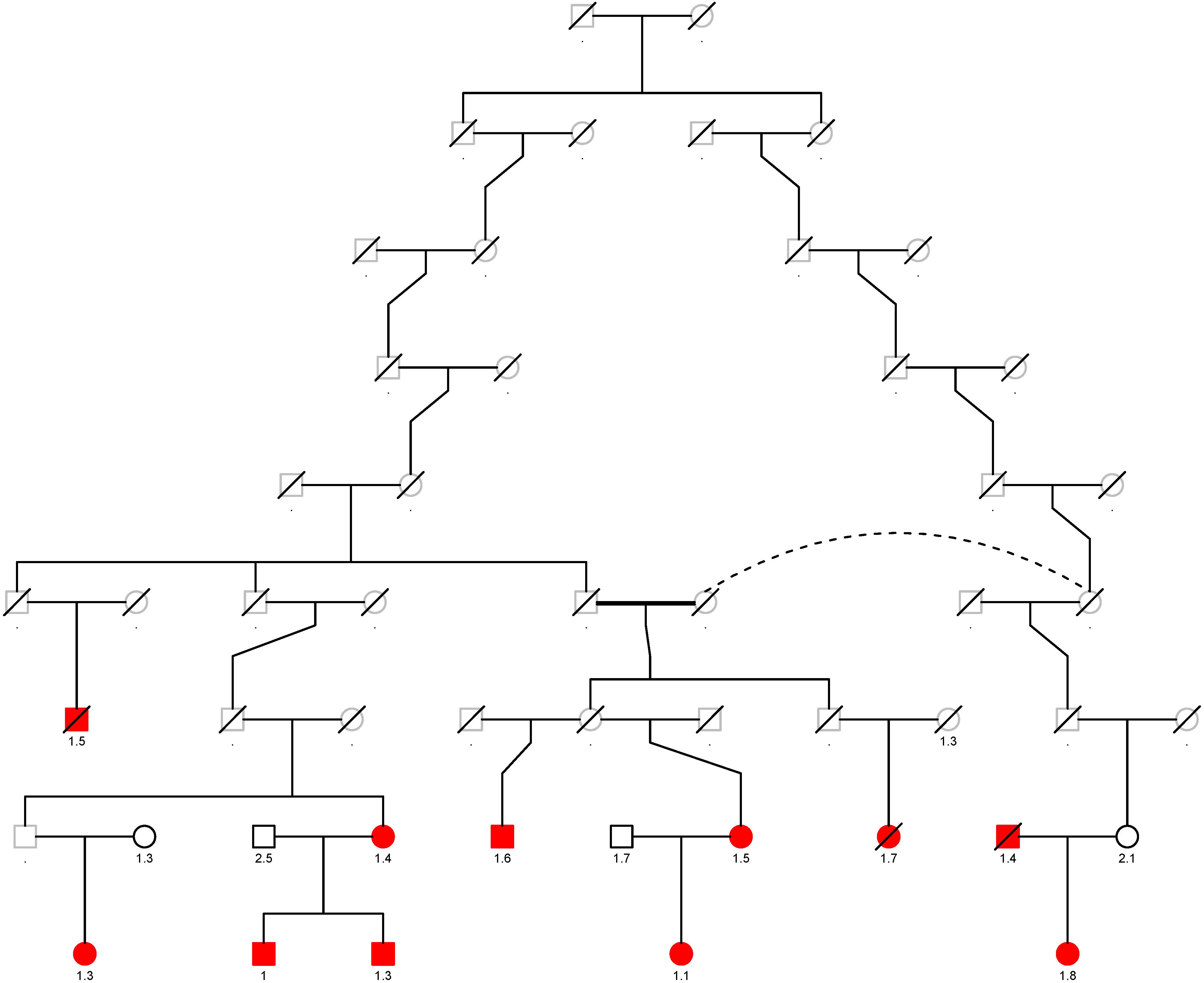
Figure 4. Segregation plot of the rare variants on chromosome 11q22.3 in the ERF study. The carriers of the rare variants located on chromosome 11q22.3 are depicted in red. The T/S ratio is added below the individuals of whom whole-exome sequencing data was available. Squares are males and rounds are females. Deceased individuals are denoted by a line through that specific individual. One person is twice in the pedigree, this is shown with the dotted line.
For replication analysis, we used WES data from two independent cohorts of European ancestry, the RS and the BHF-FHS. Results of the replication analysis are shown in Table 3, together with the results of the meta-analysis of summary statistics from all three cohorts for these variants. Six out of nine rare variants significantly associated with LTL in the ERF study (located in ACAT1, NPAT, ATM/C11orf65, EXPH5, and BTN3A1) were present in the RS and/or the BHF-FHS but were not significantly (p-value ≥ 0.21) associated with LTL (p-value < 0.025, 0.05/2 independent tests). The direction of effect of most variants was similar in the ERF study and the RS, while the BHF-FHS showed opposite direction of effect for most variants. Three variants, located in C11orf65, KDELC2, and ATM, were not present in the RS and BHF-FHS data. As these are unique to an isolated population, we were unable to confirm or reject their association with LTL in the replication cohorts.
Finally, to increase the statistical power, we performed an inverse-variance weighted meta-analysis of the association results from all three cohorts using METAL software. Variants were included in the meta-analysis if they were present in at least two out of three cohorts and had a minimum minor allele count of five in one or more cohorts, resulting in a multiple testing corrected significance threshold of 3.02 × 10–7 (0.05/165,311 variants). The top results of the meta-analysis (p-value < 3.02 × 10–4) are available in Table 4 and in Supplementary Table 1, which also contains cohort specific information. The Manhattan and QQ-plots are provided in Supplementary Figures 3, 4, respectively. The λ of 0.97 suggests the power has been low. Although there were no variants genome-wide significantly associated with LTL in the meta-analysis after adjusting for multiple testing, many of the top findings show a consistent effect across cohorts. The variant most significantly associated with LTL was a highly conserved synonymous variant (PhastCons score = 0.999) located in the RPL8 gene on chromosome 8 (p-value = 1.48 × 10–6), which is predicted to be deleterious (CADD score = 15.11). Additionally, we used the meta-analysis results to perform a look-up of variants in loci identified by previous European ancestry GWASs (Supplementary Table 2). There were 153 variants present in these loci and we found suggestive evidence (p-valuemeta < 3.02 × 10–4) for a positive association of a rare variant with LTL (rs181080831, β = 0.74, SE = 0.19, p-value = 1.18 × 10–4) in the known RTEL1 locus.
Discussion
In the family-based ERF study, we identified nine rare variants (MAF between 0.2% and 0.5%) associated with LTL by performing a WES association analysis. The eight most significantly associated variants are located in a region on chromosome 11q22.3 and segregate together with shorter LTL in one family. This region contains the ATM locus that has previously been shown to be involved in telomere maintenance and genomic stability and is thus an obvious candidate gene. In the meta-analysis of discovery and replication cohorts, we identified another rare missense variant in the RPL8 gene strongly associated with LTL. Although we were not able to replicate either of the associations, both ATM and RPL8 have been previously found to be strong predictors of telomere length (ATM) and chronological age (ATM and RPL8).
Interestingly, we identified three unique rare variants in the ATM (Ataxia Telangiectasia Mutated) gene associated with LTL in the ERF study. ATM is the homolog of the Tel1 gene in yeast (Greenwell et al., 1995) and has been implicated in important telomere maintenance processes (Pandita, 2002; Lee et al., 2015; Tong et al., 2015). The ATM protein kinase is a master controller of cell cycle checkpoint signaling pathways required for cell response to DNA damage and for genomic stability (https://www.ncbi.nlm.nih.gov/gene/?term=472). Additionally, ATM kinase is necessary for telomere elongation (Lee et al., 2015; Tong et al., 2015). ATM is involved in the genetic disorder ataxia telangiectasia (AT), which is characterized by cerebellar ataxia, oculocutaneous telangiectasia, immunodeficiency, and a predisposition to cancer (Savitsky et al., 1995). Cells of AT patients also show telomeric fusions and have accelerated telomere shortening with increasing age (Metcalfe et al., 1996). Furthermore, ATM was significantly associated with chronological age in a meta-analysis of gene expression profiles, showing lower transcript abundance in older individuals (Peters et al., 2015). Although genetic variants in ATM have been associated with various cancers (Barrett et al., 2011; Helgason et al., 2015; Ransohoff et al., 2017; Scelo et al., 2017), only one genetic variant in ATM, rs227080, was genome-wide significantly associated with LTL in a Singaporean Chinese population (Dorajoo et al., 2019). This variant was not significantly associated with LTL in the ERF study, the Rotterdam Study or the BHF-FHS, implicating it was not driving the association observed in the current study.
Segregation analysis of the variants within the chromosome 11q22.3 region in the ERF study showed that the variants segregate with shorter LTL in one family. We did not detect a specific disease that segregates in this family, which may be explained by the relatively young age of most of the carriers. In the replication analysis, we were not able to confirm the association of the nine variants associated with LTL in the RS and BHF-FHS, due to lack of association (six variants) or absence of the variant in the replication cohorts (three variants). However, it is possible this signal comes from variants that are family-specific and thus may not be transferable to the general population. Likewise, it may also be that the whole haplotype has an effect on telomere length, an haplotype that is most likely unique to the isolated ERF population. At this locus, the ATM gene is currently the most likely causative gene. Lastly, we performed a meta-analysis of the three cohorts. The top variant of the meta-analysis is located in RPL8 (Ribosomal Protein L8). This is interesting as RPL8, together with six other RPL genes, was negatively associated with chronological age (Peters et al., 2015). In this transcriptomic study, Peters et al. identified 1,497 genes whose expression level changes associated with age; this gene list includes RPL8 and ATM. We additionally found suggestive evidence for an association of a novel rare variant in the known RTEL1 locus. It would be interesting to perform a WES or whole-genome sequencing meta-analysis with larger sample size to increase the statistical power.
The advantage of our study is that we used a family-based setting that allowed us to show segregation of the variants located on chromosome 11q22.3 in a family. The ERF study population has shown a low immigration rate and a high level of inbreeding, which has increased the frequency of many rare alleles (Pardo et al., 2005). Another advantage is that all three studies used the standardized qPCR method to quantify LTL. However, there are also several limitations to our study. The first limitation is that our findings in the ERF study are not easy to generalize to the general population as these findings may be family-specific. However, we identified genes that are known to be related to telomere processes and thus are plausible candidate genes. The second limitation of our study may be that we used blood measurements of telomere length instead of tissue specific measurements, although previous studies have shown that mean telomere length in blood and other tissues are highly correlated (Okuda et al., 2002; Wilson et al., 2008) and, therefore, we expect this did not influence our findings. The third limitation is the difference in LTL distribution between the three studies, which may be explained by the different age distributions in the studies. Mean age is lowest in the ERF study (49 years), highest in the RS (75 years) and mean age in the BHF-FHS is in between (58 years). As LTL decreases significantly with age (Lindsey et al., 1991; Slagboom et al., 1994; Blackburn, 2001) and is associated with mortality (Cawthon et al., 2003; Kimura et al., 2008; Fitzpatrick et al., 2011; Honig et al., 2012; Deelen et al., 2014; Rode et al., 2015; Marioni et al., 2016), the variation in LTL becomes less with the aging populations. Mean LTL values were comparable between studies after adjusting LTL values for age and sex. To further standardize across the three cohorts, a z-transformation of the LTL values was performed resulting in comparable distributions with mean of zero and standard deviation of one. Nevertheless, these differences between studies, together with the small sample size of the BHF-FHS cohort, could potentially explain the lack of replication. The fourth limitation is that we were unable to calculate the effect of the variants on telomere length in base pairs because of the quantification method of LTL in our study together with the z-transformation that was applied to the LTL values to standardize across the cohorts.
To conclude, this first study using WES data to search for rare genetic variants associated with LTL has identified interesting variants and genes associated with shorter LTL. Eight out of nine rare variants associated with LTL are located on chromosome 11q22.3 and all variants segregate within an ERF family. As we were not able to replicate findings, future studies should further investigate this region and the other genes identified in this study to confirm their involvement in telomere length regulation.
Materials and Methods
Study Populations
Our discovery population consisted of participants from the family-based Erasmus Rucphen Family (ERF) study. The ERF study comprises approximately 3,000 inhabitants of a recent genetically isolated community in the Southwest of the Netherlands, studied as part of the Genetic Research in Isolated Population program (Pardo et al., 2005). All ERF participants are descendants of 22 founder couples who had at least six children baptized in the community church in the 18th century, or their spouses. Baseline data collection, including blood withdrawal, took place between 2002 and 2005. As the ERF population shows a low rate of immigration and a high level of inbreeding, the frequency of several rare alleles is increased in this population (Pardo et al., 2005). The ERF study was approved by the Medical Ethics Commitee of the Erasmus Medical Center (MC), Rotterdam, Netherlands. All participants provided written informed consents and all investigations were carried out in accordance with the Declaration of Helsinki.
The replication cohorts included participants from the Rotterdam Study (RS) and the British Heart Foundation Family Heart Study (BHF-FHS). The RS is a prospective cohort study ongoing since 1990 in the well-defined Ommoord district in Rotterdam, The Netherlands. The original RS cohort (RS-I) included 7,983 individuals of 55 years of age or over. At baseline, participants were interviewed at home and had an extensive set of examinations, which were repeated every 3–4 years (Ikram et al., 2017). The Rotterdam Study has been approved by the Medical Ethics Committee of the Erasmus MC and by the Dutch Ministry of Health, Welfare and Sport. The Rotterdam Study has been entered into the Netherlands National Trial Register (NTR; www.trialregister.nl) and into the WHO International Clinical Trials Registry Platform (ICTRP; www.who.int/ictrp/network/primary/en/) under shared catalogue number NTR6831. All participants provided written informed consent to participate in the study and to have their information obtained from treating physicians.
The British Heart Foundation Family Heart study recruited families with at least two siblings diagnosed with premature (<66 years) coronary artery disease (CAD) within the United Kingdom between 1998 and 2003. Full details are provided elsewhere (Samani et al., 2005, 2007).
Telomere Length
Mean LTL values in the ERF study and the BHF-FHS study were measured using a qPCR method in all samples (Cawthon, 2002). The measurements were performed in Leicester, United Kingdom, and details of the measurements were previously described (Codd et al., 2010, 2013). In summary, mean LTL was measured in leukocytes and expressed as the ratio (T/S ratio) of telomere repeat length (T) to the copy number of a single-copy gene, 36B4 (S). Samples were quantified relative to a calibrator sample used on each run (DNA from the K562 cell line) (Codd et al., 2010).
In the RS, mean LTL values were also measured using a qPCR assay based on the method described by Cawthon (2002) with minor modifications. For each sample the telomere and 36B4 assay were run in separate wells but in the same 384 wells PCR plate. Each reaction contained 5 ng DNA, 1 uM of each of the telomere primers (tel1b-forward: GGTT TGTTTGGGTTTGGGTTTGGGTTTGGGTTTGGGTT, tel2b- reverse: GGCTTGCCTTACCCTTACCCTTACCCTTACCCTTA CCCT) or 250 nM of the 36B4 primers (36B4u-forward: CAG CAAGTGGGAAGGTGTAATCC, 36B4d-reverse: CCCATTCT ATCATCAACGGGTACAA) and 1x Quantifast SYBR green PCR Mastermix (Qiagen). The reactions for both assays were performed in duplicate for each sample in a 7900HT machine (Applied Biosystems). Ct values and PCR efficiencies were calculated per plate using the MINER algorithm (Zhao and Fernald, 2005). Duplicate Ct values that had a Coefficient of Variance (CV) of more than 1% were excluded from further analysis. Using the average Ct value per sample and the average PCR efficiency per plate the samples were quantified using the formula Q = 1/(1 + PCR eff)^Ct. The relative telomere length was calculated by dividing the Q of the telomere assay by the Q of the 36B4 assay. To validate the assay 96 random samples were run twice and the CV of that experiment was 4.5%.
Exome Sequencing
The exomes of 1,336 ERF participants were sequenced at the Erasmus Center for Biomics of the department of Cell Biology, Erasmus MC, The Netherlands. The exomes of a randomly selected subset of 2,628 individuals from the RS-I population were sequenced at the Human Genotyping facility of the Department of Internal Medicine, Erasmus MC, The Netherlands. Details of the methods and quality control for ERF and RS are described elsewhere (Amin et al., 2016; van Rooij et al., 2017). In total, 1,303 ERF participants and 1,257 RS participants had both exome sequence and LTL data available and were included in this analysis. A subset of the BHF-FHS, comprising of 674 unrelated individuals of Caucasian ancestry who had previously undergone exome sequencing as part of the Leicester Myocardial Infarction Study (Khera et al., 2017) and had LTL data available (Codd et al., 2010) were included in this analysis.
Statistical Analyses
For each individual cohort quantitative trait association analysis was performed using Rare Variant tests (RVtests) software, which supports the analysis of related individuals (Zhan et al., 2016). Association analysis was performed using a score test, assuming an additive model, suitable for analysis with related and unrelated individuals. We applied a z-transformation of LTL values for the three cohorts separately to standardize values across cohorts. All analyses were adjusted for age, sex and batch effects (if needed). Furthermore, we adjusted for familial relationships in ERF using the kinship matrix estimated from the genotyped data, while in the RS we corrected for the first four principal components as the fourth principal component was significantly associated with LTL. Only variants with a minor allele count ≥ 5 were included.
In the ERF study, we calculated the pairwise LD (r2 and D′) between the top eight variants on chromosome 11 that were significantly associated with LTL using the –ld command of PLINK 1.9 software (Chang et al., 2015) (www.cog-genomics.org/plink/1.9/). Additionally, we performed an inverse-variance weighted meta-analysis using METAL software (Willer et al., 2010). In the analysis using data of the family-based ERF study, we corrected the significance thresholds for multiple testing using Bonferroni correction, resulting in a significance threshold of 1.42 × 10–7 (0.05/353,075). In the replication analysis, the multiple testing corrected p-value thresholds was 0.025 (0.05/2 independent tests). In the meta-analysis, we adjusted for the number of variants tested, resulting in a significance threshold of 3.02 × 10–7 (0.05/165,311).
Data Availability Statement
The datasets analyzed for each individual cohort can be requested by contacting the responsible Principal Investigator. Because of restrictions based on privacy regulations and informed consent of the participants, data cannot be made freely available in a public repository. For the Rotterdam Study data, requests should be directed toward the management team of the Rotterdam Study (secretariat.epi@erasmusmc.nl), which has a protocol for approving data requests.
Ethics Statement
The studies involving human participants were reviewed and approved by their respective Medical Ethics Boards and all investigations were carried out in accordance with the Declaration of Helsinki. The patients/participants provided their written informed consent to participate in this study.
Author Contributions
AS, NA, and CD designed the study and wrote the manuscript. AS, SW, CN, and SA performed the analyses. LB, PA, RB, MD, MH, JR, RK, and NA were involved in the data collection and provided the data. WI, NS, MI, AU, and CD were involved in the supervision of individual cohorts. AS, SW, CN, DV, NS, VC, NA, and CD interpreted the results. All authors critically reviewed and approved the manuscript.
Conflict of Interest
AS is an employee of the company SkylineDx.
The remaining authors declare that the research was conducted in the absence of any commercial or financial relationships that could be construed as a potential conflict of interest.
Funding
The ERF study as a part of EUROSPAN (European Special Populations Research Network) was supported by European Commission FP6 STRP grant number 018947 (LSHG-CT-2006-01947) and also received funding from the European Community’s Seventh Framework Programme (FP7/2007-2013)/grant agreement HEALTH-F4-2007-201413 by the European Commission under the programme “Quality of Life and Management of the Living Resources” of 5th Framework Programme (No. QLG2-CT-2002-01254). High-throughput analysis of the ERF data was supported by joint grant from Netherlands Organization for Scientific Research and the Russian Foundation for Basic Research (NWO-RFBR 047.017.043). Exome sequencing analysis in ERF was supported by the ZonMw grant (project 91111025). AS and CD have used exchange grants from Personalized pREvention of Chronic DIseases consortium (PRECeDI) (H2020-MSCA-RISE-2014). The generation and management of the exome sequencing data for the Rotterdam Study was executed by the Human Genotyping Facility of the Genetic Laboratory of the Department of Internal Medicine, Erasmus MC, the Netherlands. The Exome Sequencing data set was funded by the Netherlands Genomics Initiative (NGI)/Netherlands Organisation for Scientific Research (NWO) sponsored Netherlands Consortium for Healthy Aging (NCHA; project nr. 050-060-810), by the Genetic Laboratory of the Department of Internal Medicine, Erasmus MC, and by the and by a Complementation Project of the Biobanking and Biomolecular Research Infrastructure Netherlands (BBMRI-NL; www.bbmri.nl; project number CP2010-41). The Rotterdam Study was funded by Erasmus Medical Center and Erasmus University, Rotterdam, Netherlands Organization for the Health Research and Development (ZonMw), the Research Institute for Diseases in the Elderly (RIDE), the Ministry of Education, Culture and Science, the Ministry for Health, Welfare and Sports, the European Commission (DG XII), and the Municipality of Rotterdam. The BHF-FHS study was funded by the British Heart Foundation (BHF). Exome sequencing of the Leicester Myocardial Infarction Study was supported by grant 5U54HG003067 from the National Institutes of Health. CN and NS were supported by the BHF. NS is a NIHR Senior Investigator.
Acknowledgments
ERF study: we are grateful to all study participants and their relatives, general practitioners, and neurologists for their contributions and to P. Veraart for her help in genealogy, J. Vergeer for the supervision of the laboratory work and P. Snijders for his help in data collection. Rotterdam Study: we thank Mr. Pascal Arp, Ms. Mila Jhamai, and Mr. Marijn Verkerk for their help in creating the RS-Exome Sequencing database. We are grateful to the study participants, the staff from the Rotterdam Study and the participating general practitioners and pharmacists.
Supplementary Material
The Supplementary Material for this article can be found online at: https://www.frontiersin.org/articles/10.3389/fgene.2020.00337/full#supplementary-material
References
Allsopp, R. C., Vaziri, H., Patterson, C., Goldstein, S., Younglai, E. V., Futcher, A. B., et al. (1992). Telomere length predicts replicative capacity of human fibroblasts. Proc. Natl. Acad. Sci. U.S.A. 89, 10114–10118. doi: 10.1073/pnas.89.21.10114
Amin, N., Jovanova, O., Adams, H. H., Dehghan, A., Kavousi, M., Vernooij, M. W., et al. (2016). Exome-sequencing in a large population-based study reveals a rare Asn396Ser variant in the LIPG gene associated with depressive symptoms. Mol. Psychiatry 22, 537–543. doi: 10.1038/mp.2016.101
Andrew, T., Aviv, A., Falchi, M., Surdulescu, G. L., Gardner, J. P., Lu, X., et al. (2006). Mapping genetic loci that determine leukocyte telomere length in a large sample of unselected female sibling pairs. Am. J. Hum. Genet. 78, 480–486. doi: 10.1086/500052
Barrett, J. H., Iles, M. M., Harland, M., Taylor, J. C., Aitken, J. F., Andresen, P. A., et al. (2011). Genome-wide association study identifies three new melanoma susceptibility loci. Nat. Genet. 43, 1108–1113.
Benetos, A., Gardner, J. P., Zureik, M., Labat, C., Xiaobin, L., Adamopoulos, C., et al. (2004). Short telomeres are associated with increased carotid atherosclerosis in hypertensive subjects. Hypertension 43, 182–185. doi: 10.1161/01.hyp.0000113081.42868.f4
Bischoff, C., Graakjaer, J., Petersen, H. C., Hjelmborg, J., Vaupel, J. W., Bohr, V., et al. (2005). The heritability of telomere length among the elderly and oldest-old. Twin Res. Hum. Genet. 8, 433–439. doi: 10.1375/twin.8.5.433
Blackburn, E. H. (2001). Switching and signaling at the telomere. Cell 106, 661–673. doi: 10.1016/s0092-8674(01)00492-5
Blackburn, E. H., and Gall, J. G. (1978). A tandemly repeated sequence at the termini of the extrachromosomal ribosomal RNA genes in Tetrahymena. J. Mol. Biol. 120, 33–53. doi: 10.1016/0022-2836(78)90294-2
Bojesen, S. E., Pooley, K. A., Johnatty, S. E., Beesley, J., Michailidou, K., Tyrer, J. P., et al. (2013). Multiple independent variants at the TERT locus are associated with telomere length and risks of breast and ovarian cancer. Nat. Genet. 45, 371–384.
Broer, L., Codd, V., Nyholt, D. R., Deelen, J., Mangino, M., Willemsen, G., et al. (2013). Meta-analysis of telomere length in 19,713 subjects reveals high heritability, stronger maternal inheritance and a paternal age effect. Eur. J. Hum. Genet. 21, 1163–1168. doi: 10.1038/ejhg.2012.303
Brouilette, S., Singh, R. K., Thompson, J. R., Goodall, A. H., and Samani, N. J. (2003). White cell telomere length and risk of premature myocardial infarction. Arterioscler. Thromb. Vasc. Biol. 23, 842–846. doi: 10.1161/01.atv.0000067426.96344.32
Brouilette, S. W., Moore, J. S., Mcmahon, A. D., Thompson, J. R., Ford, I., Shepherd, J., et al. (2007). Telomere length, risk of coronary heart disease, and statin treatment in the West of Scotland Primary Prevention Study: a nested case-control study. Lancet 369, 107–114. doi: 10.1016/s0140-6736(07)60071-3
Cawthon, R. M., Smith, K. R., O’brien, E., Sivatchenko, A., and Kerber, R. A. (2003). Association between telomere length in blood and mortality in people aged 60 years or older. Lancet 361, 393–395. doi: 10.1016/s0140-6736(03)12384-7
Chang, C. C., Chow, C. C., Tellier, L. C., Vattikuti, S., Purcell, S. M., and Lee, J. J. (2015). Second-generation PLINK: rising to the challenge of larger and richer datasets. Gigascience 4:7.
Codd, V., Mangino, M., Van Der Harst, P., Braund, P. S., Kaiser, M., Beveridge, A. J., et al. (2010). Common variants near TERC are associated with mean telomere length. Nat. Genet. 42, 197–199. doi: 10.1038/ng.532
Codd, V., Nelson, C. P., Albrecht, E., Mangino, M., Deelen, J., Buxton, J. L., et al. (2013). Identification of seven loci affecting mean telomere length and their association with disease. Nat. Genet. 45, 422–427.
Deelen, J., Beekman, M., Codd, V., Trompet, S., Broer, L., Hagg, S., et al. (2014). Leukocyte telomere length associates with prospective mortality independent of immune-related parameters and known genetic markers. Int. J. Epidemiol. 43, 878–886. doi: 10.1093/ije/dyt267
Dorajoo, R., Chang, X., Gurung, R. L., Li, Z., Wang, L., Wang, R., et al. (2019). Loci for human leukocyte telomere length in the Singaporean Chinese population and trans-ethnic genetic studies. Nat. Commun. 10:2491.
Fitzpatrick, A. L., Kronmal, R. A., Gardner, J. P., Psaty, B. M., Jenny, N. S., Tracy, R. P., et al. (2007). Leukocyte telomere length and cardiovascular disease in the cardiovascular health study. Am. J. Epidemiol. 165, 14–21. doi: 10.1093/aje/kwj346
Fitzpatrick, A. L., Kronmal, R. A., Kimura, M., Gardner, J. P., Psaty, B. M., Jenny, N. S., et al. (2011). Leukocyte telomere length and mortality in the cardiovascular health study. J. Gerontol. A Biol. Sci. Med. Sci. 66, 421–429.
Greenwell, P. W., Kronmal, S. L., Porter, S. E., Gassenhuber, J., Obermaier, B., and Petes, T. D. (1995). TEL1, a gene involved in controlling telomere length in S. cerevisiae, is homologous to the human ataxia telangiectasia gene. Cell 82, 823–829. doi: 10.1016/0092-8674(95)90479-4
Grodstein, F., Van Oijen, M., Irizarry, M. C., Rosas, H. D., Hyman, B. T., Growdon, J. H., et al. (2008). Shorter telomeres may mark early risk of dementia: preliminary analysis of 62 participants from the nurses’ health study. PLoS One 3:e1590. doi: 10.1371/journal.pone.0001590
Harris, S. E., Deary, I. J., Macintyre, A., Lamb, K. J., Radhakrishnan, K., Starr, J. M., et al. (2006). The association between telomere length, physical health, cognitive ageing, and mortality in non-demented older people. Neurosci. Lett. 406, 260–264. doi: 10.1016/j.neulet.2006.07.055
Hastie, N. D., Dempster, M., Dunlop, M. G., Thompson, A. M., Green, D. K., and Allshire, R. C. (1990). Telomere reduction in human colorectal carcinoma and with ageing. Nature 346, 866–868. doi: 10.1038/346866a0
Haycock, P. C., Heydon, E. E., Kaptoge, S., Butterworth, A. S., Thompson, A., and Willeit, P. (2014). Leucocyte telomere length and risk of cardiovascular disease: systematic review and meta-analysis. BMJ 349:g4227. doi: 10.1136/bmj.g4227
Helgason, H., Rafnar, T., Olafsdottir, H. S., Jonasson, J. G., Sigurdsson, A., Stacey, S. N., et al. (2015). Loss-of-function variants in ATM confer risk of gastric cancer. Nat. Genet. 47, 906–910. doi: 10.1038/ng.3342
Honig, L. S., Kang, M. S., Schupf, N., Lee, J. H., and Mayeux, R. (2012). Association of shorter leukocyte telomere repeat length with dementia and mortality. Arch. Neurol. 69, 1332–1339.
Houben, J. M., Giltay, E. J., Rius-Ottenheim, N., Hageman, G. J., and Kromhout, D. (2011). Telomere length and mortality in elderly men: the zutphen elderly study. J. Gerontol. A Biol. Sci. Med. Sci. 66, 38–44. doi: 10.1093/gerona/glq164
Ikram, M. A., Brusselle, G. G. O., Murad, S. D., Van Duijn, C. M., Franco, O. H., Goedegebure, A., et al. (2017). The Rotterdam Study: 2018 update on objectives, design and main results. Eur. J. Epidemiol. 32, 807–850. doi: 10.1007/s10654-017-0321-4
Khera, A. V., Won, H. H., Peloso, G. M., O’dushlaine, C., Liu, D., Stitziel, N. O., et al. (2017). Association of rare and common variation in the lipoprotein lipase gene with coronary artery disease. JAMA 317, 937–946.
Kimura, M., Hjelmborg, J. V., Gardner, J. P., Bathum, L., Brimacombe, M., Lu, X., et al. (2008). Telomere length and mortality: a study of leukocytes in elderly Danish twins. Am. J. Epidemiol. 167, 799–806. doi: 10.1093/aje/kwm380
Lee, S. S., Bohrson, C., Pike, A. M., Wheelan, S. J., and Greider, C. W. (2015). ATM kinase is required for telomere elongation in mouse and human cells. Cell Rep. 13, 1623–1632. doi: 10.1016/j.celrep.2015.10.035
Levy, D., Neuhausen, S. L., Hunt, S. C., Kimura, M., Hwang, S. J., Chen, W., et al. (2010). Genome-wide association identifies OBFC1 as a locus involved in human leukocyte telomere biology. Proc. Natl. Acad. Sci. U.S.A. 107, 9293–9298. doi: 10.1073/pnas.0911494107
Lindsey, J., Mcgill, N. I., Lindsey, L. A., Green, D. K., and Cooke, H. J. (1991). In vivo loss of telomeric repeats with age in humans. Mutat. Res. 256, 45–48. doi: 10.1016/0921-8734(91)90032-7
Mangino, M., Christiansen, L., Stone, R., Hunt, S. C., Horvath, K., Eisenberg, D. T., et al. (2015). DCAF4, a novel gene associated with leucocyte telomere length. J. Med. Genet. 52, 157–162. doi: 10.1136/jmedgenet-2014-102681
Mangino, M., Hwang, S. J., Spector, T. D., Hunt, S. C., Kimura, M., Fitzpatrick, A. L., et al. (2012). Genome-wide meta-analysis points to CTC1 and ZNF676 as genes regulating telomere homeostasis in humans. Hum. Mol. Genet. 21, 5385–5394. doi: 10.1093/hmg/dds382
Manolio, T. A., Collins, F. S., Cox, N. J., Goldstein, D. B., Hindorff, L. A., Hunter, D. J., et al. (2009). Finding the missing heritability of complex diseases. Nature 461, 747–753.
Marioni, R. E., Harris, S. E., Shah, S., Mcrae, A. F., Von Zglinicki, T., Martin-Ruiz, C., et al. (2016). The epigenetic clock and telomere length are independently associated with chronological age and mortality. Int. J. Epidemiol. 45, 424–432. doi: 10.1093/ije/dyw041
Martin-Ruiz, C., Dickinson, H. O., Keys, B., Rowan, E., Kenny, R. A., and Von Zglinicki, T. (2006). Telomere length predicts poststroke mortality, dementia, and cognitive decline. Ann. Neurol. 60, 174–180. doi: 10.1002/ana.20869
Martin-Ruiz, C. M., Gussekloo, J., Van Heemst, D., Von Zglinicki, T., and Westendorp, R. G. (2005). Telomere length in white blood cells is not associated with morbidity or mortality in the oldest old: a population-based study. Aging Cell 4, 287–290. doi: 10.1111/j.1474-9726.2005.00171.x
McCarthy, S., Das, S., Kretzschmar, W., Delaneau, O., Wood, A. R., Teumer, A., et al. (2016). A reference panel of 64,976 haplotypes for genotype imputation. Nat. Genet. 48, 1279–1283. doi: 10.1038/ng.3643
Metcalfe, J. A., Parkhill, J., Campbell, L., Stacey, M., Biggs, P., Byrd, P. J., et al. (1996). Accelerated telomere shortening in ataxia telangiectasia. Nat. Genet. 13, 350–353. doi: 10.1038/ng0796-350
Needham, B. L., Rehkopf, D., Adler, N., Gregorich, S., Lin, J., Blackburn, E. H., et al. (2015). Leukocyte telomere length and mortality in the national health and nutrition examination survey, 1999-2002. Epidemiology 26, 528–535. doi: 10.1097/ede.0000000000000299
Njajou, O. T., Hsueh, W. C., Blackburn, E. H., Newman, A. B., Wu, S. H., Li, R., et al. (2009). Association between telomere length, specific causes of death, and years of healthy life in health, aging, and body composition, a population-based cohort study. J. Gerontol. A Biol. Sci. Med. Sci. 64, 860–864. doi: 10.1093/gerona/glp061
Okuda, K., Bardeguez, A., Gardner, J. P., Rodriguez, P., Ganesh, V., Kimura, M., et al. (2002). Telomere length in the newborn. Pediatr. Res. 52, 377–381.
Pandita, T. K. (2002). ATM function and telomere stability. Oncogene 21, 611–618. doi: 10.1038/sj.onc.1205060
Pardo, L. M., Mackay, I., Oostra, B., Van Duijn, C. M., and Aulchenko, Y. S. (2005). The effect of genetic drift in a young genetically isolated population. Ann. Hum. Genet. 69, 288–295. doi: 10.1046/j.1469-1809.2005.00162.x
Peters, M. J., Joehanes, R., Pilling, L. C., Schurmann, C., Conneely, K. N., Powell, J., et al. (2015). The transcriptional landscape of age in human peripheral blood. Nat. Commun. 6:8570.
Pooley, K. A., Bojesen, S. E., Weischer, M., Nielsen, S. F., Thompson, D., Amin Al Olama, A., et al. (2013). A genome-wide association scan (GWAS) for mean telomere length within the COGS project: identified loci show little association with hormone-related cancer risk. Hum. Mol. Genet. 22, 5056–5064. doi: 10.1093/hmg/ddt355
Ransohoff, K. J., Wu, W., Cho, H. G., Chahal, H. C., Lin, Y., Dai, H. J., et al. (2017). Two-stage genome-wide association study identifies a novel susceptibility locus associated with melanoma. Oncotarget 8, 17586–17592.
Rode, L., Nordestgaard, B. G., and Bojesen, S. E. (2015). Peripheral blood leukocyte telomere length and mortality among 64,637 individuals from the general population. J. Natl. Cancer Inst. 107:djv074.
Samani, N. J., Burton, P., Mangino, M., Ball, S. G., Balmforth, A. J., Barrett, J., et al. (2005). A genomewide linkage study of 1,933 families affected by premature coronary artery disease: the british heart foundation (BHF) family heart study. Am. J. Hum. Genet. 77, 1011–1020. doi: 10.1086/498653
Samani, N. J., Erdmann, J., Hall, A. S., Hengstenberg, C., Mangino, M., Mayer, B., et al. (2007). Genomewide association analysis of coronary artery disease. N. Engl. J. Med. 357, 443–453.
Savitsky, K., Bar-Shira, A., Gilad, S., Rotman, G., Ziv, Y., Vanagaite, L., et al. (1995). A single ataxia telangiectasia gene with a product similar to PI-3 kinase. Science 268, 1749–1753. doi: 10.1126/science.7792600
Scelo, G., Purdue, M. P., Brown, K. M., Johansson, M., Wang, Z., Eckel-Passow, J. E., et al. (2017). Genome-wide association study identifies multiple risk loci for renal cell carcinoma. Nat. Commun. 8:15724.
Slagboom, P. E., Droog, S., and Boomsma, D. I. (1994). Genetic determination of telomere size in humans: a twin study of three age groups. Am. J. Hum. Genet. 55, 876–882.
Strandberg, T. E., Saijonmaa, O., Tilvis, R. S., Pitkala, K. H., Strandberg, A. Y., Miettinen, T. A., et al. (2011). Association of telomere length in older men with mortality and midlife body mass index and smoking. J. Gerontol. A Biol. Sci. Med. Sci. 66, 815–820. doi: 10.1093/gerona/glr064
Tong, A. S., Stern, J. L., Sfeir, A., Kartawinata, M., De Lange, T., Zhu, X. D., et al. (2015). ATM and ATR signaling regulate the recruitment of human telomerase to telomeres. Cell Rep. 13, 1633–1646. doi: 10.1016/j.celrep.2015.10.041
van Rooij, J. G. J., Jhamai, M., Arp, P. P., Nouwens, S. C. A., Verkerk, M., Hofman, A., et al. (2017). Population-specific genetic variation in large sequencing data sets: why more data is still better. Eur. J. Hum. Genet. 25, 1173–1175. doi: 10.1038/ejhg.2017.110
Vasa-Nicotera, M., Brouilette, S., Mangino, M., Thompson, J. R., Braund, P., Clemitson, J. R., et al. (2005). Mapping of a major locus that determines telomere length in humans. Am. J. Hum. Genet. 76, 147–151. doi: 10.1086/426734
Willeit, P., Willeit, J., Brandstatter, A., Ehrlenbach, S., Mayr, A., Gasperi, A., et al. (2010a). Cellular aging reflected by leukocyte telomere length predicts advanced atherosclerosis and cardiovascular disease risk. Arterioscler. Thromb. Vasc. Biol. 30, 1649–1656. doi: 10.1161/atvbaha.110.205492
Willeit, P., Willeit, J., Kloss-Brandstatter, A., Kronenberg, F., and Kiechl, S. (2011). Fifteen-year follow-up of association between telomere length and incident cancer and cancer mortality. JAMA 306, 42–44.
Willeit, P., Willeit, J., Mayr, A., Weger, S., Oberhollenzer, F., Brandstatter, A., et al. (2010b). Telomere length and risk of incident cancer and cancer mortality. JAMA 304, 69–75.
Willer, C. J., Li, Y., and Abecasis, G. R. (2010). METAL: fast and efficient meta-analysis of genomewide association scans. Bioinformatics 26, 2190–2191. doi: 10.1093/bioinformatics/btq340
Wilson, W. R., Herbert, K. E., Mistry, Y., Stevens, S. E., Patel, H. R., Hastings, R. A., et al. (2008). Blood leucocyte telomere DNA content predicts vascular telomere DNA content in humans with and without vascular disease. Eur. Heart J. 29, 2689–2694. doi: 10.1093/eurheartj/ehn386
Zhan, X., Hu, Y., Li, B., Abecasis, G. R., and Liu, D. J. (2016). RVTESTS: an efficient and comprehensive tool for rare variant association analysis using sequence data. Bioinformatics 32, 1423–1426. doi: 10.1093/bioinformatics/btw079
Keywords: telomere, aging, whole exome sequencing, meta-analysis, ATM, RPL8
Citation: van der Spek A, Warner SC, Broer L, Nelson CP, Vojinovic D, Ahmad S, Arp PP, Brouwer RWW, Denniff M, van den Hout MCGN, van Rooij JGJ, Kraaij R, van IJcken WFJ, Samani NJ, Ikram MA, Uitterlinden AG, Codd V, Amin N and van Duijn CM (2020) Exome Sequencing Analysis Identifies Rare Variants in ATM and RPL8 That Are Associated With Shorter Telomere Length. Front. Genet. 11:337. doi: 10.3389/fgene.2020.00337
Received: 17 September 2019; Accepted: 20 March 2020;
Published: 30 April 2020.
Edited by:
Serena Sanna, Institute for Genetic and Biomedical Research (IRGB), ItalyReviewed by:
Eleonora Porcu, Université de Lausanne, SwitzerlandNabila Bouatia-Naji, Institut National de la Santé et de la Recherche Médicale (INSERM), France
Copyright © 2020 van der Spek, Warner, Broer, Nelson, Vojinovic, Ahmad, Arp, Brouwer, Denniff, van den Hout, van Rooij, Kraaij, van IJcken, Samani, Ikram, Uitterlinden, Codd, Amin and van Duijn. This is an open-access article distributed under the terms of the Creative Commons Attribution License (CC BY). The use, distribution or reproduction in other forums is permitted, provided the original author(s) and the copyright owner(s) are credited and that the original publication in this journal is cited, in accordance with accepted academic practice. No use, distribution or reproduction is permitted which does not comply with these terms.
*Correspondence: Cornelia M. van Duijn, Q29ybmVsaWEudmFuRHVpam5AbmRwaC5veC5hYy51aw==