- Department of Trauma and Emergency Surgeon, The Second Affiliated Hospital, Harbin Medical University, Harbin, China
Osteoporosis is a common complex human disease. Until now, large-scale genome-wide association studies (GWAS) using single genetic variant have reported some novel osteoporosis susceptibility variants. However, these risk variants only explain a small proportion of osteoporosis genetic risk, and most genetic risk is largely unknown. Interestingly, the pathway analysis method has been used in investigation of osteoporosis mechanisms and reported some novel pathways. Until now, it remains unclear whether there are other risk pathways involved in BMD. Here, we selected a lip BMD GWAS with 301,019 SNPs in 5,858 Europeans, and conducted a gene-based analysis (SET SCREEN TEST) and a pathway-based analysis (WebGestalt). On the gene level, BMD susceptibility genes reported by previous GWAS were identified to be the top 10 significant signals. On the pathway level, we identified 27 significant KEGG pathways. Three immune pathways including T cell receptor signaling pathway (hsa04660), complement and coagulation cascades (hsa04610), and intestinal immune network for IgA production (hsa04672) are ranked the top three significant signals. Evidence from the PubMed and Google Scholar databases further supports our findings. In summary, our findings provide complementary information to these nine risk pathways.
Introduction
Osteoporosis is a complex disease with reduced bone mineral density (BMD) and increased fracture risk (Wei et al., 2016). Until now, large-scale genome-wide association studies (GWAS) based on single genetic variant have reported some novel osteoporosis susceptibility variants (Guo et al., 2010; Richards et al., 2012; Wei et al., 2016). These osteoporosis genetic variants include rs2062375, rs13182402, rs7605378, rs12775980, rs494453, and rs784288 (Guo et al., 2010; Hsu et al., 2010; Kou et al., 2011; Hwang et al., 2013; Taylor et al., 2016). Meanwhile, some BMD related genetic variants were also identified (Kemp et al., 2017; Kim, 2018; Styrkarsdottir et al., 2019). In 2017, Kemp et al. performed a GWAS of heel BMD using 142,487 individuals from the UK Biobank (Kemp et al., 2017). They identified 307 conditionally independent genetic variants with genome-wide significance at 203 loci including 153 novel loci (Kemp et al., 2017). In 2018, Kim et al. conducted a GWAS of heel BMD using the data from UK Biobank, and identified 1,362 independent genetic variants with genome-wide significance around 899 loci including 613 novel loci (Kim, 2018). In 2019, Styrkarsdottir et al. performed a GWAS of hip and lumbar spine BMD, and identified 13 independent genetic variants at 12 loci (Styrkarsdottir et al., 2019).
However, these risk variants only explain a small proportion of osteoporosis genetic risk, and most genetic risk is largely unknown (Wei et al., 2016). Considering the limitations of GWAS on single genetic variant level, some improved method named pathway analysis of GWAS dataset or gene set analysis has been proposed and widely used in multiple human complex diseases (Eleftherohorinou et al., 2009; Hong et al., 2010; Liu et al., 2012; Holmans et al., 2013; Liu et al., 2013; Liu et al., 2014; Quan et al., 2015; Jiang et al., 2017; Liu et al., 2017).
Interestingly, the pathway analysis method has also been used in investigation of osteoporosis mechanisms and reported some novel pathways (Zhang et al., 2010; Lee et al., 2012; Wei et al., 2016). In addition to these risk pathways above, it remains unclear whether there are other risk pathways involved in BMD. Hence, we conducted a pathway analysis of hip BMD GWAS with 301,019 SNPs in 5,858 Europeans using a published gene-based analysis method (SET SCREEN TEST) (Moskvina et al., 2011) and a pathway-based analysis method (WebGestalt) (Zhang et al., 2005).
Materials and Methods
The BMD GWAS Dataset
This study included 5,861 Icelandic persons, and 5,858 of 5,861 persons had measurements of hip bone mineral density (Styrkarsdottir et al., 2008). For each DNA sample, the Infinium HumanHap300 or the HumanCNV370 SNP chip (Illumina) was used to genotype a total of 317,503 genetic variants (Styrkarsdottir et al., 2008). After quality control, the GWAS dataset finally included 301,019 common genetic variants (Styrkarsdottir et al., 2008). In order to evaluate the association between each genetic variant and BMD, a linear regression method was utilized (Styrkarsdottir et al., 2008). Here, we used the summary results from this BMD GWAS (Styrkarsdottir et al., 2008). More detailed results are described in the original study (Styrkarsdottir et al., 2008).
Gene-Based Testing for BMD GWAS by a Meta-Analysis Method
We selected a set screen test method implemented in PLINK to perform a gene-based test of the whole GWAS dataset (Moskvina et al., 2011). The method could combine all P values from all the genetic variants in each corresponding gene by an approximate Fisher’s test and could also adjust for the linkage disequilibrium (LD) (Moskvina et al., 2011).
For all genetic variants in a specific gene, if all these genetic variants are independent, the Fisher’s statistic could be calculated by
where N is the number of selected genetic variants in a specific gene and pi (i = 1, …, N) is the significance level about the association each genetic variant with BMD, x02 follows a chi-square distribution with the freedom degrees = 2N (Moskvina et al., 2011).
If the selected genetic variants are not independent,
where x2 follows the central chi-square distribution with 8N2/σ2 as degrees of freedom (Moskvina et al., 2011). Here, the LD information is from the HapMap CEU population.
Pathway-Based Testing for BMD GWAS Dataset
Here, we conducted a KEGG pathway analysis of the BMD risk genes using WebGestalt (Zhang et al., 2005). The enrichment analysis was performed using the hypergeometric test (Zhang et al., 2005). Here, we selected the entire entrez gene set as the reference gene assuming including N genes. We first assume that we have identified S BMD risk genes using the gene-base test. For a given pathway, we further assume that this pathway includes m genes and K BMD risk genes. The significant levels observing K BMD risk genes in a specific pathway is
Meanwhile, we limited the pathway analysis using the KEGG pathways with at least 20 and at most 300 genes. A false discovery rate (FDR) method was used to adjust the original significance levels. Here, we define a pathway with adjusted P < 0.01 to be statistically significant.
Results
Gene-Based Test for BMD GWAS Dataset
Using the gene-based test, several BMD susceptibility genes reported by previous GWAS were among the top 10 significant signals, which included CKAP5 (the most significant signal with P = 4.03E-07) (Styrkarsdottir et al., 2009), LRP4 (the most significant signal with P = 6.72E-05) (Styrkarsdottir et al., 2009), and C6orf97 (the 6th significant signal with P = 1.04E-04) (Styrkarsdottir et al., 2009). Meanwhile, we identified other new BMD susceptibility genes, such as F2 (the second significant signal with P = 1.58E-05), LALBA (the 4th significant signal with P = 6.86E-05), and CPN2 (the 5th significant signal with P = 7.21E-05). Detailed information about the 5% significant genes is described in Supplementary Table 1.
Pathway-Based Test for BMD GWAS Dataset
We used the top 5% significant signals (14,008*5% = 700 genes with P < 0.04239) from the gene-based test for following pathway analysis. We identified 27 significant KEGG pathways (adjusted P < 0.01) (Table 1). Based on the function classifications, these pathways are mainly related to immunity, cellular processes, environment, infection, cardiovascular diseases, metabolism, and circulation. The top three significant signals are related with immune system including T cell receptor signaling pathway (hsa04660), complement and coagulation cascades (hsa04610), and intestinal immune network for IgA production (hsa04672) as described in Table 1. The detailed information is provided in Supplementary Table 2.
Verification by PubMed and Google Scholar Literature Search
We further verified these findings from pathway analysis using publicly available literatures in PubMed and Google Scholar databases. Interestingly, growing evidence supports the involvement of dilated cardiomyopathy and hypertrophic cardiomyopathy, T cell receptor signaling pathway, wnt signaling pathway, and regulation of actin cytoskeleton in MBD. More detailed information is described in Table 2.
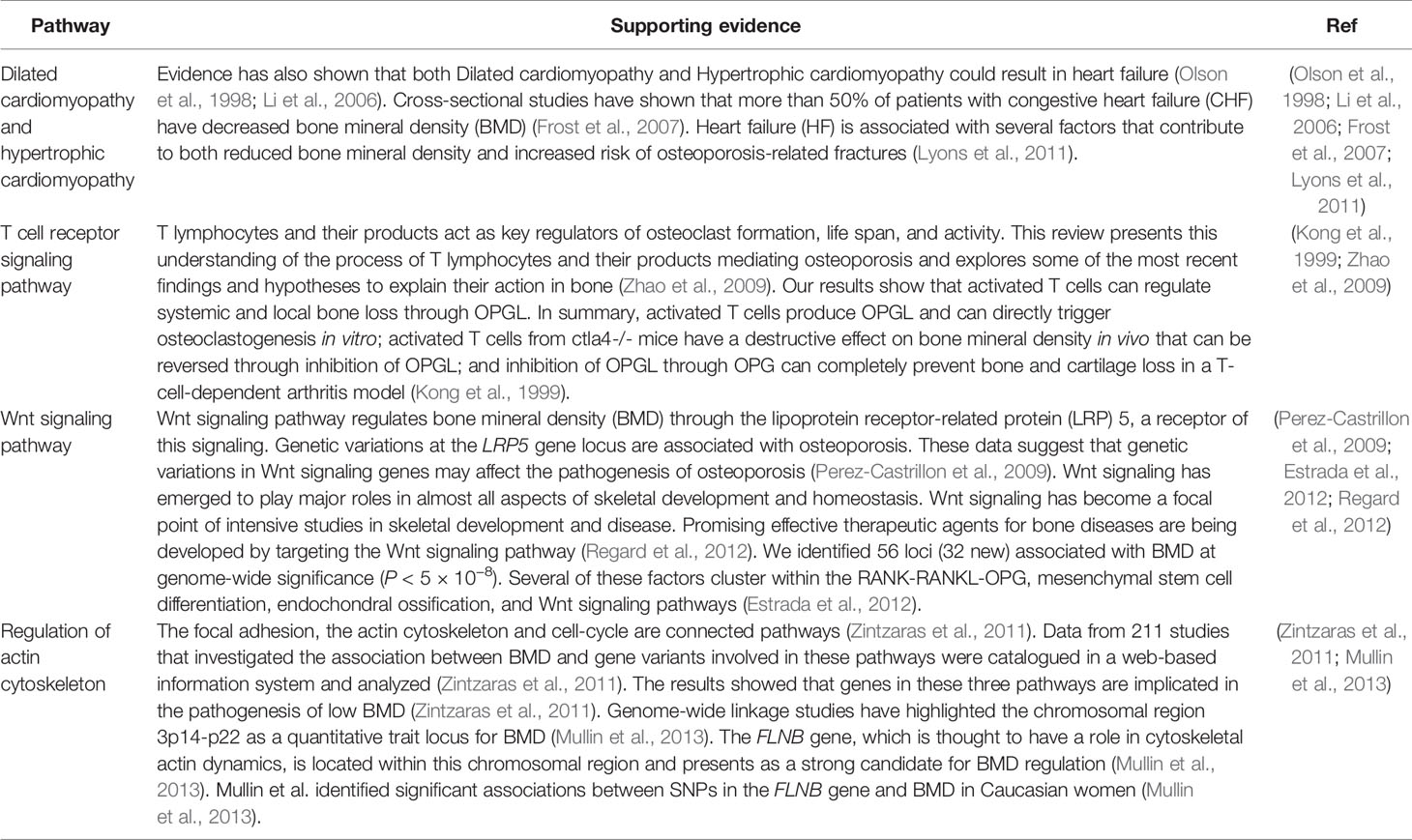
Table 2 Literature evidences supporting that genes in measles pathway are associated with bone mineral density or osteoporosis.
Discussion
Until now, pathway analysis of BMD GWAS datasets has identified several risk pathways. However, most genetic variants, risk genes, and genetic pathways influencing osteoporosis are unknown. In order to identify novel BMD risk pathways, we conducted a pathway analysis of hip BMD GWAS with 301,019 genetic variants in 5,858 Europeans using the meta-analysis method (SET SCREEN TEST) in PLINK (Moskvina et al., 2011) and a pathway-based analysis method (WebGestalt) (Zhang et al., 2005).
On the gene level, we confirmed previous identified BMD susceptibility genes such as CKAP5 (Styrkarsdottir et al., 2009), LRP4 (Styrkarsdottir et al., 2009), and C6orf97 (Styrkarsdottir et al., 2009). All these genes are ranked top 10 significant signals. We also identified some new BMD susceptibility genes, which were significantly associated with BMD with P < 0.0001. Take the second significant signal F2 for example, F2 could encode coagulation factor II, thrombin (Sato et al., 2016). Pro-thrombin is cut to generate thrombin in the coagulation cascade, and further reduce the blood loss (Sato et al., 2016). It is common that procoagulant state could active thrombin in bone fracture sites (Sato et al., 2016). Take CPN2 for example, two genetic variants rs11711157 and rs3732477 near CPN2 have been reported to be associated with BMD in Koreans (Kim et al., 2013). These findings suggest that the meta-analysis method (SET SCREEN TEST) is effective to identify BMD susceptibility genes.
On the pathway level, we identified 27 significant KEGG pathways. Three immune pathways including T cell receptor signaling pathway (hsa04660), complement and coagulation cascades (hsa04610), and intestinal immune network for IgA production (hsa04672) are ranked the top three significant signals. In addition, we also identified other kinks of pathways associated with cellular processes, environmental information processing, infectious diseases, cardiovascular diseases, metabolism, and circulatory system, are also identified. In order to further evaluate the potential roles of these newly identified risk pathways, we conducted a comprehensive literature review. Interestingly, growing evidence from the PubMed and Google Scholar databases further supports the involvement of dilated cardiomyopathy, hypertrophic cardiomyopathy, T cell receptor signaling pathway, wnt signaling pathway, and regulation of actin cytoskeleton in MBD, as provided in Table 2.
In a recent study, Guo et al. conducted a pathway and network analysis of genes related to osteoporosis (Guo et al., 2019). They first retrieved 94 osteoporosis genes from PubMed (Guo et al., 2019). They further conducted an enrichment analysis, and found that these osteoporosis genes were significantly enriched in biological processes related to bone metabolism and the immune system (Guo et al., 2019). Take wnt signaling pathway for example, we identified wnt signaling to be the 7th significant pathway, as provided in Table 1. Interestingly, Guo et al. identified wnt signaling to be the second significant pathway with P = 3.71 × 10−13 (Guo et al., 2019). Hence, our findings are consistent with recent findings.
Our study has a main difference with previous studies. In recent years, most studies focus on the single genetic variant associated with BMD, osteoporosis, or fracture (Kemp et al., 2017; Kim, 2018; Styrkarsdottir et al., 2019). However, these genetic variants only explain a small proportion of osteoporosis genetic risk (Wei et al., 2016). Hence, pathway analysis of GWAS dataset may overcome the limitations of single genetic variant. However, our current study still has some limitations. First, the sample size is relatively small and lacks validation cohort to test the robustness of these findings. In future study, we will further select other BMD GWAS datasets with large-scale sample size to demonstrate our findings. Second, we only selected one gene based method and one pathway based method. In future, we will verify our findings using other gene and pathway based methods. Third, we selected the top 5% significant signals from the gene-based test for following pathway analysis, as did in recent studies (Yoon et al., 2018; Ierodiakonou et al., 2019; White et al., 2019). Using this strategy, we selected 700 BMD risk genes with P < 0.04239. In fact, there are a total of 813 BMD risk genes with P < 0.05. Hence, our current pathway analysis findings are consistent with those using 813 BMD risk genes (data now shown). However, selection of different thresholds may have different findings, as described in recent studies (Yoon et al., 2018; Ierodiakonou et al., 2019; White et al., 2019).
In summary, two studies reported regulation-of-autophagy and other eight significant pathways (Zhang et al., 2010; Lee et al., 2012). Our findings provide complementary information to these nine risk pathways. Meanwhile, future studies using large-scale sample sizes should further verify our findings.
Data Availability Statement
Publicly available datasets were analyzed in this study. This data can be found here: https://www.nejm.org/doi/10.1056/NEJMoa0801197.
Author Contributions
FG conceived and initiated the project. FG and XL analyzed the data. All authors wrote and reviewed the manuscript.
Funding
This work was supported by funding from Heilongjiang Natural Science Foundation (Grant No. H2016025).
Conflict of Interest
The authors declare that the research was conducted in the absence of any commercial or financial relationships that could be construed as a potential conflict of interest.
Supplementary Material
The Supplementary Material for this article can be found online at: https://www.frontiersin.org/articles/10.3389/fgene.2020.00004/full#supplementary-material
References
Eleftherohorinou, H., Wright, V., Hoggart, C., Hartikainen, A. L., Jarvelin, M. R., Balding, D., et al. (2009). Pathway analysis of GWAS provides new insights into genetic susceptibility to 3 inflammatory diseases. PloS One 4, e8068. doi: 10.1371/journal.pone.0008068
Estrada, K., Styrkarsdottir, U., Evangelou, E., Hsu, Y. H., Duncan, E. L., Ntzani, E. E., et al. (2012). Genome-wide meta-analysis identifies 56 bone mineral density loci and reveals 14 loci associated with risk of fracture. Nat. Genet. 44, 491–501. doi: 10.1038/ng.2249
Frost, R. J., Sonne, C., Wehr, U., Stempfle, H. U. (2007). Effects of calcium supplementation on bone loss and fractures in congestive heart failure. Eur. J. Endocrinol. 156, 309–314. doi: 10.1530/EJE-06-0614
Guo, Y., Tan, L. J., Lei, S. F., Yang, T. L., Chen, X. D., Zhang, F., et al. (2010). Genome-wide association study identifies ALDH7A1 as a novel susceptibility gene for osteoporosis. PloS Genet. 6, e1000806. doi: 10.1371/journal.pgen.1000806
Guo, L., Han, J., Guo, H., Lv, D., Wang, Y. (2019). Pathway and network analysis of genes related to osteoporosis. Mol. Med. Rep. 20, 985–994. doi: 10.3892/mmr.2019.10353
Holmans, P., Moskvina, V., Jones, L., Sharma, M., Vedernikov, A., Buchel, F., et al. (2013). A pathway-based analysis provides additional support for an immune-related genetic susceptibility to Parkinson’s disease. Hum. Mol. Genet. 22, 1039–1049. doi: 10.1093/hmg/dds492
Hong, M. G., Alexeyenko, A., Lambert, J. C., Amouyel, P., Prince, J. A. (2010). Genome-wide pathway analysis implicates intracellular transmembrane protein transport in Alzheimer disease. J. Hum. Genet. 55, 707–709. doi: 10.1038/jhg.2010.92
Hsu, Y. H., Zillikens, M. C., Wilson, S. G., Farber, C. R., Demissie, S., Soranzo, N., et al. (2010). An integration of genome-wide association study and gene expression profiling to prioritize the discovery of novel susceptibility Loci for osteoporosis-related traits. PloS Genet. 6, e1000977. doi: 10.1371/journal.pgen.1000977
Hwang, J. Y., Lee, S. H., Go, M. J., Kim, B. J., Kou, I., Ikegawa, S., et al. (2013). Meta-analysis identifies a MECOM gene as a novel predisposing factor of osteoporotic fracture. J. Med. Genet. 50, 212–219. doi: 10.1136/jmedgenet-2012-101156
Ierodiakonou, D., Coull, B. A., Zanobetti, A., Postma, D. S., Boezen, H. M., Vonk, J. M., et al. (2019). Pathway analysis of a genome-wide gene by air pollution interaction study in asthmatic children. J. Expo. Sci. Environ. Epidemiol. 29, 539–547. doi: 10.1038/s41370-019-0136-3
Jiang, Q., Jin, S., Jiang, Y., Liao, M., Feng, R., Zhang, L., et al. (2017). Alzheimer’s disease variants with the genome-wide significance are significantly enriched in immune pathways and active in immune cells. Mol. Neurobiol. 54, 594–600. doi: 10.1007/s12035-015-9670-8
Kemp, J. P., Morris, J. A., Medina-Gomez, C., Forgetta, V., Warrington, N. M., Youlten, S. E., et al. (2017). Identification of 153 new loci associated with heel bone mineral density and functional involvement of GPC6 in osteoporosis. Nat. Genet. 49, 1468–1475. doi: 10.1038/ng.3949
Kim, Y. A., Choi, H. J., Lee, J. Y., Han, B. G., Shin, C. S., Cho, N. H. (2013). Replication of Caucasian loci associated with bone mineral density in Koreans. Osteoporos. Int. 24, 2603–2610. doi: 10.1007/s00198-013-2354-1
Kim, S. K. (2018). Identification of 613 new loci associated with heel bone mineral density and a polygenic risk score for bone mineral density, osteoporosis and fracture. PloS One 13, e0200785. doi: 10.1371/journal.pone.0200785
Kong, Y. Y., Feige, U., Sarosi, I., Bolon, B., Tafuri, A., Morony, S., et al. (1999). Activated T cells regulate bone loss and joint destruction in adjuvant arthritis through osteoprotegerin ligand. Nature 402, 304–309. doi: 10.1038/46303
Kou, I., Takahashi, A., Urano, T., Fukui, N., Ito, H., Ozaki, K., et al. (2011). Common variants in a novel gene, FONG on chromosome 2q33.1 confer risk of osteoporosis in Japanese. PloS One 6, e19641. doi: 10.1371/journal.pone.0019641
Lee, Y. H., Choi, S. J., Ji, J. D., Song, G. G. (2012). Pathway analysis of genome-wide association study for bone mineral density. Mol. Biol. Rep. 39, 8099–8106. doi: 10.1007/s11033-011-1407-9
Li, D., Parks, S. B., Kushner, J. D., Nauman, D., Burgess, D., Ludwigsen, S., et al. (2006). Mutations of presenilin genes in dilated cardiomyopathy and heart failure. Am. J. Hum. Genet. 79, 1030–1039. doi: 10.1086/509900
Liu, G., Jiang, Y., Wang, P., Feng, R., Jiang, N., Chen, X., et al. (2012). Cell adhesion molecules contribute to Alzheimer’s disease: multiple pathway analyses of two genome-wide association studies. J. Neurochem. 120, 190–198. doi: 10.1111/j.1471-4159.2011.07547.x
Liu, G., Jiang, Y., Chen, X., Zhang, R., Ma, G., Feng, R., et al. (2013). Measles contributes to rheumatoid arthritis: evidence from pathway and network analyses of genome-wide association studies. PloS One 8, e75951. doi: 10.1371/journal.pone.0075951
Liu, G., Yao, L., Liu, J., Jiang, Y., Ma, G., Chen, Z., et al. (2014). Cardiovascular disease contributes to Alzheimer’s disease: evidence from large-scale genome-wide association studies. Neurobiol. Aging 35, 786–792. doi: 10.1016/j.neurobiolaging.2013.10.084
Liu, G., Zhang, F., Jiang, Y., Hu, Y., Gong, Z., Liu, S., et al. (2017). Integrating genome-wide association studies and gene expression data highlights dysregulated multiple sclerosis risk pathways. Mult. Scler. 23, 205–212. doi: 10.1177/1352458516649038
Lyons, K. J., Majumdar, S. R., Ezekowitz, J. A. (2011). The unrecognized burden of osteoporosis-related vertebral fractures in patients with heart failure. Circ. Heart Fail 4, 419–424. doi: 10.1161/CIRCHEARTFAILURE.111.961185
Moskvina, V., O’dushlaine, C., Purcell, S., Craddock, N., Holmans, P., O’donovan, M. C. (2011). Evaluation of an approximation method for assessment of overall significance of multiple-dependent tests in a genomewide association study. Genet. Epidemiol. 35, 861–866. doi: 10.1002/gepi.20636
Mullin, B. H., Mamotte, C., Prince, R. L., Spector, T. D., Dudbridge, F., Wilson, S. G. (2013). Conditional testing of multiple variants associated with bone mineral density in the FLNB gene region suggests that they represent a single association signal. BMC Genet. 14, 107. doi: 10.1186/1471-2156-14-107
Olson, T. M., Michels, V. V., Thibodeau, S. N., Tai, Y. S., Keating, M. T. (1998). Actin mutations in dilated cardiomyopathy, a heritable form of heart failure. Science 280, 750–752. doi: 10.1126/science.280.5364.750
Perez-Castrillon, J. L., Olmos, J. M., Nan, D. N., Castillo, J., Arozamena, J., Montero, A., et al. (2009). Polymorphisms of the WNT10B gene, bone mineral density, and fractures in postmenopausal women. Calcif. Tissue Int. 85, 113–118. doi: 10.1007/s00223-009-9256-4
Quan, B., Qi, X., Yu, Z., Jiang, Y., Liao, M., Wang, G., et al. (2015). Pathway analysis of genome-wide association study and transcriptome data highlights new biological pathways in colorectal cancer. Mol. Genet. Genomics 290, 603–610. doi: 10.1007/s00438-014-0945-y
Regard, J. B., Zhong, Z., Williams, B. O., Yang, Y. (2012). Wnt signaling in bone development and disease: making stronger bone with Wnts. Cold Spring Harb. Perspect. Biol. 4, 12. doi: 10.1101/cshperspect.a007997
Richards, J. B., Zheng, H. F., Spector, T. D. (2012). Genetics of osteoporosis from genome-wide association studies: advances and challenges. Nat. Rev. Genet. 13, 576–588. doi: 10.1038/nrg3228
Sato, N., Ichikawa, J., Wako, M., Ohba, T., Saito, M., Sato, H., et al. (2016). Thrombin induced by the extrinsic pathway and PAR-1 regulated inflammation at the site of fracture repair. Bone 83, 23–34. doi: 10.1016/j.bone.2015.10.005
Styrkarsdottir, U., Halldorsson, B. V., Gretarsdottir, S., Gudbjartsson, D. F., Walters, G. B., Ingvarsson, T., et al. (2008). Multiple genetic loci for bone mineral density and fractures. N. Engl. J. Med. 358, 2355–2365. doi: 10.1056/NEJMoa0801197
Styrkarsdottir, U., Halldorsson, B. V., Gretarsdottir, S., Gudbjartsson, D. F., Walters, G. B., Ingvarsson, T., et al. (2009). New sequence variants associated with bone mineral density. Nat. Genet. 41, 15–17. doi: 10.1038/ng.284
Styrkarsdottir, U., Stefansson, O. A., Gunnarsdottir, K., Thorleifsson, G., Lund, S. H., Stefansdottir, L., et al. (2019). GWAS of bone size yields twelve loci that also affect height, BMD, osteoarthritis or fractures. Nat. Commun. 10, 2054. doi: 10.1038/s41467-019-09860-0
Taylor, K. C., Evans, D. S., Edwards, D. R. V., Edwards, T. L., Sofer, T., Li, G., et al. (2016). A genome-wide association study meta-analysis of clinical fracture in 10,012 African American women. Bone Rep. 5, 233–242. doi: 10.1016/j.bonr.2016.08.005
Wei, J., Li, M., Gao, F., Zeng, R., Liu, G., Li, K. (2016). Multiple analyses of large-scale genome-wide association study highlight new risk pathways in lumbar spine bone mineral density. Oncotarget 7, 31429–31439. doi: 10.18632/oncotarget.8948
White, M. J., Yaspan, B. L., Veatch, O. J., Goddard, P., Risse-Adams, O. S., Contreras, M. G. (2019). Strategies for pathway analysis using GWAS and WGS Data. Curr. Protoc. Hum. Genet. 100, e79. doi: 10.1002/cphg.79
Yoon, S., Nguyen, H. C. T., Yoo, Y. J., Kim, J., Baik, B., Kim, S., et al. (2018). Efficient pathway enrichment and network analysis of GWAS summary data using GSA-SNP2. Nucleic Acids Res. 46, e60. doi: 10.1093/nar/gky175
Zhang, B., Kirov, S., Snoddy, J. (2005). WebGestalt: an integrated system for exploring gene sets in various biological contexts. Nucleic Acids Res. 33, W741–W748. doi: 10.1093/nar/gki475
Zhang, L., Guo, Y. F., Liu, Y. Z., Liu, Y. J., Xiong, D. H., Liu, X. G., et al. (2010). Pathway-based genome-wide association analysis identified the importance of regulation-of-autophagy pathway for ultradistal radius BMD. J. Bone Miner. Res. 25, 1572–1580. doi: 10.1002/jbmr.36
Zhao, W., Liu, Y., Cahill, C. M., Yang, W., Rogers, J. T., Huang, X. (2009). The role of T cells in osteoporosis, an update. Int. J. Clin. Exp. Pathol. 2, 544–552.
Zintzaras, E., Doxani, C., Ziogas, D. C., Mprotsis, T., Rodopoulou, P., Karachalios, T. (2011). Bone mineral density and genetic markers involved in three connected pathways (focal adhesion, actin cytoskeleton regulation and cell cycle): the CUMAGAS-BMD information system. Biomarkers 16, 698–708. doi: 10.3109/1354750X.2011.629373
Keywords: osteoporosis, bone mineral density, genome-wide association studies, pathway analysis, immune pathways
Citation: Liu X, Zhang Y, Tian J and Gao F (2020) Analyzing Genome-Wide Association Study Dataset Highlights Immune Pathways in Lip Bone Mineral Density. Front. Genet. 11:4. doi: 10.3389/fgene.2020.00004
Received: 17 August 2019; Accepted: 06 January 2020;
Published: 10 March 2020.
Edited by:
Guiyou Liu, Tianjin Institute of Industrial Biotechnology (CAS), ChinaReviewed by:
Guangchun Han, University of Texas MD Anderson Cancer Center, United StatesYang Hu, Harbin Institute of Technology, China
Copyright © 2020 Liu, Zhang, Tian and Gao. This is an open-access article distributed under the terms of the Creative Commons Attribution License (CC BY). The use, distribution or reproduction in other forums is permitted, provided the original author(s) and the copyright owner(s) are credited and that the original publication in this journal is cited, in accordance with accepted academic practice. No use, distribution or reproduction is permitted which does not comply with these terms.
*Correspondence: Feng Gao, Z2FvZmVuZzE5NzYxMjIyQDE2My5jb20=