- 1Beijing Advanced Innovation Center for Tree Breeding by Molecular Design, National Engineering Laboratory for Tree Breeding, Key Laboratory of Genetics and Breeding in Forest Trees and Ornamental Plants, Ministry of Education, College of Biological Sciences and Technology, Beijing Forestry University, Beijing, China
- 2Key Laboratory for Forest Resources Conservation and Utilization in the Southwest Mountains of China, Southwest Forestry University, Kunming, China
- 3Department of Forest and Conservation Sciences, Faculty of Forestry, The University of British Columbia, Vancouver, BC, Canada
- 4School of Forestry, Northern Arizona University, Flagstaff, AZ, United States
Local adaptation, adaptation to specialized niches and environmental clines have been extensively reported for forest trees. Investigation of the adaptive genetic variation is crucial for forest resource management and breeding, especially in the context of global climate change. Here, we utilized a Pinus yunnanensis common garden experiments established at high and low elevation sites to assess the differences in growth and survival among populations and between the two common garden sites. The studied traits showed significant variation between the two test sites and among populations, suggesting adaptive divergence. To detect genetic variation related to environment, we captured 103,608 high quality SNPs based on RNA sequencing, and used them to assess the genetic diversity and population structure. We identified 321 outlier SNPs from 131 genes showing significant divergence in allelic frequency between survival populations of two sites. Functional categories associated with adaptation to high elevation were found to be related to flavonoid biosynthesis, response to UV, DNA repair, response to reactive oxygen species, and membrane lipid metabolic process. Further investigation of the outlier genes showed overrepresentation of the flavonoid biosynthesis pathway, suggesting that this pathway may play a key role in P. yunnanensis adaptation to high elevation environments. The outlier genes identified, and their variants, provide a basic reference for advanced investigations.
Introduction
In harsh environment such as in high elevations, natural selection may result in changes in allele frequency to maximize fitness (Hoffmann and Sgrò, 2011). Understanding the influence of natural selection on genomic variation in natural populations, and identifying the adaptive loci have received increased attention in the field of adaptive evolution and evolutionary ecology (Tiffin and Ross-Ibarra, 2014; Flood and Hancock, 2017). Organisms are adapted to diverse habitats. Highlands are characterized by intense ultraviolet radiation, low temperatures, hypoxia, and reduced pathogen incidence, providing a unique environment to study adaptation to high elevation. Survival at high elevation environments is challenging and native plants and animals have developed effective strategies through specific morphological and physiological adaptations (Monge and Leon-Velarde, 1991; Alonso-Amelot, 2008; Storz et al., 2010; Gong et al., 2018; Halbritter et al., 2018). For example, maize plants growing at high elevation often accumulate flavonoids in their leaves and silks as a mechanism for coping with high levels of UV-B exposure (Zhang et al., 2003; Casati and Walbot, 2010). The recent development of high-throughput sequencing technologies has greatly accelerated the identification of key genes and genomics research, significantly promoting the research of adaptive evolution and ecology on non-model organisms (Stapley et al., 2010; Ekblom and Galindo, 2011), including conifers (Yeaman et al., 2016; Lind et al., 2018; De La Torre and Neale, 2019; De La Torre et al., 2019; Lu et al., 2019; Tyrmi et al., 2019).
Pinus, with >100 species, is the largest conifer genus with widespread natural distribution ranging from arctic and subarctic to subtropical and tropical regions in the Northern Hemisphere (Richardson, 1999; Farjon and Farjon, 2010). Pines display diverse mountainous adaptability, with parapatric closely related species continuously distributed across varying elevation gradients (Kuan, 1981; Richardson, 1999; Farjon and Farjon, 2010). Although pines span a large elevational range, the genetics of adaptation to high elevation is still poorly understood. Pinus yunnanensis is the dominant pine in southwest China with a continuous distribution in the Yunnan–Guizhou region at elevations ranging from 700 to 3,000 m above sea level (m.a.s.l.) (Wu, 1956; Mao and Wang, 2011). P. yunnanensis morphological variation is significant across its range, and regions divided by mountain chains featuring different climatic conditions (Yu et al., 1998; Yu et al., 2000; Mao et al., 2009). Recent analysis based on maternally inherited mitochondrial (mt) and paternally inherited chloroplast (cp) DNA markers found continuous genetic differentiation over the majority of its range, and discrete isolated local clusters in the northwest and east peripheries. The discrete differentiation between the two genetic groups is coincident with their niche divergence and geographical isolation (Wang et al., 2013).
The genus Pinus and other conifers are known for their exceedingly large and complex genomes, varying from 16 to 35 Gbp (De La Torre et al., 2014; Leitch et al., 2019). Despite the decreasing costs of sequencing, whole-genome resequencing of large numbers of pine individuals is still not feasible. RNA sequencing (RNA-seq), in which the expressed part of genome is sequenced, represents a powerful alternative to whole-genome sequencing, allowing the genotyping of thousands of loci for non-model species with large genomes (Schlötterer et al., 2014; Hoban et al., 2016; Jones and Good, 2016; Oney-Birol et al., 2018). The draft genome of Pinus taeda (Wegrzyn et al., 2014; Zimin et al., 2014) may be used as a reference genome, and assist a reference-based RNA-seq approach to genotype expressed gene regions for population genomic studies.
In this study, we aim to discover the genetic variation related to adaptation to high elevation environments in P. yunnanensis, by comparing the survival of populations from common garden experiments in high and low elevations. Hundreds of thousands of single nucleotide polymorphisms (SNPs) from expressed regions including 8,595 genes were captured using RNA-Seq. Genetic diversity and population structure were resolved. SNPs, genes and pathways related to adaptation to high elevation environments were identified based on FST outlier analyses.
Materials and Methods
Sampling and Transcriptome Sequencing
In the spring of 2011, two P. yunnanensis common gardens were established at a high altitude site in Linzhi (LZ, 2,950 m.a.s.l.), Tibet and a low elevation site in Kunming (KM, 1,890 m.a.s.l.) (Figure 1), Yunnan, China. The LZ site (29°40′N, 94°20′E) is the native habitat for Pinus densata, a close relative of P. yunnanensis, and represents a high elevation environment characterized by cold and strong UV, with mean coldest-month temperature of –3.1°C, mean warmest temperature of 14.5°C, mean annual temperature of 6.5°C, average annual precipitation of 785 mm, and 185 frost-free days. While, the KM site (25°04′N, 102°46′E) is located in the central distribution of P. yunnanensis and characterized by mild, moist, and low seasonality climate, with mean coldest-month temperature of 8.1°C, mean warmest temperature of 20.2°C, mean annual temperature of 15°C, average annual precipitation of 1,035 mm and 334 frost-free days. Bulked seeds from 7 natural populations (LJ, YL, BS, GS, YX, ZD, KM) were collected to represent P. yunnanensis’ wide range of natural genetic variation (Table 1). The two common gardens were established with random block designs, with 50 or 60 seeds of each population in each block and four or five blocks for each site (LZ and KM). Survival rate (measured at 2017) and seedling height (measured at 2016) of each population were measured in the 6-7th year. In the high elevation site (LZ), all surviving trees (only five individuals) originated from the KM population were sampled (KM(LZ)). Additionally, we randomly selected 49 individuals representing 5 BS(KM), 4 GS(KM), 10 LJ(KM), 10 YL(KM), 5 YX(KM), 5 ZD(KM), and 10 KM(KM) as representatives of the low elevation site (KM) (Tables 1 and S1).
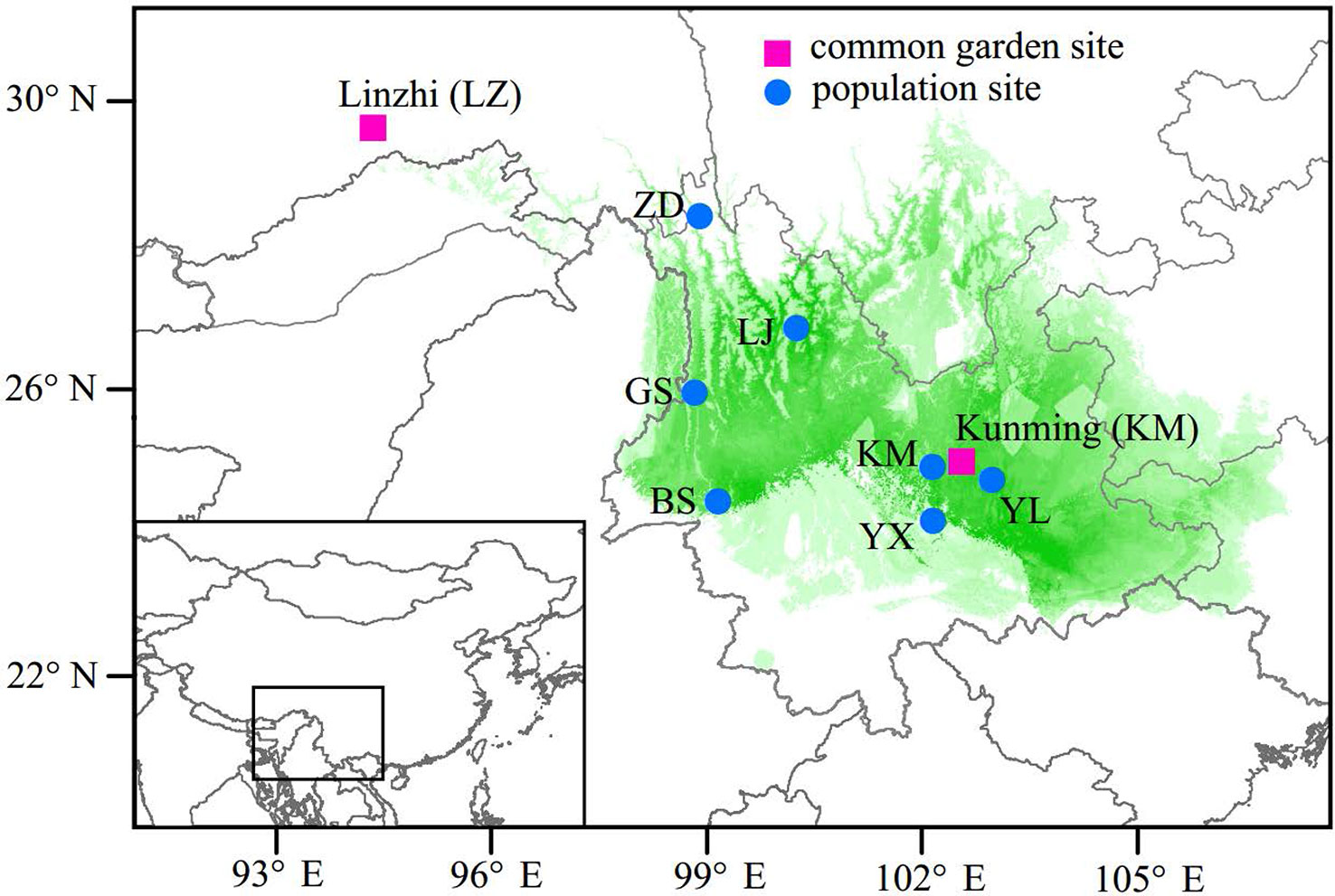
Figure 1 Geographic origins of the sampled populations and locations of the two common garden experiments. Green coloring illustrates the potential distribution of P. yunnanensis as suggested by Mao and Wang (2011).
Fresh needles from current year branches of all 54 sampled individual trees were collected for RNA-seq in June 2017, and immediately placed in liquid nitrogen and stored at –80°C until further use. Total RNAs were extracted using RNAprep pure Plant Kit (Tiangen, Beijing, China) according to the manufacturer’s protocols. NanoDrop 2000 Spectrophotometers and Agilent 2100 Bioanalyzer were used to evaluate RNA concentration and integrity. NEBNext Ultra RNA Library Prep Kit (New England BioLabs) was used for cDNA library construction. Briefly, the mRNA purification was performed with magnetic oligo (dT) beads using a Dynabeads mRNA Purification Kit (Invitrogen). The mRNA fragmentation was implemented using RNA fragmentation Kit (Ambion, Austin, TX, USA). Random hexamer primers and reverse transcriptase (Invitrogen) were used to synthesize the first-strand cDNA. Subsequently, DNA polymerase I (New England BioLabs) and RNaseH (Invitrogen) were used to synthesize the second-strand cDNA. Adapter was ligated to the double strand cDNA fragments with a single ‘A’ addition after end repair. Approximately 450 bp cDNA fragments were selected using Ampure XP beads (Beckman). The selected cDNA fragments were PCR amplified to complete library preparation. The concentration and fragment size of the cDNA library were assayed using Real-Time PCR system and Agilent 2100 Bioanalyzer, respectively. The library was sequenced on an Illumina HiSeq X Ten sequencing platform to generate 150 bp paired end raw reads.
Sequence Quality Control and Mapping
Raw reads were filtered and trimmed using Trimmomatic (Bolger et al., 2014) to remove adapter sequences and low-quality bases (Phred quality <20) from either the start or the end of the reads. After trimming, reads shorter than 36 bases were completely discarded. FastQC (http://www.bioinformatics.babraham.ac.uk/projects/fastqc/) was used to assess the quality of the raw and clean sequence data. Clean reads from each sample were mapped to the P. taeda reference genome v1.01 (Wegrzyn et al., 2014; Zimin et al., 2014) using STAR (Dobin et al., 2013). Single nucleotide polymorphism (SNP) calling was first performed using SAMtools (Li et al., 2009) and BCFtools (Li et al., 2009) with default settings. Based on the distribution of SNPs gained in the first calling, a reduced reference genome consisting of all 57,783 scaffolds with at least 1 SNP with missing rate <90% was created to decrease the computational load in the following steps. Reduced sequence alignment (in BAM format) files where only conveying reads mapped to the reduced reference genome were generated for SNP calling using the Genome Analysis Tool Kit (GATK v3.7-0) (DePristo et al., 2011). The command, “AddOrReplaceReadGroups” from GATK was used for adding of read group information and further sorting. PCR duplicates were removed using “MarkDuplicates” from Picard (http://broadinstitute.github.io/picard/). A GATK command, “SplitNCigarReads”, was used to split reads spanning splice junctions, and reassign mapping qualities to all good alignments. To minimize the mis-alignment of bases around insertions and/or deletions (indels), local realignment around indels was performed using RealignerTargetCreator and IndelRealigner from GATK. The final BAM files were produced from the local realignment for further analysis.
Variant Calling and Filtering
SNP calling was performed with “HaplotypeCaller” from GATK to produce a genomic variant call format (gVCF) file for each sample, and “GenotypeGVCFs” from GATK was then used to perform the multi-sample genotyping, which produced a raw set of joint SNPs and indels. Several filtering steps were used to minimize the number of false positive SNPs and to retain high-quality SNPs: (1) we kept only biallelic SNPs with at least 5 bp away from any indels; (2) GATK hard filtering was applied to remove SNPs with a criteria of RMSMappingQuality (MQ) < 40.0, QualByDepth (QD) < 2.0, FisherStrand (FS) > 30.0, StrandOddsRatio (SOR) > 4.0, MappingQualityRankSumTest (MQRankSum) < –12.5 and ReadPosRankSumTest (ReadPosRankSum) < –8.0; (3) SNPs with genotype quality (GQ) < 20 and depth (DP) < 10 in a single individual were treated as missing data, and we removed those SNPs with missing rate > 20%; and (4) SNPs with minor allele frequency (MAF) < 5% were removed. The remaining 103,608 SNPs were used in downstream analysis.
Functional Annotation of Outlier SNPs
We built a local database based on released gene annotation for P. taeda genome v1.01 using SnpEff (version 4.3T) (Cingolani et al., 2012). Subsequently, functional annotation for each SNP was predicted with putative functional effects defined in the SnpEff. All SNPs were partitioned into 5'UTR, coding sequence (CDS), intron, 3'UTR, splice site, and intergenic mutations. Further we categorized SNPs within CDS as synonymous and nonsynonymous categories. Plant transcription factors (TFs) database, PlantTFDB 4.0 (http://planttfdb.cbi.pku.edu.cn/), were used to retrieve TFs annotations.
Population Structure and Genetic Diversity
Principal component analysis (PCA) was performed using the R package SNPRelate (version 1.16.0) (Zheng et al., 2012). ADMIXTURE (version 1.3.0) (Alexander et al., 2009) was run to infer the population structure, with the number of genetic clusters (K) ranging from 2 to 5, and 10 replicates were run for each K. The most likely K value was identified by minimizing the cross-validation error evaluated in the 10-fold cross-validation procedure. To eliminate the effect of linkage disequilibrium (LD), we thinned the SNP set to select one SNP from each interval of 5 kb and run ADMIXTURE under both all SNP (103,608) and thinned SNP (18,329) sets.
We calculated average pairwise estimates of the number of nonsynonymous substitutions per nonsynonymous site (dN), synonymous substitutions per synonymous site (dS), and their ratio (dN/dS) for individual genes. Nucleotide diversity (π) was calculated for each gene using VCFtools (Danecek et al., 2011).
Identification of Outlier SNPs
Based on the population structure obtained by PCA and ADMIXTURE results, the population BS was excluded in subsequent analyses given its different genetic composition. To identify outlier SNPs associated with high vs. low elevation adaptation, we conducted population comparison (KM(LZ) vs. KM(KM) and YL(KM)) between the high-elevation selected population (KM(LZ)) in high elevation site (LZ) and the survival populations from Kunming (KM) and Yiliang (YL) in the low elevation site in KM (KM(KM) and YL(KM)). Here, populations from KM and YL were in an identical genetic cluster, hereafter named KM-YL(KM). In addition, a second comparison, KM(LZ) vs. ALL(KM) which included 6 survival populations in Kunming site excluding population BS, was performed with the aim of providing more information for reference, regardless of the impact of population structure which may potentially cause false positives. Population differentiation (FST) values of each SNP were calculated using VCFtools (Danecek et al., 2011).
Outlier SNPs were determined by combining FST scan with a randomization procedure that involving repeated drawing of random samples (100 times). First, SNPs with the top 1% FST values were selected as putative outlier SNPs for each comparison. Next, we removed the putative outlier SNPs that are likely to be false positives found by chance when defining population comparisons with randomization procedure. For each population comparison, 100 permutations were performed to produce 100 randomized population comparisons by randomly re-sampling the same number of individuals from the original sample set. Then, putative outlier SNPs were also detected respectively for each randomized population comparison. Finally, for each putative outlier SNP from the real population comparison, we retained only those with a recurrence rate less than 0.01 to generate the final set of outlier SNPs.
Functional Enrichment Analysis
Kyoto Encyclopedia of Genes and Genomes (KEGG) and Gene Ontology (GO) annotation were implemented using an online annotation server, KOBAS 3.0 (http://kobas.cbi.pku.edu.cn). GO and KEGG enrichment analyses were performed using ClusterProfiler (Yu et al., 2012). P values were corrected using Benjamini-Hochberg FDR (false discovery rate). GO and KO terms with a corrected P value < 0.05 were treated to be significantly enriched.
Gene enrichment analysis permutations were performed to test whether the enriched GO and kegg terms from outlier genes are likely to be observed when choosing the same number of genes randomly across the genome. For each population comparison, 100 permutations were performed by randomly resampling the same number of genes as outlier genes. We then ran ClusterProfiler for each permutation as described above and counted the number of times the significant GO and kegg terms from the outlier genes also showed up in the randomly selected genes.
Results
Growth and Survival Rate in High and Low Elevation Common Gardens
At the high elevation site (Linzhi: LZ), the seedlings of all populations suffered heavy mortality. In contrast, survival rates were comparatively high and constant at the low elevation site (Kunming: KM). By the third year, survival rates produced exceedingly contrasting results with 7.6 vs. 78.2% for the Tibet (LZ) and Kunming (KM) sites, respectively (Zhao et al., 2014). In the seventh year (2017), only five individuals which originated from population KM survived at the high-elevation site (LZ). As natural populations can harbor a great deal of standing genetic variation, these individuals may contain significant divergence in allele frequency contributing to adaptation to high elevation environments. Thus, these individuals were sampled as high elevation selected (survival) population to detect genetic variation related to adaptation to high elevation environments in this study (these results concern the 6-7th year of the common garden experiment).
In addition, significant differences of growth between populations were observed between common garden sites, suggesting adaptive divergence among P. yunnanensis natural populations. In the low elevation site (KM), the local population (KM, 249.7 ± 24.2 cm) and two exotic populations from farthest Southwest (BS, 262.9 ± 30.4; GS, 251.5 ± 23.0) generally displayed the fastest growth. The most south population (YX) showed the slowest growth (183.2 ± 26.2), and the populations from the Northwest (ZD, 215.3 ± 13.7; LJ, 221.6 ± 15.4) showed intermediate seedling heights. YL, a population close to the local population (KM), showed slower but medium growth performance (190.1 ± 15.5) in comparison to the local population.
RNA-Seq and SNP Discovery
We have successfully constructed cDNA libraries for all the 54 sampled individuals. The RNA-seq yielded a total of 2,280 million clean reads, ranging from 30 to 60 million reads for each individual with an average of 42 million reads. On average, 81.93% of the reads were uniquely aligned to the P. taeda reference genome v1.01 (Table S1). After stringent quality-filtering, we retained a total of 103,608 high quality SNPs captured across the 54 individuals, which involve 8,595 annotated genes in the reference genome. Annotation of these SNPs indicated the presence of 24,439 (23.59% of the total SNPs) synonymous, 23,589 (22.77%) nonsynonymous variants, and 3,515 (3.39%) from 5′ UTR, 5,239 (5.06%) 3′ UTR, 8,089 (7.81%) intronic, 279 (0.27%) splice sites, and 38,458 (37.12%) intergenic mutations (Table 2).
Population Structure
The population structure inferred using the full SNP set (103,608 SNPs) was identical to that from the pruned SNPs (18,329), suggesting little impacts of LD in the ADMIXTURE analysis (Figures 2B and S1). Cross-validation errors determined that the most likely K value is 2 (Figure 2A). With K = 2, one western marginal population (BS(KM)) was split from the other populations. For K = 3, three northwestern populations (GS(KM), ZD(KM), and LJ(KM)) further formed a northwest cluster and the remaining 3 central populations (KM including KM(KM) and KM(LZ), YL(KM), YX(KM)) formed another central cluster. Under the K value of 4, population YX(KM) from the most south was further separated from the central cluster. The high-elevation selected populations KM(LZ) and KM(KM) and YL(KM) were in the same genetic cluster under K = 2, 3 and 4.
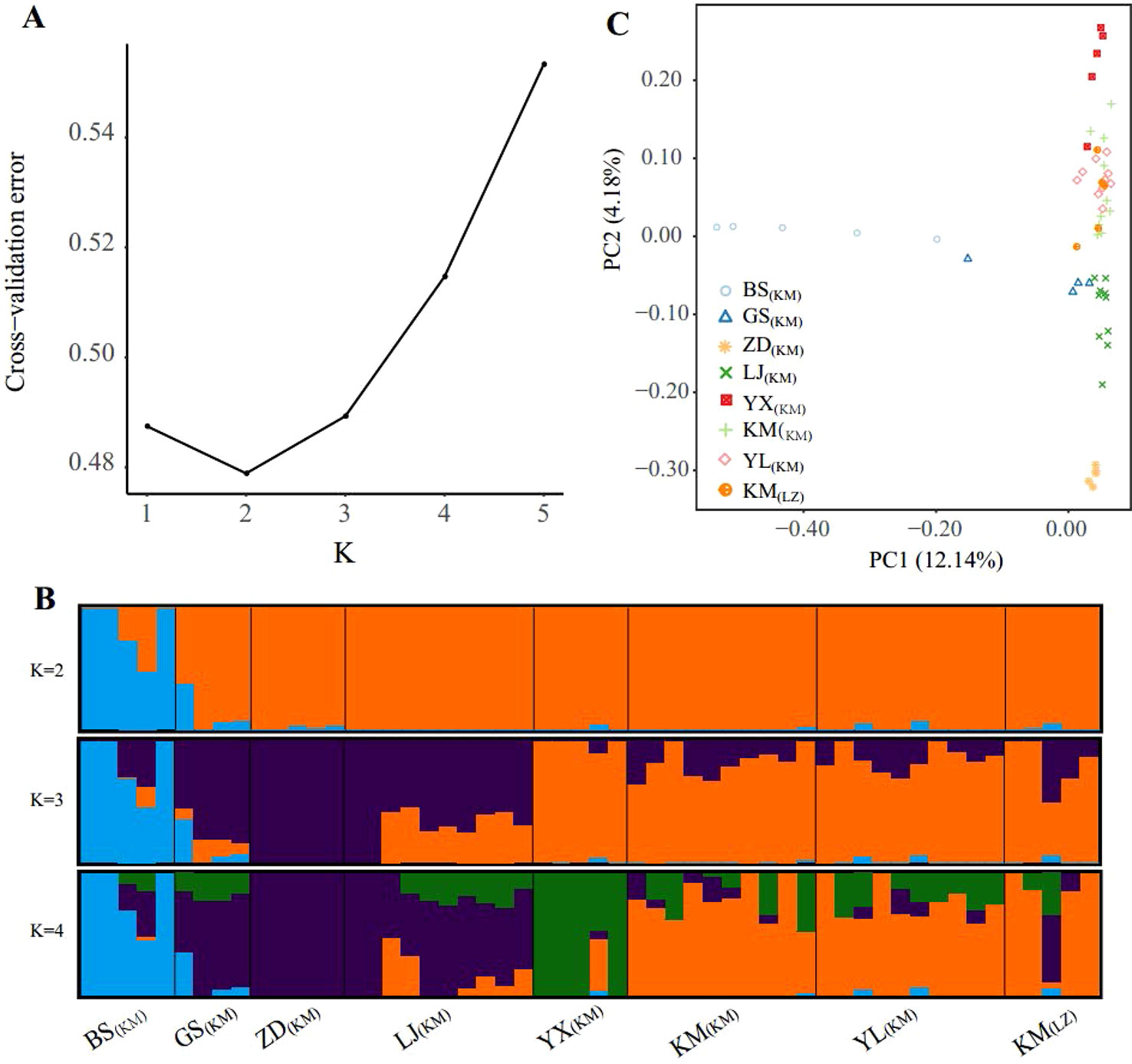
Figure 2 Population structure of the sampled individuals based on full SNP dataset. (A) Plot of Cross-validation (CV) error, (B) Genetic assignments under K = 2 – 4 based on ADMIXTURE results, (C) Plot of the two principal components and the percentage of variance explained resulting from a principal component analysis.
PCA provided further support to the patterns detected by ADMIXTURE (Figure 2C). Along the first principal component (PC1: accounting for 12.14% of total genetic variance), BS(KM) was separated from the other populations, supporting the ADMIXTURE finding at K = 2. PC2 was accounting for 4.18% of the total variance and showing 3 population clusters, YX(KM), northwest (GS(KM), ZD(KM), and LJ(KM)) and central (KM(KM), YL(KM), and KM(LZ)), which were distinguished from each other. PCA revealed a continuous genetic differentiation across the P. yunnanensis range, consistent with the ADMIXTURE results at K = 4.
Detection of Outlier Loci
Our FST procedure detected 321 outlier SNPs overlapping 131 genes for the comparison of KM(LZ) vs. KM-YL(KM), including 79 (24.61%) synonymous, 87 (27.10%) nonsynonymous, 3 (0.93%) 5′ UTR, 21 (6.54%) intronic, 22 (6.85%) 3′ UTR, 1 (0.31%) splice site, and 108 (33.64%) intergenic mutations (Tables 2 and S2). Additionally, 294 outlier SNPs for the comparison of KM(LZ) vs. ALL(KM) were also identified regardless of the impact of population structure which may cause false positives (Tables S5–S7), with 87 overlaps with the comparison of KM(LZ) vs. KM-YL(KM) (Figure 3; Tables S8–S10). The outlier SNP included higher proportion of nonsynonymous mutations (27–33%) compared to the genome average (22%) (Table 2). Compared to the whole genomic background, these 131 genes with outlier SNPs between KM(LZ) and KM-YL(KM) showed greater π across coding regions (Wilcoxon rank sum test, P <0.05), and slightly higher but not significant dN/dS values (Wilcoxon rank sum test; P > 0.05) with a mean of 0.4751 and maximum of 2.7400, in the high-elevation selected population KM(LZ) (Table 3).
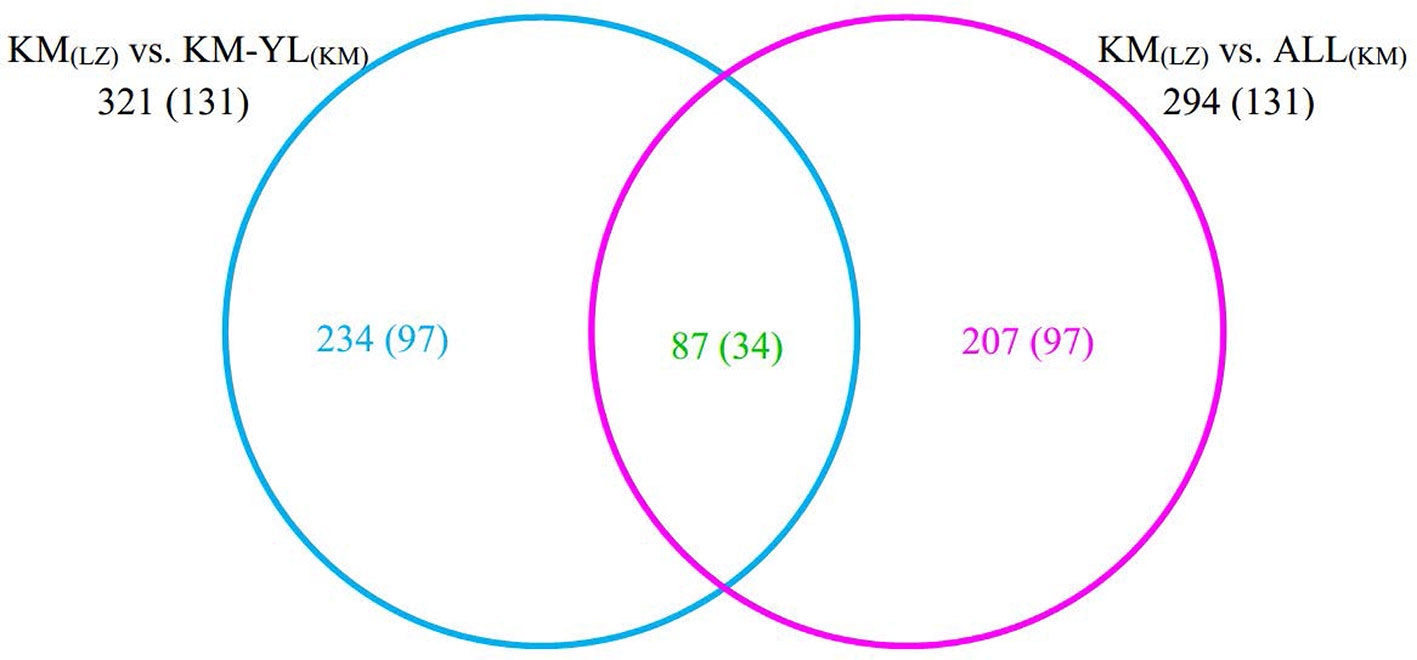
Figure 3 Overlap between outlier SNPs from the two comparisons. The numbers in parentheses indicate the number of genes overlapping with outlier SNPs.
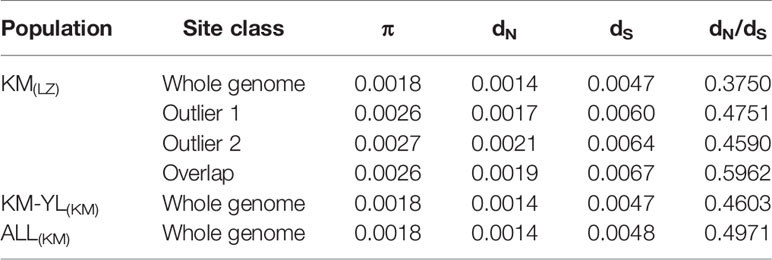
Table 3 Summary of population diversity statistics. π: nucleotide diversity across coding region for individual gene; dN: mean number of pairwise nonsynonymous substitutions per nonsynonymous site; dS: mean number of pairwise synonymous substitutions per synonymous site. Outlier 1: outlier genes between KM(LZ) and KM-YL(KM). Outlier 2: outlier genes between KM(LZ) and ALL(KM), Overlap: overlap between outlier 1 and outlier 2.
Functional Annotation
Enrichment analysis of 131 outlier genes for the comparison of KM(LZ) vs. KM-YL(KM) suggested that five “flavonoid biosynthesis pathway” genes were significantly overrepresented (Figure 4; Table S3). Of these five genes, three (gene ID: PITA_000032619-RA, PITA_000091299-RA and PITA_000042245-RA) encoded flavonoid 3-hydroxylase (F3'H) that catalyzes the formation of dihydroquercetin from dihydrokaempferol or naringenin. One gene (PITAhm_000428-RA) encoded anthocyanidin synthase (ANS) which catalyzes the oxidation of Leucoanthocyanidins (e.g., leucocyanidin, leucopelargonidin) to colored but unstable anthocyanidins (e.g., cyanidin and pelargonidin); and another one (1A_all_VO_L_2_T_4417/51331|m.1073.mrna2) encoded anthocyanidin reductase (ANR) which converts anthocyanidins to epi-flavan-3-ols (e.g., epicatechin, epigallocatechin) (Figure 4). Two SNPs causing nonsynonymous amino changes (Table 4) were found in two of the five above-mentioned genes (PITA_000032619-RA and PITA_000042245-RA). Functional analysis of these 131 outlier genes showed that five outlier genes are involved in functional categories associated with high elevation adaptation, including DNA repair (gene ID: PITA_000002229-RA), response to UV (PITA_000032619-RA and PITA_000042245-RA), response to reactive oxygen species (ROS) (PITAhm_000683-RA), and membrane lipid metabolic process (PITA_000060397-RA) (Tables 4 and S4). In addition, 3 outlier genes were annotated as TFs (1 bZIP, 1 NAC and 1 Trihelix). BZIP and NAC are known to be involved in the regulation of secondary metabolism, key components of stress response in plants (Vom Endt et al., 2002) (Table S2). Additionally, overlapping outlier genes between the two comparisons were significantly overrepresented in flavonoid biosynthesis pathway (gene IDs: PITA_000032619-RA, PITA_000042245-RA and PITA_000091299-RA), and carbon fixation in photosynthetic pathway (gene IDs: PITA_000042917-RA and PITA_000092661-RA) (Table S9), which suggests energy metabolism genes may contribute to adaptation to high elevation environments.
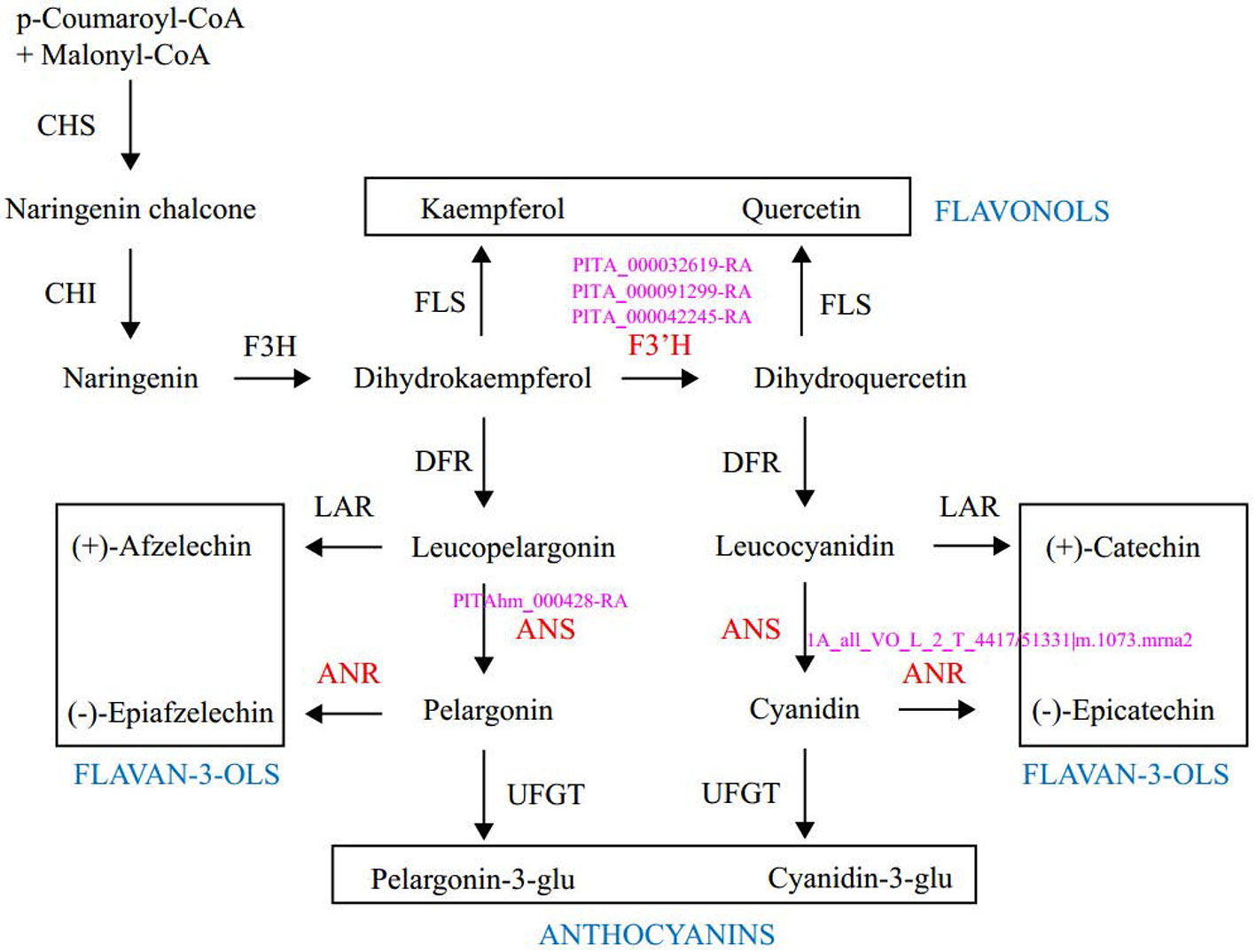
Figure 4 Outlier genes involved in the flavonoid biosynthetic pathway. Enzymes and intermediates are indicated in black. Enzymes in red are identified as targets under divergent selection, with corresponding outlier gene ID in pink. End products are placed in the square. CHS, Chalcone synthase; CHI, chalcone isomerase; F3H, fla-vanone 3-hydroxylase; F3′H, flavonoid-3′-hydroxylase; FLS, flavonol synthase; DFR, dihydroflavonol 4-reductase; LAR, leucoanthocyanidin reductase; ANS, anthocyanidin synthase; ANR, anthocyanidin reductase; UFGT, UDP-glucose: flavonoid 3-O gluco-syltransferase.
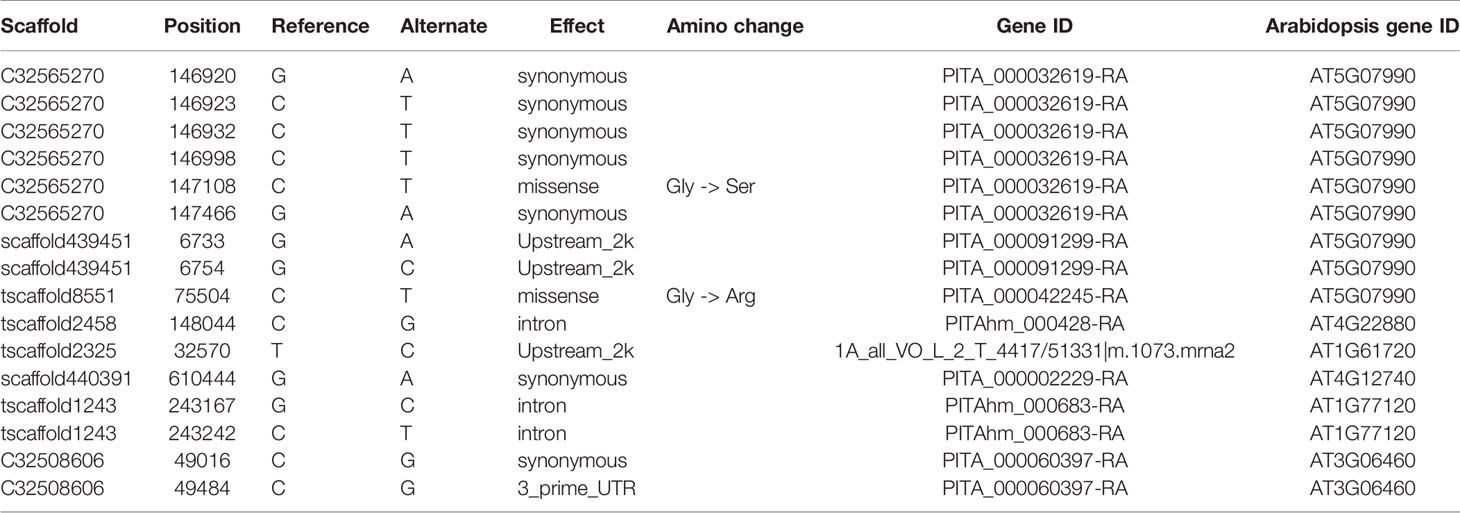
Table 4 Outlier SNPs from genes involved in flavonoid biosynthesis pathway and functional categories associated with high elevation adaptation for the comparison of KM(LZ) vs. KM-YL(KM).
To test whether the significant terms found within the outlier genes could also be found by chance when sampling genes from the genome, we performed enrichment permutations. None of the significant GO and kegg terms from our outlier analysis for the comparison of KM(LZ) vs. KM-YL(KM) reoccured during our permutations. Thus, we find that the outlier genes differ from the genomic background and that the significant terms are unlikely to be observed by chance.
Discussion
RNA sequencing allowed the identification of a large number of P. yunnanensis SNPs, most of which are from expressed genes. Based on all 103,608 high quality SNPs, a clear population structure emerged with two distinct population clusters comprising one southwestern corner population (BS) and all other populations, in which continuous genetic differentiation was found. These results are in agreement with previous findings that were based on combining mitochondrial and chloroplast DNA markers (Wang et al., 2013).
FST outlier analysis, such as FST scan and BayeScan 2.1 (Foll and Gaggiotti, 2008), is based on the assumption that nonselective processes have the same effect on all loci, while selection would only act on certain loci in the genome. Therefore, loci with very high genetic differentiation (FST) are considered to be under divergent selection (Vitti et al., 2013). However, FST outlier analysis presents two main limitations; namely, the high number of false positives produced by chance, and the lack of power to detect true positives; both of them are clearly discussed in the literature (De Mita et al., 2013; Lotterhos and Whitlock, 2014; Haasl and Payseur, 2016). Thus, it is expected that some of these putative outlier SNPs may be false positives produced by chance. Despite these caveats, FST outlier methods have been used in a large number of studies and has brought emblematic allele discoveries at genes involved in adaptation to different environment conditions (Feulner et al., 2015; Wang et al., 2015; Elgvin et al., 2017; Fustier et al., 2017). However, BayeScan has been considered more conservative in identifying outlier SNPs than other methods (Narum and Hess, 2011b; Cuervo-Alarcon et al., 2018; Gros-Balthazard et al., 2019), and its power to detect outliers depends largely on sample size and number of sampled populations (Lotterhos and Whitlock, 2015; Ahrens et al., 2018). Considering that, in this study, only two populations are involved in each comparison, so, we solely used FST scan to detect outlier SNPs. At present, there are two common strategies to reduce false positives. One strategy is to construct prediction with different algorithms, followed by assessing the consistency of signals (Narum and Hess, 2011a; De Mita et al., 2013). The other is to assess consistency of signals across biological replicates (Kawecki and Ebert, 2004; Conte et al., 2012; Tiffin and Ross-Ibarra, 2014). Additionally, in the present study, a randomization procedure (Feulner et al., 2015; Attard et al., 2018), that involved repeated drawing of random samples (100 times), was used to reduce false positives. Using top 1% FST outlier as a cutoff, we initially identified 980 putative outlier SNPs in the comparison of KM(LZ) vs. KM-YL(KM). After introducing additional FDR 0.01 by random sampling, we retained 321 outlier SNPs, suggesting that the FDR randomization procedure is potentially effective in reducing the rate of false positives.
Genetic differentiation between populations with different geographic origins have been found in ADMIXTURE and PCA analyses, and the observed differentiation between populations is an important confounding factor for detecting outliers in the comparison of KM(LZ) vs. ALL(KM). Considering that SNPs differentiated between KM(LZ) and ALL(KM) but not between KM(LZ) and KM-YL(KM) suggests they may be involved in population differentiation rather than adaptation to high elevation. Thus, we focused only on the results of the comparison of KM(LZ) vs. KM-YL(KM). An limitation of our study was the restricted number of samples surviving in the highland. However, we consider this exceedingly high mortality as evidence for maladaptation and thus we treat the results as exploratory in nature and are indicative of genetic variation to high elevation adaptation. Further functional evaluation may provide clearer insights into the genetic responses of P. yunnanensis to high elevation environments.
Natural population can harbor a great deal of standing genetic variation. Selection may result in changes of allele frequency of SNPs under selective pressures to rapidly maximize fitness in harsh environment (Hoffmann and Sgrò, 2011). In the present study, we found 321 outlier SNPs with significant divergence in allele frequency between KM(LZ) and KM-YL(KM). Genes with outlier SNPs showed significantly greater π than the genome average across coding regions and slightly higher dN/dS values in the high-elevation selected population KM(LZ). These outlier SNPs consist of 79 (24.61%) synonymous, 87 (27.10%) nonsynonymous, 3 (0.93%) 5′ UTR, 21 (6.54%) intronic, 22 (6.85%) 3′ UTR, 1 (0.31%) splice site, and 108 (33.64%) intergenic mutations. Previous studies have shown nonsynonymous, synonymous, and noncoding SNPs can show signatures of selection. Of these outlier SNPs, nonsynonymous substitutions causing amino acid substitutions and protein sequence changes have been usually considered to be the main target of natural selection. However, synonymous SNPs may affect mRNA alternative splicing, mRNA stability, translation kinetics, and protein expression and function, as previously documented (Chamary et al., 2006; Komar, 2007; Kirchner et al., 2017; Bertalovitz et al., 2018). Likewise, SNPs in noncoding regions may also be involved in the regulation of gene expression (Barrett et al., 2012). Thus, noncoding and synonymous SNPs can display a selection signal, either because they are linked to a selection site or are directly selected by natural selection.
The extremely intense UV radiation on the highland may influence plant growth and developmental processes or cause DNA and protein damage (Frohnmeyer and Staiger, 2003). Low temperatures, a major feature of high elevation, can cause lipid peroxidation and reduce fluidity of lipid membranes by causing fatty acid unsaturation, altering lipid composition and ratio of lipids to proteins in cell membrane (Wang et al., 2006). Both cold stress and strong UV radiation result in the oxidative stress due to generation of reactive oxygen species (ROS), such as hydrogen peroxide, superoxide anion, and hydroxyl radical (Halliwell, 2007; Wang et al., 2010; Lidon and Ramalho, 2011). Previous studies revealed that the DNA repair and radiation responses pathways may contribute to highland adaptation of the Crucihimalaya himalaica (Qiao et al., 2016; Zhang et al., 2019), Tibetan highland barley (Zeng et al., 2015), Tibetan antelope (Ge et al., 2013), Tibetan chicken (Zhang et al., 2016), and Tibetan hot-spring snake (Li et al., 2018). In the present study, we found that many outlier genes are involved in response to UV, DNA repair, response to ROS, and membrane lipid metabolic process. This may suggest P. yunnanensis adaptation to strong UV radiation and low temperature environments on the highland.
The strong UV-absorbing characteristics of flavonoids have been considered as a primary role to protect plant tissues from high energetic UV. Moreover, many studies have provided new evidence that UV light induces the synthesis of flavonoids (Ryan et al., 2002; Berli et al., 2010; Stracke et al., 2010; Agati et al., 2011; Kusano et al., 2011). These flavonoids can perform antioxidant roles by suppressing and scavenging free radicals such as ROS (Agati et al., 2012), as well as chelating metal ions such as iron, copper, zinc, and aluminum that generate ROS via the Fenton reaction (Williams et al., 2004). In addition, they are able to prevent the peroxidation of lipids and the oxidative damage of membrane lipids (Kumar and Pandey, 2012). The central pathways for flavonoid biosynthesis are highly conserved and well characterized (Holton and Cornish, 1995; Winkel-Shirley, 2001; Petrussa et al., 2013; Li et al., 2015). In the present study, outlier genes from the comparison of KM(LZ) vs. KM-YL(KM) were significantly enriched for flavonoid biosynthesis pathway with 5 outlier genes coding 3 key enzymes. And this pathway has been shown to help withstand harsh environments in high elevations (Zhang et al., 2003; Casati and Walbot, 2010; Agati et al., 2012). These results suggest that flavonoid biosynthesis pathway may play a key role in the adaptation of P. yunnanensis to high elevation environments. Additional functional and physiological experiments are needed to verify the contributions of these genes to high elevation adaptation (Pavlidis et al., 2012).
Conclusions
We used RNA-seq and FST scan to identify genetic variation related to high elevation adaptation in P. yunnanensis by contrasting a high-elevation selected population and low-elevation population sampled from highland and lowland common gardens. Our study provided a genome-wide evaluation of nucleotide diversity in this species, and identified variants and genes that could be involved in adaptation to high elevation environment. Our results suggest that the flavonoid biosynthesis pathway is likely an important selection target, which may play a key role in the adaptation to high elevation environments in P. yunnanensis. While these results are based on a small sample size, thus we consider this work as exploratory in nature and further research on high elevation adaptation is warranted.
Data Availability Statement
All RNA-seq data sets used in this study have been submitted to the NCBI SRA database under Bioproject Accession no. PRJNA545862.
Author Contributions
JM designed the study. YS, WZ, CX and YX completed the settlement of common garden experiment and data and sample collection. YS and WZ analyzed data. YS wrote the draft. JM, YE-K, and AT revised the manuscript. All authors reviewed the final version of the manuscript.
Funding
This research was funded by the National Natural Science Foundation of China (NO. 31670664 and 31800550) and Fundamental Research Funds for the Central Universities (2018BLCB08).
Conflict of Interest
The authors declare that the research was conducted in the absence of any commercial or financial relationships that could be construed as a potential conflict of interest.
Acknowledgments
The authors are grateful to Prof. Xiao-Ru Wang for suggestions and manuscript revision.
Supplementary Material
The Supplementary Material for this article can be found online at: https://www.frontiersin.org/articles/10.3389/fgene.2019.01405/full#supplementary-material
Figure S1 | Population structure of the sampled individuals based on the thinned SNP dataset. (A) Plot of Cross-validation (CV) error. (B) Genetic assignment under K = 2 – 4.
References
Agati, G., Biricolti, S., Guidi, L., Ferrini, F., Fini, A., Tattini, M. (2011). The biosynthesis of flavonoids is enhanced similarly by UV radiation and root zone salinity in L. vulgare leaves. J. Plant Physiol. 168, 204–212. doi: 10.1016/j.jplph.2010.07.016
Agati, G., Azzarello, E., Pollastri, S., Tattini, M. (2012). Flavonoids as antioxidants in plants: location and functional significance. Plant Sci. 196, 67–76. doi: 10.1016/j.plantsci.2012.07.014
Ahrens, C. W., Rymer, P. D., Stow, A., Bragg, J., Dillon, S., Umbers, K. D. L., et al. (2018). The search for loci under selection: trends, biases and progress. Mol. Ecol. 27, 1342–1356. doi: 10.1111/mec.14549
Alexander, D. H., Novembre, J., Lange, K. (2009). Fast model-based estimation of ancestry in unrelated individuals. Genome Res. 19, 1655–1664. doi: 10.1101/gr.094052.109
Alonso-Amelot, M. E. (2008). “High altitude plants, chemistry of acclimation and adaptation,” in Studies in Natural Products Chemistry, 1st ed. Ed. Atta-ur., R. (Holland: Elsevier), 883–982.
Attard, C., Beheregaray, L. B., Sandovalcastillo, J., Jenner, K., Gill, P. C., Jenner, M. M., et al. (2018). From conservation genetics to conservation genomics: A genome-wide assessment of blue whales (Balaenoptera musculus) in Australian feeding aggregations. R. Soc. Open Sci. 5, 170925. doi: 10.1098/rsos.170925
Barrett, L. W., Fletcher, S., Wilton, S. D. (2012). Regulation of eukaryotic gene expression by the untranslated gene regions and other non-coding elements. Cell. Mol. Life Sci. 69, 3613–3634. doi: 10.1007/s00018-012-0990-9
Berli, F. J., Moreno, D., Piccoli, P., Hespanhol-Viana, L., Silva, M. F., Bressan-Smith, R., et al. (2010). Abscisic acid is involved in the response of grape (Vitis vinifera L.) cv. Malbec leaf tissues to ultraviolet-B radiation by enhancing ultraviolet-absorbing compounds, antioxidant enzymes and membrane sterols. Plant Cell Environ. 33, 1–10. doi: 10.1111/j.1365-3040.2009.02044.x
Bertalovitz, A. C., Badhey, M. L. O., McDonald, T. V. (2018). Synonymous nucleotide modification of the KCNH2 gene affects both mRNA characteristics and translation of the encoded hERG ion channel. J. Biol. Chem. 293, 12120–12136. doi: 10.1074/jbc.RA118.001805
Bolger, A. M., Lohse, M., Usadel, B. (2014). Trimmomatic: a flexible trimmer for Illumina sequence data. Bioinformatics 30, 2114–2120. doi: 10.1093/bioinformatics/btu170
Casati, P., Walbot, V. (2010). Differential accumulation of maysin and rhamnosylisoorientin in leaves of high-altitude landraces of maize after UV-B exposure. Plant Cell Environ. 28, 788–799. doi: 10.1111/j.1365-3040.2005.01329.x
Chamary, J. V., Parmley, J. L., Hurst, L. D. (2006). Hearing silence: non-neutral evolution at synonymous sites in mammals. Nat. Rev. Genet. 7, 98–108. doi: 10.1038/nrg1770
Cingolani, P., Platts, A., Wang, L., Coon, M., Nguyen, T., Wang, L., et al. (2012). A program for annotating and predicting the effects of single nucleotide polymorphisms, SnpEff: SNPs in the genome of Drosophila melanogaster strain w1118; iso-2; iso-3. Fly 6, 80–92. doi: 10.4161/fly.19695
Conte, G. L., Arnegard, M. E., Peichel, C. L., Schluter, D. (2012). The probability of genetic parallelism and convergence in natural populations. Proc. R. Soc. B-Biol. Sci. 279, 5039–5047. doi: 10.1098/rspb.2012.2146
Cuervo-Alarcon, L., Arend, M., Müller, M., Sperisen, C., Finkeldey, R., Krutovsky, K. V. (2018). Genetic variation and signatures of natural selection in populations of European beech (Fagus sylvatica L.) along precipitation gradients. Tree Genet. Genom. 14, 84. doi: 10.1007/s11295-018-1297-2
Danecek, P., Auton, A., Abecasis, G., Albers, C. A., Banks, E., DePristo, M. A., et al. (2011). The variant call format and VCFtools. Bioinformatics 27, 2156–2158. doi: 10.1093/bioinformatics/btr330
De La Torre, A. R., Neale, D. B. (2019). Climate adaptation shaped by subtle to moderate allele frequency shifts in loblolly pine. bioRxiv 635862. doi: 10.1101/635862
De La Torre, A. R., Birol, I., Bousquet, J., Ingvarsson, P. K., Jansson, S., Jones, S. J. M., et al. (2014). Insights into conifer giga-genomes. Plant Physiol. 166, 1724–1732. doi: 10.1104/pp.114.248708
De La Torre, A. R., Puiu, D., Crepeau, M. W., Stevens, K., Salzberg, S. L., Langley, C. H., et al. (2019). Genomic architecture of complex traits in loblolly pine. New Phytol. 221, 1789–1801. doi: 10.1111/nph.15535
De Mita, S., Thuillet, A. C., Gay, L., Ahmadi, N., Manel, S., Ronfort, J., et al. (2013). Detecting selection along environmental gradients: analysis of eight methods and their effectiveness for outbreeding and selfing populations. Mol. Ecol. 22, 1383–1399. doi: 10.1111/mec.12182
DePristo, M. A., Banks, E., Poplin, R., Garimella, K. V., Maguire, J. R., Hartl, C., et al. (2011). A framework for variation discovery and genotyping using next-generation DNA sequencing data. Nat. Genet. 43, 491–498. doi: 10.1038/ng.806
Dobin, A., Davis, C. A., Schlesinger, F., Drenkow, J., Zaleski, C., Jha, S., et al. (2013). STAR: ultrafast universal RNA-seq aligner. Bioinformatics 29, 15–21. doi: 10.1093/bioinformatics/bts635
Ekblom, R., Galindo, J. (2011). Applications of next generation sequencing in molecular ecology of non-model organisms. Heredity 107, 1–15. doi: 10.1038/hdy.2010.152
Elgvin, T. O., Trier, C. N., Tørresen, O. K., Hagen, I. J., Lien, S., Nederbragt, A. J., et al. (2017). The genomic mosaicism of hybrid speciation. Sci. Adv. 3, e1602996. doi: 10.1126/sciadv.1602996
Farjon, A., Farjon, A. (2010). A Handbook of the Worlds Conifers. Leiden: Brill Academic Publishers.
Feulner, P. G., Chain, F. J., Panchal, M., Huang, Y., Eizaguirre, C., Kalbe, M., et al. (2015). Genomics of divergence along a continuum of parapatric population differentiation. PLoS Genet. 11, e1004966. doi: 10.1371/journal.pgen.1004966
Flood, P. J., Hancock, A. M. (2017). The genomic basis of adaptation in plants. Curr. Opin. Plant Biol. 36, 88–94. doi: 10.1016/j.pbi.2017.02.003
Foll, M., Gaggiotti, O. (2008). A genome-scan method to identify selected loci appropriate for both dominant and codominant markers: a Bayesian perspective. Genetics 180, 977–993. doi: 10.1534/genetics.108.092221
Frohnmeyer, H., Staiger, D. (2003). Ultraviolet-B radiation-mediated responses in plants. Balancing damage and protection. Plant Physiol. 133, 1420–1428. doi: 10.1104/pp.103.030049
Fustier, M. A., Brandenburg, J. T., Boitard, S., Lapeyronnie, J., Eguiarte, L. E., Vigouroux, Y., et al. (2017). Signatures of local adaptation in lowland and highland teosintes from whole-genome sequencing of pooled samples. Mol. Ecol. 26, 2738–2756. doi: 10.1111/mec.14082
Ge, R. L., Cai, Q., Shen, Y. Y., San, A., Ma, L., Zhang, Y., et al. (2013). Draft genome sequence of the Tibetan antelope. Nat. Commun. 4, 1858. doi: 10.1038/ncomms2860
Gong, J., Zhang, Z., Zhang, C., Zhang, J., Ran, A. (2018). Ecophysiological responses of three tree species to a high-altitude environment in the southeastern Tibetan Plateau. Forests 9, 48. doi: 10.3390/f9020048
Gros-Balthazard, M., Besnard, G., Sarah, G., Holtz, Y., Leclercq, J., Santoni, S., et al. (2019). Evolutionary transcriptomics reveals the origins of olives and the genomic changes associated with their domestication. Plant J. 100, 143–157. doi: 10.1111/tpj.14435
Haasl, R. J., Payseur, B. A. (2016). Fifteen years of genomewide scans for selection: trends, lessons and unaddressed genetic sources of complication. Mol. Ecol. 25, 5–23. doi: 10.1111/mec.13339
Halbritter, A. H., Fior, S., Keller, I., Billeter, R., Edwards, P., Holderegger, R., et al. (2018). Trait differentiation and adaptation of plants along elevation gradients. J. Evol. Biol. 31, 784–800. doi: 10.1111/jeb.13262
Halliwell, B. (2007). Biochemistry of oxidative stress. Biochem. Soc. Trans. 35, 1147–1150. doi: 10.1042/bst0351147
Hoban, S., Kelley, J. L., Lotterhos, K. E., Antolin, M. F., Bradburd, G., Lowry, D. B., et al. (2016). Finding the genomic basis of local adaptation: pitfalls, practical solutions, and future directions. Am. Nat. 188, 379–397. doi: 10.1086/688018
Hoffmann, A. A., Sgrò, C. M. (2011). Climate change and evolutionary adaptation. Nature 470, 479–485. doi: 10.1038/nature09670
Holton, T. A., Cornish, E. C. (1995). Genetics and biochemistry of anthocyanin biosynthesis. Plant Cell 7, 1071–1083. doi: 10.1105/tpc.7.7.1071
Jones, M. R., Good, J. M. (2016). Targeted capture in evolutionary and ecological genomics. Mol. Ecol. 25, 185–202. doi: 10.1111/mec.13304
Kawecki, T. J., Ebert, D. (2004). Conceptual issues in local adaptation. Ecol. Lett. 7, 1225–1241. doi: 10.1111/j.1461-0248.2004.00684.x
Kirchner, S., Cai, Z., Rauscher, R., Kastelic, N., Anding, M., Czech, A., et al. (2017). Alteration of protein function by a silent polymorphism linked to tRNA abundance. PLoS Biol. 15, e2000779. doi: 10.1371/journal.pbio.2000779
Komar, A. A. (2007). SNPs, silent but not invisible. Science 315, 466–467. doi: 10.1126/science.1138239
Kuan, C. T. (1981). Fundamental features of the distribution of Coniferae in Sichuan. Acta Phtotaxonomical. 11, 393–407.
Kumar, S., Pandey, A. K. (2012). Antioxidant, lipo-protective and antibacterial activities of phytoconstituents present in Solanum Xanthocarpum root. Int. Rev. Biophys. Chem. 3, 42–47. https://www.praiseworthyprize.org/jsm/index.php?journal=irebic&page=article&op=view&path[]=9860
Kusano, M., Tohge, T., Fukushima, A., Kobayashi, M., Hayashi, N., Otsuki, H., et al. (2011). Metabolomics reveals comprehensive reprogramming involving two independent metabolic responses of Arabidopsis to UV-B light. Plant J. 67, 354–369. doi: 10.1111/j.1365-313X.2011.04599.x
Leitch, I. J., Johnston, E., Pellicer, J., Hidalgo, O., Bennett, M. D. (2019). https://cvalues.science.kew.org/search/gymnosperm. Gymnosperm DNA C-values database (release 6.0, Apr 2019).
Li, H., Handsaker, B., Wysoker, A., Fennell, T., Ruan, J., Homer, N., et al. (2009). The sequence alignment/map format and SAMtools. Bioinformatics 25, 2078–2079. doi: 10.1093/bioinformatics/btp352
Li, C. F., Zhu, Y., Yu, Y., Zhao, Q. Y., Wang, S. J., Wang, X. C., et al. (2015). Global transcriptome and gene regulation network for secondary metabolite biosynthesis of tea plant (Camellia sinensis). BMC Genomics 16, 560. doi: 10.1186/s12864-015-1773-0
Li, J. T., Gao, Y. D., Xie, L., Deng, C., Shi, P., Guan, M. L., et al. (2018). Comparative genomic investigation of high-elevation adaptation in ectothermic snakes. PNAS 115, 8406–8411. doi: 10.1073/pnas.1805348115
Lidon, F. C., Ramalho, J. C. (2011). Impact of UV-B irradiation on photosynthetic performance and chloroplast membrane components in Oryza sativa L. J. Photochem. Photobiol. B.: Biol. 104, 457–466. doi: 10.1016/j.jphotobiol.2011.05.004
Lind, B. M., Menon, M., Bolte, C. E., Faske, T. M., Eckert, A. J. (2018). The genomics of local adaptation in trees: Are we out of the woods yet? Tree Genet. Genom. 14, 29. doi: 10.1007/s11295-017-1224-y
Lotterhos, K. E., Whitlock, M. C. (2014). Evaluation of demographic history and neutral parameterization on the performance of FST outlier tests. Mol. Ecol. 23, 2178–2192. doi: 10.1111/mec.12725
Lotterhos, K. E., Whitlock, M. C. (2015). The relative power of genome scans to detect local adaptation depends on sampling design and statistical method. Mol. Ecol. 24, 1031–1046. doi: 10.1111/mec.13100
Lu, M., Loopstra, C. A., Krutovsky, K. V. (2019). Detecting the genetic basis of local adaptation in loblolly pine (Pinus taeda L.) using whole exome-wide genotyping and an integrative landscape genomics analysis approach. Ecol. Evol. 9, 6798–6809. doi: 10.1002/ece3.5225
Mao, J. F., Wang, X. R. (2011). Distinct niche divergence characterizes the homoploid hybrid speciation of Pinus densata on the Tibetan Plateau. Am. Nat. 177, 424–439. doi: 10.1086/658905
Mao, J. F., Li, Y., Wang, X. R. (2009). Empirical assessment of the reproductive fitness components of the hybrid pine Pinus densata on the Tibetan Plateau. Evol. Ecol. 23, 447–462. doi: 10.1007/s10682-008-9244-6
Monge, C., Leon-Velarde, F. (1991). Physiological adaptation to high altitude: oxygen transport in mammals and birds. Physiol. Rev. 71, 1135–1172. doi: 10.1152/physrev.1991.71.4.1135
Narum, S. R., Hess, J. E. (2011a). Comparison of F(ST) outlier tests for SNP loci under selection. Mol. Ecol. Resour. 11 Suppl 1, 184–194. doi: 10.1111/j.1755-0998.2011.02987.x
Narum, S. R., Hess, J. E. (2011b). Comparison of FST outlier tests for SNP loci under selection. Mol. Ecol. Resour. 11 Suppl 1, 184–194. doi: 10.1111/j.1755-0998.2011.02987.x
Oney-Birol, S., Fitz-Gibbon, S., Chen, J. M., Gugger, P. F., Sork, V. L. (2018). Assessment of shared alleles in drought-associated candidate genes among southern California white oak species (Quercus sect. Quercus). BMC Genet. 19, 88. doi: 10.1186/s12863-018-0677-9
Pavlidis, P., Jensen, J. D., Stephan, W., Stamatakis, A. (2012). A critical assessment of storytelling: gene ontology categories and the importance of validating genomic scans. Mol. Biol. Evol. 29, 3237–3248. doi: 10.1093/molbev/mss136
Petrussa, E., Braidot, E., Zancani, M., Peresson, C., Bertolini, A., Patui, S., et al. (2013). Plant flavonoids——biosynthesis, transport and involvement in stress responses. Int. J. Mol. Sci. 14, 14950–14973. doi: 10.3390/ijms140714950
Qiao, Q., Wang, Q., Han, X., Guan, Y., Sun, H., Zhong, Y., et al. (2016). Transcriptome sequencing of Crucihimalaya himalaica (Brassicaceae) reveals how Arabidopsis close relative adapt to the Qinghai-Tibet Plateau. Sci. Rep. 6, 21729. doi: 10.1038/srep21729
Richardson, D. M. (1999). Ecology and biogeography of Pinus. Q. Rev. Biol. (New York, NY, USA: Cambridge University Press).
Ryan, K. G., Swinny, E. E., Markham, K. R., Winefield, C. (2002). Flavonoid gene expression and UV photoprotection in transgenic and mutant Petunia leaves. Phytochemistry 59, 23–32. doi: 10.1016/S0031-9422(01)00404-6
Schlötterer, C., Tobler, R., Kofler, R., Nolte, V. (2014). Sequencing pools of individuals — mining genome-wide polymorphism data without big funding. Nat. Rev. Genet. 15, 749. doi: 10.1038/nrg3803
Stapley, J., Reger, J., Feulner, P. G., Smadja, C., Galindo, J., Ekblom, R., et al. (2010). Adaptation genomics: the next generation. Trends Ecol. Evol. 25, 705–712. doi: 10.1016/j.tree.2010.09.002
Storz, J. F., Scott, G. R., Cheviron, Z. A. (2010). Phenotypic plasticity and genetic adaptation to high-altitude hypoxia in vertebrates. J. Exp. Biol. 213, 4125–4136. doi: 10.1242/jeb.048181
Stracke, R., Jahns, O., Keck, M., Tohge, T., Niehaus, K., Fernie, A. R., et al. (2010). Analysis of production of flavonol glycosides-dependent flavonol glycoside accumulation in Arabidopsis thaliana plants reveals MYB11-, MYB12- and MYB111-independent flavonol glycoside accumulation. New Phytol. 188, 985–1000. doi: 10.1111/j.1469-8137.2010.03421.x
Tiffin, P., Ross-Ibarra, J. (2014). Advances and limits of using population genetics to understand local adaptation. Trends Ecol. Evol. 29, 673–680. doi: 10.1016/j.tree.2014.10.004
Tyrmi, J. S., Vuosku, J., Acosta, J. J., Li, Z., Sterck, L., Savolainen, O., et al. (2019). Genomics of clinal local adaptation in Pinus sylvestris under continuous environmental and spatial genetic setting. bioRxiv. doi: 10.1101/647412
Vitti, J. J., Grossman, S. R., Sabeti, P. C. (2013). Detecting natural selection in genomic data. Annu. Rev. Genet. 47, 97–120. doi: 10.1146/annurev-genet-111212-133526
Vom Endt, D., Kijne, J. W., Memelink, J. (2002). Transcription factors controlling plant secondary metabolism: what regulates the regulators? Phytochemistry 61, 107–114. doi: 10.1016/S0031-9422(02)00185-1
Wang, X., Li, W., Li, M., Welti, R. (2006). Profiling lipid changes in plant response to low temperatures. Physiol. Plant. 126, 90–96. doi: 10.1111/j.1399-3054.2006.00622.x
Wang, S., Xie, B., Yin, L., Duan, L., Li, Z., Eneji, A. E., et al. (2010). Increased UV-B radiation affects the viability, reactive oxygen species accumulation and antioxidant enzyme activities in maize (Zea mays L.) pollen. Photochem. Photobiol. 86, 110–116. doi: 10.1111/j.1751-1097.2009.00635.x
Wang, B. S., Mao, J. F., Zhao, W., Wang, X. R. (2013). Impact of geography and climate on the genetic differentiation of the subtropical pine Pinus yunnanensis. Plos One 8, e67345. doi: 10.1371/journal.pone.0067345
Wang, M. S., Li, Y., Peng, M. S., Zhong, L., Wang, Z. J., Li, Q. Y., et al. (2015). Genomic analyses reveal potential independent adaptation to high altitude in Tibetan chickens. Mol. Biol. Evol. 32, 1880–1889. doi: 10.1093/molbev/msv071
Wegrzyn, J. L., Liechty, J. D., Stevens, K. A., Wu, L.-S., Loopstra, C. A., Vasquez-Gross, H. A., et al. (2014). Unique features of the loblolly pine (Pinus taeda L.) megagenome revealed through sequence annotation. Genetics 196, 891–909. doi: 10.1534/genetics.113.159996
Williams, R. J., Spencer, J. P. E., Rice-Evans, C. (2004). Flavonoids: antioxidants or signalling molecules? Free Radical Biol. Med. 36, 838–849. doi: 10.1016/j.freeradbiomed.2004.01.001
Winkel-Shirley, B. (2001). Flavonoid biosynthesis. a colorful model for genetics, biochemistry, cell biology, and biotechnology. Plant Physiol. 126, 485–493. doi: 10.1104/pp.126.2.485
Wu, C. L. (1956). The taxonomic revision and phytogeographical study of Chinese pines. Acta Phytotaxon. Sin. 5, 131–163.
Yeaman, S., Hodgins, K. A., Lotterhos, K. E., Suren, H., Nadeau, S., Degner, J. C., et al. (2016). Convergent local adaptation to climate in distantly related conifers. Science 353, 1431–1433. doi: 10.1126/science.aaf7812
Yu, H., Zheng, S. S., Huang, R. F. (1998). Polymorphism of male cones in populations of Pinus yunnanensis Franch. Biodiversity Sci. 6, 267–271. doi: 10.17520/biods.1998039
Yu, H., Ge, S., Huang, R., Jiang, H. (2000). A preliminary study on genetic variation and relationships of Pinus yunnanensis and its closely related species. Acta Bot. Sinica 42, 107–110.
Yu, G., Wang, L., Han, Y., He, Q. (2012). ClusterProfiler: an R package for comparing biological themes among gene clusters. OMICS 16, 284–287. doi: 10.1089/omi.2011.0118
Zeng, X., Long, H., Wang, Z., Zhao, S., Tang, Y., Huang, Z., et al. (2015). The draft genome of Tibetan hulless barley reveals adaptive patterns to the high stressful Tibetan Plateau. PNAS 112, 1095–1100. doi: 10.1073/pnas.1423628112
Zhang, P., Wang, Y., Zhang, J., Maddock, S., Snook, M., Peterson, T. (2003). A maize QTL for silk maysin levels contains duplicated Myb-homologous genes which jointly regulate flavone biosynthesis. Plant Mol. Biol. 52, 1–15. doi: 10.1023/a:1023942819106
Zhang, Q., Gou, W., Wang, X., Zhang, Y., Ma, J., Zhang, H., et al. (2016). Genome resequencing identifies unique adaptations of Tibetan chickens to hypoxia and high-dose ultraviolet radiation in high-altitude environments. GBE 8, 765–776. doi: 10.1093/gbe/evw032
Zhang, T., Qiao, Q., Novikova, P. Y., Wang, Q., Yue, J., Guan, Y., et al. (2019). Genome of Crucihimalaya himalaica, a close relative of Arabidopsis, shows ecological adaptation to high altitude. PNAS 116, 7137–7146. doi: 10.1073/pnas.1817580116
Zhao, W., Meng, J. X., Wang, B. S., Zhang, L. S., Xu, Y. L., Zeng, Q. Y., et al. (2014). Weak crossability barrier but strong juvenile selection supports ecological speciation of the hybrid pine Pinus densata on the Tibetan Plateau. Evolution 68, 3120–3133. doi: 10.1111/evo.12496
Zheng, X., Levine, D., Shen, J., Gogarten, S. M., Laurie, C., Weir, B. S. (2012). A high-performance computing toolset for relatedness and principal component analysis of SNP data. Bioinformatics 28, 3326–3328. doi: 10.1093/bioinformatics/bts606
Keywords: elevation adaptation, RNA-seq, FST outlier, flavonoid biosynthesis, nucleotide diversity
Citation: Sun Y-Q, Zhao W, Xu C-Q, Xu Y, El-Kassaby YA, De La Torre AR and Mao J-F (2020) Genetic Variation Related to High Elevation Adaptation Revealed by Common Garden Experiments in Pinus yunnanensis. Front. Genet. 10:1405. doi: 10.3389/fgene.2019.01405
Received: 04 September 2019; Accepted: 23 December 2019;
Published: 11 February 2020.
Edited by:
Renchao Zhou, Sun Yat-sen University, ChinaReviewed by:
Piotr Androsiuk, University of Warmia and Mazury in Olsztyn, PolandNaoki Tani, Japan International Research Center for Agricultural Sciences, Japan
Copyright © 2020 Sun, Zhao, Xu, Xu, El-Kassaby, De La Torre and Mao. This is an open-access article distributed under the terms of the Creative Commons Attribution License (CC BY). The use, distribution or reproduction in other forums is permitted, provided the original author(s) and the copyright owner(s) are credited and that the original publication in this journal is cited, in accordance with accepted academic practice. No use, distribution or reproduction is permitted which does not comply with these terms.
*Correspondence: Jian-Feng Mao, jianfeng.mao@bjfu.edu.cn
†These authors have contributed equally to this work