- 1Key Laboratory of Tropical Animal Breeding and Epidemic Disease Research of Hainan Province, College of Animal Science and Technology, Hainan University, Haikou, China
- 2Animal Disease Prevention and Control Center of Hanzhong, Hanzhong, China
At present, bovine mastitis is one of the most costly diseases affecting animal health and welfare. Escherichia coli (E. coli) is considered to be one of the main pathogens causing mastitis with clinical signs in dairy cattle. However, the cure rate of E. coli mastitis is low, and the pathogenesis of E. coli mastitis is not completely known. In order to develop new strategies for the rapid detection of E. coli mastitis, a comprehensive molecular investigation of E. coli mastitis is necessary. Hence, this study integrated three microarray data sets to identify the potential key candidate genes in dairy cow in response to E. coli mastitis. Differentially expressed genes (DEGs) were screened in mammary gland tissues with live E. coli infection. Furthermore, the pathways enrichment of DEGs were analyzed, and the protein–protein interaction (PPI) network was performed. In total, 105 shared DEGs were identified from the three data sets. The DEGs were significantly enriched in biological processes mainly involved in immunity. The PPI network of DEGs was constructed with 102 nodes and 546 edges. The module with the highest score through MCODE analysis was filtered from PPI; 18 central node genes were identified. However, in addition to immune-related pathways, some of the 18 DEGs were involved in signaling pathways triggered by other diseases. Considering the specificity of biomarkers for rapid detection, IL8RB, CXCL6, and MMP9 were identified as the most potential biomarker for E. coli mastitis. In conclusion, the novel DEGs and pathways identified in this study can help to improve the diagnosis and treatment strategies for E. coli mastitis in cattle.
Introduction
Domesticated cattle not only provide a significant source of nutrition but also provide a livelihood for nearly 6.6 billion humans (Elsik et al., 2009). Bacterial infection is one of the most important enemies of the cattle farming industry, causing significant losses. For example, bovine mastitis caused mostly by bacterial infection, resulting in losses of up to $2 billion per year in the United States alone. Aside from the economic losses, mastitis can impair animal welfare, and poses a threat to human health since it may be responsible for transfer of antimicrobial resistance and for food poisoning (Johler et al., 2015; Käppeli et al., 2019). Escherichia coli (E. coli) which as a prevalent environmental pathogen that routinely colonizes dairy cattle is one of the main pathogens causing mastitis (Buitenhuis et al., 2011). E. coli often leads to severe clinical mastitis and induces a distinct acute phase response (APR) (Petzl et al., 2018).
To date, the conventional method of treatment for E. coli mastitis is the use of antibiotics. However, the treatment using antibiotics is often less than 50% effective and leads to premature culling in many cases (Schmelcher et al., 2015). Alternative options are urgently needed for E. coli mastitis treatment. A deeper understanding of the molecular basis of E. coli mastitis may uncover new ways in battling this costly disease. Moreover, the comprehensive molecular investigation of E. coli mastitis may aid in the identification of new biomarkers for the rapid detection, and personalized therapy.
Microarray is a gene detection technique; using microarray can quickly detect the gene expression information in animals under infectious disease, which is particularly suitable for differentially expressed gene (DEG) screening. With the widespread use of microarray technology, a large amount of raw data about gene expression has been generated, and most of the data have been stored in public databases. The integration and reanalysis of these raw data can provide valuable information for new researches (Guo et al., 2017). In recent years, many microarray data analysis studies on E. coli mastitis have been carried out, and hundreds of DEGs have been identified (Han, 2019). For instance, a study identified 928 DEGs involved in the mammary gland with E. coli mastitis (Buitenhuis et al., 2011). Another study found 2,154 DEGs in E. coli mastitis vs. control treatment (Mitterhuemer et al., 2010). The results of different independent studies are always limited by the samples with environment, breed, population, and specific animal differences. Therefore, it is difficult to use differential genes obtained in a single independent study as biomarkers of E. coli mastitis. So far, the performance of the most mastitis detection systems do not meet the high accuracy required for clinical diagnosis needs of mastitis in cattle (Jensen et al., 2016). The aim of developmental research is to enhance the diagnostic efficiency of bovine mastitis with including several biomarkers on one test strip. The integrated bioinformatics methods combining with microarray technique will be innovative and might promote the appearance of test strip containing several biomarkers.
There are many gene expression profiles of E. coli–treated samples in the NCBI–Gene Expression Omnibus (NCBI-GEO) database. Most of these studies use primary mammary epithelial cells as test subjects to obtain data, and a small part of them were in vivo experiments using cows. However, primary mammary epithelial cells experiments are of limited significance because the in vitro cell testing does not mimic the complex environment with interactions between pathogens, antimicrobials, and components of the host’s immune response inside the mammary gland. Therefore, we integrated microarray data sets obtained from in vivo experiments with live E. coli infection to identify key candidate genes and pathways in E. coli mastitis of cows. It is anticipated that these results may provide more accurate, practically reliable biomarkers for early diagnosis and individualized prevention and therapy of bovine E. coli mastitis.
Materials and Methods
Data Set Collection and Identification of DEGs
The transcription profile data sets of bovine mammary gland with or without live E. coli infection were downloaded from NCBI-GEO database (https://www.ncbi.nlm.nih.gov/gds/). The accession number was GSE15020, GSE24217, and GSE50685. These studies used the “Affymetrix Bovine Genome Array” platform GPL2112, which contains 24,128 genes. A total of 22 E. coli mastitis cases and 19 normal mammary gland data were obtained (Table 1). The raw data (.CEL files) of these three microarray data sets were downloaded.
R software (Version 3.5.1; https://www.r-project.org/), affy package (https://bioconductor.org/packages/release/bioc/html/affy.html), and affyPLM (http://bioconductor.org/packages/release/bioc/html/affyPLM.html) package were used for raw data analysis (Li et al., 2019). The raw data in CEL format were converted into expression measures. Log scale robust multi-array analysis (RMA) background correction, quantile normalization, pmonly (perfect match correction), and median polish were performed in the R software (Li et al., 2019). RMA functions are provided by affy package (Irizarry et al., 2003). After these processes, we got the gene expression matrix. Eventually, the Linear Models for Microarray Data (LIMMA; http://www.bioconductor.org/packages/release/bioc/html/limma.html) package from Bioconductor was applied to identify DEGs by comparing expression value in mammary gland with or without E. coli infection. DEGs were identified with classical t test. A |log2 fold change (FC)| > 1 and P value < 0.05 were regarded as the cutoff criterion for DEGs.
Enrichment Analyses of DEGs
In this study, notably relevant Kyoto Encyclopedia of Genes and Genomes (KEGG) pathway and Gene Ontology (GO) analyses were carried out using the DAVID (Database for Annotation, Visualization and Integrated Discovery) Bioinformatics Resources 6.8 (https://david.ncifcrf.gov/). P < 0.01 was chosen as the cutoff criteria. R software was used for data visualization.
Identification of Key Candidate Genes With Protein–Protein Interaction (PPI) Network Analysis and Module Mining
The STRING database (Version 11.0; https://string-db.org/) was used to annotate functional interactions between DEGs and other genes. Based on this information, PPI network was visualized by Cytoscape (Version 3.7.1) (Smoot et al., 2010). Then Cytoscape plugin: MCODE and CentiScape were used to search modules of highly inter-connected nodes from the PPI network complex. Moreover, the function and pathway enrichment analysis of DEGs in the modules were performed by using DAVID.
Results
Identification of DEGs
To identify DEGs in E. coli mastitis, three original microarray data sets were downloaded from NCBI-GEO database. Using the calculating criteria of P < 0.05 and absolute log2 FC > 1, we extracted 663, 181, and 192 DEGs from the expression profile data sets GSE15020, GSE24217, and GSE50685, respectively. Employing R software and ggplot2 package, we developed Volcano Plot of the DEGs for the three expression profile data sets (Figure S1). The genes identified are in the same direction from each study. Next, we integrated the three groups of DEGs and performed bioinformatics analysis. The results are shown in Figure 1. A total of 105 DGEs were identified from the three profile data sets, including 98 up-regulated genes, 6 down-regulated genes, and 1 aberrantly expressed gene SLC2A3 in the E. coli treatment samples compared to healthy samples (Table 2).
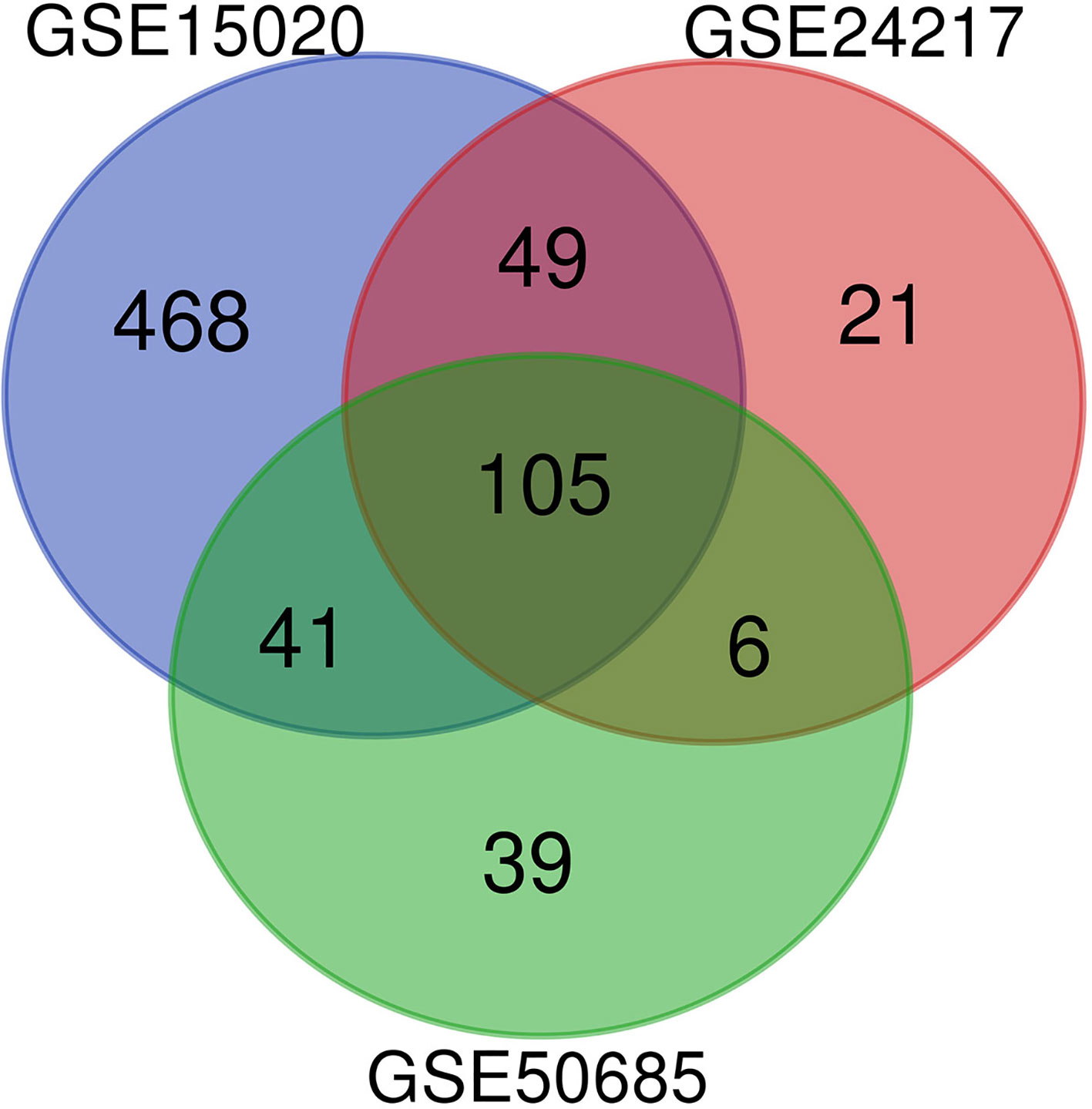
Figure 1 The DEGs between (E. coli) mastitis and normal tissues in three original microarray data sets (GSE15020, GSE24217, and GSE50685) were identified.

Table 2 One hundred and five DEGs were identified from the three profile data sets, including 98 up-regulated genes, 6 down-regulated genes, and 1 aberrantly expressed gene SLC2A3 in the E. coli treatment samples, compared to healthy samples.
Functional and Pathway Enrichment Analysis
To acquire further understanding of the functions of identified DEGs, all DEGs were uploaded to DAVID. GO biological process (BP) terms and KEGG pathways were enriched for 105 candidate DEGs in E. coli mastitis. The top 20 BP terms according to P value are shown in Figure 2. The data showed that DEGs were mainly involved in GO terms about immunity such as inflammatory response, immune response, innate immune response. DEGs mainly enriched in extracellular space, extracellular exosome, extracellular region, and external side of plasma membrane in the molecular function term. In these candidate DEGs of E. coli mastitis, 27 KEGG pathways were found to be enriched with P < 0.05 as the cutoff point, such as the TNF signaling pathway, chemokine signaling pathway, and cytokine–cytokine receptor interaction (Figure 3).
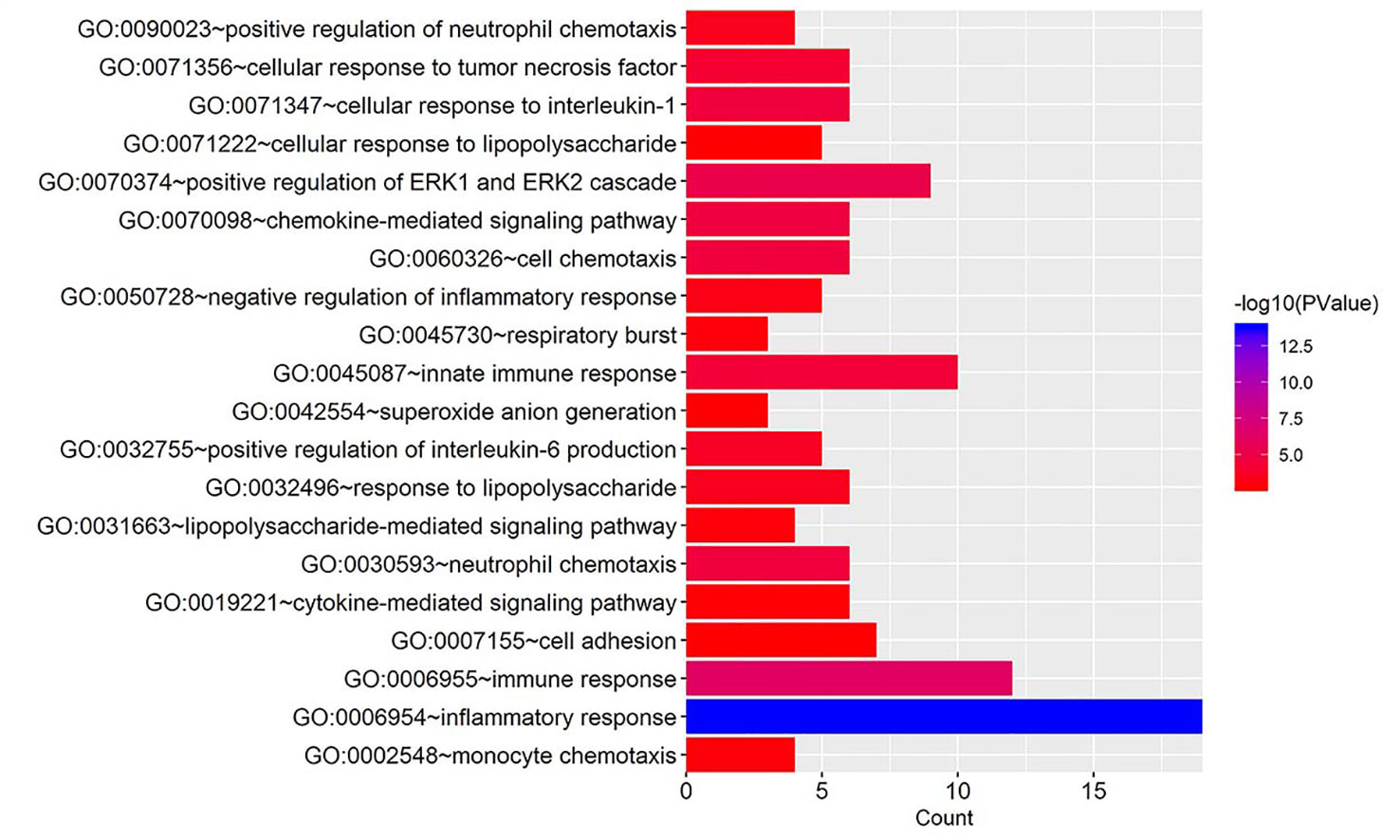
Figure 2 Gene ontology analysis of DEGs associated with E. coli mastitis. The biological process (BP) in functional enrichment of DEGs was performed using the online biological tool, DAVID, with count and P value.
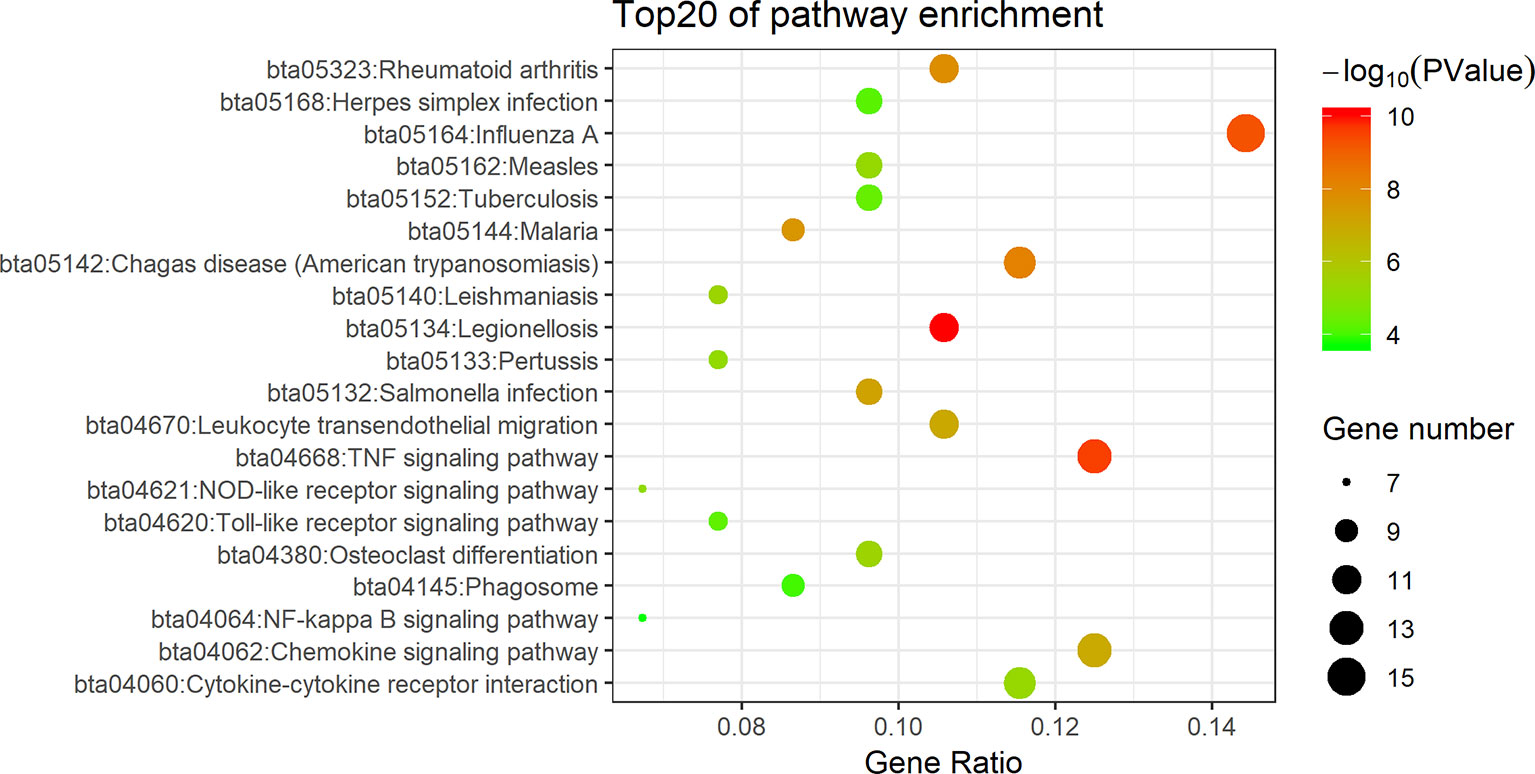
Figure 3 Signaling pathway analysis of DEGs associated with E. coli mastitis. The Kyoto Encyclopedia of Genes and Genomes (KEGG) pathways enrichment of DEGs was performed using the online biological tool DAVID with gene ratio, gene number, and P value.
Identification of Key Candidate Genes With PPI Network Analysis and Modular Analysis
The PPI network of DEGs was constructed in the STRING database which has 102 nodes and 546 edges. A total of 102 DEGs (except BASP1, BNBD-9-LIKE, and GPX1) were filtered into the DEGs PPI network complex. The PPI network was visualized by using Cytoscape software (Figure 4). Based on the STRING database, the DEGs with the highest PPI scores identified by the Cytoscape plugin: MCODE and CentiScape with three centrality methods were shown in Table 3. Among the 102 nodes, 37 central node genes were identified with the filtering of node degree >10. The most significant 10 node degree genes were shaped as diamond with yellow in Figure 4. The module with the highest score through MCODE analysis was filtered from PPI. This module consisted of 18 nodes and 144 edges (Figure 5), which are mainly associated with inflammatory response, immune response, TNF signaling pathway, and rheumatoid arthritis, etc. (Tables 4 and 5). All the 18 genes belong to high PPI score genes (Table 3). The log2 FC of these genes in three microarray data sets were summarized, and the top 10 genes with high log2 FC were listed in Table 6. These genes are significantly up-regulated in E. coli mastitis tissues. These genes are key genes associated with E. coli mastitis.
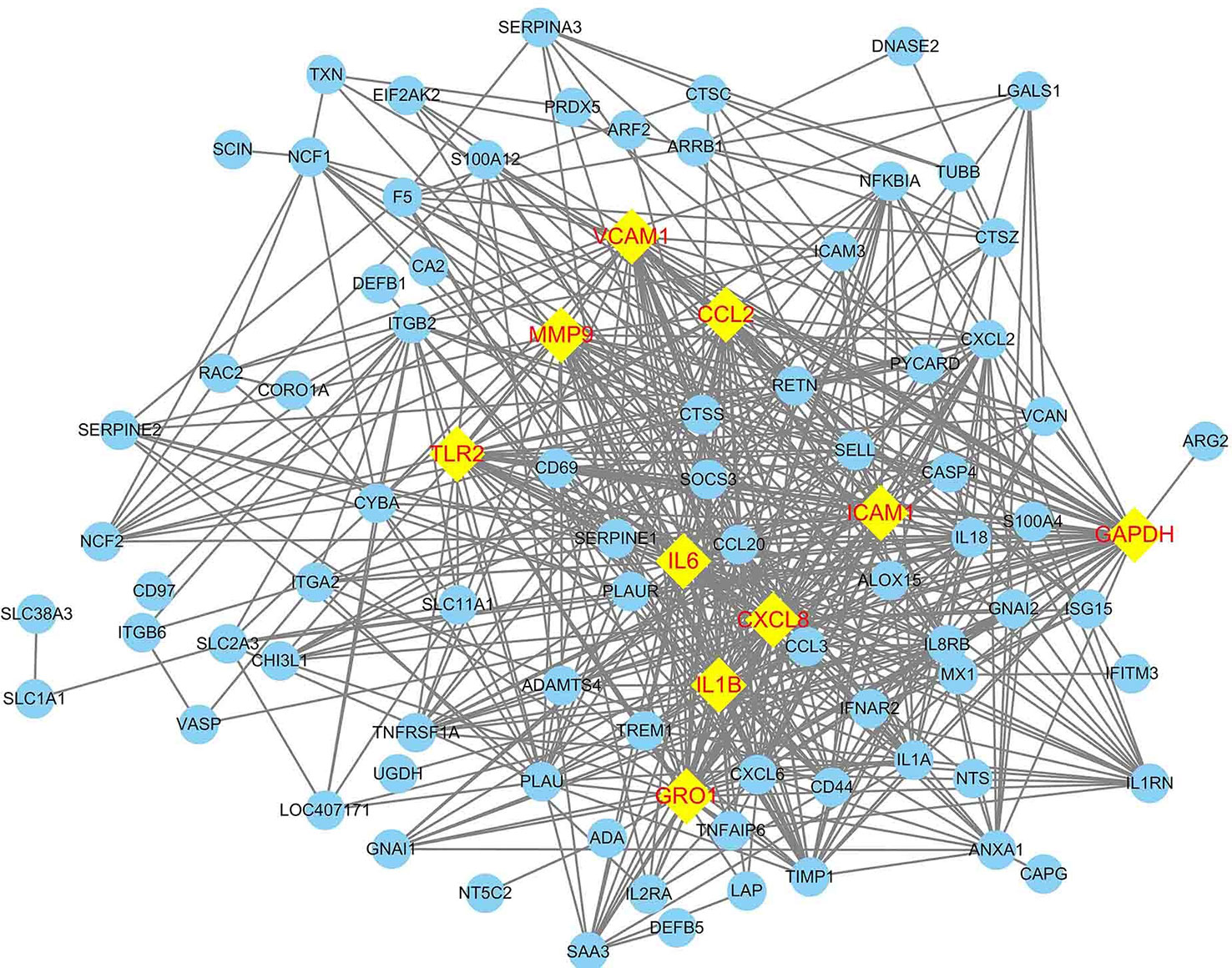
Figure 4 Construction of protein–protein interaction (PPI) network of DEGs associated with E. coli mastitis. The nodes with higher degrees were shaped as diamond in yellow.
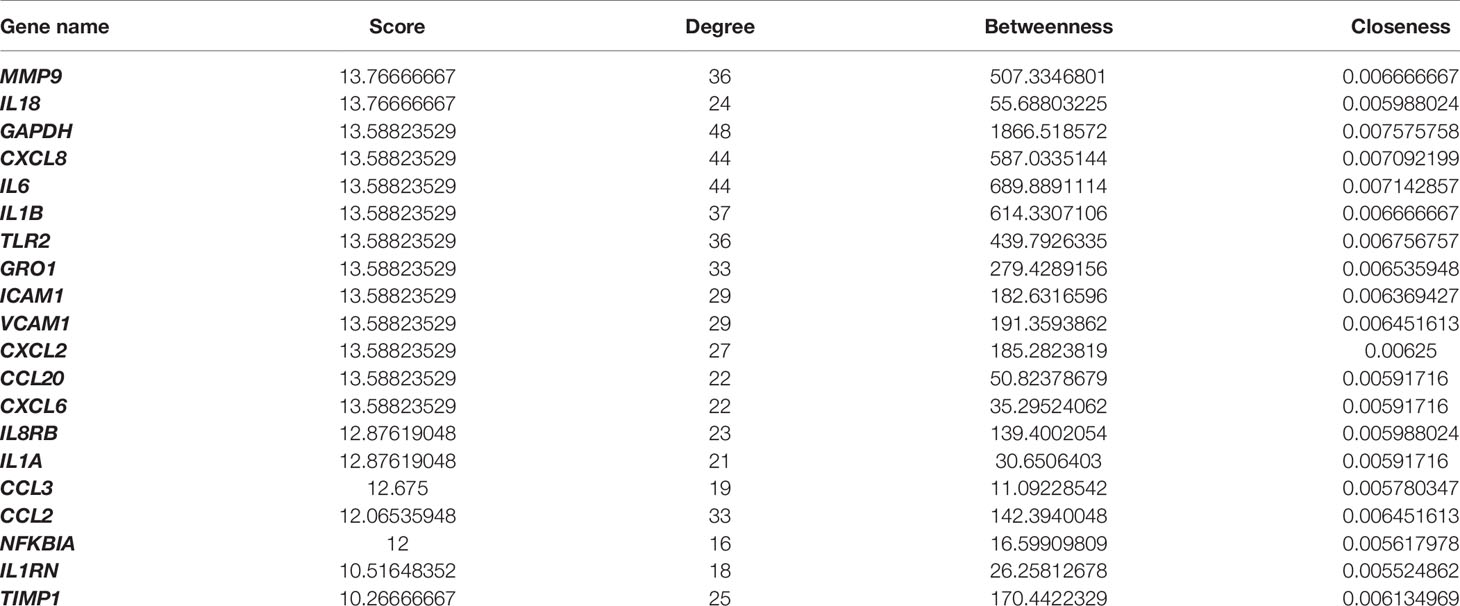
Table 3 The DEGs with PPI scores >10 identified by the MCODE and CentiScape with three centrality methods.
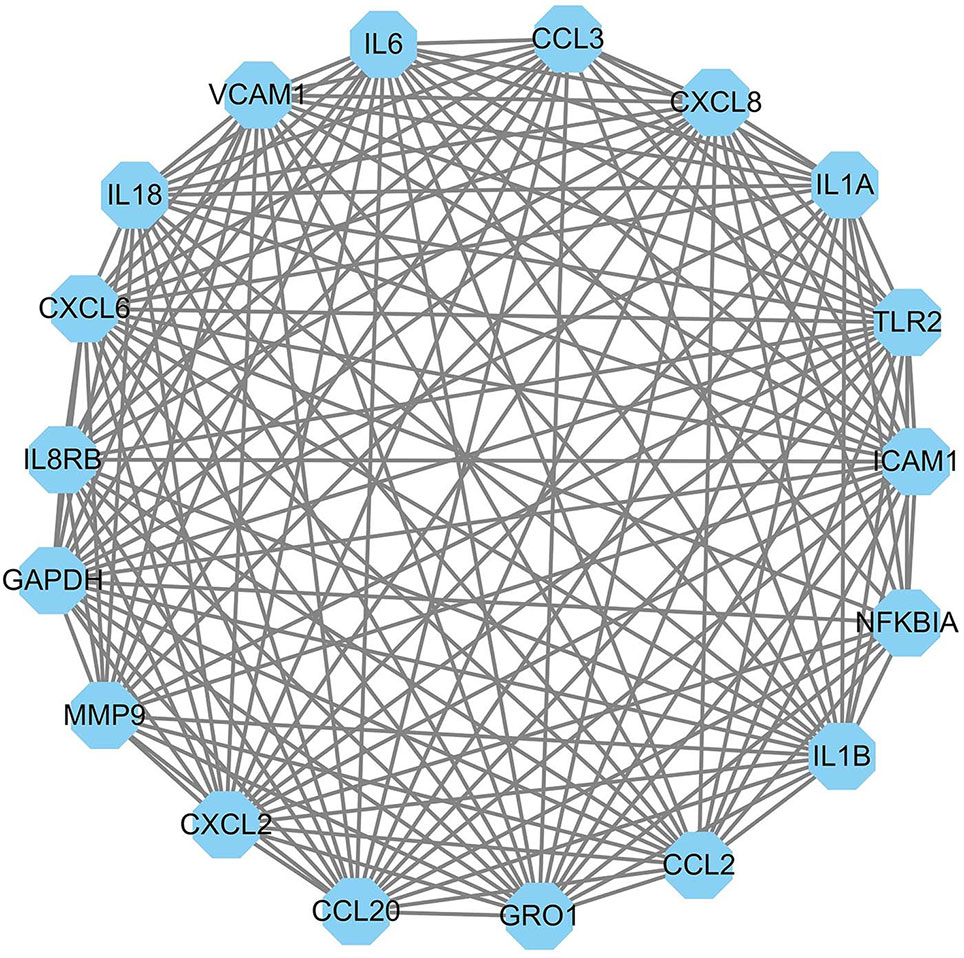
Figure 5 The most significant module of the PPI network complex of DEGs associated with E. coli mastitis. The module consists of 18 nodes and 144 edges, which are mainly associated with immune response.
Discussion
Despite the research on mastitis having lasted for a long time, the detrimental economic losses caused by mastitis remains unchanged (Petzl et al., 2018). Early diagnosis is very important due to the high costs of mastitis. However, most current detection systems do not meet the high accuracy required for clinical diagnosis needs of mastitis (Jensen et al., 2016). Nowadays, somatic cell count (SCC) and California mastitis test (CMT) are often used in the diagnosis of mastitis. Mastitis can cause significant milk changes such as the presence of clots in milk, milk discoloration, and high levels of leukocyte numbers which lead to a rise in SCC. Therefore, SCC has been used as the gold standard for decades to diagnose subclinical mastitis. As SCC requires submission of sample to a laboratory for automated cell counting, it’s very time-consuming. In addition, SCCs do not always correlate with mastitis, and they may be affected by other factors (e.g., lactation number, stage of lactation, milk production level, stress, season, and breed) (Duarte et al., 2015). CMT is based on the principle of the addition of a detergent to a milk sample with a high cell count which promotes cell lysis, nucleic acid release, and formation of a “gel-like” matrix. The CMT is quick, cheap, and simple, but the interpretation can be subjective, and this might result in false positives and negatives (Viguier et al., 2009).
Therefore, it is urgent to develop a new diagnosis system which could be adapted to rapid, on-farm diagnostic systems. Development of new biomarkers for diagnosis of mastitis has been considered as the trend of mastitis detection (Duarte et al., 2015). A biomarker is a characteristic that can be measured and evaluated as an indicator of pathological processes, or pharmacological responses to therapeutic interventions. Genes coding for proteins such as haptoglobin, cathelicidin antimicrobial peptide, and lingual antimicrobial peptide have been identified as potential biomarkers for mastitis detection (Sharifi et al., 2018). At present, antibiotics are mainly used as systemic treatment of mastitis (Porter et al., 2016). Antibiotic should be chosen based on specific mastitis pathogen. Therefore, the exploration of biomarkers for a specific pathogen is also conducive to the selection of appropriate antibiotic therapy. Combined with previous studies on biomarkers, the genes detected in this experiment have the potential to develop test strips which include several biomarkers on one test strip, and this can enhance the diagnostic efficiency.
Here, we performed bioinformatical analysis on three microarray profile data sets to identify key genes that may be significantly involved in response to bovine E. coli mastitis. To obtain unique genes and pathways associated with E. coli mastitis, for the first time, we only selected original microarray data sets obtained from in vivo experiments with live E. coli infection. The results confirmed the most important findings in previous individual studies such as induction of the responses related to immune response, inflammation, and TNF signaling pathway (Buitenhuis et al., 2011; Günther et al., 2011). In the current research, the top-ranked genes were CXCL8, CXCL2, IL8RB, CXCL6, GRO1, IL6, IL1B, MMP9, ICAM1, and TLR2 (Table 6). This result similar to previous studies such as Sharifi et al. (2018), who found CXCL8, CXCL2, and GRO1 were the top three genes associated with E. coli mastitis, and Han (2019) found CXCL2 and GRO1 were key genes in both the E. coli and the Staphylococcus aureus mastitis. The consistency of these key genes proves the validity of our study.
Importantly, new key genes and pathways associated with E. coli mastitis were obtained by this bioinformatical analysis. IL8RB and CXCL6 are key genes newly identified through our analysis. More importantly, for the first time, this study found that the DEGs associated with E. coli mastitis were involved in signaling pathways triggered by other diseases (Table 5). For example, CXCL8, CXCL2, or GRO1 were involved in rheumatoid arthritis, legionellosis, salmonella infection, and so on. The new finding may contribute to understanding the molecular basis of mastitis pathogenesis. However, this also indicates that those genes may not be suitable as a molecular biomarker of E. coli mastitis on account of biomarker should be specific for a disease and should remain unchanged by unrelated disorders. Therefore, after removing genes that cross with other diseases, only IL8RB, CXCL6, and MMP9 have the potential to be biomarker of E. coli mastitis in this study.
Interleukin 8 (IL8) is an important chemokine and plays a major role in the recruitment of neutrophils and lymphocytes from peripheral sites to the mammary gland during E. coli mastitis. IL8 has been confirmed to be produced by epithelial cells in the mammary gland respond to E. coli infection (Boehmer et al., 2008). However, these effects of IL8 must be achieved by binding to the corresponding receptors; during this process, IL8RB is the receptor gene of IL8. Moreover, IL8RB has exhibited an important role in immune function during mastitis infection, and it belongs to the promising candidate genes contributing in bovine mastitis (El Nahas et al., 2017). Consistent with the above research, in this study, IL8RB was identified as a key gene in response to E. coli mastitis. Therefore, its role during E. coli mastitis should be further studied.
Chemokines are a family of proteins with diverse functions that mediate a variety of inflammatory reactions (Gray et al., 2005). Chemokine ligand 6 (CXCL6) is mainly secreted by macrophages, epithelial cells, and stromal cells and has chemotactic granulocytes and antimicrobial and immune functions. CXCL6 is also known as granulocyte chemotactic protein 2 (GCP-2). There was evidence that indicated the up-regulation of CXCL6 gene expression in bovine mammary epithelial cell line with bacterial infection (Yu et al., 2010) and in infected gland (Rinaldi et al., 2010). Combined with the analysis of this study, it shows again that CXCL6 plays an important role in cow responses to E. coli mastitis.
Matrix metalloproteinases (MMPs) are a family of calcium-dependent zinc-containing endopeptidases which are synthesized and secreted by various cells, such as neutrophils and macrophages, and play important roles in inflammation (Vandooren et al., 2013) such as bovine mammary epithelial cell with E. coli mastitis (Zhao and Lacasse 2008). This family of proteases have five subfamilies, of which MMP9 is one of the most studied. It has been shown that the levels of expression of MMP9 in cases of bovine E. coli mastitis (Caggiano et al., 2019) and in goat mammary gland epithelial cells with S. aureus infection (Li et al., 2016) were increased. Considering all the results, MMP9 may be a good candidate for biomarker of E. coli mastitis diagnosis.
In view of the fact that the performances of the most current bovine mastitis detection systems do not meet the high accuracy required for clinical diagnosis needs of mastitis (Jensen et al., 2016), the main purpose of this study is to identify potential genes that can be used as biomarkers for the diagnosis of E. coli mastitis. Although these genes have been identified in this study and had been preliminarily confirmed in previous animal or cell experiments, these genes still need more laboratory-based studies and farm practice to confirm.
Conclusions
A total of 105 DEGs related to mastitis were identified from microarray data sets of in vivo experiments with live E. coli infection. After further integrated bioinformatical analysis, IL8RB, CXCL6, and MMP9 were identified as the most potential biomarker for E. coli mastitis. In conclusion, this study provided an extensive bioinformatics analysis of DEGs and revealed a series of targets and pathway for further study to battle with E. coli mastitis.
Data Availability Statement
Publicly available datasets were analyzed in this study. This data can be found here: GSE15020, GSE24217, GSE50685.
Author Contributions
LL designed the experiments. LL, XC, and ZC did the data analysis and wrote the paper. All authors read and approved the final manuscript.
Funding
This work was supported by a Startup Fund of Hainan University [KYQD(ZR) 1940] to LL.
Conflict of Interest
The authors declare that the research was conducted in the absence of any commercial or financial relationships that could be construed as a potential conflict of interest.
Supplementary Material
The Supplementary Material for this article can be found online at: https://www.frontiersin.org/articles/10.3389/fgene.2019.01251/full#supplementary-material
References
Boehmer, J., Bannerman, D., Shefcheck, K., Ward, J. (2008). Proteomic analysis of differentially expressed proteins in bovine milk during experimentally induced Escherichia coli mastitis. J. Dairy Sci. 91 (11), 4206–4218. doi: 10.3168/jds.2008-1297
Buitenhuis, B., Rontved, C. M., Edwards, S. M., Ingvartsen, K. L., Sorensen, P. (2011). In depth analysis of genes and pathways of the mammary gland involved in the pathogenesis of bovine Escherichia coli-mastitis. BMC Genomics 12, 130. doi: 10.1186/1471-2164-12-130
Caggiano, N., Lorenzo Smirnoff, A., Bottini, J. M., De Simone, E. A. (2019). Protease activity and protein profile in milk from healthy dairy cows and cows with different types of mastitis. Int. Dairy J. 89, 1–5. doi: 10.1016/j.idairyj.2018.07.020
Duarte, C. M., Freitas, P. P., Bexiga, R. (2015). Technological advances in bovine mastitis diagnosis: an overview. J. Vet. Diagn. Invest. 27, 665–672. doi: 10.1177/1040638715603087
El Nahas, S., Mossallem, A. A., Abdelhamid, M., Warda, M. (2017). A study on IL8RB gene polymorphism as a potential immuno-compromised adherent in exaggeration of parenteral and mammo-crine oxidative stress during mastitis in buffalo. J. Adv. Res. 8 (6), 617–625. doi: 10.1016/j.jare.2017.07.002
Elsik, C. G., Tellam, R. L., Worley, K. C. (2009). The genome sequence of taurine cattle: a window to ruminant biology and evolution. Science 324 (5926), 522–528. doi: 10.1126/science.1169588
Günther, J., Esch, K., Poschadel, N., Petzl, W., Zerbe, H., Mitterhuemer, S., et al. (2011). Comparative kinetics of Escherichia coli-and Staphylococcus aureus-specific activation of key immune pathways in mammary epithelial cells demonstrates that S. aureus elicits a delayed response dominated by interleukin-6 (IL-6) but not by IL-1A or tumor necrosis factor alpha. Infect. Immun. 79 (2), 695–707. doi: 10.1128/IAI.01071-10
Gray, C., Strandberg, Y., Donaldson, L., Tellam, R. (2005). Bovine mammary epithelial cells, initiators of innate immune responses to mastitis. Aust. J. Exp. Agr. 45 (8), 757–761. doi: 10.1071/EA05046
Guo, Y., Bao, Y., Ma, M., Yang, W. (2017). Identification of key candidate genes and pathways in colorectal cancer by integrated bioinformatical analysis. Int. J. Mol. Sci. 18, 722. doi: 10.3390/ijms18040722
Han, H. (2019). Identification of several key genes by microarray data analysis of bovine mammary gland epithelial cells challenged with Escherichia coli and Staphylococcus aureus. Gene. 683, 123–132. doi: 10.1016/j.gene.2018.10.004
Irizarry, R. A., Bolstad, B. M., Collin, F., Cope, L. M., Hobbs, B., Speed, T. P. (2003). Summaries of Affymetrix GeneChip probe level data. Nucleic Acids Res. 31, e15–e15. doi: 10.1093/nar/gng015
Jensen, D. B., Hogeveen, H., De Vries, A. (2016). Bayesian integration of sensor information and a multivariate dynamic linear model for prediction of dairy cow mastitis. J. Dairy Sci. 99 (9), 7344–7361. doi: 10.3168/jds.2015-10060
Johler, S., Weder, D., Bridy, C., Huguenin, M.-C., Robert, L., Hummerjohann, J., et al. (2015). Outbreak of staphylococcal food poisoning among children and staff at a Swiss boarding school due to soft cheese made from raw milk. J. Dairy Sci. 98, 2944–2948. doi: 10.3168/jds.2014-9123
Käppeli, N., Morach, M., Zurfluh, K., Corti, S., Nüesch-Inderbinen, M., Stephan, R. (2019). Sequence types and antimicrobial resistance profiles of Streptococcus uberis isolated from bovine mastitis. Front. Vet. Sci. 6, 234. doi: 10.3389/fvets.2019.00234
Li, Y., Li, H., Zheng, H., Li, L., Shen, X., Zang, W., et al. (2016). The effects of matrix metalloproteinase-9 on dairy goat mastitis and cell survival of goat mammary epithelial cells. PloS One 11 (8), e0160989. doi: 10.1371/journal.pone.0160989
Li, W. C., Bai, D. L., Xu, Y., Chen, H., Ma, R., Hou, W. B., et al. (2019). Identification of differentially expressed genes in synovial tissue of rheumatoid arthritis and osteoarthritis in patients. J. Cell Biochem. 120 (3), 4533–4544. doi: 10.1002/jcb.27741
Mitterhuemer, S., Petzl, W. K. S., Mehne, D., Klanner, A., Wolf, E., Zerbe, H., et al. (2010). Escherichia coli infection induces distinct local and systemic transcriptome responses in the mammary gland. BMC Genomics 11 (1), 138. doi: 10.1186/1471-2164-11-138
Petzl, W., Zerbe, H., Gunther, J., Seyfert, H. M., Hussen, J., Schuberth, H. J. (2018). Pathogen-specific responses in the bovine udder. Models Immunoprophylactic Concepts Res. Vet. Sci. 116, 55–61. doi: 10.1016/j.rvsc.2017.12.012
Porter, J., Anderson, J., Carter, L., Donjacour, E., Paros, M. (2016). In vitro evaluation of a novel bacteriophage cocktail as a preventative for bovine coliform mastitis. J. Dairy Sci. 99, 2053–2062. doi: 10.3168/jds.2015-9748
Rinaldi, M., Li, R. W., Capuco, A. V. (2010). Mastitis associated transcriptomic disruptions in cattle. Vet. Immunol. Immunop. 138 (4), 267–279. doi: 10.1016/j.vetimm.2010.10.005
Schmelcher, M., Powell, A. M., Camp, M. J., Pohl, C. S., Donovan, D. M. (2015). Synergistic streptococcal phage lambdaSA2 and B30 endolysins kill streptococci in cow milk and in a mouse model of mastitis. Appl. Microbiol. Biotechnol. 99 (20), 8475–8486. doi: 10.1007/s00253-015-6579-0
Sharifi, S., Pakdel, A., Ebrahimi, M., Reecy, J. M., Fazeli Farsani, S., Ebrahimie, E. (2018). Integration of machine learning and meta-analysis identifies the transcriptomic bio-signature of mastitis disease in cattle. PloS One 13, e0191227. doi: 10.1371/journal.pone.0191227
Sipka, A., Klaessig, S., Duhamel, G. E., Swinkels, J., Rainard, P., Schukken, Y. (2014). Impact of intramammary treatment on gene expression profiles in bovine Escherichia coli mastitis. PloS One 9 (1), e85579. doi: 10.1371/journal.pone.0085579
Smoot, M. E., Ono, K., Ruscheinski, J., Wang, P.-L., Ideker, T. (2010). Cytoscape 2.8: new features for data integration and network visualization. Bioinformatics 27 (3), 431–432. doi: 10.1093/bioinformatics/btq675
Vandooren, J., Van Den Steen, P. E., Opdenakker, G. (2013). Biochemistry and molecular biology of gelatinase B or matrix metalloproteinase-9 (MMP-9): the next decade. Crit. Rev. Biochem. Mol. 48 (3), 222–272. doi: 10.3109/10409238.2013.770819
Viguier, C., Arora, S., Gilmartin, N., Welbeck, K., O’Kennedy, R. (2009). Mastitis detection: current trends and future perspectives. Trends Biotechnol. 27, 486–493. doi: 10.1016/j.tibtech.2009.05.004
Yu, C., Shi, Z.-R., Chu, C.-Y., Lee, K.-H., Zhao, X., Lee, J.-W. (2010). Expression of bovine granulocyte chemotactic protein-2 (GCP-2) in neutrophils and a mammary epithelial cell line (MAC-T) in response to various bacterial cell wall components. Vet. J. 186 (1), 89–95. doi: 10.1016/j.tvjl.2009.07.012
Keywords: bovine mastitis, Escherichia coli, differentially expressed gene, pathway, biomarker
Citation: Li L, Chen X and Chen Z (2019) Identification of Key Candidate Genes in Dairy Cow in Response to Escherichia coli Mastitis by Bioinformatical Analysis. Front. Genet. 10:1251. doi: 10.3389/fgene.2019.01251
Received: 09 July 2019; Accepted: 13 November 2019;
Published: 06 December 2019.
Edited by:
Jesús Fernández, Instituto Nacional de Investigación y Tecnología Agraria y Alimentaria (INIA), SpainReviewed by:
Nora Lía Padola, National University of Central Buenos Aires, ArgentinaAmanda K. Lindholm-Perry, United States Department of Agriculture, United States
Copyright © 2019 Li, Chen and Chen. This is an open-access article distributed under the terms of the Creative Commons Attribution License (CC BY). The use, distribution or reproduction in other forums is permitted, provided the original author(s) and the copyright owner(s) are credited and that the original publication in this journal is cited, in accordance with accepted academic practice. No use, distribution or reproduction is permitted which does not comply with these terms.
*Correspondence: Liabin Li, 993992@hainanu.edu.cn