- 1Department of Cardiovascular Medicine, First Affiliated Hospital of Xi’an Jiaotong University, Xi’an, China
- 2Department of Biostatistics and Bioinformatics, Emory University, Atlanta, GA, United States
Identifying effective pharmacological treatments for heart failure (HF) patients remains critically important. Given that the development of drugs de novo is expensive and time consuming, drug repositioning has become an increasingly important branch. In the present study, we propose a two-step drug repositioning pipeline and investigate the novel therapeutic effects of existing drugs approved by the US Food and Drug Administration to discover potential therapeutic drugs for HF. In the first step, we compared the gene expression pattern of HF patients with drug-induced gene expression profiles to obtain preliminary candidates. In the second step, we performed a systems biology approach based on the known protein–protein interaction information and targets of drugs to narrow down preliminary candidates to obtain final candidates. Drug set enrichment analysis and literature search were applied to assess the performance of our repositioning approach. We also constructed a mode of action network for each candidate and performed pathway analysis for each candidate using genes contained in their mode of action network to uncover pathways that potentially reflect the mechanisms of candidates’ therapeutic efficacy to HF. We discovered numerous preliminary candidates, some of which are used in clinical practice and supported by the literature. The final candidates contained nearly all of the preliminary candidates supported by previous studies. Drug set enrichment analysis and literature search support the validity of our repositioning approach. The mode of action network for each candidate not only displayed the underlying mechanisms of drug efficacy but also uncovered potential biomarkers and therapeutic targets for HF. Our two-step drug repositioning approach is efficient to find candidates with potential therapeutic efficiency to HF supported by the literature and might be of particular use in the discovery of novel effective pharmacological therapies for HF.
Introduction
Heart failure (HF) remains a worsening global public health problem with high mortality (Mosterd et al., 2001; Askoxylakis et al., 2010). Although the use of neuro-hormonal interventions in the forefront of HF treatment has substantially reduced mortality and improved the prognosis, morbidity and mortality remain unacceptably high (Mosterd et al., 2001; Askoxylakis et al., 2010; Lewis et al., 2017). The mortality of HF patients within 5 years is >50%, higher than most malignancies (Askoxylakis et al., 2010). Thus, there is an urgent need to identify new effective therapeutic treatments functioning through different mechanisms compared to existing treatments in order to improve the prognoses of HF patients.
However, developing drugs de novo is expensive and time consuming. To bring a new drug to market, it takes an average of 10 years and at least $1 billion in research and development (Xue et al., 2018). Given the time and cost of developing novel drugs, drug repositioning has become an increasingly important strategy, and many different computational methods have been developed to facilitate this process (Lamb et al., 2006; March-Vila et al., 2017; Subramanian et al., 2017; Peyvandipour et al., 2018). Drug repositioning aims to identify new applications for existing drugs to treat different diseases (Novac, 2013), which eliminates the need for preclinical development and optimization, hence saving effort, expense, and avoiding the high rate of failures that are typically associated with the new drug discovery process.
Classical drug repositioning methods include ligand-based approaches (Keiser et al., 2009; Liu et al., 2010; Vasudevan et al., 2012; Anighoro et al., 2015; Iwata et al., 2015; Mervin et al., 2015; Sawada et al., 2015) and structure-based approaches (Manning et al., 2002; Zhang et al., 2004; Kinnings et al., 2009; Defranchi et al., 2010; Jalencas and Mestres, 2013; Bowman et al., 2015; Hall et al., 2015; Ehrt et al., 2016). The concept for ligand-based approaches is that similar compounds are likely to have similar biological properties. Structure-based approaches are based on the concept that proteins with similar structures tend to have similar functions and to bind similar compounds. These two approaches are based on existing information regarding drugs and proteins. Drugs identified using these two types of methods share the same mechanisms as the template drugs. For a certain disease, if the template drugs have already been used as treatments, these approaches will play a limited role, and thus, other types of drug repositioning approaches able to find therapeutic drugs with different mechanisms compared to the existing treatments are required.
As we enter the “big data” era, given the generation and wide access of disease and drug-induced gene expression profiling data, such as the Library of Integrated Network-based Cellular Signatures (LINCS) L1000 dataset (Subramanian et al., 2017) as an eminent repository for pharmacogenomics and the expansion of the Connectivity Map (Cmap) (Lamb et al., 2006), noval, data-driven approaches have been developed to explore the possibility of drug repositioning through intelligent data mining to find drugs that function through different mechanisms compared to existing treatments (Lamb et al., 2006; Hu and Agarwal, 2009; Chang et al., 2010; Jin et al., 2012; Iskar et al., 2013; Subramanian et al., 2017; Peyvandipour et al., 2018). The first class of approaches is based on transcriptomic data. By comparing the gene expression patterns of diseases and drugs, therapeutic relationships between known drugs and new disease indications can be established (Lamb et al., 2006; Subramanian et al., 2017). Another class of methods aims at organizing the relationships among disease-related genes and drug targets in the form of a network to visualize the potential therapeutic efficiency of a drug (Peyvandipour et al., 2018). The approaches based on data mining can be used to find drugs that function through different mechanisms compared to existing treatments, but how to increase the likelihood of identifying actual disease-related candidate drugs remains a critical point.
In the present study, we constructed a two-step drug repositioning pipeline by combining a transcriptome-based approach and a network-based approach to find candidate drugs approved by the US Food and Drug Administration (FDA) for HF. Drug-set enrichment analysis and literature search were applied to validate our approach. We also constructed a mode of action (MOA) network for each candidate to investigate their potential MOA. Then, a pathway analysis was performed using genes contained in the MOA network of each candidate to uncover the potential mechanisms of candidate’s therapeutic efficacy to HF (Figure 1).
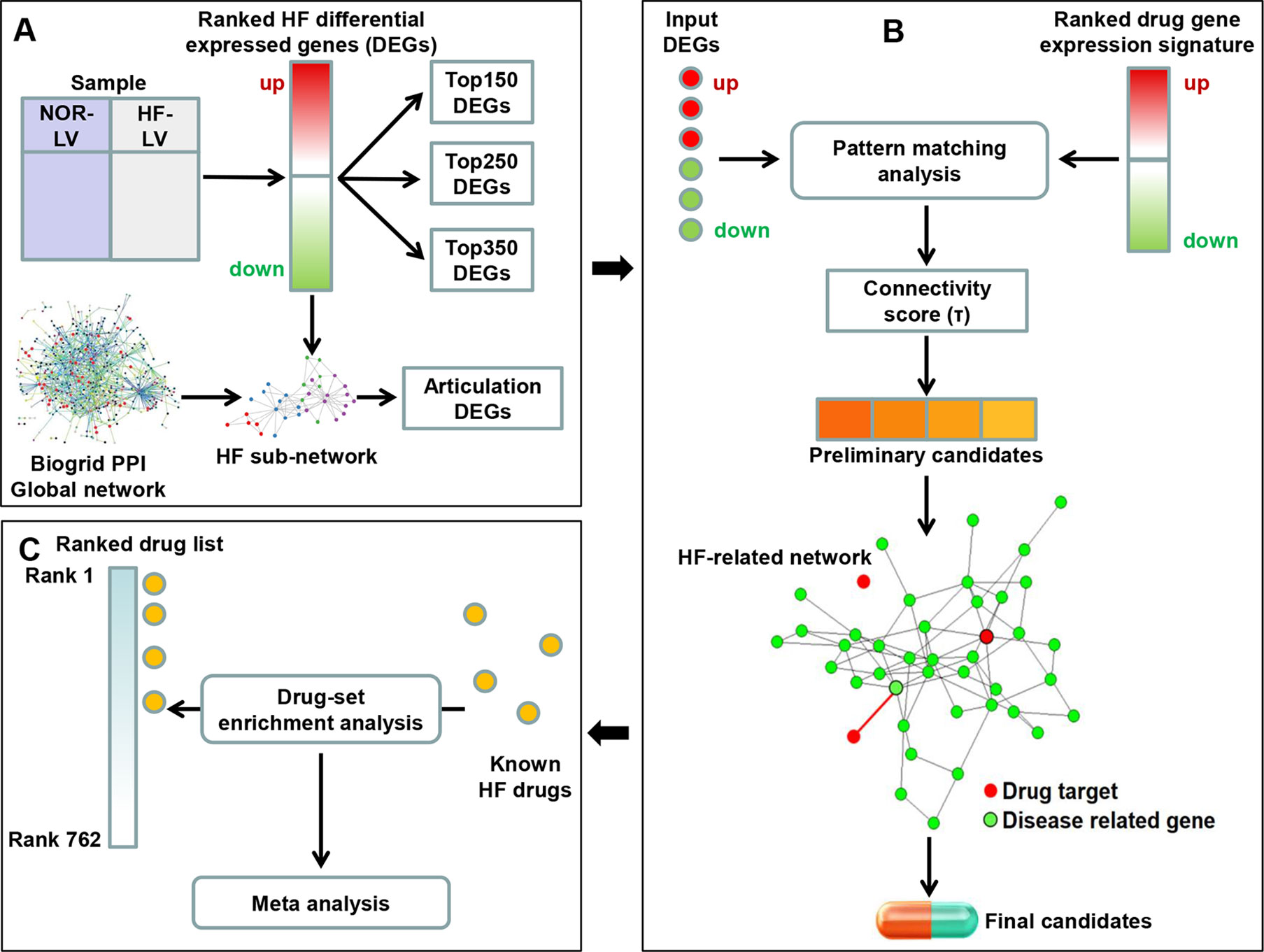
Figure 1 Framework overview. (A) We compared the gene expression profile of heart failure (HF) and normal human left ventricular (LV) tissues to obtain the differential expression genes (DEGs). The K-most top (up- and downregulated) DEGs were obtained according to the fold change values. We then extracted a HF subnetwork from the global protein–protein interaction network using all DEGs. Articulation DEGs of the subnetwork were extracted as inputs to perform subsequent drug repositioning analysis along with top K genes (K was set as 150, 250 and 350). (B) A pattern matching analysis was applied on input HF DEGs and drug-induced gene expression signatures. Connectivity score (τ) was calculated to assess the reversal of gene expression patterns and to select the preliminary candidates. Then, the interaction between the targets of preliminary candidates and HF-related network was investigated. Preliminary candidates whose targets located in the HF-related network were selected as final candidates. (C) Drug-set enrichment analysis was applied to determine whether known HF drugs are located higher in the whole drug list ranked by τ. Results across all datasets were aggregated using meta-analysis.
Materials and Methods
Date Sources
HF Gene Expression Data
Gene expression data comparing HF and healthy human left ventricular tissues with a minimum sample size of 10 were downloaded from Gene Expression Omnibus (GEO). Twenty-one datasets, representing two HF subtypes: dilated cardiomyopathy (DCM) and ischemic cardiomyopathy (ISCM), satisfied the selection criteria (Supplemental Tables 1 and 2).
Drug-Induced Gene Expression Data and Drug Target Data
Drug-induced gene expression profiles were derived from the Library of Integrated Network-Based Cellular Signatures (LINCS) L1000 dataset (Subramanian et al., 2017), an eminent pharmacogenomics repository used for diverse purposes (Wang et al., 2016; Musa et al., 2018; Musa et al., 2019). The data are available in GEO (GSE92742), which contains transcriptional gene expression data from cultured human cells exposed to over 20,000 small molecules including most of the FDA-approved drugs. The LINCS L1000 dataset compares two groups of samples (control vs. treatment) and provides differentially expressed genes (DEGs) in terms of z-score signatures. Drug targets were retrieved from the database DrugBank, which combines detailed drug data with comprehensive drug target information (Wishart et al., 2018). Overall, we extracted 762 FDA-approved drugs with both gene expression signatures and known targets to perform the subsequent drug repositioning analysis.
Protein–Protein Interaction Network
Protein–protein interaction (PPI) network information was derived from the BioGRID database, one of the largest repositories of experimentally verified PPIs (Chatr-Aryamontri et al., 2017). We extracted all genetic and protein interactions for humans to build the global PPI network. In the global PPI network, each node is a gene, and the edges represent PPIs between nodes.
Known HF-Related Genes
HF-related genes were retrieved from the Comparative Toxicogenomics Database, a robust and publicly available database containing gene–disease association information (Mattingly et al., 2006). Genes associated to systolic HF were extracted for underlying analysis.
Data Analysis
Detection of DEGs
For HF gene expression data collected from microarray, R/Bioconductor package Limma was applied (Smyth, 2004); for HF gene expression data collected from RNAseq, R/Bioconductor package edgeR was used (Robinson and Smyth, 2007). The definition of DEGs was set to an adjusted P value (<0.05). We used the top (K-most up- and downregulated) DEGs according to the fold change to represent the most significant and important DEGs of HF and perform the subsequent drug repositioning analysis. Different values of K (150, 250, and 350) were attempted.
Articulation DEGs Selection
We constructed a global network based on all BioGRID PPIs. For each HF-related dataset, we extracted a subgraph from the global network using the function induced_subgraph built in the R/Bioconductor package igraph. The nodes of the subgraph represent all DEGs, and the edges of the subgraph refer to the experimentally verified PPIs among the DEGs. Then, the articulation points of each subgraph were defined as the articulation DEGs using the function articulation_points built in the R/Bioconductor package igraph. Articulation points are key vertices in a network, whose removal increases the number of connected components in a graph. The way of finding articulation DEGs is to remove all nodes of a network one by one, and the nodes whose removal results in disconnected graph are selected as articulation DEGs. The articulation DEGs were considered as another subset of the most significant and important DEGs of HF besides top DEGs and were used to conduct the subsequent drug repositioning analysis.
Drug Repositioning Analysis
Step 1: Obtain Preliminary Candidates Using a Transcriptome-Based Approach
In this step, we follow the same strategy as in Cmap (Lamb et al., 2006; Subramanian et al., 2017).
Step 1a. Calculate the Weighted Connectivity Score
First, we conducted a pattern-matching analysis (Lamb et al., 2006) to evaluate the reversal of gene expression patterns between HF DEGs and drug-induced gene signature. HF DEGs comes from one of the four kinds of HF gene expression patterns, that is top 150 DEGs, top 250 DEGs, top 350 DEGs, and articulation DEGs. Drug-induced gene signature refers to the gene expression profile of a certain cell type treated by drugs. For the HF DEGs, we compared the sorted upregulated and sorted downregulated DEGs, respectively, with drug-induced gene signatures sorted by z-scores of which upregulated genes are located at the top and downregulated genes are located at the bottom. For a given pair of HF DEGs and drug-induced gene signature, if upregulated HF DEGs enrich at the bottom of the sorted drug-induced gene signature and downregulated DEGs of HF enrich at the top of the sorted drug-induced gene signature, they are assumed to have the reversed gene expression patterns and thus have therapeutic potential. The Kolmogorov–Smirnov (KS) statistic presented in the original Cmap paper (Lamb et al., 2006) was used to quantitatively assess the reversal of gene expression patterns.
where KS is divided into KSup and KSdown referring to the enrichment score for the sorted upregulated and sorted downregulated genes in a list of HF DEGs, respectively. The sorted upregulated HF DEGs were compared with drug-induced gene signatures sorted by z-scores to get KSup, and the sorted downregulated HF DEGs were compared with drug-induced gene signatures sorted by z-scores to get KSdown. Let n be the total number of genes in an ordered drug-induced gene signature and t be the number of the up- or downregulated genes in a list of HF DEGs. V(j) is the position of gene j in the ordered drug-induced gene signature. Gene j is any gene in the sorted up- or downregulated gene list of HF DEGs, where j = 1, 2, …, t.
The weighted connectivity score was calculated based on the KS statistic as in the original Cmap paper (Lamb et al., 2006). For a given HF DEGs (r) and drug-induced gene signature (q), the weighted connectivity score Wq,r is calculated as follows:
where KSup is the enrichment of upregulated HF DEGs in the sorted drug-induced signature, and KSdown is the enrichment of downregulated HF DEGs in the sorted drug-induced signature. The sign of KS will be negative if the upregulated DEGs of HF enrich to the bottom of the drug-induced signature or the down-regulated HF DEGs enrich to the top of the drug-induced signature. Otherwise, the sign of KS will be positive. Zero is assigned for cases where KSup and KSdown are the same sign.
Step 1b. Calculate the Normalized Connectivity Score
Since the drugs’ perturbations are profiled in several cell lines, to each candidate, we calculated the weighted connectivity score for every pair of drug-induced gene signature and HF DEGs and then normalized the vector of weighted connectivity scores following the strategy of Cmap to allow for comparing across different cell types and different drug types (Subramanian et al., 2017). To a given vector of the weighted connectivity scores (W), we normalized W using the formula described previously (Subramanian et al., 2017) to obtain normalized connectivity scores (NCS):
where NCSc,t is the normalized connectivity score for a certain pair of cell type (c) and perturbation type (t), Wc,t is the weighted connectivity score, and μ+c,t and μ−c,t are the separately evaluated signed means of the positive and negative values of the weighted connectivity scores.
Step 1c. Calculate the Terminal Connectivity Score (τ)
While NCS can be used to make meaningful comparisons of the reversal between drug-induced signatures and disease-related gene sets, it is also useful to assess if the reversal between different combinations of drug-induced signature and disease-related gene set are significantly different. Tau (τ) (Subramanian et al., 2017) was used to compare the reversal of a certain pair of HF and drug with other pairs.τ was calculated as follows:
where NCSq,r is the normalized connectivity score for a certain pair of drug-induced signature (q) and disease-related gene set (r). NCSi,r is the normalized connectivity score for the disease-related gene set (r) and any other drug-induced signature (i). N is the total number of drug-induced signatures. τ ranges from -100 to 100, and a | τ | of 90 indicates that to a certain drug-induced signature, 10% of the total drug-induced signatures showed stronger connectivity with the disease-related gene set than the certain drug-induced signature. The negative sign of τ refers to reversed gene expression patterns of drug and disease, while a positive sign indicates nonreversed gene expression patterns.
As each drug was profiled using several cell lines in Cmap, to find drugs most likely having therapeutic potential to HF, we used the minimum τ (the most negative τ) of all the cell lines as the connectivity score. The τ of the four lists of HF DEGs (top 150 genes, top 250 genes, top 350 genes, and articulation DEGs) used as inputs were averaged as the final score. For each dataset, we chose the top 5% (38) drugs with the most negative τ values as the candidate drugs. We then combined the top 5% drugs of all datasets and chose the 38 most common drugs as the preliminary candidate drugs.
Step 2: Narrow Down Preliminary Candidates Applying a Network-Based Approach
First, we built a HF-related network. To obtain representative DEGs of HF to build the network, we obtained the DEGs of each dataset and chose the most common DEGs across the majority of all datasets (three or more) as the final DEGs. Using the final DEGs, we extracted the HF-related network from the global network established previously. Then, we analyzed the relationship between candidates’ targets and the HF-related network. There are generally three different types of relationship between drug targets and disease network: (1) drug targets have no interactions with the disease network, (2) drug targets do not belong to the disease network but can interact with the nodes, and (3) drug targets are contained in the disease network. In our study, preliminary candidate drugs were selected as final candidate drugs, only if some of their targets are located in the HF-related network.
Drug-Set Enrichment Analysis of Known HF Drugs
To assess the performance of our drug repositioning method, we performed a drug-set enrichment analysis as previously described (So et al., 2017) to determine if known HF drugs are ranked significantly higher than they would have been by chance in the whole drug list ranked by τ. We performed the enrichment analysis for each dataset separately. First, we ranked drug list of each HF dataset by τ. Then, we did rank-sum test to compare the difference of ranks of drugs being used in clinical practice with the ranks of the rest drugs. Finally, we aggregated the results across all datasets using meta-analysis. We applied three different methods to summarize the results: the inverse-variance method, Fisher’s method, and Tippett’s minimum P method (So et al., 2017).
Manual Curation of the Final Candidate Drugs
To assess the results obtained from our method, we also performed a literature search with predefined search strategies to locate evidence of the therapeutic potential of candidate drugs for HF. Details regarding the query are as follows: DCM, Drug_name AND (DCM OR heart failure OR dilated cardiomyopathy OR dilated cardiomyopathies); ISCM, Drug_name AND (ISCM OR heart failure OR ischemic cardiomyopathy OR ischemic cardiomyopathy).
Investigating the Mode of Action Network of Candidate Drugs
In order to discover the potential MOA of candidate drugs, we built a MOA network for each candidate using a systems biology approach. The foundation of the MOA network is a previously described drug-disease network (DDN) (Peyvandipour et al., 2018) that considers the interactions between known drug targets and known HF-related genes. The construction of this MOA network was based on the HF-related network constructed in step 2 of our repositioning approach. Known HF-related genes extracted from Comparative Toxicogenomics Database were used to form the HF-related gene set as Sdisease = (x1…xn). Then, given the set of targets of a certain drug that were involved in the disease network as Sdrug = (y1…ym), we extracted DDN from the HF-related network as the MOA network of the drug. The DDN consists of all of the shortest paths in which a gene from either Sdisease or Sdrug can be a source or destination. To ensure the authenticity of the interaction between known drug targets and known HF-related genes, the steps from source to destination were limited to less than three.
Discovering Potential MOAs Using KEGG Pathway Analysis
We used genes involved in each MOA network as an input to perform Kyoto Encyclopedia of Genes and Genomes (KEGG) pathway analysis to uncover potential pathways through which the candidate may perform its therapeutic efficiency to HF. The KEGG pathway analysis was performed using Enrichr (Chen et al., 2013), an integrative gene set enrichment tool used to perform pathway analysis. For each input gene list, the tool calculates an enrichment score based on a modified Fisher’s exact test. The adjusted p value cutoff was set to 0.05 for the identification of significant pathways. The significant pathways of each drug were ranked according to combined scores, and the HF-related pathways within the top 10 ranked pathways were selected as potential MOAs.
Results
Preliminary Candidates for HF
HF Arising From DCM
Table 1 presents the selected top 5% (38) candidates for DCM. As expected, our analyses identified drugs used to treat HF in clinical practice, such as perindopril, telmisartan, and amiloride.
Besides, a total of 7 drugs from the list of 38 candidates had literature evidence supporting their use to treat HF. Animal studies have shown that estradiol treatment can prevent the development of HF (Beer et al., 2007; Satoh et al., 2007). Moreover, estradiol can also prevent cardiomyocyte apoptosis (Patten et al., 2004) and cardiac fibrosis (Pedram et al., 2010), which are involved in the pathological processes of HF. Chlorpromazine is a prototypical phenothiazine antipsychotic drug, and previous studies have shown that intravenous administration of chlorpromazine had hemodynamic effects and was of benefit to patients with HF (Elkayam et al., 1977; Mifune et al., 1979). Nicardipine is a calcium channel blockader exhibiting antihypertensive properties and is effective in the treatment of coronary spasms and angina. Studies showed that an acute intravenous drip infusion of nicardipine is effective in the treatment of HF (Fifer et al., 1990; Sy et al., 1993; Hirota et al., 1997). Naloxone is an opioid antagonist medication used to block the effects of opioid drugs. Animal experiments have shown that naloxone improves the systemic hemodynamics and myocardial contractile function in conscious dogs with HF (Himura et al., 1994). Atorvastatin and pravastatin are members of the drug class known as stains used to lower cholesterol. It has been suggested that the use of atorvastatin or pravastatin in HF patients attenuates adverse left ventricular remodeling and improves left ventricular systolic function as well as clinical outcomes (Sola et al., 2006; Wojnicz et al., 2006; Han et al., 2007; Lipinski et al., 2009; Correale et al., 2013). Bromocriptine is a prolactin release inhibitor. Bromocriptine treatment was reported to improve hemodynamic profiles in HF patients and was associated with a high rate of full left ventricular recovery and low morbidity and mortality in HF patients arising from peripartum cardiomyopathy (Francis et al., 1983; Hilfiker-Kleiner et al., 2017).
HF Arising From ISCM
Table 2 presents the selected top 5% (38) of candidate drugs for ISCM. Amiloride was among the candidates. We also determined that 5 out of the 38 candidate drugs have been reported as potentially being useful in HF treatment. Estradiol, atorvastatin, and naloxone, which are candidate drugs of DCM, also appeared in the preliminary candidate drug list of ISCM. Preclinical and clinical studies have indicated that thalidomide, which displays immunosuppressive and antiangiogenic activity, has potential efficacy for HF patients (Agoston et al., 2002; Gullestad et al., 2002; Gullestad et al., 2005; Orea-Tejeda et al., 2007). Furthermore, studies have indicated that nitrendipine, a calcium channel blocker with marked vasodilator action, can favorably alter performance of the failing left ventricles in HF patients (Cohn, 1986).
Refined List of Candidate Drugs
For DCM, 13 drugs remained after the second round of selection (Table 3). Perindopril and telmisartan were retained. In addition, five out of the aforementioned seven preliminary candidate drugs having been reported in the literature as having potential efficacy for HF treatment were also retained. For ISCM, 23 drugs remained following the second round of selection (Table 4). Amiloride and four out of five drugs supported by the literature in having potential therapeutic efficacy for HF were retained.
Enrichment Analysis of Known HF Drugs
We extracted all drugs (21) being demonstrated as improving the prognosis of HF patients from the 762 drugs to perform the enrichment analysis. The 21 drugs include 9 angiotensin-converting enzyme inhibitors, 7 angiotensin II-receptor blockers, 2 aldosterone receptor antagonists, and 3 beta-blockers, metoprolol, bisoprolol, and carvedilol.
Although Fisher’s methods and Tippett’s minimum P method only showed significant enrichment for DCM (Table 5), the inverse-variance method showed that the drug set was enriched for both DCM and ISCM, with significant P values of 0.0002 and 0.03, respectively (Figure 2). The forest plot also showed that the mean rank of the 21 drugs is smaller than that of the remaining 741 drugs in each data set without heterogeneity, indicating a robustness of the trend toward enrichment.
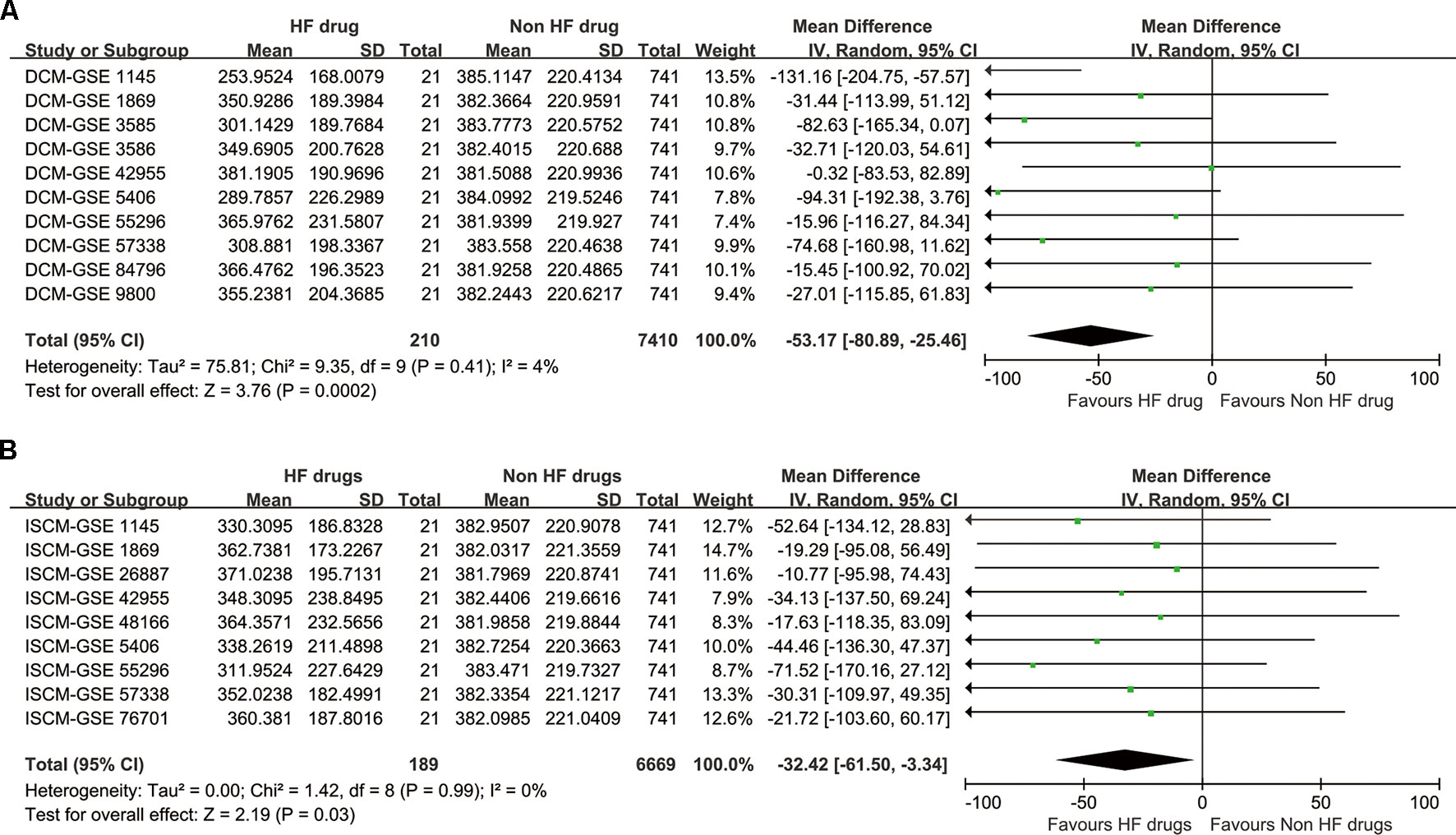
Figure 2 The synthesized result of the enrichment analysis. (A) For dilated cardiomyopathy (DCM). (B) For ischemic cardiomyopathy (ISCM).
Potential MOA of Candidates
The MOA networks displaying the relationship between drug targets and HF-related genes are shown in Figures 3 and 4. The significant pathways related to each MOA network are presented in Supplemental Tables 3 and 4.
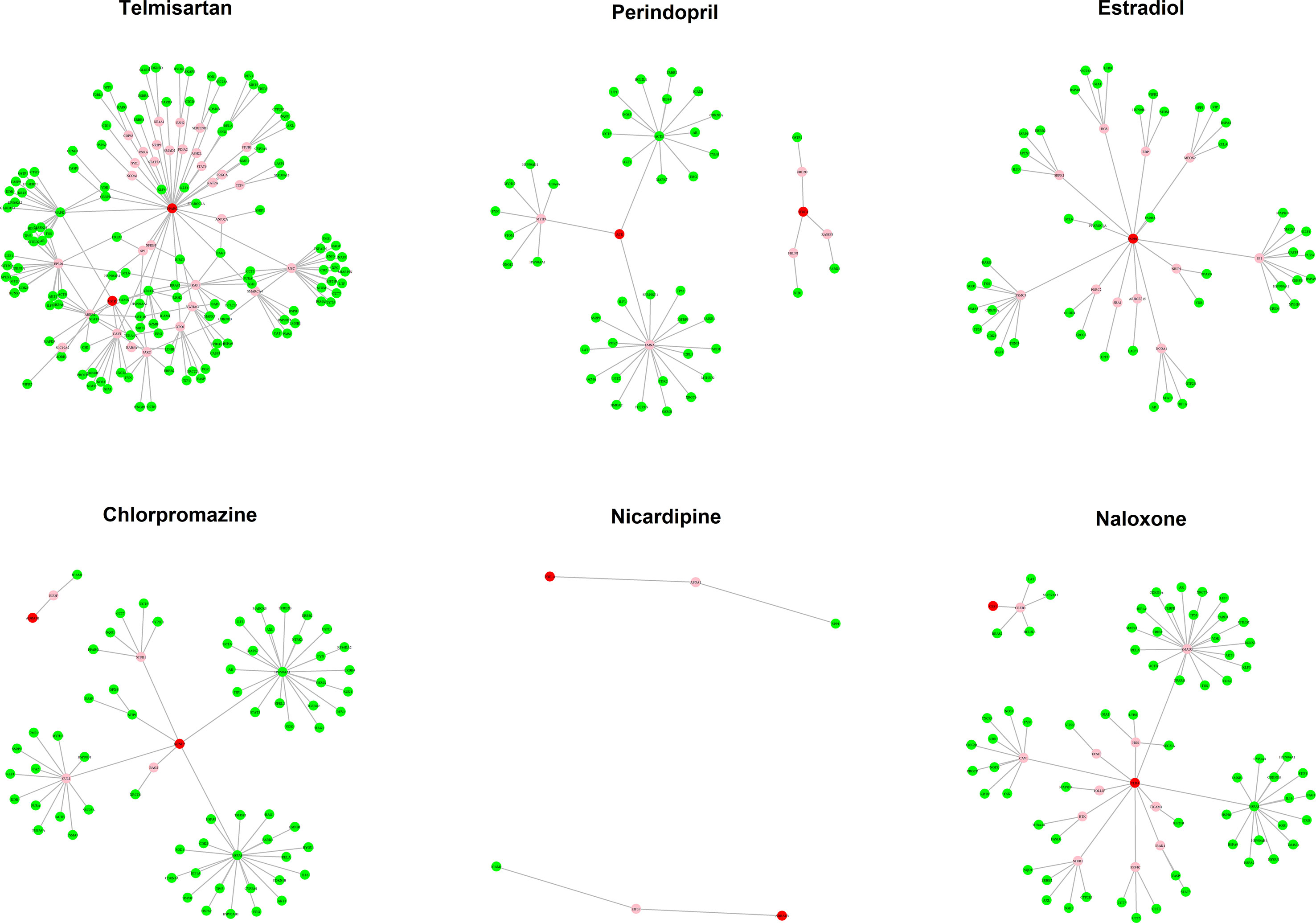
Figure 3 The mode of action (MOA) network of dilated cardiomyopathy (DCM) candidates. Green nodes present known heart failure (HF)-related genes. Red nodes represent drug targets. Pink nodes represent genes with no evidence of corresponding to HF.
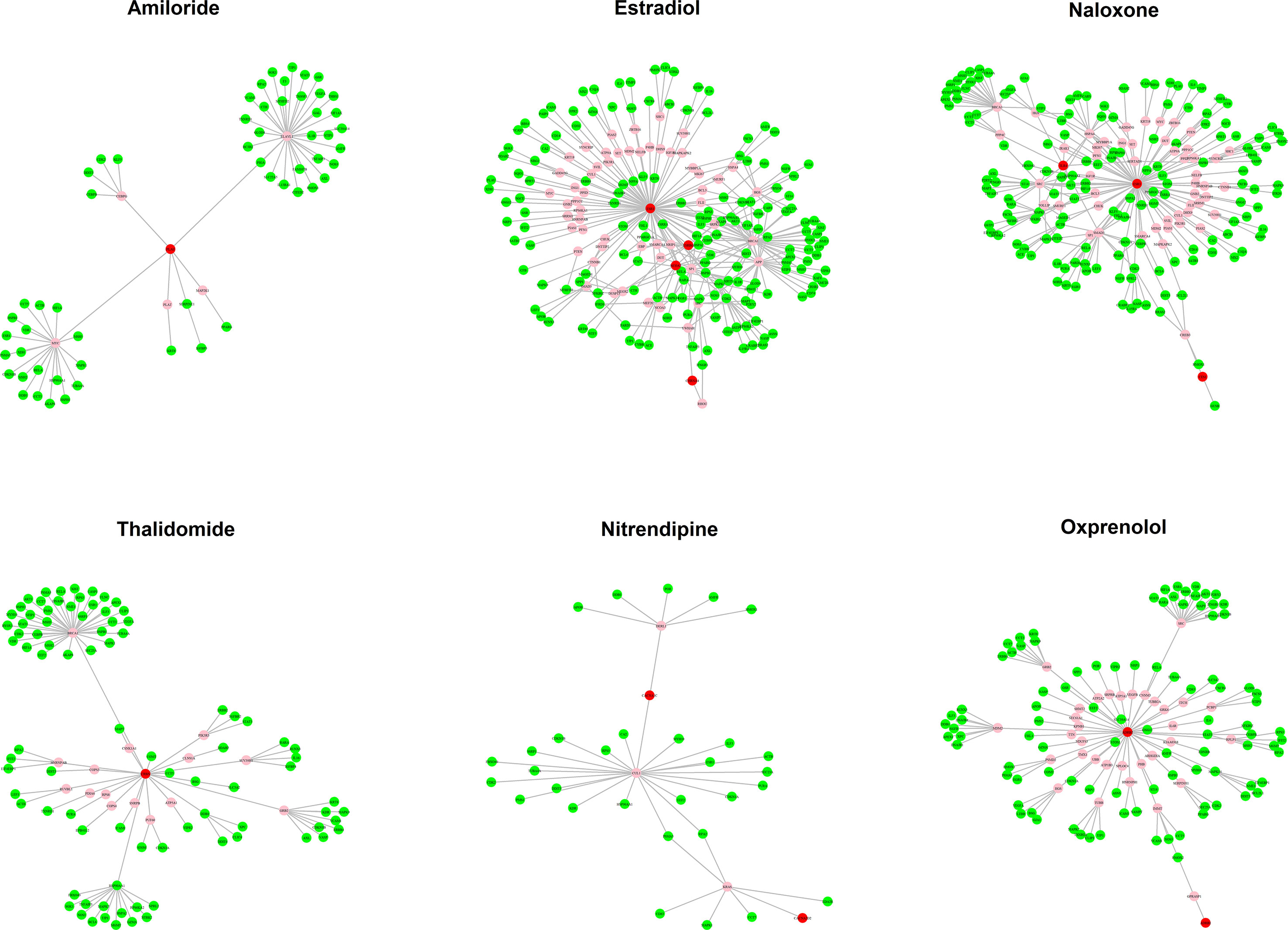
Figure 4 The mode of action (MOA) network of ischemic cardiomyopathy (ISCM) candidates. Green nodes present known heart failure (HF)-related genes. Red nodes represent drug targets. Pink nodes represent genes with no evidence of corresponding to HF.
Potential MOA of Candidates for DCM-Induced HF
Estradiol, chlorpromazine, nicardipine, naloxone, and bromocriptine were candidates with literature support regarding their potential efficacy for HF treatment. The enriched pathways using genes from their MOA network are described in the following section.
There are 59 genes contained in the MOA network of estradiol. Using these 59 genes as input for KEGG pathway analysis, the estrogen signaling pathway and hypoxia-inducible factor 1 (HIF-1) signaling pathway were deemed as significant. Notably, estrogen regulates a plethora of physiological processes in mammals through the estrogen signaling pathway, including prevention of the development of HF, cardiomyocyte apoptosis, and cardiac fibrosis (Patten et al., 2004; Beer et al., 2007; Satoh et al., 2007; Pedram et al., 2010). HIF-1 is a transcription factor functioning as a major regulator of oxygen homeostasis. One previous study investigated left ventricular tissues from HF patients and determined that the HIF pathway persists during HF (Zolk et al., 2008).
There are 72 genes in the MOA network of chlorpromazine. The significant pathways related to chlorpromazine include the phosphoinositide-3-kinase (PI3K)-Akt signaling pathway, the HIF-1 signaling pathway, and the mitogen-activated protein kinase (MAPK) signaling pathway. The PI3K-Akt signaling pathway regulates fundamental cellular functions such as transcription, translation, proliferation, growth, and survival. One study indicated that the PI3K-Akt signaling pathway is involved in the myocardial remodeling in HF patients and plays an important role in the pathogenesis of myocardial hypertrophy (Yang et al., 2005). The MAPK signaling pathway is a highly conserved module that is involved in the majority of cellular functions, including cell proliferation, differentiation, and migration. Previous studies have shown that downregulation of the MAPK signaling pathway has a cardioprotective effect against HF (Li et al., 2018).
The MOA network of nicardipine consists of six genes. The calcium signaling pathway is found to be related to the MOA network of nicardipine. Ca2+ plays an important role in connecting the excitability of cell membrane with contraction in myocardium. Previous reports have shown that Ca2+ homeostasis is defective in HF and represents a central cause of contractile dysfunction in failure myocardium (Luo and Anderson, 2013), suggesting that the calcium signaling pathway may be translated into novel therapies for HF.
There are 80 genes contained in the MOA network of naloxone. The PI3K-Akt signaling pathway and HIF-1 signaling pathway are significantly related to the MOA network of naloxone, which are both involved in the pathogenesis of HF (Yang et al., 2005; Zolk et al., 2008).
Although the MOA network of bromocriptine contains only three genes, the tumor necrosis factor (TNF) signaling pathway and nuclear factor kappa B (NF-kappa B) signaling pathway are deemed as significant. TNF can induce a wide range of intracellular signal pathways including apoptosis and cell survival as well as inflammation and immunity. Previous studies indicated that the expression of TNF receptors is upregulated in mononuclear leukocytes of HF patients (Nozaki et al., 1998). Besides, increased levels of TNF-alpha were observed in HF patients, which were high enough to reduce cardiac contractility in vitro, and the mortality of HF patients increases with higher levels of TNF-alpha (Muller-Ehmsen and Schwinger, 2004). For these reasons, TNF signaling pathway may appear to be a potential target for the treatment of HF. NF-kappa B is the generic name of the relevant transcription factor family. The NF-kappa B signaling pathway regulates genes involved in immunity, inflammation, and cell survival. The polymorphism of NF-kappa B is associated with the heart function of HF patients (Santos et al., 2010). Moreover, silencing the NF-kappa B signaling pathway can prevent cardiac hypertrophy and HF in mice HF models (Gupta et al., 2008).
Perindopril and telmisartan are currently being used in clinical practice to treat HF by targeting and inhibiting the renal–angiotensin–aldosterone system (RAAS). The significant HF-related pathways related to them include apoptosis, the PI3K-Akt signaling pathway, the HIF-1 signaling pathway, and the MAPK signaling pathway. Previous studies have revealed that cardiocyte apoptosis exists in the hearts of HF patients (Narula et al., 1996) and that the attenuation of apoptosis can delay cardiac dysfunction in murine HF models (Mandl et al., 2011). Moreover, high RAAS activity can result in apoptosis, while inhibiting RAAS can prevent apoptosis (Velez Rueda et al., 2012; Kooptiwut et al., 2015). Based on the literature, downregulating the MAPK signaling pathway can have cardioprotective effects against HF (Li et al., 2018), while inhibiting RAAS can abrogate MAPK activation (Lebeche et al., 2001).
Apart from the aforementioned drugs, other candidates were neither used in clinical practice nor did they exhibit any therapeutic efficacy to HF supported by the literature. However, there are at least two pathways corresponding to HF for each drug (results not shown), suggesting that these drugs may have potential therapeutic efficacy for HF treatment.
Potential MOA of Candidates for ISCM-Induced HF
Five candidate drugs of ISCM are used in clinical practice to treat HF or have literature support with known treatment efficacy for HF, including estradiol, naloxone, thalidomide, nitrendipine, and amiloride. Estradiol and naloxone are also DCM candidates, despite their MOA networks for DCM and ISCM being different. The MOA networks of estradiol and naloxone consist of 218 and 206 genes, respectively. The pathways that deemed as significant to them are the same, including the HIF-1 signaling pathway, MAPK signaling pathway, and PI3K-Akt signaling pathway. A total of 103 genes are involved in the MOA network of Thalidomide, and the HIF-1 signaling pathway and PI3K-Akt signaling pathway are related to this network. The MOA network of nitrendipine contains 35 genes, and the significant pathway of these genes is the PI3K-Akt signaling pathway. Amiloride, which is used as adjunctive treatment with diuretics in HF, was also contained in the final candidates list. We input the 63 genes of the MOA network of amiloride to perform the KEGG pathway analysis and determined that the HIF-1 signaling pathway, PI3K-Akt signaling pathway, and TNF signaling pathway were deemed significant, which suggests that amiloride may play other roles in the treatment of HF apart from diuretic activity. For the remaining drugs, at least two pathways corresponded to HF (results not shown), suggesting a potential therapeutic efficacy for HF prevention.
Discussion
In the present work, we presented a two-step drug repositioning pipeline and applied our methodology to HF arising from two different etiologies to identify candidate drugs. Drug-set enrichment analysis and literature search provided support to the validity of our repositioning approach. MOA network and KEGG analysis displayed the potential mechanisms of drug efficacy.
Concerning the repositioning results, we observed that the cardiovascular drugs have the largest proportion among the candidates in both DCM- and ISCM-induced HF, indicating that our proposed method could specifically focus the repositioning on disease-related tissues. For DCM, the probability of finding candidates supported by literature rise from 26.3% (10 out of 38) to 53.8% (7 out of 13) after narrowing down the preliminary candidates, and this number rise from 15.8% (6 out of 38) to 21.7% (5 out of 23) for ISCM, which indicates that our two-step drug repositioning approach can effectively increase the likelihood of finding candidates with actual therapeutic potential for HF.
It is encouraging to know that our repositioning results are supported by drug-set enrichment analysis. Our results suggest that gene expression profiling data contains useful information for drug discovery. We observed that the inverse-variance method indicates that the drug set is enriched for both DCM and ISCM, while Fisher’s method and Tippett’s minimum P method only indicate significant enrichment for DCM. There are a few possible explanations for this result. First, the sample size of drugs used to do enrichment analysis may not be large enough. The number of drugs used to perform enrichment analysis is 21, which is much smaller than the total number of drugs used for drug repositioning (762). Second, HF is known to be heterogeneous with several subtypes, which may lead to different responses to the same therapeutic interventions (McMurray et al., 2012; Ahmad et al., 2014; Ahmad et al., 2018). Some studies have reported that ISCM-induced HF patients have a worse prognosis than DCM-induced HF patients (Follath et al., 1998; Follath, 2000), suggesting that the response to treatment in DCM-induced patients is better than that of ISCM-induced patients.
To investigate the MOA for the candidate drugs, we take advantage of existing information on known HF-related genes, known drug targets, and known protein–protein interaction information to obtain the MOA network for each drug. The MOA network displays the direct interactions between drugs and diseases in a form of network through its target genes. In the MOA network, we can identify genes that have not been previously reported as HF-related but have direct interactions with known HF-related genes as well as known drug targets, suggesting that these genes may represent potential biomarkers or therapeutic targets for HF. We also observe that, although some drugs appear in the candidates list of both DCM-induced and ISCM-induced HF, the MOA network can be different, which may indicate the differences of pathogenesis in HF arising from different etiologies. For candidates which are neither used in clinical practice nor supported by previous studies, HF-related pathways can be enriched using the genes contained in their MOA networks, indicating their potential therapeutic efficacy for HF.
There are a number of advantages to our study. First, we extracted all HF-related gene expression datasets with a minimum sample size of 10 from GEO and synthesized the results of each dataset to obtain a more robust and reliable results to compensate for the limitation that most human genomic studies of HF are limited by insufficient cardiac tissues. Second, the first step of our repositioning approach is a largely hypothesis-free approach blinding to any knowledge regarding existing HF drugs, known drug targets, or known drug–disease relationships, and thus is likely to find candidate drugs of different mechanisms from the known treatment. Third, compared to the Cmap methods used as the first step of our repositioning approach, the second step of our repositioning approach can effectively increase the likelihood of identifying candidates with actual therapeutic potential for HF and can be used in conjunction with any other drug repurposing methods to effectively narrow down a list of candidate drugs. In the second step, we determined the interaction between known drug targets and HF networks to narrow down the preliminary candidates. Nearly all preliminary candidate drugs being used in clinical practice or with literature support were included in the final candidates list. Fourth, our method of constructing MOA networks can be applied to any drug repositioning studies aimed at exploring the underlying mechanisms of action of candidate drugs. Fifth, to our knowledge, this is the first systematic drug repositioning analysis conducted in relation to HF. In the face of the growing epidemiological burden of HF, our study might be of particular use in the discovery of novel effective pharmacological therapies for HF.
The present study also has a few general limitations. First, although the pattern matching method used in the first step is largely hypothesis free, the assumptions of reversed expression patterns may not be completely true for every disease–drug pair. Second, in the second step of our repositioning approach, to ensure that drugs have the greatest likelihood to interact with HF, we only chose drugs whose targets are located in the HF network as the final candidates. Owing to this selection criterion, some candidates may be missed. Moreover, the drug-induced gene expression profiles in Cmap are not measured in cardiac tissues and may represent another limitation, although the Cmap study claimed that these data can be reasonably modeled in non-neoplastic diseases (Lamb et al., 2006). In addition, our repositioning analysis is only based on gene expression information. Considering that the gene expression profile only partly reflects the mechanisms of diseases and drugs, our method may be improved by incorporating other information related to genomics, transcriptomics, proteomics, and metabolomics. Finally, although we performed drug set enrichment analysis and literature search to validate our repositioning results and obtained promising results, the current study does not provide confirmatory evidence for the repositioning candidates, and thus, adequately sized preclinical and clinical studies will be necessary to verify the repositioning predictions.
In conclusion, we have proposed a framework for drug repositioning and presented a list of repositioning candidates for HF. To our knowledge, this is the first systematic drug repositioning analysis to HF, and we believe that it could be of particular use in the discovery of novel effective pharmacological therapies for HF.
Data Availability
Publicly available datasets were analyzed in this study. This data can be found here: GSE57338, GSE5406, GSE1145, GSE55296, GSE3586, GSE3585, GSE84796, GSE42955, GSE1869, GSE9800, GSE76701, GSE48166, GSE26887, GSE92742, https://www.drugbank.ca/releases/latest, https://downloads.thebiogrid.org/BioGRID, http://ctdbase.org/downloads/#gd.
Author Contributions
GY, AM, and ZSQ conceived and designed the study. GY performed data analysis. GY, and ZSQ interpreted the data. GY and ZSQ wrote the manuscript. All authors approved the final version of the manuscript.
Conflict of Interest Statement
The authors declare that the research was conducted in the absence of any commercial or financial relationships that could be construed as a potential conflict of interest.
Supplementary Material
The Supplementary Material for this article can be found online at: https://www.frontiersin.org/articles/10.3389/fgene.2019.00916/full#supplementary-material
References
Agoston, I., Dibbs, Z. I., Wang, F., Muller, G., Zeldis, J. B., Mann, D. L., et al. (2002). Preclinical and clinical assessment of the safety and potential efficacy of thalidomide in heart failure. J. Card Fail. 8 (5), 306–314. doi: 10.1054/jcaf.2002.128684
Ahmad, T., Lund, L. H., Rao, P., Ghosh, R., Warier, P., Vaccaro, B., et al. (2018). Machine learning methods improve prognostication, identify clinically distinct phenotypes, and detect heterogeneity in response to therapy in a large cohort of heart failure patients. J. Am. Heart Assoc. 7 (8), 1–14. doi: 10.1161/JAHA.117.008081
Ahmad, T., Pencina, M. J., Schulte, P. J., O’Brien, E., Whellan, D. J., Pina, I. L., et al. (2014). Clinical implications of chronic heart failure phenotypes defined by cluster analysis. J Am Coll Cardiol 64 (17), 1765–1774. doi: 10.1016/j.jacc.2014.07.979
Anighoro, A., Stumpfe, D., Heikamp, K., Beebe, K., Neckers, L. M., Bajorath, J., et al. (2015). Computational polypharmacology analysis of the heat shock protein 90 interactome. J. Chem. Inf. Model. 55 (3), 676–686. doi: 10.1021/ci5006959
Askoxylakis, V., Thieke, C., Pleger, S. T., Most, P., Tanner, J., Lindel, K., et al. (2010). Long-term survival of cancer patients compared to heart failure and stroke: a systematic review. BMC Cancer 10, 105. doi: 10.1186/1471-2407-10-105
Beer, S., Reincke, M., Kral, M., Callies, F., Stromer, H., Dienesch, C., et al. (2007). High-dose 17beta-estradiol treatment prevents development of heart failure post-myocardial infarction in the rat. Basic Res. Cardiol. 102 (1), 9–18. doi: 10.1007/s00395-006-0608-1
Bowman, G. R., Bolin, E. R., Hart, K. M., Maguire, B. C., Marqusee, S. (2015). Discovery of multiple hidden allosteric sites by combining Markov state models and experiments. Proc. Natl. Acad. Sci. U.S.A. 112 (9), 2734–2739. doi: 10.1073/pnas.1417811112
Chang, M., Smith, S., Thorpe, A., Barratt, M. J., Karim, F. (2010). Evaluation of phenoxybenzamine in the CFA model of pain following gene expression studies and connectivity mapping. Mol. Pain 6, 56. doi: 10.1186/1744-8069-6-56
Chatr-Aryamontri, A., Oughtred, R., Boucher, L., Rust, J., Chang, C., Kolas, N. K., et al. (2017). The BioGRID interaction database: 2017 update. Nucleic Acids Res. 45 (D1), D369–D379. doi: 10.1093/nar/gkw1102
Chen, E. Y., Tan, C. M., Kou, Y., Duan, Q., Wang, Z., Meirelles, G. V., et al. (2013). Enrichr: interactive and collaborative HTML5 gene list enrichment analysis tool. BMC Bioinformatics 14, 128. doi: 10.1186/1471-2105-14-128
Cohn, J. N. (1986). Calcium antagonists and left ventricular function: effects of nitrendipine in congestive heart failure. Am. J. Cardiol. 58 (8), 27D–30D. doi: 10.1016/0002-9149(86)90421-2
Correale, M., Totaro, A., Passero, T., Abruzzese, S., Musaico, F., Ferraretti, A., et al. (2013). Treatment with atorvastatin is associated with a better prognosis in chronic heart failure with systolic dysfunction: results from The Daunia Heart Failure Registry. Neth. Heart J. 21 (9), 408–416. doi: 10.1007/s12471-013-0430-y
Defranchi, E., Schalon, C., Messa, M., Onofri, F., Benfenati, F., Rognan, D. (2010). Binding of protein kinase inhibitors to synapsin I inferred from pair-wise binding site similarity measurements. PLoS One 5 (8), e12214. doi: 10.1371/journal.pone.0012214
Ehrt, C., Brinkjost, T., Koch, O. (2016). Impact of binding site comparisons on medicinal chemistry and rational molecular design. J. Med. Chem. 59 (9), 4121–4151. doi: 10.1021/acs.jmedchem.6b00078
Elkayam, U., Rotmensch, H. H., Terdiman, R., Geller, E., Laniado, S. (1977). Hemodynamic effects of chlorpromazine in patients with acute myocardial infarction and pump failure. Chest 72 (5), 623–627. doi: 10.1378/chest.72.5.623
Fifer, M. A., Aroney, C. N., Semigran, M. J., Herrmann, H. C., Dec, G. W., Boucher, C. A. (1990). Techniques for assessing inotropic effects of drugs in patients with heart failure: application to the evaluation of nicardipine. Am. Heart J. 119 (2 Pt 2), 451–456. doi: 10.1016/S0002-8703(05)80068-7
Follath, F. (2000). [Ischemic vs nonischemic heart failure—does etiology matter?]. Ther. Umsch. 57 (5), 321–323. doi: 10.1024/0040-5930.57.5.321
Follath, F., Cleland, J. G., Klein, W., Murphy, R. (1998). Etiology and response to drug treatment in heart failure. J. Am. Coll. Cardiol. 32 (5), 1167–1172. doi: 10.1016/S0735-1097(98)00400-8
Francis, G. S., Parks, R., Cohn, J. N. (1983). The effects of bromocriptine in patients with congestive heart failure. Am. Heart J. 106 (1 Pt 1), 100–106. doi: 10.1016/0002-8703(83)90446-5
Gullestad, L., Semb, A. G., Holt, E., Skardal, R., Ueland, T., Yndestad, A., et al. (2002). Effect of thalidomide in patients with chronic heart failure. Am. Heart J. 144 (5), 847–850. doi: 10.1067/mhj.2002.125628
Gullestad, L., Ueland, T., Fjeld, J. G., Holt, E., Gundersen, T., Breivik, K., et al. (2005). Effect of thalidomide on cardiac remodeling in chronic heart failure: results of a double-blind, placebo-controlled study. Circulation 112 (22), 3408–3414. doi: 10.1161/CIRCULATIONAHA.105.564971
Gupta, S., Young, D., Maitra, R. K., Gupta, A., Popovic, Z. B., Yong, S. L., et al. (2008). Prevention of cardiac hypertrophy and heart failure by silencing of NF-kappaB. J. Mol. Biol. 375 (3), 637–649. doi: 10.1016/j.jmb.2007.10.006
Hall, D. R., Kozakov, D., Whitty, A., Vajda, S. (2015). Lessons from hot spot analysis for fragment-based drug discovery. Trends Pharmacol. Sci. 36 (11), 724–736. doi: 10.1016/j.tips.2015.08.003
Han, Z. H., Wu, X. S., Zhang, X. X., Hu, R., Zhao, H., Wang, C. M., et al. (2007). [The primary observation on the effect of pravastatin to non-ischemic heart failure]. Zhonghua Xin Xue Guan Bing Za Zhi 35 (7), 603–606. doi: 10.3760/j.issn:0253-3758.2007.07.004
Hilfiker-Kleiner, D., Haghikia, A., Berliner, D., Vogel-Claussen, J., Schwab, J., Franke, A., et al. (2017). Bromocriptine for the treatment of peripartum cardiomyopathy: a multicentre randomized study. Eur. Heart J. 38 (35), 2671–2679. doi: 10.1093/eurheartj/ehx355
Himura, Y., Liang, C. S., Imai, N., Delehanty, J. M., Woolf, P. D., Hood, W. B. Jr. (1994). Short-term effects of naloxone on hemodynamics and baroreflex function in conscious dogs with pacing-induced congestive heart failure. J. Am. Coll. Cardiol. 23 (1), 194–200. doi: 10.1016/0735-1097(94)90520-7
Hirota, Y., Kawai, C., Hori, R., Okumura, K., Kinoshita, M., Kumada, T., et al. (1997). Determining the optimum dose for the intravenous administration of nicardipine in the treatment of acute heart failure—a multicenter study. The nicardipine heart failure study group. Jpn. Circ. J. 61 (5), 367–374. doi: 10.1253/jcj.61.367
Hu, G., Agarwal, P. (2009). Human disease-drug network based on genomic expression profiles. PLoS One 4 (8), e6536. doi: 10.1371/journal.pone.0006536
Iskar, M., Zeller, G., Blattmann, P., Campillos, M., Kuhn, M., Kaminska, K. H., et al. (2013). Characterization of drug-induced transcriptional modules: towards drug repositioning and functional understanding. Mol. Syst. Biol. 9, 662. doi: 10.1038/msb.2013.20
Iwata, H., Sawada, R., Mizutani, S., Yamanishi, Y. (2015). Systematic drug repositioning for a wide range of diseases with integrative analyses of phenotypic and molecular data. J. Chem. Inf. Model. 55 (2), 446–459. doi: 10.1021/ci500670q
Jalencas, X., Mestres, J. (2013).Identification of similar binding sites to detect distant polypharmacology. Mol. Inform. 32 (11–12), 976–990. doi: 10.1002/minf.201300082
Jin, G., Fu, C., Zhao, H., Cui, K., Chang, J., Wong, S. T. (2012). A novel method of transcriptional response analysis to facilitate drug repositioning for cancer therapy. Cancer Res. 72 (1), 33–44. doi: 10.1158/0008-5472.CAN-11-2333
Keiser, M. J., Setola, V., Irwin, J. J., Laggner, C., Abbas, A. I., Hufeisen, S. J., et al. (2009). Predicting new molecular targets for known drugs. Nature 462 (7270), 175–181. doi: 10.1038/nature08506
Kinnings, S. L., Liu, N., Buchmeier, N., Tonge, P. J., Xie, L., Bourne, P. E. (2009). Drug discovery using chemical systems biology: repositioning the safe medicine Comtan to treat multi-drug and extensively drug resistant tuberculosis. PLoS Comput. Biol. 5 (7), e1000423. doi: 10.1371/journal.pcbi.1000423
Kooptiwut, S., Hanchang, W., Semprasert, N., Junking, M., Limjindaporn, T., Yenchitsomanus, P. T. (2015). Testosterone reduces AGTR1 expression to prevent beta-cell and islet apoptosis from glucotoxicity. J. Endocrinol. 224 (3), 215–224. doi: 10.1530/JOE-14-0397
Lamb, J., Crawford, E. D., Peck, D., Modell, J. W., Blat, I. C., Wrobel, M. J., et al. (2006). The connectivity map: using gene-expression signatures to connect small molecules, genes, and disease. Science 313 (5795), 1929–1935. doi: 10.1126/science.1132939
Lebeche, D., Zhao Bin, K., Hajjar, R. (2001). Candesartan abrogates G protein-coupled receptors agonist-induced MAPK activation and cardiac myocyte hypertrophy. J. Renin. Angiotensin. Aldosterone Syst. 2 (1_suppl), S154–S161. doi: 10.1177/14703203010020012701
Lewis, K. S., Butler, J., Bauersachs, J., Sandner, P. (2017). The three-decade long journey in heart failure drug development. Handb. Exp. Pharmacol. 243, 1–14. doi: 10.1007/164_2016_101
Li, L., Hao, J., Jiang, X., Li, P., Sen, H. (2018). Cardioprotective effects of ulinastatin against isoproterenol-induced chronic heart failure through the PI3KAkt, p38 MAPK and NF-kappaB pathways. Mol. Med. Rep. 17 (1), 1354–1360. doi: 10.3892/mmr.2017.7934
Lipinski, M. J., Cauthen, C. A., Biondi-Zoccai, G. G., Abbate, A., Vrtovec, B., Khan, B. V., et al. (2009). Meta-analysis of randomized controlled trials of statins versus placebo in patients with heart failure. Am. J. Cardiol. 104 (12), 1708–1716. doi: 10.1016/j.amjcard.2009.07.055
Liu, X., Ouyang, S., Yu, B., Liu, Y., Huang, K., Gong, J., et al. (2010). PharmMapper server: a web server for potential drug target identification using pharmacophore mapping approach. Nucleic Acids Res. 38 (Web Server issue), W609–W614. doi: 10.1093/nar/gkq300
Luo, M., Anderson, M. E. (2013). Mechanisms of altered Ca(2)(+) handling in heart failure. Circ Res 113 (6), 690–708. doi: 10.1161/CIRCRESAHA.113.301651
Mandl, A., Huong Pham, L., Toth, K., Zambetti, G., Erhardt, P. (2011). Puma deletion delays cardiac dysfunction in murine heart failure models through attenuation of apoptosis. Circulation 124 (1), 31–39. doi: 10.1161/CIRCULATIONAHA.110.988303
Manning, G., Whyte, D. B., Martinez, R., Hunter, T., Sudarsanam, S. (2002). The protein kinase complement of the human genome. Science 298 (5600), 1912–1934. doi: 10.1126/science.1075762
March-Vila, E., Pinzi, L., Sturm, N., Tinivella, A., Engkvist, O., Chen, H., et al. (2017). On the integration of in silico drug design methods for drug repurposing. Front. Pharmacol. 8, 298. doi: 10.3389/fphar.2017.00298
Mattingly, C. J., Rosenstein, M. C., Colby, G. T., Forrest, J. N. Jr., and Boyer, J. L. (2006). The Comparative Toxicogenomics Database (CTD): a resource for comparative toxicological studies. J. Exp. Zool. A Comp. Exp. Biol. 305 (9), 689–692. doi: 10.1002/jez.a.307
McMurray, J. J., Adamopoulos, S., Anker, S. D., Auricchio, A., Bohm, M., Dickstein, K., et al. (2012). ESC Guidelines for the diagnosis and treatment of acute and chronic heart failure 2012: the task force for the diagnosis and treatment of acute and chronic heart failure 2012 of the european society of cardiology. Developed in collaboration with the heart failure association (HFA) of the ESC. Eur. Heart J. 33 (14), 1787–1847. doi: 10.1093/eurheartj/ehs104
Mervin, L. H., Afzal, A. M., Drakakis, G., Lewis, R., Engkvist, O., Bender, A. (2015). Target prediction utilising negative bioactivity data covering large chemical space. J. Cheminform. 7, 51. doi: 10.1186/s13321-015-0098-y
Mifune, J., Kuramoto, K., Ueda, K., Matsushita, S., Kuwajima, I., Sakai, M., et al. (1979). Treatment of acute left-sided heart failure with intramuscular injection of chlorpromazine. Jpn. Heart. J. 20 (1), 33–42. doi: 10.1536/ihj.20.33
Mosterd, A., Cost, B., Hoes, A. W., de Bruijne, M. C., Deckers, J. W., Hofman, A., et al. (2001). The prognosis of heart failure in the general population: the rotterdam study. Eur. Heart J. 22 (15), 1318–1327. doi: 10.1053/euhj.2000.2533
Muller-Ehmsen, J., Schwinger, R. H. (2004). TNF and congestive heart failure: therapeutic possibilities. Expert Opin. Ther. Targets 8 (3), 203–209. doi: 10.1517/14728222.8.3.203
Musa, A., Tripathi, S., Dehmer, M., Yli-Harja, O., Kauffman, S. A., Emmert-Streib, F. (2019). Systems pharmacogenomic landscape of drug similarities from LINCS data: drug association networks. Sci. Rep. 9 (1), 7849. doi: 10.1038/s41598-019-44291-3
Musa, A., Tripathi, S., Kandhavelu, M., Dehmer, M., Emmert-Streib, F. (2018). Harnessing the biological complexity of big data from LINCS gene expression signatures. PLoS One 13 (8), e0201937. doi: 10.1371/journal.pone.0201937
Narula, J., Haider, N., Virmani, R., DiSalvo, T. G., Kolodgie, F. D., Hajjar, R. J., et al. (1996). Apoptosis in myocytes in end-stage heart failure. N. Engl. J. Med. 335 (16), 1182–1189. doi: 10.1056/NEJM199610173351603
Novac, N. (2013). Challenges and opportunities of drug repositioning. Trends Pharmacol. Sci. 34 (5), 267–272. doi: 10.1016/j.tips.2013.03.004
Nozaki, N., Yamaguchi, S., Yamaoka, M., Okuyama, M., Nakamura, H., Tomoike, H. (1998). Enhanced expression and shedding of tumor necrosis factor (TNF) receptors from mononuclear leukocytes in human heart failure. J. Mol. Cell Cardiol. 30 (10), 2003–2012. doi: 10.1006/jmcc.1998.0761
Orea-Tejeda, A., Arrieta-Rodriguez, O., Castillo-Martinez, L., Rodriguez-Reyna, T., Asensio-Lafuente, E., Granados-Arriola, J., et al. (2007). Effects of thalidomide treatment in heart failure patients. Cardiology 108 (4), 237–242. doi: 10.1159/000096829
Patten, R. D., Pourati, I., Aronovitz, M. J., Baur, J., Celestin, F., Chen, X., et al. (2004). 17beta-estradiol reduces cardiomyocyte apoptosis in vivo and in vitro via activation of phospho-inositide-3 kinase/Akt signaling. Circ. Res. 95 (7), 692–699. doi: 10.1161/01.RES.0000144126.57786.89
Pedram, A., Razandi, M., O’Mahony, F., Lubahn, D., Levin, E. R. (2010). Estrogen receptor-beta prevents cardiac fibrosis. Mol. Endocrinol. 24 (11), 2152–2165. doi: 10.1210/me.2010-0154
Peyvandipour, A., Saberian, N., Shafi, A., Donato, M., Draghici, S. (2018). A novel computational approach for drug repurposing using systems biology. Bioinformatics. 34 (16), 2817–2825. doi: 10.1093/bioinformatics/bty133
Robinson, M. D., Smyth, G. K. (2007). Moderated statistical tests for assessing differences in tag abundance. Bioinformatics 23 (21), 2881–2887. doi: 10.1093/bioinformatics/btm453
Santos, D. G., Resende, M. F., Mill, J. G., Mansur, A. J., Krieger, J. E., Pereira, A. C. (2010). Nuclear Factor (NF) kappaB polymorphism is associated with heart function in patients with heart failure. BMC Med. Genet. 11, 89. doi: 10.1186/1471-2350-11-89
Satoh, M., Matter, C. M., Ogita, H., Takeshita, K., Wang, C. Y., Dorn, G. W., et al. (2007). Inhibition of apoptosis-regulated signaling kinase-1 and prevention of congestive heart failure by estrogen. Circulation 115 (25), 3197–3204. doi: 10.1161/CIRCULATIONAHA.106.657981
Sawada, R., Iwata, H., Mizutani, S., Yamanishi, Y. (2015). Target-based drug repositioning using large-scale chemical–protein interactome data. J. Chem. Inf. Model. 55 (12), 2717–2730. doi: 10.1021/acs.jcim.5b00330
Smyth, G. K. (2004). Linear models and empirical bayes methods for assessing differential expression in microarray experiments. Stat. Appl. Genet. Mol. Biol. 3, Article3. doi: 10.2202/1544-6115.1027
So, H. C., Chau, C. K., Chiu, W. T., Ho, K. S., Lo, C. P., Yim, S. H., et al. (2017). Analysis of genome-wide association data highlights candidates for drug repositioning in psychiatry. Nat. Neurosci. 20 (10), 1342–1349. doi: 10.1038/nn.4618
Sola, S., Mir, M. Q., Lerakis, S., Tandon, N., Khan, B. V. (2006). Atorvastatin improves left ventricular systolic function and serum markers of inflammation in nonischemic heart failure. J. Am. Coll. Cardiol. 47 (2), 332–337. doi: 10.1016/j.jacc.2005.06.088
Subramanian, A., Narayan, R., Corsello, S. M., Peck, D. D., Natoli, T. E., Lu, X., et al. (2017). A next generation connectivity map: L1000 platform and the first 1,000,000 profiles. Cell 171 (6), 1437–1452 e1417. doi: 10.1016/j.cell.2017.10.049
Sy, J., Vitarelli, A., Gheorghiade, M. (1993). Nicardipine in heart failure: distinguishing its acute beneficial from its chronic effects. Minerva Cardioangiol. 41 (11), 535–541.
Vasudevan, S. R., Moore, J. B., Schymura, Y., Churchill, G. C. (2012). Shape-based reprofiling of FDA-approved drugs for the H(1) histamine receptor. J. Med. Chem. 55 (16), 7054–7060. doi: 10.1021/jm300671m
Velez Rueda, J. O., Palomeque, J., Mattiazzi, A. (2012). Early apoptosis in different models of cardiac hypertrophy induced by high renin–angiotensin system activity involves CaMKII. J. Appl. Physiol. (1985) 112 (12), 2110–2120. doi: 10.1152/japplphysiol.01383.2011
Wang, Z., Clark, N. R., and Ma’ayan, A. (2016). Drug-induced adverse events prediction with the LINCS L1000 data. Bioinformatics 32 (15), 2338–2345. doi: 10.1093/bioinformatics/btw168
Wishart, D. S., Feunang, Y. D., Guo, A. C., Lo, E. J., Marcu, A., Grant, J. R., et al. (2018). DrugBank 5.0: a major update to the DrugBank database for 2018. Nucleic Acids Res. 46 (D1), D1074–D1082. doi: 10.1093/nar/gkx1037
Wojnicz, R., Wilczek, K., Nowalany-Kozielska, E., Szygula-Jurkiewicz, B., Nowak, J., Polonski, L., et al. (2006). Usefulness of atorvastatin in patients with heart failure due to inflammatory dilated cardiomyopathy and elevated cholesterol levels. Am. J. Cardiol. 97 (6), 899–904. doi: 10.1016/j.amjcard.2005.09.142
Xue, H., Li, J., Xie, H., Wang, Y. (2018). Review of drug repositioning approaches and resources. Int. J. Biol. Sci. 14 (10), 1232–1244. doi: 10.7150/ijbs.24612
Yang, Y. J., Zhu, W. L., Zhang, X., Zhou, X. W., Zhu, Z. M. (2005). [PTEN/PI3K pathways are involved in the signal transduction of myocardial remodeling in patients with congestive heart failure]. Zhonghua Yi Xue Za Zhi 85 (17), 1201–1204. doi: 10.3760/j:issn:0376-2491.2005.17.014
Zhang, Y. M., Cockerill, S., Guntrip, S. B., Rusnak, D., Smith, K., Vanderwall, D., et al. (2004). Synthesis and SAR of potent EGFR/erbB2 dual inhibitors. Bioorg. Med. Chem. Lett. 14 (1), 111–114. doi: 10.1016/j.bmcl.2003.10.010
Keywords: heart failure, drug repositioning, gene signature, systems biology, connectivity map
Citation: Yang G, Ma A and Qin ZS (2019) An Integrated System Biology Approach Yields Drug Repositioning Candidates for the Treatment of Heart Failure. Front. Genet. 10:916. doi: 10.3389/fgene.2019.00916
Received: 29 May 2019; Accepted: 29 August 2019;
Published: 25 September 2019.
Edited by:
Zhengqing Ouyang, Jackson Laboratory for Genomic Medicine, United StatesReviewed by:
Sungwon Jung, Gachon University, South KoreaFrank Emmert-Streib, University of Tampere, Finland
Copyright © 2019 Yang, Ma and Qin. This is an open-access article distributed under the terms of the Creative Commons Attribution License (CC BY). The use, distribution or reproduction in other forums is permitted, provided the original author(s) and the copyright owner(s) are credited and that the original publication in this journal is cited, in accordance with accepted academic practice. No use, distribution or reproduction is permitted which does not comply with these terms.
*Correspondence: Zhaohui S. Qin, zhaohui.qin@emory.edu