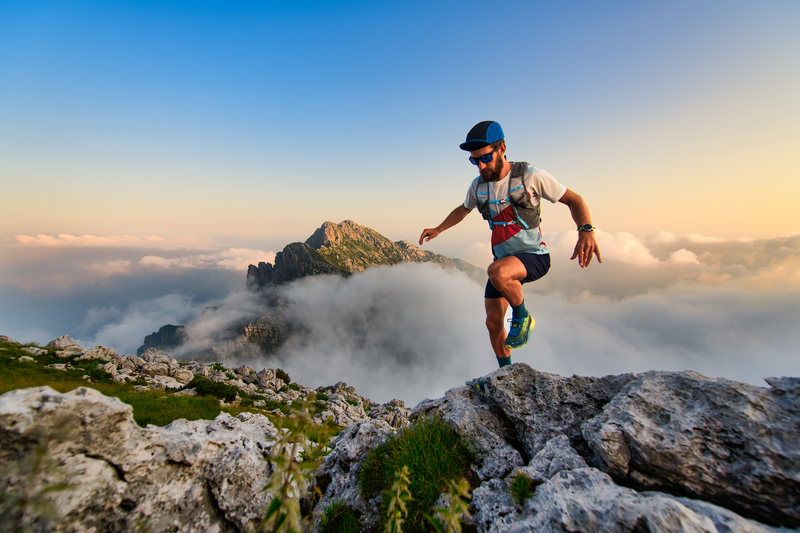
94% of researchers rate our articles as excellent or good
Learn more about the work of our research integrity team to safeguard the quality of each article we publish.
Find out more
ORIGINAL RESEARCH article
Front. Genet. , 30 August 2019
Sec. Applied Genetic Epidemiology
Volume 10 - 2019 | https://doi.org/10.3389/fgene.2019.00765
Deletion of glutathione S-transferase µ1 (GSTM1) is common in populations and has been asserted to associate with chronic kidney disease progression in some research studies. The association needs to be validated. We estimated GSTM1 copy number using whole exome sequencing data in the DiscovEHR cohort. Kidney failure was defined as requiring dialysis or receiving kidney transplant using data from the electronic health record and linkage to the United States Renal Data System, or the most recent eGFR < 15 ml/min/1.73 m2. In a cohort of 46,983 unrelated participants, 28.8% of blacks and 52.1% of whites had 0 copies of GSTM1. Over a mean of 9.2 years follow-up, 645 kidney failure events were observed in 46,187 white participants, and 28 in 796 black participants. No significant association was observed between GSTM1 copy number and kidney failure in Cox regression adjusting for age, sex, BMI, smoking status, genetic principal components, or comorbid conditions (hypertension, diabetes, heart failure, coronary artery disease, and stroke), whether using a genotypic, dominant, or recessive model. In sensitivity analyses, GSTM1 copy number was not associated with kidney failure in participants that were 45 years or older at baseline, had baseline eGFR < 60 ml/min/1.73 m2, or with baseline year between 1996 and 2002. In conclusion, we found no association between GSTM1 copy number and kidney failure in a large cohort study.
Glutathione S-transferase µ1 (GSTM1), belongs to the family of glutathione-S-transferases that metabolize a broad range of reactive oxygen species and aldehydes (Hayes et al., 2005; Yang et al., 2009). Loss of GSTM1 is very common in the population; ∼50% of whites and 27% of blacks have 0 copies of GSTM1 (Garte et al., 2001). Deletion of one or both copies of the gene results in reduced amount of GSTM1 (Board et al., 1990) and could lead to increased oxidative stress due to diminished ability to neutralize reactive chemical species. An association between loss of GSTM1 and chronic kidney disease (CKD) progression has been reported in the African American Study of Kidney and Hypertension (AASK) (Chang et al., 2013). The association between GSTM1 copy number and kidney failure was reported in the Atherosclerosis Risk in Communities (ARIC) study, which had a larger sample size, both in blacks and whites (Tin et al., 2017). However, data from smaller case–control studies have had mixed findings (Yang et al., 2004; Agrawal et al., 2007; Tiwari et al., 2009; Gutierrez-Amavizca et al., 2013; Nomani et al., 2016). The inconsistent results may be due to insufficient sample size and different populations and disease background.
The Geisinger MyCode® Community Health Initiative is an EHR-linked biobank for precision medicine research (Carey et al., 2016). The population served by Geisinger have low rates of out migration; thus, the electronic health record (EHR) data are relatively complete longitudinally, with a median of 14 years of follow-up (Carey et al., 2016). In addition, a high proportion of participants were found to have first- and second-degree relatedness through cryptic relatedness analysis (Staples et al., 2018). Through the ongoing DiscovEHR collaboration with the Regeneron Genetic Center, whole exome sequence (WES) data are available from ∼92,000 MyCode® participants to date (Abul-Husn et al., 2016; Carey et al., 2016; Dewey et al., 2016). These comprehensive clinical data are linked to matched genetic data and provide power to identify disease-genetic associations (Rader and Damrauer, 2016).
Confirming whether or not loss of GSTM1 increases the risk of kidney failure is important, given the high prevalence of GSTM1 loss in the population and the serious morbidity and mortality associated with kidney failure. In this study, we examine the relationship between GSTM1 copy number and incident kidney failure in the Geisinger MyCode cohort.
The study population included 72,756 participants in the Geisinger–Regeneron DiscovEHR cohort with WES data and at least three serum creatinine values. We excluded participants who had baseline eGFR < 15 ml/min/1.73 m2 or history of dialysis or transplant (n = 417), baseline age <18 (n = 1,960), missing BMI values (n = 230), and unknown smoking status (n = 2,694). Cryptic relatedness was evaluated using IBD method in PLINK1.9 (Chang et al., 2015). One of the related pair of participants with PI_HAT ≥ 0.125 were removed to reduce confounding from other shared genetic/environmental factors that could not be assessed, resulting in 46,983 participants (Supplement Figure 1). We estimated eGFR using the CKD-Epidemiology Collaboration equation (Levey et al., 2009; Levey and Stevens, 2010). We defined the study period, from a baseline time of the second serum creatinine to the time of a renal endpoint, or the last serum creatinine test.
An assignment of kidney failure was made if any of the following criteria was met: 1) the last available eGFR was <15 ml/min/1.73 m2; 2) the EHR showed an International Classification of Disease (ICD) code for end stage renal disease (ESRD) (ICD9: 585.6, ICD10: N18.6); and 3) receipt of dialysis or transplant per linkage to the United States Renal Data System (USRDS). For participants who met more than one criteria, the earliest documented date of the criterion was considered the kidney failure date.
The following data elements were extracted from the EHR: baseline age, sex, self-reported race, body mass index [BMI, BMI = weight (kg)/height (m)2], serum creatinine, smoking status, and ICD-9/10 coded diagnosis of hypertension, diabetes, coronary artery disease, heart failure, and stroke. Genetic principal components were calculated by PLINK1.9 (www.cog-genomics.org/plink/1.9/) (Chang et al., 2015).
DNA was sequenced in two batches at the Regeneron Genetic Center. The WES data processing has been described elsewhere (Dewey et al., 2016; Staples et al., 2018), and details are also provided in the supplementary methods. Copy number of GSTM1 was estimated using the method reported previously (Tin et al., 2017). Briefly, sequence coverage was normalized using CLAMMS software (Packer et al., 2016). Normalized coverage of all eight exons of GSTM1 was summed for each participant. Thresholds for GSTM1 copy number were determined empirically according to the distribution of sum of normalized coverage (Supplementary Figure 2). The copy number of GSTM5 was estimated the same way as GSTM1 (Supplementary notes).
Hardy–Weinberg equilibrium (HWE) was estimated using chi-square test for GSTM1 copy number. A more stringent significance level of 1e−5 was selected due to the large sample size (46,187) for whites. Nominal significance level of 0.05 was used for blacks as the small sample size (796). Missing baseline BMI values were imputed using mean BMI values calculated from all available BMI values for each participant, and missing smoking status at baseline was imputed using the most recent recorded smoking status in the EHR (Supplemental Figure 3). Baseline characteristics were compared using ANOVA for continuous variables and chi-squared tests for categorical variables. A p < 0.05/N was considered statistically significant after Bonferroni adjustment for multiple comparisons, where N is the number of variables compared. Kaplan–Meier curves were plotted by GSTM1 copy number, and survival differences between genotype groups were assessed using a log-rank test. All subsequent analyses were stratified by race given the difference in allele frequency and sample size. Two models were evaluated. In model 1, Cox proportional hazards model was adjusted for age, sex, and the first four genetic principle components; based on model 1, model 2 was additionally adjusted for risk factors including baseline eGFR, smoking status, BMI, hypertension, diabetes, coronary artery disease, heart failure, and stroke. Three genetic models were evaluated: 1) genotypic model, using copy numbers 0, 1, and 2 as three categories; 2) dominant model (0 or 1 copy vs. 2 copies GSTM1); and 3) recessive model (1 or 2 copies vs. 0 copies of GSTM1). To explore whether the effect of GSTM1 loss was stronger in specific higher-risk subgroups, sensitivity analyses were completed including subsets of 1) older participants (baseline age ≥ 45 years), 2) participants with CKD (baseline eGFR < 60 ml/min/1.73 m2), and 3) participants with longer follow-up (baseline year 1996–2002). Power was estimated using powerCT() function in R package powerSurvEpi 0.1.0. Given the current sample size and event number, we have power of ≥0.8 to test hazard ratio of at least 1.4 and 2.8 for white and black cohort, respectively. All analyses were performed using R (version 3.4.3).
There were 46,983 unrelated participants included in the main analysis. Among these, 46,187 (98.3%) were white, and 796 (1.7%) were black. Frequency of GSTM1 copy number differed between blacks and whites (Supplemental Figure 4). Almost half of the blacks (49.2%) had one copy of GSTM1 compared with only 39.6% of whites, and more than half (52.1%) of whites had zero copies of GSTM1 compared with 28.8% of blacks. Chi-square test indicated GSTM1 copy number follows the HWE (Table 1; p values were 1 × 10−4 for whites and 0.618 for blacks).
Table 1 Characteristics of participants at baseline by GSTM1 copy number and follow-up kidney failure cases.
Baseline demographic and clinical characteristics of the participants stratified by race and GSTM1 copy numbers are provided in Table 1. The average baseline age for whites and blacks was 51 and 44 years old, respectively. The mean baseline eGFRs were 91 and 92 ml/min/1.73 m2. Prevalence of hypertension was 29% in whites and 44% in blacks, and prevalence of type 2 diabetes was 13% in whites and 21% in blacks at baseline. No statistically significant differences were observed for baseline characteristics among the three genotype groups when adjusted for multiple comparisons.
Over a mean follow-up of 9.2 years, there were a total of 645 kidney failure events in 46,187 white participants. Over a mean follow-up of 5.5 years, there were 28 events in 796 blacks. Kidney failure cases showed more males, older ages, lower eGFR in both white and black participants (Table 1). Unsurprisingly, kidney failure cases had higher percentage of risk diseases including hypertension, diabetes and cardiovascular diseases in white participants (Table 1). The case number in blacks was too small to have enough statistical power. No significant difference in kidney failure-free survival was found across GSTM1 copy numbers by log-rank test (p = 0.9 and 0.5 for whites and blacks, respectively, Figure 1).
Figure 1 Kaplan–Meier survival curves of time to kidney failure by GSTM1 copy number in white and black participants. In both races, participants without GSTM1 (black line) had longer kidney failure-free survival time than those with 1 or 2 copies of GSTM1 (blue and cyan lines). No significant difference was found by log-rank test. Tables below the figure showed the number of patients at risk with start time at month 0, 50, 100, 150, and 200.
Findings were similar in Cox regression analyses adjusting for other covariates (Table 2). In the genotypic model, after adjusting for age, sex, and the first four PCs, there was no difference in risk of kidney failure among white participants with 0 copies [hazard ratio (HR) 1.01, 95% confidence interval (CI): 0.81–1.48)] or 1 copy (HR 1.08, 95% CI: 0.80–1.47), compared to those with 2 copies. Findings were similar for black participants in the genotypic model adjusting for age, sex, and the first four PCs (0 copies HR 0.68, 95% CI: 0.22–2.15; 1 copy HR 1.13, 95% CI: 0.44–2.90). In the dominant model, after adjusting for age, sex, and the first four PCs, there was again no difference in risk of kidney failure among participants with 0 or 1 copies for white participants (HR 1.10, 95% CI: 0.82–1.46) or black participants with 0 or 1 copies (HR 0.96, 95% CI: 0.39–2.34), compared to their counterparts with 2 copies. In the recessive model, after adjusting for age, sex, and the first four PCs, there was no difference in risk of kidney failure among white participants with 0 copies (1.03, 95% CI: 0.88–1.20) or black participants with 0 copies (HR 0.63, 95% CI: 0.25–1.56), compared to their counterparts with 1 or 2 copies. Adjustment for additional renal risk factors in model 2 yielded similar results (Table 2 and Supplemental Tables 1–3).
Analyses were performed on white participants who were older than 45 years of age at baseline (n = 31,406), had baseline eGFR < 60 ml/min/1.73 m2 (n = 3,129), or had index date before 2003 (n = 14,572). No statistically significant differences in the risk of kidney failure were identified for participants older than 45 years of age at baseline (0 copies: HR 1.03. 95% CI: 0.74–1.42; 1 copy: HR 0.99. 95% CI: 0.71–1.37) or those with baseline eGFR < 60 ml/min/1.73 m2 (0 copies: HR 1.13, 95% CI: 0.75–1.69; 1 copy HR 1.04, 95% CI: 0.69–1.57) or for those with baseline year before 2003 (0 copies: HR 0.88, 95% CI: 0.59–1.32; 1 copy HR 0.88. 95% CI: 0.59–1.33) (Table 2 and Supplemental Tables 1–3).
To our knowledge, this is the largest study to investigate the association of GSTM1 copy number variation with kidney failure. There were a total of 46,187 unrelated whites and 796 blacks in the study with an average of 9.3 years’ follow-up. The frequency of the GSTM1 copy numbers were very similar to those reported previously (Garte et al., 2001; Chang et al., 2013; Tin et al., 2017). Our results showed no significant association between GSTM1 copy number and risk of kidney failure in unadjusted or adjusted analyses whether using a genotypic, dominant, or recessive genetic model.
Data from the ARIC and AASK cohorts suggested that the loss of GSTM1 increased the risk of kidney failure or accelerated CKD progression (Chang et al., 2013; Bodonyi-Kovacs et al., 2016; Tin et al., 2017). In ARIC, a community-based cohort of middle-aged black and white participants, there were 3,461 white participants with WES reads. In fully adjusted models among whites in ARIC, loss of GSTM1 was associated with risk of kidney failure (0 or 1 copy vs. 2 copies: HR 2.54; 95% CI: 1.32–4.88). It is possible that differences in baseline characteristics of the study populations could explain differing findings. Our cohort had a lower prevalence of smoking (never smoking 48 vs. 38%), a higher prevalence of diabetes (14 vs. 8%), and was more contemporary (median baseline year 2004 vs. ARIC baseline year 1987–1989). However, in sensitivity analyses in 14,572 white participants with baseline year between 1996 and 2002 in our cohort, there was no association between GSTM1 loss and risk of kidney failure.
There is also the possibility that GSTM1 may only be deleterious when GFR falls below certain levels or in the setting of specific types of kidney disease. In the AASK study, a randomized trial comparing different antihypertensive medications and levels of blood pressure control in blacks with CKD attributed to hypertension, 692 participants had GSTM1 genotyping completed. Loss of GSTM1 in AASK was associated with increased risk of CKD progression (HR 0 copy: HR 1.88; 95% CI: 1.07–3.30; HR 1 copy: 1.68; 95% CI: 1.00–2.84). While there were few blacks in our study population with CKD, we found no association between loss of GSTM1 and risk of kidney failure in 3,129 white participants with baseline eGFR < 60 ml/min/1.73 m2. Some smaller case–control studies also tested a recessive genetic model (0 copies vs. 1 or 2 copies) of GSTM1 and found that GSTM1 0 copy was associated with kidney failure in several case–control studies (Agrawal et al., 2007; Chang et al., 2013; Gutierrez-Amavizca et al., 2013; Suvakov et al., 2013). In our study, however, we examined both recessive and dominant genetic models and found no associations between GSTM1 copy number and risk of kidney failure.
Some limitations of this study are worth noting. First, the genotypes of GSTM1 in this study were derived from WES, and the thresholds were determined empirically from the histogram. Multiplex PCR validation was not performed for this study. However, this method was used in the ARIC study, which showed 99.3% agreement when comparing the results of 0 copy with ≥ 1 copy from PCR (Tin et al., 2017). Moreover, the frequency of GSTM1 copy numbers in this study is similar to those reported previously for both whites and blacks (Chang et al., 2013; Tin et al., 2017), and it follows the Hardy–Weinberg equilibrium. We also examined the possibility of mismapping due to the complexity of GSTM locus. The histogram of the sum of normalized coverage of GSTM5, the paralog gene of GSTM1, is unimodal distribution. Based on these evidences, we believe that the copy number results derived from WES had a low error rate and can be used with confidence for analysis. Second, the sample size of blacks was small with only 796 patients available for analysis, limiting the power to examine an association between GSTM1 and kidney failure in this population. The strength of this study is the large sample size of whites, which included 46,187 patients, allowing for large subgroup analyses in older patients, those with baseline eGFR < 60 ml/min/1.73 m2, and those with longer duration of follow-up. Finally, other factors such as SNPs and haplotypes in the GSTM cluster, CNV of other GST genes, such as GSTT1, and environmental factors such as diet were not examined. A recent study identified substantial differences of SNP-based haplotype in GSTM cluster among groups of individuals with different GSTM1 CNV, suggesting a role for local genetic context in the deletion frequency thus the deletion-related effects on various disease (Khrunin et al., 2016). Studies that examined the combined effect of GSTM1 and GSTT1 deletion showed increased risk for ESRD in patients with null genotype of both GSTM1 and GSTT1 (Agrawal et al., 2007; Suvakov et al., 2013; Nomani et al., 2016). GSTM1 deletion is known to be protective for lung cancer among individuals with a high intake of cruciferous vegetable and deleterious among those with low intake of cruciferous vegetable (London et al., 2000; Zhao et al., 2001; Wang et al., 2004; Brennan et al., 2005; Carpenter et al., 2009). We were not able to analyze the effect modification between GSTM1 copy number and cruciferous vegetable intake.
In conclusion, our study does not support an association between loss of GSTM1 and increased risk of kidney failure in whites. Additional research is needed to confirm whether loss of GSTM1 increases risk of kidney failure in certain subgroups such as blacks. The interaction with diet, and genetic context will also be examined in future.
The individual EHR and genetics datasets (even de-identified) used and/or analyzed during the current study are not publicly available. The availability restriction is a condition of the ethical approval and Geisinger policy and terms with RGC. Collaboration requests and data use agreements with Geisinger are necessary to obtain access to the deidentified EHR data.
All participants provided their informed written consent, and the study was approved by the Geisinger Institutional Review Board.
AT, AC, and ML designed the study; DH extracted data; YZ and WZ performed the analysis and drafted the manuscript. MW, ML, and AC did critical review on the paper. RGC supported the study and reviewed the manuscript. All authors approved the final version of the manuscript.
The Regeneron Genetics Center funded the collection of genomic study samples, the generation of genotype data, and genotype imputation. Geisinger provided funding for clinical data extraction and genetic association analysis. AC is supported by the National Institutes of Health/National Institute of Diabetes and Digestive and Kidney Diseases grant K23 DK106515-01
Regeneron Genetics Center authors were employed by Regeneron Pharmaceuticals. The remaining authors declare that the research was conducted in the absence of any commercial or financial relationships that could be construed as a potential conflict of interest.
The authors thank the staff and participants of MyCode. The Regeneron Genetics Center funded the collection of study samples and the generation of whole exome sequencing data. Data reported here were supplied by the US Renal Data System. The interpretation and reporting of these data are the responsibility of the authors and in no way should be seen as an official policy or interpretation of the US government. The authors thank Ilene G. Ladd, who is the project manager at Genomic Medicine Institute, for English editing. Regeneron Genetics Center contributors were the following: Goncalo Abecasis, Aris Baras, Michael Cantor, Giovanni Coppola, Aris Economides, John D. Overton, Jeffrey G. Reid, Alan Shuldiner, Christina Beechert, Caitlin Forsythe, Erin D. Fuller, Zhenhua Gu, Michael Lattari, Alexander Lopez, Thomas D. Schleicher, Maria Sotiropoulos Padilla, Karina Toledo, Louis Widom, Sarah E. Wolf, Manasi Pradhan, Kia Manoochehri, Ricardo H. Ulloa, Xiaodong Bai, Suganthi Balasubramanian, Leland Barnard, Andrew Blumenfeld, Yating Chai, Gisu Eom, Lukas Habegger, Young Hahn, Alicia Hawes, Shareef Khalid, Evan K. Maxwell, John Penn, Jeffrey C. Staples, Ashish Yadav, Nilanjana Banerjee, Michael Cantor, Goncalo Abecasis, Amy Damask, Lauren Gurski, Alexander Li, Nan Lin, Daren Liu, Jonathan Marchini, Anthony Marcketta, Shane McCarthy, Colm O’Dushlaine, Charles Paulding, Claudia Schurmann, Dylan Sun, Tanya Teslovich, Cristopher Van Hout, Bin Ye, Jan Freudenberg, Nehal Gosalia, Claudia Gonzaga-Jauregui, Julie Horowitz, Kavita Praveen, Paloma M. Guzzardo, Marcus B. Jones, and Lyndon J. Mitnaul.
The Supplementary Material for this article can be found online at: https://www.frontiersin.org/articles/10.3389/fgene.2019.00765/full#supplementary-material
Abul-Husn, N. S., Manickam, K., Jones, L. K., Wright, E. A., Hartzel, D. N., Gonzaga-Jauregui, C., et al. (2016). Genetic identification of familial hypercholesterolemia within a single U.S. health care system. Science 354 (6319), aaf700. doi: 10.1126/science.aaf7000
Agrawal, S., Tripathi, G., Khan, F., Sharma, R., Baburaj, V. P. (2007). Relationship between GSTs gene polymorphism and susceptibility to end stage renal disease among North Indians. Ren Fail. 29 (8), 947–953. doi: 10.1080/08860220701641314
Board, P., Coggan, M., Johnston, P., Ross, V., Suzuki, T., Webb, G. (1990). Genetic heterogeneity of the human glutathione transferases: a complex of gene families. Pharmacol. Ther. 48 (3), 357–369. doi: 10.1016/0163-7258(90)90054-6
Bodonyi-Kovacs, G., Ma, J. Z., Chang, J., Lipkowitz, M. S., Kopp, J. B., Winkler, C. A., et al. (2016). Combined effects of GSTM1 null allele and APOL1 renal risk alleles in CKD progression in the African American study of kidney disease and hypertension trial. J. Am. Soc. Nephrol. 27 (10), 3140–3152. doi: 10.1681/ASN.2015050487
Brennan, P., Hsu, C. C., Moullan, N., Szeszenia-Dabrowska, N., Lissowska, J., Zaridze, D., et al. (2005). Effect of cruciferous vegetables on lung cancer in patients stratified by genetic status: a mendelian randomisation approach. Lancet 366 (9496), 1558–1560. doi: 10.1016/S0140-6736(05)67628-3
Carey, D. J., Fetterolf, S. N., Davis, F. D., Faucett, W. A., Kirchner, H. L., Mirshahi, U., et al. (2016). The Geisinger MyCode community health initiative: an electronic health record-linked biobank for precision medicine research. Genet. Med. 18 (9), 906–913. doi: 10.1038/gim.2015.187
Carpenter, C. L., Yu, M. C., London, S. J. (2009). Dietary isothiocyanates, glutathione S-transferase M1 (GSTM1), and lung cancer risk in African Americans and Caucasians from Los Angeles County, California. Nutr. Cancer 61 (4), 492–499. doi: 10.1080/01635580902752270
Chang, C. C., Chow, C. C., Tellier, L. C., Vattikuti, S., Purcell, S. M., Lee, J. J. (2015). Second-generation PLINK: rising to the challenge of larger and richer datasets. Gigascience 4, 7. doi: 10.1186/s13742-015-0047-8
Chang, J., Ma, J. Z., Zeng, Q., Cechova, S., Gantz, A., Nievergelt, C., et al. (2013). Loss of GSTM1, a NRF2 target, is associated with accelerated progression of hypertensive kidney disease in the African American Study of Kidney Disease (AASK). Am. J. Physiol. Renal Physiol. 304 (4), F348–F355. doi: 10.1152/ajprenal.00568.2012
Dewey, F. E., Murray, M. F., Overton, J. D., Habegger, L., Leader, J. B., Fetterolf, S. N., et al. (2016). Distribution and clinical impact of functional variants in 50,726 whole-exome sequences from the DiscovEHR study. Science 354 (6319), aaf6814. doi: 10.1126/science.aaf6814
Garte, S., Gaspari, L., Alexandrie, A. K., Ambrosone, C., Autrup, H., Autrup, J. L., et al. (2001). Metabolic gene polymorphism frequencies in control populations. Cancer Epidemiol. Biomarkers Prev. 10 (12), 1239–1248. http://cebp.aacrjournals.org/content/10/12/1239.long
Gutierrez-Amavizca, B. E., Orozco-Castellanos, R., Ortiz-Orozco, R., Padilla-Gutierrez, J., Valle, Y., Gutierrez-Gutierrez, N., et al. (2013). Contribution of GSTM1, GSTT1, and MTHFR polymorphisms to end-stage renal disease of unknown etiology in Mexicans. Indian J. Nephrol. 23 (6), 438–443. doi: 10.4103/0971-4065.120342
Hayes, J. D., Flanagan, J. U., Jowsey, I. R. (2005). Glutathione transferases. Annu. Rev. Pharmacol. Toxicol. 45, 51–88. doi: 10.1146/annurev.pharmtox.45.120403.095857
Khrunin, A. V., Filippova, I. N., Aliev, A. M., Tupitsina, T. V., Slominsky, P. A., Limborska, S. A. (2016). GSTM1 copy number variation in the context of single nucleotide polymorphisms in the human GSTM cluster. Mol. Cytogenet 9, 30. doi: 10.1186/s13039-016-0241-0
Levey, A. S., Stevens, L. A. (2010). Estimating GFR using the CKD Epidemiology Collaboration (CKD-EPI) creatinine equation: more accurate GFR estimates, lower CKD prevalence estimates, and better risk predictions. Am. J. Kidney Dis. 55 (4), 622–627. doi: 10.1053/j.ajkd.2010.02.337
Levey, A. S., Stevens, L. A., Schmid, C. H., Zhang, Y. L., Castro, AF, 3rd, Feldman, H. I., et al. (2009). A new equation to estimate glomerular filtration rate. Ann. Intern Med. 150 (9), 604–612. doi: 10.7326/0003-4819-150-9-200905050-00006
London, S. J., Yuan, J. M., Chung, F. L., Gao, Y. T., Coetzee, G. A., Ross, R. K., et al. (2000). Isothiocyanates, glutathione S-transferase M1 and T1 polymorphisms, and lung-cancer risk: a prospective study of men in Shanghai, China. Lancet 356 (9231), 724–729. doi: 10.1016/S0140-6736(00)02631-3
Nomani, H., Hagh-Nazari, L., Aidy, A., Vaisi-Raygani, A., Kiani, A., Rahimi, Z., et al. (2016). Association between GSTM1, GSTT1, and GSTP1 variants and the risk of end stage renal disease. Ren Fail. 38 (9), 1455–1461. doi: 10.1080/0886022X.2016.1214054
Packer, J. S., Maxwell, E. K., O’Dushlaine, C., Lopez, A. E., Dewey, F. E., Chernomorsky, R., et al. (2016). CLAMMS: a scalable algorithm for calling common and rare copy number variants from exome sequencing data. Bioinformatics 32 (1), 133–135. doi: 10.1093/bioinformatics/btv547
Rader, D. J., Damrauer, S. M. (2016). “Pheno” menal value for human health. Science 354 (6319), 1534–1536. doi: 10.1126/science.aal4573
Staples, J., Maxwell, E. K., Gosalia, N., Gonzaga-Jauregui, C., Snyder, C., Hawes, A., et al. (2018). Profiling and leveraging relatedness in a precision medicine cohort of 92,455 exomes. Am. J. Hum. Genet. 102 (5), 874–889. doi: 10.1016/j.ajhg.2018.03.012
Suvakov, S., Damjanovic, T., Stefanovic, A., Pekmezovic, T., Savic-Radojevic, A., Pljesa-Ercegovac, M., et al. (2013). Glutathione S-transferase A1, M1, P1 and T1 null or low-activity genotypes are associated with enhanced oxidative damage among haemodialysis patients. Nephrol. Dial Transplant 28 (1), 202–212. doi: 10.1093/ndt/gfs369
Tin, A., Scharpf, R., Estrella, M. M., Yu, B., Grove, M. L., Chang, P. P., et al. (2017). The loss of GSTM1 associates with kidney failure and heart failure. J. Am. Soc. Nephrol. 28 (11), 3345–3352. doi: 10.1681/ASN.2017030228
Tiwari, A. K., Prasad, P., B K T, Kumar, K. M., Ammini, A. C., Gupta, A., et al. (2009). Oxidative stress pathway genes and chronic renal insufficiency in Asian Indians with type 2 diabetes. J. Diabetes Complications 23 (2), 102–111. doi: 10.1016/j.jdiacomp.2007.10.003
Wang, L. I., Giovannucci, E. L., Hunter, D., Neuberg, D., Su, L., Christiani, D. C. (2004). Dietary intake of cruciferous vegetables, glutathione S-transferase (GST) polymorphisms and lung cancer risk in a Caucasian population. Cancer Causes Control 15 (10), 977–985. doi: 10.1007/s10552-004-1093-1
Yang, Y., Kao, M. T., Chang, C. C., Chung, S. Y., Chen, C. M., Tsai, J. J., et al. (2004). Glutathione S-transferase T1 deletion is a risk factor for developing end-stage renal disease in diabetic patients. Int. J. Mol. Med. 14 (5), 855–859. doi: 10.3892/ijmm.14.5.855
Yang, Y., Parsons, K. K., Chi, L., Malakauskas, S. M., Le, T. H. (2009). Glutathione S-transferase-micro1 regulates vascular smooth muscle cell proliferation, migration, and oxidative stress. Hypertension 54 (6), 1360–1368. doi: 10.1161/HYPERTENSIONAHA.109.139428
Zhao, B., Seow, A., Lee, E. J., Poh, W. T., Teh, M., Eng, P., et al. (2001). Dietary isothiocyanates, glutathione S-transferase -M1, -T1 polymorphisms and lung cancer risk among Chinese women in Singapore. Cancer Epidemiol. Biomarkers Prev. 10 (10), 1063–1067. http://cebp.aacrjournals.org/content/10/10/1063.long
Keywords: GSTM1, kidney failure, copy number, large cohort, electronic health records
Citation: Zhang Y, Zafar W, Hartzel DN, Williams MS, Tin A, Chang AR and Lee MTM (2019) GSTM1 Copy Number Is Not Associated With Risk of Kidney Failure in a Large Cohort. Front. Genet. 10:765. doi: 10.3389/fgene.2019.00765
Received: 11 April 2019; Accepted: 19 July 2019;
Published: 30 August 2019.
Edited by:
Dana C. Crawford, Case Western Reserve University, United StatesReviewed by:
Hui-Qi Qu, Children’s Hospital of Philadelphia, United StatesCopyright © 2019 Zhang, Zafar, Hartzel, Williams, Tin, Chang and Lee. This is an open-access article distributed under the terms of the Creative Commons Attribution License (CC BY). The use, distribution or reproduction in other forums is permitted, provided the original author(s) and the copyright owner(s) are credited and that the original publication in this journal is cited, in accordance with accepted academic practice. No use, distribution or reproduction is permitted which does not comply with these terms.
*Correspondence: Ming Ta Michael Lee, bWxlZTJAZ2Vpc2luZ2VyLmVkdQ==
†These authors have contributed equally to this work
Disclaimer: All claims expressed in this article are solely those of the authors and do not necessarily represent those of their affiliated organizations, or those of the publisher, the editors and the reviewers. Any product that may be evaluated in this article or claim that may be made by its manufacturer is not guaranteed or endorsed by the publisher.
Research integrity at Frontiers
Learn more about the work of our research integrity team to safeguard the quality of each article we publish.