- 1Engelhardt Institute of Molecular Biology, Russian Academy of Sciences, Moscow, Russia
- 2National Medical Research Radiological Center, Ministry of Health of the Russian Federation, Moscow, Russia
- 3Federal State Autonomous Educational Institution of Higher Education, I.M. Sechenov First Moscow State Medical University of the Ministry of Health of the Russian Federation, Moscow, Russia
- 4Vavilov Institute of General Genetics, Russian Academy of Sciences, Moscow, Russia
Older age is one of the main risk factors for cancer development. The incidence of prostate cancer, as a multifactorial disease, also depends upon demographic factors, race, and genetic predisposition. Prostate cancer most frequently occurs in men over 60 years of age, indicating a clear association between older age and disease onset. Carcinogenesis is followed by the deregulation of many genes, and some of these changes could serve as biomarkers for diagnosis, prognosis, prediction of drug therapy efficacy, as well as possible therapeutic targets. We have performed a bioinformatic analysis of a The Cancer Genome Atlas (TCGA) data and RNA-Seq profiling of a Russian patient cohort to reveal prognostic markers of locally advanced lymph node-negative prostate cancer (lymph node-negative LAPC). We also aimed to identify markers of the most common molecular subtype of prostate cancer carrying a fusion transcript TMPRSS2-ERG. We have found several genes that were differently expressed between the favorable and unfavorable prognosis groups and involved in the enriched KEGG pathways based on the TCGA (B4GALNT4, PTK6, and CHAT) and Russian patient cohort data (AKR1C1 and AKR1C3). Additionally, we revealed such genes for the TMPRSS2-ERG prostate cancer molecular subtype (B4GALNT4, ASRGL1, MYBPC1, RGS11, SLC6A14, GALNT13, and ST6GALNAC1). Obtained results contribute to a better understanding of the molecular mechanisms behind prostate cancer progression and could be used for further development of the LAPC prognosis marker panel.
Introduction
Aging is a complex phenomenon, which is characterized by the deregulation of various cellular processes and involves metabolic, epigenomic, transcriptomic, genomic, and proteomic alterations, and ultimately leads to death. Aberrant gene expression, somatic mutations, DNA damage caused by exposure to ionizing radiation, reactive oxygen species, and chemicals accumulate throughout the life of a cell. These alterations can initiate activation of oncogenes and deactivation of tumor suppressor genes resulting in tumorigenesis. Moreover, one of the main causes of cancers is the disruption of cellular senescence, which is associated with proliferative arrest blocking multiplication of potentially malignant cells. The association between aging and cancer is well documented in the literature, and several cancer types are classified as age-related diseases (Kudryavtseva et al., 2016).
Being a multifactorial age-related disease, prostate cancer (PC) also depends upon demographic factors, race, and genetic predisposition (Belova et al., 2017). A clear association between older age and prostate cancer is confirmed by the elevation of incidence in men over 60 years of age (Vinjamoori et al., 2012). PC is associated with aging through the disruption of glandular homeostasis, which is caused by an imbalance between cell proliferation and death. The imbalance between mitosis and apoptosis also leads to various diseases, including prostate cancer (Plati et al., 2011).
Carcinogenesis is associated with the disruption in the functioning of many genes that lead to deregulations of metabolic and signaling pathways (Cherkasova et al., 2011; Oparina et al., 2013; Fedorova et al., 2015; Snezhkina et al., 2016a; Fedorova et al., 2017; Kudryavtseva et al., 2018; Snezhkina et al., 2018a; Pudova et al., 2018). Some of these changes can serve as markers for diagnosis and prognosis, predicting the efficacy of drug therapy (Krasnov et al., 2015; Knyazev et al., 2016; Jhun et al., 2017; Kudryavtseva et al., 2019), as well as possible therapeutic targets. However, a large number of studies indicate difficulties in the choice of universal prognostic markers (Dmitriev et al., 2015) that are equally effective for various stages of the disease. Moreover, most cancer types and histological subgroups of cancer are divided into subtypes. These tumor subtypes differ in clinical and molecular genetic characteristics, and each could be identified using a set of markers that predict the aggressiveness of the disease and evaluate sensitivity to medicines (Dmitriev et al., 2014; Krasnov et al., 2015; Snezhkina et al., 2016b; Krasnov et al., 2017; Snezhkina et al., 2018b).
Therefore, a detailed study of the molecular genetic characteristics of different PC subgroups, as well as the selection of markers for each molecular subtype and propensity for metastasis, is particularly important.
Based on the data from “The Cancer Genome Atlas” (TCGA, https://portal.gdc.cancer.gov) project, several molecular subtypes of PC have been identified. The most common ones comprise three groups characterized by the formation of the fusion transcripts (TMPRSS2-ERG, TMPRSS2-ETV1, and TMPRSS2-ETV4) and a group that has somatic mutations in the SPOP gene (The Cancer Genome Atlas Research Network, 2015). Making well informed decisions on the applicability of adjuvant therapy following radical PC surgical treatment remains an unanswered problem. This problem is relevant for cohorts of patients with locally advanced prostate cancer (LAPC) without lymphatic dissemination (LD), and particularly for those patients with the most widely represented molecular subtype of PC associated with the expression of the fusion transcript TMPRSS2-ERG (approximately 40% of PC patients). Many researchers attribute this transcript to factors associated with unfavorable disease prognosis. One of the most effective approaches for identification of prognostic markers is to use transcriptome profiling data deposited in the TCGA consortium database (approximately 500 prostate cancer samples) (The Cancer Genome Atlas Research Network, 2015).
Currently, there are several commercially available services that help to predict the aggressiveness of the disease and to choose optimal treatment strategies. However, these decision-making gene expression panels were initially developed and validated on patient cohorts with a significantly different ethnic composition to that of Russian patients.
Thus, the aim of this study was to identify reliable prognostic markers of both locally advanced lymph node-negative prostate cancer and the most common PC molecular subtype, TMPRSS2-ERG.
Materials and Methods
Tumor Samples
Prostate cancer samples and adjacent histologically normal tissues were obtained from patients that underwent surgical intervention in the P.A. Hertzen Moscow Oncology Research Center (branch of the National Medical Research Radiological Center, Ministry of Health of the Russian Federation) in 2015–2016. All materials were collected and characterized by the Organization’s Pathology Department according to the WHO Classification of Tumours of the Urinary System and Male Genital Organs (Moch et al., 2016). Each sample contained a minimum 70% of tumor cells. Tissue samples were immediately frozen and stored in liquid nitrogen following surgical resection.
In the current study, we used 32 lymph node-negative LAPC (adenocarcinoma) samples obtained from patients that underwent surgical treatment and did not receive neoadjuvant therapy. The samples have the following characteristics: pT3a and pT3b categories, negative resection margins, any PSA value, and any Gleason score (Supplementary Table S1). We did not include normal prostate tissues in the current study.
All patients have had at least 1 year of follow-up. The serum level of PSA was recorded every 6 months. Biochemical reoccurrence of the disease was determined as an increase in PSA level > 0.2 ng/ml in three consecutive measurements with an interval of not less than 2 weeks. Based on these data, patients were divided into the favorable prognosis group (no relapse within a year) and unfavorable prognosis group (biochemical relapse within a year).
The study was approved by The Ethics committee of P.A. Hertzen Moscow Oncology Research Center, Ministry of Health of the Russian Federation. The study was done in accordance with the principles outlined in the Declaration of Helsinki (1964). All patients gave their informed consent for participation in the study.
Isolation of RNA, Library Preparation, and Next Generation Sequencing (NGS)
Total RNA was isolated from 32 tumor samples using a MagNA Pure Compact RNA Isolation Kit (Roche, Switzerland). The RNA concentration was determined using a Qubit 2.0 Fluorometer (Thermo Fisher Scientific, USA). The RIN (RNA Integrity Number) parameter, which characterizes the integrity of RNA, was evaluated using an Agilent 2100 Bioanalyzer (Agilent Technologies, USA). RIN for all samples studied was no less than 8.0.
Libraries were prepared using a TruSeq® Stranded mRNA LT Kit (Illumina, USA) according to the manufacturer’s protocol. The average size of prepared cDNA libraries was 300 bp. Libraries were validated prior to sequencing using a quantitative PCR (qPCR).
Sequencing was performed on a NextSeq500 System (Illumina) at the EIMB RAS “Genome” Center [http://www.eimb.ru/rus/ckp/ccu_genome_c.php]. Read length was 75 bp (single-end mode).
RNA-Seq Data Analysis of the Russian Patient Cohort
Read quality was evaluated using FastqQC [http://www.bioinformatics.babraham.ac.uk/publications.html]. Read pseudo-alignment to the reference genome (assembly GRCh38) and their counting were performed using Kallisto (Bray et al., 2016) [https://pachterlab.github.io/kallisto/]. The read count table was exported and used in the statistical environment R [https://www.r-project.org/]. To analyze the differential expression of genes, the Bioconductor package DESeq2 (Love et al., 2014) [http://www.bioconductor.org/packages/release/bioc/html/DESeq2.html] was used. Gene expression-level differences were accepted as statistically significant if they underwent a 2-fold change or greater and had a p-value of less than 0.05. Tumor tissue samples demonstrate heterogeneous gene expression profiles that do not have Gaussian or any other parametric distribution across the cohort. Hence, standard edgeR or DESeq2 tests should be used followed with a non-parametric test (e.g., Mann–Whitney U test). The decision should be made after the mandatory manual examination of expression profiles across the sampling. In the study, we supplied our data with Mann–Whitney p-values (the lowest rank was assigned to all samples with CPM [read counts per million] less than 0.5). Differences in gene expression levels (fold changes) between the favorable and unfavorable prognosis groups were transformed into the binary logarithms of fold changes (LogFC). Gene Ontology and KEGG pathway enrichment analysis were done using the clusterProfiler Bioconductor package (Yu et al., 2012) [http://bioconductor.org/packages/release/bioc/html/clusterProfiler.html].
Bioinformatic Analysis of the TCGA Data
To identify transcriptomic markers of unfavorable prognosis, the bioinformatic analysis was carried out using the results of TCGA transcriptome sequencing data generated on an Illumina platform (HiSeq 1500 System) and deposited into the TCGA database. We focused on the study of Caucasian patients, as this cohort is closest to the Russian population (Caucasians are identified in the database as “white”). The total sample size was 134 individuals. Next, we isolated a group of patients with locally advanced lymph node-negative prostate cancer according to the pathologic classification (pTNM). We only included patients that had undergone a prostatectomy, which is generally accompanied by lymphadenectomy (“Nx” was unacceptable). Patients who received neoadjuvant therapy were not included in the study. Finally, in the analysis, we included 113/21 patients with favorable/unfavorable prognosis, among them—42/10 patients of TMPRSS2-ERG group.
The transcriptome sequencing data from the TCGA (read counts) were exported for further analysis in R environment with the DESeq2 and clusterProfiler packages as described above.
Results
Bioinformatic Analysis of the TCGA Data
For lymph node-negative LAPC samples, a comparison between the favorable (no relapse for the entire observation period) and unfavorable (biochemical reoccurrence) prognosis groups was made, and 173 differentially expressed genes (DEGs) were revealed (Supplementary Table S2). This list includes genes for which the expression level underwent a 2-fold or higher changes and that were passed DESeq2 p < 0.05 threshold. Using these data, we performed Mann–Whitney test and identified 44 genes with Mann–Whitney p < 0.05 that could be potential markers of unfavorable prognosis in lymph node-negative LAPC (Figure 1). Next, KEGG pathway enrichment analysis was carried out using all set of identified DEGs (173 genes). This allowed to reveal biological pathways that are most likely responsible for phenotypic and clinical differences between favorable and unfavorable prognosis groups (Figure 2, Supplementary Table S5). Three genes (B4GALNT4, CHAT, and PTK6) with Mann–Whitney p < 0.05 were involved in the enriched KEGG pathways (Figure 3).
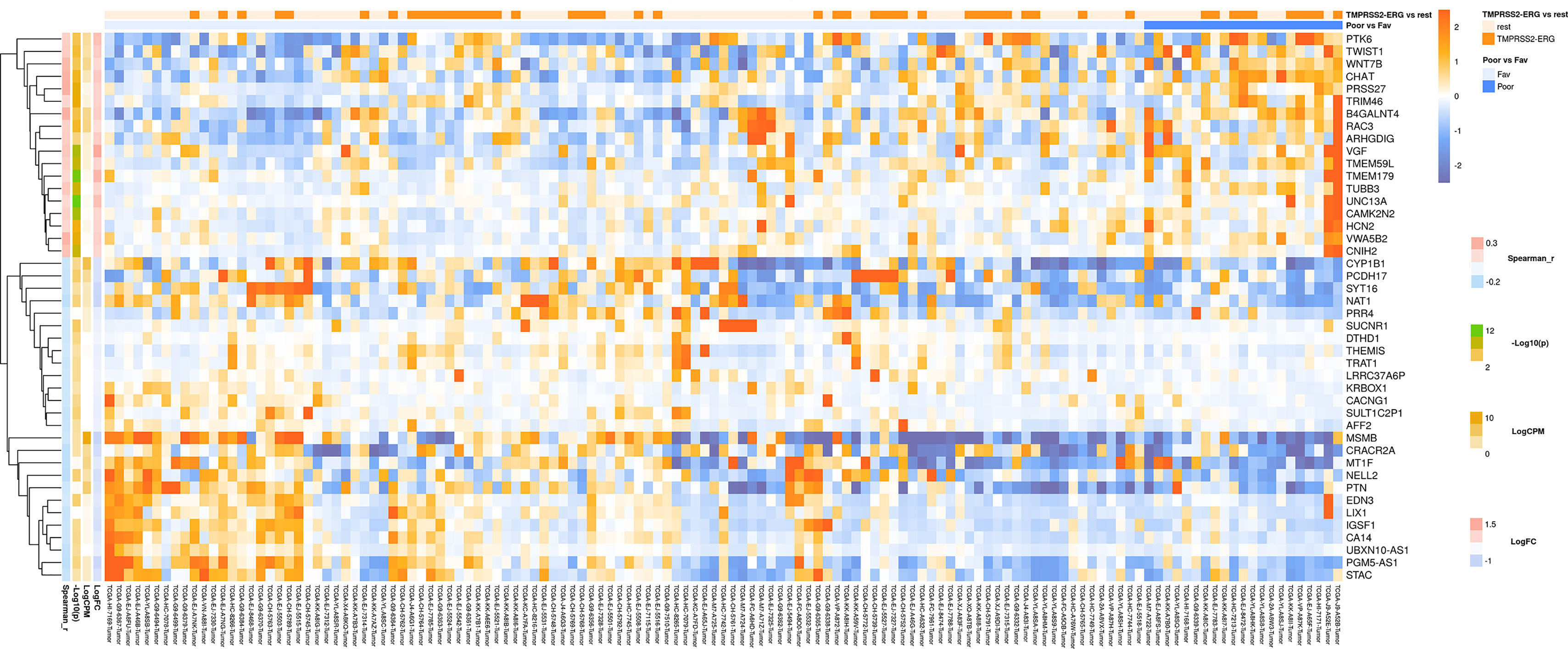
Figure 1 Heatmap demonstrating log relative expression level of top differentially expressed genes between the groups of adverse and favorable prognosis (TCGA data). Cell colors (blue-white-orange gradient) correspond the binary logarithm of the ratio of the expression level in a current sample to the average level across all the samples (per each gene). Blue - expression level is below the average, orange - above the average. Spearman_r—Spearman’s rank correlation coefficient. -Log10(p) is the negative logarithm of DESeq’s p-value. LogCPM—average binary logarithm of read counts per million (CPM). LogFC—binary logarithm of expression level fold change (samples with adverse prognosis compared to favorable prognosis).
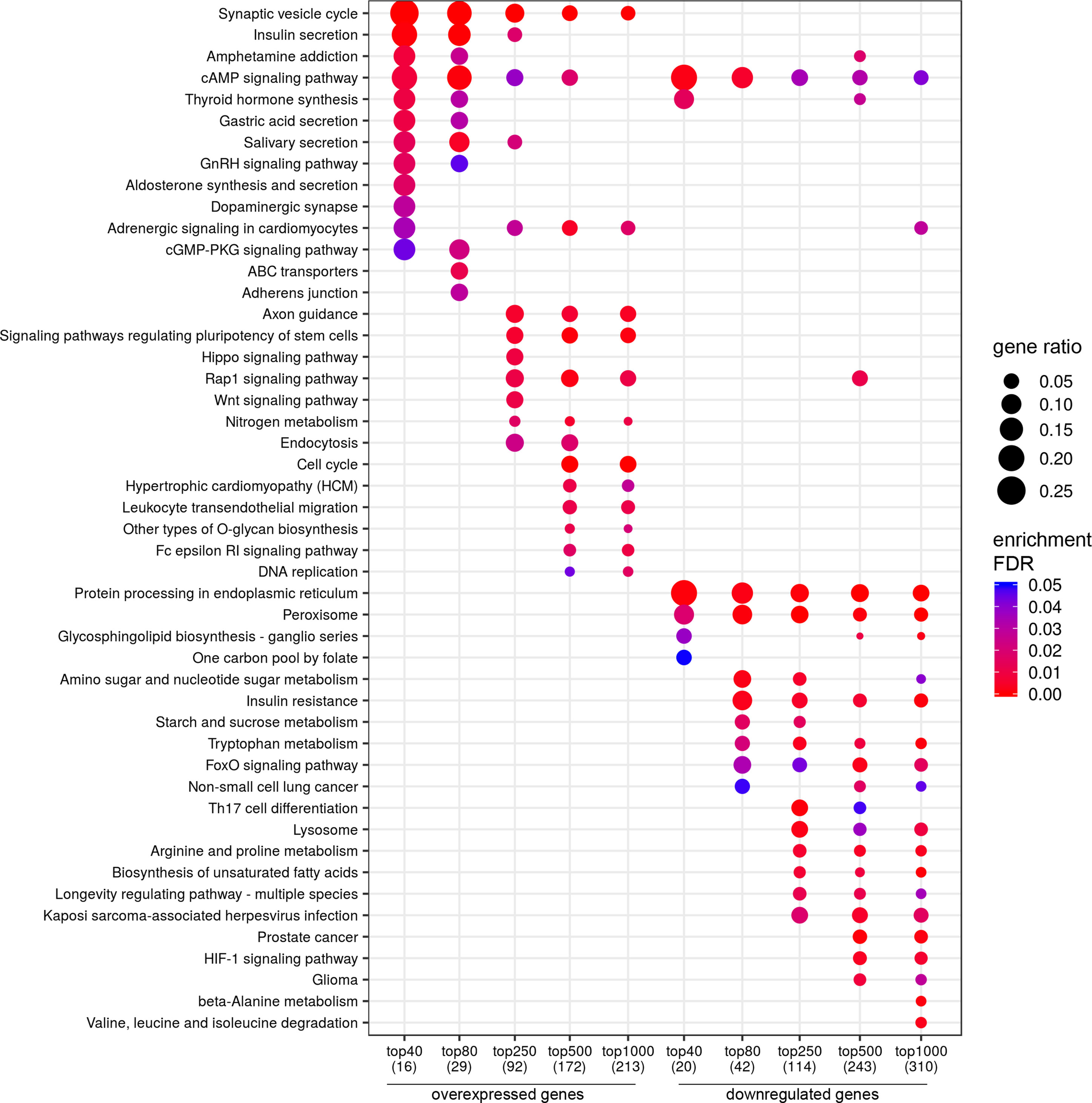
Figure 2 Dotplot showing the results of KEGG pathways enrichment analyses performed for top-40, 80, 250, 500, and 1,000 differentially expressed genes (either overexpressed or downregulated) between the groups of adverse and favorable prognosis (TCGA data). All the genes have passed the following thresholds: DESeq p-value < 0.01, Mann–Whitney p-value < 0.05, average expression-level fold change > 25%. Dot size indicates k/n ratio (“gene ratio”), where k is the number of genes participating in the current KEGG pathway (within top-40, top-80, or other top lists), and n is the number of genes (also within top-40, top-80, or other top lists) annotated as participants of any KEGG pathway (these numbers are provided in the brackets in the bottom of the figure). Dot color indicates the enrichment test FDR (Fisher’s exact test).
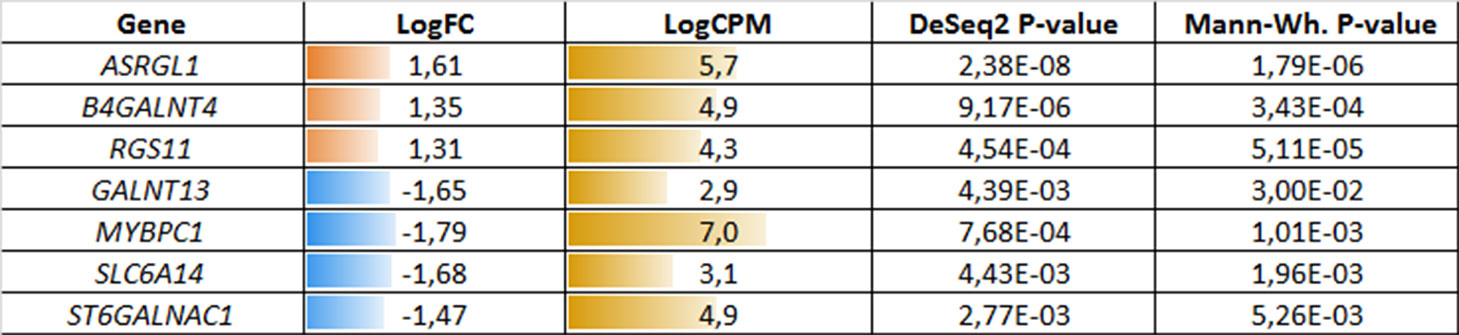
Figure 3 Potential markers of unfavorable prognosis in lymph node-negative locally advanced prostate cancer (LAPC) involved in the enriched KEGG pathways (TCGA data). LogFC—trimmed binary logarithm of average expression level fold change (between the groups of favorable and unfavorable prognosis); LogCPM—binary logarithm of read counts per million (CPM).
In a similar manner, we performed bioinformatic analysis for the TCGA sample groups characterized by the expression of the fusion transcript TMPRSS2-ERG. Comparative analysis between the groups with favorable and unfavorable prognosis revealed 127 DEGs (Supplementary Table S3). We identified 61 genes that were characterized by Mann–Whitney p < 0.05 (Figure 4). The KEGG pathway analysis revealed several over-represented ones; these are present in Figure 5 and Supplementary Table S6. Figure 6 represents the genes involved in the enriched KEGG pathways that can be potential marker in lymph node-negative LAPC TMPRSS2-ERG subtype.
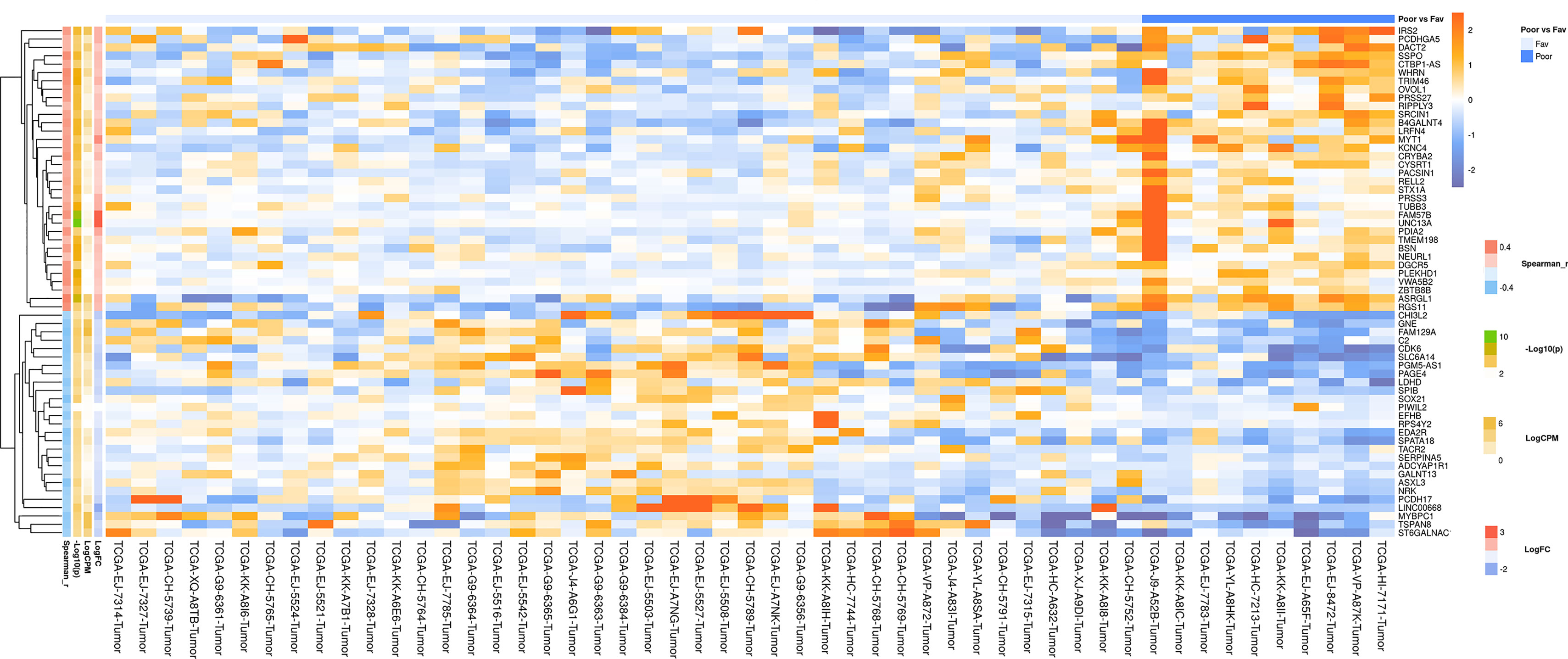
Figure 4 Heatmap demonstrating log relative expression level of top differentially expressed genes between the groups of adverse and favorable prognosis (TCGA data; TMPRSS2-ERG-positive samples). Cell colors (blue-white-orange gradient) correspond the binary logarithm of the ratio of the expression level in a current sample to the average level across all the samples (per each gene). Blue - expression level is below the average, orange - above the average. Spearman_r—Spearman’s rank correlation coefficient. -Log10(p) is the negative logarithm of DESeq’s p-value. LogCPM—average binary logarithm of read counts per million (CPM). LogFC—binary logarithm of expression-level fold change (sample with adverse prognosis compared to favorable prognosis).
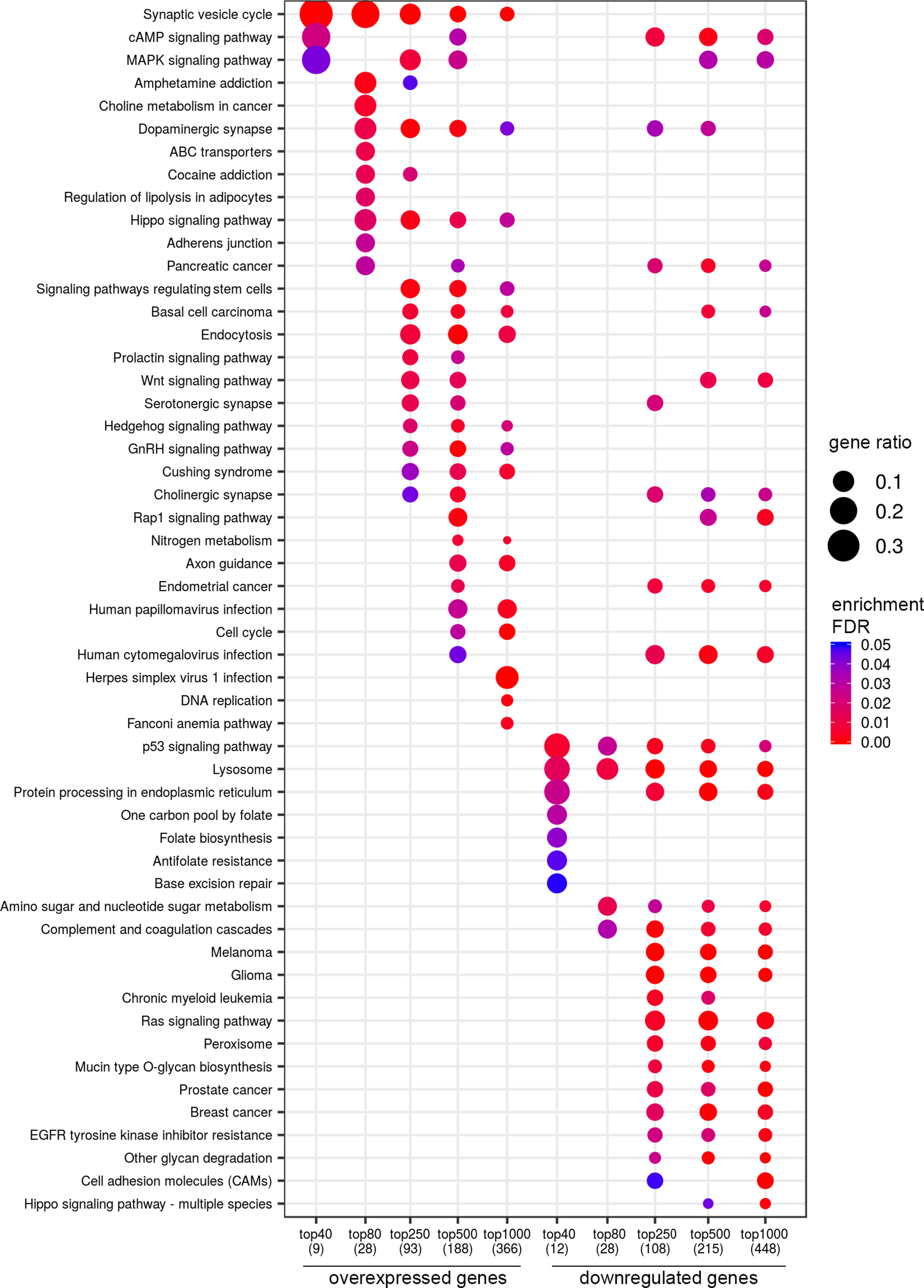
Figure 5 Dotplot showing the results of KEGG pathways enrichment analyses performed for top-40, 80, 250, 500, and 1,000 differentially expressed genes (either overexpressed or downregulated) between the groups of adverse and favorable prognosis (TCGA data; TMPRSS2-ERG-positive samples). All the genes have passed the following thresholds: DESeq p-value < 0.01, Mann–Whitney p-value < 0.05, average expression-level fold change > 25%. Dot size indicates k/n ratio (“gene ratio”), where k is the number of genes participating in the current KEGG pathway (within top-40, top-80, or other top lists), and n is the number of genes (also within top-40, top-80, or other top lists) annotated as participants of any KEGG pathway (these numbers are provided in the brackets in the bottom of the figure). Dot color indicates the enrichment test FDR (Fisher’s exact test).

Figure 6 Potential markers of unfavorable prognosis in lymph node-negative LAPC involved in the enriched KEGG pathways (TCGA data, TMPRSS2-ERG-positive samples). LogFC—trimmed binary logarithm of average expression-level fold change (between the groups of favorable and unfavorable prognosis); LogCPM—binary logarithm of CPM.
Bioinformatic Analysis of the Russian Patient Cohort
According to the results of bioinformatic analysis of lymph node-negative LAPC samples from the Russian patient cohort, we identified 63 DEGs with the expression-level changes of 2-fold or greater between the prognostic groups studied that are passed DESeq2 threshold (Supplementary Table S4). We then used Mann–Whitney test and revealed six potential markers of unfavorable prognosis (Figure 7). We performed KEGG pathway enrichment analysis for 81 DEGs identified (Figure 8, Supplementary Table S7). Several enriched KEGG pathways were identified: phospholipase D signaling pathway, GnRH signaling pathway, cell adhesion molecules (CAMs), allograft rejection, and some metabolic pathways (including sphingolipid metabolism) were found to be correlated with the tumor prognosis. These pathways included two genes (AKR1C1 and AKR1C3) with Mann–Whitney p < 0.05 (Figure 9).
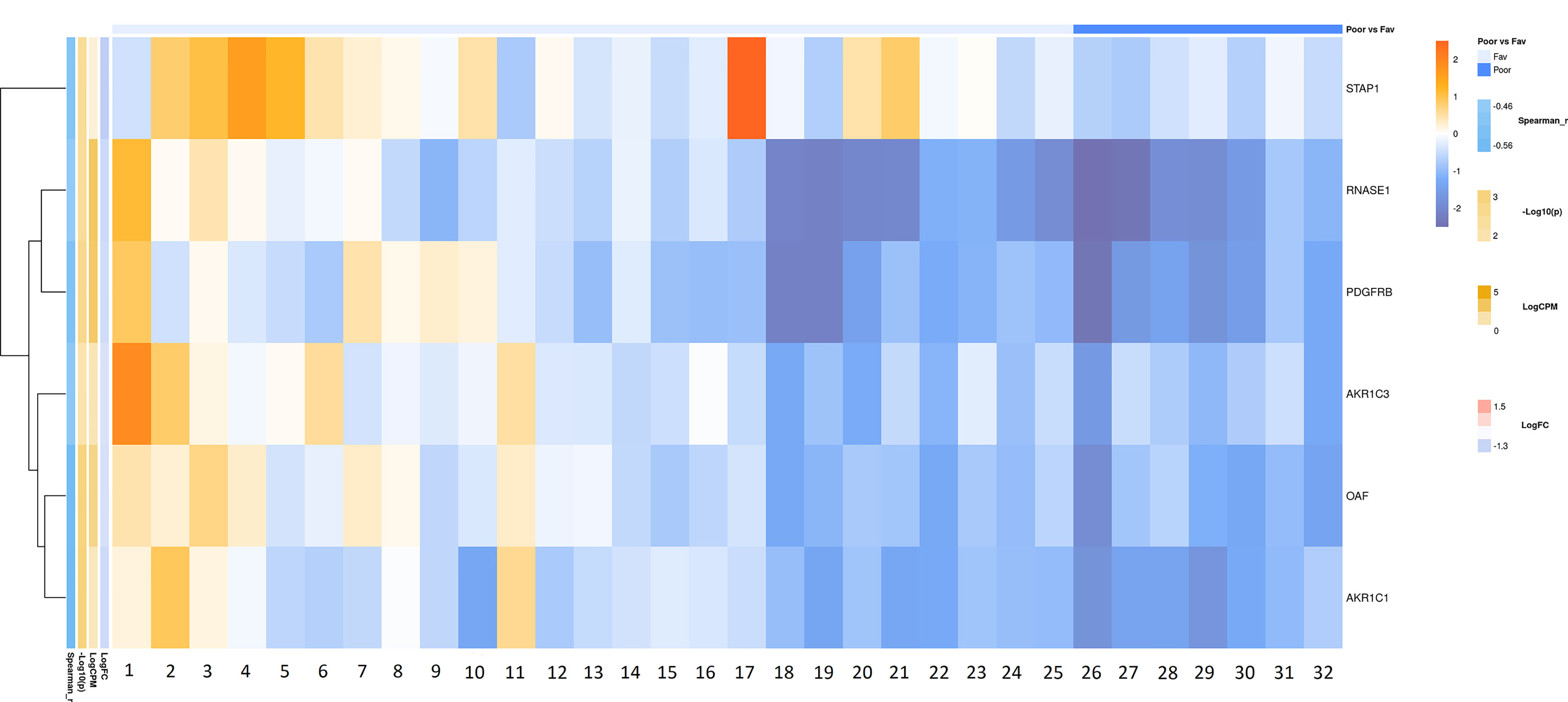
Figure 7 Heatmap demonstrating log relative expression level of top differentially expressed genes between the groups of adverse and favorable prognosis (Russian patient cohort). Cell colors (blue-white-orange gradient) correspond the binary logarithm of the ratio of the expression level in a current sample to the average level across all the samples (per each gene). Blue - expression level is below the average, orange - above the average. Spearman_r—Spearman’s rank correlation coefficient. -Log10(p) is the negative logarithm of DESeq’s p-value. LogCPM—average binary logarithm of read counts per million (CPM). LogFC—binary logarithm of expression-level fold change (sample with adverse prognosis compared to favorable prognosis).
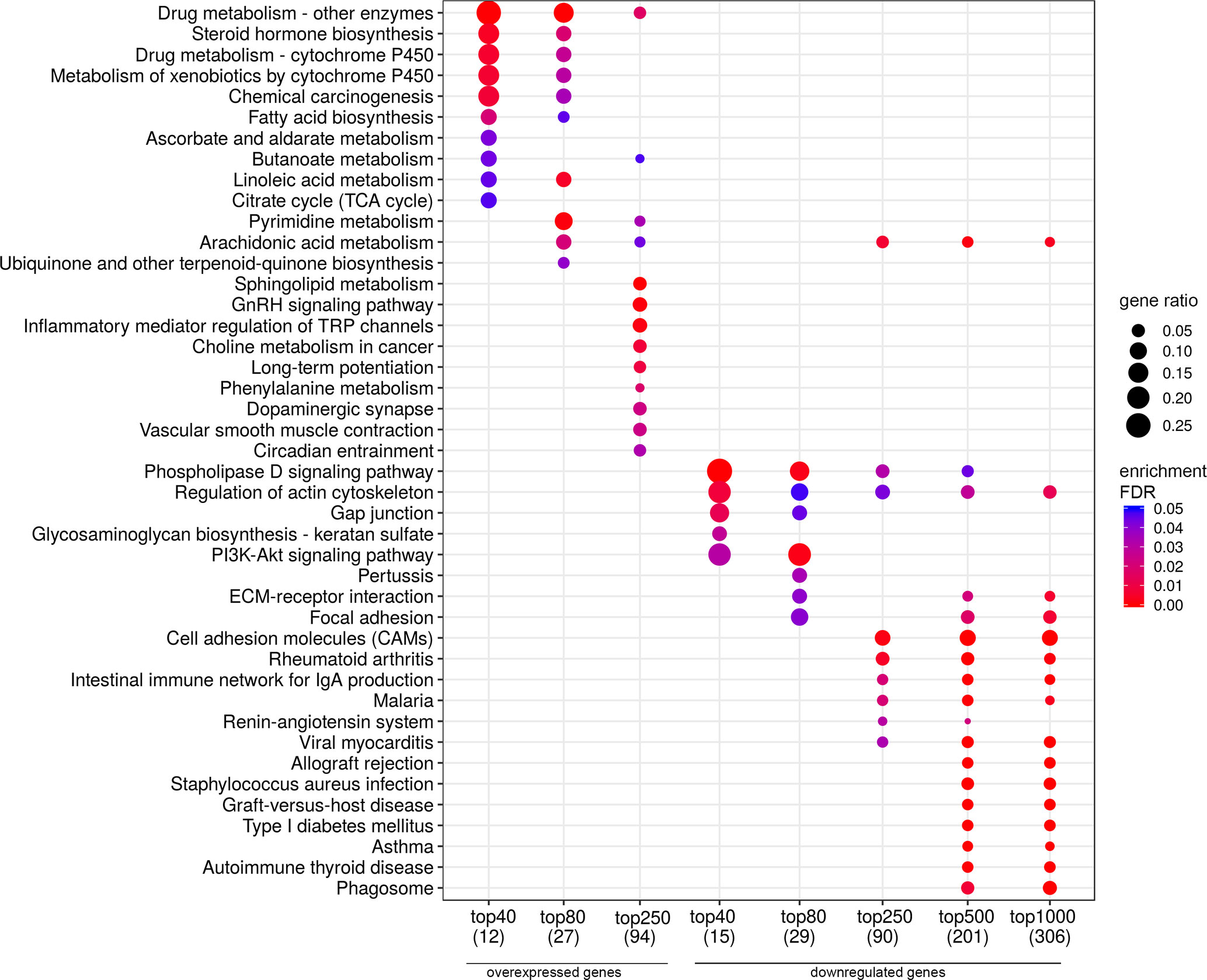
Figure 8 Dotplot showing the results of KEGG pathways enrichment analyses performed for top-40, 80, 250, 500, and 1,000 differentially expressed genes (either overexpressed or downregulated) between the groups of adverse and favorable prognosis (Russian patient cohort). All the genes have passed the following thresholds: DESeq p-value < 0.01, Mann–Whitney p-value < 0.05, average expression-level fold change > 25%. Dot size indicates k/n ratio (“gene ratio”), where k is the number of genes participating in the current KEGG pathway (within top-40, top-80 or other top lists), and n is the number of genes (also within top-40, top-80, or other top lists) annotated as participants of any KEGG pathway (these numbers are provided in the brackets in the bottom of the figure). Dot color indicates the enrichment test FDR (Fisher’s exact test).

Figure 9 Potential markers of unfavorable prognosis in lymph node-negative LAPC involved in the enriched KEGG pathways (Russian patient cohort). LogFC—trimmed binary logarithm of average expression-level fold change (between the groups of favorable and unfavorable prognosis); LogCPM—binary logarithm of read counts per million (CPM).
Discussion
Prostate cancer, an age-dependent disease, is caused by alterations in various molecular genetic mechanisms, including mutations and epigenetic changes followed by perturbations in cell signaling and metabolic pathways. Genes, which participate in these pathways, can serve as either diagnostic or prognostic biomarkers.
Our study describes the results of transcriptome profiling of locally advanced lymph node-negative prostate cancer. We have identified several genes that are potentially associated with the unfavorable prognosis of the tumors. The study can be divided into four parts. We first analyzed RNA-Seq data of lymph node-negative LAPC patients from both the TCGA and Russian cohort. Then, we separately studied the tumors of most common PC molecular subtype carrying fusion transcript TMPRSS2-ERG across the patients grouped by favorable and unfavorable prognosis.
In the first part of the study, we examined the TCGA data and identified 60 genes that were differentially expressed between favorable and unfavorable prognosis groups (with Mann–Whitney p < 0.05) and could be potential prognostic markers. Three genes (B4GALNT4, PTK6, and CHAT) were involved in the enriched KEGG pathways.
B4GALNT4 encodes for a beta-1,4-N-acetyl-galactosaminyltransferase 4. It was found to be upregulated in prostate, breast, and lung cancers (Fukushima et al., 2010; Potapenko et al., 2010; Zhang et al., 2017). The encoded protein is involved in the glycan metabolism and seems to be responsible for beta-N-acetylgalactosaminylation of a prostate-specific antigen (PSA) during prostate carcinogenesis (Fukushima et al., 2010).
PTK6 encodes for a cytoplasmic nonreceptor tyrosine kinase that may act as an intracellular signal transducer in epithelial tissues. Overexpression of PTK6 in mammary epithelial cells leads to sensitization of the cells to epidermal growth factor and results in a partially transformed phenotype (GeneCards). PTK6 is highly expressed in multiple tumor types, including prostate, ovarian, and breast cancers, and participates in the regulation of tumor cell proliferation, migration, and survival (Ito et al., 2017). The encoded protein represents a prominent therapeutic target (Park et al., 2015). In prostate cancer, PTK6 contributes to increased phosphorylation and activation of its substrates, such as AKT serine/threonine kinase 1 (AKT), CAS scaffolding protein family member 1 (p130CAS), and focal adhesion kinase (FAK), thereby promoting the tumor progression (Zheng and Tyner, 2013).
CHAT gene encodes for choline O-acetyltransferase catalyzing the biosynthesis of the neurotransmitter acetylcholine. Acetylcholine is the main mediator in the parasympathetic nervous system providing neuromuscular transmission. It was shown that in the prostate epithelium, CHAT participates in autocrine cholinergic signaling potentially involved in prostate cancer invasion and metastasis (Wang et al., 2015).
The second part of our results refers to lymph node-negative LAPC samples obtained from the Russian patients. Totally, we identified 12 DEGs, potential prognostic markers (passed Mann–Whitney p < 0.05 threshold). Two genes from them were involved in the enriched KEGG pathways, the steroid hormone biosynthesis, and arachidonic acid metabolism. As most primary prostate cancers are strongly hormone-dependent, the steroid hormone (including testosterone) biosynthesis pathway plays a pivotal role in the tumor growth, particularly, when androgens are actively synthesized within tumor cells (Nelson et al., 2002; Imamoto et al., 2008; van der Sluis et al., 2012). We have identified two DEGs, AKR1C1 and AKR1C3, encoding for aldo-keto reductase family 1 member C1 and aldo-keto reductase family 1 member C3, respectively. These enzymes participate in the steroid hormone biosynthesis and were shown to be highly expressed in different cancer types (Lewis et al., 2004; Sayagues et al., 2016).
AKR1C1 converts progesterone into its inactive form, a 20-alpha-dihydroxyprogesterone (Nishizawa et al., 2000). Overexpression of AKR1C1 is associated with cancer progression; however, the mechanism, by which AKR1C1 promotes metastasis, has not been understood (Ji et al., 2004; Chien et al.; 2009). Several studies suggest a critical role for the canonical function of AKR1C1 in the aggressiveness of hormone-related cancers, which is especially interesting in case of prostate cancer (Ji et al., 2004; Rizner et al., 2006).
AKR1C3, which is also involved in arachidonic acid metabolism, plays a role in the progression of prostate cancer and its resistance to hormone therapy (Adeniji et al., 2013; Chen et al., 2015; Powell et al., 2015). Elevated expression of AKR1C3 is associated with the progression and aggressiveness of prostate cancer (Stanbrough et al., 2006; Wako et al., 2008).
The third part of our analysis was focused on the identification of prognostic markers in the TMPRSS2-ERG PC molecular subtype based on the TCGA data. The bioinformatic analysis revealed 74 DEGs between favorable and unfavorable prognosis groups with Mann–Whitney p < 0.05. Among them, several genes were involved in the enriched KEGG pathways: ASRGL1, MYBPC1, RGS11, SLC6A14, GALNT13, ST6GALNAC1, and B4GALNT4.
ASRGL1 gene encodes for asparaginase–like protein 1 probably belonging to the β-aspartyl peptidase family (Cantor et al., 2009). ASRGL1 catalyzes the hydrolysis of L-asparagine and β-aspartyl dipeptides. In the recent study based on the TCGA data, elevated expression of ASRGL1 gene was found to be associated with biochemical recurrence of prostate cancer (Chu et al., 2018). On the contrary, decreased expression of ASRGL1 was significantly associated with poor prognosis and reduced disease-specific survival in endometrioid endometrial adenocarcinoma (Edqvist et al., 2015).
MYBPC1 gene encodes for a member of the myosin-binding protein C family that plays an important role in muscle contraction by recruiting muscle-type creatine kinase to myosin filaments. Increased expression of MYBPC1 gene was observed in breast cancer (Hu et al., 2014). It is assumed that this gene can take part in the progesterone signaling that is associated with the progression of hormone-associated cancers (Ji et al., 2004).
Regarding the other DEGs, there are many already known cancer-associated ones. Overexpression of RGS11 gene, encoding for a regulator of G protein signaling 11, promotes cell migration and is associated with advanced stages and aggressiveness of lung adenocarcinoma (Yang et al., 2016). Amino acid transporter, SLC6A14, might represent a possible therapeutic target for pancreatic cancer, and it was found to be upregulated in patient-derived xenografts and primary pancreatic tumors, as well as colorectal cancer (Gupta et al., 2005; Coothankandaswamy et al., 2016).
ST6GALNAC1 gene encodes for sialyltransferase, which is involved in mucin-type O-glycan biosynthesis. Mucin-type O-glycan is of special interest in the context of carcinogenesis since ectopic expression of mucin-type glycoproteins is a hallmark of epithelial tumors. Different alterations in mucin-type O-glycan pathways are associated with unfavorable prognosis in various tumor types (Byrd and Bresalier, 2004). ST6GALNAC1 gene is directly activated by androgens and is frequently overexpressed in prostate cancer (Munkley et al., 2015; Munkley et al., 2016). The encoded protein catalyzes the synthesis of the cancer-associated sialyl-Tn antigen (sTn), which is present in a variety of carcinomas and is associated with metastasis and unfavorable prognosis (Pinho et al., 2007). High expression of sTn has been detected in up to half of the high-grade prostate tumors (Myers et al., 1994). It was also found that the expression of ST6GALNAC1 in prostate cancer cells changes global gene expression profiles toward mesenchymal-like pattern increasing cell mobility and decreasing cell adhesion (Wei et al., 2008). The results of these studies confirm the potential prognostic significance of ST6GALNAC1 gene for prostate cancer.
The GALNT13 gene encodes for the polypeptide N-acetylgalactosaminyltransferase 13, which is a member of the GalNAcT family involved in the initiation of O-linked glycosylation of mucins. This gene is expressed in most primary and metastatic epithelial malignant tumors (Wang et al., 1997).
B4GALNT4 gene was also found to be differentially expressed between favorable and unfavorable prognosis groups in the whole set of LAPC samples from TCGA data (first part of the study). This gene demonstrates great potential prognostic value.
As the results of this study, we identified several genes that can represent potential prognostic markers for LAPC. These genes are participants of several processes associated with the development of various types of cancer; however, none of these genes has an established association with the prognosis of prostate cancer. The results of our research could be used as a basis for the test system development of improving the prognosis, treatment strategy, and management of prostate cancer patients.
Ethics Statement
The study was approved by The Ethics committee of P.A. Hertzen Moscow Oncology Research Center, Ministry of Health of the Russian Federation. The study was done in accordance with the principles outlined in the Declaration of Helsinki (1964). All patients gave their informed consent for participation in the study.
Author Contributions
EP, EL, ADK, KN, NV, GE, BA, and AVK conceived and designed the work; EP, AB, KK, AS, DM, and AZ performed the experiments; EL, MK, GK, AAK, and MS analyzed the data; EP, EL, AS, NM, and AVK wrote the manuscript. All authors read and approved the final manuscript.
Funding
This work and publication costs were funded by the Russian Science Foundation, grant 18-75-10127.
Conflict of Interest Statement
The authors declare that the research was conducted in the absence of any commercial or financial relationships that could be construed as a potential conflict of interest.
Acknowledgments
Authors thank the National Medical Research Radiological Center for supplying samples, Sechenov First Moscow State Medical University for fusion transcript TMPRSS2-ERG identification and Vavilov Institute of General Genetics for the provided computational resources. This work was performed using the equipment of EIMB RAS “Genome” center (http://www.eimb.ru/rus/ckp/ccu_genome_c.php).
Supplementary Material
The Supplementary Material for this article can be found online at: https://www.frontiersin.org/articles/10.3389/fgene.2019.00730/full#supplementary-material
References
Adeniji, A. O., Chen, M., Penning, T. M. (2013). AKR1C3 as a target in castrate resistant prostate cancer. J. Steroid. Biochem. Mol. Biol. 137, 136–149. doi: 10.1016/j.jsbmb.2013.05.012
Belova, A. A., Sosnovtseva, A. O., Lipatova, A. V., Njushko, K. M., Volchenko, N. N., Belyakov, M. M., et al. (2017). Biomarkers of Prostate Cancer Sensitivity to the Sendai Virus. Mol. Biol. 51 (1), 80–88. doi: 10.1134/S0026893317010046
Bray, N. L., Pimentel, H., Melsted, P., Pachter, L. (2016). Near-optimal probabilistic RNA-seq quantification. Nat. Biotechnol. 34 (5), 525–527. doi: 10.1038/nbt.3519
Byrd, J. C., Bresalier, R. S. (2004). Mucins and mucin binding proteins in colorectal cancer. Cancer. Metastasis. Rev. 23 (1-2), 77–99. doi: 10.1023/A:1025815113599
Cantor, J. R., Stone, E. M., Chantranupong, L., Georgiou, G. (2009). The human asparaginase-like protein 1 hASRGL1 is an Ntn hydrolase with beta-aspartyl peptidase activity. Biochemistry 48 (46), 11026–11031. doi: 10.1021/bi901397h
Chen, E. J., Sowalsky, A. G., Gao, S., Cai, C., Voznesensky, O., Schaefer, R., et al. (2015). Abiraterone treatment in castration-resistant prostate cancer selects for progesterone responsive mutant androgen receptors. Clin. Cancer Res. 21 (6), 1273–1280. doi: 10.1158/1078-0432.CCR-14-1220
Cherkasova, E., Malinzak, E., Rao, S., Takahashi, Y., Senchenko, V. N., Kudryavtseva, A. V., et al. (2011). Inactivation of the von Hippel-Lindau tumor suppressor leads to selective expression of a human endogenous retrovirus in kidney cancer. Oncogene 30 (47), 4697–4706. doi: 10.1038/onc.2011.179
Chien, C. W., Ho, I. C., Lee, T. C. (2009). Induction of neoplastic transformation by ectopic expression of human aldo-keto reductase 1C isoforms in NIH3T3 cells. Carcinogenesis 30 (10), 1813–1820. doi: 10.1093/carcin/bgp195
Chu, J., Li, N., Gai, W. (2018). Identification of genes that predict the biochemical recurrence of prostate cancer. Oncol. Lett. 16 (3), 3447–3452. doi: 10.3892/ol.2018.9106
Coothankandaswamy, V., Cao, S., Xu, Y., Prasad, P. D., Singh, P. K., Reynolds, C. P., et al. (2016). Amino acid transporter SLC6A14 is a novel and effective drug target for pancreatic cancer. Br. J. Pharmacol. 173 (23), 3292–3306. doi: 10.1111/bph.13616
Dmitriev, A. A., Rosenberg, E. E., Krasnov, G. S., Gerashchenko, G. V., Gordiyuk, V. V., Pavlova, T. V., et al. (2015). Identification of Novel Epigenetic Markers of Prostate Cancer by NotI-Microarray Analysis. Dis. Markers 2015, 241301. doi: 10.1155/2015/241301
Dmitriev, A. A., Rudenko, E. E., Kudryavtseva, A. V., Krasnov, G. S., Gordiyuk, V. V., Melnikova, N. V., et al. (2014). Epigenetic alterations of chromosome 3 revealed by NotI-microarrays in clear cell renal cell carcinoma. Biomed. Res. Int. 2014, 735292. doi: 10.1155/2014/735292
Edqvist, P. H., Huvila, J., Forsstrom, B., Talve, L., Carpen, O., Salvesen, H. B., et al. (2015). Loss of ASRGL1 expression is an independent biomarker for disease-specific survival in endometrioid endometrial carcinoma. Gynecol. Oncol. 137 (3), 529–537. doi: 10.1016/j.ygyno.2015.03.055
Fedorova, M. S., Kudryavtseva, A. V., Lakunina, V. A., Snezhkina, A. V., Volchenko, N. N., Slavnova, E. N., et al. (2015). Downregulation of OGDHL expression is associated with promoter hypermethylation in colorectal cancer. Mol. Biol. 49 (4), 608–617. doi: 10.1134/S0026893315040044
Fedorova, M. S., Snezhkina, A. V., Pudova, E. A., Abramov, I. S., Lipatova, A. V., Kharitonov, S. L., et al. (2017). Upregulation of NETO2 gene in colorectal cancer. BMC Genet. 2818 (Suppl 1), 117. doi: 10.1186/s12863-017-0581-8
Fukushima, K., Satoh, T., Baba, S., Yamashita, K. (2010). alpha1,2-Fucosylated and beta-N-acetylgalactosaminylated prostate-specific antigen as an efficient marker of prostatic cancer. Glycobiology 20 (4), 452–460. doi: 10.1093/glycob/cwp197
Gupta, N., Miyauchi, S., Martindale, R. G., Herdman, A. V., Podolsky, R., Miyake, K., et al. (2005). Upregulation of the amino acid transporter ATB0,+ (SLC6A14) in colorectal cancer and metastasis in humans. Biochim. Biophys. Acta. 1741 (1-2), 215–223. doi: 10.1016/j.bbadis.2005.04.002
Hu, H., Wang, J., Gupta, A., Shidfar, A., Branstetter, D., Lee, O., et al. (2014). RANKL expression in normal and malignant breast tissue responds to progesterone and is up-regulated during the luteal phase. Breast Cancer Res. Treat. 146 (3), 515–523. doi: 10.1007/s10549-014-3049-9
Imamoto, T., Suzuki, H., Yano, M., Kawamura, K., Kamiya, N., Araki, K., et al. (2008). The role of testosterone in the pathogenesis of prostate cancer. Int. J. Urol. 15 (6), 472–480. doi: 10.1111/j.1442-2042.2008.02074.x
Ito, K., Park, S. H., Katsyv, I., Zhang, W., De Angelis, C., Schiff, R., et al. (2017). PTK6 regulates growth and survival of endocrine therapy-resistant ER+ breast cancer cells. NPJ Breast Cancer 3, 45. doi: 10.1038/s41523-017-0047-1
Jhun, M. A., Geybels, M. S., Wright, J. L., Kolb, S., April, C., Bibikova, M., et al. (2017). Gene expression signature of Gleason score is associated with prostate cancer outcomes in a radical prostatectomy cohort. Oncotarget 8 (26), 43035–43047. doi: 10.18632/oncotarget.17428
Ji, Q., Aoyama, C., Nien, Y. D., Liu, P. I., Chen, P. K., Chang, L., et al. (2004). Selective loss of AKR1C1 and AKR1C2 in breast cancer and their potential effect on progesterone signaling. Cancer Res. 64 (20), 7610–7617. doi: 10.1158/0008-5472.CAN-04-1608
Rizner, T. L., Smuc, T., Rupreht, R., Sinkovec, J., Penning, T. M. (2006). AKR1C1 and AKR1C3 may determine progesterone and estrogen ratios in endometrial cancer. Mol. Cell. Endocrinol. 248 (1–2), 126–135. doi: 10.1016/j.mce.2005.10.009
Knyazev, E. N., Fomicheva, K. A., Mikhailenko, D. S., Nyushko, K. M., Samatov, T. R., Alekseev, B. Y., et al. (2016). Plasma Levels of hsa-miR-619-5p and hsa-miR-1184 Differ in Prostatic Benign Hyperplasia and Cancer. Bull. Exp. Biol. Med. 161 (1), 108–111. doi: 10.1007/s10517-016-3357-7
Krasnov, G. S., Dmitriev, A. A., Sadritdinova, A. F., Volchenko, N. N., Slavnova, E. N., Danilova, T. V., et al. (2015). Molecular genetic mechanisms of drug resistance in prostate cancer. Mol. Biol. 49 (5), 638–648. doi: 10.1134/S0026893315050118
Krasnov, G. S., Puzanov, G. A., Kudryavtseva, A. V., Dmitriev, A. A., Beniaminov, A. D., Kondratieva, T. T., et al. (2017). Differential expression of an ensemble of the key genes involved in cell-cycle regulation in lung cancer. Mol. Biol. 51 (5), 740–747. doi: 10.1134/S0026893317050107
Kudryavtseva, A. V., Krasnov, G. S., Dmitriev, A. A., Alekseev, B. Y., Kardymon, O. L., Sadritdinova, A. F., et al. (2016). Mitochondrial dysfunction and oxidative stress in aging and cancer. Oncotarget 7 (29), 44879–44905. doi: 10.18632/oncotarget.9821
Kudryavtseva, A. V., Lukyanova, E. N., Kharitonov, S. L., Nyushko, K. M., Krasheninnikov, A. A., Pudova, E. A., et al. (2019). Bioinformatic identification of differentially expressed genes associated with prognosis of locally advanced lymph node-positive prostate cancer. J. Bioinform. Comput. Biol. 17 (1), 1950003. doi: 10.1142/S0219720019500033
Kudryavtseva, A. V., Nyushko, K. M., Zaretsky, A. R., Shagin, D. A., Sadritdinova, A. F., Fedorova, M. S., et al. (2018). Suppression of NR0B2 gene in clear cell renal cell carcinoma is associated with hypermethylation of its promoter. Mol. Biol. 52 (3), 414–418. doi: 10.1134/S0026893318030081
Lewis, M. J., Wiebe, J. P., Heathcote, J. G. (2004). Expression of progesterone metabolizing enzyme genes (AKR1C1, AKR1C2, AKR1C3, SRD5A1, SRD5A2) is altered in human breast carcinoma. BMC Cancer 4, 27. doi: 10.1186/1471-2407-4-27
Love, M. I., Huber, W., Anders, S. (2014). Moderated estimation of fold change and dispersion for RNA-seq data with DESeq2. Genome. Biol. 15 (12), 550. doi: 10.1186/s13059-014-0550-8
Moch, H., Humphrey, P. A., Ulbright, T. M., Reuter, V. E. (2016). WHO classification of tumours of the urinary system and male genital organs. Fourth ed., WHO Press.
Munkley, J., Oltean, S., Vodak, D., Wilson, B. T., Livermore, K. E., Zhou, Y., et al. (2015). The androgen receptor controls expression of the cancer-associated sTn antigen and cell adhesion through induction of ST6GalNAc1 in prostate cancer. Oncotarget 6 (33), 34358-34374. doi: 10.18632/oncotarget.6024
Munkley, J., Vodak, D., Livermore, K. E., James, K., Wilson, B. T., Knight, B., et al. (2016). Glycosylation is an androgen-regulated process essential for prostate cancer cell viability. E. Bio. Med. 8, 103–116. doi: 10.1016/j.ebiom.2016.04.018
Myers, R. B., Meredith, R. F., Schlom, J., LoBuglio, A. F., Bueschen, A. J., Wheeler, R. H., et al. (1994). Tumor associated glycoprotein-72 is highly expressed in prostatic adenocarcinomas. J. Urol. 152 (1), 243–246. doi: 10.1016/S0022-5347(17)32870-7
Nelson, P. S., Clegg, N., Arnold, H., Ferguson, C., Bonham, M., White, J., et al. (2002). The program of androgen-responsive genes in neoplastic prostate epithelium. Proc. Natl. Acad. Sci. U. S. A. 99 (18), 11890–11895. doi: 10.1073/pnas.182376299
Nishizawa, M., Nakajima, T., Yasuda, K., Kanzaki, H., Sasaguri, Y., Watanabe, K., et al. (2000). Close kinship of human 20alpha-hydroxysteroid dehydrogenase gene with three aldo-keto reductase genes. Genes. Cells 5 (2), 111–125. doi: 10.1046/j.1365-2443.2000.00310.x
Oparina, N.Yu., Snezhkina, A. V., Sadritdinova, A. F., Veselovskii, V. A., Dmitriev, A. A., Senchenko, V. N., et al. (2013). Differential expression of genes that encode glycolysis enzymes in kidney and lung cancer in humans. Russ. J. Genet. 49 (7), 707–716. doi: 10.1134/S1022795413050104
Park, S. H., Ito, K., Olcott, W., Katsyv, I., Halstead-Nussloch, G., Irie, H. Y. (2015). PTK6 inhibition promotes apoptosis of Lapatinib-resistant Her2(+) breast cancer cells by inducing Bim. Breast Cancer Res. 17, 86. doi: 10.1186/s13058-015-0594-z
Pinho, S., Marcos, N. T., Ferreira, B., Carvalho, A. S., Oliveira, M. J., Santos-Silva, F., et al. (2007). Biological significance of cancer-associated sialyl-Tn antigen: modulation of malignant phenotype in gastric carcinoma cells. Cancer Lett. 249 (2), 157–170. doi: 10.1016/j.canlet.2006.08.010
Plati, J., Bucur, O., Khosravi-Far, R. (2011). Apoptotic cell signaling in cancer progression and therapy. Integr. Biol. (Camb.) 3 (4), 279–296. doi: 10.1039/c0ib00144a
Potapenko, I. O., Haakensen, V. D., Luders, T., Helland, A., Bukholm, I., Sorlie, T., et al. (2010). Glycan gene expression signatures in normal and malignant breast tissue; possible role in diagnosis and progression. Mol. Oncol. 4 (2), 98–118. doi: 10.1016/j.molonc.2009.12.001
Powell, K., Semaan, L., Conley-LaComb, M. K., Asangani, I., Wu, Y. M., Ginsburg, K. B., et al. (2015). ERG/AKR1C3/AR constitutes a feed-forward loop for ar signaling in prostate cancer cells. Clin. Cancer. Res. 21 (11), 2569–2579. doi: 10.1158/1078-0432.CCR-14-2352
Pudova, E. A., Kudryavtseva, A. V., Fedorova, M. S., Zaretsky, A. R., Shcherbo, D. S., Lukyanova, E. N., et al. (2018). HK3 overexpression associated with epithelial-mesenchymal transition in colorectal cancer. BMC Genomics 919 (Suppl 3), 113. doi: 10.1186/s12864-018-4477-4
Sayagues, J. M., Corchete, L. A., Gutierrez, M. L., Sarasquete, M. E., Del Mar Abad, M., Bengoechea, O., et al. (2016). Genomic characterization of liver metastases from colorectal cancer patients. Oncotarget 7 (45), 72908–72922. doi: 10.18632/oncotarget.12140
Snezhkina, A. V., Krasnov, G. S., Lipatova, A. V., Sadritdinova, A. F., Kardymon, O. L., Fedorova, M. S., et al. (2016a). The dysregulation of polyamine metabolism in colorectal cancer is associated with overexpression of c-myc and c/ebpβ rather than enterotoxigenic bacteroides fragilis infection. Oxid. Med. Cell Longev. 2016, 2353560. doi: 10.1155/2016/2353560
Snezhkina, A. V., Krasnov, G. S., Zaretsky, A. R., Zhavoronkov, A., Nyushko, K. M., Moskalev, A. A., et al. (2016b). Differential expression of alternatively spliced transcripts related to energy metabolism in colorectal cancer. BMC Genomics 17 (Suppl 14), 1011. doi: 10.1186/s12864-016-3351-5
Snezhkina, A. V., Krasnov, G. S., Zhikrivetskaya, S. O., Karpova, I. Y., Fedorova, M. S., Nyushko, K. M., et al. (2018b). Overexpression of microRNAs miR-9, -98, and -199 correlates with the downregulation of hk2 expression in colorectal cancer. Mol. Biol. 52 (2), 190–199. doi: 10.1134/S0026893318020140
Snezhkina, A. V., Nyushko, K. M., Zaretsky, A. R., Shagin, D. A., Sadritdinova, A. F., Fedorova, M. S., et al. (2018a). Transcription factor sap30 is involved in the activation of neto2 gene expression in clear cell renal cell carcinoma. Mol. Biol. 52 (3), 385–392. doi: 10.1134/S0026893318020152
Stanbrough, M., Bubley, G. J., Ross, K., Golub, T. R., Rubin, M. A., Penning, T. M., et al. (2006). Increased expression of genes converting adrenal androgens to testosterone in androgen-independent prostate cancer. Cancer Res. 66 (5), 2815–2825. doi: 10.1158/0008-5472.CAN-05-4000
The Cancer Genome Atlas Research Network (2015). The molecular taxonomy of primary prostate cancer. Cell 163 (4), 1011–1025. doi: 10.1016/j.cell.2015.10.025
van der Sluis, T. M., Vis, A. N., van Moorselaar, R. J., Bui, H. N., Blankenstein, M. A., Meuleman, E. J., et al. (2012). Intraprostatic testosterone and dihydrotestosterone. Part I: concentrations and methods of determination in men with benign prostatic hyperplasia and prostate cancer. BJU Int. 109 (2), 176–182. doi: 10.1111/j.1464-410X.2011.10651.x
Vinjamoori, A. H., Jagannathan, J. P., Shinagare, A. B., Taplin, M. E., Oh, W. K., Van den Abbeele, A. D., et al. (2012). Atypical metastases from prostate cancer: 10-year experience at a single institution. AJR Am. J. Roentgenol. 199 (2), 367–372. doi: 10.2214/AJR.11.7533
Wako, K., Kawasaki, T., Yamana, K., Suzuki, K., Jiang, S., Umezu, H., et al. (2008). Expression of androgen receptor through androgen-converting enzymes is associated with biological aggressiveness in prostate cancer. J. Clin. Pathol. 61 (4), 448–454. doi: 10.1136/jcp.2007.050906
Wang, B. L., Springer, G. F., Carlstedt, S. C. (1997). Quantitative computerized image analysis of Tn and T (Thomsen-Friedenreich) epitopes in prognostication of human breast carcinoma. J. Histochem. Cytochem. 45 (10), 1393–1400. doi: 10.1177/002215549704501007
Wang, N., Yao, M., Xu, J., Quan, Y., Zhang, K., Yang, R., et al. (2015). Autocrine activation of chrm3 promotes prostate cancer growth and castration resistance via cam/camkk-mediated phosphorylation of akt. Clin. Cancer Res. 21 (20), 4676–4685. doi: 10.1158/1078-0432.CCR-14-3163
Wei, J., Xu, G., Wu, M., Zhang, Y., Li, Q., Liu, P., et al. (2008). Overexpression of vimentin contributes to prostate cancer invasion and metastasis via src regulation. Anticancer. Res. 28 (1A), 327–334.
Yang, S. H., Li, C. F., Chu, P. Y., Ko, H. H., Chen, L. T., Chen, W. W., et al. (2016). Overexpression of regulator of G protein signaling 11 promotes cell migration and associates with advanced stages and aggressiveness of lung adenocarcinoma. Oncotarget 7 (21), 31122–31136. doi: 10.18632/oncotarget.8860
Yu, G., Wang, L. G., Han, Y., He, Q. Y. (2012). clusterProfiler: an R package for comparing biological themes among gene clusters. OMICS 16 (5), 284–287. doi: 10.1089/omi.2011.0118
Zhang, F., Chen, X., Wei, K., Liu, D., Xu, X., Zhang, X., et al. (2017). Identification of key transcription factors associated with lung squamous cell carcinoma. Med. Sci. Monit. 23, 172–206. doi: 10.12659/MSM.898297
Keywords: locally advanced prostate cancer, prognostic markers, TCGA, RNA-Seq, TMPRSS2-ERG
Citation: Pudova EA, Lukyanova EN, Nyushko KM, Mikhaylenko DS, Zaretsky AR, Snezhkina AV, Savvateeva MV, Kobelyatskaya AA, Melnikova NV, Volchenko NN, Efremov GD, Klimina KM, Belova AA, Kiseleva MV, Kaprin AD, Alekseev BY, Krasnov GS and Kudryavtseva AV (2019) Differentially Expressed Genes Associated With Prognosis in Locally Advanced Lymph Node-Negative Prostate Cancer. Front. Genet. 10:730. doi: 10.3389/fgene.2019.00730
Received: 03 August 2018; Accepted: 11 July 2019;
Published: 09 August 2019.
Edited by:
Elena G. Pasyukova, Institute of Molecular Genetics (RAS), RussiaReviewed by:
Nick Barlev, Institute of Cytology (RAS), RussiaNeeraj Agarwal, Cedars-Sinai Medical Center, United States
Copyright © 2019 Pudova, Lukyanova, Nyushko, Mikhaylenko, Zaretsky, Snezhkina, Savvateeva, Kobelyatskaya, Melnikova, Volchenko, Efremov, Klimina, Belova, Kiseleva, Kaprin, Alekseev, Krasnov and Kudryavtseva. This is an open-access article distributed under the terms of the Creative Commons Attribution License (CC BY). The use, distribution or reproduction in other forums is permitted, provided the original author(s) and the copyright owner(s) are credited and that the original publication in this journal is cited, in accordance with accepted academic practice. No use, distribution or reproduction is permitted which does not comply with these terms.
*Correspondence: Anna V. Kudryavtseva, rhizamoeba@mail.ru
†These authors have contributed equally to this work.