- 1Department of Biostatistics, Gillings School of Global Public Health, University of North Carolina at Chapel Hill, Chapel Hill, NC, United States
- 2Charles Bronfman Institute for Personalized Medicine, Icahn School of Medicine at Mount Sinai, New York, NY, United States
- 3Division of Nephrology, Department of Medicine, Icahn School of Medicine at Mount Sinai, New York, NY, United States
- 4Department of Biostatistics, Vanderbilt University Medical Center, Nashville, TN, United States
- 5Vanderbilt Genetics Institute, Vanderbilt University Medical Center, Nashville, TN, United States
- 6Department of Epidemiology, Gillings School of Global Public Health, University of North Carolina at Chapel Hill, Chapel Hill, NC, United States
- 7The University of Texas Health Science Center at Houston, Houston, TX, United States
- 8Department of Genetics, Rutgers University, Piscataway, NJ, United States
- 9Epidemiology Program, University of Hawaii Cancer Center, Honolulu, HI, United States
- 10Division of Public Health Sciences, Fred Hutchinson Cancer Research Center, Seattle, WA, United States
- 11Department of Epidemiology, University of Washington, Seattle, WA, United States
- 12Department of Preventive Medicine, University of Southern California, Los Angeles, CA, United States
- 13Information Sciences Institute, University of Southern California, Marina del Rey, CA, United States
- 14Department of Biostatistics, University of Liverpool, Liverpool, United Kingdom
- 15Wellcome Centre for Human Genetics, University of Oxford, Oxford, United Kingdom
- 16Mindich Child Health and Development Institute, Icahn School of Medicine at Mount Sinai, New York, NY, United States
- 17Applied Sciences, Premier, Inc., Charlotte, NC, United States
Background: Chronic kidney disease (CKD) is common and disproportionally burdens United States ethnic minorities. Its genetic determinants may differ by disease severity and clinical stages. To uncover genetic factors associated CKD severity among high-risk ethnic groups, we performed genome-wide association studies (GWAS) in diverse populations within the Population Architecture using Genomics and Epidemiology (PAGE) study.
Methods: We assembled multi-ethnic genome-wide imputed data on CKD non-overlapping cases [4,150 mild to moderate CKD, 1,105 end-stage kidney disease (ESKD)] and non-CKD controls for up to 41,041 PAGE participants (African Americans, Hispanics/Latinos, East Asian, Native Hawaiian, and American Indians). We implemented a generalized estimating equation approach for GWAS using ancestry combined data while adjusting for age, sex, principal components, study, and ethnicity.
Results: The GWAS identified a novel genome-wide associated locus for mild to moderate CKD nearby NMT2 (rs10906850, p = 3.7 × 10-8) that replicated in the United Kingdom Biobank white British (p = 0.008). Several variants at the APOL1 locus were associated with ESKD including the APOL1 G1 rs73885319 (p = 1.2 × 10-9). There was no overlap among associated loci for CKD and ESKD traits, even at the previously reported APOL1 locus (p = 0.76 for CKD). Several additional loci were associated with CKD or ESKD at p-values below the genome-wide threshold. These loci were often driven by variants more common in non-European ancestry.
Conclusion: Our genetic study identified a novel association at NMT2 for CKD and showed for the first time strong associations of the APOL1 variants with ESKD across multi-ethnic populations. Our findings suggest differences in genetic effects across CKD severity and provide information for study design of genetic studies of CKD in diverse populations.
Introduction
Chronic kidney disease (CKD) affects 15% of United States. adults and is a leading cause of death globally (Global Burden of Disease 2016 Causes of Death Collaborators, 2017). CKD is classified based on its causes, kidney function (estimated glomerular filtration rate, eGFR), and markers of kidney damage (Levin and Stevens, 2014). The risk of adverse outcomes and disability greatly increases in advanced CKD (Go et al., 2004; Saran et al., 2018). There is a high burden of CKD in non-European ancestry groups, including African Americans and Hispanics/Latinos (Collins et al., 2011). Genetic susceptibility explains in part ethnic differences in the burden of CKD, as illustrated by the African-ancestry APOL1 G1 and G2 genotypes that contribute to increased CKD risk in individuals with African ancestry (Genovese et al., 2010; Kramer et al., 2017). Approximately 13% of African Americans carry two APOL1 risk genotypes G1 (composed of two missense variants), G2 (a 6-base pair in-frame deletion) or are compound heterozygous of G1 and G2 genotypes. APOL1 encodes an HDL cholesterol-binding protein but mechanisms related to CKD risk are unknown.
Few genome-wide association studies (GWAS) have been published for CKD as the primary outcome. These include studies of CKD progression such as the Chronic Renal Insufficiency Cohort Study (Parsa et al., 2017), and cause-specific CKD such as GWAS consortia that compared individuals with diabetic nephropathy with non-CKD diabetes controls (Iyengar et al., 2015; van Zuydam et al., 2018), in addition to studies of glomerular diseases (for example, IgA nephropathy, membranous nephropathy) (GWAS Catalog, 2019). CKD is a heterogeneous condition and its genetic determinants may vary by CKD severity, with more advanced CKD possibly reflecting stronger genetic risk. The genetic determinants of CKD severity have not been previously studied, particularly among individuals of diverse ancestries that vary in their genetic susceptibility.
Our recent research in diverse populations within the Continental Origins and Genetic Epidemiology Network (COGENT) Kidney Consortium identified 93 novel loci for eGFR, displaying homogenous effects across four major ancestries (Morris et al., 2019). Using Mendelian Randomization, we have shown that identified single nucleotide variants (SNVs) were causally related to a clinical diagnosis of CKD from International Classification of Disease (ICD) diagnosis billing codes in the United Kingdom Biobank. These SNVs, identified from the trans-ethnic analyses and showing homogenous effects across ancestries, more likely capture CKD genetic risk across diverse populations.
To identify novel risk loci associated with CKD severity (stages), we assembled multi-ethnic data on cases (4,150 mild to moderate CKD and 1,105 ESKD) and non-CKD controls for samples up to 41,041 participants within the Population Architecture using Genomics and Epidemiology (PAGE) study (Bien et al., 2016). We also examined the association of eGFR-identified GWAS variants with CKD stages using genetic variants reported by the COGENT-Kidney consortium.
Materials and Methods
PAGE Study Description
The PAGE consortium includes eligible minority participants from four studies. The Women’s Health Initiative (WHI) is a long-term, prospective, multi-center, and multi-ethnic cohort study investigating post-menopausal women’s health recruited from 1993 to 1998 at 40 centers across the United States (Anderson et al., 2003). WHI participants of European descent were excluded from analyses. The Hispanic Community Health Study/Study of Latinos (HCHS/SOL) is a multi-center study of Hispanic/Latinos with the goal of determining the role of acculturation in the prevalence and development of diseases relevant to Hispanic/Latino health. Starting in 2006, household sampling was used to recruit self-identified Hispanic/Latinos from four sites in San Diego, CA, Chicago, IL, Bronx, NY, and Miami, FL (Sorlie et al., 2010). The Multiethnic Cohort (MEC) is a population-based prospective cohort study recruiting men and women aged 45–75 from Hawaii and Los Angeles, California, in 1993–1996, that examines lifestyle risk factors and genetic susceptibility for cancer across five racial/ethnic groups (Kolonel et al., 2000). Only the African American, Japanese American, and Native Hawaiian participants for MEC were included in analyses. The BioMe BioBank is an Electronic Medical Record-linked biobank that integrates research data and clinical care information for consented patients at the Mount Sinai Medical Center, which serves diverse local communities of upper Manhattan with broad health disparities. Recruitment began in 2007 and continues at 30 clinical care sites throughout New York City. BioMe participants were African American, Hispanic/Latino, primarily of Caribbean origin (36%), Caucasian (30%), and Others who did not identify with any of the available options (9%) (Nadkarni et al., 2014). All PAGE participants have provided informed consent. Up to 41,041 participants with kidney phenotype information were included in analyses.
Genotypes and Imputation
The genotyping and quality control (QC) in PAGE has been previously described (Bien et al., 2016). Briefly, 53,426 samples were genotyped centrally at the Center for Inherited Disease Research (CIDR) in the Johns Hopkins University using the Multi-Ethnic Genotyping Array (MEGA), Consortium version, consisting of 1,705,969 single nucleotide variants (SNV). Genotypes were called using the GenomeStudio version 2001.1, Genotyping Module 1.9.4, and GenTrain version 1.0. Extensive QC was performed to the combined genotyping data, which included checks for gender discrepancies, Mendelian inconsistencies, unexpected duplication, unexpected non-duplication, poor performance, or DNA mixture. Samples with identity issues, restricted consent, and duplicates were also removed (final sample 51,520 subjects). SNVs were filtered if they had a missing call rate ≥ 2%, more than 6 discordant calls in 988 study duplicates, > 1 Mendelian errors in 282 trios and 1,439 duos, a Hardy–Weinberg p-value < 10-4, sex difference in allele frequency ≥ 0.2 and sex difference in heterozygosity >0.3 for autosome chromosomes. After SNV QC, a total of 1,438,399 SNVs were available for analyses.
Imputation was done centrally at the University of Washington in combined samples. The study samples were phased with SHAPEIT2 (Delaneau et al., 2013) and imputed with IMPUTE2 (Howie et al., 2009) to the 1000 Genomes Project Phase 3 data release. Reference panel variants were restricted to a minor allele count (MAC) ≥2 across all 1000. Kinship coefficients were estimated using PC-Relate (Conomos et al., 2016). Principal components (PCs) were estimated in unrelated individuals within the global study population using SNVRelate package (Zheng et al., 2012). The first 10 PCs explained most of the genetic variation in the PAGE study population.
CKD Phenotypes
For studies with available serum creatinine (HCHS/SOL, WHI), we calculated eGFR using the CKD-EPI equation and baseline cohort data (Inker et al., 2012). Mild to moderate CKD (referred as CKD) was defined by an eGFR between 15 and 60 ml/min/1.73 m2 (HCHS/SOL, WHI) or by an ICD-9 or 10 code in medical claims (585.1-585.5, 585.9, N18.1-N18.5, N18.9) (MEC, BioMe) (Nadkarni et al., 2014). Advanced CKD (referred as ESKD) was defined by an eGFR < 15 ml/min/1.73 m2 (HCHS/SOL), an ICD-9 or 10 code of 585.6 or N18.6 related to ESKD (MEC) or ESKD obtained through linkage to the United States Renal Data System (BioMe). CKD and ESKD cases were mutually exclusive. Controls were individuals with an eGFR > 60 ml/min/1.73 m2 or without ICD codes related to CKD or ESKD. In sensitivity analyses, we used two additional definitions for mild to moderate CKD: one based on ICD codes (MEC and BioMe data) and one based on eGFR from cohort studies (HCHS/SOL, WHI).
Statistical Analyses
We performed genome-wide association analyses of the combined data using the software SUGEN, which implements a generalized estimating equation approach and empirically estimates within-family correlations without modeling the correlation structures of complex pedigrees (Lin et al., 2014). SUGEN adopts a modified version of the sandwich variance estimator, which replaces the empirical covariance matrix of the score vectors by the Fisher information matrix for unrelated subjects. We used logistic regression to analyze categorical phenotypes, and included age, sex, ten PCs, study, center (if available), and ethnicity as covariates. We filtered variants with an effective number <50 based on minor allele frequency of cases (MAF), number of participants (N) and imputation score from impute2 (info) using the following calculation [2∗MAF∗(1-MAF)∗N∗info] where N = total sample for a given phenotype. P-values were generated by score tests. Significant threshold for GWAS was p < 5.0 × 10-8 and suggestive threshold was a p < 1.0 × 10-7.
For SNVs with p < 10-7, we used the clumping procedure INDEP in Easystrata to identify independent signals at each locus which included on 1 Mb genomic interval flanking the lead SNVs. We also examined if the published loci for eGFR were associated with CKD or ESKD. We prioritized eGFR variants identified in the multi-ethnic COGENT-Kidney consortium and also used variants available in the Genome catalog for CKD and ESKD. For the APOL1 locus, we performed analysis conditioning on the most significant SNV in the region. In sensitivity analysis, we assessed the significance of SNVs identified in the GWAS for CKD using CKD definition based on ICD codes or eGFR thresholds.
Associations in the United Kingdom Biobank
We assessed the association of our identified variants in the United Kingdom Biobank for SNVs available in European ancestry listed in Tables 2, 3. We extracted p-values from United Kingdom Biobank using GeneATLAS (2019) for ICD-10 diagnosis codes N18 (chronic renal failure, 4,905 cases, and 447,359 controls), N19 (unspecified renal failure including uremia, kidney failure, azotemia, 1,516 cases and 450,748 controls) and renal/kidney failure (759 cases and 451,505 controls) (Gene ATLAS). Publicly available replication samples for CKD/ESKD in non-European ancestry were not available.
Results
Participant Characteristics
Overall, 41,041 individuals contributed data for CKD analyses (10.1% cases) and 31,694 individuals to ESKD analyses (3.4% cases) with non-overlapping cases. Cases were older and had a lower proportion of women compared to controls, and more comorbidities (Table 1). The study-specific contribution for cases and controls is shown in Supplementary Table 1.
Multi-Ethnic GWAS Findings
The main findings from the GWAS of CKD and ESKD in combined multi-ethnic samples are shown in Tables 2, 3. Manhattan plots are shown in Figure 1 and quantile-quantile (QQ) are shown in Supplementary Figure 1. The genomic control lambdas were 1.025 for CKD and 1.026 for ESKD. These analyses identified two genome-wide associated loci: a chromosome 10 locus nearby NMT2 associated with CKD (rs10906850, allele frequency 0.23, p = 3.7 × 10-8) (Table 2 and Figure 2A) and the chromosome 22 APOL1 locus associated with ESKD (four common SNVs, including the two highly correlated APOL1 G1 missense variants rs73885319 and rs60910145) (Figure 2B and Supplementary Table 2). APOL1 G2 indel was not available in our data. Conditional analysis on the most significant SNV supported an independent association at the APOL1 locus.
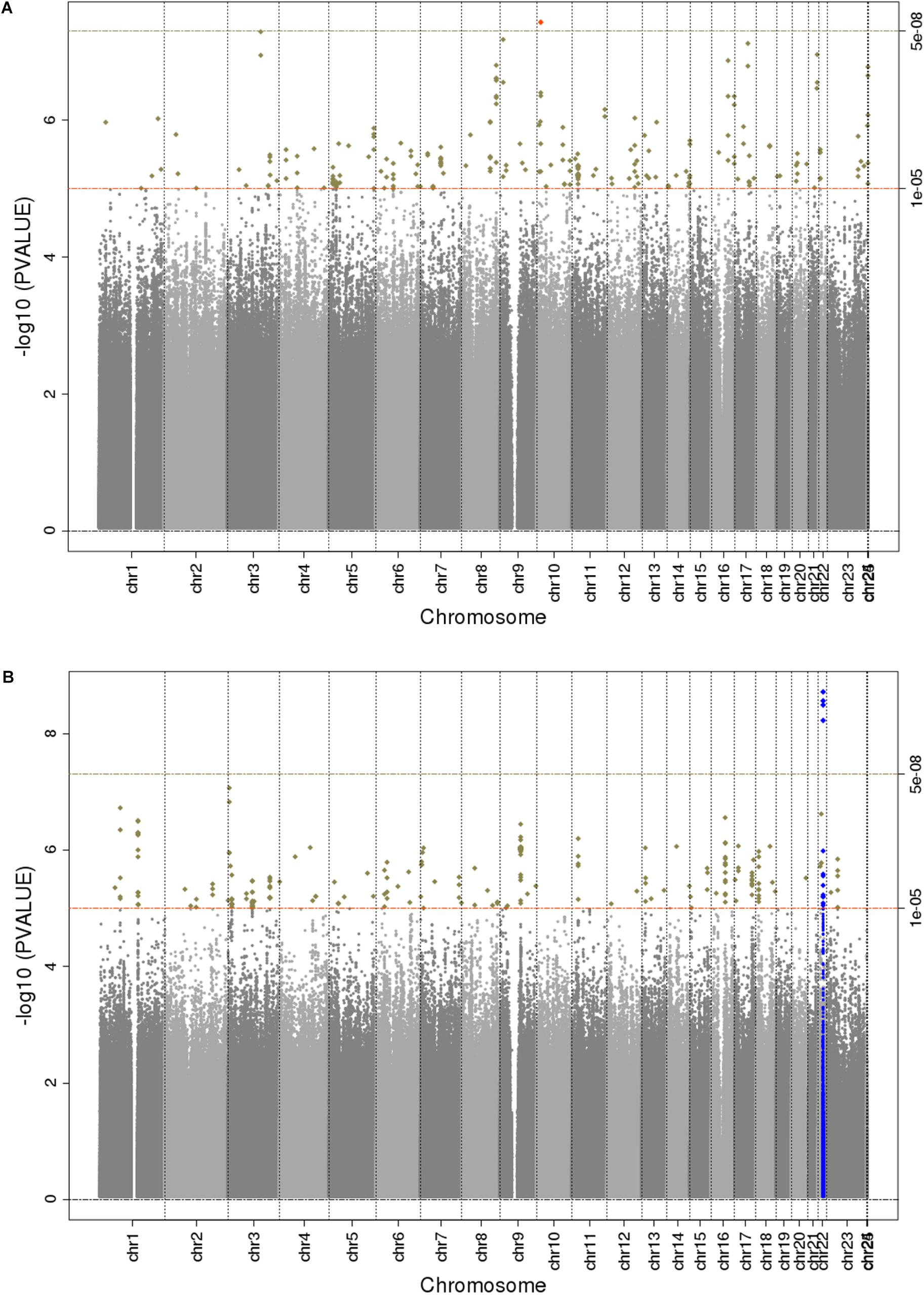
Figure 1. Manhattan plots for trans-ethnic GWAS of CKD (A) and ESKD (B). Significant novel (red) and known (blue) loci are highlighted.
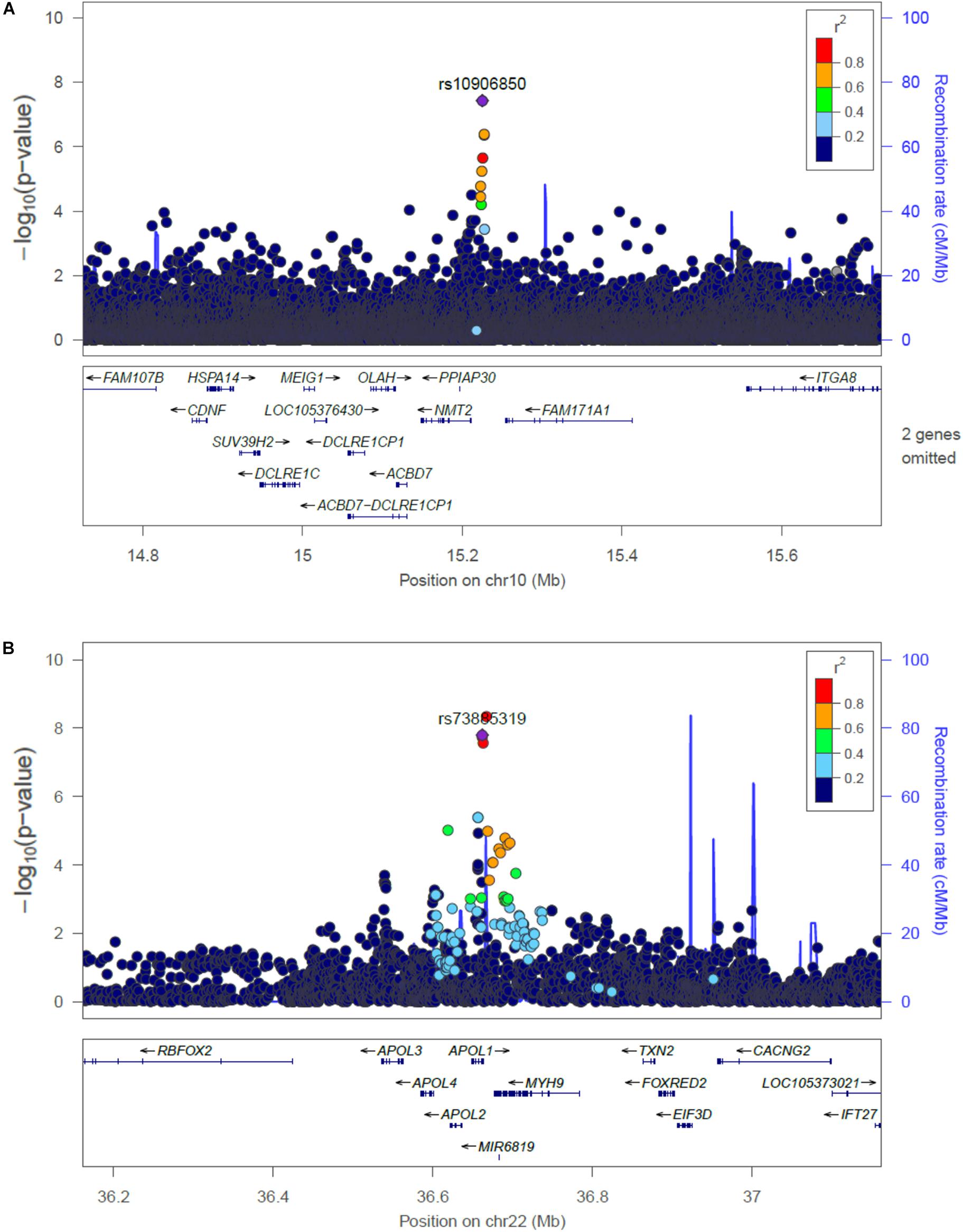
Figure 2. Regional plots of the association at the NMT2 (A) with CKD, and the APOL1 locus associated with ESKD (B). Linkage disequilibrium was estimated from the PAGE multi-ethnic data.
Several loci with low frequency SNVs had suggestive evidence for association including SNVs located nearby RPN1 (p = 5.1 × 10-8), TYRP1 (p = 6.7 × 10-8), and LUC7L3 (p = 7.6 × 10-8) associated with CKD, and MIR4790 (p = 8.6 × 10-8) associated with ESKD. Except for LUC7L3, the most significant SNV at these loci was rare or not present in European ancestry. For example, the TYRP1 variant allele frequency in 1000 Genomes Project is 0.02 in African ancestry and 0.001 in European ancestry, and RPN1 and MIR4790 SNVs are not available in 1000 Genomes Project European ancestry samples. Additional SNVs associated with ESKD at p < 10-7 included a low frequency intronic variant in FTO (rs7189997, p = 2.8 -10-7) and a common variant nearby IRX3 (rs8050506, p = 9.94 × 10-7). Both SNVs were also more common in African ancestry reference panels (Table 3).
Nine of the SNVs with significant or suggestive association with CKD or ESKD (listed in Tables 2, 3) were available for replication in the United Kingdom Biobank white British samples. The SNV rs10906850 nearby NMT2 was significantly associated with ICD code for renal/kidney failure (p = 0.008) and rs11645800 nearby CDH8 was nominally associated with renal/kidney failure (Table 4). The common indel rs138873021 was not available in the United Kingdom Biobank.
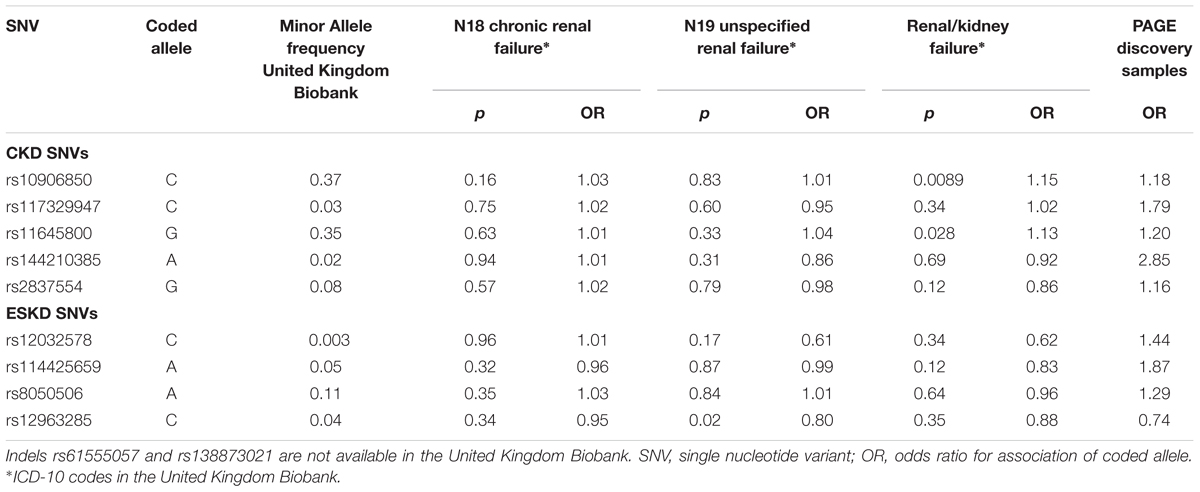
Table 4. Association of SNVs identified for CKD or ESKD in white British in the United Kingdom Biobank for three CKD diagnosis.
Cross-Association of Significant SNVs for CKD and ESKD and Sensitivity Analyses
At the NMT2 locus associated for CKD, the most significant SNV was not associated with ESKD (p = 0.82). At the APOL1 locus associated with ESKD, rs73885319 (and other variants) were not associated with CKD (p = 0.76). To explore heterogeneity in the definition for CKD that could explain our findings, we examined the association of the genome-wide associated significant SNVs in samples stratified by CKD definition based on ICD code (n = 4,698 cases, n = 18,764 controls) or eGFR thresholds (n = 3,179 cases, n = 18,550 controls). The NMT2 SNV rs10906850 was associated with CKD using both definitions (p = 2.4 × 10-6 for ICD codes and p = 8.2 × 10-3 for eGFR thresholds) and there was consistency in direction of effects. Conversely, APOL1 rs73885319 was not associated with CKD using either definition (p > 0.05).
Association of Previously Reported eGFR SNVs From the COGENT-Kidney Consortium With CKD Stages in PAGE
Given PAGE studies included diverse (non-European) participants, we selected 93 eGFR SNVs identified in the trans-ethnic COGENT-Kidney Consortium to assess their association with CKD and ESKD in PAGE. Seventeen loci were associated with CKD and six loci were associated with ESKD at nominal p-values (p < 0.05). These SNVs had concordant effect estimates between the COGENT-Kidney eGFR lowering allele that showed increased odds of CKD or ESKD (Supplementary Table 3). The PDILT/UMOD was the only locus that was associated with both CKD and ESKD.
Discussion
The main finding of this study is the identification of a new locus for mild to moderate CKD nearby NMT2 for a SNV common across ancestries. The study also shows for the first time genome-wide associations of the APOL1 SNVs with ESKD across multi-ethnic populations. Several other leading low frequency variants showed suggestive association with CKD traits. Most of the low frequency associated SNVs were more common in reference datasets of African ancestry and rare or absent in individuals of European ancestry, particularly for findings related to ESKD. These findings were driven by our discovery samples, which is composed of a large number of non-European ancestry including African Americans (34%) and Hispanics/Latinos (46%). Only nine SNVs were available in the United Kingdom Biobank for a sample of European ancestry. We replicated the association at the NMT2 locus, which also showed consistent direction of effects for alleles between PAGE and the United Kingdom Biobank samples. Of the remaining eight SNVs brought for replication, four had consistent direction of effect between CKD discovery and the United Kingdom Biobank ICD code N18 replication, and one showed consistent direction of effect between ESKD discovery and United Kingdom Biobank ICD code N19 and renal/kidney failure replication, although p-values were not significant. We were unable to replicate other variants due to their low frequency or unavailability in samples of European ancestry, and lack of comparable publicly available summary results for CKD traits in non-European populations.
Single nucleotide variant rs10906850 is an intergenic variant located nearby NMT2, a gene that encodes a protein involved in regulating the function and localization of signaling proteins. The SNV is an expression quantitative trait (eQTL) for NMT2 in tibial artery (p = 4.7 × 10-9), adipose tissue (p = 5.7 × 10-8) and skin (p = 1.6 × 10-7). This locus has not been previously associated with kidney traits. Additional locus identified at p < 10-7 for CKD includes ADCY8, which has been previously described in the CRIC study for association with eGFR decline among non-diabetic African Americans, although not at genome-wide significant level (Parsa et al., 2017). Our SNV in the region (rs138873021) is in linkage disequilibrium with the SNV identified in the CRIC study (rs4492355, p = 1.3 × 10-7 in the CRIC study, D’ = 0.90, r2 = 0.01 in 1000 Genomes Project African ancestry), although rs4492355 is not associated with CKD in the study (p = 0.53). Two loci associated with ESKD have been previously associated with obesity traits (FTO and IRX3), but our SNVs are low frequency and more common in African ancestry. Our findings related to the association of eGFR SNVs identified in the COGENT-Kidney consortium with CKD stages supports heterogeneity in genetic effects across CKD stages. We found a larger number of COGENT-Kidney eGFR lowering SNVs associated with increased CKD than eGFR lowering SNVs associated with increased ESKD. Only one locus was associated with both CKD and ESKD in PAGE: rs77924615 at the PDILT/UMOD locus, which showed nominal associations with these traits.
Chronic kidney disease is a heterogeneous disease in its etiology and clinical manifestation, with varying rates of progression to advanced stages. There is still little understanding on the mechanisms related to these varying patterns of disease severity even within the same disease etiology, for example, diabetic nephropathy. An interesting finding of our study is that there may be differences in genetic susceptibility based on the severity of the disease manifestation. We found little overlap of the most significant loci associated with the CKD phenotype (that includes mild to moderate CKD stages) and ESKD (which reflects advanced CKD). For example, the known APOL1 G1 genotype was not associated with CKD. The NMT2 SNV was significantly associated with CKD but not with ESKD. Although our study lacks information on the APOL1 G2 SNV, we did not identify additional associations at the chromosome 22 locus in conditional analyses. The APOL1 risk genotypes, including G1, were identified in admixture mapping for ESKD attributed to hypertension, FSGS or HIV (Genovese et al., 2010), and associations have been replicated in population studies although not at the genome-wide significant level (Foster et al., 2013; Kramer et al., 2017). Our study provides evidence for APOL1 association with ESKD among diverse populations and for ESKD not selected for a specific disease etiology.
There are several possible explanations for the different genetic findings by CKD stages. Advanced CKD (ESKD) may have a stronger genetic component related to CKD progression, whereas mild to moderate CKD may capture more genetic factors related to CKD initiation. Mild to moderate CKD likely has less genetic influences due to inclusion of older individuals (aging process) and due to environmental factors. Alternatively, there is greater heterogeneity in our definition of mild to moderate CKD, opening the possibility of misclassified cases among individuals with an eGFR around the threshold of 60 ml/min/1.73 m2. However, our sensitivity analyses in CKD subgroups defined by ICD codes or eGFR thresholds did not show differences in the association for our significant NMT2 locus. Overall, these findings provide important information for the study design of genetic studies for CKD, which should consider phenotype heterogeneity and severity of disease particularly when CKD is defined using ICD billing codes and when the definition includes a mixed case of CKD identified through biomarkers or clinical disease.
In conclusion, our multi-ethnic study identified a novel locus for mild to moderate CKD and replicated a known locus for ESKD. Our results highlight the need for more studies in diverse populations to identify genetic risk factors in populations at higher risk for CKD. It also underscores the current limitations of genetic research in these populations, including the lack of suitable replication samples for non-European ancestry variants.
Ethics Statement
All human research was approved by the relevant institutional review boards and conducted according to the Declaration of Helsinki. All participants provided written informed consent.
Author Contributions
NF, GN, and CW conceived and designed the experiments. NF and CW coordinated the project. JA, RT, MG, HMH, SB, TM, and D-YL performed the quality control of genotypes and phenotype data, or support for statistical methods. BL, NF, and CW performed the statistical analyses. BL, NF, LW, and RT drafted the manuscript. All authors critically revised the manuscript.
Funding
NF was supported by the NIH (R01-MD-012765, R56-DK-104806, and R01-DK-117445-01A1). HMH is supported by NHLBI training grant T32 HL007055 and T32 HL129982. PAGE program was funded by the National Human Genome Research Institute (NHGRI) with co-funding from the National Institute on Minority Health and Health Disparities (NIMHD), supported by U01HG007416 (CALiCo), U01HG007417 (ISMMS), U01HG007397 (MEC), U01HG007376 (WHI), and U01HG007419 (Coordinating Center). The contents of this paper are solely the responsibility of the authors and do not necessarily represent the official views of the NIH. Funding support for the Genetic Epidemiology of Causal Variants Across the Life Course (CALiCo) program was provided through the NHGRI PAGE program (U01 HG007416 and U01 HG004803). The following studies contributed to this manuscript and are funded by the following agencies: The Hispanic Community Health Study/Study of Latinos was carried out as a collaborative study supported by contracts from the National Heart, Lung, and Blood Institute (NHLBI) to the University of North Carolina (N01-HC65233), University of Miami (N01-HC65234), Albert Einstein College of Medicine (N01-HC65235), Northwestern University (N01-HC65236), and San Diego State University (N01-HC65237). The following Institutes/Centers/Offices contribute to the HCHS/SOL through a transfer of funds to the NHLBI: National Institute on Minority Health and Health Disparities, National Institute on Deafness and Other Communication Disorders, National Institute of Dental and Craniofacial Research, National Institute of Diabetes and Digestive and Kidney Diseases, National Institute of Neurological Disorders and Stroke, NIH Institution-Office of Dietary Supplements. Funding support for the PAGE IPM BioMe Biobank study was provided through NHGRI (U01 HG007417). Phenotype data collection was supported by The Andrea and Charles Bronfman Philanthropies. The Multiethnic Cohort study (MEC) characterization of epidemiological architecture is funded through the NHGRI PAGE program (U01 HG007397, U01HG004802 and its NHGRI ARRA supplement). The MEC study is funded through the National Cancer Institute (R37CA54281, R01 CA63, P01CA33619, U01CA136792, and U01CA98758). Funding support for the “Exonic variants and their relation to complex traits in minorities of the WHI ” study is provided through the NHGRI PAGE program (U01HG007376 and U01HG004790). The WHI program was funded by the National Heart, Lung, and Blood Institute, National Institutes of Health, United States Department of Health and Human Services through contracts HHSN268201100046C, HHSN268201100001C, HHSN268 201100002C, HHSN268201100003C, HHSN268201100004C, and HHSN271201100004C. The datasets used for the analyses described in this manuscript were obtained from dbGaP under accession numbers phs000220, phs000227, phs000555 (HCHS/SOL), and phs000925.
Conflict of Interest Statement
The authors declare that the research was conducted in the absence of any commercial or financial relationships that could be construed as a potential conflict of interest.
Acknowledgments
The PAGE consortium thanks the staff and participants of all PAGE studies for their important contributions. The complete list of PAGE members can be found at http://www.pagestudy.org. Samples and data of The Charles Bronfman Institute for Personalized Medicine (IPM) BioMe Biobank used in this study were provided by The Charles Bronfman Institute for Personalized Medicine at the Icahn School of Medicine at Mount Sinai (New York). The authors also thank the WHI investigators and staff for their dedication, and the study participants for making the program possible. A listing of WHI investigators can be found at: https://www.whi.org/researchers/Documents%20%20Write%20a%20Paper/WHI%20Investigator%20Short%20List.pdf.
Supplementary Material
The Supplementary Material for this article can be found online at: https://www.frontiersin.org/articles/10.3389/fgene.2019.00494/full#supplementary-material
References
Anderson, G. L., Manson, J., Wallace, R., Lund, B., Hall, D., and Davis, S. (2003). Implementation of the women’s health initiative study design. Ann. Epidemiol. 13, S5–S17.
Bien, S. A., Wojcik, G. L., Zubair, N., Gignoux, C. R., Martin, A. R., and Kocarnik, J. M. (2016). Study, strategies for enriching variant coverage in candidate disease loci on a multiethnic genotyping array. PLoS One 11:e0167758. doi: 10.1371/journal.pone.0167758
Collins, A. J., Foley, R. N., Gilbertson, D. T., and Chen, S. C. (2011). United States renal data system public health surveillance of chronic kidney disease and end-stage renal disease. Kidney Int. Suppl. 5, 2–7. doi: 10.1038/kisup.2015.2
Conomos, M. P., Reiner, A. P., Weir, B. S., and Thornton, T. A. (2016). Model-free estimation of recent genetic relatedness. Am. J. Hum. Genet. 98, 127–148. doi: 10.1016/j.ajhg.2015.11.022
Delaneau, O., Zagury, J. F., and Marchini, J. (2013). Improved whole-chromosome phasing for disease and population genetic studies. Nat. Methods 10, 5–6. doi: 10.1038/nmeth.2307
Foster, M. C., Coresh, J., Fornage, M., Astor, B. C., Grams, M., and Franceschini, N. (2013). APOL1 variants associate with increased risk of CKD among African Americans. J. Am. Nephrol. 24, 1484–1491. doi: 10.1681/ASN.2013010113
GeneATLAS Gene ATLAS is a Large Database of Associations Between Hundreds of Traits and Millions of Variants using the UK Biobank cohort. Available at: http://geneatlas.roslin.ed.ac.uk/ (accessed March 14, 2019).
Genovese, G., Friedman, D. J., Ross, M. D., Lecordier, L., Uzureau, P., and Freedman, B. I. (2010). Association of trypanolytic ApoL1 variants with kidney disease in African Americans. Science 329, 841–845. doi: 10.1126/science.1193032
Global Burden of Disease 2016 Causes of Death Collaborators (2017). Global, regional, and national age-sex specific mortality for 264 causes of death, 1980-2016: a systematic analysis for the global burden of disease study 2016. Lancet 390, 1151–1210.
Go, A. S., Chertow, G. M., Fan, D., McCulloch, C. E., and Hsu, C. Y. (2004). Chronic kidney disease and the risks of death, cardiovascular events, and hospitalization. N. Engl. J. Med. 351, 1296–1305. doi: 10.1056/nejmoa041031
GWAS Catalog The NHGRI-EBI Catalog of Published Genome-Wide Association studies. Available at: https://www.ebi.ac.uk/gwas/ (accessed March 14, 2019).
Howie, B. N., Donnelly, P., and Marchini, J. (2009). A flexible and accurate genotype imputation method for the next generation of genome-wide association studies. PLoS Genet. 5:e1000529. doi: 10.1371/journal.pgen.1000529
Inker, L. A., Schmid, C. H., Tighiouart, H., Eckfeldt, J. H., Feldman, H. I., and Greene, T. (2012). Estimating glomerular filtration rate from serum creatinine and cystatin C. N. Engl. J. Med. 367, 20–29. doi: 10.1056/NEJMoa1114248
Iyengar, S. K., Sedor, J. R., Freedman, B. I., Kao, W. H., Kretzler, M., and Keller, B. J. (2015). Genome-wide association and trans-ethnic meta-analysis for advanced diabetic kidney disease: family investigation of nephropathy and diabetes (FIND). PLoS Genet. 11:e1005352. doi: 10.1371/journal.pgen.1005352
Kolonel, L. N., Henderson, B. E., Hankin, J. H., Nomura, A. M., Wilkens, L. R., and Pike, M. C. (2000). A multiethnic cohort in Hawaii and Los Angeles: baseline characteristics. Am. J. Epidemiol. 151, 346–357. doi: 10.1093/oxfordjournals.aje.a010213
Kramer, H. J., Stilp, A. M., Laurie, C. C., Reiner, A. P., Lash, J., and Daviglus, M. L. (2017). African ancestry-specific alleles and kidney disease risk in hispanics/latinos. J. Am. Soc. Nephrol. 28, 915–922. doi: 10.1681/ASN.2016030357
Levin, A., and Stevens, P. E. (2014). Summary of KDIGO 2012 CKD guideline: behind the scenes, need for guidance, and a framework for moving forward. Kidney Int. 85, 49–61. doi: 10.1038/ki.2013.444
Lin, D. Y., Tao, R., Kalsbeek, W. D., Zeng, D., Gonzalez, F. II, and Fernandez-Rhodes, L. (2014). Genetic association analysis under complex survey sampling: the hispanic community health study/study of latinos. Am. J. Hum. Genet. 95, 675–688. doi: 10.1016/j.ajhg.2014.11.005
Morris, A. P., Le, T. H., Wu, H., Akbarov, A., van der Most, P. J., and Hemani, G. (2019). Trans-ethnic kidney function association study reveals putative causal genes and effects on kidney-specific disease aetiologies. Nat. Commun. 10:29. doi: 10.1038/s41467-018-07867-7
Nadkarni, G. N., Gottesman, O., Linneman, J. G., Chase, H., Berg, R. L., and Farouk, S. (2014). Development and validation of an electronic phenotyping algorithm for chronic kidney disease. AMIA Annu. Symp. Proc. 2014, 907–916.
Parsa, A., Kanetsky, P. A., Xiao, R., Gupta, J., Mitra, N., and Limou, S. (2017). Genome-wide association of CKD progression: the chronic renal insufficiency cohort study. J. Am. Soc. Nephrol. 28, 923–934. doi: 10.1681/ASN.2015101152
Saran, R., Robinson, B., Abbott, K. C., Agodoa, L. Y. C., Bhave, N., and Bragg-Gresham, J. (2018). US renal data system 2017 annual data report: epidemiology of kidney disease in the United States. Am. J. Kidney Dis. 71:A7.
Sorlie, P. D., Aviles-Santa, L. M., Wassertheil-Smoller, S., Kaplan, R. C., Daviglus, M. L., and Giachello, A. L. (2010). Design and implementation of the hispanic community health study/study of latinos. Ann. Epidemiol. 20, 629–641. doi: 10.1016/j.annepidem.2010.03.015
van Zuydam, N. R., Ahlqvist, E., Sandholm, N., Deshmukh, H., and Rayner, N. W. (2018). A genome-wide association study of diabetic kidney disease in subjects with type 2 diabetes. Diabetes Metab. Res. Rev. 67, 1414–1427.
Keywords: genetics, chronic kidney disease stages, genome-wide association studies, APOL1, end stage kidney disease, diverse populations, single nucleotide polymorphisms
Citation: Lin BM, Nadkarni GN, Tao R, Graff M, Fornage M, Buyske S, Matise TC, Highland HM, Wilkens LR, Carlson CS, Park SL, Setiawan VW, Ambite JL, Heiss G, Boerwinkle E, Lin D-Y, Morris AP, Loos RJF, Kooperberg C, North KE, Wassel CL and Franceschini N (2019) Genetics of Chronic Kidney Disease Stages Across Ancestries: The PAGE Study. Front. Genet. 10:494. doi: 10.3389/fgene.2019.00494
Received: 31 January 2019; Accepted: 06 May 2019;
Published: 24 May 2019.
Edited by:
Martin H. De Borst, University Medical Center Groningen, NetherlandsReviewed by:
Jessica Van Setten, University Medical Center Utrecht, NetherlandsTheodora Katsila, National Hellenic Research Foundation, Greece
Copyright © 2019 Lin, Nadkarni, Tao, Graff, Fornage, Buyske, Matise, Highland, Wilkens, Carlson, Park, Setiawan, Ambite, Heiss, Boerwinkle, Lin, Morris, Loos, Kooperberg, North, Wassel and Franceschini. This is an open-access article distributed under the terms of the Creative Commons Attribution License (CC BY). The use, distribution or reproduction in other forums is permitted, provided the original author(s) and the copyright owner(s) are credited and that the original publication in this journal is cited, in accordance with accepted academic practice. No use, distribution or reproduction is permitted which does not comply with these terms.
*Correspondence: Nora Franceschini, bm9yYWZAdW5jLmVkdQ==
†These authors have contributed equally to this work