- 1Department of Anatomy, School of Medicine, Pusan National University, Yangsan, South Korea
- 2Department of Premedicine, School of Medicine, Pusan National University, Yangsan, South Korea
- 3Division of Drug Process Development, New Drug Development Center, Osong Medical Innovation Foundation, Cheongju, South Korea
- 4Department of Molecular and Human Genetics, Baylor College of Medicine, Houston, TX, United States
- 5Department of Pathology and Immunology, Baylor College of Medicine, Houston, TX, United States
- 6Center for Skeletal Medicine and Biology, Baylor College of Medicine, Houston, TX, United States
- 7Department of Biochemistry, Pusan National University School of Medicine, Yangsan, South Korea
- 8Department of Convergence Medical Science, Pusan National University School of Medicine, Yangsan, South Korea
- 9Department of Anatomy, Biomedical Research Institute, School of Medicine, Pusan National University, Yangsan, South Korea
- 10Department of Biomedical Informatics, Biomedical Research Institute, School of Medicine, Pusan National University, Yangsan, South Korea
Transmembrane p24 trafficking protein 3 (TMED3) is a metastatic suppressor in colon cancer and hepatocellular carcinoma. However, its function in the progression of clear cell renal cell carcinoma (ccRCC) is unknown. Here, we report that TMED3 could be a new prognostic marker for ccRCC. Patient data were extracted from cohorts in the Cancer Genome Atlas (TCGA) and the International Cancer Genome Consortium (ICGC). Differential expression of TMED3 was observed between the low stage (Stage I and II) and high stage (Stage III and IV) patients in the TCGA and ICGC cohorts and between the low grade (Grade I and II) and high grade (Grade III and IV) patients in the TCGA cohort. Further, we evaluated TMED3 expression as a prognostic gene using Kaplan-Meier survival analysis, multivariate analysis, the time-dependent area under the curve (AUC) of Uno’s C-index, and the AUC of the receiver operating characteristics at 5 years. The Kaplan-Meier analysis revealed that TMED3 overexpression was associated with poor prognosis for ccRCC patients. Analysis of the C-indices and area under the receiver operating characteristic curve further supported this. Multivariate analysis confirmed the prognostic significance of TMED3 expression levels (P = 0.005 and 0.006 for TCGA and ICGC, respectively). Taken together, these findings demonstrate that TMED3 is a potential prognostic factor for ccRCC.
Introduction
The transmembrane emp24 domain (TMED) protein family is involved in the vesicular trafficking of proteins and innate immune signaling (Strating and Martens, 2009; Zheng et al., 2016). TMED proteins contain a Golgi dynamics domain and function in Golgi dynamics and intracellular protein trafficking (Jenne et al., 2002; Carney and Bowen, 2004; Luo et al., 2007;Jerome-Majewska et al., 2010). Recent studies have implicated TMED7 in the regulation of TLR4 signaling (Palsson-McDermott et al., 2009; Doyle et al., 2012; Liaunardy-Jopeace et al., 2014), and TMED1 is involved in the ST2L-IL33 axis (Connolly et al., 2013). In addition, a recent study showed that TMED3 overexpression was significantly correlated with an aggressive phenotype of HCC and poor prognosis (Zheng et al., 2016). In HCC, TMED3 promotes metastasis through IL-11/STAT3 signaling. However, the clinical significance of TMED3 and its role in other malignancies are unknown.
Kidney cancer is among the top 10 cancers, and 30% of patients with kidney cancer present with metastatic disease (Nickerson et al., 2008). Renal cell carcinoma (RCC) accounts for 90% of kidney cancers (Choueiri and Motzer, 2017; Siegel et al., 2018), and clear cell renal cell carcinoma (ccRCC) is the most common type of kidney cancer (Srigley et al., 2013). However, 30% of patients with ccRCC have been diagnosed with advanced cancer (Karakiewicz et al., 2007), and the therapeutics available for renal cancer is not very effective. Therefore, there is a great need for new drugs and biomarkers for ccRCC.
Thus far, the prognostic significance of TMED3 in ccRCC is unknown. In this study, we present the first data on TMED3 expression in ccRCC in a well-defined cohort from the TGCA (Cerami et al., 2012; Cancer Genome Atlas Research Network et al., 2013) and ICGC (International Cancer Genome Consortium et al., 2010) primary ccRCC cohorts. The statistical analysis suggested that TMED3 could be a useful prognostic factor in ccRCC.
Materials and Methods
Patient Data Acquisition and Statistical Analysis
The data were downloaded from TCGA (Cerami et al., 2012; Cancer Genome Atlas Research Network et al., 2013) and ICGC (International Cancer Genome Consortium et al., 2010) from the ICGC data portal1 in March 2018. We downloaded mRNA expression (TCGA, RSEM normalization; ICGC, RPKM normalization) and clinical information. Samples with insufficient information (gene expression values and survival information) were excluded from the analysis. GSE11024 (Affymetrix U133 Plus 2.0 Array) (Kort et al., 2008), GSE12606 (Affymetrix U133 Plus 2.0 Array) (Stickel et al., 2009), and GSE14762 (Affymetrix U133 Plus 2.0 Array) (Wang et al., 2009) were downloaded from GEO database using “GEOquery” R package. In the stage-related analysis, only the “Not Available (NA)” value of the stage was excluded. When the grade-related analysis was performed, only the “NA” value of the grade was excluded. These analyses were performed using R software version 3.5.0 (R Core Team, 2018).
To identify the differences of TMED3 expression values between low stages (I and II) and high stages (III and IV), we performed Wilcoxon rank sum test using “coin” R package because the differences were not a normal distribution. We used Kruskal–Wallis test with Bonferroni adjustment to identify the differential expression of TMED3 in different T stages using appropriate statistical methods (GSE11024 and GSE14762, Welch two sample t-test; GSE12606, paired t-test). Survival analyses were performed to predict overall survival (OS). We used three methods, (1) Uno’s C-index in a time-dependent Area Under the Curve (AUC) analysis, (2) AUC values in receiver operating characteristics (ROC) at 5 years, and (3) Kaplan-Meier survival analysis, to evaluate the accuracy of the discrimination, as described previously (Cho et al., 2018; Han et al., 2018). These values were obtained using the R packages “survival” and “survAUC.” The C-index is a global measure of the fitness of a survival model for continuous event time in clinical studies (Uno et al., 2011; Kim et al., 2017a,b). In the Kaplan-Meier analyses, we determined the optimal cutoff value that had the maximal Uno’s C-index by fivefold cross-validation (Table 2). We then used univariate and multivariate Cox regression to compare the effect of TMED3 expression level as a categorical value on prognosis, along with other clinical variables. In the multivariate analysis with the stepwise selection, we included clinical factors that were not associated with survival in the univariate analysis. All statistical analyses were performed using R.
Results
Overexpression of TMED3
The study included 446 patients from the TCGA and 91 patients from the ICGC (Hoshida et al., 2009; International Cancer Genome Consortium et al., 2010; Cerami et al., 2012; Cancer Genome Atlas Research Network et al., 2013; Shtraizent et al., 2017). Patient information that was used in the current study is shown in Table 1. The expression of TMED3 was compared between low (Stage I and II) and high stage (Stage III and IV) ccRCC patients in the TCGA and ICGC cohorts, and between low (Grade I and II) and high grade (Grade III and IV) ccRCC patients from the TCGA cohort. The TMED3 expression levels in the high stage and grade cohorts were much higher than in the low stage and grade cohorts (Figure 1). The groups with statistically significant TMED3 differences were only two groups (T1 vs. T2 and T1 vs. T3 in TCGA) (Supplementary Figure S1). Additionally, we confirmed TMED3 expression in cancer tissues are higher than normal tissues by using GSE11024, GSE12606, and GSE14762) (Supplementary Figure S2).
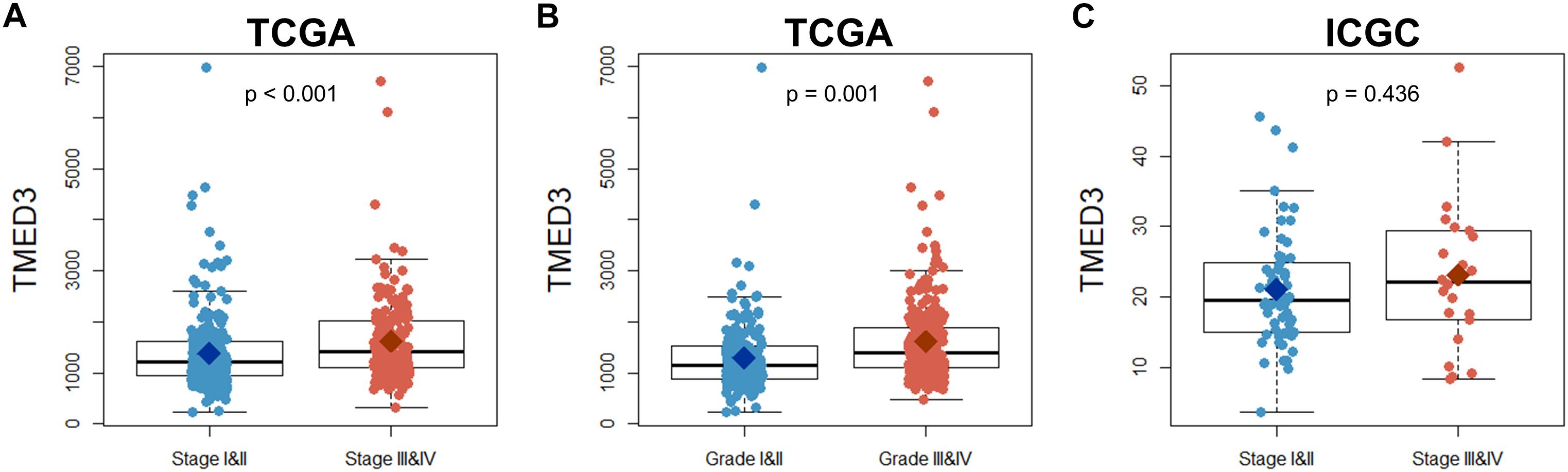
Figure 1. Comparison of TMED3 gene expression between low (Stage I and II) and high stage (Stage III and IV) patients in the TCGA and ICGC ccRCC cohorts as well as the low (Grade I and II) and high grade (Grade III and IV) patients in the TCGA ccRCC cohort. (A,B) TMED3 expression levels in ccRCC patients from the TCGA cohort. (C) TMED3 expression levels in ccRCC cases from the ICGC cohort.
Prognostic Value of TMED3 Expression in ccRCC Patients
To evaluate the prognostic value of TMED3 in ccRCC, we analyzed Kaplan-Meier survival curves for TMED3 gene expression and survival from the TCGA (Figure 2) and ICGC (Figure 3) cohorts. The high TMED3 expression group had a significantly shorter survival than the low TMED3 expression group in the TCGA (Figure 2) and ICGC cohorts (Figure 3). The prognostic value was further confirmed using multivariate analysis (P = 0.005 and 0.006 for the TCGA and ICGC cohorts, respectively; Table 3).
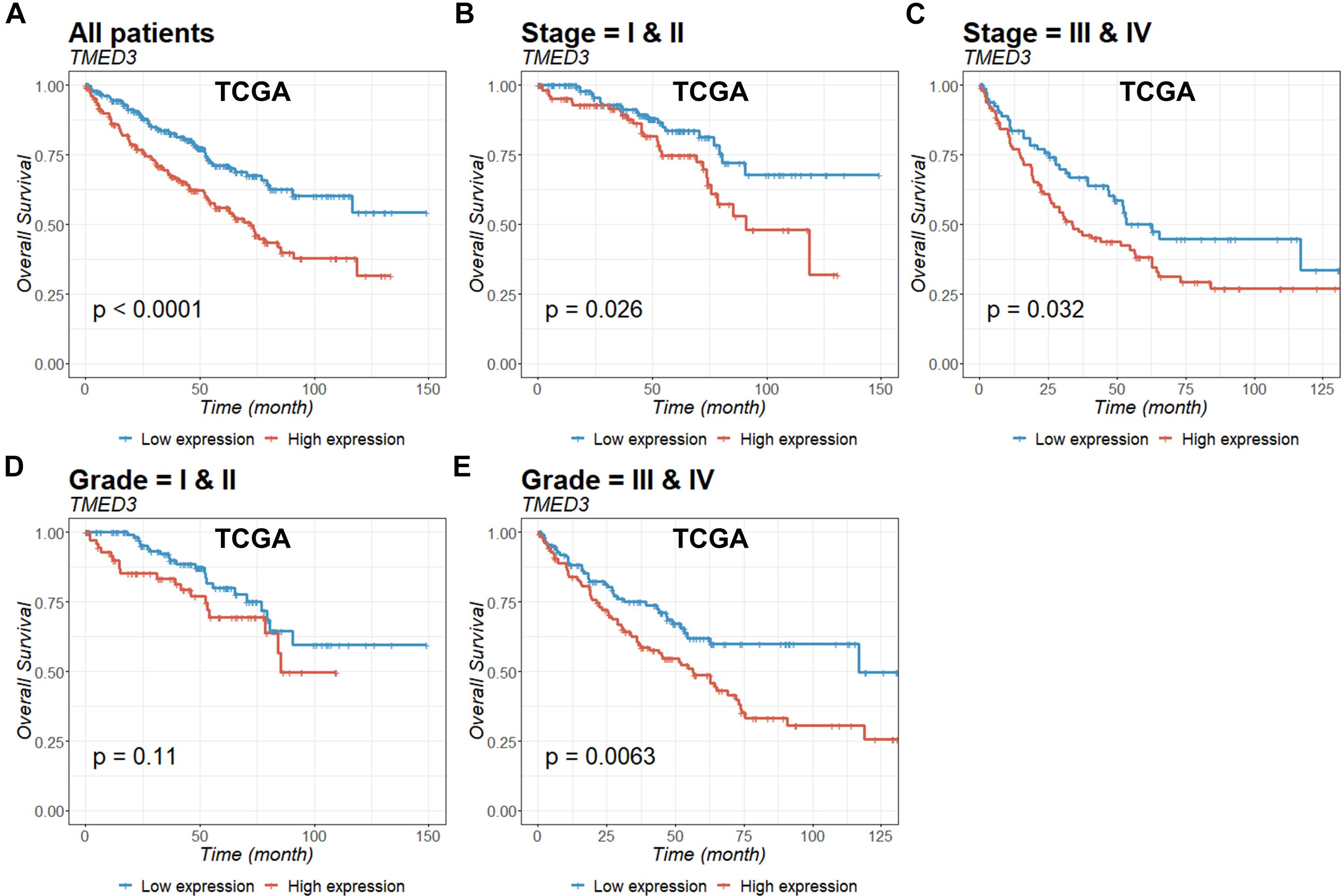
Figure 2. Kaplan-Meier survival curves of ccRCC patients according to TMED3 expression levels. Overall survival of all (A), stage I and II (B), stage III and IV (C), grade I and II (D), and grade III and IV (E) patients in the TCGA cohort.
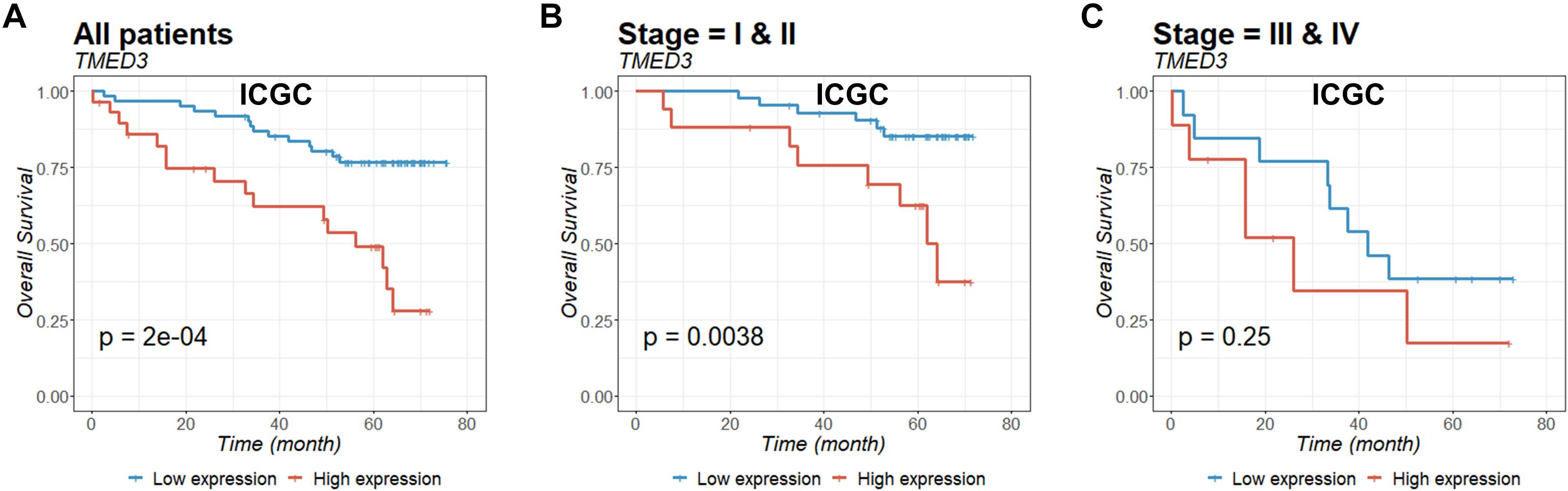
Figure 3. Overall survival of all (A), stage I and II (B), and stage III and IV (C) patients in the ICGC cohort were examined according to TMED3 gene expression levels. P-values were calculated by the log-rank test and are shown at the bottom left of each panel.
To assess the utility of TMED3 expression as a biomarker for ccRCC, we examined Uno’s C-index in a time-dependent AUC analysis and the AUC values for ROCs at 5 years for the TCGA (Figure 4) and ICGC cohorts (Figure 5). TMED3 had high C-index values in the two independent cohorts (TCGA: 0.610 and ICGC: 0.602; Figures 4A, 5A, respectively). The 5-year ROC graphs also showed high AUC values for the TCGA and ICGC cohorts (TCGA: 0.579 and ICGC: 0.594; Figures 4B, 5B, respectively).
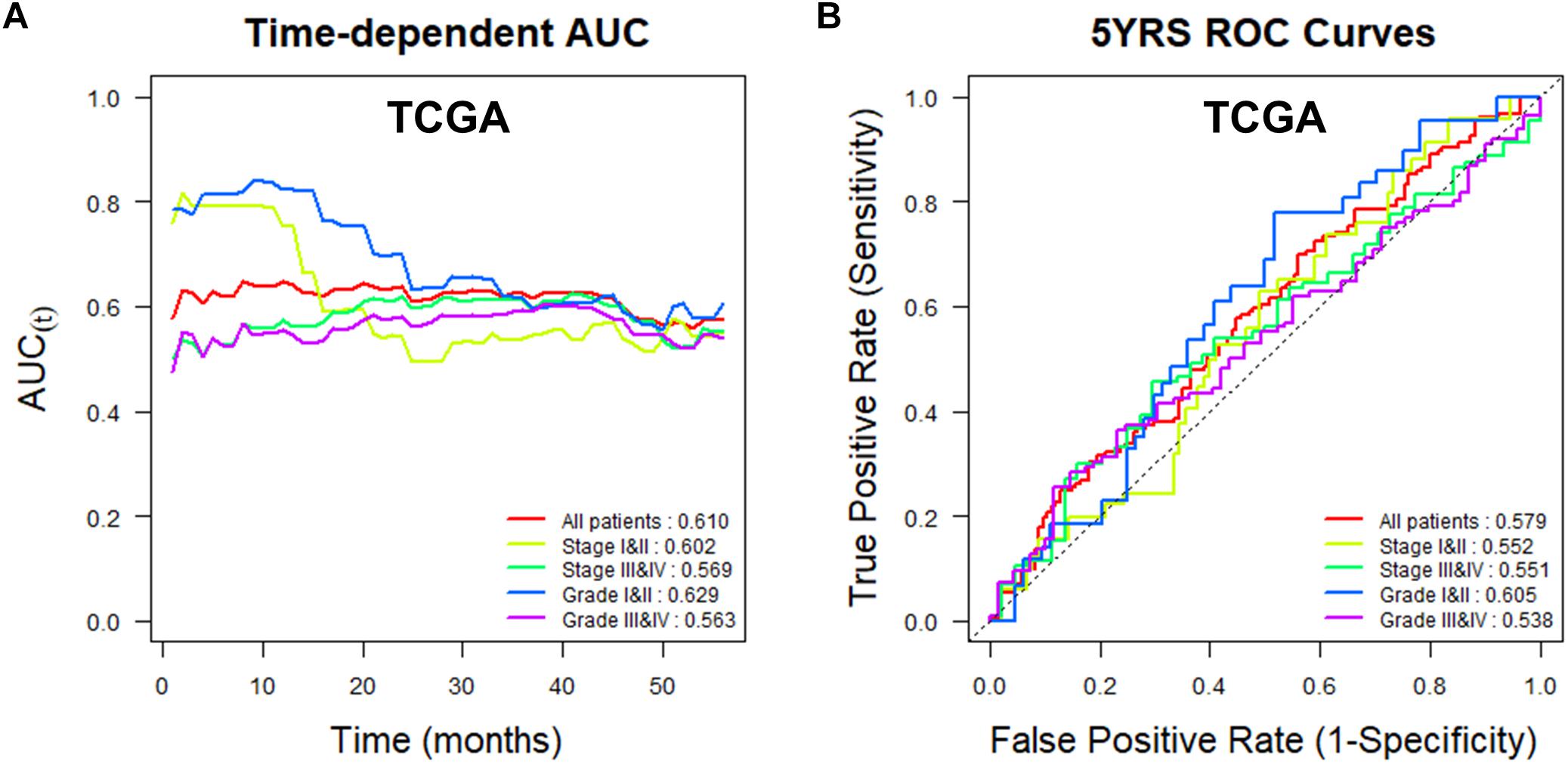
Figure 4. Time-dependent area under the curve (AUC) and receiver operating characteristic (ROC) curves at 5 years according to TMED3 expression levels in the TCGA cohort. (A) Time-dependent AUC and (B) ROC curves at 5 years for patients in the TCGA cohort according to TMED3 expression levels. C-index values are shown at the bottom right in (A). AUC values at 5 years are shown at the bottom right in (B).
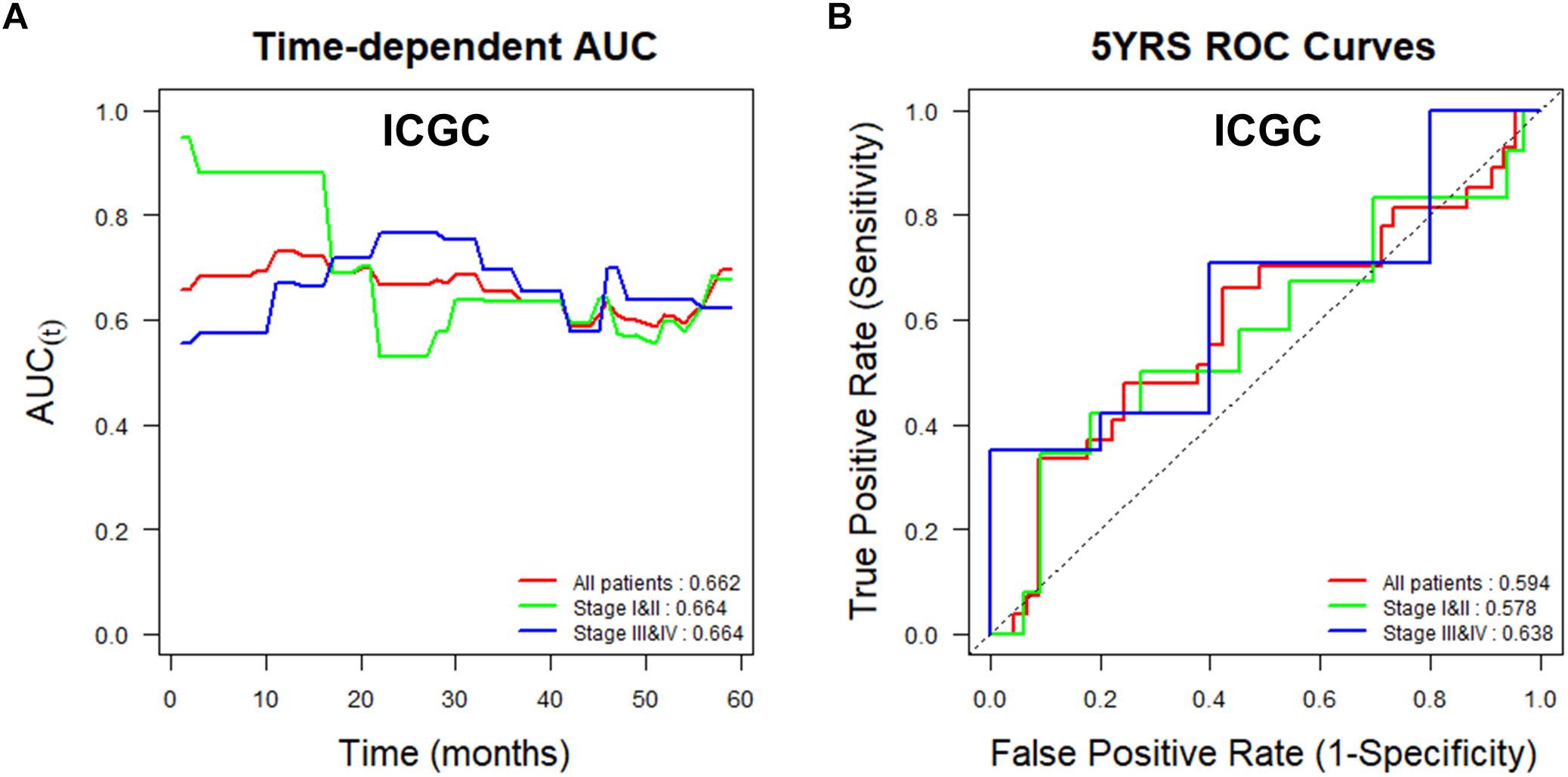
Figure 5. Time-dependent area under the curve (AUC) and receiver operating characteristic (ROC) curves at 5 years according to TMED3 expression levels in the ICGC cohort. (A) Time-dependent AUC and (B) ROC curves at 5 years for patients in the ICGC cohort according to TMED3 expression levels. C-index values are shown at the bottom right in (A). AUC values at 5 years are shown at the bottom right in (B).
Discussion
The main purpose of our study is to strengthen the foundation of precision medicine by analyzing big genome data. There is a growing need to find novel prognostic genes for ccRCC. We analyzed the TMED3 gene from two large independent cohorts as prognostic markers for ccRCC. In the present study, we confirmed that the TMED3 gene fulfills a sufficient role as a universal prognostic marker for ccRCC. From survival analysis, we found a very good marker (TMED3) to predict the prognosis of renal cell carcinoma patients.
TMED3 showed good predictive power in patients with low- and high-stage ccRCC, and low- and high-grade disease in the TCGA cohort and in patients with low- and high-stage cancer in the ICGC cohort (Figures 4, 5). In addition, TMED3 overexpression is associated with poor prognosis of ccRCC. A recent study showed that TMED3 is overexpressed in HCC and that TMED3 promotes HCC metastasis through IL-11/STAT3 signaling (Zheng et al., 2016). Moreover, STAT3 activation is correlated with TMED3 expression in HCC. Further, TMED3 may contribute to the progression of colon cancer (Duquet et al., 2014).
The current treatments for advanced ccRCC are VEGF, VEGFR and mammalian target of rapamycin (mTOR) (Wang et al., 2018) – targeted therapy, but surgical treatment remains the most effective clinical therapy for ccRCC. The ccRCC can easily invade local tissues and metastasize (Yan et al., 2009). In addition, patients with RCC typically respond poorly to radiation and conventional chemotherapy (Linehan, 2012) and ccRCC cells are unsatisfactory and resistant to currently available therapeutics. Further, the rates of recurrence and metastasis for ccRCC remain high due to long-term interactions with the microenvironment (Subramanian and Haas, 2018; Wang et al., 2018). Understanding the mechanisms underlying ccRCC pathogenesis will support the development of more effective therapeutic strategies, including new drugs and biomarkers. With recent advances in biotechnology, the field of bioinformatics has developed rapidly and more potential biomarkers have been discovered (Guan et al., 2018). There are a number of free databases available to the public, including GEO and TCGA databases that contain extensive gene expression data useful to finding heretofore unknown biomarkers and provide a wealth of information that can be used to identify biomarkers (Gao et al., 2018). These new molecular markers can be used in combination with the current staging systems.
Based on our findings in both cohorts, the higher the TMED3 expression level, the worse the patient prognosis. Although there are limitations in transcriptome-based studies of TMED3, we believe that our results are sufficient to suggest the possibility of TMED3 as a new prognostic biomarker for ccRCC.
Author Contributions
YK, DL, M-EH, and S-OO contributed to conception and design of the study. DC, WK, J-HK, KL, DP, and C-DK acquired the data. MH and HM analyzed and interpreted the data. DL and YK wrote and reviewed the manuscript. YK supervised the study. All authors read and approved the final version of this manuscript.
Funding
This work was supported by the MRC program (NRF-2015R1A5A2009656) and by grants from the National Research Foundation of Korea (NRF-2018R1C1B6001290 to DL and NRF-2016R1A6A3A11931738) funded by the Korean Government (MSIT). This work was also supported by the Convergence Medical Institute of Technology R&D project (CMIT2019-03), Pusan National University Hospital to DL.
Conflict of Interest Statement
The authors declare that the research was conducted in the absence of any commercial or financial relationships that could be construed as a potential conflict of interest.
Supplementary Material
The Supplementary Material for this article can be found online at: https://www.frontiersin.org/articles/10.3389/fgene.2019.00355/full#supplementary-material
FIGURE S1 | Comparison of TMED3 gene expression according to T stages in the TCGA (A) and ICGC (B) ccRCC cohorts.
FIGURE S2 | Comparison of TMED3 gene expression between cancer and normal kidney in GSE11024 (A), GSE12606 (B), and GSE14762 (C).
Footnotes
References
Cancer Genome Atlas Research Network, Weinstein, J. N., Collisson, E. A., Mills, G. B., Shaw, K. R., Ozenberger, B. A., et al. (2013). The cancer genome atlas pan-cancer analysis project. Nat. Genet. 45, 1113–1120. doi: 10.1038/ng.2764
Carney, G. E., and Bowen, N. J. (2004). P24 proteins, intracellular trafficking, and behavior: Drosophila melanogaster provides insights and opportunities. Biol. Cell 96, 271–278. doi: 10.1016/j.biolcel.2004.01.004
Cerami, E., Gao, J., Dogrusoz, U., Gross, B. E., Sumer, S. O., Aksoy, B. A., et al. (2012). The cBio cancer genomics portal: an open platform for exploring multidimensional cancer genomics data. Cancer Discov. 2, 401–404. doi: 10.1158/2159-8290.CD-12-0095
Cho, S. H., Pak, K., Jeong, D. C., Han, M. E., Oh, S. O., and Kim, Y. H. (2018). The AP2M1 gene expression is a promising biomarker for predicting survival of patients with hepatocellular carcinoma. J. Cell. Biochem. 120, 4140–4146. doi: 10.1002/jcb.27699
Choueiri, T. K., and Motzer, R. J. (2017). Systemic therapy for metastatic renal-cell carcinoma. N. Engl. J. Med. 376, 354–366. doi: 10.1056/NEJMra1601333
Connolly, D. J., O’Neill, L. A., and McGettrick, A. F. (2013). The GOLD domain-containing protein TMED1 is involved in interleukin-33 signaling. J. Biol. Chem. 288, 5616–5623. doi: 10.1074/jbc.M112.403899
Doyle, S. L., Husebye, H., Connolly, D. J., Espevik, T., O’Neill, L. A., and McGettrick, A. F. (2012). The GOLD domain-containing protein TMED7 inhibits TLR4 signalling from the endosome upon LPS stimulation. Nat. Commun. 3:707. doi: 10.1038/ncomms1706
Duquet, A., Melotti, A., Mishra, S., Malerba, M., Seth, C., Conod, A., et al. (2014). A novel genome-wide in vivo screen for metastatic suppressors in human colon cancer identifies the positive WNT-TCF pathway modulators TMED3 and SOX12. EMBO Mol. Med. 6, 882–901. doi: 10.15252/emmm.201303799
Gao, C., Zhou, C., Zhuang, J., Liu, L., Liu, C., Li, H., et al. (2018). MicroRNA expression in cervical cancer: novel diagnostic and prognostic biomarkers. J. Cell. Biochem. 119, 7080–7090. doi: 10.1002/jcb.27029
Guan, L., Tan, J., Li, H., and Jin, X. (2018). Biomarker identification in clear cell renal cell carcinoma based on miRNA-seq and digital gene expression-seq data. Gene 647, 205–212. doi: 10.1016/j.gene.2017.12.031
Han, M. E., Kim, J. Y., Kim, G. H., Park, S. Y., Kim, Y. H., and Oh, S. O. (2018). SAC3D1: a novel prognostic marker in hepatocellular carcinoma. Sci. Rep. 8:15608. doi: 10.1038/s41598-018-34129-9
Hoshida, Y., Nijman, S. M., Kobayashi, M., Chan, J. A., Brunet, J. P., Chiang, D. Y., et al. (2009). Integrative transcriptome analysis reveals common molecular subclasses of human hepatocellular carcinoma. Cancer Res. 69, 7385–7392. doi: 10.1158/0008-5472.CAN-09-1089
International Cancer Genome Consortium, Hudson, T. J., Anderson, W., Artez, A., Barker, A. D., Bell, C., et al. (2010). International network of cancer genome projects. Nature 464, 993–998. doi: 10.1038/nature08987
Jenne, N., Frey, K., Brugger, B., and Wieland, F. T. (2002). Oligomeric state and stoichiometry of p24 proteins in the early secretory pathway. J. Biol. Chem. 277, 46504–46511. doi: 10.1074/jbc.M206989200
Jerome-Majewska, L. A., Achkar, T., Luo, L., Lupu, F., and Lacy, E. (2010). The trafficking protein Tmed2/p24beta(1) is required for morphogenesis of the mouse embryo and placenta. Dev. Biol. 341, 154–166. doi: 10.1016/j.ydbio.2010.02.019
Karakiewicz, P. I., Briganti, A., Chun, F. K., Trinh, Q. D., Perrotte, P., Ficarra, V., et al. (2007). Multi-institutional validation of a new renal cancer-specific survival nomogram. J. Clin. Oncol. 25, 1316–1322. doi: 10.1200/JCO.2006.06.1218
Kim, Y. H., Jeong, D. C., Pak, K., Goh, T. S., Lee, C. S., Han, M. E., et al. (2017a). Gene network inherent in genomic big data improves the accuracy of prognostic prediction for cancer patients. Oncotarget 8, 77515–77526. doi: 10.18632/oncotarget.20548
Kim, Y. H., Jeong, D. C., Pak, K., Han, M. E., Kim, J. Y., Liangwen, L., et al. (2017b). SLC2A2 (GLUT2) as a novel prognostic factor for hepatocellular carcinoma. Oncotarget 8, 68381–68392. doi: 10.18632/oncotarget.20266
Kort, E. J., Farber, L., Tretiakova, M., Petillo, D., Furge, K. A., Yang, X. J., et al. (2008). The E2F3-Oncomir-1 axis is activated in Wilms’ tumor. Cancer Res. 68, 4034–4038. doi: 10.1158/0008-5472.CAN-08-0592
Liaunardy-Jopeace, A., Bryant, C. E., and Gay, N. J. (2014). The COP II adaptor protein TMED7 is required to initiate and mediate the delivery of TLR4 to the plasma membrane. Sci. Signal 7:ra70. doi: 10.1126/scisignal.2005275
Linehan, W. M. (2012). Genetic basis of kidney cancer: role of genomics for the development of disease-based therapeutics. Genome Res. 22, 2089–2100. doi: 10.1101/gr.131110.111
Luo, W., Wang, Y., and Reiser, G. (2007). p24A, a type I transmembrane protein, controls ARF1-dependent resensitization of protease-activated receptor-2 by influence on receptor trafficking. J. Biol. Chem. 282, 30246–30255. doi: 10.1074/jbc.M703205200
Nickerson, M. L., Jaeger, E., Shi, Y., Durocher, J. A., Mahurkar, S., Zaridze, D., et al. (2008). Improved identification of von Hippel-Lindau gene alterations in clear cell renal tumors. Clin. Cancer Res. 14, 4726–4734. doi: 10.1158/1078-0432.CCR-07-4921
Palsson-McDermott, E. M., Doyle, S. L., McGettrick, A. F., Hardy, M., Husebye, H., Banahan, K., et al. (2009). TAG, a splice variant of the adaptor TRAM, negatively regulates the adaptor MyD88-independent TLR4 pathway. Nat. Immunol. 10, 579–586. doi: 10.1038/ni.1727
R Core Team (2018). R: A Language and Environment for Statistical Computing. Vienna: R Foundation for Statistical Computing. Available at: https://www.r-project.org/
Shtraizent, N., DeRossi, C., Nayar, S., Sachidanandam, R., Katz, L. S., Prince, A., et al. (2017). MPI depletion enhances O-GlcNAcylation of p53 and suppresses the Warburg effect. eLife 6:e22477. doi: 10.7554/eLife.22477
Siegel, R. L., Miller, K. D., and Jemal, A. (2018). Cancer statistics, 2018. CA Cancer J. Clin. 68, 7–30. doi: 10.3322/caac.21442
Srigley, J. R., Delahunt, B., Eble, J. N., Egevad, L., Epstein, J. I., Grignon, D., et al. (2013). The international society of urological pathology (ISUP) vancouver classification of renal neoplasia. Am. J. Surg. Pathol. 37, 1469–1489. doi: 10.1097/PAS.0b013e318299f2d1
Stickel, J. S., Weinzierl, A. O., Hillen, N., Drews, O., Schuler, M. M., Hennenlotter, J., et al. (2009). HLA ligand profiles of primary renal cell carcinoma maintained in metastases. Cancer Immunol. Immunother. 58, 1407–1417. doi: 10.1007/s00262-008-0655-6
Strating, J. R., and Martens, G. J. (2009). The p24 family and selective transport processes at the ER-Golgi interface. Biol. Cell 101, 495–509. doi: 10.1042/BC20080233
Subramanian, P., and Haas, N. B. (2018). Recent advances in localized RCC: a focus on VEGF and immuno-oncology therapies. Urol. Oncol. 36, 23–30. doi: 10.1016/j.urolonc.2017.09.008
Uno, H., Cai, T., Pencina, M. J., D’Agostino, R. B., and Wei, L. J. (2011). On the C-statistics for evaluating overall adequacy of risk prediction procedures with censored survival data. Stat. Med. 30, 1105–1117. doi: 10.1002/sim.4154
Wang, Y., Chen, L., Wang, G., Cheng, S., Qian, K., Liu, X., et al. (2018). Fifteen hub genes associated with progression and prognosis of clear cell renal cell carcinoma identified by coexpression analysis. J. Cell. Physiol. 234, 10225–10237. doi: 10.1002/jcp.27692
Wang, Y., Roche, O., Yan, M. S., Finak, G., Evans, A. J., Metcalf, J. L., et al. (2009). Regulation of endocytosis via the oxygen-sensing pathway. Nat. Med. 15, 319–324. doi: 10.1038/nm.1922
Yan, B. C., Mackinnon, A. C., and Al-Ahmadie, H. A. (2009). Recent developments in the pathology of renal tumors: morphology and molecular characteristics of select entities. Arch. Pathol. Lab. Med. 133, 1026–1032. doi: 10.1043/1543-2165-133.7.1026
Keywords: TMED3, TCGA, ICGC, clear cell renal cell carcinoma, prognosis
Citation: Ha M, Moon H, Choi D, Kang W, Kim J-H, Lee KJ, Park D, Kang C-D, Oh S-O, Han M-E, Kim YH and Lee D (2019) Prognostic Role of TMED3 in Clear Cell Renal Cell Carcinoma: A Retrospective Multi-Cohort Analysis. Front. Genet. 10:355. doi: 10.3389/fgene.2019.00355
Received: 08 January 2019; Accepted: 02 April 2019;
Published: 17 April 2019.
Edited by:
William W. Guo, Central Queensland University, AustraliaReviewed by:
Bo Dai, Fudan University Shanghai Cancer Center, ChinaNitish Kumar Mishra, University of Nebraska Medical Center, United States
Copyright © 2019 Ha, Moon, Choi, Kang, Kim, Lee, Park, Kang, Oh, Han, Kim and Lee. This is an open-access article distributed under the terms of the Creative Commons Attribution License (CC BY). The use, distribution or reproduction in other forums is permitted, provided the original author(s) and the copyright owner(s) are credited and that the original publication in this journal is cited, in accordance with accepted academic practice. No use, distribution or reproduction is permitted which does not comply with these terms.
*Correspondence: Yun Hak Kim, yunhak10510@pusan.ac.kr Dongjun Lee, lee.dongjun@pusan.ac.kr
†These authors have contributed equally to this work