- 1School of Biomedical Informatics, The University of Texas Health Science Center at Houston, Houston, TX, United States
- 2BGI-Shenzhen, Shenzhen, China
- 3Department of Epidemiology, The University of Alabama at Birmingham, Birmingham, AL, United States
- 4Department of Biostatistics, The University of Alabama at Birmingham, Birmingham, AL, United States
- 5Division of Statistical Genomics, Department of Genetics, Washington University School of Medicine, St. Louis, MO, United States
- 6USDA/ARS Children’s Nutrition Research Center, Baylor College of Medicine, Houston, TX, United States
- 7Division of Endocrinology, Diabetes and Nutrition, Department of Medicine, University of Maryland School of Medicine, Baltimore, MD, United States
- 8Nutrition and Genomics Laboratory, Jean Mayer United States Department of Agriculture Human Nutrition Research Center on Aging, Tufts University, Boston, MA, United States
- 9IMDEA Alimentación, Madrid, Spain
- 10Centro Nacional de Investigaciones Cardiovasculares, Madrid, Spain
- 11Department of Experimental and Clinical Pharmacology, University of Minnesota, Minneapolis, MN, United States
- 12Division of Cardiovascular Medicine, University of Utah, Salt Lake City, UT, United States
- 13Genetic Analysis Center, Department of Biostatistics, University of Washington, Seattle, WA, United States
- 14College of Public Health, University of Kentucky, Lexington, KY, United States
- 15School of Public Health, The University of Texas Health Science Center at Houston, Houston, TX, United States
Background: Associations of both common and rare genetic variants with fasting blood lipids have been extensively studied. However, most of the rare coding variants associated with lipids are population-specific, and exploration of genetic data from diverse population samples may enhance the identification of novel associations with rare variants.
Results: We searched for novel coding genetic variants associated with fasting lipid levels in 894 samples from the Genetics of Lipid Lowering Drugs and Diet Network (GOLDN) with exome-wide sequencing-based genotype data. In single variant tests, one variant (rs11171663 in ITGA7) was associated with fasting triglyceride levels (P = 7.66E-08), explaining approximately 3.2% of the total trait variance. In gene-based tests, we found statistically significant associations between ITGA7 (P = 1.77E-07) and SLCO2A1 (P = 7.18E-07) and triglycerides, as well as between POT1 (P = 3.00E-07) and low-density lipoprotein cholesterol. In another independent replication cohort consisting of 3,183 African American samples from Hypertension Genetic Epidemiology Network (HyperGEN) and the Genetic Epidemiology Network of Arteriopathy (GENOA), the top genes achieved P-values of 0.04 (ITGA7), 0.08 (SLCO2A1), and 0.02 (POT1). In GOLDN, gene transcript levels of ITGA7 and SLCO2A1 were associated with fasting triglycerides (P = 0.07 and P = 0.02), highlighting functional relevance of our findings.
Conclusion: In this study, we present preliminary evidence of novel rare variant determinants of fasting lipids, and reveal potential underlying molecular mechanisms. Moreover, these results were replicated in an independent cohort. Our findings may inform novel biomarkers of disease risk and treatment targets.
Introduction
The understanding of the biology behind lipid-metabolism has increased exponentially in the past two decades, enabled by the vast interrogation of the human genome. To date, genome-wide association studies have identified over 170 candidate single-nucleotide variants (SNVs), with many residing in common regions of the genome (Teslovich et al., 2010; Do et al., 2013). However, two major limitations exist in the current exploration for causal loci associated with lipid metabolism: (1) Identification of SNVs in non-coding regions or SNVs in large regions that span several candidate genes; and (2) missing detection of candidate genes altogether, particularly if population-specific (Assimes and Quertermous, 2014). Exome-wide association studies naturally expand on findings from genome-wide association studies through their exploration of the functional region of the genome (Majewski et al., 2011).
Exome-wide association studies have been extensively used to dissect the genetic architecture of complex diseases and quantitative traits (Lee et al., 2014). Exonic variants, particularly loss-of-function variants, tend to show the most dramatic effect sizes, yielding the greatest power for detection. Recent evidence on lipid traits provides support that rare variants can be ancestry-specific (Lu et al., 2017). Therefore, examining exonic variants across diverse ancestry groups likely augments the identification of novel loci.
The Genetics of Lipid Lowering Drugs and Diet Network (GOLDN) cohort, recruited from families of European ancestry residing in the United States, provides an opportunity to study the effects of rare genetic variants on clinically measured lipid levels. Prior studies in GOLDN have identified common variants controlling lipid fasting level (An et al., 2014). To augment the discoveries which were limited to common variants and identify additional rare functional loci contributing to variation in fasting plasma lipid level, we performed an exome-wide sequencing study in 894 GOLDN participants to identify novel associations of coding variants and fasting lipid traits. To further explore population-specific effects, we also performed replication analyses in two diverse external validation cohorts: (1) the Heredity and Phenotype Intervention (HAPI) Heart Study, composed of Old Order Amish individuals; and (2) the Hypertension Genetic Epidemiology Network (HyperGEN) and the Genetic Epidemiology Network of Arteriopathy (GENOA), which both recruited African Americans. Finally, we interrogated the functional relevance of our significant findings using DNA methylation and RNA-Seq data available in GOLDN.
Materials and Methods
Study Populations
Genetics of Lipid Lowering Drugs and Diet Network (clinicaltrials.gov-NCT00083369) recruited and sequenced 894 participants from 186 families of European ancestry at two centers (Minneapolis, MN, United States and Salt Lake City, UT, United States) to characterize genetic and epigenetic determinants of lipid levels (Wojczynski et al., 2015). The population size indicates we have statistical power ranging from 0.5 for h2locus = 0.02 to 1.00 for h2locus = 0.05 or above in the single variant test, and from 0.8 for h2locus = 0.02 to 1.00 for h2locus = 0.05 or above in the gene-based test for SNVs with MAF < 0.05. The participants were healthy without diabetes or cardiovascular disease, and they were asked to discontinue any lipid-lowering agents (pharmaceuticals or nutraceuticals) for at least 4 weeks prior to the initial visit. Demographic and clinical characteristics of the study participants are listed in Table 1.
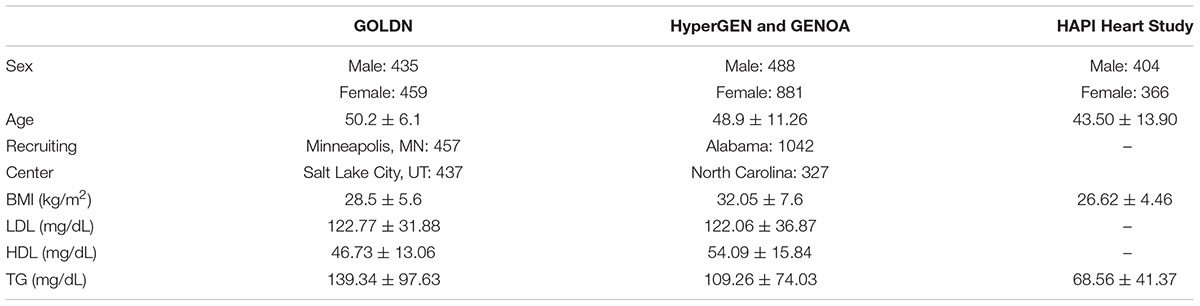
Table 1. Demographic and clinical characteristics of samples in GOLDN, HyperGEN and GENOA, and HAPI Heart Study.
We sought to replicate the significant association in two studies. The effect directions of the associated variants were also compared in different ethnic populations. The first study is HAPI Heart Study consisting of 770 Old Order Amish participants. The triglyceride (TG) levels were relatively low in the physically active Amish population (Mitchell et al., 2008; Table 1). It was allowed to use HAPI Heart Study for replication of the TG findings, not the LDL-C for POT1. The second study, which consisted of 3,183 African Americans from pooled samples of HyperGEN and GENOA, was used to replicate high-density lipoprotein cholesterol (HDL-C), low-density lipoprotein cholesterol (LDL-C), and TG findings. In the replication test, only the top genes found in GOLDN were analyzed. All the exonic variants within these top genes in the replication populations were included in the gene-based test.
Genotype, Phenotype, and Statistical Analysis
The sequencing, genotyping, and quality control procedures were described in a prior study (Geng et al., 2018). Briefly, genomic DNA from peripheral blood nucleated cells was extracted using QIAmp 96 DNA Blood Kits (Qiagen, Hilden, Germany). After Illumina paired end small fragment libraries were constructed, they were run on a HiSeq 2000 V3 2 bp × 101 bp sequencing run. Illumina sequencing data in FASTQ format were aligned to the GRCh37-lite reference sequence using BWA (Li and Durbin, 2010) version 0.5.9. SNVs were called using the Atlas-SNP2 first at the Subject-level and then combined (Challis et al., 2012). Only biallelic mutations were kept after filtered using VCFtools (Danecek et al., 2011). For the genotype level QC, genotypes with read depth less than 20 or genotyping quality less than 30 were excluded. For the variant level QC, the mutations were filtered out if their missing rate exceeded 5%. The project-level VCF was further annotated using ANNOVAR (Wang K. et al., 2010) according to hg19 genome assembly/dbSNP version 138. Four classes of functional variants (splicing, non-synonymous, stop-loss, and stop-gain) on chromosomes 1–22 were used for association tests (Lange et al., 2014). We required that >70% of target bases were covered at >20×; samples below that threshold received additional (top-up) sequencing. To confirm sample purity and identity, we compared high-density SNVarray genotypes (Aslibekyan et al., 2012) (Illumina Omni Express) to the SNV calls, and each sample achieved >90% genotype concordance.
HDL-C, LDL-C, and TG were measured in this study in the fasting state as previously reported (Liu et al., 2008). All the lipid values were natural log transformed to achieve normality of residuals. Genetic associations were assessed using linear mixed models using RAREMETALWORKER and RAREMETAL (version 4.13.6). All the associations were adjusted for sex, age (linear, quadratic, and cubic terms), and recruiting center as fixed effects, and a kinship coefficient was used to adjust for family relatedness as a random effect. Single variant analyses and gene-based analyses were both conducted. For gene-based analyses, sequence kernel association test (SKAT), simple burden test, Madsen and Browning weighted burden test (MB), and variable threshold test (VT) were utilized for rare variants with MAF < 1% (Madsen and Browning, 2009; Price et al., 2010; Wu et al., 2011).
Functional Validation
We also sought to explore the relationships between our top findings and other omic layers using the following GOLDN data: DNA methylation (measured with the Illumina Infinium 450K chip, n = 991) (Irvin et al., 2014) and gene expression (measured with RNA-Seq, n = 100 unrelated participants) as previously described (Sayols-Baixeras et al., 2016). The CpG sites of CD4+ T-cells within the genes containing significantly associated variants and the intergenic CpG sites near these genes were examined to test whether their methylation levels were associated with lipid levels or not. For transcriptional profiling, GOLDN participants were selected from the extremes of the BMI distribution. RNA was extracted from buffy coats using the TRIzol method (Thermo Fisher Scientific, Waltham, MA, United States) and the quality was evaluated using Bioanalyzer (Agilent Technologies, Santa Clara, CA, United States). We also fitted linear mixed models to test for associations of DNA methylation and gene-expression. We hypothesized CD4+ T-cells should reflect underlying epigenetic variation influencing blood lipids. Many key genes (e.g., PPARs) involved in lipid metabolism are expressed in lymphocytes and other immune cells (Chinetti et al., 2000; Bouwens et al., 2008). It was demonstrated that peripheral blood mononuclear cell gene expression profiles reflect nutrition-related metabolic changes. Responsive genes including CPT1, ACAA2, and SCL25A20 were enriched for fatty acid metabolizing enzymes (Bouwens et al., 2007).
Results
Association Test Results
In single variant tests, we found rs11171663 in ITGA7 to be significantly associated with fasting TG levels (P = 7.66E-08), explaining approximately 3.2% of the total trait variance. The 16 participants carrying this variant exhibited significantly higher TG compared with non-carriers (mean of 260 mg/dL in carriers vs. 114 mg/dL in non-carriers, P < 0.05) (Figure 1 and Table 2). In the gene-based test, three genes were significantly associated with lipid traits, specifically ITGA7 (TG, P = 1.77E-07), SLCO2A1 (TG, P = 7.18E-07), and POT1 (LDL-C, P = 3.00E-07). In the ITGA7 gene-based test, the signal was driven by the same variant (rs11171663) identified in the single variant test. The severities of rare variant effects, predicted by PolyPhen2 (Ramensky et al., 2002) or SIFT (Ng and Henikoff, 2003), are listed in Table 2. The Bonferroni-corrected significance thresholds for single variant tests and gene-level tests were P < 5.98E-07 (0.05/83,577 rare variants) and P < 3.44E-06 (0.05/14,521 genes), respectively.
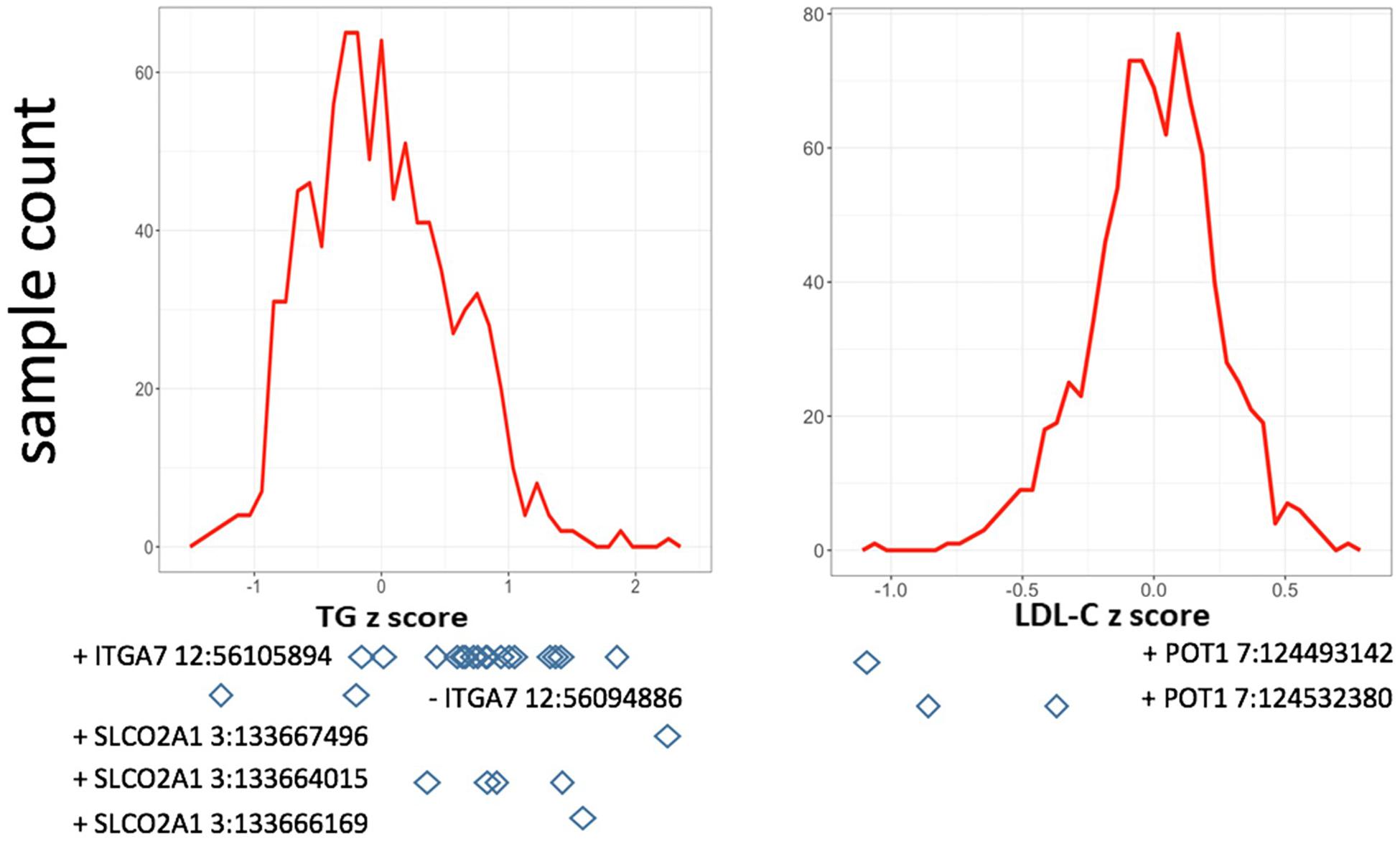
Figure 1. Distribution of residuals from the regression of phenotypes on their covariates. The diamonds indicate corresponding residual values of rare variant carriers. X-axis lists the z-score for residuals; Y-axis represents the sample counts.
Replication Results
In the pooled HyperGEN and GENOA samples, POT1, ITGA7, and SLCO2A1 approached significant associations (P = 0.020, P = 0.036 and 0.082, respectively) in the gene-based test (Supplementary Table 1). After Bonferroni correction, the significant threshold for association in this replication gene-based test is 0.05/3. Among the top single variants in these three genes in GOLDN, only one in ITGA7 (rs11171663) and another one in SLCO2A1 (rs150345667) were shared by HyperGEN and GENOA samples, but both associations failed to replicate (P > 0.05).
Our top TG findings were allowed to be tested for association with the corresponding trait in the HAPI Heart Study, which were recruited from the Amish community of Lancaster County, PA, who are descendants of about 200 original founding individuals. None of the ITGA7 or SLCO2A1 SNVs with P < 0.05 in GOLDN were found in HAPI Heart samples which are from a unique founder population. Variants in these two genes according to single variant test or these two genes according to gene-based test were not significantly associated with TG in HAPI Heart Study.
Functional Validation
Transcripts of all the three top genes were observed from the buffy coats. The significant threshold for functional validation of gene-expression is 0.05/3 for multiple testing. In the gene transcript analysis, the expression levels of SLCO2A1 and ITGA7 approached a significant association with TG (P = 0.02 and P = 0.07, respectively), and POT1 was not significantly associated with LDL-C (P > 0.1). The associations between the methylation status of CpG sites within or neighboring the three significant genes with lipid levels were not statistically significant after Bonferroni correction.
Discussion
Genetic associations with fasting blood lipids have been extensively studied. Here, we sought to expand on that work by examining data from heterogeneous communities to leverage the population-specific nature of rare coding variants, striving for a more thorough understanding of the mechanisms underlying lipid metabolism.
In this study, we present preliminary evidence of rare variant determinants of circulating lipids. Three genes – ITGA7, SLCO2A1, and POT1 – were significantly associated with fasting lipid levels in the discovery cohort. Two of these findings (ITGA7 and POT1) were nominally replicated in the African American HyperGEN/GENOA sample but not in Old Order Amish, likely due to the genetic isolation of the latter. Additionally, the expression level of SLCO2A1 was associated with fasting TG, suggesting regulatory relevance. Importantly, lack of replication in ethnically distinct cohorts supports the population-specific effect of rare variants on lipid traits, particularly because SNVs with P < 0.05 in the top three genes in GOLDN (the discovery cohort) were not found in HAPI Heart at all, and only few were shared with HyperGEN/GENOA.
The three genes harboring novel loci identified in our study have been implicated in lipid homeostasis. SLCO2A1 is a lipid transporter protein, which could inhibit TG accumulation (Gimeno, 2007). ITGA2, an ITGA7 homolog, was reported to be associated with coronary atherosclerosis in the Chinese Han population (Wang Y. et al., 2010), drawing attention to the ITGA gene family in the cardiovascular context. We also observed associations between ITGA7 and LDL-C response to fenofibrate in another GOLDN study (Geng et al., 2018). In the conditional analysis after including LDL-C response to fenofibrate as a covariate, ITGA7 is still significantly associated with fasting TG (P < 0.05). The associations between the top three genes and lipid levels were in part supported by our replication and functional assessments. The putative biological mechanism of POT1 needs further clarification.
In addition to identifying novel associations, we also tested variants within known lipid genes and corresponding traits. In GOLDN, variants within APOE, PCSK9, LDLR, and APOB were nominally associated with fasting LDL-C in single variant association tests (P < 0.05), and PCSK9 and LDLR were nominally associated with fasting LDL-C in gene-based tests (P < 0.05). These findings are consistent with a previous study, which reported associations between LDL-C and APOE, PCSK9, LDLR, APOB, and PNPLA5 (Lange et al., 2014). However, rare variants in PNPLA5 (Lange et al., 2014) were not present in GOLDN and nor was the gene significantly associated with LDL-C, further highlighting the population-specific nature of some genetic determinants of lipids.
Our work also suggests that rare variants may play different roles in different populations. Heterogeneous effects of the same variant across different ethnic groups are due to differences in the genetic background or environment (Lin et al., 2007; Lu et al., 2017). Subjected to natural selection or genetic drift, inter-ancestry differences in the identification of rare coding variants across populations were observed as expected (Lu et al., 2017). In our study, rare variant rs11171663 in ITGA7 was significantly associated with TG in Caucasian samples from GOLDN, but the direction of effect was opposite in African American samples from HyperGEN and GENOA, yet the variant still approached significance (P = 0.053). The hypothesis that this rare variant – or others identified in our study – may be positively or negatively associated with fasting lipid levels depending on the population merits attention in future studies in individuals of diverse ancestry. One limitation of our study is that we did not take the environmental factors for participants into account, which may interact with the variants and account for partial population difference as well.
Conclusion
In this study, we presented preliminary evidence of novel rare variant determinants of fasting lipids, which may inform novel biomarkers of disease risk and treatment targets. We found statistically significant associations between ITGA7 (P = 1.77E-07) and SLCO2A1 (P = 7.18E-07) and triglycerides, as well as between POT1 (P = 3.00E-07) and low-density lipoprotein cholesterol. Replication analyses yielded mixed results: in 3,183 African American samples from HyperGEN and GENOA study, the top genes achieved P-values of 0.04 (ITGA7), 0.08 (SLCO2A1), and 0.02 (POT1), but no associations approached significance in 770 samples from the HAPI Heart Study (P > 0.05), which implies exploration of genetic data from diverse population samples may enhance the identification of novel associations with rare variants. In GOLDN, the P-values for the association between gene transcript levels of ITGA7 and SLCO2A1 and fasting triglycerides were 0.07 and 0.02, respectively, highlighting functional relevance of our findings.
Data Availability
The datasets generated for this study can be found in dbGaP https://www.ncbi.nlm.nih.gov/projects/gap/cgi-bin/study.cgi?study_id=phs000741.v2.p1. The other datasets used and/or analyzed during the current study are available from the corresponding author on reasonable request.
Ethics Statement
Our study has been approved by the appropriate internal review boards at the University of Texas Health Science Center at Houston and all other participating institutions, and it abides by the Declaration of Helsinki principles.
Author Contributions
MI, BH, SA, HT, JO, DA, and DZ designed the study. XG, VS, and DZ analyzed the data. TD, KR, and BM conducted the replication in HAPI heart study. XG, RS, BH, SA, and DZ wrote the manuscript. All authors participated in data interpretation, reviewed the manuscript, and approved the final version of the manuscript.
Funding
The GOLDN study has been funded by the National Institutes of Health (NIH) National Heart, Lung, and Blood Institute (NHLBI) Grants U01HL072524 and R01HL091357. SA is additionally funded by NHLBI K01 HL136700 and JO by the U.S. Department of Agriculture, under agreement Nos. 8050-51000-098-00D and USDA-AFRI 2017-67017-26719. HyperGEN and GENOA have been funded by NIH R01HL055673 and U01HL075572. The HAPI Heart Study was supported by NIH grants U01 HL072515 and P30 DK072488. Whole genome sequencing of Amish subjects was provided by the Trans-Omics for Precision Medicine program through the National Heart, Lung and Blood Institute (NHLBI) and funded by 3R01HL121007-01S1, 3R01HL-117626-02S1, and 3R01HL-120393-02S1. XG and DZ are partially supported by Agriculture and Food Research Initiative Competitive Grant no. 2015-67015-22975 from the USDA National Institute of Food and Agriculture, and USDA Aquaculture Research Program Competitive Grant no. 2014-70007-22395.
Conflict of Interest Statement
The authors declare that the research was conducted in the absence of any commercial or financial relationships that could be construed as a potential conflict of interest.
Supplementary Material
The Supplementary Material for this article can be found online at: https://www.frontiersin.org/articles/10.3389/fgene.2019.00158/full#supplementary-material
References
An, P., Straka, R. J., Pollin, T. I., Feitosa, M. F., Wojczynski, M. K., Daw, E. W., et al. (2014). Genome-wide association studies identified novel loci for non-high-density lipoprotein cholesterol and its postprandial lipemic response. Hum. Genet. 133, 919–930. doi: 10.1007/s00439-014-1435-3
Aslibekyan, S., Goodarzi, M. O., Frazier-Wood, A. C., Yan, X., Irvin, M. R., Kim, E., et al. (2012). Variants identified in a GWAS meta-analysis for blood lipids are associated with the lipid response to fenofibrate. PloS One 7:e48663. doi: 10.1371/journal.pone.0048663
Assimes, T. L., and Quertermous, T. (2014). Study of exonic variation identifies incremental information regarding lipid-related and coronary heart disease genes. Circ. Res. 115, 478–480. doi: 10.1161/CIRCRESAHA.114.304693
Bouwens, M., Afman, L. A., and Müller, M. (2007). Fasting induces changes in peripheral blood mononuclear cell gene expression profiles related to increases in fatty acid β-oxidation: functional role of peroxisome proliferator–activated receptor α in human peripheral blood mononuclear cells–. Am. J. Clin. Nutr. 86, 1515–1523. doi: 10.1093/ajcn/86.5.1515
Bouwens, M., Afman, L. A., and Müller, M. (2008). Activation of peroxisome proliferator-activated receptor alpha in human peripheral blood mononuclear cells reveals an individual gene expression profile response. BMC Genomics 9:262. doi: 10.1186/1471-2164-9-262
Challis, D., Yu, J., Evani, U. S., Jackson, A. R., Paithankar, S., Coarfa, C., et al. (2012). An integrative variant analysis suite for whole exome next-generation sequencing data. BMC Bioinformatics 13:8. doi: 10.1186/1471-2105-13-8
Chinetti, G., Fruchart, J.-C., and Staels, B. (2000). Peroxisome proliferator-activated receptors (PPARs): nuclear receptors at the crossroads between lipid metabolism and inflammation. Inflamm. Res. 49, 497–505. doi: 10.1007/s000110050622
Danecek, P., Auton, A., Abecasis, G., Albers, C. A., Banks, E., DePristo, M. A., et al. (2011). The variant call format and VCFtools. Bioinformatics 27, 2156–2158. doi: 10.1093/bioinformatics/btr330
Do, R., Willer, C. J., Schmidt, E. M., Sengupta, S., Gao, C., Peloso, G. M., et al. (2013). Common variants associated with plasma triglycerides and risk for coronary artery disease. Nat. Genet. 45, 1345–1352. doi: 10.1038/ng.2795
Geng, X., Irvin, M. R., Hidalgo, B., Aslibekyan, S., Srinivasasainagendra, V., An, P., et al. (2018). An exome-wide sequencing study of lipid response to high-fat meal and fenofibrate in Caucasians from the GOLDN cohort. J. Lipid Res. 59, 722–729. doi: 10.1194/jlr.P080333
Gimeno, R. E. (2007). Fatty acid transport proteins. Curr. Opin. Lipidol. 18, 271–276. doi: 10.1097/MOL.0b013e3281338558
Irvin, M. R., Zhi, D., Joehanes, R., Mendelson, M., Aslibekyan, S., Claas, S. A., et al. (2014). Epigenome-wide association study of fasting blood lipids in the genetics of lipid lowering drugs and diet network study. Circulation 130, 565–572. doi: 10.1161/CIRCULATIONAHA.114.009158
Lange, L. A., Hu, Y., Zhang, H., Xue, C., Schmidt, E. M., Tang, Z.-Z., et al. (2014). Whole-exome sequencing identifies rare and low-frequency coding variants associated with LDL cholesterol. Am. J. Hum. Genet. 94, 233–245. doi: 10.1016/j.ajhg.2014.01.010
Lee, S., Abecasis, G. R., Boehnke, M., and Lin, X. (2014). Rare-variant association analysis: study designs and statistical tests. Am. J. Hum. Genet. 95, 5–23. doi: 10.1016/j.ajhg.2014.06.009
Li, H., and Durbin, R. (2010). Fast and accurate long-read alignment with Burrows–Wheeler transform. Bioinformatics 26, 589–595. doi: 10.1093/bioinformatics/btp698
Lin, P.-I., Vance, J. M., Pericak-Vance, M. A., and Martin, E. R. (2007). No gene is an island: the flip-flop phenomenon. Am. J. Hum. Genet. 80, 531–538. doi: 10.1086/512133
Liu, Y., Ordovas, J. M., Gao, G., Province, M., Straka, R. J., Tsai, M. Y., et al. (2008). The SCARB1 gene is associated with lipid response to dietary and pharmacological interventions. J. Hum. Genet. 53, 709–717. doi: 10.1007/s10038-008-0302-2
Lu, X., Peloso, G. M., Liu, D. J., Wu, Y., Zhang, H., Zhou, W., et al. (2017). Exome chip meta-analysis identifies novel loci and East Asian–specific coding variants that contribute to lipid levels and coronary artery disease. Nat. Genet. 49, 1722–1730. doi: 10.1038/ng.3978
Madsen, B. E., and Browning, S. R. (2009). A groupwise association test for rare mutations using a weighted sum statistic. PLoS Genet. 5:e1000384. doi: 10.1371/journal.pgen.1000384
Majewski, J., Schwartzentruber, J., Lalonde, E., Montpetit, A., and Jabado, N. (2011). What can exome sequencing do for you? J. Med. Genet. 48, 580–589. doi: 10.1136/jmedgenet-2011-100223
Mitchell, B. D., McArdle, P. F., Shen, H., Rampersaud, E., Pollin, T. I., Bielak, L. F., et al. (2008). The genetic response to short-term interventions affecting cardiovascular function: rationale and design of the Heredity and Phenotype Intervention (HAPI) heart study. Am. Heart J. 155, 823–828. doi: 10.1016/j.ahj.2008.01.019
Ng, P. C., and Henikoff, S. (2003). SIFT: predicting amino acid changes that affect protein function. Nucleic Acids Res. 31, 3812–3814. doi: 10.1093/nar/gkg509
Price, A. L., Kryukov, G. V., de Bakker, P. I., Purcell, S. M., Staples, J., Wei, L.-J., et al. (2010). Pooled association tests for rare variants in exon-resequencing studies. Am. J. Hum. Genet. 86, 832–838. doi: 10.1016/j.ajhg.2010.04.005
Ramensky, V., Bork, P., and Sunyaev, S. (2002). Human non-synonymous SNPs: server and survey. Nucleic Acids Res. 30, 3894–3900. doi: 10.1093/nar/gkf493
Sayols-Baixeras, S., Subirana, I., Lluis-Ganella, C., Civeira, F., Roquer, J., Do, A., et al. (2016). Identification and validation of seven new loci showing differential DNA methylation related to serum lipid profile: an epigenome-wide approach. The REGICOR study. Hum. Mol. Genet. 25, 4556–4565. doi: 10.1093/hmg/ddw285
Teslovich, T. M., Musunuru, K., Smith, A. V., Edmondson, A. C., Stylianou, I. M., Koseki, M., et al. (2010). Biological, clinical and population relevance of 95 loci for blood lipids. Nature 466, 707–713. doi: 10.1038/nature09270
Wang, K., Li, M., and Hakonarson, H. (2010). ANNOVAR: functional annotation of genetic variants from high-throughput sequencing data. Nucleic Acids Res. 38:e164. doi: 10.1093/nar/gkq603
Wang, Y., Fu, W., Xie, F., Wang, Y., Chu, X., Wang, H., et al. (2010). Common polymorphisms in ITGA2, PON1 and THBS2 are associated with coronary atherosclerosis in a candidate gene association study of the Chinese Han population. J. Hum. Genet. 55, 490–494. doi: 10.1038/jhg.2010.53
Wojczynski, M. K., Parnell, L. D., Pollin, T. I., Lai, C. Q., Feitosa, M. F., O’Connell, J. R., et al. (2015). Genome-wide association study of triglyceride response to a high-fat meal among participants of the NHLBI Genetics of Lipid Lowering Drugs and Diet Network (GOLDN). Metabol. Clin. Exp. 64, 1359–1371. doi: 10.1016/j.metabol.2015.07.001
Keywords: whole exome sequencing, rare variant, HDL, LDL, triglyceride, cholesterol, genetics, epidemiology
Citation: Geng X, Irvin MR, Hidalgo B, Aslibekyan S, Srinivasasainagendra V, An P, Frazier-Wood AC, Tiwari HK, Dave T, Ryan K, Ordovas JM, Straka RJ, Feitosa MF, Hopkins PN, Borecki I, Province MA, Mitchell BD, Arnett DK and Zhi D (2019) An Exome-Wide Sequencing Study of the GOLDN Cohort Reveals Novel Associations of Coding Variants and Fasting Plasma Lipids. Front. Genet. 10:158. doi: 10.3389/fgene.2019.00158
Received: 15 July 2018; Accepted: 13 February 2019;
Published: 26 February 2019.
Edited by:
Daniel Shriner, National Human Genome Research Institute (NHGRI), United StatesReviewed by:
Sarah Buxbaum, Jackson State University, United StatesMaris Alver, University of Tartu, Estonia
Copyright © 2019 Geng, Irvin, Hidalgo, Aslibekyan, Srinivasasainagendra, An, Frazier-Wood, Tiwari, Dave, Ryan, Ordovas, Straka, Feitosa, Hopkins, Borecki, Province, Mitchell, Arnett and Zhi. This is an open-access article distributed under the terms of the Creative Commons Attribution License (CC BY). The use, distribution or reproduction in other forums is permitted, provided the original author(s) and the copyright owner(s) are credited and that the original publication in this journal is cited, in accordance with accepted academic practice. No use, distribution or reproduction is permitted which does not comply with these terms.
*Correspondence: Xin Geng, xin.geng@uth.tmc.edu; gxouc@hotmail.com Donna K. Arnett, donna.arnett@uky.edu Degui Zhi, Degui.Zhi@uth.tmc.edu