Erratum: Construction of a Suite of Computable Biological Network Models Focused on Mucociliary Clearance in the Respiratory Tract
- PMI R&D, Philip Morris Products S.A., Neuchâtel, Switzerland
Mucociliary clearance (MCC), considered as a collaboration of mucus secreted from goblet cells, the airway surface liquid layer, and the beating of cilia of ciliated cells, is the airways’ defense system against airborne contaminants. Because the process is well described at the molecular level, we gathered the available information into a suite of comprehensive causal biological network (CBN) models. The suite consists of three independent models that represent (1) cilium assembly, (2) ciliary beating, and (3) goblet cell hyperplasia/metaplasia and that were built in the Biological Expression Language, which is both human-readable and computable. The network analysis of highly connected nodes and pathways demonstrated that the relevant biology was captured in the MCC models. We also show the scoring of transcriptomic data onto these network models and demonstrate that the models capture the perturbation in each dataset accurately. This work is a continuation of our approach to use computational biological network models and mathematical algorithms that allow for the interpretation of high-throughput molecular datasets in the context of known biology. The MCC network model suite can be a valuable tool in personalized medicine to further understand heterogeneity and individual drug responses in complex respiratory diseases.
Introduction
The respiratory tract is under constant challenge to provide the body with oxygen while monitoring air quality for pollutants and microorganisms. The mucous membranes in the airways, which are lined with microtubule-based projections, the cilia, represent a powerful first-line defense. In response to irritants and infection, mucus is secreted by goblet cells, and cilia on the surface of ciliated cells move mucus upward in coordinated waving and beating motions. Eventually, particles are expelled through sneeze and cough (Wanner et al., 1996). This self-clearing mechanism, mucociliary clearance (MCC), ensures proper functioning of the respiratory tract.
Cilia have attracted increasing attention because of the growing number of diseases caused by mutations in genes that impact cilium assembly, function, and turnover (Fliegauf et al., 2007; Kempeneers and Chilvers, 2018). Traditionally, cilia are classified as primary or motile (Wheatley, 1995; Satir and Christensen, 2007). Primary cilia are present on almost all cell types and are involved in tissue homeostasis (Gerdes et al., 2009; Nozawa et al., 2013). Motile cilia often occur as clusters of several hundred protrusions covering cells and direct fluid flow (Choksi et al., 2014).
Cilia assembly and resorption often depend on the cell cycle (Kim and Tsiokas, 2011), with a neatly interwoven mode of regulation assuring timely and developmentally precise control of cilium biogenesis. The regulatory factor X (RFX) family of transcription factors is a key regulator of both primary and motile cilia assembly programs (reviewed in Thomas et al., 2010; Choksi et al., 2014). A master regulator of motile cilia assembly across the vertebrates is forkhead box J (FOXJ1), a member of the forkhead/winged-helix family of transcription factors (Murphy et al., 1997; Chen et al., 1998; Brody et al., 2000), which is under control of multiciliate differentiation and DNA synthesis-associated cell cycle protein (MCIDAS) and geminin coiled-coil domain-containing (GMNC) protein in the respiratory epithelium (Stubbs et al., 2012; Arbi et al., 2016). Mutations in MCIDAS and its downstream effector cyclin O are implicated in an MCC disorder known as reduced generation of multiple motile cilia (RGMC) (Boon et al., 2014). In RGMC patients, cilia numbers are reduced, resulting in impaired MCC, airway obstruction, and recurring respiratory infections.
An alternative mechanism of ciliary regulation is the disassembly of the organelle by aurora A kinase (AURKA), which also regulates the entry into mitosis (Pan et al., 2004; Pugacheva et al., 2007). AURKA phosphorylates histone deacetylase 6 (HDAC6), stimulating HDAC6-dependent deacetylation of axonemal microtubules (Hubbert et al., 2002), destabilization of the ciliary shaft, and subsequent collapse of the cilium.
Exposure to air pollutants, cigarette smoke, drugs, or infectious agents can affect ciliary beating frequency (CBF) (Workman and Cohen, 2014; Yaghi and Dolovich, 2016). On the molecular level, CBF increases in response to high mucus viscosity (Fernandes et al., 2008) and fluctuations in the levels of second messengers, such as cyclic adenosine 3′,5′-mono- phosphate (cAMP), cyclic guanidine 3′,5′-mono- phosphate (cGMP), intracellular Ca2+, calmodulin, nitric oxide (Jain et al., 1993; Korngreen and Priel, 1996; Yang et al., 1996; Wyatt et al., 1998; Zagoory et al., 2001, 2002), and intracellular pH (Sutto et al., 2004). Mechanistically, CBF increases as a result of cAMP- and cGMP-mediated activation of respective protein kinases via Ca2+ release or by a calcium-independent mechanism.
While mucus secretion is a normal defense response, mucin synthesis in goblet cells and mucus secretion are amplified in respiratory diseases such as asthma or chronic obstructive pulmonary disease (COPD). In addition, the number of goblet cells can increase by proliferation (hyperplasia) and by airway epithelial cell transdifferentiation (metaplasia), further contributing to increased mucus production (Blyth et al., 1998; Rogers, 2007; Turner and Jones, 2009; Boucherat et al., 2013; Ramos et al., 2014). This airway epithelial remodeling decreases ciliated cell numbers and ciliary beating efficiency, reducing MCC and aggravating airway plugging (Nini et al., 2012; Yaghi et al., 2012; Yaghi and Dolovich, 2016).
There is overwhelming evidence that oxidative stress and oxidative damage play a pivotal role in the pathogenesis of COPD (Rahman and MacNee, 1999; Rahman and Adcock, 2006; Anderson and Macnee, 2009; Kim and Criner, 2015; Matera et al., 2016). Oxidative stress is a well-described trigger of the epidermal growth factor receptor (EGFR) signaling pathway that leads to mucus hypersecretion (Takeyama et al., 1999, 2001; Perrais et al., 2002; Hewson et al., 2004; Casalino-Matsuda et al., 2006; Hao et al., 2014). We recently published an adverse outcome pathway that describes the events that follow oxidative stress-mediated EGFR activation to goblet cell hyperplasia/metaplasia and decreased lung function following mucus overproduction (Luettich et al., 2017).
Signaling downstream of interleukin (IL) 13 is involved in the pathogenesis of asthma (Wills-Karp, 2004). The IL13 receptor complex initiates several cascades of molecular events that result in goblet cell metaplasia/hyperplasia. One important downstream effector of IL13 is the sterile alpha motif pointed domain-containing ETS transcription factor (SPDEF), which is directly involved in mucin gene expression (Park et al., 2007; Chen et al., 2009).
The vast volume and diversity of biological data available on cilium assembly, CBF, and goblet cell hyperplasia/metaplasia require that the information be integrated for better visualization and understanding of the processes that underlie respiratory diseases. Biological network models offer a framework for understanding biological processes and diseases and aid in drawing new, often unpredicted conclusions. Over the years, we have built several causal biological network (CBN) models that capture biological processes that are impacted in COPD. These models, stored in the CBN database, are emerging as an innovative and powerful tool to quantify the impact of exposure or potentially affected biological processes in disease (Cho et al., 2012; Martin et al., 2014; Boue et al., 2015; Talikka et al., 2017). The major advantage of the CBN approach is that it transforms unstructured data into interconnected and organized knowledge that describes biological processes precisely and accurately (Schlage et al., 2011; Westra et al., 2011, 2013; Cho et al., 2012; Gebel et al., 2013; De Leon et al., 2014; Martin et al., 2014; Szostak et al., 2016).
In this study, we present a suite of causal biological models that describe important molecular events involved in MCC, from cilium assembly to ciliary beating, goblet cell hyperplasia/metaplasia, and mucus hypersecretion. We also show how transcriptomic data are scored onto these network models and how the models can provide mechanistic understanding of gene expression changes.
Materials and Methods
Literature Curation
Biological Expression Language (BEL)1 version 1.0 is used for scientific text curation. BEL is a computable language that converts causal and correlative biological observations to statements consisting of two biological entities connected by a relationship predicate1. Relevant original research articles for curation were identified from pertinent review articles in the field. The journal impact factor or any other means to rank the publications was not considered. If the statements in the original research articles were sufficiently supported by the results presented in figures, the information was considered reliable and captured. To retrieve causal relationships the result sections were extracted from these articles for curation. The introduction, discussion and conclusion sections were avoided because the evidences therein largely contain data from earlier studies, repetition of the results, hypotheses and assumptions. Although several evidences supporting an interaction would provide more confidence on the edge, we capture the interactions even when a single experiment is provided in the literature, in order to not omit the relevant information. Contradicting statements were captured without preferential treatment and with proper annotations (model organism, tissue, cell line, treatment/disease, experimental setup). The experimental information from the relevant peer-reviewed scientific articles is semi-automatically processed through the BEL Information Extraction workFlow (BELIEF) platform (Szostak et al., 2015, 2016). BELIEF contains a text-mining software that recognizes biological terms in the text and assembles them into BEL statements. The curation interface allows review, correction, and annotations (cell/tissue type, disease if applicable, species, and experimental design) of the statements that BELIEF proposes. The literature curation is an iterative process. After the curation of initial articles, a gap analysis is performed, and more literature is identified based on gaps in the network models.
Network Model Assembly and Visualization
BEL statements are then compiled to generate a cohesive knowledge assembly model using the OpenBEL framework 3.0.0, an open source compilation framework. The network model consists of nodes that are the biological entities in the network models connected by edges (i.e., the relationships between the biological entities). Any RNA nodes are removed from the model backbone and used in the downstream layer for model scoring as described in Martin et al. (2014). The Cytoscape web application2 is used to visualize and analyze the network properties (Shannon et al., 2003). Cytoscape supports powerful visual mapping whereby biological entities are depicted as defined-shaped nodes connected by the relationship edges. The network visualization is used also during the curation process to identify the gaps and to trim the network models. The trimming here means that any nodes that are “hanging” and do not lead to a biological process described in the model are removed, or further curation is performed to add molecular relationships to connect such nodes to the biological process.
The network model suite is available in the CBN database. The NPA algorithm as well as some measurable “downstream” relationships (backbone node to mRNA) can be downloaded as R packages from the GitHub project pages https://github.com/pmpsa-hpc/NPA and https://github.com/pmpsa-hpc/NPAModels.
Network Model Scoring
The network perturbation amplitude (NPA) methodology is used to obtain a quantitative assessment of how each of the models interprets the transcriptomic changes in the datasets we selected (GSE22430, GSE37693, and GSE5264). This methodology allows for the translation of gene expression fold-changes to differential values for each network node as well as enabling a network-level summary to provide a quantitation of the degree of network model perturbation (Hoeng et al., 2012; Martin et al., 2014; Sewer et al., 2015; Szostak et al., 2016). Raw data were obtained from Gene Expression Omnibus (GEO) repository and normalized following a standard pipeline based on robust multiarray normalization implemented in the R environment for statistical computing (Smyth, 2004). The differential expression values and statistics were calculated using the Bioconductor LIMMA package with appropriate experimental comparisons. “O” and “K” statistics was used to test the specificity of the network models (including the “downstream edges” that connect the network nodes to gene differential expression nodes according to the underlying reverse-causal concept; Catlett et al., 2013). They compare the actual NPA value to the distributions of alternative NPA values obtained by permuting the edges of the networks (the connections between nodes for “K” and the connection between nodes and gene differential expression nodes for “O”). If the actual NPA value is significantly different from these “background” non-biological values, then we consider it as significantly specific.
The leading node analysis allows to focus on a fewer number of nodes in the network by ranking the nodes based on their contribution (%). Using an empirical 80% collective contribution instead of the actual rank, does not limit the number of the nodes, when the contribution of several nodes is almost equal (Martin et al., 2014).
Results
Model Description
Cilium Assembly Model
The cilium assembly network model is a collection of intertwined biological entities and processes that are supported by 59 relevant peer-reviewed articles. The network contains 209 nodes and 319 edges that represent relationships between nodes (Figure 1). When the connections between the nodes in the network were analyzed, many poorly connected nodes and a few highly connected ones, “hubs,” were observed. The most connected node (63 indegree edges) was the biological process “cilium assembly,” and the transcription factor FOXJ1, which is downstream of MCIDAS and GMNC, had the most outdegree edges (Figure 1).
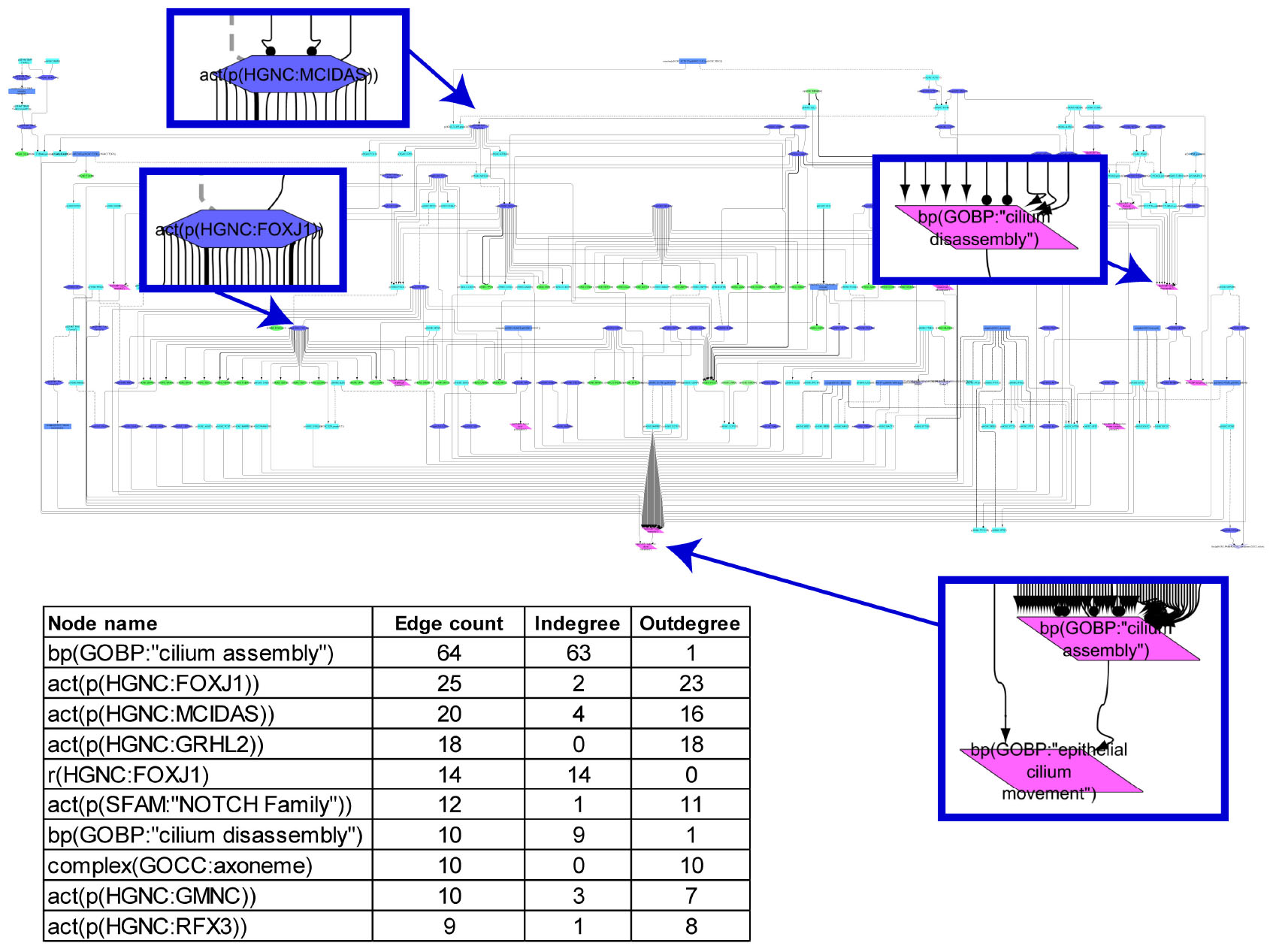
Figure 1. Causal biological network model for cilium assembly. The table shows the top 10 highly connected nodes and their degrees of distribution. The vocabulary for the BEL is provided in http://www.openbel.org/. The Cytoscape layout is the Yfiles hierarchical layout. The network model can be downloaded from causalbionet.com.
A number of pathways, including delta-like canonical notch ligand (DLL)/NOTCH (through MCIDAS/GMNC), smoothened/hedgehog, and grainyhead-like transcription factor 2, converge into a FOXJ1/RFX module that triggers cilium assembly in the network model. This shows a high level of cooperativity between FOXJ1 and RFX factors; FOXJ1 can induce RFX2 and RFX3 expression, FOXJ1 gene expression is partially dependent on RFX3 activity, and a subset of FOXJ1 and RFX target genes overlap (Figure 1). This assures timely and developmentally precise control of cilium biogenesis. In addition, numerous molecules and complexes necessary for structural integrity of cilia, such as the axoneme constituents, BBSome complex (structural components of the basal body), and exocyst complex (membrane transport to cilium), support the “cilium assembly” hub as immediate neighbor nodes. With regard to the “cilium disassembly” hub, as expected, the AURKA-HDAC6 axis and their upstream regulators emerged as a supporting subnetwork.
Ciliary Beating Model
The ciliary beating network model was computed from 52 articles and comprises 80 nodes and 137 edges. The network illustrates the path from various stimuli through intermediate signaling molecules converging into consecutive biological processes, with “mucociliary clearance” as the final node (Figure 2). “Epithelial cilium movement” has the most inward connections in the network, and adenosine triphosphate has the most outward connections. Calcium and “nitric oxide synthase family” are central hubs in the network, with several incoming and outgoing edges. The model shows the CBF increases as a result of cAMP- and cGMP-mediated activation of the respective protein kinases through Ca2+ release or by a calcium-independent mechanism. The model also captures cystic fibrosis transmembrane conductance regulator, whose activation triggers the adenylate cyclase (ADCY)/cAMP pathway. Several other stimuli, such as serotonin or macrophage-stimulating protein, via corresponding receptors (HTR and MST1R, respectively), lead to increased ciliary motion in the model. Another level of regulation is added through sex hormone-dependent modulation, such as progesterone-mediated decreases or estrogen-mediated increases in CBF.
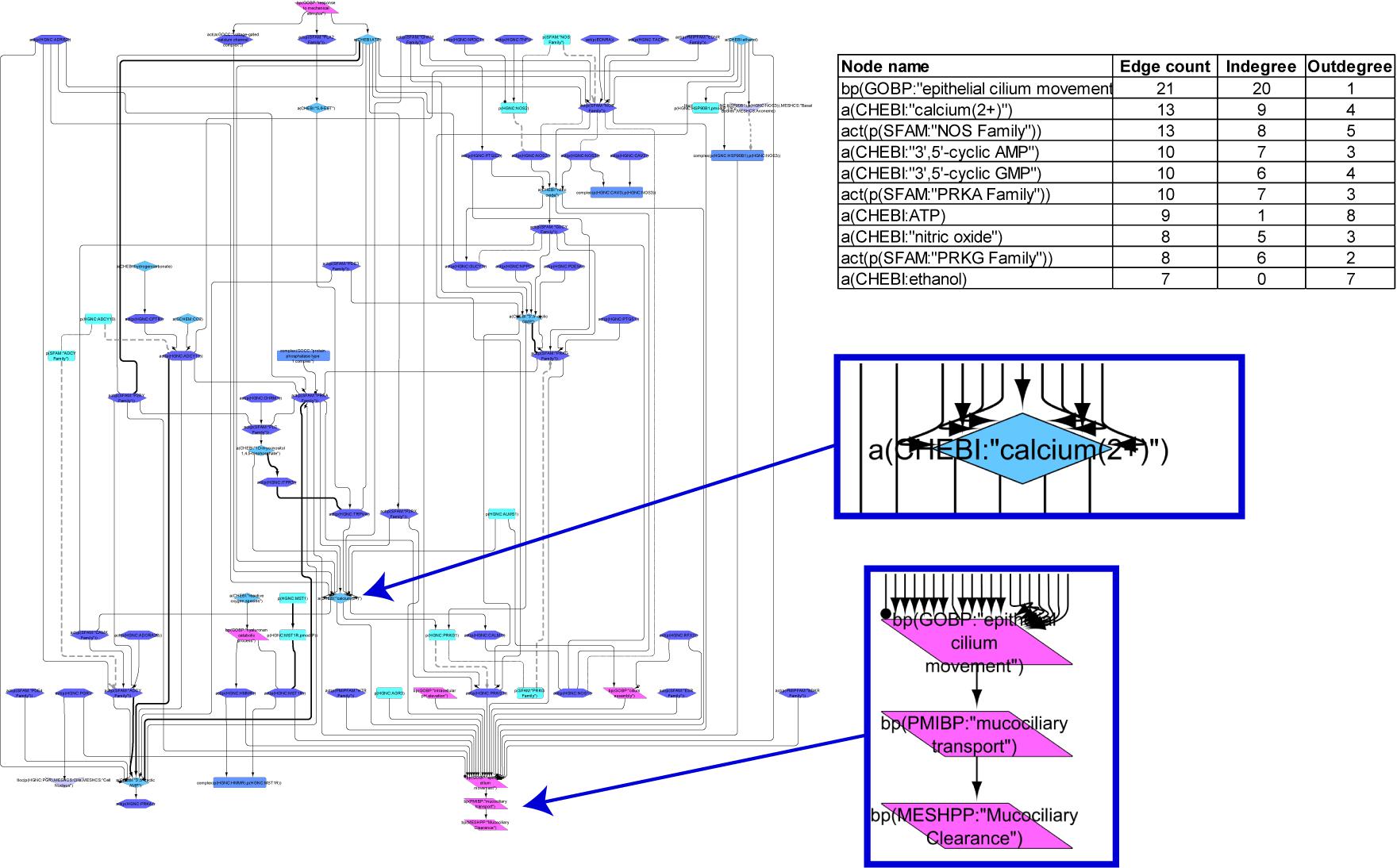
Figure 2. Causal biological network model for ciliary beating. The table shows the top 10 highly connected nodes and their degrees of distribution. The vocabulary for the BEL is provided in http://www.openbel.org/. The Cytoscape layout is the Yfiles hierarchical layout. The network model can be downloaded from causalbionet.com.
Goblet Cell Hyperplasia/Metaplasia Model
The goblet cell hyperplasia/metaplasia model covers 172 nodes and 335 edges that were obtained from 58 articles. The hierarchical view of the network model clearly indicates that, as expected, the biological process “mucus secretion” is the endpoint of the model (Figure 3). The network model hinges on EGFR and IL signaling pathways (Figure 3). An array of growth factors such as epidermal growth factor, transforming growth factor, tumor necrosis factor, amphiregulin, IL4, IL6, IL7, IL8, and IL13 initiate goblet cell-specific mucus secretion by activating their respective receptors (EGFR and IL6R, IL13R, IL17R) and subsequent signaling events, notably through Ras/Raf/mitogen-activated protein kinase kinase/mitogen-activated protein kinase (MAPK)/extracellular signal-regulated kinase 1/2 (ERK1/2) or janus kinase/signal transducer and activator of transcription/SPDEF effectors, modulating mucin gene expression. Multiple additional factors leading to mucus hypersecretion and their interactions are also depicted in the network model. FOXA2 transcription factor, in contrast, limits goblet cell differentiation in the lung and directly represses mucin gene expression. The network displays the inhibition of FOXA2 by EGFR and IL13 pathways that results in goblet cell hyperplasia and mucus secretion.
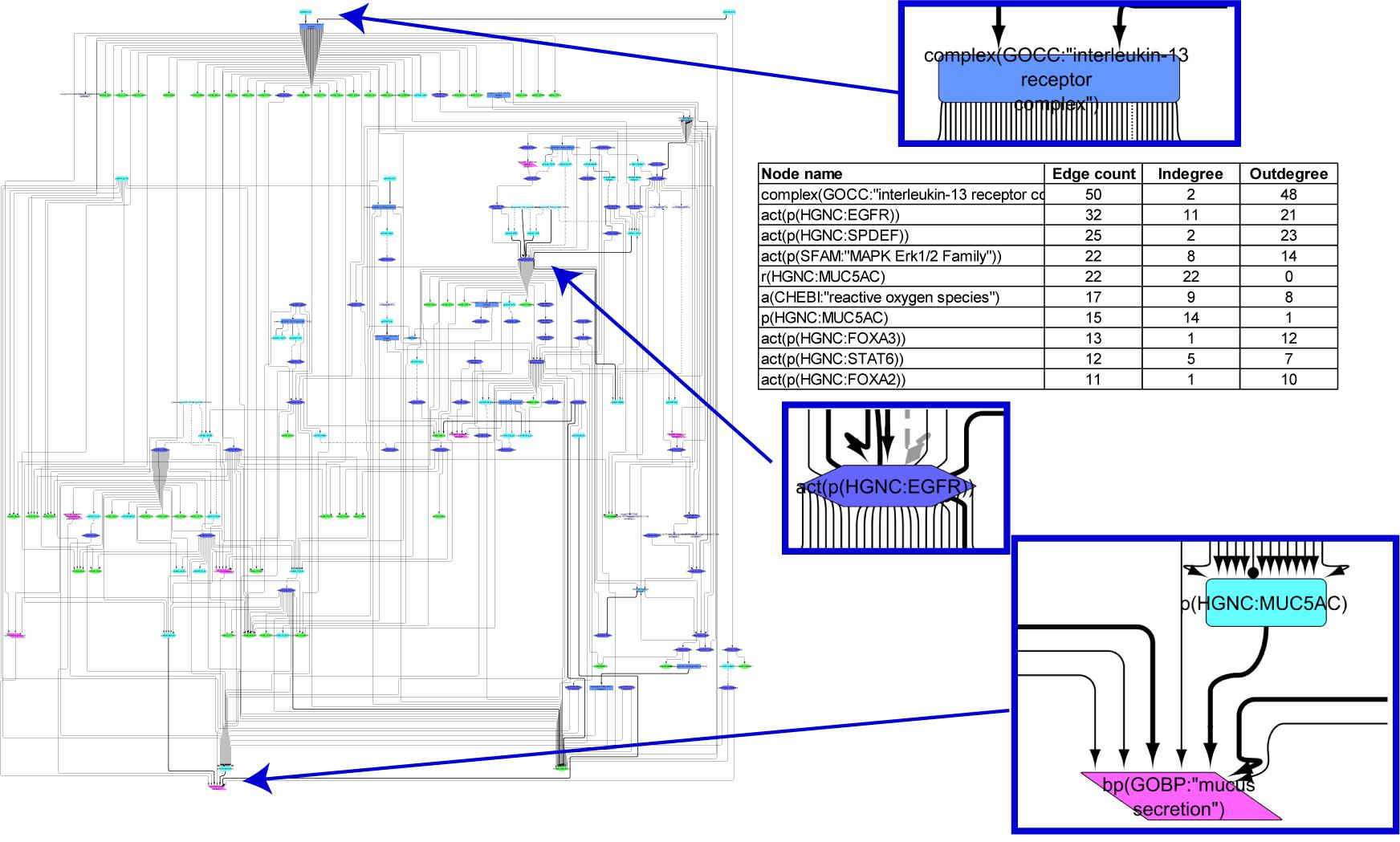
Figure 3. Causal biological network model for goblet cell hyperplasia/metaplasia. The table shows the top 10 highly connected nodes and their degrees of distribution. The vocabulary for the BEL is provided in http://www.openbel.org/. The Cytoscape layout is the Yfiles hierarchical layout. The network model can be downloaded from causalbionet.com.
Model Scoring With Transcriptomic Data
NPA
Network scoring with transcriptomic data is based on the inference of activities of the molecular entities in the network from gene expression changes. This backward reasoning employs a downstream layer with information on gene expression changes known to be induced by the backbone entities (Martin et al., 2014). To test the ability of the MCC network models to provide a quantitative measure of MCC, we identified publicly available datasets in Gene Expression Omnibus. The first dataset selected for model scoring (GSE22430) was from lung epithelial cells treated with the redox-active toxin pyocyanin from Pseudomonas aeruginosa that stimulates EGFR (Rada et al., 2011). Dataset GSE5264 was derived from an in vitro experiment, in which airway epithelial cells were allowed to differentiate to a pseudostratified epithelium at the air-liquid interface (Ross et al., 2007). Finally, we used a transcriptomic dataset from IL13-treated human airway epithelial cells (GSE37693) (Alevy et al., 2012).
The cilium assembly network model responded strongly to the treatment of lung cells with pyocyanin and to the time-course of bronchial epithelial cell differentiation with increasing amplitude over time. There was no impact on the models in response to the IL13 treatment (Figure 4). When the same datasets were used to score the cilia beating network models, the largest amplitude of network perturbation was observed in response to pyocyanin treatment of lung cells (Figure 4). Similar to the cilium assembly model, the amplitude of cilia beating network perturbation increased with advanced mucociliary differentiation, and the model did not respond to the IL13 treatment. The scoring of the goblet cell hyperplasia/metaplasia network model again showed a very strong response to the pyocyanin treatment and, to a lesser extent, to mucociliary differentiation of airway cells. This model responded to IL13 treatment (Figure 4).
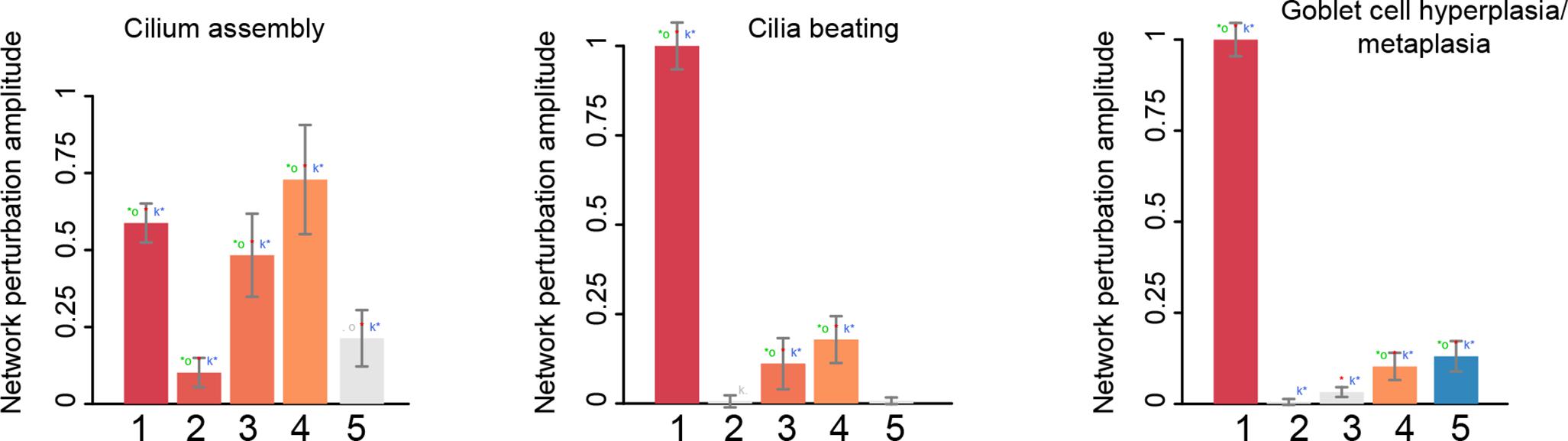
Figure 4. The network perturbation amplitude (NPA). The NPA scores are shown with their confidence interval, accounting for experimental variation. The red star indicates that NPA is statistically different from 0. In addition, companion statistics derived to inform on the specificity of the NPA score with respect to the network structure are shown as ∗O and K∗, respectively, if their p-values are below the significance level of 0.05, and by O and K when the corresponding p-values are between 0.05 and 0.1. ∗ indicates O and K statistic p-values below 0.05 (in color), O and K p-values between 0.05 and 0.1 (in gray). Lanes: 1. GSE22430, pyocyanin-treated vs. control; 2. GSE5264, early time points of mucociliary differentiation in human airway epithelial cells; 3. GSE5264, intermediate time points of mucociliary differentiation in human airway epithelial cells; 4. GSE5264, late time points of mucociliary differentiation in human airway epithelial cells; 5. GSE37693, IL13-treated vs. control.
Leading Node Analysis
To investigate the mechanistic foundation underlying the perturbations of the network models from transcriptomic data and to further validate the biology in the models, we used the leading node analysis (Martin et al., 2014). Leading nodes are the entities in the network models upon which the impact contributes 80% of the observed effect on the network as a whole. Leading node analysis also allows for the assessment of the directionality (activation or inhibition) of the inferred effect on each node. All leading nodes for all contrasts and models are provided in Supplementary Data Sheets S1–S3.
Cilium assembly model
Figure 5 shows the leading node analysis of the cilium assembly network model scored with transcriptomic data from early, intermediate, and late time points of human airway epithelial cell mucociliary differentiation. At the early time point, bone morphogenic protein (BMP) signaling was inferred to be upregulated. The mechanistic target of rapamycin (mTOR), platelet-derived growth factor A (PDGFA), and protein kinase B (AKT) signaling were inferred to be downregulated, in contrast with the inferred upregulation of cilium assembly. At the same time, NudE neurodevelopment protein 1 like 1 (NDEL1) was inferred to be downregulated, resulting in downregulation of cyclin A2 (CCNA2) and cell cycle arrest. At the intermediate and late time points of mucociliary differentiation, BMP signaling was no longer inferred to be upregulated. Instead, DLL1/NOTCH1 signaling was inferred to be downregulated, resulting in an increase in MCIDAS and FOXJ1, the master transcription factors required for the formation of motile cilia. RFX3, also known to induce FOXJ1, was inferred to be upregulated at the intermediate and late time points. PDGFA, mTOR, AKT, and NDEL1/CCNA2 continued to be downregulated in the leading node analysis.
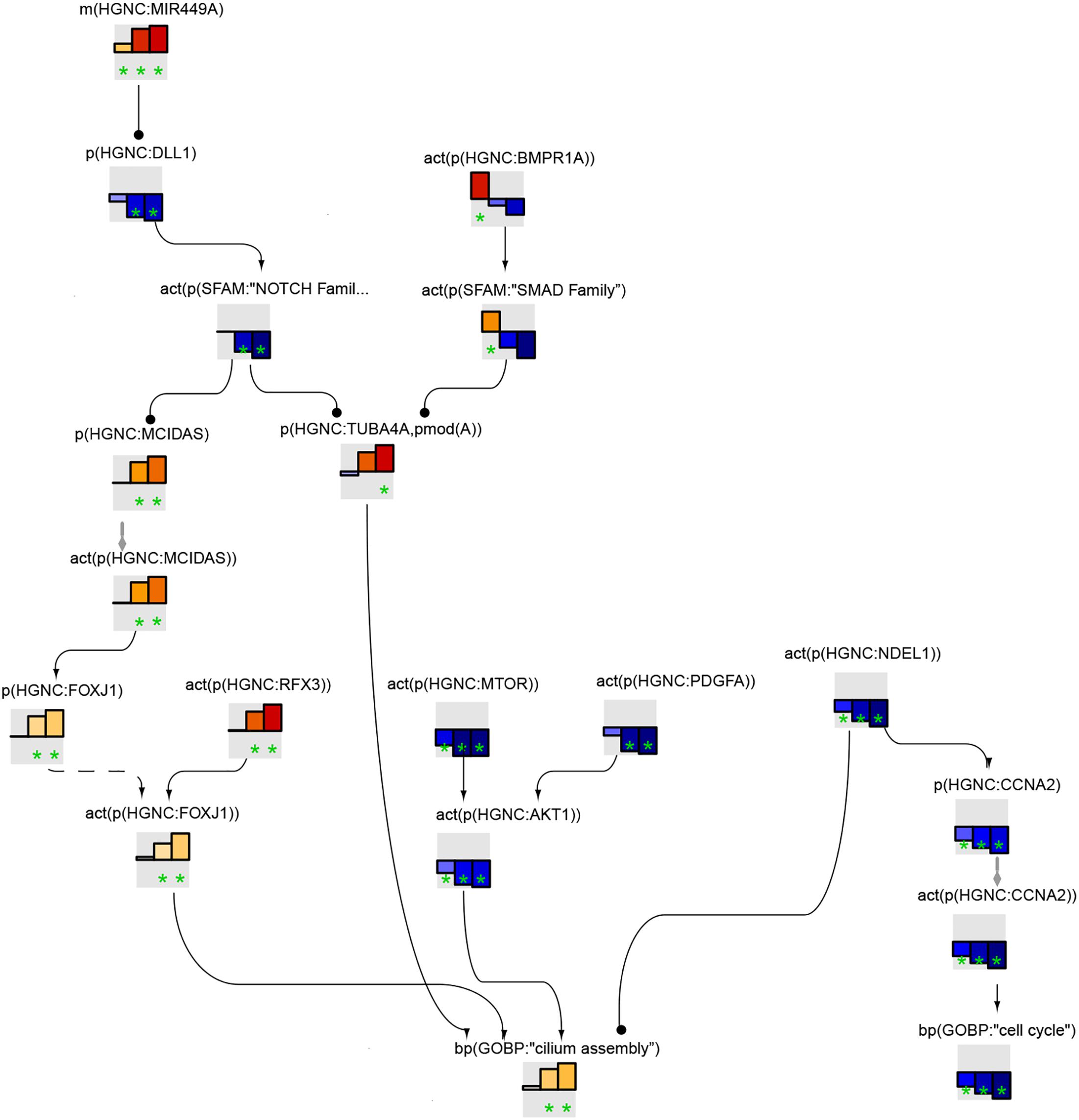
Figure 5. Cilium assembly subnetwork based on leading nodes. The graphical representation shows an example of connected leading nodes common for early (first lane), intermediate (second lane), and late (third lane) time points of human airway epithelial cell mucociliary differentiation. The top 10 leading nodes were prioritized and connected with other leading nodes from the analysis. The backbone NPA values with directionalities of inferred regulation are shown as bar graphs for each node. Orange/red bars indicate inferred upregulation and blue bars indicate inferred downregulation. The green asterisk indicates that the node is a leading node. The vocabulary for the BEL is provided in http://www.openbel.org/.
The leading node analysis of the pyocyanin treatment data scored on the cilium assembly network model indicated the upregulation of PDGFA and downregulation of BMP signaling (Supplementary Data Sheet S1).
Ciliary beating model
Figure 6 shows the leading node analysis of the ciliary beating network model scored with transcriptomic data from early, intermediate, and late time points of human airway epithelial cell mucociliary differentiation. The β2-adrenergic receptor/ADCY signaling pathway, leading to an increase in cAMP levels and subsequent Ca2+ increase via the activation of the PRKA family, was inferred to be upregulated. The analysis also inferred the activation of cGMP-dependent protein kinase 1 (PRKG1). In addition, the leading node analysis of the pyocyanin treatment data scored on the ciliary beating network model indicated the upregulation of ADCY and calcium signaling (Supplementary Data Sheet S2).
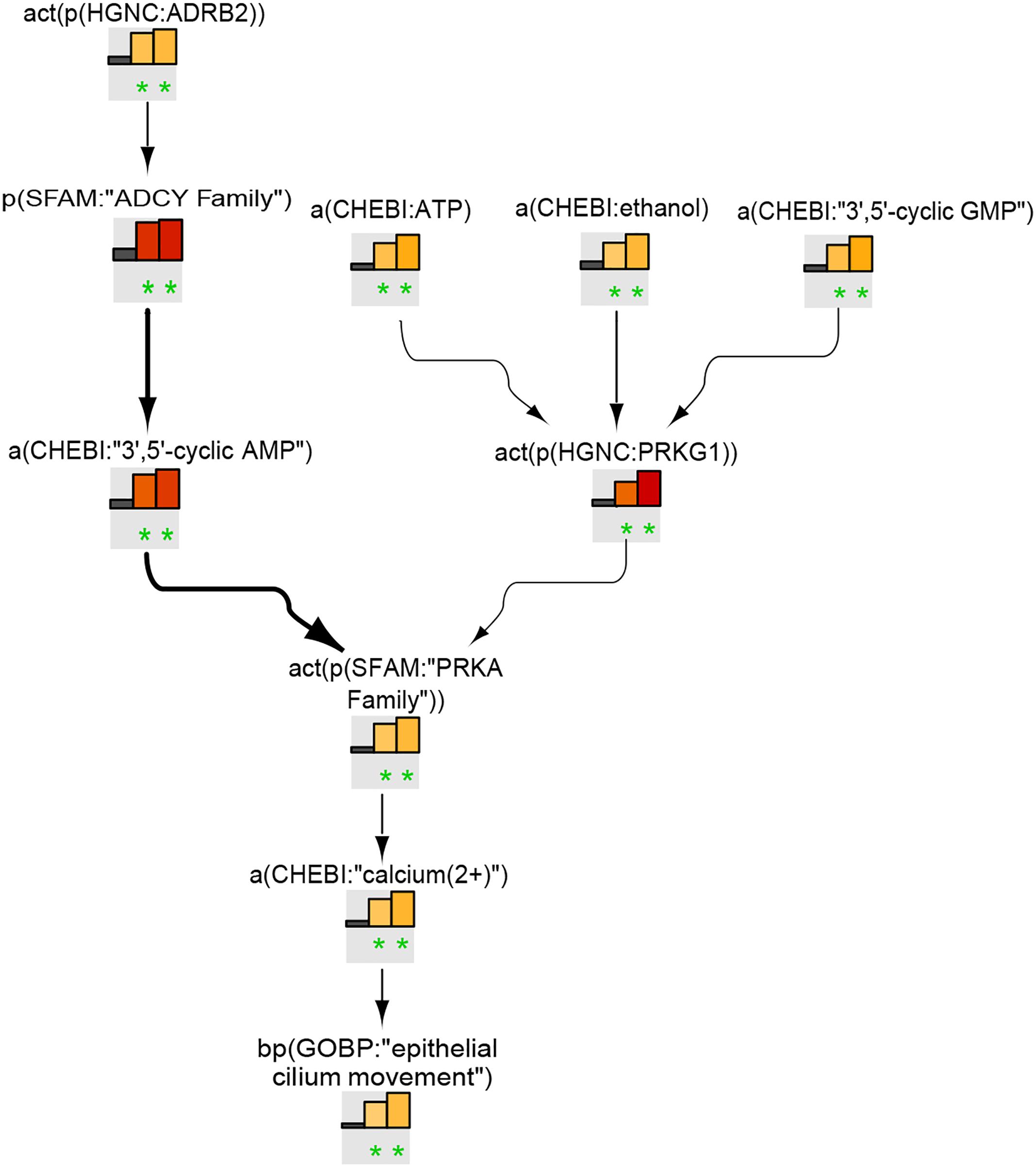
Figure 6. Ciliary beating subnetwork based on leading nodes. The graphical representation shows an example of connected leading nodes common for early (first lane), intermediate (second lane), and late (third lane) time points of human airway epithelial cell mucociliary differentiation. The top 10 leading nodes were prioritized and connected with other leadin g nodes from the analysis. The backbone NPA values with directionalities of inferred regulation are shown as bar graphs for each node. Orange/red bars indicate inferred upregulation and blue bars indicate inferred downregulation. Bold edges indicate “direct regulation.” The green asterisk indicates that the node is a leading node. The vocabulary for the BEL is provided in http://www.openbel.org/.
Goblet cell hyperplasia/metaplasia model
Figure 7 shows the leading-node analysis of the goblet cell hyperplasia/metaplasia model with the pyocyanin and IL13 datasets. The levels of reactive oxygen species (ROS) were inferred to increase with subsequent activation of EGFR and ERK1/2, followed by an inferred increase in mucin production. Three other branches of the network that were highlighted and led to increases in mucins included the AP-1, FOXA3/SPDEF, and IL13/SPDEF pathways. The inferred activation of the IL13 receptor complex mirrored the activation of the matrix metalloproteinase family. The inferred activation of the p38 MAPK family upstream of MUC5AC was unique to the pyocyanin dataset. While the NPA analysis showed a significant network perturbation in response to human airway epithelial cell mucociliary differentiation, a closer examination of the leading nodes clearly indicated inferred downregulation of ROS and the EGFR and MAPK ERK1/2 pathways (Supplementary Data Sheet S3).
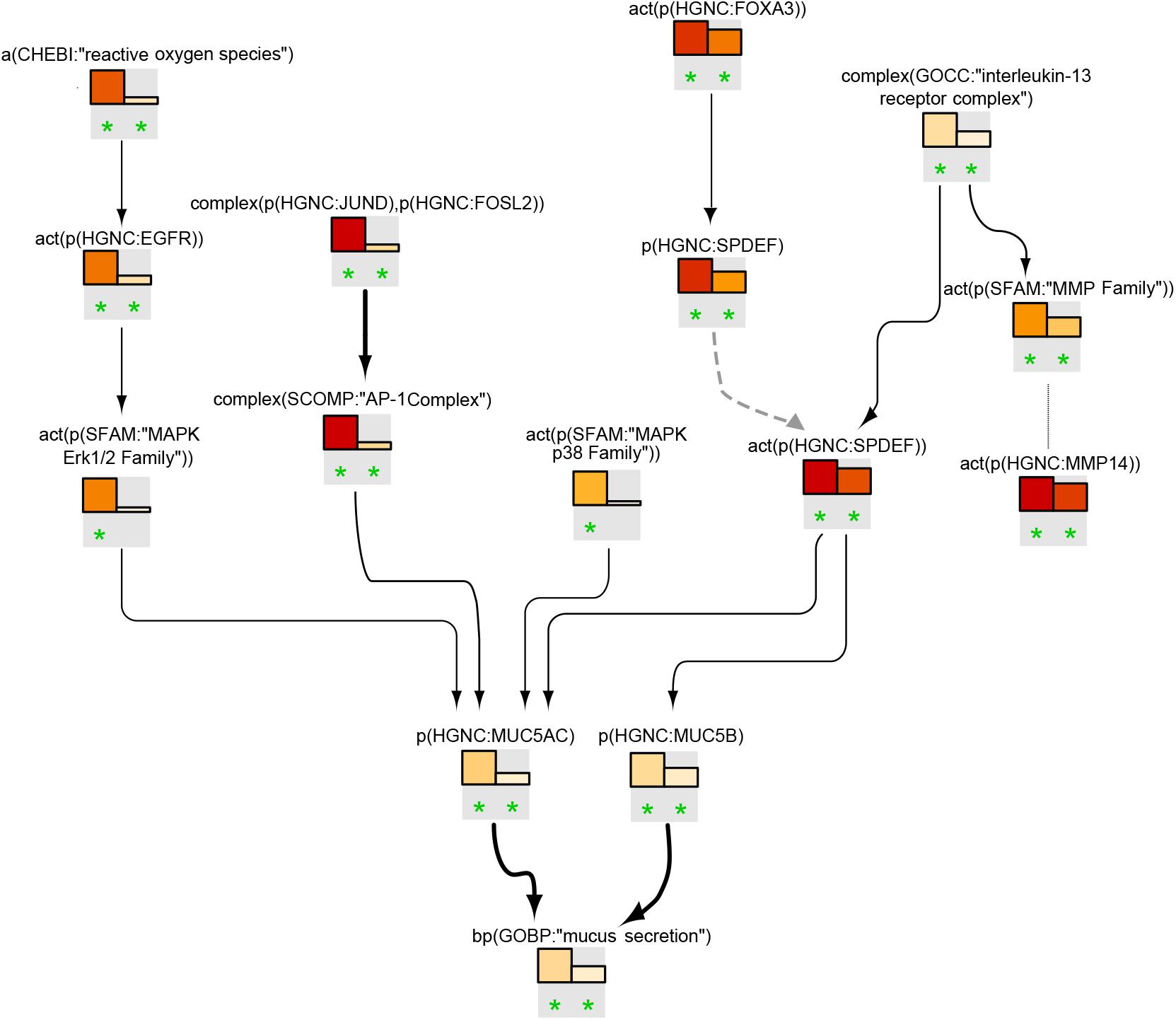
Figure 7. Goblet cell hyperplasia/metaplasia subnetwork based on leading nodes. The graphical representation shows an example of connected leading nodes for pyocyanin treatment (first lane) and IL13 treatment (second lane) compared with experimental controls. The top 10 leading nodes were prioritized and connected with other leading nodes from the analysis. Orange/red bars indicate inferred upregulation. Bold edges indicate “direct regulation.” The dotted edge denotes a member of a protein family. The green asterisk indicates that the node is a leading node in a given contrast. The vocabulary for the BEL is provided in http://www.openbel.org/.
Discussion
MCC is an important defense mechanism that protects the respiratory tract, and thus the body, from infections and airborne pollutants. In this article, we presented a suite of CBN models that describe relevant molecular processes related to MCC. Derived from original articles, the BEL-scripted scientific statements were assembled into three separate network models that capture molecular processes involved in cilium assembly, ciliary beating, and goblet cell hyperplasia/metaplasia accurately. The key factors involved in these processes are part of the backbone that interconnects various entities in the network models. As an example, the cilium assembly hub integrates the diversity of the cascades that are determined by the variety of cilia types, each requiring precise regulation (for review, see Choksi et al., 2014).
As part of network model validation, we conducted network scoring with gene expression data from experiments that were expected to trigger perturbation of the MCC models. The scoring also allowed us to look farther from the static network view into the key factors that impact the network and assess the behavior (activation or inhibition) of molecular entities in the model backbone based on differential gene expression in the selected datasets.
As expected, the biology in the redox-active pyocyanin treatment experiment was best reflected in the goblet cell hyperplasia/metaplasia model, with EGFR and downstream MAPK ERK1/2 factors predicted to be activated, leading to mucin production. This was in line with other experimental observations of increased numbers of goblet cells and increased mucin production in response to pyocyanin treatment (Rada et al., 2011).
Impact on the cilium-focused network models could be explained by the cell redox state and ROS levels affecting multiple cellular signaling pathways, some of which overlap with cilium biology. As an example, the activity of the nitric oxide synthase (NOS) family as well as the nitric oxide (NO) chemical node were inferred to be upregulated by pyocyanin treatment in the ciliary beating network model (Supplementary Data Sheet S2). NO is a redox molecule that regulates tissue oxidative balance through direct and indirect mechanisms of action and can lead to an increase in ciliary beat frequency through the activation of NOS family.
Network scoring with the airway epithelial cell differentiation dataset clearly showed time-dependent activation of pathways leading to cilium assembly and ciliary beating. At the early stage, BMP signaling was inferred to be upregulated, indicating the lack of cilium assembly, while at later time points, BMP released the inhibitory effect on cilium assembly, with the MCIDAS/FOXJ1/RFX3 pathway inferred to be activated to promote cilium assembly. Network scoring, however, indicated that mTOR and AKT were downregulated in the dataset, contradictory to the causal connection from mTOR and AKT to cilium assembly that was inferred to be upregulated. These relationships were derived from articles describing primary cilium assembly and may not appropriately reflect the biology in the respiratory tract, where the operating process is the motile cilia assembly program that culminates in the FOXJ1/RFX3 module (Wang et al., 2015; Suizu et al., 2016).
Downregulation of CCNA2 and cell cycle arrest in the cilium assembly network model could indicate a slowing down in cell proliferation to enforce cell differentiation. This was in accordance with the inferred inhibition of the mTOR/AKT pathway in the cilium assembly model. The results were further enforced by the scoring of the goblet cell network. The inferred reduction in EGFR signaling could indicate loss of proliferative potential in cultures differentiating to pseudostratified epithelium. This result also suggests that the network model discriminates between a physiological (i.e., differentiation) and pathological (i.e., COPD-related) increase in the number of goblet cells in airways. Finally, the cilia beating model appropriately captured the activation of cAMP/PRKA and cGMP/PRKG signaling that elevates cellular Ca2+ levels, leading to increases in cilia beating.
Scoring the three network models with the datasets from IL13-treated lung cells highlighted the specificities of the different networks: IL13-induced airway mucus production affected several hubs in the hyperplasia/metaplasia model, notably the SPDEF transcription factor, while impacts on the cilium assembly and ciliary beating models did not reach statistical significance.
In conclusion, the representation of cilium assembly, ciliary beating, and airway remodeling processes through CBN models is a potential powerful tool for systems medicine (Talikka et al., 2017). MCC networks can be used as a substrate for scoring high-throughput data for mechanistic understanding of the differences between diseased and healthy tissue. The MCC network model suite presented here, along with gene expression data from well-controlled clinical studies, could be used in individuals with MCC disorders for subject classification, identification of mode of action of novel drug candidates, or prediction of treatment outcome. Ultimately, the MCC network model suite provides perspectives for tailored drug therapy and precision medicine.
Author Contributions
MT, JH, and MP conceived and designed the experiments. HY, SV, and MT performed the study. HY and MT wrote the manuscript with the support of KL. FM, AS, and SG analyzed the data. All authors made critical revision and approved the final version of the manuscript.
Funding
Philip Morris International is the sole source of funding and sponsor of this research.
Conflict of Interest Statement
All authors are employees of Philip Morris International.
Acknowledgments
We would like to thank Nicholas Karoglou and Elena Scotti for editorial assistance as well as Stephanie Boue for preparing and uploading the network models in the CBN database.
Supplementary Material
The Supplementary Material for this article can be found online at: https://www.frontiersin.org/articles/10.3389/fgene.2019.00087/full#supplementary-material
DATA SHEET S1 | Leading node analysis for cilium assembly network model scored with transcriptomic data from pyocyanin treatment, IL-13 treatment, and mucociliary differentiation datasets. For each node, an ∗ indicates it is a leading node and the number denotes its rank among the leading nodes. + or - in parenthesis indicates inferred up- or down-regulation, respectively. Value in % is the contribution of the node to the overall NPA.
DATA SHEET S2 | Leading node analysis for ciliary beating network model scored with transcriptomic data from pyocyanin treatment, IL-13 treatment, and mucociliary differentiation datasets. For each node, an ∗ indicates it is a leading node and the number denotes its rank among the leading nodes. + or - in parenthesis indicates inferred up- or down-regulation, respectively. Value in % is the contribution of the node to the overall NPA.
DATA SHEET S3 | Leading node analysis for goblet cell hyperplasia/metaplasia network model scored with transcriptomic data from pyocyanin treatment, IL-13 treatment, and mucociliary differentiation datasets. For each node, an ∗ indicates it is a leading node and the number denotes its rank among the leading nodes. + or - in parenthesis indicates inferred up- or down-regulation, respectively. Value in % is the contribution of the node to the overall NPA.
Footnotes
References
Alevy, Y. G., Patel, A. C., Romero, A. G., Patel, D. A., Tucker, J., Roswit, W. T., et al. (2012). IL-13–induced airway mucus production is attenuated by MAPK13 inhibition. J. Clin. Invest. 122, 4555–4568. doi: 10.1172/JCI64896
Anderson, D., and Macnee, W. (2009). Targeted treatment in COPD: a multi-system approach for a multi-system disease. Int. J. Chron. Obstruct. Pulmon. Dis. 4, 321–335. doi: 10.2147/COPD.S2999
Arbi, M., Pefani, D. E., Kyrousi, C., Lalioti, M. E., Kalogeropoulou, A., Papanastasiou, A. D., et al. (2016). GemC1 controls multiciliogenesis in the airway epithelium. EMBO Rep. 17, 400–413. doi: 10.15252/embr.201540882
Blyth, D. I., Pedrick, M. S., Savage, T. J., Bright, H., Beesley, J. E., and Sanjar, S. (1998). Induction, duration, and resolution of airway goblet cell hyperplasia in a murine model of atopic asthma: effect of concurrent infection with respiratory syncytial virus and response to dexamethasone. Am. J. Respir. Cell Mol. Biol. 19, 38–54. doi: 10.1165/ajrcmb.19.1.2930
Boon, M., Wallmeier, J., Ma, L., Loges, N. T., Jaspers, M., Olbrich, H., et al. (2014). MCIDAS mutations result in a mucociliary clearance disorder with reduced generation of multiple motile cilia. Nat. Commun. 5:4418. doi: 10.1038/ncomms5418
Boucherat, O., Boczkowski, J., Jeannotte, L., and Delacourt, C. (2013). Cellular and molecular mechanisms of goblet cell metaplasia in the respiratory airways. Exp. Lung Res. 39, 207–216. doi: 10.3109/01902148.2013.791733
Boue, S., Talikka, M., Westra, J. W., Hayes, W., Di Fabio, A., Park, J., et al. (2015). Causal biological network database: a comprehensive platform of causal biological network models focused on the pulmonary and vascular systems. Database 2015:bav030. doi: 10.1093/database/bav030
Brody, S. L., Yan, X. H., Wuerffel, M. K., Song, S. K., and Shapiro, S. D. (2000). Ciliogenesis and left-right axis defects in forkhead factor HFH-4-null mice. Am. J. Respir. Cell Mol. Biol. 23, 45–51. doi: 10.1165/ajrcmb.23.1.4070
Casalino-Matsuda, S. M., Monzon, M. E., and Forteza, R. M. (2006). Epidermal growth factor receptor activation by epidermal growth factor mediates oxidant-induced goblet cell metaplasia in human airway epithelium. Am. J. Respir. Cell Mol. Biol. 34, 581–591. doi: 10.1165/rcmb.2005-0386OC
Catlett, N. L., Bargnesi, A. J., Ungerer, S., Seagaran, T., Ladd, W., Elliston, K. O., et al. (2013). Reverse causal reasoning: applying qualitative causal knowledge to the interpretation of high-throughput data. BMC Bioinformatics 14:340. doi: 10.1186/1471-2105-14-340
Chen, G., Korfhagen, T. R., Xu, Y., Kitzmiller, J., Wert, S. E., Maeda, Y., et al. (2009). SPDEF is required for mouse pulmonary goblet cell differentiation and regulates a network of genes associated with mucus production. J. Clin. Invest. 119, 2914–2924. doi: 10.1172/JCI39731
Chen, J., Knowles, H. J., Hebert, J. L., and Hackett, B. P. (1998). Mutation of the mouse hepatocyte nuclear factor/forkhead homologue 4 gene results in an absence of cilia and random left-right asymmetry. J. Clin. Invest. 102, 1077–1082. doi: 10.1172/JCI4786
Cho, D. Y., Kim, Y. A., and Przytycka, T. M. (2012). Chapter 5: network biology approach to complex diseases. PLoS Comput. Biol. 8:e1002820. doi: 10.1371/journal.pcbi.1002820
Choksi, S. P., Lauter, G., Swoboda, P., and Roy, S. (2014). Switching on cilia: transcriptional networks regulating ciliogenesis. Development 141, 1427–1441. doi: 10.1242/dev.074666
De Leon, H., Boue, S., Schlage, W. K., Boukharov, N., Westra, J. W., Gebel, S., et al. (2014). A vascular biology network model focused on inflammatory processes to investigate atherogenesis and plaque instability. J. Transl. Med. 12:185. doi: 10.1186/1479-5876-12-185
Fernandes, J., Lorenzo, I. M., Andrade, Y. N., Garcia-Elias, A., Serra, S. A., Fernandez-Fernandez, J. M., et al. (2008). IP3 sensitizes TRPV4 channel to the mechano- and osmotransducing messenger 5′-6′-epoxyeicosatrienoic acid. J. Cell Biol. 181, 143–155. doi: 10.1083/jcb.200712058
Fliegauf, M., Benzing, T., and Omran, H. (2007). When cilia go bad: cilia defects and ciliopathies. Nat. Rev. Mol. Cell Biol. 8, 880–893. doi: 10.1038/nrm2278
Gebel, S., Lichtner, R. B., Frushour, B., Schlage, W. K., Hoang, V., Talikka, M., et al. (2013). Construction of a computable network model for DNA damage, autophagy, cell death, and senescence. Bioinform. Biol. Insights 7, 97–117. doi: 10.4137/BBI.S11154
Gerdes, J. M., Davis, E. E., and Katsanis, N. (2009). The vertebrate primary cilium in development, homeostasis, and disease. Cell 137, 32–45. doi: 10.1016/j.cell.2009.03.023
Hao, Y., Kuang, Z., Jing, J., Miao, J., Mei, L. Y., Lee, R. J., et al. (2014). Mycoplasma pneumoniae modulates STAT3-STAT6/EGFR-FOXA2 signaling to induce overexpression of airway mucins. Infect. Immun. 82, 5246–5255. doi: 10.1128/IAI.01989-14
Hewson, C. A., Edbrooke, M. R., and Johnston, S. L. (2004). PMA induces the MUC5AC respiratory mucin in human bronchial epithelial cells, via PKC, EGF/TGF-alpha, Ras/Raf, MEK, ERK and Sp1-dependent mechanisms. J. Mol. Biol. 344, 683–695. doi: 10.1016/j.jmb.2004.09.059
Hoeng, J., Deehan, R., Pratt, D., Martin, F., Sewer, A., Thomson, T. M., et al. (2012). A network-based approach to quantifying the impact of biologically active substances. Drug Discov. Today 17, 413–418. doi: 10.1016/j.drudis.2011.11.008
Hubbert, C., Guardiola, A., Shao, R., Kawaguchi, Y., Ito, A., Nixon, A., et al. (2002). HDAC6 is a microtubule-associated deacetylase. Nature 417, 455–458. doi: 10.1038/417455a
Jain, B., Rubinstein, I., Robbins, R. A., Leise, K. L., and Sisson, J. H. (1993). Modulation of airway epithelial cell ciliary beat frequency by nitric oxide. Biochem. Biophys. Res. Commun. 191, 83–88. doi: 10.1006/bbrc.1993.1187
Kempeneers, C., and Chilvers, M. A. (2018). To beat, or not to beat, that is question! The spectrum of ciliopathies. Pediatr. Pulmonol. 53, 1122–1129. doi: 10.1002/ppul.24078
Kim, S., and Tsiokas, L. (2011). Cilia and cell cycle re-entry: more than a coincidence. Cell Cycle 10, 2683–2690. doi: 10.4161/cc.10.16.17009
Kim, V., and Criner, G. J. (2015). The chronic bronchitis phenotype in chronic obstructive pulmonary disease: features and implications. Curr. Opin. Pulm. Med. 21, 133–141. doi: 10.1097/MCP.0000000000000145
Korngreen, A., and Priel, Z. (1996). Purinergic stimulation of rabbit ciliated airway epithelia: control by multiple calcium sources. J. Physiol. 497(Pt 1), 53–66. doi: 10.1113/jphysiol.1996.sp021749
Luettich, K., Talikka, M., Lowe, F. J., Haswell, L. E., Park, J., Gaca, M. D., et al. (2017). The adverse outcome pathway for oxidative stress-mediated EGFR activation leading to decreased lung function. Appl. Vitro Toxicol. 3, 99–109. doi: 10.1089/aivt.2016.0032
Martin, F., Sewer, A., Talikka, M., Xiang, Y., Hoeng, J., and Peitsch, M. C. (2014). Quantification of biological network perturbations for mechanistic insight and diagnostics using two-layer causal models. BMC Bioinformatics 15:238. doi: 10.1186/1471-2105-15-238
Matera, M. G., Calzetta, L., and Cazzola, M. (2016). Oxidation pathway and exacerbations in COPD: the role of NAC. Expert Rev. Respir. Med. 10, 89–97. doi: 10.1586/17476348.2016.1121105
Murphy, D. B., Seemann, S., Wiese, S., Kirschner, R., Grzeschik, K. H., and Thies, U. (1997). The human hepatocyte nuclear factor 3/fork head gene FKHL13: genomic structure and pattern of expression. Genomics 40, 462–469. doi: 10.1006/geno.1996.4587
Nini, G., Raica, M., Neamtiu, V., and Onel, M. (2012). Morphological study of bronchial mucosa in the chronic obstructive pulmonary disease under the influence of therapeutic algorithm. Rom. J. Morphol. Embryol. 53, 121–134.
Nozawa, Y. I., Lin, C., and Chuang, P. T. (2013). Hedgehog signaling from the primary cilium to the nucleus: an emerging picture of ciliary localization, trafficking and transduction. Curr. Opin. Genet Dev. 23, 429–437. doi: 10.1016/j.gde.2013.04.008
Pan, J., Wang, Q., and Snell, W. J. (2004). An aurora kinase is essential for flagellar disassembly in Chlamydomonas. Dev. Cell 6, 445–451. doi: 10.1016/S1534-5807(04)00064-4
Park, K. S., Korfhagen, T. R., Bruno, M. D., Kitzmiller, J. A., Wan, H., Wert, S. E., et al. (2007). SPDEF regulates goblet cell hyperplasia in the airway epithelium. J. Clin. Invest. 117, 978–988. doi: 10.1172/JCI29176
Perrais, M., Pigny, P., Copin, M. C., Aubert, J. P., and Van Seuningen, I. (2002). Induction of MUC2 and MUC5AC mucins by factors of the epidermal growth factor (EGF) family is mediated by EGF receptor/Ras/Raf/extracellular signal-regulated kinase cascade and Sp1. J. Biol. Chem. 277, 32258–32267. doi: 10.1074/jbc.M204862200
Pugacheva, E. N., Jablonski, S. A., Hartman, T. R., Henske, E. P., and Golemis, E. A. (2007). HEF1-dependent Aurora A activation induces disassembly of the primary cilium. Cell 129, 1351–1363. doi: 10.1016/j.cell.2007.04.035
Rada, B., Gardina, P., Myers, T. G., and Leto, T. L. (2011). Reactive oxygen species mediate inflammatory cytokine release and EGFR-dependent mucin secretion in airway epithelial cells exposed to Pseudomonas pyocyanin. Mucosal Immunol. 4, 158–171. doi: 10.1038/mi.2010.62
Rahman, I., and Adcock, I. M. (2006). Oxidative stress and redox regulation of lung inflammation in COPD. Eur. Respir. J. 28, 219–242. doi: 10.1183/09031936.06.00053805
Rahman, I., and MacNee, W. (1999). Lung glutathione and oxidative stress: implications in cigarette smoke-induced airway disease. Am. J. Physiol. 277, L1067–L1088. doi: 10.1152/ajplung.1999.277.6.L1067
Ramos, F. L., Krahnke, J. S., and Kim, V. (2014). Clinical issues of mucus accumulation in COPD. Int. J. Chron. Obstruct. Pulmon. Dis. 9, 139–150. doi: 10.2147/COPD.S38938
Rogers, D. F. (2007). Physiology of airway mucus secretion and pathophysiology of hypersecretion. Respir. Care 52, 1134–1146;discussion 1146–1139.
Ross, A. J., Dailey, L. A., Brighton, L. E., and Devlin, R. B. (2007). Transcriptional profiling of mucociliary differentiation in human airway epithelial cells. Am. J. Respir. Cell Mol. Biol. 37, 169–185. doi: 10.1165/rcmb.2006-0466OC
Satir, P., and Christensen, S. T. (2007). Overview of structure and function of mammalian cilia. Annu. Rev. Physiol. 69, 377–400. doi: 10.1146/annurev.physiol.69.040705.141236
Schlage, W. K., Westra, J. W., Gebel, S., Catlett, N. L., Mathis, C., Frushour, B. P., et al. (2011). A computable cellular stress network model for non-diseased pulmonary and cardiovascular tissue. BMC Syst. Biol. 5:168. doi: 10.1186/1752-0509-5-168
Sewer, A., Martin, F., Schlage, W. K., Hoeng, J., and Peitsch, M. C. (2015). “Quantifying the Biological Impact of Active Substances Using Causal Network Models,” in Computational Systems Toxicology, eds J. Hoeng and M. Peitsch (New York, NY: Humana Press), 223–256.
Shannon, P., Markiel, A., Ozier, O., Baliga, N. S., Wang, J. T., Ramage, D., et al. (2003). Cytoscape: a software environment for integrated models of biomolecular interaction networks. Genome Res. 13, 2498–2504. doi: 10.1101/gr.1239303
Smyth, G. K. (2004). Linear models and empirical bayes methods for assessing differential expression in microarray experiments. Stat. Appl. Genet Mol. Biol. 3:Article3. doi: 10.2202/1544-6115.1027
Stubbs, J. L., Vladar, E. K., Axelrod, J. D., and Kintner, C. (2012). Multicilin promotes centriole assembly and ciliogenesis during multiciliate cell differentiation. Nat. Cell Biol. 14, 140–147. doi: 10.1038/ncb2406
Suizu, F., Hirata, N., Kimura, K., Edamura, T., Tanaka, T., Ishigaki, S., et al. (2016). Phosphorylation-dependent Akt-Inversin interaction at the basal body of primary cilia. EMBO J. 35, 1346–1363. doi: 10.15252/embj.201593003
Sutto, Z., Conner, G. E., and Salathe, M. (2004). Regulation of human airway ciliary beat frequency by intracellular pH. J. Physiol. 560, 519–532. doi: 10.1113/jphysiol.2004.068171
Szostak, J., Ansari, S., Madan, S., Fluck, J., Talikka, M., Iskandar, A., et al. (2015). Construction of biological networks from unstructured information based on a semi-automated curation workflow. Database 2015:bav057. doi: 10.1093/database/bav057
Szostak, J., Martin, F., Talikka, M., Peitsch, M. C., and Hoeng, J. (2016). Semi-automated curation allows causal network model building for the quantification of age-dependent plaque progression in ApoE-/- mouse. Gene Regul. Syst. Bio. 10, 95–103. doi: 10.4137/GRSB.S40031
Takeyama, K., Dabbagh, K., Lee, H. M., Agusti, C., Lausier, J. A., Ueki, I. F., et al. (1999). Epidermal growth factor system regulates mucin production in airways. Proc. Natl. Acad. Sci. U.S.A. 96, 3081–3086. doi: 10.1073/pnas.96.6.3081
Takeyama, K., Jung, B., Shim, J. J., Burgel, P. R., Dao-Pick, T., Ueki, I. F., et al. (2001). Activation of epidermal growth factor receptors is responsible for mucin synthesis induced by cigarette smoke. Am. J. Physiol. Lung Cell Mol. Physiol. 280, L165–L172. doi: 10.1152/ajplung.2001.280.1.L165
Talikka, M., Bukharov, N., Hayes, W. S., Hofmann-Apitius, M., Alexopoulos, L., Peitsch, M. C., et al. (2017). Novel approaches to develop community-built biological network models for potential drug discovery. Expert Opin. Drug Discov. 12, 849–857. doi: 10.1080/17460441.2017.1335302
Thomas, J., Morle, L., Soulavie, F., Laurencon, A., Sagnol, S., and Durand, B. (2010). Transcriptional control of genes involved in ciliogenesis: a first step in making cilia. Biol. Cell 102, 499–513. doi: 10.1042/BC20100035
Turner, J., and Jones, C. E. (2009). Regulation of mucin expression in respiratory diseases. Biochem. Soc. Trans. 37, 877–881. doi: 10.1042/BST0370877
Wang, S., Livingston, M. J., Su, Y., and Dong, Z. (2015). Reciprocal regulation of cilia and autophagy via the MTOR and proteasome pathways. Autophagy 11, 607–616. doi: 10.1080/15548627.2015.1023983
Wanner, A., Salathe, M., and O’Riordan, T. G. (1996). Mucociliary clearance in the airways. Am. J. Respir. Crit. Care Med. 154, 1868–1902. doi: 10.1164/ajrccm.154.6.8970383
Westra, J. W., Schlage, W. K., Frushour, B. P., Gebel, S., Catlett, N. L., Han, W., et al. (2011). Construction of a computable cell proliferation network focused on non-diseased lung cells. BMC Syst. Biol. 5:105. doi: 10.1186/1752-0509-5-105
Westra, J. W., Schlage, W. K., Hengstermann, A., Gebel, S., Mathis, C., Thomson, T., et al. (2013). A modular cell-type focused inflammatory process network model for non-diseased pulmonary tissue. Bioinform. Biol. Insights 7, 167–192. doi: 10.4137/BBI.S11509
Wheatley, D. N. (1995). Primary cilia in normal and pathological tissues. Pathobiology 63, 222–238. doi: 10.1159/000163955
Wills-Karp, M. (2004). Interleukin-13 in asthma pathogenesis. Immunol. Rev. 202, 175–190. doi: 10.1111/j.0105-2896.2004.00215.x
Workman, A. D., and Cohen, N. A. (2014). The effect of drugs and other compounds on the ciliary beat frequency of human respiratory epithelium. Am. J. Rhinol. Allergy 28, 454–464. doi: 10.2500/ajra.2014.28.4092
Wyatt, T. A., Spurzem, J. R., May, K., and Sisson, J. H. (1998). Regulation of ciliary beat frequency by both PKA and PKG in bovine airway epithelial cells. Am. J. Physiol. 275, L827–L835. doi: 10.1152/ajplung.1998.275.4.L827
Yaghi, A., and Dolovich, M. B. (2016). Airway Epithelial Cell Cilia and Obstructive Lung Disease. Cells 5:40. doi: 10.3390/cells5040040
Yaghi, A., Zaman, A., Cox, G., and Dolovich, M. B. (2012). Ciliary beating is depressed in nasal cilia from chronic obstructive pulmonary disease subjects. Respir. Med. 106, 1139–1147. doi: 10.1016/j.rmed.2012.04.001
Yang, B., Schlosser, R. J., and McCaffrey, T. V. (1996). Dual signal transduction mechanisms modulate ciliary beat frequency in upper airway epithelium. Am. J. Physiol. 270, L745–L751. doi: 10.1152/ajplung.1996.270.5.L745
Zagoory, O., Braiman, A., Gheber, L., and Priel, Z. (2001). Role of calcium and calmodulin in ciliary stimulation induced by acetylcholine. Am. J. Physiol. Cell Physiol. 280, C100–C109. doi: 10.1152/ajpcell.2001.280.1.C100
Keywords: mucociliary clearance, network models, biological expression language, respiratory tract, network perturbation amplitude
Citation: Yepiskoposyan H, Talikka M, Vavassori S, Martin F, Sewer A, Gubian S, Luettich K, Peitsch MC and Hoeng J (2019) Construction of a Suite of Computable Biological Network Models Focused on Mucociliary Clearance in the Respiratory Tract. Front. Genet. 10:87. doi: 10.3389/fgene.2019.00087
Received: 05 October 2018; Accepted: 29 January 2019;
Published: 15 February 2019.
Edited by:
Marco Antoniotti, Università degli Studi di Milano Bicocca, ItalyReviewed by:
Richard R. Rodrigues, Oregon State University, United StatesAdriano Velasque Werhli, Fundação Universidade Federal do Rio Grande, Brazil
Copyright © 2019 Yepiskoposyan, Talikka, Vavassori, Martin, Sewer, Gubian, Luettich, Peitsch and Hoeng. This is an open-access article distributed under the terms of the Creative Commons Attribution License (CC BY). The use, distribution or reproduction in other forums is permitted, provided the original author(s) and the copyright owner(s) are credited and that the original publication in this journal is cited, in accordance with accepted academic practice. No use, distribution or reproduction is permitted which does not comply with these terms.
*Correspondence: Marja Talikka, bWFyamEudGFsaWtrYUBwbWkuY29t