- 1Australian Prostate Cancer Research Centre – Queensland, Translational Research Institute, Queensland University of Technology, Brisbane, QLD, Australia
- 2Cancer Program, School of Biomedical Sciences, Institute of Health and Biomedical Innovation, Queensland University of Technology, Brisbane, QLD, Australia
- 3Menzies Health Institute Queensland, Griffith University, Gold Coast, QLD, Australia
- 4Cancer Research Centre, Cancer Council Queensland, Brisbane, QLD, Australia
With an estimated 1.1 million men worldwide diagnosed with prostate cancer yearly, effective and more specific biomarkers for early diagnosis could lead to better patient outcome. As such, novel genetic markers are sought for this purpose. The tribbles homologue 1 gene (TRIB1) has recently shown to have a role in prostate tumorigenesis and data-mining of prostate cancer expression data confirmed clinical significance of TRIB1 in prostate cancer. For the first time, a polymorphic microsatellite in this gene was studied for its potential association with prostate cancer risk and aggressiveness. Genomic DNA was extracted from a cohort of 1,152 prostate cancer patients and 1,196 cancer-free controls and the TTTTG-TRIB1 microsatellite was genotyped. The socio-demographic and clinical characteristics were analyzed using the non-parametric t-test and two-way ANOVA. Association of the TTTTG-TRIB1 microsatellite and prostate cancer risk and aggressiveness were analyzed by binary logistic regression and confirmed by bootstrapping. Total and prostate cancer mortality was analyzed using the Kaplan Meier test. Genotype and allele correlation with TRIB1 mRNA levels was analyzed using the non-parametric Kolmogorov–Smirnov test. To predict the effect that the TTTTG-TRIB1 polymorphisms had on the mRNA structure, the in silico RNA folding predictor tool, mfold, was used. By analyzing the publicly available data, we confirmed a significant over-expression of TRIB1 in prostate cancer compared to other cancer types, and an over-expression in prostate cancerous tissue compared to adjacent benign. Three alleles (three–five repeats) were observed for TTTTG-TRIB1. The three-repeat allele was associated with prostate cancer risk at the allele (OR = 1.16; P = 0.044) and genotypic levels (OR = 1.70; P = 0.006) and this association was age-independent. The four-repeat allele was inversely associated with prosatet cancer risk (OR = 0.57; P < 0.0001). TRIB1 expression was upregulated in tumors when compared to adjacent cancer-free tissue but was not allele specific. In silico analysis suggested that the TTTTG-TRIB1 alleles may alter TRIB1 mRNA structure. In summary, the three-repeat allele was significantly associated with prostate cancer risk, suggesting a biomarker potential for this microsatellite to predict prostate cancer. Further studies are needed to elucidate the functional role of this microsatellite in regulating TRIB1 expression, perhaps by affecting the TRIB1 mRNA structure and stability.
Introduction
Australia and New Zealand, followed by North America, were the countries with the highest prostate cancer incidence in the developed world in 2012, according to the World Health Organization (Jemal et al., 2011). In 2016, 18,138 males were diagnosed with prostate cancer in Australia, accounting for 25.2% of all new male cancer cases and 12.8% of all male deaths caused by cancer (Welfare Aloha, 2016). In an increasing aging population, the numbers of newly diagnosed men with prostate cancer are likely to rise. Despite all the accumulated knowledge of the disease, the prostate-specific antigen (PSA) test remains one of the most common methods of screening since its introduction 30 years ago. However, the PSA test has gathered criticism for its potential over-diagnosis (Etzioni et al., 2002) and overtreatment consequences (Howrey et al., 2013). In 2012, The American Society of Clinical Oncology (Basch et al., 2012) and the U.S. Preventive Services Task Force (Moyer and U. S. Preventive Services Task Force, 2012) recommended against its use as a routine screening test. In this light, new and more specific biomarkers are needed.
Microsatellites, or short tandem repeats (STRs), are typically consecutive repeats of 2–5 base pairs (bp) of DNA in the genome. STRs are attractive biomarkers in human disease due to their highly polymorphic nature, abundancy, and wide distribution throughout the genome (Schlotterer, 2004; Willems et al., 2014). They have been used for high-resolution human genome mapping (Kong et al., 2002), population studies (Edwards et al., 1992; Budowle et al., 2002), and associated with up to 40 human monogenic diseases such as oculopharyngeal muscular dystrophy (Willems et al., 2014), as well as with more complex diseases such as cystic fibrosis and asthma (Hefferon et al., 2004; Batra et al., 2007).
Genome-Wide Association Studies (GWAS) genotype large cohorts of patients and controls, using high-throughput screening platforms to identify single-nucleotide polymorphisms (SNPs) that are associated with prostate cancer. This approach has been successful in identifying over 150 susceptibility loci for prostate cancer in a European cohort (Al Olama et al., 2014; Srinivasan et al., 2016; Schumacher et al., 2018). However, GWAS conducted to date can explain only up to ∼33% of prostate cancer heredity (Al Olama et al., 2014). We hypothesize that some of the missing prostate cancer genetic component can be explained by other genetic variants such as STRs. We used existing expression databases and bioinformatics tools that detect functional STRs lying in differentially expressed genes in prostate cancer as described previously by us (Lai et al., 2017), whereby we identified a penta-STR within the 3′UTR of the tribbles homologue 1 gene (TRIB1): TTTTG-TRIB1. Recent studies have associated the TRIB1 gene with the development of several tumors including colorectal (Wang et al., 2017), leukemia (Yoshida et al., 2013), and hepatocellular (Ye et al., 2017) cancers. Also, in prostate cancer it has recently shown to have a role in cancer cell proliferation, survival and tumor growth (Mashima et al., 2014). Furthermore, independent clinical studies have reported higher expression of TRIB1 in prostate cancer when compared to other cancers (Su et al., 2001; Ramaswamy et al., 2003) and an up-regulation of the TRIB1 gene in prostate cancer tissue when compared to adjacent cancer-free cells (Liu et al., 2006; Grasso et al., 2012). However, the mechanisms by which its expression is upregulated in prostate cancer are not yet understood (Yu et al., 2004; Taylor et al., 2010; Grasso et al., 2012; Mashima et al., 2014). Additionally, we and others have observed a down-regulation of the TTTTG-TRIB1 STR and the TRIB1 gene (Munkley et al., 2015; Lai et al., 2017) in LNCaP cells after androgen treatment, supporting a link between TRIB1 and prostate cancer. Interestingly, a recent study has observed an increase of TRIB1 DNA copies and mRNA levels in breast cancer patients with a poorer survival outcome and a more aggressive phenotype in (Gendelman et al., 2017) after regulating proliferation, apoptosis, and cytokine production. Here, we undertook the largest genotyping analysis of a microsatellite repeat in this gene and analyzed its association with prostate cancer risk, aggressiveness and survival.
Materials and Methods
TRIB1 Expression in Cancer
The Oncomine gene mining database (Rhodes et al., 2004) was used to determine how the TRIB1 gene expression in prostate cancer compares with other cancers (Figures 1A,B) and to confirm if its expression was higher in prostate tumor tissue when compared to non-tumor prostatic tissue (Figures 1C,D). This mining web-tool extracts differential gene expression data from microarray analysis which originate from numerous samples such as cancer physiological fluids and tissues. Clinical and pathological data is also made available to the user. All the data presented is normalized and statistically analyzed.
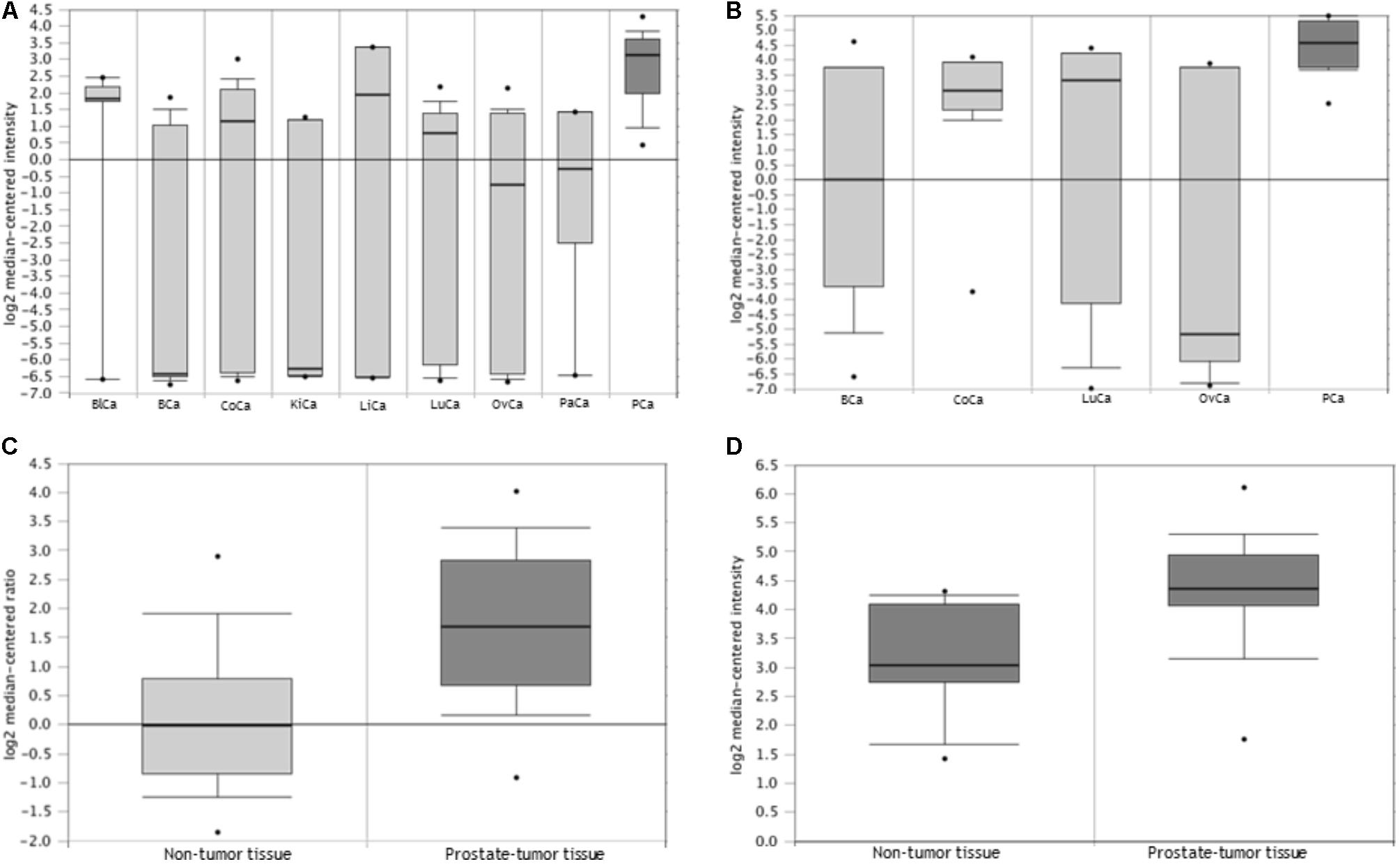
FIGURE 1. TRIB1 is overexpressed in prostatate cancer. The Oncomine database showed that TRIB1 was overexpressed in (A) prostate cancer when compared to eight (n = 174; Su et al., 2001), (B) and four other cancer types (n = 76; Ramaswamy et al., 2003) and in prostate cancer when compared to adjacent cancer-free tissue with in (C) Grasso et al. (2012) dataset (n = 122) and (D) Liu et al. (2006) dataset (n = 57); BlCa, bladder cancer; BCa, breast cancer; CoCa, colorectal cancer; KiCa, kidney cancer; LiCa, liver cancer; LuCa, lung cancer; OvCa, ovarian cancer; PaCa, pancreatic cancer; PCa, prostate cancer.
In order to analyze the expression of the TRIB1 gene in multiple cancer types, two multi-cancer studies were selected. The selection criteria were based on their number of samples and data availability for the prostate cancer. In the first study (Figure 1A) by Su et al. (2001) TRIB1 gene expression data was available from prostate tumor as well as eight other cancers (n = 174; bladder, breast, colorectal, kidney, liver, lung, ovarian, and pancreatic). In the second study (Figure 1B), Ramaswamy et al. (2003) analyzed the TRIB1 gene expression in prostate malignant tissue and compared it to four other cancer types (n = 76; breast, colorectal, lung and ovarian). The arrays used in the first study were the Human Genome U95A-Av2 Array while the HumanGeneFL Array, Hu35KsubA Array was used in the second study.
We then searched for reported differential expression of the TRIB1 gene in prostate cancer when compared to prostatic benign tissue. Two studies that reflect this differential expression were selected for their number of samples available. The first study selected was carried out by Grasso et al. (2012; Figure 1C), where a total of 122 samples were analyzed (59 localized prostate carcinoma, and 28 benign prostate tissue specimens) using the Agilent Human Genome 44K array. The second selected study was presented by Liu et al. (2011; Figure 1D), where a total of 57 samples (44 prostate carcinoma and 13 adjacent normal samples) using the Human Genome U133A Array.
Prostate Cancer Patients and Cancer-Free Controls
A total of 1,152 prostate patients were analyzed, including 138 men recruited via collaborations with urologists, 347 men from the QLD node of the Australian Prostate Cancer BioResource (APCB) and 667 men recruited in collaboration with the Cancer Council Queensland ProsCan study as detailed in our previous studies (Batra et al., 2011a; Lose et al., 2012; Eeles et al., 2013).
An extensive medical record for each patient that includes parameters such as age at diagnosis, family history of prostate cancer, ethnicity, PSA levels and survival data were collected, as well as pathology reports, including Gleason scores. Survival data was obtained through Cancer registry, which is maintained by Cancer Council Queensland (last extraction-2016). The registry operates under an Act of Parliament that requires mandatory notification of all cancer cases in Queensland by all hospitals (public, private, and psychiatric), nursing homes, and pathology laboratories. Death certificates are accessed to identify if the cause of death is cancer.
A total of 1,196 cancer-free control participants were included in the study. None of the controls included had been diagnosed with prostate cancer at the time of collection or presented any symptoms. From these, 527 men recruited through the Electoral Roll were age- and postal code-matched to patients from the ProsCan study. A further 669 male controls were recruited through the Australian Red Cross Blood Services. All cancer-free volunteers completed a detailed questionnaire that included information such as age, height and weight at the time of recruitment, and it also included risk factors and sociodemographic variables like family history of prostate cancer, whether they have had a vasectomy, as well as epidemiological factors such as, smoking, drinking and education. The scale used for all the variables are summarized in Table 1. All cases and controls were of European ancestry. This study was approved by the Queensland University of Technology’s Human Ethics Committee (Ethics’ Approval Number: 1000001171), and all participants provided informed written consent to participate in prostate cancer genetic studies.
Genomic DNA Extraction and STR Genotyping
White blood cells were obtained from 10 ml of venous blood that were collected in EDTA tubes. Buffy coat was separated within 24 h and stored at -20°C until further processing. Genomic DNA was extracted from the buffy coats using the QIAamp DNA Mini Kit as per the manufacturer’s instructions (Qiagen, Hilden, Germany) as described previously (Batra et al., 2011b; Lose et al., 2012) and a multiplex PCR was performed in a 96 well plates. Briefly, the multiplex PCR kit (QIAGEN, Chadstone, VIC, Australia) was used in a 40 cycles’ PCR following the manufacturer’s guidelines. NED-fluorescently labeled primers (forward: 5′-GAGAAATGGCACAAAAACAGG-3′ and reverse: 5′-TTCTGTCAAGGTAATATTGCCAA-3′) were obtained from Sigma-Aldrich (Castle Hill, NSW, Australia). The primers were designed to amplify the STRs’ region at chromosome 8:126450287–126450311 position with a predicted product length of 299 bp (according to UCSC hg19). A total of thirty-one 96 well plates were subsequently analyzed according to their fragment sizes by capillary electrophoresis using the 3500 Genetic Analyzer platform (Applied Biosystems). Every plate had a well with water as a blank to determine the specificity of our PCR product and a total of 175 technical replicates were included across the plates to determine the reproducibility of the results. Technical replicates had to match in at least 90% of the hits detected to confirm the robustness of the assay. Due to the nature of the amplified and genotyped product, a 10% failure was allowed for potential mistakes at the PCR and/or genotypic levels. The results were then analyzed with GeneMapper v.5.0 (Thermo Fisher Scientific, Waltham, MA, United States).
TTTTG-TRIB1 RT-qPCR
The APCB prostate tumor bank provided formalin fixed and paraffin embedded (FFPE) blocks of prostate tissue, which contained both prostate tumor and adjacent non-tumor cells. The blocks were then sectioned sequentially in 20 μm sections and placed on glass slides which were methyl green stained. The pathologist marked tumor areas with their respective Gleason scores (Supplementary Table 1). mRNA was isolated using the RNeasy FFPE Kit (QIAGEN, Chadstone, VIC, Australia), mRNA was reversed transcribed (RT) using Superscript III (Life Technologies, Scoresby, VIC, Australia) as previously described (Lai et al., 2017). RNA purity and quantity were analyzed using the nanodropTM 2000/2000c Spectrophotometers and quantitative PCR (RT-qPCR) was performed using the SYBR Green method (Life Technologies, Scoresby, VIC, Australia). Twenty available patients’ tumor and adjacent non-tumor prostate tissue were selected based on their genotype as follows: 3/3 repeats (n = 2), 3/4 repeats (n = 8), and 4/4 repeats (n = 10). Their Gleason grades are detailed in Supplementary Table 1. The sequence at chr8:126450219-126450357 (UCSC hg19) was amplified with a predicted PCR product length of 139 bp using the forward, 5′-GAATGCCGTGTATACCTCACG-3′, and reverse, 5′-CGCAGGTTATTCAGACAGACA-3′ primers set. Applying the geometrical mean (geomean) of multiple housekeeping genes in RT-qPCR assists in removing non-specific gene variation expression, minimizing differences in the samples gene expression detection due to variables such as the RNA quantity and quality (Vandesompele et al., 2002). We therefore used geomean of the two control genes as reference, HPRT1 and RPL32. We then proceeded to evaluate the STR expression by calculating the ΔCT values = Ct TRIB1 – Ct Geomean of HPRT1 and RPL32.
Statistical Analysis
After checking if age and body mass index (BMI) followed a Gaussian distribution, they were analyzed using a non-parametric, unpaired t-test (GraphPad Prism 7.00). For other parameters such as smoking, drinking, and marital status, their frequencies in both cases and controls were calculated and analyzed using a paired, non-parametric t-test. For those parameters where only two pairs of values were available such as family history, alcohol consumption and vasectomy status, a two-way ANOVA test was used. To test if the STR was within Hardy–Weinberg equilibrium (HWE), a chi-square test with a confidence level of 0.05 was used (Hwe, 2016). STR genotype and allele association with prostate cancer risk and aggressiveness analysis were performed using binary logistic regression and the odds ratios (ORs) were estimated as follows. For the allele analysis, the dependent variable was the prostate cancer status and the categorical covariate was the allele. In this analysis no reference was used as the covariate variable was either presence/absence of the allele (equal to 1 or 0, respectively). For the genotype analysis, the dependent variable was the prostate cancer status and the covariate variable was the genotypes. In here, the analysis used the major allele (4/4) as the reference. To verify that age was not biasing the results, a re-analysis was performed using the allele/genotype as the categorical covariate again, age as the second covariate and case-control status or Gleason score as the dependent variables. All tests were repeated using random sampling with replacement by bootstrapping analysis. The tests were seeded 1,000,000 times in 1,000 samples. Next, we analyzed the association of TTTTG-TRIB1 STR with prostate cancer aggressiveness. A Gleason score of 8 and above is associated with a poorly differentiated or high-grade disease (Association of Directors of Anatomic and Surgical Pathology, 2018). We then grouped patients with a Gleason score of less than 8 and equal or greater than 8. Furthermore, since it has also been reported that the Gleason score pattern for a Gleason score of 7 can be an informative prognostic tool (Stark et al., 2009), where a higher primary Gleason score predicts a more lethal form of the disease, we then subdivided into 3+4 and 4+3 patients with a Gleason score = 7. Survival analysis was undertaken using the Kaplan Meier test [Log-Rank (Mantel-Cox)]. Data was plotted using GraphPad Prism 7.00. Hazard ratios (HR) were calculated using the Cox-Regression analysis for both total and prostate cancer related mortality. In this case the analysis was adjusted for age, Gleason score and PSA values. All analyses were conducted using IBM SPSS Statistics; 23.0 unless stated otherwise.
Differential TRIB1 expression in prostate cancer tissue and adjacent normal tissue and correlation of genotype and allele data with STR mRNA levels were analyzed using the non-parametric Mann–Whitney test and plotted using GraphPad Prism 7.00. A two tailed P < 0.05 value was considered significant for all the analysis.
TTTTG-TRIB1 mRNA Structure Prediction
In order to assess the potential impact that the two most common alleles of TTTTG-TRIB1 have on the TRIB1 mRNA folding structure, the in silico RNA folding predictor tool, mfold web server v.31, was used (Zuker, 2003). There are two transcript variants of TRIB1 according to the RefSeq genes data set: transcript variant 1, NM_025195.3, and transcript variant 2, NM_025195.1. Both were analyzed with the two most common alleles, three- and four-TTTTG-TRIB1 repeats. Since mfold calculates the most energetic favorable secondary structures that compose a mRNA molecule (given by their minimum free energy thermodynamic parameter, ΔG°), we hypothesized that the alleles may have an effect in the final folded structure and, potentially, in the final stability of the molecule, affecting the functionality and/or levels of the translated protein.
The Ingenuity Pathway Analysis (IPA; QIAGEN, Redwood City, CA, United States) was used to identify a list of miRNAs that bind to TRIB1 and are deregulated in prostate cancer. From these, we sought for further confirmation in the miRNet database (Fan et al., 2016). Next, microRNA.org was used to find predicted miRNAs to bind near the STR (Betel et al., 2008). Finally, RNAhybrid (Rehmsmeier et al., 2004) was applied to detect if the miRNAs’ seed region of any of these miRNAs was altered by the allele change.
Results
TRIB1 Is Highly Expressed in Prostate Cancer
Oncomine analysis (www.oncomine.org (Rhodes et al., 2004)) revealed TRIB1 is highly expressed in prostate cancer when compared to other types of cancers in two multi-cancer clinical datasets, suggesting a specific role of TRIB1 in this particular disease. Su et al. (2001) showed an overexpression (Figure 1A) in prostate cancer tissue when compared to bladder, breast, colorectal, kidney, liver, lung, ovarian, and pancreatic cancers (n = 174). Additionally, Ramaswamy et al. (2003) reported TRIB1 to be overexpressed in prostate tumor tissue when compared to breast, colorectal, lung and ovarian cancer tissues (n = 76; Figure 1B). Furthermore, two independent data sets also showed an increase of mRNA expression in prostate cancer when compared to adjacent cancer-free tissue (Figures 1C,D; Liu et al., 2006; Grasso et al., 2012, respectively), which suggests that TRIB1 may be associated with prostate cancer initiation and/or development.
Demographic and Clinical Characteristics of the Cohort
Epidemiological data is shown in Table 1. The socio-demographic and clinical characteristics analysis showed no significant differences between cases and controls for all of the parameters analyzed (Table 1). Therefore, these parameters were not used further. Only the mean of the age was significantly different between the two groups (P < 0.05). This was expected due to the nature of the recruitment of some healthy controls, where samples from random blood donors were collected and some were younger participants than the patient cohort.
The TTTTG-TRIB1 STR Is Associated With Prostate Cancer Risk but Not With Aggressiveness
In some instances, one of the technical replicates failed, either due to amplification and/or electrophoresis issues. Of the genotyped duplicates, 92% showed concordant results, satisfying the quality control criteria of STR genotyping. We then proceed to analyze our STR genotyping results. Three alleles were observed for the TTTTG-TRIB1 STR, varying from three- to five-repeats. All alleles were in HWE (P = 0.51). The three-repeat allele was associated with prostate cancer risk at the allelic (OR = 1.16; 95%; CI = 1–1.34; P = 0.044) and genotypic levels (OR = 1.70; 95% CI = 1.16–2.49; P = 0.006; Table 2). The four-repeat allele inversely associated with risk of prostate cancer at the allele level (OR = 0.57; 95% CI = 0.44–0.75; P < 0.0001; Table 2). Similar results were obtained after age adjusting and bootstrap reanalysis (Table 2). The rest of the genotypes (3/5, 4/5, and 5/5) were not analyzed due to the low number of observations (<1%).
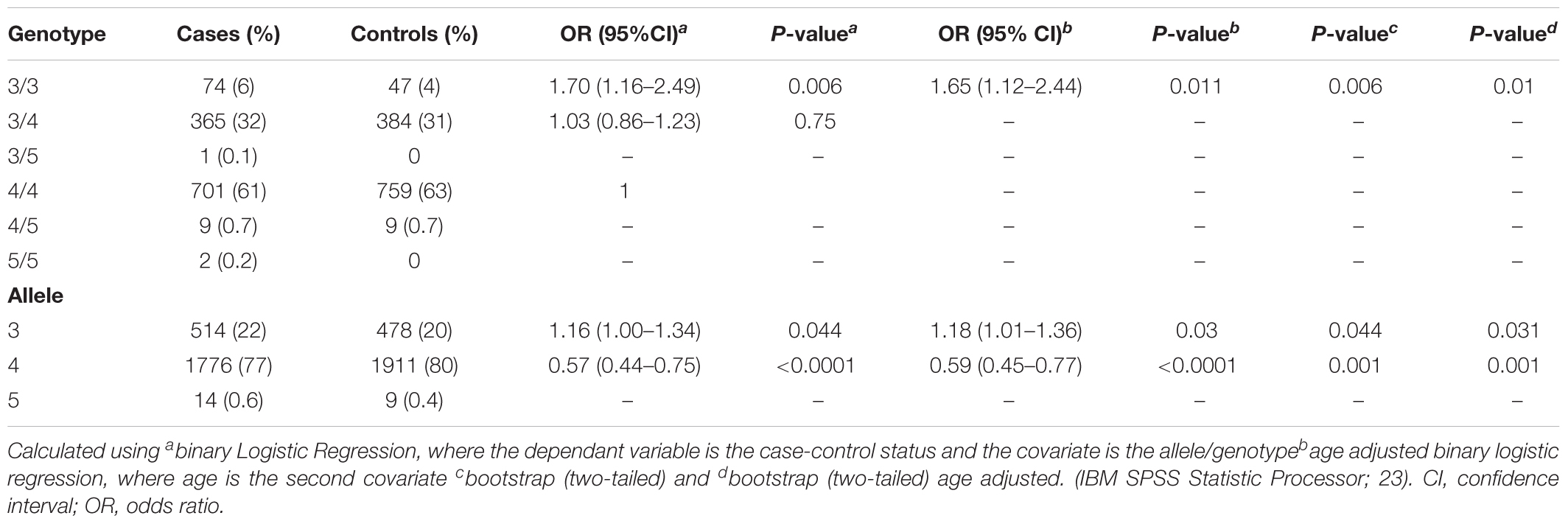
TABLE 2. Allele and genotype risk association analysis of TTTTG-TRIB1 STR with prostate cancer risk.
No significant association betweeen the genotypes and Gleason scores was obseved (P > 0.05; Supplementary Table 2), suggesting the TTTTG-TRIB1 STR alleles have no effect in the disease’s aggressiveness.
A total of 67 deaths were reported from the total cohort of 1,152 patients, of which 25 were prostate cancer-related. No significant differences in mortality were observed between the 3/3, 3/4, and 4/4 TTTTG-TRIB1 genotypes etiher for total mortality data [Log-Rank(Mantel-Cox) P = 0.695; Figure 2A] or prostate cancer related deaths [Log-Rank (Mantel-Cox) P = 0.466; Figure 2B] and these results were not affected by age, Gleason scores and PSA values (HR and their respective P-values are in Figure 2). Those patients with 5 TTTTG-TRIB1 repeats (3/5, 4/5, and 5/5) were not included in the analysis due to their low incidence (n = 1, 3 and 7, respectively).
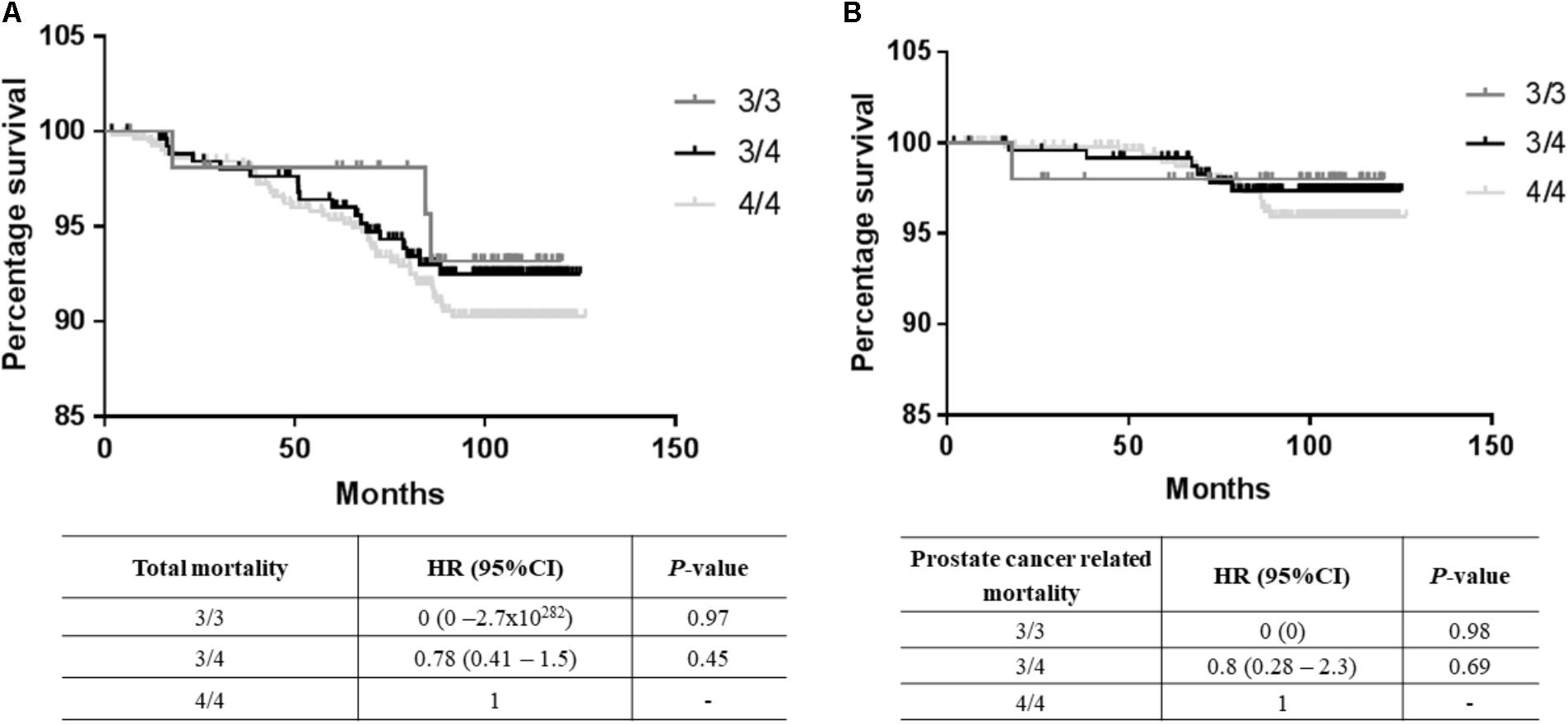
FIGURE 2. Survival data analysis. (A) Total mortality data [Log-Rank (Mantel-Cox) P-value = 0.16], (B) prostate cancer mortality data [Log-Rank (Mantel-Cox) P-value = 0.43] where TTTTG-TRIB1 genotypes (3/3, 3/4, 4/4) are represented as a function of percentage survival over 125 months. HR and P-values were calculated using Cox-Regression analysis and adjusted for age, Gleason score and PSA values. HR, hazard ratio; CI, confidence interval.
TRIB1 mRNA Expression Is Not Associated With the STR Allele
The TRIB1 gene expression showed a higher expression in prostate cancer tumor when compared to adjacent non-malignant tissue (P = 0.0005; Figure 3A). To identify if the STR alleles regulate TR1BI expression in tumor tissue, we analyzed the patient’s RNA from genotype two groups, i.e., carrying the three repeats allele or carrying the four repeats allele. No allele and TR1B1 mRNA expression correlation was observed either in the tumor (Figure 3B) or in the adjacent benign tissue (Figure 3C).
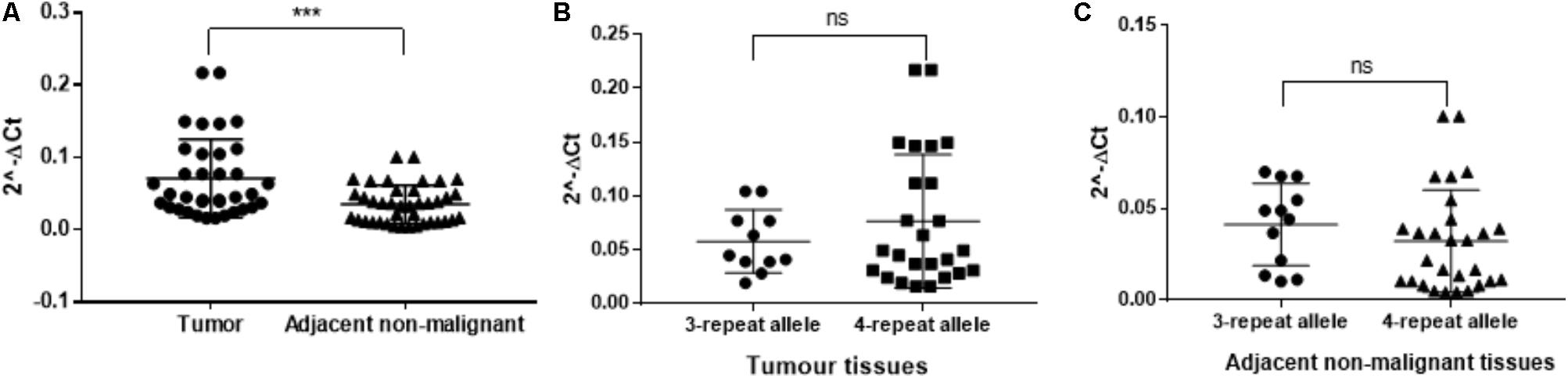
FIGURE 3. RT-qPCR from twenty patient tissue samples for TTTTG-TRIB1. Expression of the (A) TRIB1 gene in tumor tissues when compared to adjacent non-malignant tissues and expression of the 3- and 4-repeats alleles in (B) tumor tissues and (C) adjacent non-malignant tissues. ∗∗∗P = 0.0005, value calculated using the Mann–Whitney test (GraphPad Prism 7.00).
TTTTG-TRIB1 Alleles May Alter the mRNA Secondary Structure
From the 45–50 mRNA folded structures predicted by the in silico mfold tool, we focused on the top ten most energetically favorable ones, and compared how the change from three- to four-TTTTG repeats affected the structures of the two TRIB1 transcripts mentioned before. Four mRNA structures from the top ten were different as a consequence of the allelic change in the TRIB1 transcript variant 1 (NM_025195.3; example shown in Figures 4A,B). When the TRIB1 transcript variant 2 (NM_025195.1) was analyzed, there were eight mRNA predicted structures from the top ten that were different as a consequence of the allelic modification, including the most favorable structure (Figures 4C,D). This suggests the TTTTG-TRIB1 alleles influence the mRNA folding structure and potentially this could also have an impact in the expression of the translated protein, which could explain the differences in prostate cancer risk observed in this study.
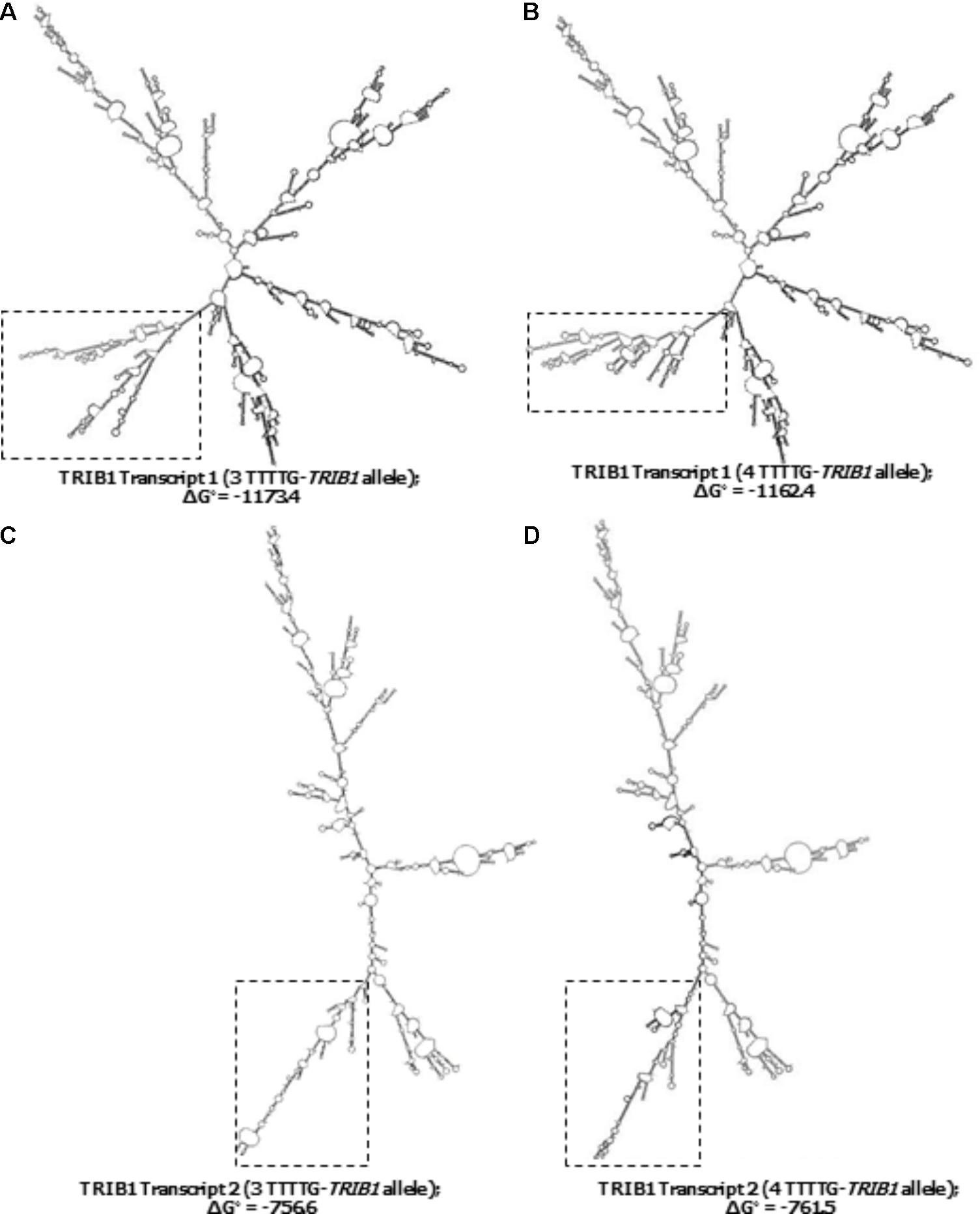
FIGURE 4. Predicted secondary structures of the two TRIB1 mRNA transcripts. Showing two examples of the differences observed in the predicted TRIB1 mRNA structure of the TRIB1 transcript variant one with the three (A) and four (B) TTTTG-TRIB1 repeats alleles; and of the TRIB1 transcript variant two with the three (C) and four (D) TTTTG-TRIB1 repeats alleles. The dotted squares highlight the secondary structures differences in the final structure. Data obtained from mfold web server v.3 (http://www.bioinfo.rpi.edu/applications/mfold.).
Since the TTTTG-TRIB1 STR is within the 3′UTR, we investigated if the alleles have an effect in regulating the TRIB1 gene expression by affecting the miRNA binding site region and interfering in the miRNA–mRNA duplex formation. The alleles of the TTTTG-TRIB1 STR were not predicted to have an effect on any of the analyzed miRNAs binding.
Discussion
Short tandem repeats are attractive biomarkers in human disease due to their highly polymorphic nature, abundancy and wide distribution throughout the genome (Schlotterer, 2004; Willems et al., 2014). They have been used for linkage mapping in several organisms, including humans (Weissenbach et al., 1992) and they have been associated with genetically simple and complex human diseases (Hefferon et al., 2004; Willems et al., 2014), including neurological disorders (Brouwer et al., 2009). A recent publication has shown massive STR sequencing (STR-Seq) is possible using the CRISPR-Cas9 technology (Shin et al., 2017). This study, published after our analysis was done, not only highlights the power of STRs as tools for genetic diagnosis, but it also shows the on-going interest of pursuing the sequencing of STRs for genetic association studies. However, this novel STR-Seq approach may not always be suitable to implement as it may depend on the resources available. In this study, we used a pre-screening approach where existing databases and bioinformatics tools were applied to detect STRs within differentially expressed genes in prostate cancer as shown in our recent publication (Lai et al., 2017). Using such methodology, we identified a penta-STR within the 3′UTR of the TRIB1 gene: TTTTG. This gene is part of the tribbles family, comprised of three genes: TRIB1, TRIB2, and TRIB3. It is a highly conserved pseudokinase (Yokoyama and Nakamura, 2011) that lacks the protein-to-protein interaction domains and possibly any catalytic activity (Wei et al., 2012). Instead of directly phosphorylating proteins, this family of genes acts as an adaptor protein that regulates several cell pathways by enabling the degradation of targeted proteins after interacting with different cell mediators (Wei et al., 2012; Dugast et al., 2013). Intracellularly, TRIB1 has an important role of regulating the C/EBP family of transcription factors (Yokoyama and Nakamura, 2011), responsible of processes such as transcription/translation and isoform formation (Hattori et al., 2003). TRIB1 is also a known regulator of the mitogen-activated protein kinase (MAPK) pathway (Sung et al., 2007; Yokoyama and Nakamura, 2011), which activates/inhibits key cell processes such as growth, proliferation, differentiation, migration, and apoptosis, all of which have a pivotal role in cancer development and progression (Dhillon et al., 2007; Roberts and Der, 2007). In fact, TRIB1 has been described as a myeloid oncogene due to its strong link with leukemia (Rothlisberger et al., 2007; Yokoyama and Nakamura, 2011). In a previously mentioned recent study, the TRIB1 gene has also shown to play a significant role in prostate cancer, where its knockdown decreased the proliferation and survival of prostate cancer cells in a three-dimensional in vitro model, as well as it promoted prostate tumorigenesis in an in vivo xenograft model after gene over-expression (Mashima et al., 2014). The increase in prostate tumor growth observed was reversed in a TRIB1 knockdown model. In addition, TRIB1 has also been shown to be downregulated by androgens in LNCaP prostate cancer cells (Munkley et al., 2015; Lai et al., 2017). Since androgens are a key signaling molecule in prostate cancer development and the main current therapeutic target (androgen deprivation therapy), this suggests TRIB1 plays a role in the onset and/or development of the disease. It is not surprising then that the publicly available database Oncomine (Rhodes et al., 2004), revealed numerous independent clinical datasets that showed an overexpression of this gene in prostate cancer tissue when compared to adjacent cancer-free cells (LaTulippe et al., 2002; Varambally et al., 2005; Liu et al., 2006; Taylor et al., 2010; Grasso et al., 2012). Interestingly, Oncomine analysis also showed that TRIB1 is highly expressed in prostate cancer when compared to other types of cancers in two independent multi-cancer studies (Su et al., 2001; Ramaswamy et al., 2003, respectively). All this evidence supports a role of TRIB1 in the onset and/or progression of the disease.
In this study, we genotyped over 2,000 patients and matching controls for the TTTTG-TRIB1 STR and found three alleles (three, four, and five repeats) in this population. The three-repeat allele showed a prostate cancer risk association at both the allele (P = 0.044) and genotype levels (P = 0.006) and this was stronger in the genotype analysis (OR = 1.16 vs. OR = 1.70). This difference could be due to a synergistic effect of the two alleles when combined. This study also showed that the allele/genotype does not affect the disease aggressiveness. Both results showed not to be associated with age after being age-adjusted. Additionally, no association with prostate cancer survival was observed, possibly due to the low number of reported deaths in our data set. Further follow ups, where more prostate cancer related deaths may be reported, could elucidate the role of this STR as a prognostic tool if such role indeed exists. We did not observe age, Gleason score or PSA had an effect on survival analysis in our cohort despite Gleason scores have previously shown to influence the prognosis of prostate cancer patients (Wright et al., 2009). Although not significant, an opposite effect of the 4/4 genotype with survival compared to its risk association with prostate cancer was observed. This could be due to the context dependent function of these alleles in the tumor microenvironment of an aggressive cancer vs. less aggressive tumor conditions, which has also been reported in recent publications such as SNP association studies (Wiklund et al., 2009; Pomerantz et al., 2010, 2011; Ahn et al., 2011). For example, a risk allele of the MSMB gene has been reported to be associated with prostate cancer compared to controls, but its association was stronger for non-fatal prostate cancer. Furthermore, the frequency of the risk allele was higher for non-fatal prostate cancer compared with fatal prostate cancer, but it was also associated with a decreased rate of progression to prostate cancer specific mortality (Wiklund et al., 2009; Pomerantz et al., 2010). Ahn et al. (2011), also reported that a risk allele in the MSMB and 8q24 genes were associated with an increased risk of metastatic prostate cancer compared with controls but not with time of recurrence in prostate cancer cases following diagnosis. Therefore, the observation that that the 4/4 genotype individuals have poorer survival (although not significant) and it is inversely associated with risk of prostate cancer is not surprising. Another limitation of this study was that our access to prostate tumor tissue and their adjacent non-tumor cells was limited in number: n = 10 for the three-TTTTG repeats and n = 18 for the four-TTTTG repeats allele, and therefore the results should be read with caution. This could explain why no significant differences in allele specific mRNA expression were observed. Future analysis, where additional samples can be included may clarify if the TRIB1 expression in prostate tumor tissue contributes to a poorer survival outcome as recently shown in a breast cancer study (Gendelman et al., 2017).
Since this STR is in the 3′UTR and its two most common alleles, three- and four-TTTTG repeats, have a difference of five nucleotides, we hypothesized this could affect either the miRNAs binding site or the final mRNA folding, and therefore have an effect in the stability and translation of the protein, potentially affecting the TRIB1 protein levels. Our miRNA analysis showed no differences in the 3′ UTR seed region when changing the STR allele from three- to four-repeats, suggesting the risk differences observed in this study are due to other regulatory mechanisms. Alternatively, there could be one or more miRNAs not yet identified that are affected by the polymorphism of the STR. We then focused on how the alleles could affect the TRIB1 mRNA structure. The predictor tool, mfold v3.0, showed the TTTTG-TRIB1 alleles altered not only the order in which a given structure was most favorable but they also promoted unique structures for the two known TRIB1 transcripts. It would be interesting to investigate further the role of these two transcripts in prostate cancer development and if the TTTTG-TRIB1 STR found herein has indeed an effect on TRIB1 mRNA stability, by possibly promoting or interfering in the translation of the mRNA. However, it is important to note that the minimum free energy method used by the mfold predictor tool has its own limitations such as assuming the RNA molecule is in equilibrium, that it has a single conformation and the nearest-neighbor effects are non-existent (Mathews, 2015). For these reasons, additional computational methods should be used to confirm the physiological significance of these findings and they also need to be experimentally validated by analyzing the allele specific protein expression of the TR1BI with a recently validated antibody (Soubeyrand et al., 2017). Finally, although we identified the TR1B1 STR through a method completely independent of GWAS, and for the first time analyzed the association of a polymorphism within the TRIB1 gene, it must be mentioned that several SNPs associated with prostate cancer have been detected by GWAS in the same locus than the TRIB1 gene (Gudmundsson et al., 2007, 2012; Srinivasan et al., 2016). It would therefore be imperative to establish the TR1B1 STR as an independent marker to GWAS identified SNPs at 8q24 for prostate cancer by undertaking the regression analysis conditioned on the GWAS loci.
In summary, the TRIB1 gene is significantly overexpressed in prostate cancer when compared to other types of cancer and it is upregulated in prostate tumor tissue when compared to adjacent cancer-free cells. After genotyping over 2,000 prostate cancer patients and controls, we found the TTTTG-TRIB1 STR is polymorphic and its three repeats allele has an association with prostate cancer risk at both the allelic and genotypic levels. However, no associations with mortality or aggressive disease were found. Further association studies in a larger cohort are warranted to confirm the outcome of our study. Our in silico predictions indicated that the TRIB1 mRNA structures are allele dependent. This repeat length could be affecting the mRNA stability and hence their expression level. Additional mechanisms by which this STR regulates TRIB1 expression in an allele specific manner need to be further explored. Collectively, these findings validate our biomarker discovery approach methodology and highlight the utility of pursuing the use of STRs as biomarkers.
Author Contributions
JL and JB conceived and designed the study and carried out bioinformatics analysis. LM and JB drafted the manuscript. LM, SS, and JP conducted the FFPE RNA extractions. LM and AH undertook the qRT-PCRs and Fragment analysis. LM and SS extracted DNA from patients’ blood samples. SC, JC, and the APCB provided the clinical samples. LM conducted the statistical analysis. JC provided vital feedback. JB supervised the study. All authors read and approved the final manuscript.
Funding
This study was supported by the National Health and Medical Research Council (NHMRC) grants (JB), IHBI ECR Grant (JB), Cancer Australia grant APP1086830 (JB), and Career Development Fellowship APP1090505 (JB). The establishment of the Australian Prostate Cancer BioResource was supported by an NHMRC Enabling Grant APP1614296 and the Prostate Cancer Foundation of Australia. JC was an NHMRC Principal Research Fellow. The Translational Research Institute was supported by a grant from the Australian Government.
Conflict of Interest Statement
The authors declare that the research was conducted in the absence of any commercial or financial relationships that could be construed as a potential conflict of interest.
Acknowledgments
We thank all the study participants who kindly donated tissue to the Australian Prostate Cancer BioResource (APCB). The APCB includes QLD node participants (T. Yeadon, P. Saunders, A. Eckert, J.A. Clements, P. Heathcote, G. Wood, G. Malone, H. Samaratunga, A. Collins, M. Turner, and K. Kerr, from the IHBI-QUT, the Brisbane Urology Clinic, Aquesta Pathology, Sullivan Nicolaides Pathology).
Supplementary Material
The Supplementary Material for this article can be found online at: https://www.frontiersin.org/articles/10.3389/fgene.2018.00428/full#supplementary-material
Footnotes
References
Ahn, J., Kibel, A. S., Park, J. Y., Rebbeck, T. R., Rennert, H., Stanford, J. L., et al. (2011). Prostate cancer predisposition loci and risk of metastatic disease and prostate cancer recurrence. Clin. Cancer Res. 7, 1075–1081. doi: 10.1158/1078-0432.ccr-10-0881
Al Olama, A. A., Kote-Jarai, Z., Berndt, S. I., Conti, D. V., Schumacher, F., Han, Y., et al. (2014). A meta-analysis of 87, 040 individuals identifies 23 new susceptibility loci for prostate cancer. Nat. Genet. 46, 1103–1109. doi: 10.1038/ng.3094
Association of Directors of Anatomic and Surgical Pathology (2018). Understanding Your Pathology Report: Prostate Cancer. Atlanta, GA: American Cancer Society.
Basch, E., Oliver, T. K., Vickers, A., Thompson, I., Kantoff, P., Parnes, H., et al. (2012). Screening for prostate cancer with prostate-specific antigen testing: american society of clinical oncology provisional clinical opinion. J. Clin. Oncol. 30, 3020–3025. doi: 10.1200/JCO.2012.43.3441
Batra, J., Lose, F., O’Mara, T., Marquart, L., Stephens, C., Alexander, K., et al. (2011a). Association between Prostinogen (KLK15) genetic variants and prostate cancer risk and aggressiveness in Australia and a meta-analysis of GWAS data. PLoS One 6:e26527. doi: 10.1371/journal.pone.0026527
Batra, J., Nagle, C. M., O’Mara, T., Higgins, M., Dong, Y., Tan, O. L., et al. (2011b). A Kallikrein 15 (KLK15) single nucleotide polymorphism located close to a novel exon shows evidence of association with poor ovarian cancer survival. BMC Cancer 11:119. doi: 10.1186/1471-2407-11-119
Batra, J., Rajpoot, R., Ahluwalia, J., Devarapu, S. K., Sharma, S. K., Dinda, A. K., et al. (2007). A hexanucleotide repeat upstream of eotaxin gene promoter is associated with asthma, serum total IgE and plasma eotaxin levels. J. Med. Genet. 44, 397–403. doi: 10.1136/jmg.2006.046607
Betel, D., Wilson, M., Gabow, A., Marks, D. S., and Sander, C. (2008). The microRNA.org resource: targets and expression. Nucleic Acids Res. 36, D149–D153. doi: 10.1093/nar/gkm995
Brouwer, J. R., Willemsen, R., and Oostra, B. A. (2009). Microsatellite repeat instability and neurological disease. Bioessays 31, 71–83. doi: 10.1002/bies.080122
Budowle, B., Chidambaram, A., Strickland, L., Beheim, C. W., Taft, G. M., and Chakraborty, R. (2002). Population studies on three native Alaska population groups using STR loci. Forensic Sci. Int. 129, 51–57. doi: 10.1016/S0379-0738(02)00209-8
Dhillon, A. S., Hagan, S., Rath, O., and Kolch, W. (2007). MAP kinase signalling pathways in cancer. Oncogene 26, 3279–3290. doi: 10.1038/sj.onc.1210421
Dugast, E., Kiss-Toth, E., Soulillou, J.-P., Brouard, S., and Ashton-Chess, J. (2013). Current Molecular Medicine. Sharjah: Bentham Science Publishers.
Edwards, A., Hammond, H. A., Jin, L., Caskey, C. T., and Chakraborty, R. (1992). Genetic variation at five trimeric and tetrameric tandem repeat loci in four human population groups. Genomics 12, 241–253. doi: 10.1016/0888-7543(92)90371-X
Eeles, R. A., Olama, A. A., Benlloch, S., Saunders, E. J., Leongamornlert, D. A., Tymrakiewicz, M., et al. (2013). Identification of 23 new prostate cancer susceptibility loci using the iCOGS custom genotyping array. Nat. Genet. 45, 385–391, 391e1-2. doi: 10.1038/ng.2560
Etzioni, R., Penson, D. F., Legler, J. M., di Tommaso, D., Boer, R., Gann, P. H., et al. (2002). Overdiagnosis due to prostate-specific antigen screening: lessons from U.S. Prostate Cancer Incidence Trends. J. Natl. Cancer Inst. 94, 981–990. doi: 10.1093/jnci/94.13.981
Fan, Y., Siklenka, K., Arora, S. K., Ribeiro, P., Kimmins, S., and Xia, J. (2016). miRNet - dissecting miRNA-target interactions and functional associations through network-based visual analysis. Nucleic Acids Res. 44, W135–W141. doi: 10.1093/nar/gkw288
Gendelman, R., Xing, H., Mirzoeva, O. K., Sarde, P., Curtis, C., Feiler, H., et al. (2017). Bayesian network inference modeling identifies TRIB1 as a novel regulator of cell cycle progression and survival in cancer cells. Cancer Res. 77, 1575–1585. doi: 10.1158/0008-5472.can-16-0512
Grasso, C. S., Wu, Y. M., Robinson, D. R., Cao, X., Dhanasekaran, S. M., Khan, A. P., et al. (2012). The mutational landscape of lethal castration-resistant prostate cancer. Nature 487, 239–243. doi: 10.1038/nature11125
Gudmundsson, J., Sulem, P., Gudbjartsson, D. F., Masson, G., Agnarsson, B. A., Benediktsdottir, K. R., et al. (2012). A study based on whole-genome sequencing yields a rare variant at 8q24 associated with prostate cancer. Nat. Genet. 44, 1326–1329. doi: 10.1038/ng.2437
Gudmundsson, J., Sulem, P., Manolescu, A., Amundadottir, L. T., Gudbjartsson, D., Helgason, A., et al. (2007). Genome-wide association study identifies a second prostate cancer susceptibility variant at 8q24. Nat. Genet. 39, 631–637. doi: 10.1038/ng1999
Hattori, T., Ohoka, N., Inoue, Y., Hayashi, H., and Onozaki, K. (2003). C/EBP family transcription factors are degraded by the proteasome but stabilized by forming dimer. Oncogene 22, 1273–1280. doi: 10.1038/sj.onc.1206204
Hefferon, T. W., Groman, J. D., Yurk, C. E., and Cutting, G. R. (2004). A variable dinucleotide repeat in the CFTR gene contributes to phenotype diversity by forming RNA secondary structures that alter splicing. Proc. Natl. Acad. Sci. U.S.A. 101, 3504–3509. doi: 10.1073/pnas.0400182101
9Howrey, B. T., Kuo, Y.-F., Lin, Y.-L., and Goodwin, J. S. (2013). The impact of PSA screening on prostate cancer mortality and overdiagnosis of prostate cancer in the United States. J. Gerontol. A Biol. Sci. Med. Sci. 68, 56–61. doi: 10.1093/gerona/gls135
Hwe, A. (2016). Hardy-Weinberg Equilibrium Calculator. Available at: http://www.had2know.com/academics/hardy-weinberg-equilibrium-calculator-3-alleles.html
Jemal, A., Bray, F., Center, M. M., Ferlay, J., Ward, E., and Forman, D. (2011). Global cancer statistics. CA Cancer J. Clin. 61, 69–90. doi: 10.3322/caac.20107
Kong, A., Gudbjartsson, D. F., Sainz, J., Jonsdottir, G. M., Gudjonsson, S. A., Richardsson, B., et al. (2002). A high-resolution recombination map of the human genome. Nat. Genet. 31, 241–247. doi: 10.1038/ng917
Lai, J., Moya, L., An, J., Hoffman, A., Srinivasan, S., Panchadsaram, J., et al. (2017). A microsatellite repeat in PCA3 long non-coding RNA is associated with prostate cancer risk and aggressiveness. Sci. Rep. 7:16862. doi: 10.1038/s41598-017-16700-y
LaTulippe, E., Satagopan, J., Smith, A., Scher, H., Scardino, P., Reuter, V., et al. (2002). Comprehensive gene expression analysis of prostate cancer reveals distinct transcriptional programs associated with metastatic disease. Cancer Res. 62, 4499–4506.
Liu, C., Kelnar, K., Liu, B., Chen, X., Calhoun-Davis, T., Li, H., et al. (2011). The microRNA miR-34a inhibits prostate cancer stem cells and metastasis by directly repressing CD44. Nat. Med. 17, 211–215. doi: 10.1038/nm.2284
Liu, P., Ramachandran, S., Ali Seyed, M., Scharer, C. D., Laycock, N., Dalton, W. B., et al. (2006). Sex-determining region Y box 4 is a transforming oncogene in human prostate cancer cells. Cancer Res. 66, 4011–4019. doi: 10.1158/0008-5472.can-05-3055
Lose, F., Srinivasan, S., O’Mara, T., Marquart, L., Chambers, S., Gardiner, R. A., et al. (2012). Genetic association of the KLK4 locus with risk of prostate cancer. PLoS One 7:e44520. doi: 10.1371/journal.pone.0044520
Mashima, T., Soma-Nagae, T., Migita, T., Kinoshita, R., Iwamoto, A., Yuasa, T., et al. (2014). TRIB1 supports prostate tumorigenesis and tumor-propagating cell survival by regulation of endoplasmic reticulum chaperone expression. Cancer Res. 74, 4888–4897. doi: 10.1158/0008-5472.can-13-3718
Mathews, D. (2015). Lab III: Computational Biology and RNA Structure Prediction. Rochester, NY: University of Rochester Medical Center.
Moyer, V. A., and U. S. Preventive Services Task Force. (2012). Screening for prostate cancer: US preventive services task force recommendation statement. Ann. Intern. Med. 157, 120–134. doi: 10.7326/0003-4819-157-2-201207170-00459
Munkley, J., Lafferty, N. P., Kalna, G., Robson, C. N., Leung, H. Y., Rajan, P., et al. (2015). Androgen-regulation of the protein tyrosine phosphatase PTPRR activates ERK1/2 signalling in prostate cancer cells. BMC Cancer 15:9. doi: 10.1186/s12885-015-1012-8
Pomerantz, M. M., Shrestha, Y., Flavin, R. J., Regan, M. M., Penney, K. L., Mucci, L. A., et al. (2010). Analysis of the 10q11 cancer risk locus implicates MSMB and NCOA4 in human prostate tumorigenesis. PLoS Genet. 6:e1001204. doi: 10.1371/journal.pgen.1001204
Pomerantz, M. M., Werner, L., Xie, W., Regan, M. M., Lee, G. S., Sun, T., et al. (2011). Association of prostate cancer risk Loci with disease aggressiveness and prostate cancer-specific mortality. Cancer Prev Res. 4, 719–728. doi: 10.1158/1940-6207.capr-10-0292
Ramaswamy, S., Ross, K. N., Lander, E. S., and Golub, T. R. (2003). A molecular signature of metastasis in primary solid tumors. Nat. Genet. 33, 49–54. doi: 10.1038/ng1060
Rehmsmeier, M., Steffen, P., Hochsmann, M., and Giegerich, R. (2004). Fast and effective prediction of microRNA/target duplexes. RNA 10, 1507–1517. doi: 10.1261/rna.5248604
Rhodes, D. R., Yu, J., Shanker, K., Deshpande, N., Varambally, R., Ghosh, D., et al. (2004). ONCOMINE: a cancer microarray database and integrated data-mining platform. Neoplasia 6, 1–6. doi: 10.1016/S1476-5586(04)80047-2
Roberts, P. J., and Der, C. J. (2007). Targeting the Raf-MEK-ERK mitogen-activated protein kinase cascade for the treatment of cancer. Oncogene 26, 3291–3310. doi: 10.1038/sj.onc.1210422
Rothlisberger, B., Heizmann, M., Bargetzi, M. J., and Huber, A. R. (2007). TRIB1 overexpression in acute myeloid leukemia. Cancer Genet. Cytogenet. 176, 58–60. doi: 10.1016/j.cancergencyto.2007.03.003
Schlotterer, C. (2004). The evolution of molecular markers [mdash] just a matter of fashion? Nat. Rev. Genet. 5, 63–69. doi: 10.1038/nrg1249
Schumacher, F., Al Olama, A. A., Berndt, S., Benlloch, S., Ahmed, E., Saunders, E., et al. (2018). Association analyses of more than 140, 000 men identifies 63 novel prostate cancer susceptibility loci. Nat. Genet. 50, 928–936. doi: 10.1038/s41588-018-0142-8
Shin, G., Grimes, S. M., Lee, H., Lau, B. T., Xia, L. C., and Ji, H. P. (2017). CRISPR-Cas9-targeted fragmentation and selective sequencing enable massively parallel microsatellite analysis. Nat. Commun. 8:14291. doi: 10.1038/ncomms14291
Soubeyrand, S., Martinuk, A., and McPherson, R. (2017). TRIB1 is a positive regulator of hepatocyte nuclear factor 4-alpha. Sci. Rep. 7:5574. doi: 10.1038/s41598-017-05768-1
Srinivasan, S., Clements, J. A., and Batra, J. (2016). Single nucleotide polymorphisms in clinics: fantasy or reality for cancer? Crit. Rev. Clin. Lab. Sci. 53, 29–39. doi: 10.3109/10408363.2015.1075469
Stark, J. R., Perner, S., Stampfer, M. J., Sinnott, J. A., Finn, S., Eisenstein, A. S., et al. (2009). Gleason score and lethal prostate cancer: does 3 + 4 = 4 + 3? J. Clin. Oncol. 27, 3459–3464. doi: 10.1200/jco.2008.20.4669
Su, A. I., Welsh, J. B., Sapinoso, L. M., Kern, S. G., Dimitrov, P., Lapp, H., et al. (2001). Molecular classification of human carcinomas by use of gene expression signatures. Cancer Res. 61, 7388–7393.
Sung, H. Y., Guan, H., Czibula, A., King, A. R., Eder, K., Heath, E., et al. (2007). Human tribbles-1 controls proliferation and chemotaxis of smooth muscle cells via MAPK signalling pathways. J. Biol. Chem. 282, 18379–18387. doi: 10.1074/jbc.M610792200
Taylor, B. S., Schultz, N., Hieronymus, H., Gopalan, A., Xiao, Y., Carver, B. S., et al. (2010). Integrative genomic profiling of human prostate cancer. Cancer Cell 18, 11–22. doi: 10.1016/j.ccr.2010.05.026
Vandesompele, J., De Preter, K., Pattyn, F., Poppe, B., Van Roy, N., De Paepe, A., et al. (2002). Accurate normalization of real-time quantitative RT-PCR data by geometric averaging of multiple internal control genes. Genome Biol. 3, research0034.1– research0034.11. doi: 10.1186/gb-2002-3-7-research0034
Varambally, S., Yu, J., Laxman, B., Rhodes, D. R., Mehra, R., Tomlins, S. A., et al. (2005). Integrative genomic and proteomic analysis of prostate cancer reveals signatures of metastatic progression. Cancer Cell 8, 393–406. doi: 10.1016/j.ccr.2005.10.001
Wang, Y., Wu, N., Pang, B., Tong, D., Sun, D., Sun, H., et al. (2017). TRIB1 promotes colorectal cancer cell migration and invasion through activation MMP-2 via FAK/Src and ERK pathways. Oncotarget 8, 47931–47942. doi: 10.18632/oncotarget.18201
Wei, S. C., Rosenberg, I. M., Cao, Z., Huett, A. S., Xavier, R. J., and Podolsky, D. K. (2012). Tribbles 2 (Trib2) is a novel regulator of Toll-like receptor 5 signaling. Inflamm. Bowel Dis. 18, 877–888. doi: 10.1002/ibd.22883
Weissenbach, J., Gyapay, G., Dib, C., Vignal, A., Morissette, J., Millasseau, P., et al. (1992). A second-generation linkage map of the human genome. Nature 359, 794–801. doi: 10.1038/359794a0
Welfare Aloha (2016). Australian Cancer Incidence and Mortality (ACIM) Books. Bruce: Australian Institute of Health and Welfare.
Wiklund, F. E., Adami, H. O., Zheng, S. L., Stattin, P., Isaacs, W. B., Gronberg, H., et al. (2009). Established prostate cancer susceptibility variants are not associated with disease outcome. Cancer Epidemiol. Biomarkers Prev. 18, 1659–1662. doi: 10.1158/1055-9965.epi-08-1148
Willems, T., Gymrek, M., Highnam, G., 1000 Genomes Project Consortium, Mittelman, D., and Erlich, Y. (2014). The landscape of human STR variation. Genome Res. 24, 1894–1904. doi: 10.1101/gr.177774.114
Wright, J. L., Salinas, C. A., Lin, D. W., Kolb, S., Koopmeiners, J., Feng, Z., et al. (2009). Prostate cancer specific mortality and Gleason 7 disease differences in prostate cancer outcomes between cases with Gleason 4 + 3 and Gleason 3 + 4 tumors in a population based cohort. J. Urol. 182, 2702–2707. doi: 10.1016/j.juro.2009.08.026
Ye, Y., Wang, G., Wang, G., Zhuang, J., He, S., Song, Y., et al. (2017). The oncogenic role of tribbles 1 in hepatocellular carcinoma is mediated by a feedback loop involving microRNA-23a and p53. Front. Physiol. 8:789. doi: 10.3389/fphys.2017.00789
Yokoyama, T., and Nakamura, T. (2011). Tribbles in disease: signaling pathways important for cellular function and neoplastic transformation. Cancer Sci. 102, 1115–1122. doi: 10.1111/j.1349-7006.2011.01914.x
Yoshida, A., Kato, J. Y., Nakamae, I., and Yoneda-Kato, N. (2013). COP1 targets C/EBPalpha for degradation and induces acute myeloid leukemia via Trib1. Blood 122, 1750–1760. doi: 10.1182/blood-2012-12-476101
Yu, Y. P., Landsittel, D., Jing, L., Nelson, J., Ren, B., Liu, L., et al. (2004). Gene expression alterations in prostate cancer predicting tumor aggression and preceding development of malignancy. J. Clin. Oncol. 22, 2790–2799. doi: 10.1200/jco.2004.05.158
Keywords: short tandem repeats (STRs), microsatellite, tribbles homologue 1 gene (TRIB1), prostate cancer, biomarker
Citation: Moya L, Lai J, Hoffman A, Srinivasan S, Panchadsaram J, Chambers S, Clements JA, Batra J and Australian Prostate Cancer BioResource (2018) Association Analysis of a Microsatellite Repeat in the TRIB1 Gene With Prostate Cancer Risk, Aggressiveness and Survival. Front. Genet. 9:428. doi: 10.3389/fgene.2018.00428
Received: 19 January 2018; Accepted: 10 September 2018;
Published: 04 October 2018.
Edited by:
Logan Walker, University of Otago, New ZealandReviewed by:
Weikuan Gu, The University of Tennessee Health Science Center, United StatesSharon E. Johnatty, QIMR Berghofer Medical Research Institute, Australia
Copyright © 2018 Moya, Lai, Hoffman, Srinivasan, Panchadsaram, Chambers, Clements, Batra and Australian Prostate Cancer BioResource. This is an open-access article distributed under the terms of the Creative Commons Attribution License (CC BY). The use, distribution or reproduction in other forums is permitted, provided the original author(s) and the copyright owner(s) are credited and that the original publication in this journal is cited, in accordance with accepted academic practice. No use, distribution or reproduction is permitted which does not comply with these terms.
*Correspondence: Jyotsna Batra, jyotsna.batra@qut.edu.au
†The participants of the Australian Prostate Cancer BioResource are listed in the Acknowledgements section.