- State Key Laboratory for Pig Genetic Improvement and Production Technology, Jiangxi Agricultural University, Nanchang, China
The whole-genome sequences of progenies with low-density single-nucleotide polymorphism (SNP) genotypes can be imputed with high accuracy based on the deep-coverage sequences of key ancestors. With this imputation technology, a more powerful genome-wide association study (GWAS) can be carried out using imputed whole-genome variants and the phenotypes of interest to overcome the shortcomings of low-power detection and the large confidence interval derived from low-density SNP markers in classic association studies. In this study, 19 ancestors of a large-scale swine F2 White Duroc × Erhualian population were deeply sequenced for their genome with an average coverage of 25×. Considering 98 pigs from 10 different breeds with high-quality deep sequenced genomes, we imputed the whole genomic variants of 1020 F2 pigs genotyped by the PorcineSNP60 BeadChip with high accuracy and obtained 14,851,440 sequence variants after quality control. Based on this, 87 novel quantitative traits loci (QTLs) for 18 hematological traits at three different physiological stages of the F2 pigs were identified, among which most of the novel QTLs have been repeated in two of the three stages. Literature mining pinpointed that the FGF14 and LCLAT1 genes at SSC11 and SSC3 may affect the MCH at day 240 and MCV at day 18, respectively. The present study shows that combining high-quality imputed genomic variants and correlated phenomic traits into GWAS can improve the capability to detect QTL considerably. The large number of different QTLs for hematological traits identified at multiple growth stages implies the complexity and time specificity of these traits.
Introduction
Hematological traits, which are associated with the immune system (Oddgeirsson et al., 1988; Muller and Brem, 1991), have been widely used as biomarkers to detect the signs of diseases and disease severity in human and animals. Hematological cells consist of three different kinds of cells: leukocytes (white blood cells, WBCs), erythrocytes (red blood cells, RBCs), and platelets, which perform different roles in blood (Tullis, 1952). WBCs are called immune cells and play the function of defending against bacteria or fungi and protecting an organism from disease. RBCs exchange oxygen and carbon dioxide in the respiration of vertebrate animals. Platelets play key roles in wound healing and inflammatory response (Toby Simon, 2001). Identifying highly associated SNPs and deciphering the genetic mechanism of hematological traits would help in dealing with hematological diseases.
The researchers have paid considerable attention to hematological traits in humans. The heritabilities of RBC, WBC, and platelet count have been estimated to be 0.42, 0.62, and 0.57, respectively, indicating moderately or highly heritable traits (Garner et al., 2000). Some heritability studies on twins have proven that hematological traits are highly heritable and tightly interactive (Evans et al., 1999; Garner et al., 2000). In 2012, common genetic factors for hematological traits in humans were reviewed in detail by Okada and Kamatani (2012). In the last 5 years, several missense variants in CXCR2 associated with the reduction of WBC count, a common missense variant in CPS1 and a rare synonymous variant in GFI1B that caused a lower platelet count, and a mass of genes and loci-related hematological parameters have been detected by adopting the genome-wide association studies (GWAS) approach (van der Harst et al., 2012; Auer et al., 2014; Polfus et al., 2016; Ulirsch et al., 2016). Due to the strong similarity with human physiological characteristics, the domestic pig serves as a suitable model animal in elucidating the genetic mechanism of hematological traits in humans (Swindle et al., 2012).
To date, a total of 356 quantitative trait loci (QTLs) associated with swine hematological traits have been identified by linkage mapping recorded in the AnimalQTLdb database (Hu et al., 2007), but because of the large confidence intervals and large number of genes in these QTL regions, the identification of potential causal genes would be obstructed to some extent. With the discovery of massive numbers of genetic markers and the advent of high-throughput technology to genotype animals for hundreds of thousands of single-nucleotide polymorphisms (SNPs) in a cost-effective way, GWAS is widely applied in the fields of human and livestock for traits of interest. For the hematological traits in pigs, hundreds of significant SNPs located in the whole genome except chromosomes 14 and 16 were identified using the PorcineSNP60 BeadChip (Illumina Inc., San Diego, CA, United States) based on GWAS in diversified pig populations (Luo et al., 2012; Wang et al., 2013; Zhang et al., 2013, 2014; Jung et al., 2014; Ponsuksili et al., 2016). The effects of uncovered significant loci are population- or breed-specific and only a small fraction of loci are consistent among these studies, implying the complexity and heterogeneity of hematological traits. On the other hand, those massive significant SNPs can explain only a relatively small fraction of phenotypic variance, ranging from 4.75 to 19.41% of hematological traits (Zhang et al., 2014). The remaining and missing heritability has been discussed in 2009 (Manolio et al., 2009). A fairly stringent statistical threshold, numerous small-effect loci affecting traits, and the relative low linkage disequilibrium (LD) among markers should be most likely responsible for the missing heritability. All these genes with small effects can together explain the majority of the genetic variance for most of these traits (Yang et al., 2010).
In our previous study, we performed a GWAS analysis in a White Duroc × Erhualian F2 resource population using the PorcineSNP60 BeadChip, and we identified 185 significant SNPs distributed on chromosomes 1, 4, 5, 7, 8, 10, 11, 12, 13, 17, and 18, affecting hematological traits at three different growth stages (Zhang et al., 2013). To obtain the majority of the remaining genetic variance and to overcome the weakness of low efficiency caused by low LD in detecting small-effect QTLs when using low-density BeadChip (Supplementary Figure S1), we carried out a single- and multitrait association study using imputation-based whole-genome sequence data in the same F2 resource population. As causal variants and higher LD may have been included in the whole-genome sequences, the detection power for small-effect variants would improve. We herein aim to explore more loci associated with hematological traits using a whole-genome sequence based on imputation in pigs.
Materials And Methods
Ethics Statement
All the processes involving animals are in accordance with the care and the guidelines of experimental animals established by the Ministry of Agriculture and Rural Affairs of the China. The ethics committee of Jiangxi Agricultural University specifically approved this study.
Animals and Phenotypic Measurements
The description of the White Duroc × Erhualian F2 resource population and the related method of phenotypic measurement have been described in detail in our previous publications (Zou et al., 2008; Guo et al., 2009; Yang et al., 2009). In short, a total of 1912 F2 individuals were produced from two White Duroc boars and seventeen Erhualian sows. All F2 piglets were fed under the same circumstance in a pig farm at Jiangxi Agricultural University. Eighteen hematological parameters from 1449 F2 individuals were measured at three growth-age stages, i.e., days at 18, 46, and 240. These hematological parameters include seven erythroid parameters [hematocrit (HCT), hemoglobin (HGB), mean corpuscular hemoglobin (MCH), mean corpuscular hemoglobin concentration (MCHC), mean corpuscular volume (MCV), red blood cell count (RBC), and red blood cell volume distribution width (RDW)], seven leukocyte parameters [granulocyte count (GRAN), granulocyte count percentage (GRAR), monocyte count (MON), monocyte count percentage (MONR), lymphocyte count (LYM), lymphocyte count percentage (LYMA), and white blood cell count (WBC)], and four platelet parameters [plateletcrit (PCT), platelet distribution width (PDW), platelet count (PLT), and mean platelet volume (MPV)].
Processing With Reference and Target Panels
One hundred and seventeen individuals with whole-genome sequence data produced by the Illumina HiSeq 2000 platform with an average depth of ∼25 coverages were introduced as the reference panel; of the individuals, 19 F0 individuals are the progenitors of the 933 F2 resource populations (target panel) and 98 unrelated pigs are from nine diverse breeds and one wild boar population. Sixty-nine unrelated pigs were used in a study of adaptation and introgression project (Ai et al., 2015). Briefly, these 69 pigs comprising 6 Bamaxiang, 6 Luchuan, 6 Wuzhishan, 6 Jiangxi Wild boar, 5 Erhualian, 6 Hetao, 6 Laiwu, 6 Min, 4 Gansu Tibetan, 6 Sichuan Tibetan, 6 Tibet Tibetan, and 6 Yunnan Tibetan represent both low- and high-latitude populations of pigs in China. After being sequenced, the raw reads were firstly trimmed based on a quality score threshold >15; reads that passed the chastity filtering were then aligned to the reference porcine genome assembly Sus-scrofa 10.2 (Groenen et al., 2012) using the Burrows–Wheeler aligner tool (Li and Durbin, 2009). Variants were called following the genome analysis toolkit (GATK) (McKenna et al., 2010) best practice protocol. PCR duplications were firstly marked by Picard MarkDuplicates1, and local realignments were performed with the GATK IndelRealigner option. Then, variants were filtered with the GATK VariantFiltration option, and insertions and deletions were performed with VCFtools (Danecek et al., 2011).
A subset of this resource population with 62,163 loci detected by PorcineSNP60 BeadChip was treated as the target panel. The target panel included 19 F0, 68 F1 and 933 F2. The 19 F0 ancestors included both resequencing variants and 60K genotypes. Individuals were genotyped by the PorcineSNP60 BeadChip following a standard protocol on an iScan system. A more complete description, including methods and the criteria of quality control (QC) of this SNP dataset can be found in our previous report (Zhang et al., 2013). To keep the alleles consistent with the reference panel, the following analysis was carried out. The information of forward or reverse strands of each SNP in the PorcineSNP60 BeadChip was firstly annotated by BLAST (Altschul et al., 1990). Then, the reversed SNPs in the target panel were flipped by PLINK (v1.9) software (Chang et al., 2015). The SNPs without specific information of chromosomes and (or) positions were excluded from further analysis.
Haplotype Construction of Reference Panel and Target Panel
The accuracy of the haplotypes of the ancestors (F0) is critical for high-quality imputation of the F2 population. The number of individuals present in a population is a crucial factor in determining how well the phase can be estimated for haplotype construction. Browning and Browning (2011) and Williams et al. (2012) have fully demonstrated the relationship between sample size and haplotype accuracy; the more individuals, the better the haplotype estimation. In our study, to obtain more accurate phases of the 19 founders, an additional 98 unrelated pigs were added into the reference panel. The haplotypes of the reference panel were constructed by SHAPEIT (v2 r837) (Delaneau et al., 2013). To improve the accuracy of the construction of haplotypes, phase informative reads (PIRs) that span at least two heterozygous sites were firstly extracted from aligned bam files by using the extractPIRs program from SHAPEIT appendix, setting the –base-quality and –read-quality options to 20 and 30, respectively. Combined with the variants files, PIRs were then involved in haplotype construction using the standard Markov chain Monte Carlo iterative procedure of the phasing module (-assemble) in SHAPEIT and the window was set to 0.2 Mb by the –window parameter. Haplotypes of the target panel were constructed and improved by taking advantage of both linkage and LD information using DualPhase software; It firstly phased a large proportion of loci and individuals based on Mendelian rules and linkage information using the pedigree relationship of the F2 population; then, the remaining small part of the unphased loci were haplotyped based on a hidden Markov model (Druet and Georges, 2010).
Imputation of Sequence Variants
Subsequently, the genotype imputation between the target and reference panels with known phase information were performed by IMPUTE2 (v.2.3.2) (Howie et al., 2009). Specifically, the option of -use_prehased_g was required to set IMPUTE2 in the prephasing mode. Then, the size of the region to be imputed on the current chromosome was set to a 5 Mb window with the -int option. Finally, the other options used in the imputation follow the default setting. Imputation accuracy was automatically assessed by an internal cross-validation strategy in IMPUTE2. Briefly, it masked the variants of one SNP in the target panel at a time, and then the masked genotypes were imputed by the information of the reference panel and the nearby studied variants. Then, the genotypic concordance rate and the squared correlation (R2) between the best-guess imputed and the original variants were calculated as imputation accuracy.
To investigate the imputation accuracy further, we carried out whole-genome resequencing for five experimental animals in the present study population including two F1 and three F2 individuals. The SNP calling of these five individuals was performed following the pipeline described above. The R2 value between the sequenced variants and the best-guess imputed variants across chromosomes was calculated.
Furthermore, to obtain relatively accurate imputation results that would be used for the following GWAS, a series of methods were applied into the GEN file format from the imputation result. As the GEN file contains the information of genotype probability, we used a cutoff of 0.5 to convert the GEN file into a common PED file by GTOOL2 as it best balanced the imputation accuracy and the missing proportion in the next analysis process. Then, variants with a call rate <90% and a minor allele frequency (MAF) <0.01 were further excluded by PLINK.
Phenotypic and Genetic Correlation
The estimated breeding value (EBV) of all experimental animals for 54 hematological traits were estimated by Bayesian sparse linear mixed models implemented in Gemma (v.0.94) (Zhou et al., 2013). The phenotypic correlation and the genetic correlation were calculated by using the cor function using the Pearson correlation coefficient in the stats package.
Single-Trait GWAS Analysis
A univariate linear mixed model (see Eq. 1) implemented in GEMMA (v.0.94) is employed for the single-marker association test between variants and phenotypes (Zhou and Stephens, 2012), and is described in the following equation:
where y is the vector of phenotypic observation; W is a design matrix of covariates, including a column of 1 s; α is a vector of fixed effects (e.g., gender); x is a vector of genotypes at each locus; β is the effect of the marker; u is a vector of random effects following the multivariate normal distribution (see Eq. 1), in which τ-1 is the variance of the residual errors, λ is the ratio between τ-1 and the variance of polygenetic effects, and K is a kinship matrix estimated from whole-genome sequence variants; 𝜀 is a vector of errors following the multivariate normal distribution (see Eq. 1) and In is an identity matrix. Using naïve Bonferroni corrections of 0.05 divided by the number of examined SNPs to correct multiple comparisons would lead to an overly conservative threshold in our study because these SNPs were highly correlated with each other. Pe’er et al. (2008) and Johnson et al. (2010) suggested that 5E-08 could serve as a genome-wide significant threshold in human GWASs based on independent haplotype blocks of an African population structure. Based on the assumption that an equal number of independent haplotype segments between pigs and humans are held, we used the same genome-wide threshold in our study.
Multitrait GWAS Analysis
Hierarchical clustering of the traits in multitrait-GWAS was performed by using the function corrplot in the corrplot R package with the agglomeration method of “complete,” which employs the longest distance principle to generate clusters. Based on the clusters of phenotypic and genetic correlation from different stages, the multitrait association tests between variants and multiple correlated phenotypes were carried out by the multivariate linear mixed model (see Eq. 2) implemented in GEMMA (v. 0.94) (Zhou and Stephens, 2014), which is described in the following equation:
where n and d are the numbers of individuals and phenotypes, respectively. Y is a n by d matrix of phenotypic observation; W is an n by c design matrix of covariates, including a column of 1 s; A is a c by d matrix of fixed effects (e.g., gender); x is a vector of genotypes at each locus; β is a d vector of marker effect sizes for d phenotypes; U is an n by d matrix of random effects following the n × d matrix normal distribution (see Eq. 2), in which K is a relatedness matrix generated by genotypes of n individuals, and Vg is a d by d matrix of genetic variance components; E is an n by d matrix of errors, in which In×n is an n by n identity matrix, and Ve is a d by d matrix of environmental variance components. The genome-wide significant threshold in multitrait GWAS was also set to 5E-08 as mentioned above.
Results
Phenotypic and Genetic Correlations
In this study, 1020 individuals in three generations, including 19 F0, 68 F1, and 933 F2 were genotyped by the PorcineSNP60 BeadChip. The number of individuals measured for each trait ranged from 181 to 924 (Supplementary Table S1). The detailed descriptive statistics of these 18 hematological traits were presented in our previous report (Zhang et al., 2013).
The detailed information about phenotypic correlation and genetic correlation across the three different stages were presented (Supplementary Figure S2). Some traits were excluded because there were too few records in those traits (Supplementary Table S1). There is a highly similar correlation pattern between the phenotypic correlation and genetic correlation for these traits; for example, traits with a high positive phenotypic correlation also have a high positive genetic correlation and vice versa. To further explore potential common genetic factors for multiple traits, we performed clustering analysis for the hematological traits using their genetic correlation matrix. Briefly, HCT, HGB, MCH, and MCV at day 18 were clustered to one group (Supplementary Figure S2A). At day 46, there was also only one cluster with many significant positive correlations among traits including GRAN, GRAR, MCH, and MCHC (Supplementary Figure S2B). For day 240, RDW, PCT, PLT, LYM, and LYMA were clustered to one group and MON, MONR, GRAN, GRAR, RBC, HCT, and HGB traits were clustered into another group (Supplementary Figure S2C). Here, a correlation cluster including at least four traits was used in the multitrait GWAS analysis process.
Summary Imputation
The distributions of proportion of the variants across MAF for 60 K and imputation results before QC are compared in Figure 1A. Both of them had a more than 15% homozygote rate in this population. In general, the imputed variants had a larger proportion of low frequency loci (below 0.2) when compared to 60K SNP classes. Finally, 1020 individuals with 40,057 sites were regarded as the target panel. More than 20 million variants were imputed for 1020 animals. After trimming the genotype probability with a threshold of 0.5, several variants contained missing values in PED files. We repeated the QC process using thresholds of missing rate of each variant and MAF. 2,572,745 variants with a low call rate (0.9) and 4,200,615 variants with a low MAF (0.01) were discarded further in the QC process. Thus, a total of 14,851,440 variants were retained for the following GWAS process (Figure 1B).
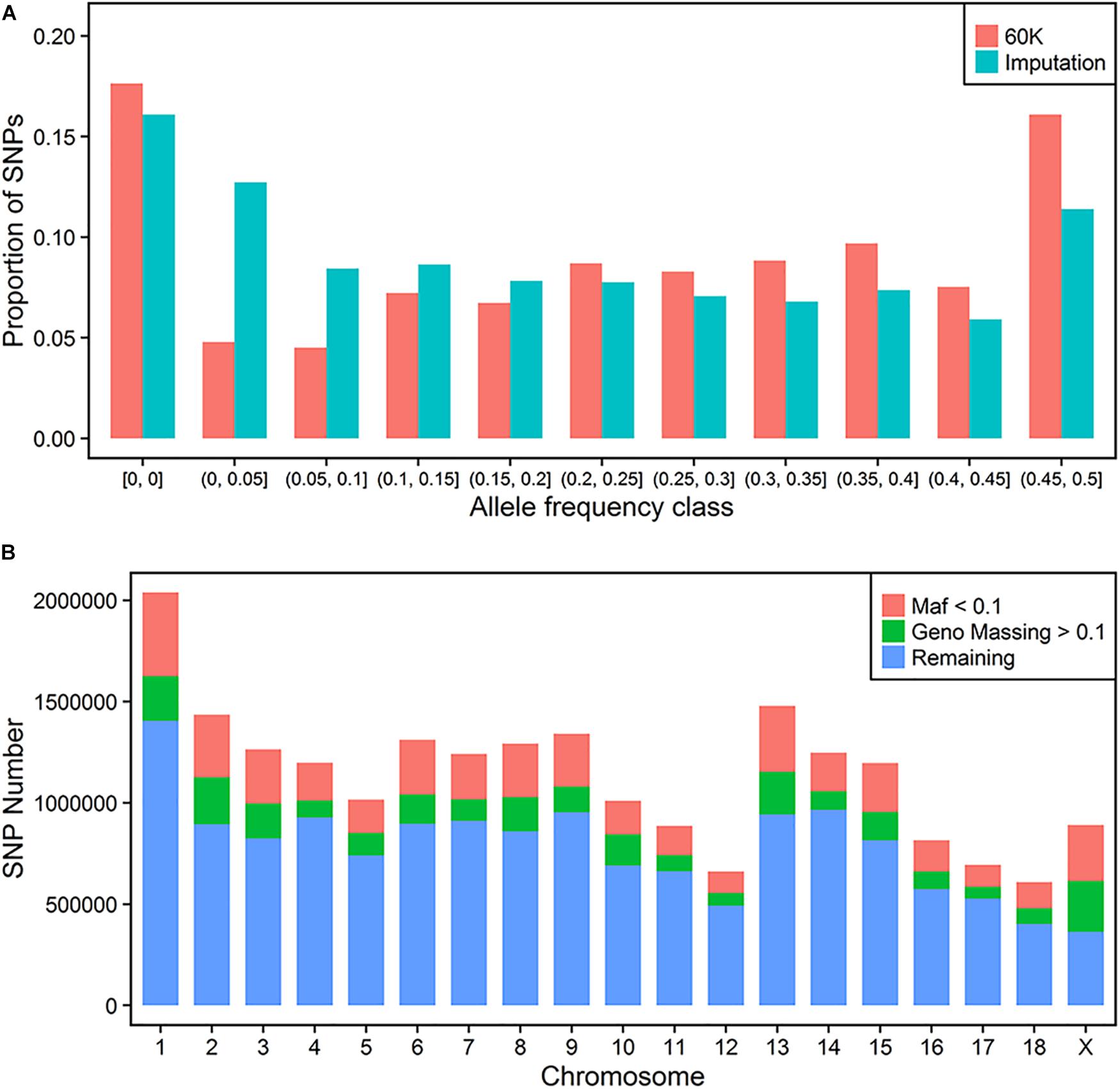
FIGURE 1. Imputation of whole-genome sequence variants. Distribution of SNP frequencies of 60 K and imputed variants across MAF classes (A). Quality control for imputed variants with MAF and genotype missing rate (B).
To investigate the imputation accuracy across MAF in detail, we studied the genotypic concordance rate and R2 according to MAF and chromosome (Figures 2A,B). Genotypic concordance rate and R2 between the best-guess imputed and true variants in the cross-validation reached an average of 89 and 80%, respectively. With the increasing of MAF, R2 increased from 23 to 88%, while genotypic concordance rate decreased from 97% to approximately 86% (Figure 2A). The whole-genome genotypic concordance rate fluctuated between 85 and 92%, with R2 fluctuating between 73 and 85%, and varying across chromosomes (Figure 2B). R2 between variants in five sequenced individuals and best-guess imputed variants, fluctuated between 79 (s35) and 87% (s1143) across individuals (Figure 2C).
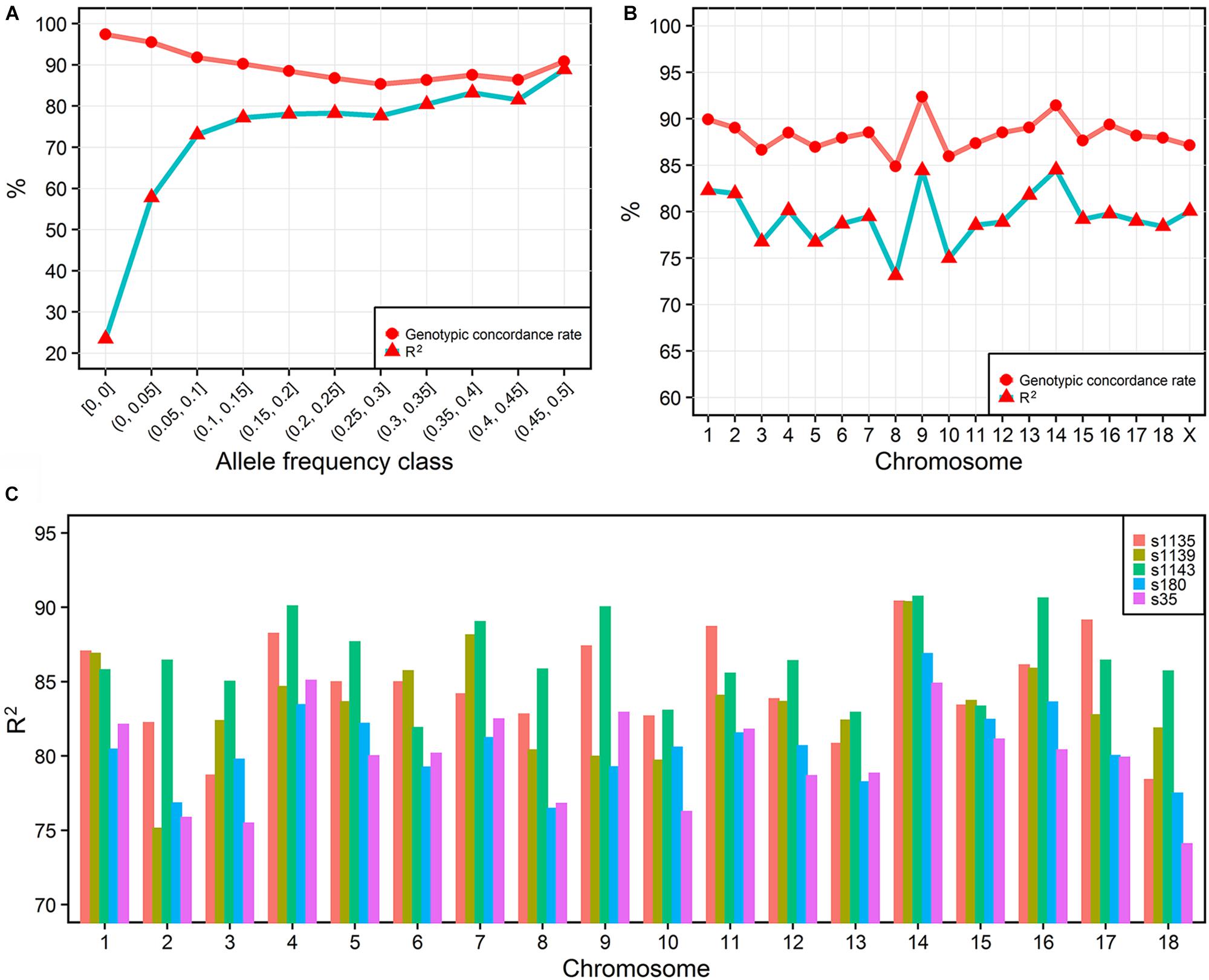
FIGURE 2. Imputation accuracy. The imputation accuracy from IMPUTE2 internal cross-validation across MAFs (A) and chromosomes (B). R2 is the squared correlation. The squared correlation between five sequenced and imputed individuals (C).
Single-Trait GWAS
Totally, we identified 197 QTLs for all hematological traits at three different stages. Among them, we obtained 75, 86, and 36 QTLs for hematological traits at day 18, day 46, and at day 240, respectively. With regard to the three classes of hematological traits, we identified 108, 76, and 13 QTLs for erythroid parameters, leukocyte parameters, and platelet parameters, respectively. To detect the number of independent QTLs identified in this study, we defined a minimum distance of 5 Mb between two top SNPs to differentiate two independent QTLs. Thus, we identified 95 unique QTLs, 87 of which are novel compared to the 60 K GWAS in the same population. Furthermore, we identified a series of significant variants located on some annotated protein-coding genes.
Loci for Erythrocyte Traits
One hundred and eight QTLs significantly associated with the seven erythrocyte traits in different stages were identified. The Manhattan plots for these association results and detailed information across the three different stages are presented in Figure 3, Table 1, Supplementary Figures S3, S4, and Supplementary Table S2. Among the 108 QTLs, 22, 68, and 18 QTLs were identified for days 18, 46, and 240, respectively. There is no significant variant association with RDW at day 18, and HGB and RBC at day 46 (P > 5E-08). Variants affecting HCT, HGB, and RBC at day 18 are located on SSC9 and near within 1 Mb, and are treated as identical QTLs (P = 1.18E-14, P = 1.01E-13, and P = 2.27E-11). The nearest gene in this region is the serine/threonine kinase 33 (STK33) gene. We also identified a QTL at 304,622,788 bp located on SSC1 that possibly affected HCT and HGB at day 18. The most significant SNP (P = 1.51E-09) was located on the far upstream element-binding protein 3 (FUBP3) gene. Several significant loci were detected for erythrocyte traits at day 46, especially for HCT, MCHC, and MCV (Supplementary Figure S3). Regarding the traits at day 240, one and two novel QTLs were detected for MCH and MCHC, respectively. And some QTLs located on SSCX were identified for MCV and RDW. The novel locus associated with MCH was located at ∼77 Mb on SSC11 including 23 significant SNPs (P < 5E-08). The most significant SNPs (P = 1.97E-09) were exactly located within the fibroblast growth factor 14 (FGF14) gene. The two novel QTLs associated with MCHC were located on SSC6 and SSC17, respectively. More information about significant SNPs are listed in Table 1 and Supplementary Table S2.
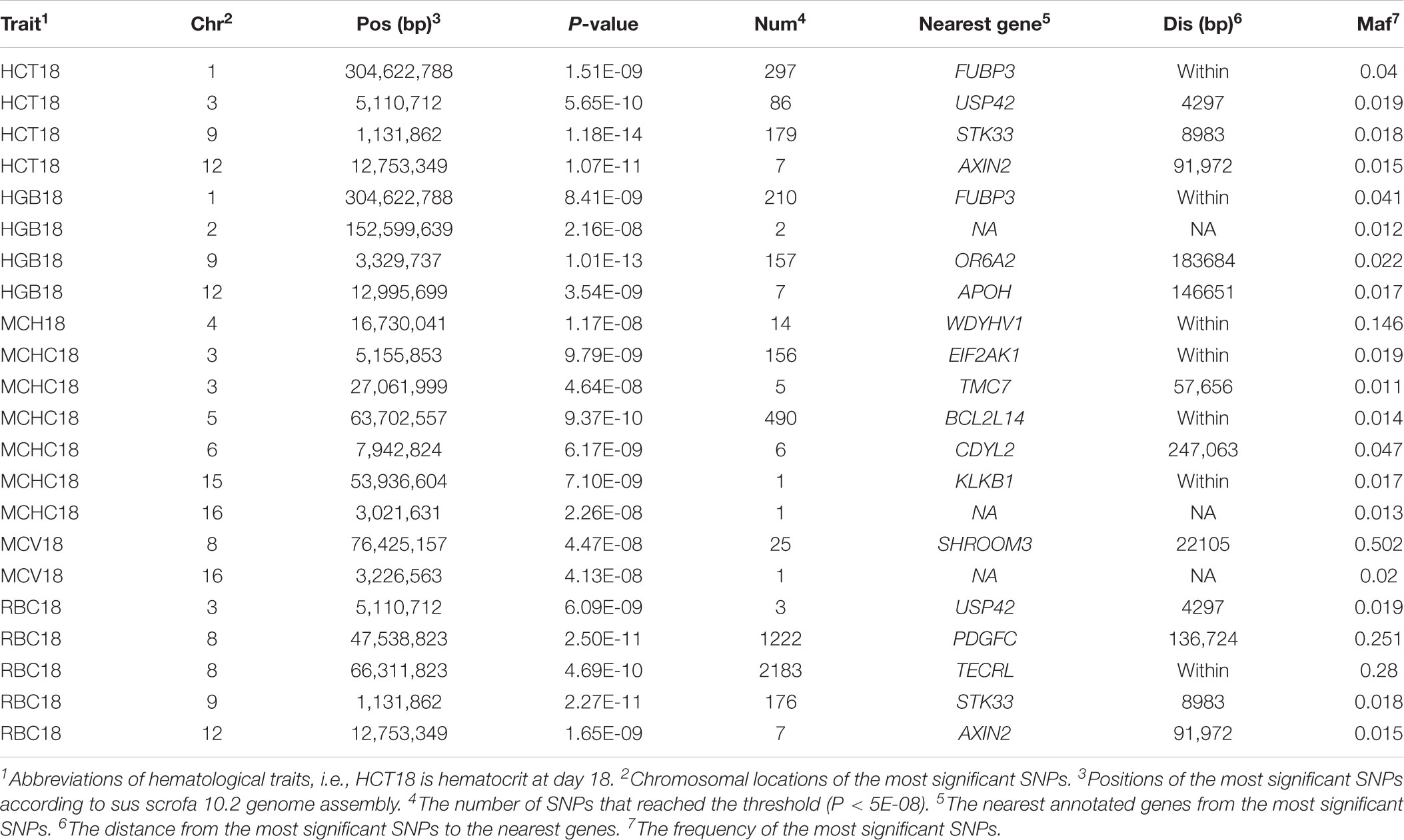
TABLE 1. Genome-wide significant loci associated with erythroid traits at day 18 obtained by single-trait GWAS.
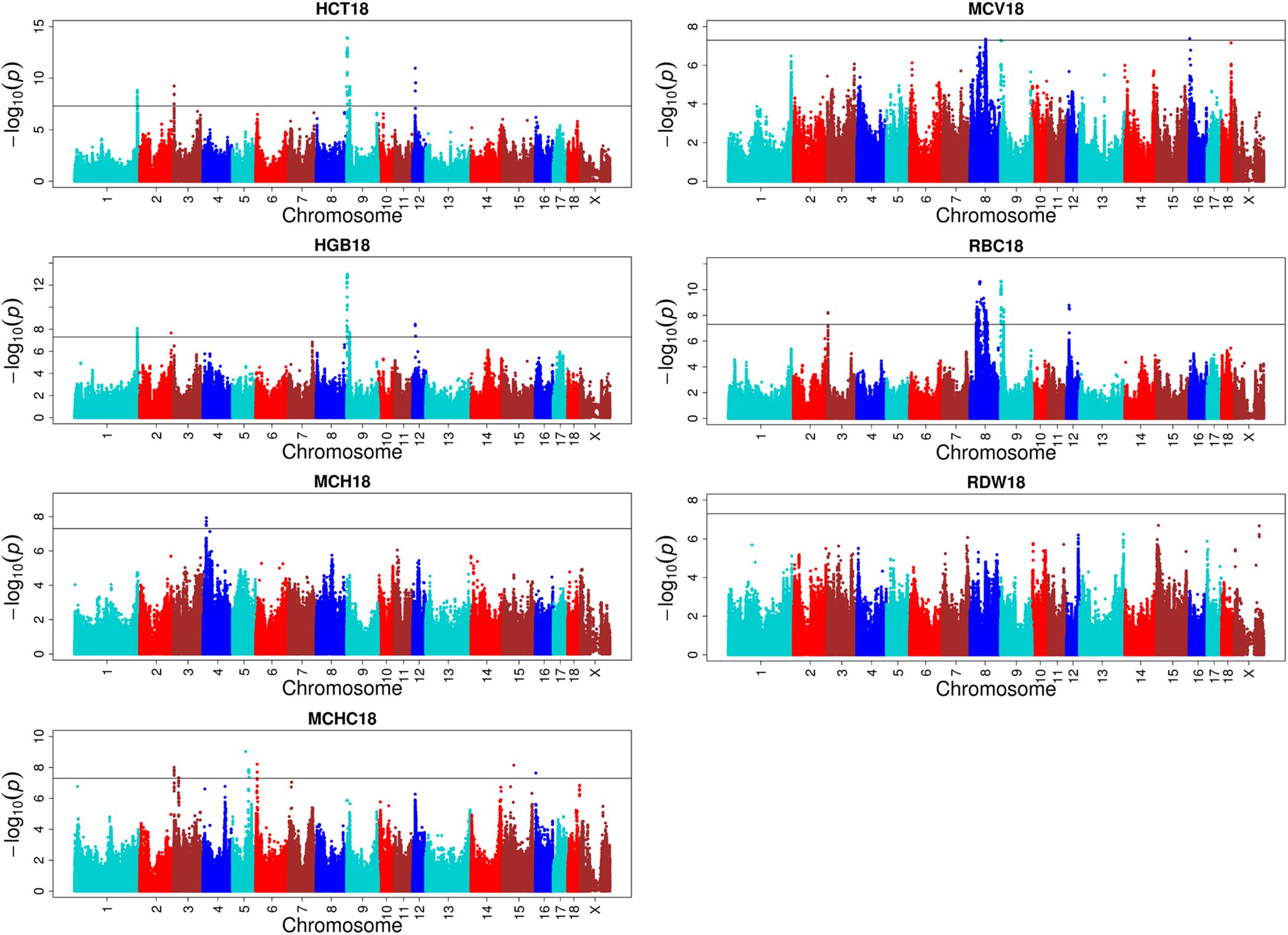
FIGURE 3. Single-marker GWAS results for seven erythrocyte traits at day 18. The y-axis and the x-axis represent the negative log10 P-value of the SNPs and the genomic positions separated by chromosomes, respectively, and the black solid lines indicate the significance threshold (negative log10 5E-08).
Loci for Leukocyte Traits
Seventy six loci significantly associated with the seven leukocyte traits at different stages were detected. The related Manhattan plots and detailed information across three different stages are presented in Supplementary Figures S5–S7 and Supplementary Table S2. Among the 76 loci, 47, 11, and 18 QTLs were identified to be responsible for the seven leukocyte traits at the three different stages, respectively. At day 18, many QTLs were identified for MON, MONR, and WBC. One locus near the FGF14 gene located on SSC11 was simultaneously associated with GRAN, LYM, and WBC at day 18. There was only one possible QTL located on SSC8 that affected GRAR at day 18. This region held 35 significant SNPs, where the most significant SNP was at the position of 10,485,550 bp and located in the coiled-coil and c2 domain containing 2A (CC2D2A) gene. At day 46, the majority of QTLs were identified associated with MONR. At day 240, some identical QTLs were uncovered for MON and MONR. There is no significant association for lymphocyte count (LYM) and WBC (P > 5E-08). The detailed descriptions of significant SNPs across the three different stages of QTLs are presented in Supplementary Table S2.
Loci for Platelet Traits
Unlike the plenty of loci identified for erythrocyte and leukocyte parameters, only a few loci were identified for platelet traits (Supplementary Figure S8 and Supplementary Table S2). Six and seven loci were associated with platelet traits at days 18 and 46, respectively. There was no significant association with platelet traits at day 240. Although some SNPs were significantly associated with MPV at day 18, no annotated gene was found around a distance of 700 Kb near the most significant SNP (P = 4.44E-10). For PDW at day 18, a region of 1.56 Mb on SSC5 was identified including 13 significant SNPs, and the most significant SNP (P = 2.2E-08) was located in the activating transcription factor 7 interacting protein (ATF7IP) gene. Fourteen significant SNPs located on SSC11 were detected for PDW at day 46. The most significant SNP (P = 1.19E-09) was located in the integrin subunit beta like 1 (ITGBL1) gene.
Multitrait GWAS
For these four multitrait GWAS of correlated traits, we identified eight, one, four, and three novel significant loci, respectively, compared to single-trait GWAS (Figure 4, Supplementary Figure S9 and Supplementary Table S3). The multitrait GWAS (including HCT, HGB, MCH, and MCV traits) at day 18 identified two, one, two, one, and two loci located on SSC3, SSC5, SSC7, SSC9, and SSC10, respectively (Supplementary Table S3). The most significant SNP (P = 2.33E-08) was located on SSC3 at 115 Mb near to an annotated gene called the lysocardiolipin acyltransferase 1 (LCLAT1) gene. The other significant SNPs are listed in Supplementary Table S3.
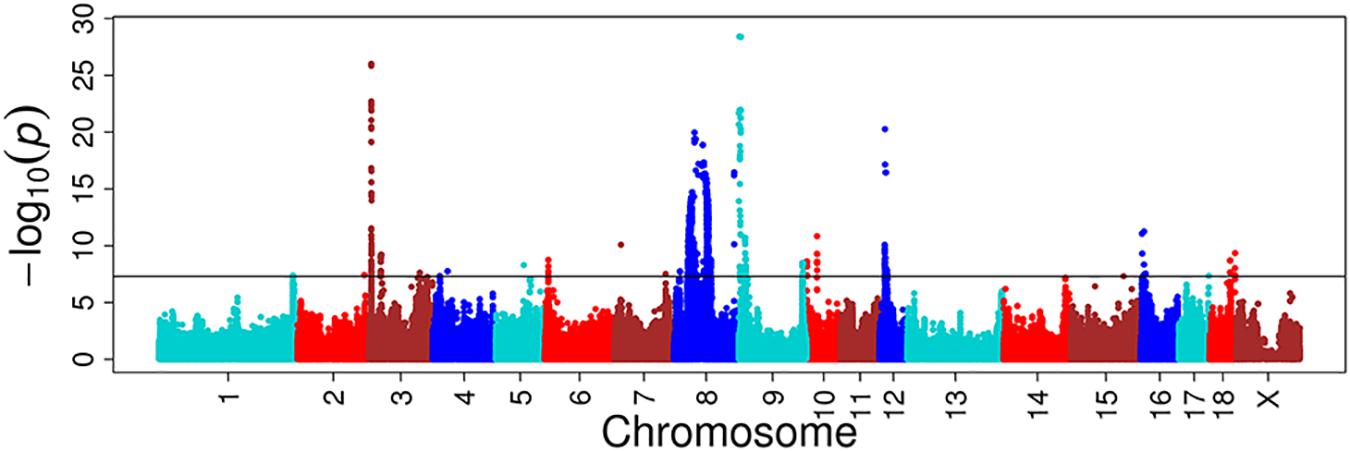
FIGURE 4. Multitrait GWAS results for correlated hematological traits at day 18. The multiple traits include HCT, HGB, MCH, and MCV at day 18. The y-axis and the x-axis represent the negative log10 P-value of the SNPs and the genomic positions separated by chromosomes, respectively, and the black solid lines indicate the significance threshold (negative log10 5E-08).
Discussion
Genotype Imputation
In the current study, we obtained 14,851,440 variants with a high imputation accuracy. Genotypic concordance rate and R2 between the best-guess imputed and true variants in the cross-validation reached an average of 89 and 80%. In the case of the pig, most studies focused on the imputation from low-density genotypes to 60 K variants with correlations ranging from 0.938 to 0.992 for imputation from 3 to 60 K (Cleveland and Hickey, 2013), and with R2 ranging from 0.88 to 0.95 for imputation from 9 to 60 K (Badke et al., 2014). However, few studies reported the imputation accuracy from 60 K to a whole-genome sequence in the pig. Genotypic concordance rate was reported to be 92.1% from 80 to 650 K and 85.6% from 650K to whole-genome sequence variants using a stepwise imputation strategy in 1,363 Duroc pigs (Zhang et al., 2018), and was lower compared to the present study (89%). Another study showed an average correlation of 80% between the true and imputed genotypes ranging from 0.74 to 0.86 with imputing 60K to whole-genome sequence variants in Sutai pigs (Yan et al., 2017). Sometimes, comparing the imputation accuracy among different studies is difficult since it is defined in different ways. Genotypic concordance rate is highly sensitive to MAF and is not appropriate for comparing genotypes with different MAF. To directly compare other studies, we adopted both genotypic concordance rate and R2 to estimate imputation accuracy.
Many factors affect the accuracy of imputation from low- or medium-density genotypes to whole-genome sequence data. As the whole-genome sequence data contains a huge percent of low MAF variants compared to chip data, imputation accuracy will be affected by those low MAF variants more in the imputation to whole-genome sequence data. In the present study, R2 decreased from 72 to 22% when the MAF decreased from 0.1 to 0. In other studies, the same trend was found (Daetwyler et al., 2014; van Binsbergen et al., 2014). The relationships between the target and reference panels is another key factor that influences imputation accuracy (van Binsbergen et al., 2014). We sequenced 19 ancestors of F2 to achieve a high imputation accuracy. LD and reference size will also affect the imputation accuracy according to many studies (van Binsbergen et al., 2014). Usually, a smaller distance between markers in the target panel and in reference would lead to a higher imputation accuracy. Thus, imputation accuracy can be improved by considering different factors, which will lead to a high accuracy in GWAS and genomic selection.
Whole-Genome Association vs. 60K GWAS and Potential Novel Candidate Genes
With the development of advanced statistical models and the decrease of genotyping cost, GWAS has become a considerably effective and popular method to search for the association between the genetic variants and complex traits across the whole genome (Hirschhorn and Daly, 2005). Previously, by performing a single-marker GWAS and a LONG-GWAS in the same population of 18 hematological traits at three different stages using PorcineSNP60 BeadChip (Zhang et al., 2013), we identified 38 genome-wide significant regions distributed on SSC1, 4, 5, 7, 8, 10, 11, 12, 13, 17, and 18, which only confirmed 10 QTLs (Supplementary Figure S10 and Supplementary Table S4) from the linkage mapping result obtained using 194 microsatellites for traits of seven erythroid parameters at three stages, and five leukocyte and four platelet parameters at day 240 within the same population (Zou et al., 2008; Yang et al., 2009). Because of the relatively shorter LD (10.5 kb) decay distance (threshold: r2 = 0.3) in most native pigs than in western pigs (Ai et al., 2013), and the density of the current 60 K BeadChip cannot effectively cover the LD block (∼80 kb), if there is no marker in tight LD with casual variants in a such broader region, no significant association signal would be detected as LD decreased rapidly. Thus, some QTLs were missing when we conducted the 60 K GWAS. In comparison, the whole-genome sequence including almost genetics variants across genome as well as causative mutation for traits of interesting association between causative variants and phenotypes can meet the shortcoming of low-density BeadChip based association.
After performing the whole-genome association study, we identified 108, 76, and 13 QTLs for erythroid, leukocyte, and platelet traits, respectively. Among these QTLs, 95 QTLs are unique and 87 QTLs are novel when compared to the 60K GWAS result in the same population (Supplementary Table S4), of which 39, 41, and 7 are novel QTLs for erythroid, leukocyte, and platelet traits, respectively. These novel QTLs distributed on almost all chromosomes except chromosome 18 across the whole genome. Because of the numerous novel results, we herein paid more attention to the gene which is function-related to each trait or the gene which the most significant SNP is rightly located on for each trait. We identified one locus that affected LYM and WBC at day 18 with the most significant position of 50, 736, and 762 bp (PLY M = 2.03E-10 and PWBC = 7.10E-10), which is near the TOX2 gene. TOX2 is a transcription factor that shares a highly conserved high-mobility group DNA-binding domain with the other TOX family members. A recent study shows that TOX2 can regulate the development of natural killer cells with the control in T-BET expression (Vong et al., 2014). Another study illustrates that TOX is a requirement for the differentiation of common lymphoid progenitors into innate lymphoid cells in vivo (Seehus et al., 2015). These evidences imply that TOX2 gene may be a candidate gene for WBC and LYM at day 18. Furthermore, except the QTL on SSC8, we identified another novel QTL located on SSC11 associated with MCH at day 240. This region including 23 significant SNPs, with the top SNP located at the position of 77,754,820 bp (MAF = 0.212, P = 1.97E-09) and posited the fibroblast growth factor 14 (FGF14) gene. Fibroblast growth factors (FGFs) family are involved in a variety of biological processes. Among the Fgf family, FGF14 is one member of the intracellular subfamily, also known as iFGFs (Itoh and Ornitz, 2008). The iFGFs function as intracellular proteins in an FGFR-independent way (Wang et al., 2000; Goldfarb, 2005). FGF14 was identified to be associated with neuronal signaling in mice (Wang et al., 2002). Recent studies on the FGF14 gene focused on neurology in humans and in mice (Amado et al., 2017; Di Re et al., 2017; Hsu et al., 2017). Interestingly, this gene was also identified to be a candidate for teat number in Duroc pigs (Tan et al., 2017), implying the pleiotropy of FGF14. These new candidate genes could help to complete the gene regulatory network of hematological traits.
We almost confirmed all the QTLs identified in our prior 60K GWAS based on a single-marker, except the QTLs located on SSC5, SSC7, and SSC13 for MCH at day 18, WBC at day 240, and PDW at day 46. The undetected signal is probably because of the higher threshold in the whole-genome GWAS. In particular, as to the QTLs located on SSC7 or SSC8 for HCT, MCH, and MCV at day 240, respectively, we did not discuss these QTLs in detail here, because the conditional GWAS was performed and results have been elaborately discussed by Zhang et al. (2013) in the same population using SNP60 BeadChip data. Although we identified many novel QTLs, most of them are time-specific except for the region on SSC8 harboring the kit proto-oncogene receptor tyrosine kinase (KIT) gene, implying that the same hematological trait at different stages may be affected by different genes.
Result Consistency With Other Studies
To the best of our knowledge, there are only six published studies demonstrating the association between either all 18 hematological traits or part of these traits and genomic loci using the Porcine SNP60 BeadChip based on GWAS in pigs (Luo et al., 2012; Wang et al., 2013; Zhang et al., 2013, 2014; Jung et al., 2014; Ponsuksili et al., 2016). The KIT gene was proposed as a candidate gene for hematological traits by some different studies. We found that the candidate gene of KIT should be responsible for the MCV trait (days 18, 46, and 240), and MCH and RBC traits (day 240) in our previous study (Zhang et al., 2013). The same result was obtained in Landrace × Korean native pigs F2 population at the age of 140 days (Jung et al., 2014). However, Luo et al. only identified the candidate gene of KIT associated with two erythroid traits including MCH and MCV at day 240 in White × Minzhu F2 population (Luo et al., 2012). In this study, we found that this candidate gene can also significantly affect the PDW trait at day 18, although the SNPs in that region did not reach the significant threshold (P = 1.35E-07). The relatively low significance may be because of the small samples for this trait, i.e., only 250 samples that held phenotypes were used for GWAS. If we can increase number of the valid samples, the association between PDW and the genotypes may emerge. Beside the KIT gene claimed as the candidate gene for hematological traits in majority studies at different stages (i.e., days 140 and 240), some other candidate genes were identified, including neuroligin 4, x-linked (NLGN4X) and high-mobility group box family member 2 (TOX2) gene, which was identified in the Commercial Landrace population (Ponsuksili et al., 2016). In the GWAS study in the Commercial Landrace population, Ponsuksili et al. (2016) identified a candidate gene named NLGN4X associated with MCH, MCV, and RDW traits at an average day 170 using the Bayesian GWAS approach. The mutations of NLGN4X are generally linked with autism and Asperger syndrome in different human populations (Jamain et al., 2003; Volaki et al., 2013; Landini et al., 2016). We found that this NLGN4X gene is not only associated with MCV traits at day 46 but also associated with HCT, MCHC, and PCT at day 46. In summary, although we have confirmed the associations of different QTLs or candidate genes with these hematological traits reported in other studies, the different genetic backgrounds and populations and the different stages when the traits are measured would lead to different association results.
Multitrait GWAS
A multivariate linear mixed model has been recently considered as a remarkably efficient method in GWAS, not only because of its stronger ability in correcting sample relatedness (Meyer, 1991; Amos, 1994; Korte et al., 2012) and population stratification (Yu et al., 2006), but also because of the advantage over standard univariate analysis in terms of increasing statistical power (Korte et al., 2012). Multivariate analysis can increase detection power by accumulating common genetic factors together for multiple correlated traits and increasing the sample size (Stephens, 2013). We thus used multiple correlated traits implemented in a multitrait linear mixed model to discover more novel QTLs. The genetic correlation and the phenotype correlation are highly similar. The statistical power of multitrait GWAS on HCT, HGB, MCH, and MCV at day 18 were increased in most chromosomes. The novel loci with the most significant SNP at the position of 115,277,228 bp (P = 2.33E-08) located on SSC3 near the lysocardiolipin acyltransferase 1 (LCLAT1) gene were found to be responsible for MCH and MCV at day 18. A study reported that LCLAT played an important role in the development of hematopoietic and endothelial lineages, probably acting at the level of the hemangioblast in mice (Wang et al., 2007).
Conclusion
Whole-genome association analyses based on imputed sequence variants increase the possibility to identify more QTLs and the potential causative mutation. Multitrait GWAS has been proven to be a remarkably powerful statistical method to uncover the variants that affect multiple correlated traits. This study identified more than 87 QTL novel regions that affected 18 hematological traits at three different stages by single and multitrait GWAS, based on the whole-genome imputation data. We propose that the FGF14 and LCLAT1 genes located at SSC11 and SSC3 may affect MCH at day 240 and MCH as well as MCV at stage at day 18, respectively.
Data Availability
The genotypic data of 933 F2 individuals analyzed for this study can be found in the Dryad Digital Repository (https://doi.org/10.5061/dryad.7kn7r) (Ma et al., 2013). The raw reads of the whole-genome sequence can be found from the NCBI sequence read archive (SRA) under the accession codes SRA065461 (Ai et al., 2015), SRP047260 (Moon et al., 2015), and SRP159212.
Author Contributions
LH and ZZ conceived and designed the experiments. TG, GY, ZZ, and SX performed the experiments. GY, ZZ, FZ, TH, and YL analyzed the data. SX, WXi, and WXu contributed to materials and analysis tools. GY, TG, ZZ, and LH wrote the manuscript. All authors read and approved the final manuscript.
Funding
This work was supported by the National Natural Science Foundation of China (31640046 and 31760656), and the Guangdong Sail Plan Introduction of Innovative and Entrepreneurship Research Team Program (2016YT03H062).
Conflict of Interest Statement
The authors declare that the research was conducted in the absence of any commercial or financial relationships that could be construed as a potential conflict of interest.
Acknowledgments
We are grateful to all the members who participated in this study from the State Key Laboratory for Pig Genetic Improvement and Production Technology.
Supplementary Material
The Supplementary Material for this article can be found online at: https://www.frontiersin.org/articles/10.3389/fgene.2018.00401/full#supplementary-material
Footnotes
- ^http://broadinstitute.github.io/picard/
- ^http://www.well.ox.ac.uk/cfreeman/software/gwas/gtool.html
References
Ai, H., Fang, X., Yang, B., Huang, Z., Chen, H., Mao, L., et al. (2015). Adaptation and possible ancient interspecies introgression in pigs identified by whole-genome sequencing. Nat. Genet. 47, 217–225. doi: 10.1038/ng.3199
Ai, H., Huang, L., and Ren, J. (2013). Genetic diversity, linkage disequilibrium and selection signatures in Chinese and Western pigs revealed by genome-wide SNP markers. PLoS One 8:e56001. doi: 10.1371/journal.pone.0056001
Altschul, S. F., Gish, W., Miller, W., Myers, E. W., and Lipman, D. J. (1990). Basic local alignment search tool. J. Mol. Biol. 215, 403–410. doi: 10.1016/S0022-2836(05)80360-2
Amado, A., Blanco, M. O., and Reparaz-Andrade, A. (2017). Spinocerebellar ataxia 27: clinical phenotype of twin sisters with FGF14 deletion. Neuropediatrics 48:131. doi: 10.1055/s-0037-1598110
Amos, C. I. (1994). Robust variance-components approach for assessing genetic linkage in pedigrees. Am. J. Hum. Genet. 54, 535–543.
Auer, P. L., Teumer, A., Schick, U., O’Shaughnessy, A., Lo, K. S., Chami, N., et al. (2014). Rare and low-frequency coding variants in CXCR2 and other genes are associated with hematological traits. Nat. Genet. 46, 629–634. doi: 10.1038/ng.2962
Badke, Y. M., Bates, R. O., Ernst, C. W., Fix, J., and Steibel, J. P. (2014). Accuracy of estimation of genomic breeding values in pigs using low-density genotypes and imputation. G3 4, 623–631. doi: 10.1534/g3.114.010504
Browning, S. R., and Browning, B. L. (2011). Haplotype phasing: existing methods and new developments. Nat. Rev. Genet. 12, 703–714. doi: 10.1038/nrg3054
Chang, C. C., Chow, C. C., Tellier, L. C., Vattikuti, S., Purcell, S. M., and Lee, J. J. (2015). Second-generation PLINK: rising to the challenge of larger and richer datasets. Gigascience 4:7. doi: 10.1186/s13742-015-0047-8
Cleveland, M. A., and Hickey, J. M. (2013). Practical implementation of cost-effective genomic selection in commercial pig breeding using imputation. J. Anim. Sci. 91, 3583–3592. doi: 10.2527/jas.2013-6270
Daetwyler, H. D., Capitan, A., Pausch, H., Stothard, P., van Binsbergen, R., Brondum, R. F., et al. (2014). Whole-genome sequencing of 234 bulls facilitates mapping of monogenic and complex traits in cattle. Nat. Genet. 46, 858–865. doi: 10.1038/ng.3034
Danecek, P., Auton, A., Abecasis, G., Albers, C. A., Banks, E., DePristo, M. A., et al. (2011). The variant call format and VCFtools. Bioinformatics 27, 2156–2158. doi: 10.1093/bioinformatics/btr330
Delaneau, O., Howie, B., Cox, A. J., Zagury, J. F., and Marchini, J. (2013). Haplotype estimation using sequencing reads. Am. J. Hum. Genet. 93, 687–696. doi: 10.1016/j.ajhg.2013.09.002
Di Re, J., Wadsworth, P. A., and Laezza, F. (2017). Intracellular fibroblast growth factor 14: emerging risk factor for brain disorders. Front. Cell. Neurosci. 11:103. doi: 10.3389/fncel.2017.00103
Druet, T., and Georges, M. (2010). A hidden markov model combining linkage and linkage disequilibrium information for haplotype reconstruction and quantitative trait locus fine mapping. Genetics 184, 789–798. doi: 10.1534/genetics.109.108431
Evans, D. M., Frazer, I. H., and Martin, N. G. (1999). Genetic and environmental causes of variation in basal levels of blood cells. Twin Res. 2, 250–257. doi: 10.1375/136905299320565735
Garner, C., Tatu, T., Reittie, J. E., Littlewood, T., Darley, J., Cervino, S., et al. (2000). Genetic influences on F cells and other hematologic variables: a twin heritability study. Blood 95, 342–346.
Goldfarb, M. (2005). Fibroblast growth factor homologous factors: evolution, structure, and function. Cytokine Growth Factor Rev. 16, 215–220. doi: 10.1016/j.cytogfr.2005.02.002
Groenen, M. A., Archibald, A. L., Uenishi, H., Tuggle, C. K., Takeuchi, Y., Rothschild, M. F., et al. (2012). Analyses of pig genomes provide insight into porcine demography and evolution. Nature 491, 393–398. doi: 10.1038/nature11622
Guo, Y., Mao, H., Ren, J., Yan, X., Duan, Y., Yang, G., et al. (2009). A linkage map of the porcine genome from a large-scale White Duroc x Erhualian resource population and evaluation of factors affecting recombination rates. Anim. Genet. 40, 47–52. doi: 10.1111/j.1365-2052.2008.01802.x
Hirschhorn, J. N., and Daly, M. J. (2005). Genome-wide association studies for common diseases and complex traits. Nat. Rev. Genet. 6, 95–108. doi: 10.1038/nrg1521
Howie, B. N., Donnelly, P., and Marchini, J. (2009). A flexible and accurate genotype imputation method for the next generation of genome-wide association studies. PLoS Genet. 5:e1000529. doi: 10.1371/journal.pgen.1000529
Hsu, W. J., Wildburger, N. C., Haidacher, S. J., Nenov, M. N., Folorunso, O., Singh, A. K., et al. (2017). PPARgamma agonists rescue increased phosphorylation of FGF14 at S226 in the Tg2576 mouse model of Alzheimer’s disease. Exp. Neurol. 295, 1–17. doi: 10.1016/j.expneurol.2017.05.005
Hu, Z. L., Fritz, E. R., and Reecy, J. M. (2007). AnimalQTLdb: a livestock QTL database tool set for positional QTL information mining and beyond. Nucleic Acids Res. 35, D604–D609. doi: 10.1093/nar/gkl946
Itoh, N., and Ornitz, D. M. (2008). Functional evolutionary history of the mouse Fgf gene family. Dev. Dyn. 237, 18–27. doi: 10.1002/dvdy.21388
Jamain, S., Quach, H., Betancur, C., Rastam, M., Colineaux, C., Gillberg, I. C., et al. (2003). Mutations of the X-linked genes encoding neuroligins NLGN3 and NLGN4 are associated with autism. Nat. Genet. 34, 27–29. doi: 10.1038/ng1136
Johnson, R. C., Nelson, G. W., Troyer, J. L., Lautenberger, J. A., Kessing, B. D., Winkler, C. A., et al. (2010). Accounting for multiple comparisons in a genome-wide association study (GWAS). BMC Genomics 11:724. doi: 10.1186/1471-2164-11-724
Jung, E. J., Park, H. B., Lee, J. B., Yoo, C. K., Kim, B. M., Kim, H. I., et al. (2014). Genome-wide association study identifies quantitative trait loci affecting hematological traits in an F2 intercross between Landrace and Korean native pigs. Anim. Genet. 45, 534–541. doi: 10.1111/age.12175
Korte, A., Vilhjalmsson, B. J., Segura, V., Platt, A., Long, Q., and Nordborg, M. (2012). A mixed-model approach for genome-wide association studies of correlated traits in structured populations. Nat. Genet. 44, 1066–1071. doi: 10.1038/ng.2376
Landini, M., Merelli, I., Raggi, M. E., Galluccio, N., Ciceri, F., Bonfanti, A., et al. (2016). Association analysis of noncoding variants in neuroligins 3 and 4X genes with autism spectrum disorder in an italian cohort. Int. J. Mol. Sci. 17:E1765. doi: 10.3390/ijms17101765
Li, H., and Durbin, R. (2009). Fast and accurate short read alignment with Burrows-Wheeler transform. Bioinformatics 25, 1754–1760. doi: 10.1093/bioinformatics/btp324
Luo, W., Chen, S., Cheng, D., Wang, L., Li, Y., Ma, X., et al. (2012). Genome-wide association study of porcine hematological parameters in a large White x Minzhu F2 resource population. Int. J. Biol. Sci. 8, 870–881. doi: 10.7150/ijbs.4027
Ma, J., Yang, J., Zhou, L., Zhang, Z., Ma, H., Xie, X., et al. (2013). Genome-wide association study of meat quality traits in a White Duroc x Erhualian F2 intercross and Chinese Sutai pigs. PLoS One 8:e64047. doi: 10.1371/journal.pone.0064047
Manolio, T. A., Collins, F. S., Cox, N. J., Goldstein, D. B., Hindorff, L. A., Hunter, D. J., et al. (2009). Finding the missing heritability of complex diseases. Nature 461, 747–753. doi: 10.1038/nature08494
McKenna, A., Hanna, M., Banks, E., Sivachenko, A., Cibulskis, K., Kernytsky, A., et al. (2010). The genome analysis toolkit: a MapReduce framework for analyzing next-generation DNA sequencing data. Genome Res. 20, 1297–1303. doi: 10.1101/gr.107524.110
Meyer, K. (1991). Estimating variances and covariances for multivariate animal models by restricted maximum likelihood. Genet. Sel. Evol. 23, 1–17. doi: 10.1186/1297-9686-23-1-67
Moon, S., Kim, T. H., Lee, K. T., Kwak, W., Lee, T., Lee, S. W., et al. (2015). A genome-wide scan for signatures of directional selection in domesticated pigs. BMC Genomics 16:130. doi: 10.1186/s12864-015-1330-x
Muller, M., and Brem, G. (1991). Disease resistance in farm animals. Experientia 47, 923–934. doi: 10.1007/BF01929883
Oddgeirsson, O., Simpson, S. P., Morgan, A. L., Ross, D. S., and Spooner, R. L. (1988). Relationship between the bovine major histocompatibility complex (BoLA), erythrocyte markers and susceptibility to mastitis in Icelandic cattle. Anim. Genet. 19, 11–16. doi: 10.1111/j.1365-2052.1988.tb00783.x
Okada, Y., and Kamatani, Y. (2012). Common genetic factors for hematological traits in humans. J. Hum. Genet. 57, 161–169. doi: 10.1038/jhg.2012.2
Pe’er, I., Yelensky, R., Altshuler, D., and Daly, M. J. (2008). Estimation of the multiple testing burden for genomewide association studies of nearly all common variants. Genet. Epidemiol. 32, 381–385. doi: 10.1002/gepi.20303
Polfus, L. M., Khajuria, R. K., Schick, U. M., Pankratz, N., Pazoki, R., Brody, J. A., et al. (2016). Whole-exome sequencing identifies loci associated with blood cell traits and reveals a role for alternative GFI1B splice variants in human hematopoiesis. Am. J. Hum. Genet. 99, 481–488. doi: 10.1016/j.ajhg.2016.06.016
Ponsuksili, S., Reyer, H., Trakooljul, N., Murani, E., and Wimmers, K. (2016). Single- and bayesian multi-marker genome-wide association for haematological parameters in pigs. PLoS One 11:e0159212. doi: 10.1371/journal.pone.0159212
Seehus, C. R., Aliahmad, P., de la Torre, B., Iliev, I. D., Spurka, L., Funari, V. A., et al. (2015). The development of innate lymphoid cells requires TOX-dependent generation of a common innate lymphoid cell progenitor. Nat. Immunol. 16, 599–608. doi: 10.1038/ni.3168
Stephens, M. (2013). A unified framework for association analysis with multiple related phenotypes. PLoS One 8:e65245. doi: 10.1371/journal.pone.0065245
Swindle, M. M., Makin, A., Herron, A. J., Clubb, F. J. Jr., and Frazier, K. S. (2012). Swine as models in biomedical research and toxicology testing. Vet. Pathol. 49, 344–356. doi: 10.1177/0300985811402846
Tan, C., Wu, Z., Ren, J., Huang, Z., Liu, D., He, X., et al. (2017). Genome-wide association study and accuracy of genomic prediction for teat number in Duroc pigs using genotyping-by-sequencing. Genet. Sel. Evol. 49:35. doi: 10.1186/s12711-017-0311-8
Ulirsch, J. C., Nandakumar, S. K., Wang, L., Giani, F. C., Zhang, X., Rogov, P., et al. (2016). Systematic functional dissection of common genetic variation affecting red blood cell traits. Cell 165, 1530–1545. doi: 10.1016/j.cell.2016.04.048
van Binsbergen, R., Bink, M. C., Calus, M. P., van Eeuwijk, F. A., Hayes, B. J., Hulsegge, I., et al. (2014). Accuracy of imputation to whole-genome sequence data in Holstein Friesian cattle. Genet. Sel. Evol. 46:41. doi: 10.1186/1297-9686-46-41
van der Harst, P., Zhang, W., Mateo Leach, I., Rendon, A., Verweij, N., Sehmi, J., et al. (2012). Seventy-five genetic loci influencing the human red blood cell. Nature 492, 369–375. doi: 10.1038/nature11677
Volaki, K., Pampanos, A., Kitsiou-Tzeli, S., Vrettou, C., Oikonomakis, V., Sofocleous, C., et al. (2013). Mutation screening in the Greek population and evaluation of NLGN3 and NLGN4X genes causal factors for autism. Psychiatr. Genet. 23, 198–203. doi: 10.1097/YPG.0b013e3283643644
Vong, Q. P., Leung, W. H., Houston, J., Li, Y., Rooney, B., Holladay, M., et al. (2014). TOX2 regulates human natural killer cell development by controlling T-BET expression. Blood 124, 3905–3913. doi: 10.1182/blood-2014-06-582965
Wang, C., Faloon, P. W., Tan, Z., Lv, Y., Zhang, P., Ge, Y., et al. (2007). Mouse lysocardiolipin acyltransferase controls the development of hematopoietic and endothelial lineages during in vitro embryonic stem-cell differentiation. Blood 110, 3601–3609. doi: 10.1182/blood-2007-04-086827
Wang, J. Y., Luo, Y. R., Fu, W. X., Lu, X., Zhou, J. P., Ding, X. D., et al. (2013). Genome-wide association studies for hematological traits in swine. Anim. Genet. 44, 34–43. doi: 10.1111/j.1365-2052.2012.02366.x
Wang, Q., Bardgett, M. E., Wong, M., Wozniak, D. F., Lou, J., McNeil, B. D., et al. (2002). Ataxia and paroxysmal dyskinesia in mice lacking axonally transported FGF14. Neuron 35, 25–38. doi: 10.1016/S0896-6273(02)00744-4
Wang, Q., McEwen, D. G., and Ornitz, D. M. (2000). Subcellular and developmental expression of alternatively spliced forms of fibroblast growth factor 14. Mech. Dev. 90, 283–287. doi: 10.1016/S0925-4773(99)00241-5
Williams, A. L., Patterson, N., Glessner, J., Hakonarson, H., and Reich, D. (2012). Phasing of many thousands of genotyped samples. Am. J. Hum. Genet. 91, 238–251. doi: 10.1016/j.ajhg.2012.06.013
Yan, G., Qiao, R., Zhang, F., Xin, W., Xiao, S., Huang, T., et al. (2017). Imputation-based whole-genome sequence association study rediscovered the missing QTL for lumbar number in Sutai Pigs. Sci. Rep. 7:615. doi: 10.1038/s41598-017-00729-0
Yang, J., Benyamin, B., McEvoy, B. P., Gordon, S., Henders, A. K., Nyholt, D. R., et al. (2010). Common SNPs explain a large proportion of the heritability for human height. Nat. Genet. 42, 565–569. doi: 10.1038/ng.608
Yang, S., Ren, J., Yan, X., Huang, X., Zou, Z., Zhang, Z., et al. (2009). Quantitative trait loci for porcine white blood cells and platelet-related traits in a white Duroc x Erhualian F resource population. Anim. Genet. 40, 273–278. doi: 10.1111/j.1365-2052.2008.01830.x
Yu, J., Pressoir, G., Briggs, W. H., Vroh Bi, I., Yamasaki, M., Doebley, J. F., et al. (2006). A unified mixed-model method for association mapping that accounts for multiple levels of relatedness. Nat. Genet. 38, 203–208. doi: 10.1038/ng1702
Zhang, C., Kemp, R. A., Stothard, P., Wang, Z., Boddicker, N., Krivushin, K., et al. (2018). Genomic evaluation of feed efficiency component traits in Duroc pigs using 80K, 650K and whole-genome sequence variants. Genet. Sel. Evol. 50:14. doi: 10.1186/s12711-018-0387-9
Zhang, F., Zhang, Z., Yan, X., Chen, H., Zhang, W., Hong, Y., et al. (2014). Genome-wide association studies for hematological traits in Chinese Sutai pigs. BMC Genet. 15:41. doi: 10.1186/1471-2156-15-41
Zhang, Z., Hong, Y., Gao, J., Xiao, S., Ma, J., Zhang, W., et al. (2013). Genome-wide association study reveals constant and specific loci for hematological traits at three time stages in a White Duroc x Erhualian F2 resource population. PLoS One 8:e63665. doi: 10.1371/journal.pone.0063665
Zhou, X., Carbonetto, P., and Stephens, M. (2013). Polygenic modeling with bayesian sparse linear mixed models. PLoS Genet. 9:e1003264. doi: 10.1371/journal.pgen.1003264
Zhou, X., and Stephens, M. (2012). Genome-wide efficient mixed-model analysis for association studies. Nat. Genet. 44, 821–824. doi: 10.1038/ng.2310
Zhou, X., and Stephens, M. (2014). Efficient multivariate linear mixed model algorithms for genome-wide association studies. Nat. Methods 11, 407–409. doi: 10.1038/nmeth.2848
Keywords: GWAS, imputation, hematology, pig, whole-genome sequence
Citation: Yan G, Guo T, Xiao S, Zhang F, Xin W, Huang T, Xu W, Li Y, Zhang Z and Huang L (2018) Imputation-Based Whole-Genome Sequence Association Study Reveals Constant and Novel Loci for Hematological Traits in a Large-Scale Swine F2 Resource Population. Front. Genet. 9:401. doi: 10.3389/fgene.2018.00401
Received: 07 April 2018; Accepted: 03 September 2018;
Published: 22 October 2018.
Edited by:
Meng-Hua Li, Institute of Zoology (CAS), ChinaReviewed by:
Zhonglin Tang, Institute of Animal Sciences (CAAS), ChinaRuiHua Huang, Nanjing Agricultural University, China
Copyright © 2018 Yan, Guo, Xiao, Zhang, Xin, Huang, Xu, Li, Zhang and Huang. This is an open-access article distributed under the terms of the Creative Commons Attribution License (CC BY). The use, distribution or reproduction in other forums is permitted, provided the original author(s) and the copyright owner(s) are credited and that the original publication in this journal is cited, in accordance with accepted academic practice. No use, distribution or reproduction is permitted which does not comply with these terms.
*Correspondence: Zhiyan Zhang, bioducklily@hotmail.com Lusheng Huang, lushenghuang@hotmail.com
†These authors have contributed equally to this work