- 1Institute for Marine and Antarctic Studies, University of Tasmania, Hobart, TAS, Australia
- 2CSIRO, Oceans and Atmosphere, Hobart, TAS, Australia
- 3Centre for Marine Socioecology, University of Tasmania, Hobart, TAS, Australia
- 4Norwegian Polar Institute, Fram Centre, Tromsø, Norway
- 5The Levin Lab, Ecology and Evolutionary Biology, Princeton University, Princeton, NJ, United States
- 6British Antarctic Survey, Cambridge, United Kingdom
- 7Marine Affairs Program, Dalhousie University, Halifax, NS, Canada
- 8Centro Austral de Investigaciones Científicas (CADIC-CONICET), Ushuaia, Argentina
- 9Earth, Ocean and Atmospheric Sciences Department, Institute for the Oceans and Fisheries, The University of British Columbia, Vancouver, BC, Canada
- 10Hakai Institute, Heriot Bay, BC, Canada
- 11National Institute of Water and Atmospheric Research (NIWA), Wellington, New Zealand
- 12Instituto de Ciencias, Universidad Nacional de General Sarmiento (UNGS), Buenos Aires, Argentina
- 13Sydney Institute of Marine Science, Sydney, NSW, Australia
- 14Royal Belgian Institute for Natural Sciences, OD-Nature, Brussels, Belgium
- 15Marine Biology Lab, Université Libre de Bruxelles, Brussels, Belgium
- 16Laboratory of Biodiversity and Evolutionary Genomics, KU Leuven, Leuven, Belgium
- 17Antarctic Climate and Ecosystems, Cooperative Research Centre, Hobart, TAS, Australia
- 18Australian Antarctic Division, Department of Agriculture, Water and Environment, Kingston, TAS, Australia
Globally important services are supported by Southern Ocean ecosystems, underpinned by the structure, function, and dynamics of complex interconnected and regionally distinctive food webs. These food webs vary in response to a combination of physical and chemical processes that alter productivity, species composition and the relative abundance and dynamics of organisms. Combined with regional and seasonal variability, climate-induced changes and human activities have and are expected to continue to drive important structural and functional changes to Southern Ocean food webs. However, our current understanding of food web structure, function, status, and trends is patchy in space and time, and methods for systematically assessing and comparing community-level responses to change within and across regional and temporal scales are not well developed. Insights gained from food web modelling studies—ranging from theoretical analyses of ecosystem resilience and adaptation, to qualitative and quantitative descriptions of the system—can assist in resolving patterns of energy flow and the ecological mechanisms that drive food web structure, function, and responses to drivers (such as fishing and climate change). This understanding is required to inform robust management strategies to conserve Southern Ocean food webs and the ecosystem services they underpin in the face of change. This paper synthesises the current state of knowledge regarding Southern Ocean pelagic food webs, highlighting the distinct regional food web characteristics, including key drivers of energy flow, dominant species, and network properties that may indicate system resilience. In particular, the insights, gaps, and potential integration of existing knowledge and Southern Ocean food web models are evaluated as a basis for developing integrated food web assessments that can be used to test the efficacy of alternative management and policy options. We discuss key limitations of existing models for assessing change resulting from various drivers, summarise priorities for model development and identify that significant progress could be made to support policy by advancing the development of food web models coupled to projected biogeochemical models, such as in Earth System models.
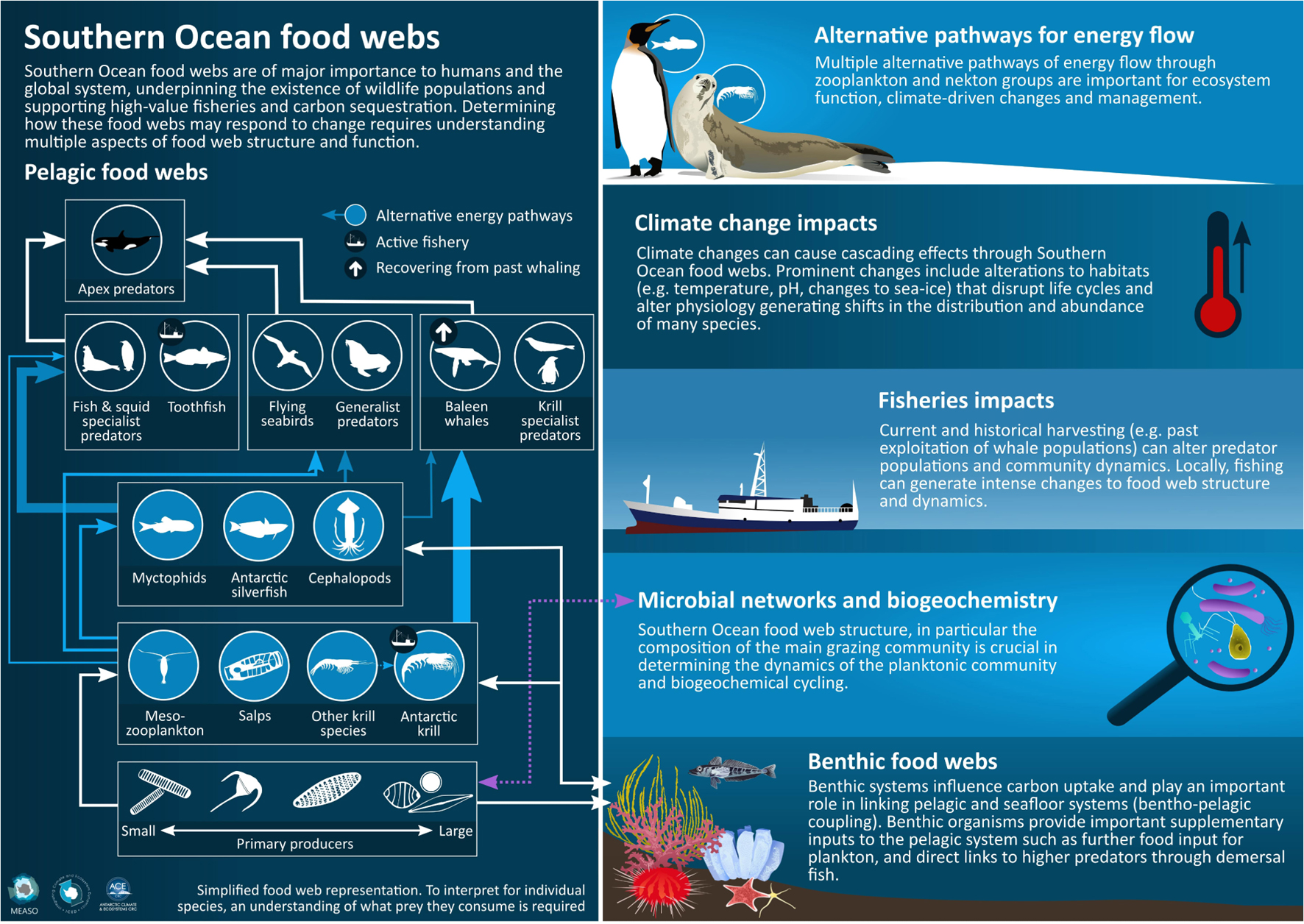
Graphical Abstract. Graphical summary of multiple aspects of Southern Ocean food web structure and function including alternative energy pathways through pelagic food webs, climate change and fisheries impacts and the importance of microbial networks and benthic systems.
Introduction
Southern Ocean marine ecosystems support globally important values and services such as the sequestration of atmospheric carbon, commercial fisheries, polar biodiversity, the existence of iconic wildlife populations, and cultural connections (Grant et al., 2013; Cavanagh et al., 2021; Murphy et al., 2021; Roberts et al., 2021). These unique ecosystems and the services they support are underpinned by the structure, function, and dynamics of complex interconnected and regionally distinctive food webs (McCormack et al., 2020; Hill et al., 2021). Food web structure can be understood in terms of energy transfer and biomass flow between different components of the ecosystem, either flowing from primary producers to top predators in the pelagos or exported to the benthos through the sinking of detritus and dead organisms as well as through active transport, thereby serving as an energy source for many benthic organisms (Isla et al., 2006). These energy pathways connect different trophic levels, geographic places, as well as the water column and benthic environment. The types of pathways also determine how much energy and biomass ends up in different parts of the system—both spatially and within the trophic structure—whether that be in the form of the bodies of iconic wildlife and fished species, or in the form of carbon that is sequestered in deep waters through sinking and mineralisation. As such, food web ecology has considerable relevance to policy makers, as management decisions have to rely on knowledge of food web structure and function to predict and mitigate the effects of change (Constable et al., 2017; Trebilco et al., 2020).
Understanding and predicting the dynamics of Southern Ocean food webs is challenging, complicated by a myriad of direct and indirect (networks of) interactions amongst species, many of which have highly variable spatial and seasonal dynamics (Murphy et al., 2012). Combined with this variability are the past, current, and future effects of climate-induced changes (Morley et al., 2020) and human activities (Grant et al., 2021) on habitats and biology in the Southern Ocean (Constable et al., 2014; Turner et al., 2014). These drivers of change can cause rapid and significant effects on species, habitats, broader ecosystem functioning, and resilience. The complex adaptive nature of oceanic ecosystems further complicates our ability to determine the range of responses that food webs may exhibit to perturbations.
Most Southern Ocean species have broad distributions with trophic interactions varying across seasons, regions, and habitats. Such variation impacts trajectories of network reorganisation following disturbances, illustrating the importance of accounting for spatial heterogeneity (Cordone et al., 2020; also see Section “Ecological Network Models” and Box 1 for a description of ecological networks and network modelling in a food web context). Whilst it is impossible to empirically document the spatial variability and scales of all trophic interactions across the Southern Ocean, significant progress has been made in recent decades toward characterising Southern Ocean food webs at a regional scale (see Figure 1 for locations). Here, we consider that Southern Ocean food webs include species that wholly reside in the Southern Ocean, south of the Subtropical Front (Morley et al., 2020), as well as migratory species that obtain much of their sustenance within the Southern Ocean, such as whales and flying birds (Constable, 2005).
BOX 1. Network science as a powerful approach to describe the structure and dynamics of food webs.
Network science has become a powerful interdisciplinary approach for describing, quantifying, and analysing the structure and function of ecological communities. Through application of well-developed mathematical concepts from graph theory (Iñiguez et al., 2020), network science approaches allow for the exploration of questions across various ecological scales, ranging from individual species to community-level analyses (Poisot et al., 2016). Graph theory, the branch of mathematics concerned with pairwise relations between objects, has provided a range of measures to quantify and interpret interactions, scaling from pair-wise interactions between species to complex whole ecosystem approaches (Delmas et al., 2019).
Network science has been applied across various spatial scales in the Southern Ocean from characterisation of the general Antarctic food web (Carscallen and Romanuk, 2012; de Santana et al., 2013) to more localised studies of the complexity, structure, and function of several coastal and open-ocean ecosystems. Important insights have been gained into mechanisms for energy flow, the relative importance and traits of individual species and the influence of environmental variables (e.g., sea-ice) on the structure of local food webs (Jacob et al., 2011; Marina et al., 2018a; Rossi et al., 2019). Evolving state-of-the art techniques are opening up new possibilities of applying network science to investigate Southern Ocean food webs that go beyond simple measures and correlation analysis. Based on the concept of causal networks (Runge et al., 2019) and complex adaptive systems (Griffith, 2020; Eyring et al., 2021), they have the potential to help identify the causal effects on food web structure and functioning from the complex interplay between environmental and human induced changes on food webs.
We consider that there are a range of future applications of network science building on the progress to date. Priority areas of research include: (1) A generic food model should be developed as a common structural base for further work and as a base for collective knowledge (Murphy et al., 2016). This can initially be a qualitative or semi-quantitative food web model (see section “Qualitative Modelling and Network Approaches”). (2) Quantifying the interaction strengths or fluxes in food webs. This is currently considered difficult for the Southern Ocean requiring intensive experimental and observational efforts. A viable solution is to extend the qualitative model or use a more sophisticated model and use mathematical proxies to calculate interaction effects such as energy fluxes. One approach is to assume that energy fluxes are driven by a top-down effect (energy demands of top predators). This can also be extended to a general model using allometric rules (Gauzens et al., 2018). (3) Extending species-based food web models to ones based on body-size architecture and species traits (e.g., Blanchard et al., 2017). For instance, this has the potential to identify critical interactions and linkages between energy pathways that stabilise food webs (e.g., Blanchard et al., 2011; Brose et al., 2019). (4) Deciding on a common group of measures to characterise the different regional properties of Southern Ocean food webs and to feed into research and monitoring plans such as for Marine Protected Areas. Four metrics from graph theory that are applicable are Degree, Betweenness centrality, Google Page Rank, and Modularity. (5) Uncovering the building blocks of Southern Ocean food webs regionally and across gradients applying the network science concept of motifs and higher-order connectivity patterns (Benson et al., 2016). These patterns have important consequences to dynamic stability and how food webs may adapt to perturbations. (6) Extending Southern Ocean food web research to include social- ecological interactions including governance. (7) Integrating more sophisticated network science analysis with whole ecosystem models. For instance, to identify which species or traits are more important for transferring the complex effects of multiple stressors (Griffith et al., 2018). (8) Developing a circumpolar and/or regional Dynamic Bayesian Networks as a Decision Support tool to help establish potential adaptation strategies.
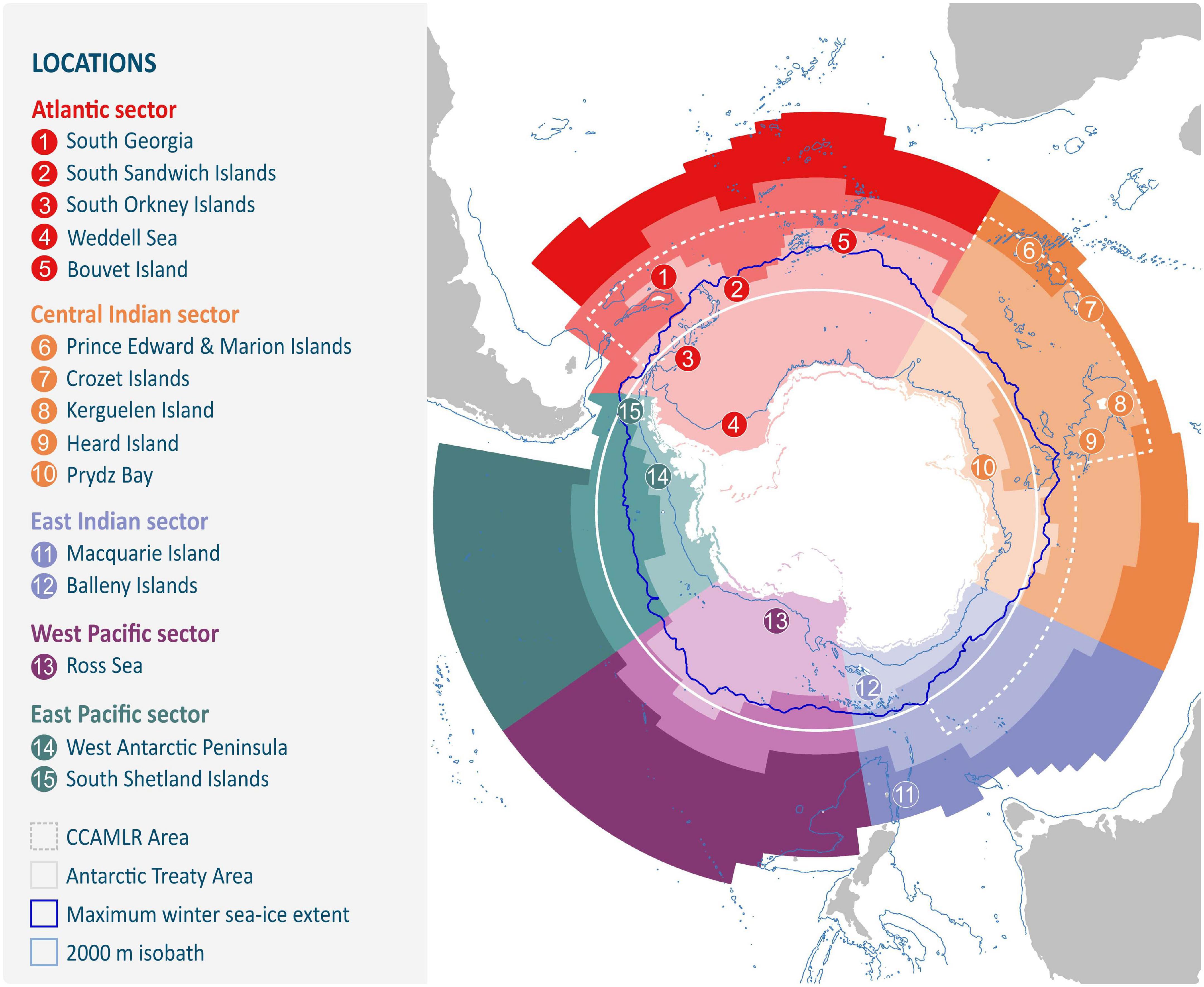
Figure 1. Boundaries for the Marine Ecosystem Assessment for the Southern Ocean (MEASO) areas including five ocean sectors (Atlantic, Central Indian, East Indian, West Pacific, and East Pacific) each with three zone divisions. Sectors are divided meridionally. Zones extend from the coast to the Southern Antarctic Circumpolar Current Front (Antarctic Zone), to the Subantarctic Front (Subantarctic Zone) and to the Subtropical Front (Northern Zone). Islands are marked in white. Antarctica includes ice shelves. Antarctic Treaty is denoted by the solid white line and the area of the Convention on the Conservation of Antarctic Marine Living Resources is shown with a dashed line, which approximately follows the Antarctic Polar Front. The 2,000 m isobath is shown in light blue with the approximate position of the maximum winter sea ice extent shown in dark blue. Numbers refer to locations mentioned throughout the manuscript or with relevance to food web studies and correspond to those listed in the key.
Two parallel activities have been expanding over the last decade since the programme Integrating Climate and Ecosystem Dynamics of the Southern Ocean (ICED) first reviewed the gaps and priorities for understanding Southern Ocean food webs and what was needed to support decision-makers (Murphy et al., 2012). The first set of activities relates to building a greater depth of understanding of food web linkages using enhanced diet studies involving isotopes, genetics, and other trophic markers (e.g., McCormack et al., 2019a; 2020). The second has been the development of models of multispecies interactions, food webs, and ecosystems, derived from diet and foraging studies (discussed in section “Current Developments in Food Web Modelling”). Insights gained from the modelling studies, ranging from theoretical analyses of ecosystem resilience and adaptation to qualitative and quantitative descriptions of food webs, have assisted in resolving patterns of energy flow and the ecological mechanisms that drive food web structure and function at a regional level (Figure 2; previously reviewed in Murphy et al., 2012).
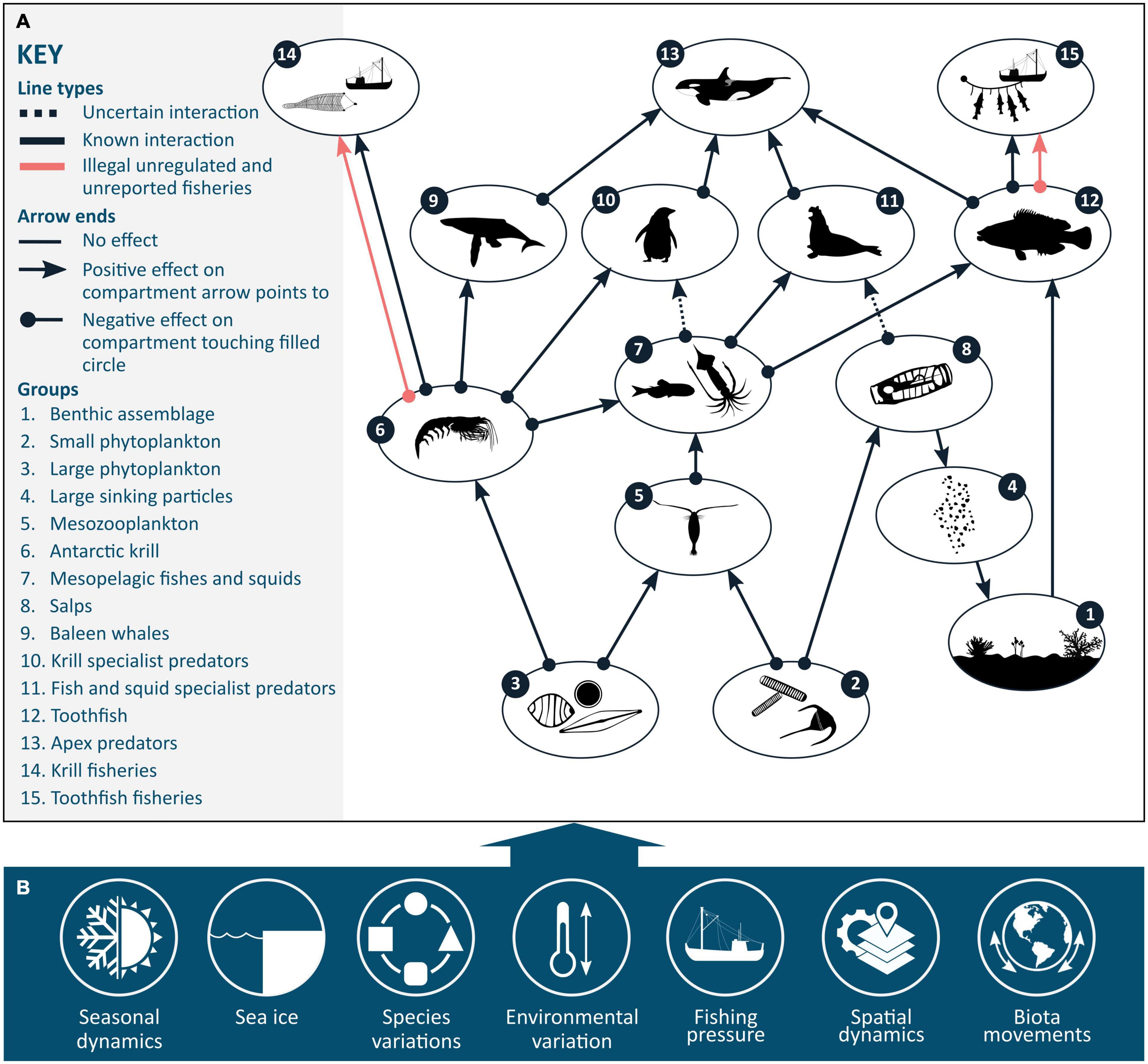
Figure 2. (A) Simplified representation of a Southern Ocean food web, incorporating links to dependent fisheries. (B) Key controls underlying food web dynamics. Figure adapted from Trebilco et al. (2020).
The interactions of individual species with prey, including foraging ecology, are examined in detail in other papers associated with the Marine Ecosystem Assessment for the Southern Ocean (MEASO, 2020). In this paper, we contribute to MEASO by evaluating the current state of food web and ecosystem modelling for supporting assessments of current and future change in Southern Ocean ecosystems and for informing decisions relating to the management and conservation of those ecosystems. We divide this contribution into a further five sections. Section “Approach” details the approach we have taken and its rationale. Section “Characterising Southern Ocean Food Webs” examines regional variations in the characteristics of Southern Ocean food webs, including key drivers of energy flow and the dominant species giving rise to different energy pathways, regional variation in the relative importance of those pathways and how those pathways may change. In section “Attributes of Food Web Models for Supporting Policy,” we examine the attributes of food web models for supporting policy by examining food web (network) properties that may provide indicators of change as well as system resilience. We describe how food web and ecosystem models can be used to inform policy makers on scenarios for and risks in the future, what field measures are needed to help validate models and refine them over time, and how the models can be used to test the efficacy of management strategies. Section “Current Developments in Food Web Modelling” reviews the current developments in food web modelling and their capabilities in supporting policy, and the key issues to be resolved in building models to support policy. Lastly, section “Concluding Remarks—Addressing Needs of Policy Makers” considers the capability of food web and ecosystem models to address topical issues, current constraints/limitations, and outlines priorities for model development.
Approach
The focus of this paper is on progress in development of modelling of Southern Ocean food webs as part of the broader ecosystem. In this context, food web models incorporate representation of all trophic levels, the potential for competition within trophic levels, and non-trophic transfers such as bentho-pelagic flux. This definition may be seen to include biogeochemical models that typically include representation of nutrients (N), primary production (P), secondary production (Z = zooplankton), and carbon export (D = detritus). In this paper, we do not review biogeochemical models in detail but instead consider the models that help address policy questions relating to food web interactions at higher trophic levels, which need greater flexibility in considering (unpacking) different functional groups and relationships.
While not always the case, we have in mind that food web models are informed by, if not embedded within, end-to-end ecosystem approaches to modelling. In that respect, compartments of the model would typically involve interactions between physics, chemistry, and biology, where food webs would be the biological part. The term “food web” relates to energy flow. In this respect, the interpretation of “food web” needs to be broader than a trophic network but also include the potential for non-trophic mortality or competition for resources other than food. These processes are common within the benthic environment, where benthic disturbance and competition for space may be important for structuring benthic assemblages and their influence on benthic-pelagic coupling and carbon sequestration. That said, the ability to include models of non-trophic interactions is poor at present for Southern Ocean ecosystems, despite some important progress over the last decade (e.g., Kéfi et al., 2015). As a result, we focus this review on pelagic food webs, taking note wherever possible of what needs to be done to improve the representation of benthic processes and bentho-pelagic coupling in Southern Ocean ecosystem dynamics.
Our discussion of progress in Southern Ocean food web modelling is informed by broader reviews of marine ecosystem and food web modelling elsewhere (e.g., Fulton and Link, 2014; Melbourne-Thomas et al., 2017; Fulton et al., 2019) and by the knowledge of Southern Ocean biological interactions contained within the MEASO research topic (MEASO, 2020) and other recent reviews. We do not undertake similar reviews here but summarise those reviews in Table 1. Instead, we describe the important characteristics of Southern Ocean food webs that should be represented in models and the attributes that models need to have to support policy. Finally, we consider the progress being made toward that level of modelling and the gaps and priorities for the future.
Characterising Southern Ocean Food Webs
Despite the highly dynamic nature of Southern Ocean ecosystems at a large geographic scale, consistent patterns in food web structure exist (Murphy et al., 2012). Such regularities can help determine ecosystem functioning (e.g., productivity, nutrient cycling, energy flow) (Griffith et al., 2019). Identifying these consistent patterns and their links to function contributes to our understanding of the key controls that underlie food web dynamics (Figure 2). One of the most common descriptions of food web structure and function is the relative importance of various species and trophic relationships in dictating how energy flows through an ecosystem (i.e., energy pathways). Multiple studies have described the various energy pathways that exist within Southern Ocean ecosystems, with their dominance varying across regions and between seasons (Ballerini et al., 2014; Suprenand and Ainsworth, 2017; Dahood et al., 2019; McCormack et al., 2019b, 2020; Hill et al., 2021).
The food chain from phytoplankton through Antarctic krill (Euphausia superba) to various marine mammal and bird species is typically dominant in Antarctic food webs (Hill et al., 2006; Murphy et al., 2012; McCormack et al., 2020). This represents an efficient energy pathway from primary producers to higher trophic levels, with the potential to sustain a large biomass (Clarke, 1985). Such central dependence of higher trophic levels on krill is unique to the Antarctic, leading to krill often being classified as a key “forage fish” in the context of general ecosystem model structure (Essington and Plagányi, 2014; Plagányi and Essington, 2014). For instance, the diets of baleen whales include a more diverse range of prey species (e.g., fish) in other systems such as the northern hemisphere. The importance of Antarctic krill in providing the majority of energy needed to sustain higher trophic level production has been well demonstrated in various regions such as the Antarctic Peninsula (Ballerini et al., 2014; Dahood et al., 2019).
In addition to the archetypal krill pathway, alternative pathways exist for this transfer of energy to top predators; these pathways sustain other predators less reliant on krill, notably toothfish, toothed whales, and a number of seals, penguins, and flying birds (Figure 2A; Atkinson et al., 2004; Murphy et al., 2016; McCormack et al., 2019b, 2020; Subramaniam et al., 2020a), or provide alternative pathways to the suite of krill dependent predators (e.g., Saunders et al., 2019). Various studies have emphasised the importance of these alternative configurations for energy flow, usually involving various combinations of other zooplankton, fish, and squid species (Ballerini et al., 2014; Suprenand and Ainsworth, 2017; Dahood et al., 2019; McCormack et al., 2019b). These diverse energy pathways maintain a range of ecosystem services (e.g., high-value fisheries, carbon sequestration; Cavanagh et al., 2021) where distinct suites of species provide unique contributions (Barnes and Sands, 2017; Constable et al., 2017). However, what determines the relative dominance of different energy pathways in space and time generally remains poorly understood (Constable et al., 2017).
Southern Ocean food webs are highly temporally and spatially variable across multiple scales (Murphy et al., 2012). There is marked seasonality, which varies in intensity from the permanently ice-covered region of the southern Weddell and Ross Seas (see Figure 1) to the northern areas of the Polar Frontal Zone and Sub-Antarctic Front. There is also a high level of interannual variability in regional ocean temperatures and sea ice conditions associated with atmospheric variation across the Southern Hemisphere. The asymmetric nature of the Antarctic continent and the complex bottom topography results in varied patterns of ocean circulation and seasonal sea ice dynamics across the Southern Ocean. This temporal and spatial variability generates different physical and chemical conditions, which are crucial determinants of regional food web structure (Murphy et al., 2012). There is also, however, a high degree of connectivity among regions as a result of transport in association with both ocean currents and sea ice drift along with the movement of organisms during development, foraging and migration (Figure 2B; see also Murphy et al., 2012; Thorpe et al., 2019; Treasure et al., 2019). This combination of variability and openness of ecosystems across multiple scales challenges simple definitions of regional boundaries between food webs, and hence also characterisation of the structure of food webs (McCormack et al., 2020).
Insights gained from regional analyses of food web structure and the mechanisms for energy flow (McCormack et al., 2020; Hill et al., 2021) have provided the foundation for developing a generalised view of Southern Ocean food webs, one that highlights the importance of alternative energy pathways and regional variability in the relative importance in those pathways (Murphy et al., 2012). For example, Murphy et al. (2013) drew upon earlier quantitative food web analyses (Hill et al., 2012; Sailley et al., 2013; Ballerini et al., 2014), to compare ecosystem structure in two major regions: South Georgia and the west Antarctic Peninsula. They showed structural similarity in the food webs, highlighting the importance of ecological connectivity across the Scotia Sea from sea ice dominated regions in the south to warmer open ocean regions in the north. Despite the similarities, the study also emphasised that the dominant species at mid-trophic levels involved in the major pathways of energy flow are not the same across the different habitats (Murphy et al., 2013). In northern regions, and north of the Polar Front, copepods and mesopelagic fish (myctophids or lantern fish) are key mid-trophic levels species, while across much of the Antarctic Circumpolar Current (ACC) south of the Polar Front, Antarctic krill become a dominant feature with mesopelagic fish less so. In the most southerly areas of regular sea ice cover, it is ice-krill and silverfish that are the most important mid-trophic level species (Murphy et al., 2013, 2016; Ballerini et al., 2014; McCormack et al., 2019b). Squids can also be important in some areas (Subramaniam et al., 2020a). Salps have long been considered to be an alternative dominant herbivore to Antarctic krill (Murphy et al., 2012), possibly even competing but more likely occurring in different water masses (Johnston et al., in this research). The role of salps in the food web is less known but becoming increasingly recognised as an alternative energy pathway to krill and copepods in some areas (Kelly et al., 2020).
Further comparative analysis of the structure and pathways of energy in food webs at South Georgia and the west Antarctic Peninsula was undertaken by Murphy et al. (2016, Supplementary Information). The study reanalysed food web data, re-aggregated the species and groups into size groups and recalculated energy flows between groups. The study further demonstrated the basic similarity of food web structure and the crucial role of Antarctic krill in both ecosystems. The comparative analysis of these food webs suggests that the South Georgia food web is more dependent on external productivity (e.g., import of secondary production from external regions) than that in the west Antarctic Peninsula region. This may, however, reflect differences in initial food web analysis and data rather than real differences between the actual food webs.
The view that polar pelagic food webs are dominated by relatively few major pathways of energy flow involving a small number of mid-trophic level species was further developed for the wider Southern Ocean and polar regions more generally in Murphy et al. (2016). They noted that the crucial role that these key mid-trophic level species play in Southern Ocean food webs is related to their life history traits and the associated habitats in which they dominate. This led to the recommendation that development of understanding of polar food webs and the impact of change not only requires analyses of food webs but also quantitative studies and models of the life history processes of the key species (Murphy et al., 2016).
Major gaps remain in information on food web structure for most areas of the Southern Ocean and on the species involved in major flows of energy, the degree of sub-system connectivity (e.g., pelagic—benthic—sea-ice) and seasonal changes in interactions. An increased focus on the development of comparative analyses of food web structure will be crucial for improving understanding and generating and validating models for projecting the potential impacts of future change. A concerted and systematic effort is required to quantify and compare food web structure and variation throughout the Southern Ocean (Murphy et al., 2016; Newman et al., 2019). Such studies would also need to quantify the seasonal and interannual variation, food web subsidies (input of external energy: allochthonous production) and inter-food web connectivity (Murphy et al., 2007, 2013, 2016; Treasure et al., 2019). Understanding of the life-histories of many of the less-studied key mid-trophic level species is improving (e.g., Moteki et al., 2017; Queirós et al., 2018; Groeneveld et al., 2020; Kelly et al., 2020; Lin et al., 2020; Saunders et al., 2020; Zhu et al., 2020), however, for most species, quantitative understanding of life-cycles and population dynamics is still very limited (Constable et al., 2014, 2017). Models of distribution and population processes of these species are required to provide inputs into food web models.
Attributes of Food Web Models for Supporting Policy
Assessing variability and change in Southern Ocean food webs and their underlying causes is required to inform policy and guide management. Constable et al. (2016) discuss four general, inter-related classes of ecosystem properties relevant to food web structure and function that may be used to develop statements on status and trends to inform policymaking:
1. Primary production: Production of organic material by photosynthetic and chemosynthetic autotrophs.
2. Structure: Abundances of taxa in space and time, related to patchiness of the organisms; size structure of populations and functional groups; relationships between biota (including trophic connections); and processes/responses that give rise to structure (note that the meaning of “structure” here encompasses both food web network structure and the structure of populations).
3. Production: Production of organic material at different trophic levels of the food web in a region. This may include factors that affect production such as non-trophic interactions and disease.
4. Energy transfer: Efficiency in transferring/utilising energy in the food web, which will need to account for spatial and temporal overlap of consumers and resources, which in turn will be affected by habitat characteristics and behaviour.
Climate-driven changes in physical and chemical conditions are expected to generate shifts in the distribution of organisms during the coming decades, which will affect food web structure as a consequence (e.g., Hill et al., 2012; Murphy et al., 2012, 2013, 2016; Ballerini et al., 2014; Suprenand and Ainsworth, 2017; Dahood et al., 2019; McCormack et al., 2019b). Developing projections of change in food web structures will require models that can represent the major alternative structures and energy transfer pathways that exist now or may occur in the future, requiring a nuanced understanding of potential shifts in the food web. Development of models for multiple regional food webs with a high degree of species resolution is useful for improving understanding. However, given the current relatively poor state of knowledge of the structure of food webs throughout much of the Southern Ocean, and the low resolution of models used to generate future projections, such high-resolution food web modelling is less useful at this time for considering large scale, Southern Ocean-wide responses to change. Instead, a more generalised and generic approach, in which the major components of food webs throughout the Southern Ocean can be represented in a single model structure, is likely to be more useful in generating projections and understanding the potential impacts of future change (Trebilco et al., 2020). In current ocean-scale models, biogeochemical processes and plankton dynamics are represented by generalised and often very simple models that can capture major structural and process variations. A similar approach has been proposed for Southern Ocean ecosystems, aiming to generate generic representations of the wider (meta-) food web that provides a basis for predicting the major pathways of energy flow and food web structure in different regions and the potential impacts of future change (Murphy et al., 2012, 2013, 2016).
A challenge for researchers and for observing systems is to identify and measure meaningful indicators of these ecosystem properties. So-called ecosystem Essential Ocean Variables (eEOVs) are defined as biological and ecological variables selected for regular measurement by observing systems, that can be used as indicators of status and change in ecosystem properties (as well as to attribute the causes of change), and that are feasible to collect at appropriate spatial and temporal scales (Constable et al., 2016). eEOVs can also inform the development of ecological models (e.g., qualitative, statistical/empirical, dynamic mathematical models) to support assessments. The identification of eEOVs for Southern Ocean ecosystems is an ongoing process (Newman et al., 2019; Muelbert et al., to be published in this research topic) and the identification of key data streams that constrain biological energy pathways by better characterising the transfer of mass through food webs, into carbon export, and into fish stocks has been identified as a priority to inform Southern Ocean ecosystem modelling, assessment, and management (Constable et al., 2017; Newman et al., 2019).
Indicators of food web processes of utility to policy making can also relate directly to Southern Ocean ecosystem services (Cavanagh et al., 2021). For example, Trebilco et al. (2020) considered four types of indicators relating to ecosystem services, using network models to investigate their response to perturbations in a simplified food web. These indicators included target species of fisheries (Antarctic krill and toothfish), predator groups of conservation importance (krill specialists such as Adelie penguins, and fish/squid specialists such as elephant seals), baleen whales and carbon export potential. Modelling changes in these indicators under different scenarios for climate change impacts and human use can help in identifying where there might be counterintuitive changes in ecosystem services due to feedback effects in Southern Ocean food webs.
Current Developments in Food Web Modelling
Food web and ecosystem models have been fundamental and highly influential to the provision of robust, policy-relevant scientific advice about status and change, particularly in the context of ecosystem-based management of fisheries (Essington and Plagányi, 2014). Such models continue to be used in assessments of change, investigations of causality and the prediction of potential impacts. These models vary in their representation of ecological systems, ranging from conceptual models, qualitative mathematical models, statistical algorithms, and dynamic quantitative simulation models (see Fulton and Link, 2014 for a review of model types and applications) (Figure 3). They allow for the exploration of alternative conservation and management strategies in relation to achieving specified objectives. Through synthesising information and representing an ecosystem or species in a coherent form, particular questions of interest can be explored to generate scientific advice.
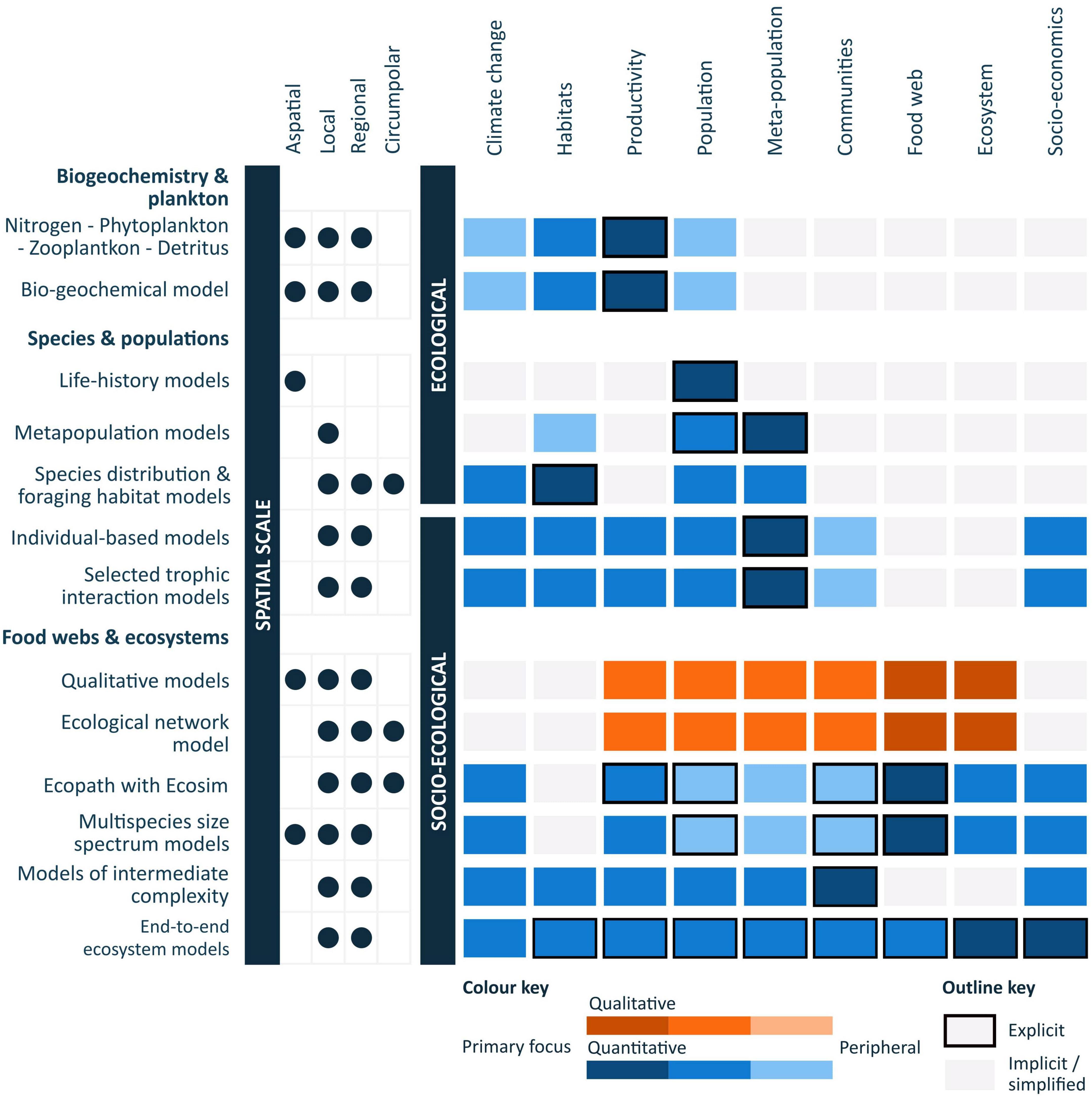
Figure 3. Summary of various ecological modelling approaches and the associated spatial and socio-ecological scales at which they can be applied (concept visualisation based on Fulton and Link, 2014; Melbourne-Thomas et al., 2017). Coloured rectangles indicate the inclusion and level of focus (i.e., dark—primary focus, light—peripheral) of each of the components for the summarised qualitative (orange) and quantitative (blue) models. Reference to spatial scales relate to Southern Ocean applications.
Whilst modelling approaches and applications across the Southern Ocean vary considerably in scope (i.e., socio-ecological and spatial representation), each provide a degree of insight into various aspects of Southern Ocean food webs. This section discusses approaches to modelling Southern Ocean ecosystems, outlining the insights and gaps highlighted by various models (published following Murphy et al., 2012) in relation to food web structure, function, and change. The key limitations of these existing models and their potential to be integrated in support of Southern Ocean food web assessments to inform policy are evaluated in section “Concluding Remarks—Addressing Needs of Policy Makers.”
Qualitative Modelling and Network Approaches
Qualitative Models
Qualitative models can help to capture, communicate, and represent knowledge of food webs. They can be used in a multi-step process starting with a simple conceptual model of the pair-wide relationships (e.g., feeding interactions) for specific research questions, to being used in “real time” workshop sessions to collectively pool knowledge across many researchers and stakeholders. To date, these models have been used for the Southern Ocean to identify interactions to aid quantitative model development and for assessing the consequences of simulated changes on interaction pathways (Melbourne-Thomas et al., 2013a; Goedegebuure et al., 2017; Figure 3). These types of models remain underutilised in the Southern Ocean context particularly for integrating and communicating food web research results along with other concepts and research results to aid policy decisions (e.g., Trebilco et al., 2020). Decision-support software (e.g., www.mentalmodeler.com; or QPress in R, Melbourne-Thomas et al., 2012; https://github.com/SWotherspoon/QPress) exist that allow a scenario interface to be easily developed to allow stakeholders to run and compare changes under different potential scenarios representing collective knowledge and then revisit and revise in the light of new information.
Ecological Network Models
Network science, meaning the study of interactions to quantify the structure and dynamics of complex systems such as food webs (see Box 1), has proved useful in evaluating the diverse effects of climate change (i.e., local extinctions, sea-ice dynamics) on the structure and functioning of Southern Ocean food webs (Jacob et al., 2011; Cordone et al., 2018; Rossi et al., 2019). A comparison between pelagic regions in the Arctic and Antarctic using standard network measures such as degree distribution (frequency distribution of the number of trophic links) suggests that the most connected prey species in Antarctic food webs, such as E. superba are more vulnerable to extinctions (de Santana et al., 2013). Network science suggests that many food webs exhibit the so-called “small-world” pattern that involves high clustering and short interaction path lengths between species conferring resistance to perturbations (such as species removal) (Montoya and Solé, 2002). Marina et al. (2018b) studied the prevalence of such a property in food webs of two Southern Ocean locations: Potter Cove (South Shetland Islands) and Weddell Sea. Interestingly, Potter Cove and the Weddell Sea food webs showed opposite results, meaning that the latter network presents a “small-world” pattern while the former does not. This implies that understanding how each local food web self-organises is essential to gain precise knowledge about the impact of perturbations (e.g., climate change) on local food web structure and function.
Two recent studies of Arctic food webs using a network science approach suggest that polar food webs can self-organise through new interaction pathways. What had previously been considered as a system-wide regime shift with changing climate and species invasion was shown to be limited to changes in a limited set of species interactions (Yletyinen et al., 2016). In another study, the core ecological processes of an Arctic food web have been shown to be resilient to cumulative perturbations (ocean warming, decreasing sea-ice and arrival of invasive species) by the self-organisation of direct and indirect species prey interactions. Both studies caution that the resilience may be short-lived (Griffith et al., 2019; Yletyinen, 2019). Resilience in this case is defined from a complex adaptive systems perspective (Hagstrom and Levin, 2017) that the resilience of food webs to on-going perturbations emerges from the dynamic self-organisation of small-scale interactions. It remains unclear if Antarctic food webs show a similar pattern to perturbation. A probabilistic model that accounts for trophic structure, colonisation, and extinction events from the Antarctic metaweb suggests that the modification of habitats due to climate change will probably modify individual populations distributions and abundances (Saravia et al., 2021). However, food web structure could rearrange without producing abrupt changes (regime shifts) (Saravia et al., 2021). This may be due to internal dynamical feedback (Suweis and D’Odorico, 2014; Ward et al., 2018).
Dynamic Modelling
Modelling Key Species and Trophic Interactions
Developing a suite of models that vary in scope and their spatial and socio-ecological representations (Figure 3) is required to support management approaches in complex ecological systems (Murphy et al., 2012; Fulton et al., 2015; Melbourne-Thomas et al., 2017). Through offering varying representations of ecological scales (e.g., individual, local, regional, global) and dimensions (e.g., physical, ecological, social, management) (Figure 3), integrated modelling approaches can provide flexibility for supporting decision making and for constraining the abstracted views developed for an ecosystem (Murphy et al., 2012; Melbourne-Thomas et al., 2017). Insights gained from models that focus on particular species of interest (see Table 2 for Southern Ocean examples) can provide knowledge into key food web processes and guide the development and implementation of multi-species management tools (Plagányi, 2007).
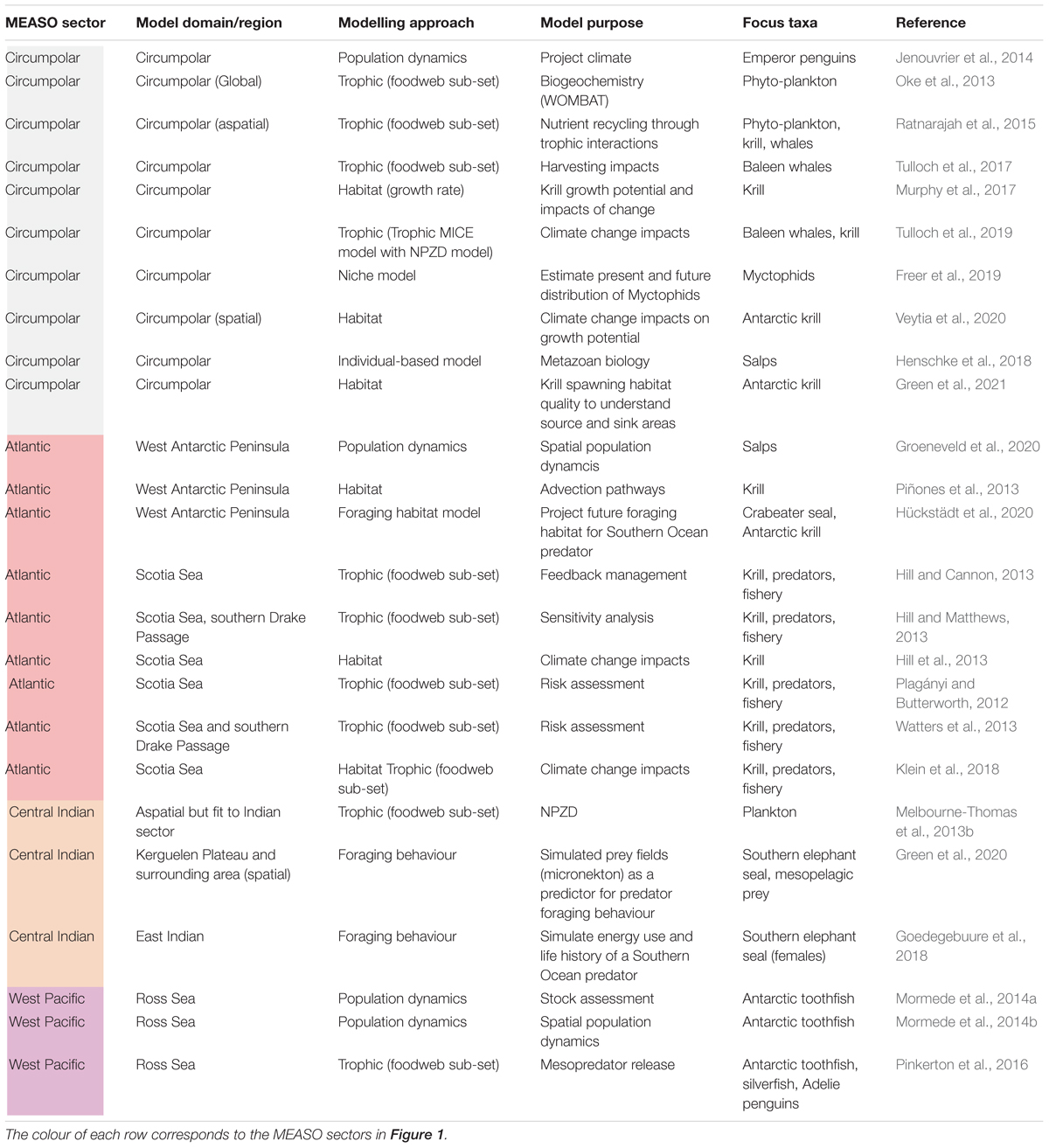
Table 2. Examples of published models (since 2012) for the Southern Ocean which capture habitat, population and trophic interactions for key species (not fully comprehensive).
In Table 2 we assess the spatial coverage of Southern Ocean focussed models published since the last assessment of Southern Ocean food webs in 2012 (see Murphy et al., 2012), with respect to each MEASO sector (Figure 1). Table 2 also distinguishes between the following classes of models, all of which can be used to inform the development of food web models and/or to validate them:
i) habitat models (including species distribution models, niche models and foraging habitat models) that might influence interpretation of food web models i.e., “where might a particular species do best”;
ii) population dynamics models, including both non-spatial (life-history modelling) and spatial (metapopulation) approaches e.g., matrix models and stock assessment models; and
iii) trophic models (including predator-prey models and Models of Intermediate Complexity (MICE; Plagányi et al., 2014) that focus on a particular species and represent a subset of the food web.
Bridging the gap between single-species assessment models and more comprehensive ecosystem models are those that consider system operation and change based on a subset of the ecosystem and key processes, i.e., selected trophic interaction models (such as predator–prey models) and MICE models (Figure 3). These models have a long history of use in the Southern Ocean, particularly for evaluating the nature and impact of fishing on predators that share a fished resource. Beginning with the work of May (1979) and the subsequent agreement for the Convention on the Conservation of Marine Living Resources (CCAMLR), explicit acknowledgement of the importance of maintaining the integrity of food webs to support dependent species was developed, laying the foundation for the input of modelled insights into management and policy decisions globally (Plagányi, 2007).
Historically, the nature and impact of fishing on predators that share a fished resource has been an important consideration in ecosystem-based fisheries management (Constable, 2001). In the Antarctic, predator–prey modelling procedures have been used to assess the impacts of Antarctic krill harvesting on krill predator populations and to inform subsequent recommendations for setting annual krill catch levels. For example, initial modelling estimated the level of krill harvesting that would reduce the availability of krill and hence impact predator populations (Thomson et al., 2000). More recently, modelling procedures have been developed to consider these krill predation issues and advise on the spatial allocation of krill catches (e.g., in the Scotia Sea; Plagányi and Butterworth, 2012). Watters et al. (2013) developed a dynamic model of Antarctic krill, its predators and fishery, to explore potential krill fisheries impacts in the Southwest Atlantic. Klein et al. (2018) modified the model to simulate ocean warming impacts on krill growth by incorporating the results of Hill et al. (2013). These results suggested spatial disconnect between the strongest potential impacts of krill biomass and those on the abundance of its predators.
Whilst there is a general acceptance that parameter estimates for krill are often highly uncertain and subject to spatial, seasonal and interannual variability (Hill et al., 2006; Atkinson et al., 2012a), many models have developed innovative methods for dealing with such uncertainty (Hill et al., 2012; Watters et al., 2013). For example, Plagányi and Butterworth (2012) used models that are composed of alternative combinations of parameters to bound the uncertainty. Nevertheless, better understanding of the fine-scale interactions between both predators and fisheries with krill in relation to fishing operations and foraging trips (Cresswell et al., 2008; Waluda et al., 2010) will help refine some of these uncertainties along with identifying critical gaps in representations of krill in food-web models (Atkinson et al., 2012a).
Whole-of-Food Web Models
Ecopath with Ecosim (EwE) (Christensen and Walters, 2004) has been one of the most widely applied modelling frameworks for assessing the impacts of fishing and environmental change on Southern Ocean food webs. Indeed, there are only a few examples of whole-of-food web models for the Southern Ocean that use modelling approaches other than EwE (see Table 3), and there are no published whole-of-ecosystem models for any region of the Southern Ocean. There are many approaches to evaluating how change propagates through modelled networks using EwE including manipulation of specific ecosystem components, analytical methods (e.g., metrics built into the EwE software) and more intensive methods such as the forcing of parameters in dynamic Ecosim models to represent the direct impacts of a specified driver.
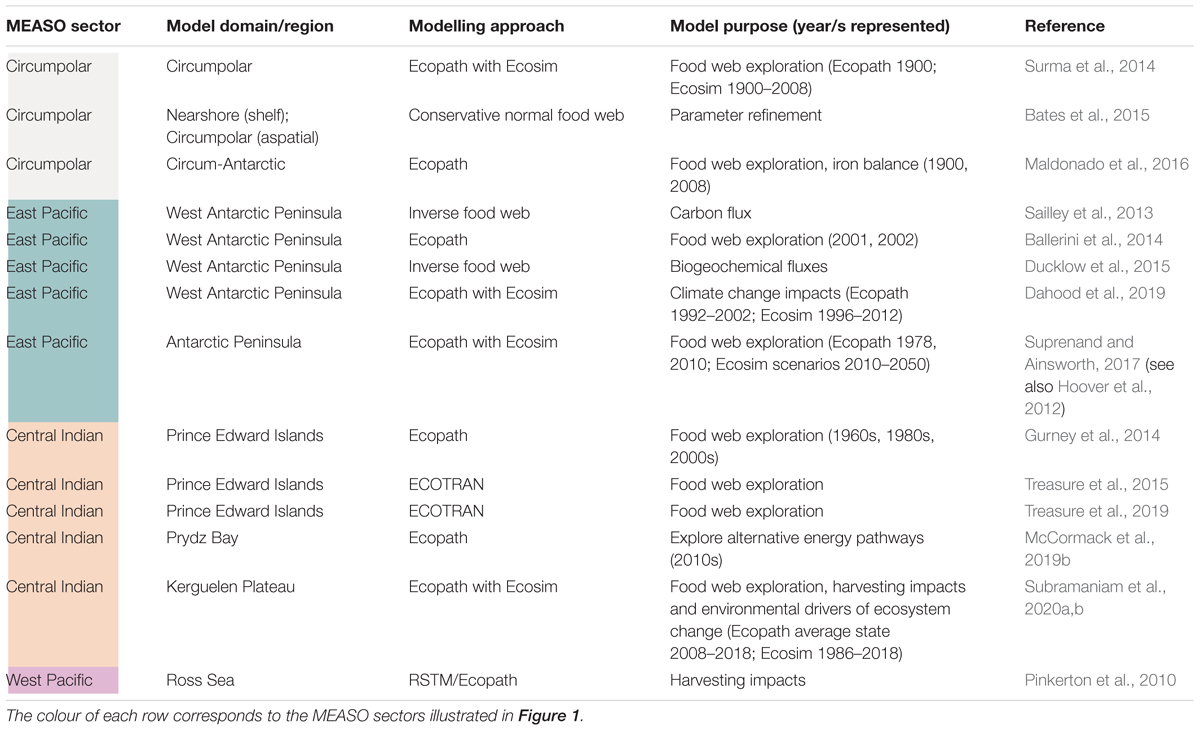
Table 3. Examples of Southern Ocean EwE and whole-of-food web models published since 2012 (not fully comprehensive).
Based on mass-balance constraints, Ecopath describes food webs in terms of the biomass, consumption, production and diets of species or life stages, which can be aggregated into functional groups according to trophic similarity and/or data availability. Applications of Ecopath in the Southern Ocean (see Table 3) have contributed significantly to our understanding of food web characteristics and emerging patterns following change across multiple regions and latitudes. Commonly characterised as a top-down model (i.e., trophic interactions are represented in relation to predator diets), Ecopath can be inverted to represent interactions in terms of the fate of prey biomass (Steele and Ruzicka, 2011). This simplified calculation can help tease apart the response of higher trophic levels to changes exhibited by lower trophic levels. Ballerini et al. (2014) used this approach to explore five scenarios of change in an Ecopath dataset representing an area adjacent to the western Antarctic Peninsula. The simulated changes included reduction in the size of unicellular phytoplankton, which predicted a reduced production of Antarctic krill and the top predators that feed on it.
An alternative approach is to manipulate the biomass of functional groups within an Ecopath dataset and to introduce compensatory changes elsewhere in the food web during the balancing process (Hill et al., 2012). McCormack et al. (2019b) and Saunders et al. (2019) used this approach to examine the consequences of reduced krill biomass for other trophic pathways in the Scotia Sea and Prydz Bay. Whereas the krill pathway remained dominant in the former, pathways involving squid and fish became dominant in the latter area. Similarly, the Mixed Trophic Impact (MTI) metric built into the EwE software provides an indication of the effect of a change in the biomass of one functional group on another (Libralato et al., 2006; Christensen et al., 2008). Subramaniam et al. (2020a) used this method to explore the direct and indirect impacts of the Patagonian toothfish (Dissostichus eleginoides) and mackeral icefish (Champsocephalus gunnari) fisheries activities (catch and by-catch) on trophic interactions on the Kerguelen Plateau. This identified the functional groups that frequently interact with fishery target species, and the importance of fished species in the transfer of energy through the food web.
Several studies have used Ecosim to explore the time-dynamic variations of Southern Ocean food webs (Surma et al., 2014; Suprenand and Ainsworth, 2017; Dahood et al., 2019; Subramaniam et al., 2020b). This approach fits models from Ecopath to time-series and/or fisheries data for functional groups (Christensen et al., 2008). Most models are fitted to the data by manipulating how consumption is influenced by changes in predator and prey biomass (vulnerability parameter; Christensen et al., 2008) while others also use forcing functions (e.g., Dahood et al., 2019). Since 2012, Ecosim has been used to explore the effects of whaling and bottom-up forcing, concluding that they may have had counteracting impacts on krill biomass (Surma et al., 2014). Suprenand and Ainsworth (2017) explored potential future change, with most scenarios resulting in reduced biodiversity and increased trophic level. Dahood et al. (2019) evaluated changes to sea-ice cover as an environmental driver of predator–prey interactions, finding that the sea-ice regime was needed to recreate observed biomass trends and Subramaniam et al. (2020b) correlated time-dynamic food web interactions with environmental variables, highlighting sea surface temperature, zonal wind, and the Southern Annular Mode as important drivers of ecosystem change.
An additional component of EwE is Ecospace, which simulates Ecosim models over a two-dimensional grid using habitat maps and movement rates (see Christensen et al., 2014). Ecospace presents an advantage and incentive to build EwE models as food web models that incorporate spatial information are few in the Southern Ocean. One published spatially explicit food web model currently exists for the Southern Ocean, exploring scenarios relating to ecosystem-based fisheries management (Dahood et al., 2020). The model evaluated scenarios regarding the designation of marine protected areas and future changes in sea ice and krill fishing effort. Results from the study indicate that establishing marine protected areas in the right locations could be important for mitigating the negative effects of climate change.
Steps toward addressing model reliability and parameter uncertainty have resulted in additional tools, guides, and improvements to the EwE software. Heymans et al. (2016) provide guidelines for testing and reviewing EwE models for management, detailing pre-balancing diagnostics and thermodynamic and ecological rules for balancing models. Ainsworth and Walters (2015) outline the top ten mistakes made by EwE modellers to help new users side-step pitfalls. Current tools address uncertainties for management strategy evaluation (Steenbeek et al., 2016) and find best statistical fits to Ecosim input (stepwise fitting procedure, Scott et al., 2016). A new development addresses EwE-wide parameter uncertainty by recording alternate mass-balanced parameter sets in the Monte Carlo routine (Ecosampler; Steenbeek et al., 2018). These guidelines and improvements to the software provide a path forward for developing reliable models for ecosystem-based management, including multi-model ensemble approaches to predict ecosystem response to change (Heymans et al., 2016; Hill et al., 2021).
Although the suite of regional food web models has expanded since 2012, the only attempt at a circumpolar food web model (de Santana et al., 2013) is based on a simple interrogation of the Southern Ocean Diet and Energetics Database (Scientific Committee on Antarctic Research, 2020). This is a static network model (see section “Ecological Network Models”) that considers only the links between species, with no possibilities to compensate for the significant spatial heterogeneity in sampling effort. As suggested previously a significant improvement for this model would be to consider weights for the links based on the probability of interactions due to species distributions (Poisot et al., 2015). Most Southern Ocean food web models consider relatively small scales whereas the dynamic models often consider regional (>1 million km2) or circumpolar (>10 million km2) scales. The food web models predominantly consider continental shelf ecosystems. This reflects data availability: intensive ecosystem studies across multiple trophic scales tend to be localised within the area of interest of particular Antarctic operators, which in turn tends to be associated with land-based research stations. The SCAR Southern Ocean Diet and Energetics Database, collated by the SCAR Expert Groups on Antarctic Biodiversity Informatics (EG-ABI) and Birds and Marine Mammals (EG-BAMM), provides data related to diet and energy flow from conventional (e.g., gut content) and modern (e.g., molecular) studies, stable isotopes, fatty acids, and energetic content. At larger spatial scales, the available data are limited to remote sensed chlorophyll data and population or distribution estimates for high profile taxa including Antarctic krill, marine mammals, and penguins. Despite the higher level of detail at intensively studied sites, most Southern Ocean food web models incorporate parameter estimates which are not based on direct observation of the modelled site.
Building on Murphy et al. (2012, 2013), Murphy et al. (2016) suggest that development of a generic food web model approach (i.e., representative of all Southern Ocean regions) is both possible and useful in analyses of Southern Ocean food webs. A number of potential general model structures for food webs have been suggested, involving different sets of species or functional groups or alternative representations of groups of organisms and interactions (e.g., based on classifications of guilds or organism size) (Murphy et al., 2012, 2013, 2016). These provide a valuable basis for the development of generic computational models of Southern Ocean food webs. The aim of such an approach is to apply a single model throughout the Southern Ocean, and on the basis of a series of environmental constraints/drivers (physical and biogeochemical), identify the major structural form of regional food webs and the main species/groups involved in the major pathways of energy flow. Such an approach can be extended to include more complex representations of the food web if there is sufficient understanding and data to validate model outcomes.
While the on-going development of more complex food web models is providing greater understanding of Southern Ocean ecosystems, there is also a growing awareness, particularly from management, of the uncertainties of this approach. Given the complexity and associated uncertainty, ecosystem models face many challenges which are complicated further by the additional uncertainties associated with representing environmental and harvesting scenarios (Travers et al., 2007; Plagányi et al., 2011). General uncertainties include the lack of observational data, concerns on the ability of such models to capture the underlying ecosystem dynamics and the ability to identify emergent behaviour, regime shifts and evolution of food web structure and function (Griffith and Fulton, 2014).
The interactions between species are generally defined by correlations. If the underlying dynamics are non-linear then mirage correlations can occur in which the causally connected interaction can be positive or negative or neutral over different periods of time (Fogarty et al., 2016). The “dynamical systems” perspective offers an alternative and complementary approach to viewing marine food webs (Griffith, 2020). It assumes that the food web results from the complex interplay between multiple processes. Viewing Southern Ocean food webs in this way allows us to leverage some powerful approaches from dynamical systems theory. These concepts are particularly useful in the Southern Ocean context where observing all the species that inhabit an ecosystem is near impossible, and knowledge of which of the observed variables are relevant and causally related is lacking. Rather than attempting to investigate system dynamics through complex equation-based models (e.g., Ecopath models) that explicitly account for each interaction, the “non-linear dynamic” perspective draws on the concept from state-space reconstruction which implies that any variable in a state-dependent dynamic system encodes the history of its interactions with other system variables (Munch et al., 2020). That is, the data itself provides the evidentiary portal to identifying the causal relationships in complex real-world system dynamics. Previously, this approach has been limited by requiring long-time series. Recent work shows that integrating an equation free non-linear approach with a Bayesian model can overcome the problem of short ecological time series (Johnson et al., 2020). To many in marine management, this concept is challenging despite recent work demonstrating the utility of this approach to a wide range of topics in marine ecology (Griffith, 2020). In future work, sensibly applied and complementary to existing Southern Ocean food web models, this approach may provide valuable insights into understanding and predicting causal food web interactions.
Concluding Remarks—Addressing Needs of Policy Makers
Policy makers need information on how changing climate and environmental drivers (Henley et al., 2020; Morley et al., 2020) and local drivers, such as fisheries and pollution (Grant et al., 2021), will impact on the conservation and long-term maintenance of Southern Ocean species, biodiversity and productivity. Modelling to date has shown how these drivers have cascading effects through food webs and ecosystems. The parallel changes that are occurring through climate change, increasing ocean acidification, increasing krill fishing, and recovery of whales means that not only are individual species being affected but, also, the relative importance of different energy pathways may change (Trebilco et al., 2020). Policy makers will need to develop ecosystem-based strategies for achieving their conservation and sustainability objectives that take account of the changing ecosystems (Constable et al., 2017). A good example of this requirement is embedded in Article II of CCAMLR, such that fisheries must be managed in such a way to ensure.
“prevention of changes or minimisation of the risk of changes in the marine ecosystem which are not potentially reversible over two or three decades, taking into account the state of available knowledge of the direct and indirect impact of harvesting, the effect of the introduction of alien species, the effects of associated activities on the marine ecosystem and of the effects of environmental changes, with the aim of making possible the sustained conservation of Antarctic marine living resources.”
Changing system productivity is naturally embedded in existing approaches to managing fisheries under CCAMLR (Constable, 2001, 2002). However, the potential for changing food web structures could lead to unwanted outcomes if they are not countenanced, not only in terms of shifting the relative importance of production of different fished species but also in the conservation of biodiversity generally (Constable et al., 2017). Food web and ecosystem models could be used to support policy makers at present but there is much room for improvement, particularly with respect to the challenges of climate change.
Based on our review here, we provide an expert synthesis to summarise the state of ecosystem models to support policy makers in Table 4. We also summarise the expected requirements of models that may be used in three different forms of advice. The use of food web and ecosystem models for testing specific scenarios is becoming common place, such as through FishMIP (Tittensor et al., 2018) or in specific circumstances (Holsman et al., 2019). Typically, future scenarios are from Earth System models. As a result, end-to-end modelling approaches are needed to simulate and predict changes in the state of marine ecosystems and remain to be developed for the Southern Ocean. Testing scenarios helps form hypotheses about, or frame, plausible futures for which management strategies need to be developed. End-to-end ecosystem models, or simpler versions of them, can be used to evaluate management strategies and for assessing risks of failing to meet objectives (Constable, 2002, 2011; Melbourne-Thomas et al., 2017; Plagányi and Fulton, 2017). A great challenge in these cases is to provide satisfactory representation but with computational economy in order to explore the many and varied uncertainties that plague decision-makers. Novel representations of the properties and dynamics of ecosystems can be very useful in these circumstances if they satisfactorily represent the decision environment and its relationship to the real world (Griffith, 2020).
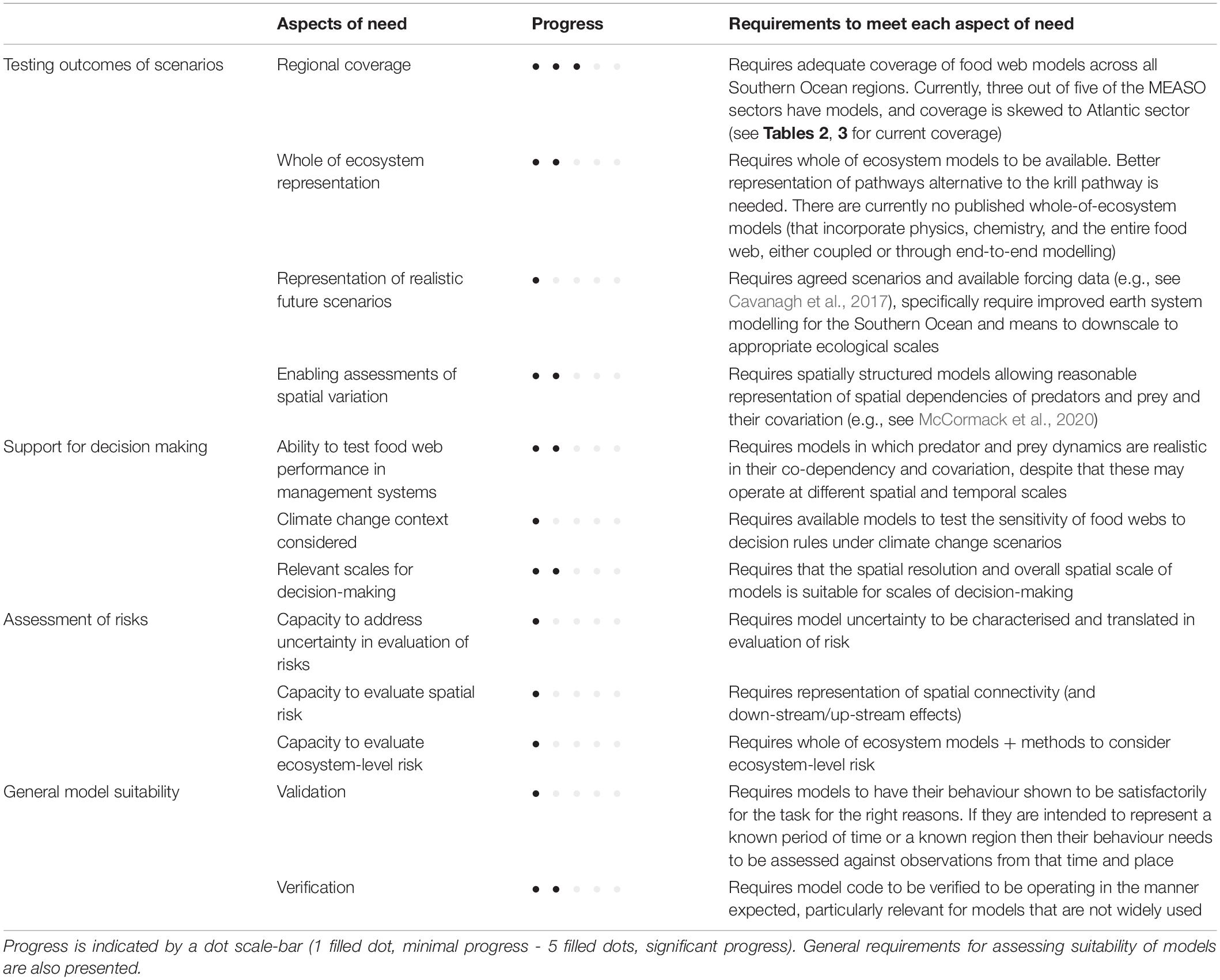
Table 4. Summary of progress in the development of Southern Ocean food web models to meet three different approaches to informing policy makers and supporting decision making—testing outcomes of future scenarios, support of decision-making, and assessment of risks.
Table 4 shows that considerable effort and resources remain to be invested in developing ecosystem and food web models that satisfactorily represent the whole of the ecosystem in a manner suitable for policy makers. A major advance since Murphy et al. (2012) has been better characterisation of food webs around the Southern Ocean. However, three critical challenges remain to be addressed by the scientific community and policy makers. First, end-to-end ecosystem models or food web models coupled to Earth System model outputs are urgently needed for developing future scenarios under climate change, and to frame the ecosystem issues for policy makers to address in the coming decades. A second challenge, and no less important, is to establish sustained observations of food webs in more than just the Antarctic Peninsula and Scotia Arc (Newman et al., 2019). Without these observations, it will be very difficult to detect changes in food webs, validate ecosystem models of the region and support management in areas other than the south Atlantic and Antarctic Peninsula. The third challenge is to establish processes and procedures for validating ecosystem and food web models and for verifying that their behaviours are “right for the right reasons,” a process that still challenges the Earth System modelling community (Russell et al., 2018). The importance of validation and verification cannot be overstated. Russell et al. (2018) articulates very well that fitting a model to data, while centrally important to the validation process, does not guarantee that the model will provide satisfactory projections into the future. Models need to also have behaviours of state variables as well as spatial and temporal correlations amongst variables that make sense when compared to known broader patterns, understanding, and theory. With appropriate validation and weighting of each model’s utility for the task, ensembles of different models can help with understanding the impacts of model uncertainty on the conclusion about different scenarios.
Managers rely on models, heuristic or formal, to make decisions. Ecosystem models enable conversations about what processes are important, how species interact and where the greatest risks and vulnerability to ecosystem structure and function may lie. They also allow scientists and policy makers to frame the possible futures that we face. Earth System models point to the vulnerability and diminution of polar systems. At present, we can foresee how species will be affected by physical changes and what the consequent pathways of effects might be. In the absence of ecosystem models, we are unable to foresee what the system-level response will be for Southern Ocean ecosystems once climate recovery begins.
Author Contributions
SM led the development and writing of the manuscript, figures, and tables. SM, JM-T, RT, and AC developed the approach. All authors contributed text to the manuscript and input to the figures and tables.
Funding
SM was supported by the Antarctic Climate & Ecosystems Cooperative Research Centre and PEW Charitable Trusts. MP was supported by MBIE (C01X1710, ANTA180).
Conflict of Interest
The authors declare that the research was conducted in the absence of any commercial or financial relationships that could be construed as a potential conflict of interest.
Publisher’s Note
All claims expressed in this article are solely those of the authors and do not necessarily represent those of their affiliated organizations, or those of the publisher, the editors and the reviewers. Any product that may be evaluated in this article, or claim that may be made by its manufacturer, is not guaranteed or endorsed by the publisher.
Acknowledgments
This work is a contribution to the first Marine Ecosystem Assessment for the Southern Ocean (MEASO) of IMBeR’s program ICED. We thank the MEASO Support Group and Steering Committee for assisting with figures, coordination and editing of the text. Thank you to Ben Raymond and Laura Blamey for reviewing a previous version of this paper, and to the two reviewers for useful comments that greatly improved the manuscript. Additional thanks to WWF, PEW, and COLTO who supported the MEASO project and SM whilst writing this manuscript.
References
Ainsworth, C. H., and Walters, C. J. (2015). Ten common mistakes made in Ecopath with Ecosim modelling. Ecol. Model. 308, 14–17. doi: 10.1016/j.ecolmodel.2015.03.019
Alvain, S., and Ovidio, F. D. (2014). “Chapter 6.2. Phytoplankton diversity in the Southern Ocean: a satellite view,” in Biogeographic Atlas of the Southern Ocean, eds C. De Broyer, P. Koubbi, H. J. Griffiths, B. Raymond, C. D. Udekem d’Acoz, et al. (Cambridge: Scientific Committee on Antarctic Research), 260–265.
Angel, M. V., and Blachowiak-Samolyk, K. (2014). “Chapter 6.7. Halocyprid Ostracods of the Southern Ocean,” in Biogeographic Atlas of the Southern Ocean, eds C. De Broyer, P. Koubbi, H. J. Griffiths, B. Raymond, C. D. Udekem d’Acoz, et al. (Cambridge: Scientific Committee on Antarctic Research), 297–302.
Atkinson, A., Nicol, S., Kawaguchi, S., Pakhomov, E., Quetin, L., Ross, R., et al. (2012a). Fitting Euphausia superba into Southern Ocean food-web models: a review of data sources and their limitations. CCAMLR Sci. 19, 219–245.
Atkinson, A., Siegel, V., Pakhomov, E., and Rothery, P. (2004). Long-term decline in krill stock and increase in salps within the Southern Ocean. Nature 432, 100–103. doi: 10.1038/nature0299
Atkinson, A., Ward, P., Pakhomov, E., and Hosie, G. (2012b). An overview of Southern Ocean zooplankton data: abundance, biomass, feeding and functional relationships. CCAMLR Sci. 19, 171–218.
Ballerini, T., Hofmann, E. E., Ainley, D. G., Daly, K., Marrari, M., Ribic, C., et al. (2014). Productivity and linkages of the food web of the southern region of the western Antarctic Peninsula continental shelf. Prog. Oceanogr. 122, 10–29. doi: 10.1016/j.pocean.2013.11.007
Barnes, D. K. A., and Sands, C. J. (2017). Functional group diversity is key to Southern Ocean benthic carbon pathways. PLoS One 12:e0179735. doi: 10.1371/journal.pone.0179735
Bates, M. L., Nash, S. M. B., Hawker, D. W., Norbury, J., Stark, J. S., and Cropp, R. A. (2015). Construction of a trophically complex near-shore Antarctic food web model using the Conservative Normal framework with structural coexistence. J. Mar. Syst. 145, 1–14. doi: 10.1016/j.jmarsys.2014.12.002
Benson, A. R., Gleich, D. F., and Leskovec, J. (2016). Higher-order organization of complex networks. Science 353, 163–166. doi: 10.1126/science.aad9029
Bestley, S., Ropert-Coudert, Y., Nash, S. B., Brooks, C. M., Cotté, C., Dewar, M., et al. (2020). Marine Ecosystem Assessment for the Southern Ocean: birds and marine mammals in a changing climate. Front. Ecol. Evol. 8:566936. doi: 10.3389/fevo.2020.566936
Blanchard, J. L., Heneghan, R. F., Everett, J. D., Trebilco, R., and Richardson, A. J. (2017). From bacteria to whales: using functional size spectra to model marine ecosystems. Trends Ecol. Evol. 32, 174–186. doi: 10.1016/j.tree.2016.12.003
Blanchard, J. L., Law, R., Castle, M. D., and Jennings, S. (2011). Coupled energy pathways and the resilience of size-structured food webs. Theor. Ecol. 4, 289–300. doi: 10.1007/s12080-010-0078-9
Borboroglu, P. G., and Boersma, P. D. (2015). Penguins: natural history and conservation. Seattle, London: University of Washington Press, 328.
Brasier, M. J., Barnes, D., Bax, N., Brandt, A., Christianson, A. B., Constable, A. J., et al. (2021). Responses of Southern Ocean seafloor habitats and communities to global and local drivers of change. Front. Mar. Sci. 8:622721. doi: 10.3389/fmars.2021.622721
Brose, U., Archambault, P., Barnes, A. D., Bersier, L. F., Boy, T., Canning-Clode, J., et al. (2019). Predator traits determine food-web architecture across ecosystems. Nat. Ecol. Evol. 3, 919–927. doi: 10.1038/s41559-019-0899-x
Caccavo, J. A., Christiansen, H., Constable, A. J., Ghigliotti, L., Trebilco, R., Brooks, C. M., et al. (2021). Productivity and change in fish and squid in the Southern Ocean. Front. Ecol. Evol. 9:624918. doi: 10.3389/fevo.2021.624918
Carscallen, M., and Romanuk, T. (2012). Structure and robustness to species loss in Arctic and Antarctic ice-shelf meta-ecosystem webs. Ecol. Model. 245, 208–218. doi: 10.1016/j.ecolmodel.2012.03.027
Cavan, E. L., Belcher, A., Atkinson, A., Hill, S. L., Kawaguchi, S., McCormack, S., et al. (2019). The importance of Antarctic krill in biogeochemical cycles. Nat. Commun. 10:4742. doi: 10.1038/s41467-019-12668-7
Cavanagh, R. D., Melbourne-Thomas, J., Grant, S. M., Barnes, D. K. A., Hughes, K. A., Halfter, S., et al. (2021). Corrigendum: future risk for southern ocean ecosystem services under climate change. Front. Mar. Sci. 8:707934. doi: 10.3389/fmars.2021.707934
Cavanagh, R. D., Murphy, E. J., Bracegirdle, T. J., Turner, J., Knowland, C. A., Corney, S. P., et al. (2017). A synergistic approach for evaluating climate model output for ecological applications. Front. Mar. Sci. 4:308. doi: 10.3389/fmars.2017.00308
Christensen, V., and Walters, C. J. (2004). Ecopath with Ecosim: methods, capabilities and limitations. Ecol. Model. 172, 109–139. doi: 10.1016/jecolmodel.2003.09.003
Christensen, V., Coll, M., Steenbeek, J., Buszowski, J., Chagaris, D., and Walters, C. J. (2014). Representing variable habitat quality in a spatial food web model. Ecosystems 17, 1397–1412. doi: 10.1007/s10021-014-9803-3
Christensen, V., Walters, C. J., Pauly, D., and Forrest, R. (2008). Ecopath with Ecosim Version 6: User Guide. Vancouver, BC: Fisheries Centre; University of British Columbia.
Clarke, A. (1985). “Energy Flow in the Southern Ocean Food Web,” in Antarctic Nutrient Cycles and Food Webs, eds W. R. Siegfried, P. R. Condy, and R. M. Laws (Berlin, Heidelberg: Springer Berlin Heidelberg), 573–580. doi: 10.1007/978-3-642-82275-9_78
Constable, A. J. (2001). The ecosystem approach to managing fisheries: achieving conservation objectives for predators of fished species. CCAMLR Science. 8, 37–64.
Constable, A. J. (2002). CCAMLR ecosystem monitoring and management: future work. CCAMLR Sci. 9, 233–253.
Constable, A. J. (2005). A possible framework in which to consider plausible models of the Antarctic marine ecosystem for evaluating krill management procedures. CCAMLR Sci. 12, 99–117.
Constable, A. J. (2011). Lessons from CCAMLR on the implementation of the ecosystem approach to managing fisheries. Fish Fish. 12, 138–151. doi: 10.1111/j.1467-2979.2011.00410.x
Constable, A. J., Costa, D. P., Schofield, O., Newman, L., Urban, E. R. Jr., Fulton, E. A., et al. (2016). Developing priority variables (“ecosystem Essential Ocean Variables” – eEOVs) for observing dynamics and change in Southern Ocean ecosystems. J. Mar. Syst. 161, 26–41. doi: 10.1016/j.jmarsys.2016.05.003
Constable, A. J., Melbourne-Thomas, J., Corney, S. P., Arrigo, K. R., Barbraud, C., Barnes, D. K. A., et al. (2014). Climate change and Southern Ocean ecosystems I: how changes in physical habitats directly affect marine biota. Glob. Chang. Biol. 20, 3004–3025. doi: 10.1111/gcb.12623
Constable, A. J., Melbourne-Thomas, J., Trebilco, R., Press, A. J., and Haward, M. (2017). ACE CRC position analysis: managing change in Southern Ocean ecosystems. Hobart, Australia: Antarctic Climate and Ecosystems Cooperative Research Centre.
Cordone, G., Marine, T. I., Salinas, V., Doyle, S. R., Saravia, L. A., and Momo, F. R. (2018). Effects of macroalgae loss in an Antarctic marine food web: applying extinction thresholds to food web studies. PeerJ. 6:e5531. doi: 10.7717/peerj.5531
Cordone, G., Salinas, V., Marina, T. I., Doyle, S. R., Pasotti, F., Saravia, L. A., et al. (2020). Green vs brown food web: effects of habitat type on multidimensional stability proxies for a highly-resolved Antarctic food web. Food Webs. 25:e00166. doi: 10.1016/j.fooweb.2020.e00166
Cresswell, K. A., Wiedenmann, J., and Mangel, M. (2008). Can macaroni penguins keep up with climate- and fishing-induced changes in krill? Polar Biol. 31, 641–649. doi: 10.1007/s00300-007-0401-0
Cuzin-Roudy, J., Irisson, J.-O., Penot, F., Kawaguchi, S., and Vallet, C. (2014). “Chapter 6.9. Southern Ocean Euphausiids,” in Biogeographic Atlas of the Southern Ocean, eds C. De Broyer, P. Koubbi, H. J. Griffiths, B. Raymond, C. D. Udekem d’Acoz, et al. (Cambridge: Scientific Committee on Antarctic Research), 309–320.
Dahood, A., de Mutsert, K., and Watters, G. M. (2020). Evaluating Antarctic marine protected area scenarios using a dynamic food web model. Biol. Conserv. 251:108766. doi: 10.1016/j.biocon.2020.108766
Dahood, A., Watters, G. M., and de Mutsert, K. (2019). Using sea-ice to calibrate a dynamic trophic model for the Western Antarctic Peninsula. PLoS One 14:e0214814. doi: 10.1371/journal.pone.0214814
de Santana, C. N., Rozenfeld, A. F., Marquet, P. A., and Duarte, C. M. (2013). Topological properties of polar food webs. Mar. Ecol. Prog. Ser. 474, 15–26. doi: 10.3354/meps10073
Delmas, E., Besson, M., Brice, M. H., Burkle, L. A., Dalla Riva, G. V., Fortin, M. J., et al. (2019). Analysing ecological networks of species interactions. Biol. Rev. 94, 16–36. doi: 10.1111/brv.12433
Deppeler, S. L., and Davidson, A. T. (2017). Southern ocean phytoplankton in a changing climate. Front. Mar. Sci. 4:40. doi: 10.3389/fmars.2017.00040
Ducklow, H. W., Doney, S. C., and Sailley, S. F. (2015). Ecological controls on biogeochemical fluxes in the western Antarctic Peninsula studied with an inverse foodweb model. Adv. Polar Sci. 26, 122–139. doi: 10.13679/j.advps.2015.2.00122
Duhamel, G., Hulley, P.-A., Causse, R., Koubbi, P., Vacchi, M., Pruvost, P., et al. (2014). “Chapter 7. Biogeographic patterns of fish,” in Biogeographic Atlas of the Southern Ocean, eds C. De Broyer, P. Koubbi, H. J. Griffiths, B. Raymond, C. D. Udekem d’Acoz, et al. (Cambridge: Scientific Committee on Antarctic Research), 328–362.
Essington, T. E., and Plagányi, E. E. (2014). Pitfalls and guidelines for “recycling” models for ecosystem-based fisheries management: evaluating model suitability for forage fish fisheries. ICES J. Mar. Sci. 71, 118–127. doi: 10.1093/icesjms/fst047
Eyring, V., Mishra, V., Griffith, G. P., Chen, L., Keenan, T., Turetsky, M. R., et al. (2021). Reflections and projections on a decade of climate science. Nat. Clim. Chang. 11, 279–285. doi: 10.1038/s41558-021-01020-x
Fogarty, M. J., Gamble, R., and Perretti, C. T. (2016). Dynamic complexity in exploited marine ecosystems. Front. Ecol. Evol. 4:68. doi: 10.3389/fevo.2016.00068
Freer, J. J., Tarling, G. A., Collins, M. A., Partridge, J. C., and Genner, M. J. (2019). Predicting future distributions of lanternfish, a significant ecological resource within the Southern Ocean. Divers. Distrib. 25, 1259–1272. doi: 10.1111/ddi.12934
Fulton, E. A., and Link, J. S. (2014). “Modelling approaches for marine ecosystem-based management,” in The sea: marine ecosystem-based management, Vol. 16, eds M. J. Fogarty and J. J. McCarthy (Cambridge: Harvard University Press), 121–170.
Fulton, E. A., Blanchard, J. L., Melbourne-Thomas, J., Plagányi, E. E., and Tulloch, V. J. D. (2019). Where the ecological gaps remain, a modelers’ perspective. Front. Ecol. Evol. 7:424. doi: 10.3389/fevo.2019.00424
Fulton, E. A., Boschetti, F., Sporcic, M., Jones, T., Little, R., Dambacher, J. M., et al. (2015). A multi-model approach to engaging stakeholder and modellers in complex environmental problems. Environ. Sci. Policy 48, 44–56. doi: 10.1016/j.envsci.2014.12.006
Gauzens, B., Barnes, A., Giling, D. P., Hines, J., Jochum, M., Lefcheck, J. S., et al. (2018). fluxweb: an R package to easily estimate energy fluxes in food webs. Methods Ecol. Evol. 10, 270–279. doi: 10.1111/2041-210X.13109
Geary, W. L., Bode, M., Doherty, T. S., Fulton, E. A., Nimmo, D. G., Tulloch, A. I. T., et al. (2020). A guide to ecosystem models and their environmental applications. Nat. Ecol. Evol. 4, 1459–1471. doi: 10.1038/s41559-020-01298-8
Goedegebuure, M., Melbourne-Thomas, J., Corney, S. P., Hindell, M. A., and Constable, A. J. (2017). Beyond blue fish: the case for more detailed representations of top predators in marine ecosystem models. Ecol. Model. 359, 182–192. doi: 10.1016/j.ecolmodel.2017.04.004
Goedegebuure, M., Melbourne-Thomas, J., Corney, S. P., McMahon, C. R., and Hindell, M. A. (2018). Modelling southern elephant seals Mirounga leonine using an individual-based model coupled with a dynamic energy budget. PLoS One 13:e0194950. doi: 10.1371/journal.pone.0194950
Grant, S. M., Hill, S. L., Trathan, P. N., and Murphy, E. J. (2013). Ecosystem services of the Southern Ocean: trade-offs in decision-making. Antarct. Sci. 25, 603–617. doi: 10.1017/S0954102013000308
Grant, S. M., Waller, C. L., Morley, S. A., Barnes, D. K. A., Brasier, M. J., Double, M. C., et al. (2021). Local drivers of change in southern ocean ecosystems: human activities and policy implications. Front. Ecol. Evol. 9:624518. doi: 10.3389/fevo.2021.624518
Green, D. B., Bestley, S., Corney, S. P., Trebilco, R., Lehodey, P., and Hindell, M. A. (2021). Modeling Antarctic krill circumpolar spawning habitat quality to identify regions with potential to support high larval production. Geophys. Res. Lett. 48, 1–11. doi: 10.1029/2020GL091206
Green, D. B., Bestley, S., Trebilco, R., Corney, S. P., Lehodey, P., McMahon, C. R., et al. (2020). Modelled mid-trophic pelagic prey fields improve understanding of marine predator foraging behaviour. Ecography 43, 1014–1026. doi: 10.1111/ecog.04939
Griffith, G. P. (2020). Closing the gap between causality, prediction, emergence and applied marine management. ICES J. Mar. Sci. 77, 1456–1462. doi: 10.1093/icesjms/fsaa087
Griffith, G. P., and Fulton, E. A. (2014). New approaches to simulating the complex interaction effects of multiple human impacts on the marine environment. ICES J. Mar. Sci. 71, 764–774. doi: 10.1093/icesjms/fst196
Griffith, G. P., Hop, H., Vihtakari, M., Wold, A., Kalhagen, K., and Gabrielsen, G. W. (2019). Ecological resilience of Arctic marine food webs to climate change. Nat. Clim. Change 9, 868–872. doi: 10.1038/s41558-019-0601-y
Griffith, G. P., Strutton, P. G., Semmens, J. M., and Fulton, E. A. (2018). Identifying important species that amplify or mitigate the interactive effects of human impacts on marine food webs. Conserv. Biol. 33, 403–412. doi: 10.1111/cobi.13202
Groeneveld, J., Berger, U., Henschke, N., Pakhomov, E. A., Reiss, C. S., and Meyer, B. (2020). Blooms of a key grazer in the Southern Ocean – an individual-based model of Salpa thompsoni. Prog. Oceanog. 185:102339. doi: 10.1016/j.pocean.2020.102339
Gurney, L. J., Pakhomov, E. A., and Christensen, V. (2014). An ecosystem model of the Prince Edward Island Archipelago. Ecol. Model. 294, 117–136. doi: 10.1016/j.ecolmodel.2014.09.008
Hagstrom, G. I., and Levin, S. A. (2017). Managing marine ecosystems as complex adaptive systems: Emergent patterns, critical transitions, and public goods. Ecosystems 20, 458–476. doi: 10.1101/056838
Henley, S. F., Cavan, E. L., Fawcett, S. E., Kerr, R., Monteiro, T., Sherrell, R. M., et al. (2020). Changing biogeochemistry of the Southern Ocean and its ecosystem implications. Front. Mar. Sci. 7:581. doi: 10.3389/fmars.2020.00581
Henschke, N., Pakhomov, E. A., Groeneveld, J., and Meyer, B. (2018). Modelling the life cycle of Salpa thompsoni. Ecol. Model. 387, 17–26. doi: 10.1016/j.ecolmodel.2018.08.017
Heymans, J. J., Coll, M., Link, J. S., Mackinson, S., Steenbeek, J., Walters, C., et al. (2016). Best practice in Ecopath with Ecosim food-web models for ecosystem-based management. Ecol. Model 331, 173–184. doi: 10.1016/j.ecolmodel.2015.12.007
Hill, S. L., Keeble, K., Atkinson, A., and Murphy, E. J. (2012). A foodweb model to explore uncertainties in the South Georgia shelf pelagic ecosystem. Deep Sea Res. Part II Top. Stud. Oceanogr. 59-60, 237–252. doi: 10.1016/j.dsr2.2011.09.001
Hill, S. L., Murphy, E. J., Reid, K., Trathan, P. N., and Constable, A. J. (2006). Modelling southern ocean ecosystems: krill, the food-web, and the impacts of harvesting. Biol. Rev. 81, 581–608. doi: 10.1017/S1464793106007123
Hill, S. L., Phillips, T., and Atkinson, A. (2013). Potential climate change effects on the habitat of Antarctic krill in the Weddell quadrant of the Southern Ocean. PLoS One 8:e72246. doi: 10.1371/journal.pone.0072246
Hill, S. L., Pinkerton, M. H., Ballerini, T., Cavan, E. L., Gurney, L. J., Martins, I., et al. (2021). Robust model-based indicators of regional differences in food-web structure in the Southern Ocean. J. Mar. Syst. 220:103556. doi: 10.1016/j.jmarsys.2021.103556
Hill, S., and Cannon, M. (2013). A potential feedback approach to ecosystem-based management: model predictive control of the Antarctic krill fishery. CCAMLR Sci. 20, 119–137.
Hill, S., and Matthews, J. (2013). The sensitivity of multiple output statistics to input parameters in a krill–predator–fishery ecosystem dynamics model. CCAMLR Sci. 20, 97–118.
Hindell, M. A., Reisinger, R. R., Ropert-Coudert, Y., Hückstädt, L. A., Trathan, P. N., Bornemann, H., et al. (2020). Tracking of marine predators to protect Southern Ocean ecosystems. Nature 58, 87–92. doi: 10.1038/s41586-020-2126-y
Holsman, K. K., Hazen, E. L., Haynie, A., Gourguet, S., Hollowed, A., Bograd, S. J., et al. (2019). Towards climate resiliency in fisheries management. ICES J. Mar. Sci. 76, 1368–1378. doi: 10.1093/icesjms/fsz031
Hoover, C., Pitcher, T., Pakhomov, E., and Wabnitz, C. (2012). “The Antarctic Peninsula marine ecosystem model and simulations 1978 - present,” in From the Tropics to the Poles: ecosystem models of Hudson Bay, Kaloko-Honokohau, Hawai’i, and the Antarctic Peninsula, eds C. C. C. Wabnitz and C. Hoover (Vancouver, British Columbia: Fisheries Centre, University of British Columbia), 108–188.
Hosie, G., Mormède, S., Kitchener, J., Takahashi, K., and Raymond, B. (2014). “Chapter 10.3. Near-surface zooplankton communities,” in Biogeographic Atlas of the Southern Ocean, eds C. De Broyer, P. Koubbi, H. J. Griffiths, B. Raymond, C. D. Udekem d’Acoz, et al. (Cambridge: Scientific Committee on Antarctic Research), 422–430.
Hückstädt, L. A., Piñones, A., Palacios, D. M., McDonald, B. I., Dinniman, M. S., Hofmann, E. E., et al. (2020). Projected shifts in the foraging habitat of crabeater seals along the Antarctic Peninsula. Nat. Clim. Change 10, 472–477. doi: 10.1038/s41558-020-0745-9
Iñiguez, G., Battiston, F., and Karsai, M. (2020). Bridging the gap between graphs and networks. Commun. Phys. 3:88. doi: 10.1038/s42005-020-0359-6
Isla, E., Palanques, A., Gili, J. M., Gerdes, D., and Arntz, W. (2006). Biochemical composition of marine sediment from the eastern Weddell Sea (Antarctica): High nutritive value in a high benthic-biomass environment. J. Mar. Syst. 60, 255–267. doi: 10.1016/j.jmarsys.2006.01.006
Jacob, U., Thierry, A., Brose, U., Arntz, W. E., Berg, S., Brey, T., et al. (2011). The role of body size in complex food webs: A cold case. Adv. Ecol. Res. 45, 181–223. doi: 10.1016/B978-0-12-386475-8.00005-8
Jenouvrier, S., Holland, M., Stroeve, J., Serreze, M., Barbraud, C., Weimerskirch, H., et al. (2014). Projected continent-wide declines of the emperor penguin under climate change. Nat. Clim. Change 41, 715–718. doi: 10.1038/NCLIIMATE2280
Johnson, B., Gomez, M., and Munch, S. B. (2020). Leveraging spatial information to forecast nonlinear ecological dynamics. Methods Ecol. Evol. 12, 266–279. doi: 10.1111/2041-210X.13511
Kéfi, S., Berlow, E. L., Wieters, E. A., Joppa, L. N., Wood, S. A., Brose, U., et al. (2015). Network structure beyond food webs: mapping non-trophic and trophic interactions on Chilean rocky shores. Ecology. 96, 291–303. doi: 10.1890/13-1424.1
Kelly, P., Corney, S. P., Melbourne-Thomas, J., Kawaguchi, S., Bestley, S., Fraser, A., et al. (2020). Salpa thompsoni in the Indian sector of the Southern Ocean: environmental drivers and life history parameters. Deep Sea Res. Part II Top. Stud. Oceanog. 174:104789. doi: 10.1016/j.dsr2.2020.104789
Klein, E. S., Hill, S. L., Hinke, J. T., Phillips, T., and Watters, G. M. (2018). Impacts of rising sea temperature on krill increase risks for predators in the Scotia Sea. PLoS One 13:e0191011. doi: 10.1371/journal.pone.0191011
Kock, K.-H., Barrera-Oro, E., Belchier, M., Collins, M. A., Duhamel, G., Hanchet, S., et al. (2012). The role of fish as predators of krill (Euphausia superba) and other pelagic resources in the Southern Ocean (submitted to the 2008 Joint CCAMLR-IWC Workshop). CCAMLR Sci. 19, 115–169.
Kouwenberg, J. H. M., Razouls, C., and Desreumaux, N. (2014). “Chapter 6.6. Southern Ocean Pelagic Copepods,” in Biogeographic Atlas of the Southern Ocean, eds C. De Broyer, P. Koubbi, H. J. Griffiths, B. Raymond, C. D. Udekem d’Acoz, et al. (Cambridge: Scientific Committee on Antarctic Research), 290–296.
Leaper, R., Bannister, J. L., Branch, T. A., Clapham, P. J., Donovan, G. P., Matsuoka, K., et al. (2008a). A Review of Abundance, Trends and Foraging Parameters of Baleen Whales in the Southern Hemisphere. Impington: International Whaling Commission.
Leaper, R., Best, P. B., Branch, T. A., Donovan, G. P., Murase, H., and Waerebeek, K. (2008b). Report of Review Group of Data Sources on Odontocetes in the Southern Ocean in Preparation for IWC/CCAMLR Workshop in August 2008. Impington: International Whaling Commission.
Libralato, S., Christensen, V., and Pauly, D. (2006). A method for identifying keystone species in food web models. Ecol. Model. 195, 153–171. doi: 10.1016/j.ecolmodel.2005.11.029
Lin, D., Walters, A., Bestley, S., Zhu, G., Chen, X., and Trebilco, R. (2020). Distribution of larval and juvenile pelagic squids in the Kerguelen Axis region: Oceanographic influence on size structure and evidence of spawning locations. Deep Sea Res. Part II Top. Stud. Oceanog. 174, 1–13. doi: 10.1016/j.dsr2.2019.07.003
Lindsay, D., Fuentes, V., Guerrero, E., and Grossmann, M. (2014). “Chapter 6.3. Southern Ocean Gelatinous Zooplankton,” in Biogeographic Atlas of the Southern Ocean, eds C. De Broyer, P. Koubbi, H. J. Griffiths, B. Raymond, C. D. Udekem d’Acoz, et al. (Cambridge: Scientific Committee on Antarctic Research), 266–275.
Maldonado, M. T., Surma, S., and Pakhomov, E. A. (2016). Southern Ocean biological iron cycling in the pre-whaling and present ecosystems. Philos. Trans. R. Soc. A 374:20150292. doi: 10.1098/rsta.2015.0292
Marina, T. I., Salinas, V., Cordone, G., Campana, G., Moreira, E., Deregibus, D., et al. (2018a). The food web of Potter Cove (Antarctica): complexity, structure and function. Estuar. Coast. Mar. Sci. 200, 141–151. doi: 10.1016/j.ecss.2017.10.015
Marina, T. I., Saravia, L. A., Cordone, G., Salinas, V., Doyle, S. R., and Momo, F. R. (2018b). Architecture of marine food webs: To be or not be a ‘small-world’. PLoS One 13:e0198217. doi: 10.1371/journal.pone.0198217
May, R. M. (1979). Ecological interactions in the Southern Ocean. Nature 277, 86–89. doi: 10.1038/277086a0
McCormack, S. A., Melbourne-Thomas, J., Trebilco, R., Blanchard, J. L., and Constable, A. (2019b). Alternative energy pathways in Southern Ocean food webs: Insights from a balance model of Prydz Bay, Antarctica. Deep Sea Res. Part II Top. Stud. Oceanogr. 174:104613. doi: 10.1016/j.dsr2.2019.07.001
McCormack, S. A., Melbourne-Thomas, J., Trebilco, R., Blanchard, J. L., Raymond, B., and Constable, A. (2020). Decades of dietary data demonstrate regional food web structures in the Southern Ocean. Ecol. Evol. 11, 227–241. doi: 10.1002/ece3.7017
McCormack, S. A., Trebilco, R., Melbourne-Thomas, J., Blanchard, J. L., Fulton, E. A., and Constable, A. J. (2019a). Using stable isotope data to advance marine food web modelling. Rev. Fish Biol. 29, 277–296. doi: 10.1007/s11160-019-09552-4
MEASO (2020). Marine Ecosystem Assessment for the Southern Ocean. Available Online at: https://www.frontiersin.org/research-topics/10606/marine-ecosystem-assessment-for-the-southern-ocean-meeting-the-challenge-for-conserving-earth-ecosys#articles [Accessed August 6, 2021]
Melbourne-Thomas, J., Constable, A. J., Fulton, E. A., Corney, S. P., Trebilco, R., Hobday, A. J., et al. (2017). Integrated modelling to support decision-making for marine social-ecological systems in Australia. ICES J. Mar. Sci. J. Conseil. 74, 2298–2308. doi: 10.1093/icesjms/fsx078
Melbourne-Thomas, J., Constable, A., Wotherspoon, S., and Raymond, R. (2013a). Testing paradigms of ecosystem change under climate warming in Antarctica. PLoS One 8:e55039. doi: 10.1371/journal.pone.0055093
Melbourne-Thomas, J., Wotherspoon, S., Corney, S., Molina-Balari, E., Marini, O., and Constable, A. (2013b). Optimal control and system limitation in a Southern Ocean ecosystem model. Deep Sea Res. Part II Top. Stud. Oceanogr. 114, 1–10. doi: 10.1016/j.dsr2.2013.02.017
Melbourne-Thomas, J., Wotherspoon, S., Raymond, B., and Constable, A. (2012). Comprehensive evaluation of model uncertainty in qualitative network analyses. Ecol. Monogr. 82, 505–519. doi: 10.1890/12.0207.1
Meyer, B., Atkinson, A., Bernard, K. S., Brierley, A. S., Driscoll, R., Hill, S. L., et al. (2020). Successful ecosystem-based management of Antarctic krill should address uncertainties in krill recruitment, behaviour and ecological adaptation. Commun. Earth Environ. 1:28. doi: 10.1038/s43247-020-00026-1
Montoya, J. M., and Solé, R. V. (2002). Small world patterns in food webs. J. Theor. Biol. 214, 405–412. doi: 10.1006/jtbi.2001.2460
Morley, S. A., Abele, D., Barnes, D. K. A., Cárdenas, C. A., Cotté, C., Gutt, J., et al. (2020). Global drivers on southern ocean ecosystems: changing physical environments and anthropogenic pressures in an Earth system. Front. Mar. Sci. 7:547188. doi: 10.3389/fmars.2020.547188
Mormede, S., Dunn, A., and Hanchet, S. M. (2014a). A stock assessment model of Antarctic toothfish (Dissostichus mawsoni) in the Ross Sea region incorporating multi-year mark-recapture data. CCAMLR Sci. 21, 39–62.
Mormede, S., Dunn, A., Hanchet, S., and Parker, S. (2014b). Spatially explicit population dynamics models for Antarctic toothfish in the Ross Sea region. CCAMLR Sci. 21, 19–37.
Moteki, M., Tsujimura, E., and Hulley, P.-A. (2017). Developmental intervals during the larval and juvenile stages of the Antarctic myctophid fish Electrona antarctica in relation to changes in feeding and swimming functions. Polar Sci. 12, 88–98. doi: 10.1016/j.polar.2017.02.006
Munch, S. B., Brias, A., Sugihara, G., and Rogers, T. L. (2020). Frequently asked questions about nonlinear dynamics and empirical dynamic modelling. ICES J. Mar. Sci. 77, 1463–1479. doi: 10.1093/icesjms/fsz209
Murphy, E. J., Cavanagh, R. D., Drinkwater, K. F., Grant, S. M., Heymans, J. J., Hofmann, E. E., et al. (2016). Understanding the structure and functioning of polar pelagic ecosystems to predict the impacts of change. Proc. Royal Soc. B Biol. Sci. 283:1844. doi: 10.1098/rspb.2016.1646
Murphy, E. J., Cavanagh, R. D., Hofmann, E. E., Hill, S. L., Constable, A. J., Costa, D. P., et al. (2012). Developing integrated models of Southern Ocean food webs: Including ecological complexity, accounting for uncertainty and the importance of scale. Prog. Oceanogr. 102, 74–92. doi: 10.1016/j.pocean.2012.03.006
Murphy, E. J., Hofmann, E. E., Watkins, J. L., Johnston, N. M., Piñones, A., Ballerini, T., et al. (2013). Comparison of the structure and function of Southern Ocean regional ecosystems: the Antarctic Peninsula and South Georgia. J. Mar. Syst. 109-110, 22–42. doi: 10.1016/j.jmarsys.2012.03.011
Murphy, E. J., Johnston, N. M., Hofmann, E. E., Phillips, R. A., Jackson, J. A., Constable, A. J., et al. (2021). Global connectivity of southern ocean ecosystems. Front. Ecol. Evol. 9:624451. doi: 10.3389/fevo.2021.624451
Murphy, E. J., Thorpe, S. E., Tarling, G. A., Watkins, J. L., Fielding, S., and Underwood, F. (2017). Restricted regions of enhanced growth of Antarctic krill in the circumpolar Southern Ocean. Nat. Sci. Rep. 7:6963. doi: 10.1038/s41598-017-07205-9
Murphy, E. J., Watkins, J. L., Trathan, P. N., Reid, K., Meredith, M. P., Thorpe, S. E., et al. (2007). Spatial and temporal operation of the Scotia Sea ecosystem: a review of large-scale links in a krill centred food web. Philos. Trans. R. Soc. Lond. B. Biol. Sci. 362, 113–148. doi: 10.1098/rstb.2006.1957
Newman, L., Heil, P., Trebilco, R., Katsumata, K., Constable, A., van Wijk, E., et al. (2019). Delivering sustained, coordinated, and integrated observations of the Southern Ocean for global impact. Front. Mar. Sci. 6:433. doi: 10.3389/fmars.2019.00433
Oke, P. R., Griffin, D. A., Schiller, A., Matear, R. J., Fiedler, R., Mansbridge, J., et al. (2013). Evaluation of a near-global eddy-resolving ocean model. Geosci. Model Dev. 6, 591–615. doi: 10.5194/gmd-6-591-2013
Pinkerton, M. H., Boyd, P. W., Deppeler, S., Hayward, A., Höfer, J., and Moreau, S. (2021). Evidence for the impact of climate change on primary producers in the Southern Ocean. Front. Ecol. Evol. 9:592027. doi: 10.3389/fevo.2021.592027
Pinkerton, M. H., Bradford-Grieve, J. M., and Hanchet, S. M. (2010). A balanced model of the food web of the Ross Sea, Antarctica. CCAMLR Sci. 17, 1–31.
Pinkerton, M. H., Decima, M., Kitchener, J., Takahashi, K., Robinson, K., Stewart, R., et al. (2020). Zooplankton in the Southern Ocean form the continuous plankton recorder: distributions and long-term change. Deep Sea Res. Part I Oceanogr. Res. Pap. 162:103303. doi: 10.1016/j.dsr.2020.103303
Pinkerton, M. H., Lyver, P. O. B., Stevens, D. W., Forman, J., Eisert, R., and Mormede, S. (2016). Increases in Adélie penguins in the Ross Sea: Could the fishery for Antarctic toothfish be responsible? Ecol. Model. 337, 262–271. doi: 10.1016/j.ecolmodel.2016.07.007
Piñones, A., Hofmann, E. E., Daly, K., Dinniman, M. S., and Klinck, J. M. (2013). Modeling the remote and local connectivity of Antarctic krill populations along the Western Antarctic Peninsula. Mar. Ecol. Prog. Ser. 481, 69–92. doi: 10.3354/meps10256
Plagányi, É, Punt, A., Hillary, R., Morello, E., Thébaud, O., Hutton, T., et al. (2014). Multi-species fisheries management and conservation: tactical applications using models of intermediate complexity. Fish Fish. 15, 1–22. doi: 10.1111/j.1467-2979.2012.00488.x
Plagányi, E. E. (2007). Models for an ecosystem approach to fisheries. FAO Fisheries Technical Paper no. 477. Rome: Food and Agriculture Organization of the United Nations.
Plagányi, E. E., and Butterworth, D. S. (2012). The Scotia Sea krill fishery and its possible impacts on dependent predators: modelling localized depletion of prey. Ecol. Appl. 22, 748–761. doi: 10.1890/11-0441.1
Plagányi, E. E., and Essington, T. E. (2014). When the SURFs up, forage fish are key. Fish. Res. 159, 68–74. doi: 10.1016/j.fishres.2014.05.011
Plagányi, E. E., Bell, J. D., Bustamante, R. H., Dambacher, J. M., Dennis, D. M., Dichmont, C. M., et al. (2011). Modelling climate-change effects on Australian and Pacific aquatic ecosystems: a review of analytical tools and management implications. Mar. Freshw. Res. 62, 1132–1147. doi: 10.1071/MF10279
Plagányi, ÉE., and Fulton, E. A. (2017). “The Future of Modeling to Support Conservation Decisions in the Anthropocene Ocean,” in Conservation for the Anthropocene Ocean: Interdisciplinary Science in Support of Nature and People, eds P. S. Levin and M. R. Poe (London: Academic Press Ltd Elsevier Science Ltd), 423–446. doi: 10.1016/b978-0-12-805375-1.00020-9
Poisot, T., Stouffer, D. B., and Gravel, D. (2015). Beyond species: why ecological interaction networks vary through space and time. Oikos 124, 243–251. doi: 10.1111/oik.01719
Poisot, T., Stouffer, D. B., and Kéfi, S. (2016). Describe, understand and predict: why do we need networks in ecology? Funct. Ecol. 30, 1878–1882. doi: 10.1111/1365-2435.12799
Post, A. L., Meijers, A. J. S., Fraser, A. D., Meiners, K. M., Ayers, J., Bindoff, N. L., et al. (2014). “Chapter 4. Environmental Setting,” in Biogeographic Atlas of the Southern Ocean, eds C. De Broyer, P. Koubbi, H. J. Griffiths, B. Raymond, C. D. Udekem d’Acoz, et al. (Cambridge: Scientific Committee on Antarctic Research), 46–64.
Queirós, J. P., Cherel, Y., Ceia, F. R., Hilário, A., Roberts, J., and Xavier, J. (2018). Ontogenic changes in habitat and trophic ecology in the Antarctic squid Kondakovia longimana derived from isotopic analysis on beaks. Polar Biol. 41, 2409–2421. doi: 10.1007/s00300-018-2376-4
Ratcliffe, N., and Trathan, P. (2011). A review of the diet and at-sea distribution of penguins breeding within the CCAMLR convention area. CCAMLR Sci. 19, 75–114.
Ratnarajah, L., Melbourne-Thomas, J., Marzloff, M. P., Lannuzel, D., Meiners, K., Chever, F., et al. (2015). A preliminary model of iron fertilisation by baleen whales and Antarctic krill in the southern ocean: Sensitivity of primary productivity estimates to parameter uncertainty. Ecol. Model. 320, 203–212. doi: 10.1016/j.ecolmodel.2015.10.007
Roberts, D., Hopcroft, R. R., and Hosie, G. W. (2014). “Chapter 6.4. Southern Ocean Pteropods,” in Biogeographic Atlas of the Southern Ocean, eds C. De Broyer, P. Koubbi, H. J. Griffiths, B. Raymond, C. D. Udekem d’Acoz, et al. (Cambridge: Scientific Committee on Antarctic Research), 276–283.
Roberts, L., Kutay, C., Melbourne-Thomas, J., Petrou, K., Benson, T. M., Fiore, D., et al. (2021). Enabling enduring evidence-based policy for the southern ocean through cultural arts practices. Front. Ecol. Evol. 9:616089. doi: 10.3389/fevo.2021.616089
Rodhouse, P. G. K., Griffiths, H. J., and Xavier, J. (2014). “Chapter 6.5. Southern Ocean squid,” in Biogeographic Atlas of the Southern Ocean, eds C. De Broyer, P. Koubbi, H. J. Griffiths, B. Raymond, C. D. Udekem d’Acoz, et al. (Cambridge: Scientific Committee on Antarctic Research), 284–289.
Rogers, A. D., Frinault, B. A. V., Barnes, D. K. A., Bindoff, N. L., Downie, R., Ducklow, H. W., et al. (2019). Antarctic futures: an assessment of climate-driven changes in ecosystem structure, function, and service provisioning in the Southern Ocean. Annu. Rev. Mar. Sci. 12, 87–120. doi: 10.1146/annurev-marine-010419-011028
Ropert-Coudert, Y., Hindell, M. A., Phillips, R., Charassin, J. B., Trudelle, L., and Raymond, B. (2014). “Chapter 8. Biogeographic patterns of birds and mammals,” in Biogeographic Atlas of the Southern Ocean, eds C. De Broyer, P. Koubbi, H. J. Griffiths, B. Raymond, C. D. Udekem d’Acoz, et al. (Cambridge: Scientific Committee on Antarctic Research), 364–387.
Rossi, L., Caputi, S. S., Calizza, E., Careddu, G., Oliverio, M., Schiaparelli, S., et al. (2019). Antarctic food web architecture under varying dynamics of sea ice cover. Sci. Rep. 9:12454. doi: 10.1038/s41598-019-48245-7
Runge, J., Nowack, P., Kretschmer, M., Flaxman, S., and Sejdinovic, D. (2019). Detecting and quantifying causal associations in large nonlinear time series datasets. Sci. Adv. 5:11. doi: 10.1126/sciadv.aau4996
Russell, J. L., Kamenkovich, I., Bitz, C., Ferrari, R., Gille, S. T., Goodman, P. J., et al. (2018). Metrics for the evaluation of the southern ocean in coupled climate models and earth system models. J. Geophys. Res. Oceans 123, 3120–3143. doi: 10.1002/2017jc013461
Sailley, S. F., Ducklow, H. W., Moeller, H. V., Fraser, W. R., Schofield, O., Steinberg, D., et al. (2013). Carbon fluxes and pelagic ecosystem dynamics near two western Antarctic Peninsula Adélie penguin colonies: an inverse model approach. Mar. Ecol. Prog. Ser. 492, 253–272. doi: 10.3354/meos10534
Saravia, L. A., Marina, T. I., Kristensen, N. P., De Troch, M., and Momo, F. R. (2021). Ecological network assembly: how the regional metaweb influences local food webs. bioRxiv [Preprint]. doi: 10.1101/340430
Saunders, R. A., Hill, S. L., Tarling, G. A., and Murphy, E. J. (2019). Myctophid fish (family Myctophidae) are central consumers in the food web of the Scotia Sea (Southern Ocean). Front. Mar. Sci. 6:530. doi: 10.3389/fmars.2019.00530
Saunders, R. A., Lourenco, S., Vieira, R. P., Collins, M. A., Assis, C. A., and Xavier, J. C. (2020). Age and growth of Brauer’s lanternfish Gymnoscopelus braueri and rhombic lanternfish Krefftichthys anderssoni (Family Myctophidae) in the Scotia Sea, Southern Ocean. J. Fish. Biol. 96, 364–377. doi: 10.1111/jfb.14206
Schaafsma, F. L., Cherel, Y., Flores, H., van Franeker, J. A., Lea, M.-A., Raymond, B., et al. (2018). Review: the energetic value of zooplankton and nekton species of the Southern Ocean. Mar. Biol. 165:129. doi: 10.1007/s00227-018-3386-z
Scientific Committee on Antarctic Research (2021). Southern Ocean Diet and Energetics Database. doi: 10.5281/zenodo.5072528
Scott, E., Serpetti, N., Steenbeek, J., and Heymans, J. J. (2016). A stepwise fitting procedure for automated fitting of Ecopath with Ecosim models. SoftwareX. 5, 25–30. doi: 10.1016/j.softx.2016.02.002
Southwell, C., Bengtson, J., Bester, M., Schytee Blix, A., Bornemann, H., Boveng, P., et al. (2012). A review of data on abundance, trends in abundance, habitat use and diet of ice-breeding seals in the Southern Ocean. CCAMLR Sci. 19, 49–74.
Steele, J. H., and Ruzicka, J. J. (2011). Constructing end-to-end models using ECOPATH data. J. Mar. Syst. 87, 227–238. doi: 10.1016/j.jmarsys.2011.04.005
Steenbeek, J., Buszowski, J., Christensen, V., Akoglu, E., Aydin, K., Ellis, N., et al. (2016). Ecopath with Ecosim as a model-building toolbox: source code capabilities, extensions, and variations. Ecol. Model. 319, 178–189. doi: 10.1016/j.ecolmodel.2015.06.031
Steenbeek, J., Corrales, X., Platts, M., and Coll, M. (2018). Ecosampler: a new approach to assessing parameter uncertainty in Ecopath with Ecosim. SoftwareX. 7, 198–204. doi: 10.1016/j.softx.2018.06.004
Subramaniam, R. C., Corney, S. P., Swadling, K. M., and Melbourne-Thomas, J. (2020a). Exploring ecosystem structure and function of the northern Kerguelen Plateau using a mass-balanced food web model. Deep Sea Res. Part II Top. Stud. Oceanogr. 174:104787. doi: 10.1016/j.dsr2.2020.104787
Subramaniam, R. C., Melbourne-Thomas, J., Corney, S. P., Alexander, K. A., Péron, C., Ziegler, P., et al. (2020b). Time-dynamic food web modelling to explore environmental drivers of ecosystem change on the Kerguelen Plateau. Front. Mar. Sci. 7:641. doi: 10.3389/fmars.2020.00641
Suprenand, P. M., and Ainsworth, C. H. (2017). Trophodynamic effects of climate change-induced alterations to primary production along the western Antarctic Peninsula. Mar. Ecol. Prog. Series. 569, 37–54. doi: 10.3354/meps12100
Surma, S., Pakhomov, E. A., and Pitcher, T. J. (2014). Effects of whaling on the structure of the Southern Ocean food web: insights on the “krill surplus” from ecosystem modelling. PLoS One 9:e114978. doi: 10.1371/journal.pone.0114978
Suweis, S., and D’Odorico, P. (2014). Early warning signs in social-ecological networks. PloS One. 9:e101851. doi: 10.1371/journal.pone.0101851
Swadling, K. M. (2014). “Chapter 6.10. Sea-ice Fauna,” in Biogeographic Atlas of the Southern Ocean, eds C. De Broyer, P. Koubbi, H. J. Griffiths, B. Raymond, C. D. Udekem d’Acoz, et al. (Cambridge: Scientific Committee on Antarctic Research), 321–325.
Thomson, R. B., Butterworth, D. S., Boyd, I. L., and Croxall, J. P. (2000). Modeling the consequences of Antarctic krill harvesting on Antarctic fur seals. Ecol. Appl. 10, 1806–1819. doi: 10.2307/2641240
Thorpe, S. E., Tarling, G. A., and Murphy, E. J. (2019). Circumpolar patterns in Antarctic krill larval recruitment: an environmentally driven model. Mar. Ecol. Prog. Ser. 613, 77–96. doi: 10.3354/meps12887
Tittensor, D., Eddy, T., Lotze, H., Galbraith, E., Cheung, W., Barange, M., et al. (2018). A protocol for the intercomparison of marine fishery and ecosystem models: Fish-MIP v1.0. Geosci. Model Dev. 11, 1421–1442. doi: 10.5194/gmd-11-1421-2018
Travers, M., Shin, Y. J., Jennings, S., and Cury, P. M. (2007). Towards end-to-end models for investigating the effects of climate and fishing in marine ecosystems. Prog. Oceanog. 75, 751–770. doi: 10.1016/j.pocean.2007.08.001
Treasure, A. M., Ruzhicka, J. J., Moloney, C. L., Gurney, L. J., and Ansorge, I. J. (2015). Land-sea interactions and consequences for Sub-Antarctic marine food webs. Ecosystems. 18, 752–768. doi: 10.1007/s10021-015-9860-2
Treasure, A. M., Ruzicka, J. J., Pakhomov, E. A., and Ansorge, I. J. (2019). Physical transport mechanisms driving Sub-Antarctic island marine ecosystems. Ecosystems. 22, 1069–1087. doi: 10.1007/s10021-018-0326-1
Trebilco, R., Melbourne-Thomas, J., and Constable, A. (2020). The policy relevance of Southern Ocean food web structure: implications of food web change for fisheries, conservation and carbon sequestration. Mar. Policy 115:103832. doi: 10.1016/j.marpol.2020.103832
Trites, A. W., and Pauly, D. (1998). Estimating mean body masses of marine mammals from maximum body lengths. Can. J. Zool. 76, 886–896. doi: 10.1139/cjz-76-5-886
Tulloch, V. J. D., Plagányi, ÉE., Brown, C., Richardson, A. J., and Matear, R. (2019). Future recovery of baleen whales is imperiled by climate change. Glob. Change Biol. 25, 1263–1281. doi: 10.1111/gcb.14573
Tulloch, V. J. D., Plagányi, ÉE., Matear, R., Brown, C. J., and Richardson, A. J. (2017). Ecosystem modelling to quantify the impact of historical whaling on Southern Hemisphere baleen whales. Fish. Fish. 19, 117–137. doi: 10.1111/faf.12241
Turner, J., Barrand, N. E., Bracegirdle, T. J., Convey, P., Hodgson, D. A., Jarvis, M., et al. (2014). Antarctic climate change and the environment: an update. Polar Rec. 50, 237–259. doi: 10.1017/S0032247413000296
Veytia, D., Corney, S., Meiners, K. M., Kawaguchi, S., Murphy, E. K., and Bestley, S. (2020). Circumpolar projections of Antarctic krill growth potential. Nat. Climate Change. 10, 568–575. doi: 10.1038/s41558-020-0758-4
Waluda, C. M., Collins, M. A., Black, A. D., Staniland, I. J., and Trathan, P. N. (2010). Linking predator and prey behaviour: contrasts between Antarctic fur seals and macaroni penguins at South Georgia. Mar. Biol. 157, 99–112. doi: 10.1007/s00227-009-1299-6
Ward, B. A., Wilson, J. D., Death, R. M., Monteiro, F. M., Yool, A., and Ridgwell, A. (2018). EcoGEnIE 1.0: plankton ecology in the cGEnIE Earth system model. Geosci. Model Dev. 11, 4241–4267. doi: 10.5194/gmd-11-4241-2018
Watters, G. M., Hill, S. L., Hinke, J. T., Matthews, J., and Reid, K. (2013). Decision-making for ecosystem-based management: evaluating options for a krill fishery with an ecosystem dynamics model. Ecol. Appl. 23, 710–725. doi: 10.1890/12-1371.1
Wiencke, C., Amsler, C. D., Clayton, M. N., Gooday, A. J., Rothe, N., Bowser, S. S., et al. (2014). “Part 5. Biogeographic patterns of benthos,” in Biogeographic Atlas of the Southern Ocean, eds C. De Broyer, P. Koubbi, H. J. Griffiths, B. Raymond, C. D. Udekem d’Acoz, et al. (Cambridge: Scientific Committee on Antarctic Research), 364–387.
Yletyinen, J. (2019). Arctic climate resilience. Nat. Clim. Chang. 9, 805–806. doi: 10.1038/s41558-019-0616-4
Yletyinen, J., Bodin, Ö, Weigel, B., Nordström, M. C., Bonsdorff, E., and Blenckner, T. (2016). Regime shifts in marine communities: a complex systems perspective on food web dynamics. Proc. Royal Soc. B Biol. Sci. 283:20152569. doi: 10.1098/rspb.2015.2569
Zeidler, W., and De Broyer, C. (2014). “Chapter 6.8. Amphipoda Hyperiidea,” in Biogeographic Atlas of the Southern Ocean, eds C. De Broyer, P. Koubbi, H. J. Griffiths, B. Raymond, C. D. Udekem d’Acoz, et al. (Cambridge: Scientific Committee on Antarctic Research), 303–308.
Zhu, G., Duan, M., Wei, L., Trebilco, R., Bestley, S., and Walters, A. (2020). Determination and precision of otolith growth zone estimates of Electrona antarctica in the Southern Kerguelen Plateau region in the Indian sector of the Southern Ocean. Deep Sea Res. Part II Top. Stud. Oceanogr. 174, 1–35. doi: 10.1016/j.dsr2.2020.104778
Keywords: ecosystem models, food web assessment, marine policy, Antarctic, ecosystem-based management
Citation: McCormack SA, Melbourne-Thomas J, Trebilco R, Griffith G, Hill SL, Hoover C, Johnston NM, Marina TI, Murphy EJ, Pakhomov EA, Pinkerton M, Plagányi É, Saravia LA, Subramaniam RC, Van de Putte AP and Constable AJ (2021) Southern Ocean Food Web Modelling: Progress, Prognoses, and Future Priorities for Research and Policy Makers. Front. Ecol. Evol. 9:624763. doi: 10.3389/fevo.2021.624763
Received: 01 November 2020; Accepted: 30 August 2021;
Published: 14 October 2021.
Edited by:
Orsolya Valkó, Centre for Ecological Research, Hungarian Academy of Science, HungaryReviewed by:
Mohammad Imam Hasan Reza, Centre for Environment and Sustainability, Presidency Education, BangladeshBodil Annikki Bluhm, Arctic University of Norway, Norway
Copyright © 2021 McCormack, Melbourne-Thomas, Trebilco, Griffith, Hill, Hoover, Johnston, Marina, Murphy, Pakhomov, Pinkerton, Plagányi, Saravia, Subramaniam, Van de Putte and Constable. This is an open-access article distributed under the terms of the Creative Commons Attribution License (CC BY). The use, distribution or reproduction in other forums is permitted, provided the original author(s) and the copyright owner(s) are credited and that the original publication in this journal is cited, in accordance with accepted academic practice. No use, distribution or reproduction is permitted which does not comply with these terms.
*Correspondence: Stacey A. McCormack, stacey.mccormack@utas.edu.au; orcid.org/0000-0002-8949-4793