- 1Department of Entomology, College of Plant Protection, Nanjing Agricultural University, Nanjing, China
- 2Department of Geosciences and Geography, University of Helsinki, Helsinki, Finland
Metacommunity theory provides a useful framework to describe the underlying factors (e.g., environmental and dispersal-related factors) influencing community structure. The strength of these factors may vary depending on the properties of the region studied (e.g., environmental heterogeneity and spatial location) and considered biological groups. Here, we examined environmental and dispersal-related controls of stream macroinvertebrates and diatoms in three regions in China using the distance-decay relationship analysis. We performed analyses for the whole stream network and separately for two stream network locations (headwater and downstream sites) to test the network position hypothesis (NPH), which states that the strength of environmental and dispersal-related controls varies between headwater and downstream communities. Community dissimilarities were significantly related to environmental distances, but not geographical distances. These results suggest that communities are structured strongly by environmental filtering, but weakly by dispersal-related factors such as dispersal limitation. More importantly, we found that, at the whole network scale, environmental control was the highest in the regions with highest environmental heterogeneity. Results further showed that the influence of environmental control was strong in both headwaters and downstream sites, whereas spatial control was generally weak in all sites. This suggests a lack of consistent support for the NPH in our studied stream networks. Moreover, we found that local-scale variables relative to basin-scale variables better explained community dissimilarities for diatoms than for macroinvertebrates. This indicates that diatoms and macroinvertebrates responded to environment at different scales. Collectively, these results suggest that the importance of drivers behind the metacommunity assembly varied among regions with different level of environmental heterogeneity and between organism groups, potentially indicating context dependency among stream systems and taxa.
Introduction
The assembly rules of biotic communities are among the leading concerns of community ecology. Metacommunity theory suggests that the assembly of local communities results from a combination of dispersal, environmental filtering, stochastic colonization and extinction events, and biological interactions (Leibold et al., 2004). Based on these assembly processes, Leibold et al. (2004) suggested four paradigms or archetypes of metacommunities: species sorting, mass effects, neutral model and patch dynamics. Recent studies suggest that metacommunities form a continuum structured by different assembly mechanisms varying in their relative importance, rather than a typological classification based on four accurately bordered archetypes (Winegardner et al., 2012; Brown et al., 2017; Leibold and Chase, 2017). The assembly processes within metacommunities might vary among different study systems and this variability may be related to the environmental and spatial characteristics of the study region (Heino et al., 2012, 2015a).
Environmental heterogeneity prevailing in the study region is typically one of the main factors affecting metacommunity assembly (but see Bini et al., 2014). For example, the importance of environmental filtering is expected to vary among regions that cover different levels of environmental heterogeneity (Leibold et al., 2004). Such an effect would be more likely to be found when studying metacommunities that show intermediate among-site dispersal and intermediate spatial extent (e.g., within a river basin, Heino et al., 2015b). This is because the “true” effect of environmental filtering can be masked by limited or excessive dispersal, which likely occur at large or small spatial scales, respectively (Ng et al., 2009; Heino et al., 2015c). However, empirical support for such an expectation (i.e., the importance of environmental filtering on community composition is expected to be greater within regions that have higher environmental heterogeneity) is relatively weak, particularly in stream ecosystems (Landeiro et al., 2012; Grönroos et al., 2013; Heino et al., 2015a).
Spatial location of a site may also affect metacommunity organization. In an influential study on stream macroinvertebrates, Brown and Swan (2010) predicted that headwater metacommunities are strongly determined by environmental filtering because headwaters are more isolated and more environmentally heterogeneous, whereas downstream metacommunities are potentially more influenced by mass effects due to a surplus of dispersal across well-connected downstream sites and the likely strong influence of movements from headwaters to downstream. These predictions were described as the network position hypothesis (NPH) by Schmera et al. (2018). However, recent studies found that the relative roles of environmental and dispersal-related factors on community composition are likely to depend on network level differences in environmental heterogeneity and connectivity configurations, rather than simply on headwater-downstream differences in environmental and connectivity variables (Eros, 2017; Schmera et al., 2018; Eros and Lowe, 2019; Henriques-Silva et al., 2019). For example, Henriques-Silva et al. (2019) found a lack of general support for the NPH predictions across multiple catchments and suggested that catchment properties (e.g., network connectivity) generated considerable context dependency in NPH predictions. These findings thus underline the need for testing the core predictions of the NPH in different regions.
Previous studies suggest that the relative roles of environmental and dispersal-related factors could also differ between biological groups with different traits such as body size (De Bie et al., 2012; Farjalla et al., 2012), dispersal ability (Grönroos et al., 2013), environmental tolerance and environmental optima. Diatoms are unicellular organisms and could be expected to be stronger dispersers than larger sized macroinvertebrates due to their small size and high abundance (Astorga et al., 2012; Heino et al., 2012). They may thus be better able to track environmental variation and show a stronger degree of environmental control than macroinvertebrates (Astorga et al., 2012). This is because diatoms can disperse passively via air and animal vectors (Kristiansen, 1996) and may overcome dispersal barriers more easily than macroinvertebrates restricted to dispersal via watercourses (Shurin et al., 2009; De Bie et al., 2012; Tonkin et al., 2017). However, some studies observed that the level of environmental control was surprisingly weaker for diatoms when compared with macroinvertebrates (Heino et al., 2012; Soininen, 2014). We note though that these studies considered the whole environment in only one single model without making distinction between different scales (e.g., local scale and basin scale). However, organisms with different biological traits may respond environmental variables at multiple scales differently (Johnson et al., 2007; Liu et al., 2016; Heino et al., 2017). For example, (Liu et al., 2016) found that catchment-level variables (e.g., land use diversity) explained a larger amount of variation in macroinvertebrate community composition than small-scale variables (e.g., substrates). In contrast, Pan et al. (1996) suggested that local variables (e.g., pH) played a more important role in structuring diatom communities than broad-scale variables (e.g., climatic variables). Recognition of such scale-related responses implies the need for simultaneous disentangling of multi-scale (e.g., local scale vs. basin scale) environmental effects on diatom and macroinvertebrate communities.
Here we aimed at addressing the role of environmental filtering and dispersal-related processes (e.g., dispersal limitation and mass effect) in structuring communities of stream diatoms and macroinvertebrates from the same set of sites at three intermediate-sized regions in China. The three regions differed in the level of environmental heterogeneity and were located spatially distant from each other. Stream assemblages across a set of sites within a region were defined here as a metacommunity. We performed independent analyses at different spatial hierarchies using data from the whole stream network and separately from headwater and downstream sites. We hypothesized that (H1) the effect of environmental filtering would be the highest in the region with highest environmental variation and (H2) the NPH predictions would receive inconsistent support across three regions and between two organismal groups. As (i) previous studies found a mixture of outcomes for the differences in environmental and dispersal-related controls between diatoms and macroinvertebrates, and (ii) only few studies have examined the differences in local-scale and basin-scale environmental controls between these two biological groups, we did not form a specific hypothesis regarding the differences in assembly processes between diatoms and macroinvertebrates.
Materials and Methods
Study Area
In this study, we used a data set containing three geographically distant (minimum distance between regions is ~2,000 km) regions: the Irtysh River (ITR) in Xijiang autonomous region, the middle section of Qiantang River (QTR) in Zhejiang Province, and the upper section of the Mekong River (MKR) in Xishuangbanna prefecture in China (Figure 1). These regions are ideal intermediate-sized systems (i.e., within a drainage basin) for our study with spatial extent ranging between 168 and 311 km. The study regions located in different climate zones: ITR, QTR and MKR in temperate arid climate, subtropical monsoon climate and tropical monsoon climate, respectively (Wang et al., 2012; Ding et al., 2017; Chen et al., 2019). They are also evidently different in human land use characteristics. We focus our investigation on streams ranging from first to fourth Strahler orders. We classified orders 1–2 as headwater (mean geographical distances between sites were 130, 72, and 85 km in the ITR, QTR, and MKR regions, respectively) sites and orders 3-4 as downstream (mean geographical distances between sites were 115, 80, and 79 km in the ITR, QTR, and MKR regions, respectively), sites (Henriques-Silva et al., 2019). We selected the same number of headwater (n = 15) and downstream (n = 15) sites within each region based on two restrictions: (1) we included sites where both macroinvertebrates and diatoms were collected, and (2) we included headwater sites that had the highest position in the river network, and downstream sites that had the lowest position in the river network to distinguish between different-sized streams as well as possible. For example, in the QTR region, we included all (n = 14) orders 1, and the order 2 with the narrowest wetted width. After the selection procedure, headwater sites were significantly (p < 0.0001) narrower and shallower than downstream sites within each region (Table 1). We conducted all analyses using only the selected sites in each region (Figure 1).
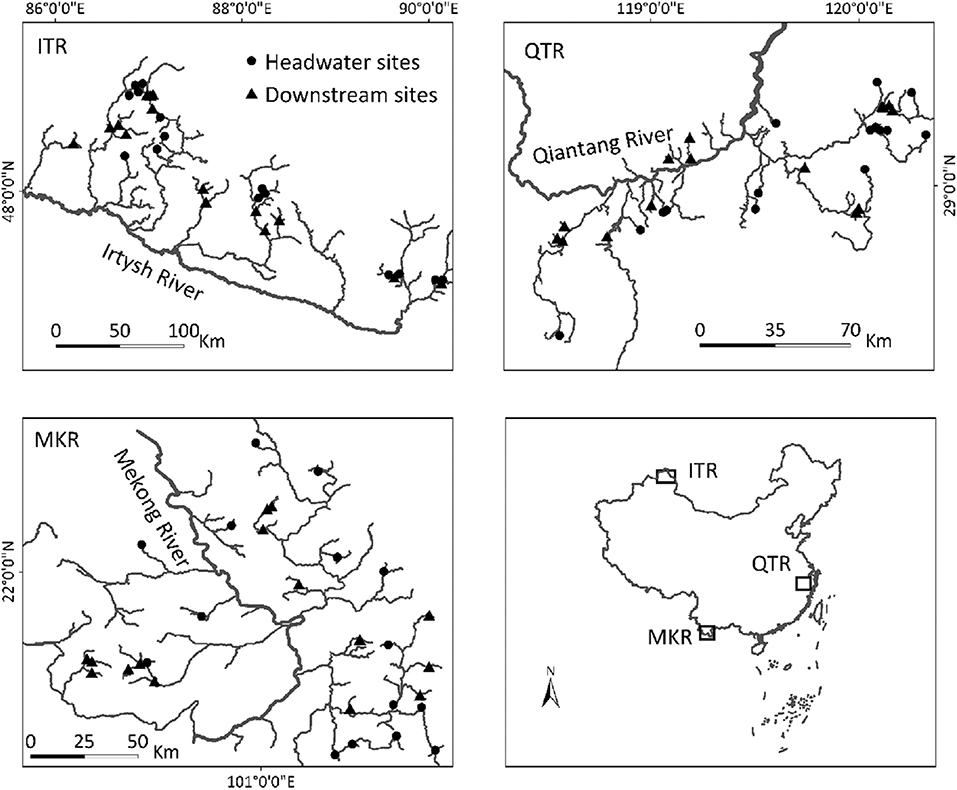
Figure 1. Geographic locations of sampling site in three regions in China: the Irtysh River (ITR) in Xijiang autonomous region, the middle section of Qiantang River (QTR) in Zhejiang Province, and the upper section of the Mekong River (MKR) in Xishuangbanna prefecture.

Table 1. Comparison of wetted width and water depth (mean ± standard deviation) between headwater and downstream sites in three regions in China: the Irtysh River (ITR) in Xijiang autonomous region, the middle section of Qiantang River (QTR) in Zhejiang Province, and the upper section of the Mekong River (MKR) in Xishuangbanna prefecture.
Environmental Variables
Chemical and physical data – We measured physical habitat and water chemical data from local scale for each site. These environmental data were measured simultaneously with the collection of macroinvertebrates and diatoms. We used a METTLER TOLEDO meter (model SG23, Mettler) to measure water temperature (WT), pH, total dissolved solids (TDS) and conductivity (Cond) in situ. We used a portable meter HI93752 (Hanna, Italy) to measure calcium (Ca2+) and magnesium (Mg2+) concentrations. We measured mean channel width and water depth on transects with equal distance interval across channel sections (Song et al., 2009). We also estimated the percentages of different substrate categories (i.e., % sands, % gravels, % cobbles and % boulders) (Wolman, 1954; Kondolf, 1997). Prior to the field measurements and biotic sampling, we collected one 500 ml water sample at each riffle and stored them in a portable refrigerator at <4°C. In the laboratory, we analyzed these samples for total nitrogen (TN), total phosphorus (TP), ammonia nitrogen (NH4-N), phosphate (PO4-P) contents and determined the potassium permanganate index (CODMn).
Land use and climate data- We followed Chen et al. (2015) to delineate the watershed boundaries for each site using the Multi-Watershed Delineation Tool and ArcGIS 9.3 software (Esri, Redlands, CA, USA) with 30-m resolution digital elevation models provided by the Chinese Academy of Sciences (http://www.cnic.cn/). We then included a digital land-use raster layer provided by GLOBELAND30 (http://www.globallandcover.com/) to estimate the percentages of three land-use types (i.e., % forest, % farmland, and % urban) within each watershed. We also used 19 bioclimatic variables available in the WorldClim database (http://www.worldclim.org/), at a resolution of 2.5' (~25 km2). These variables included data about annual trends (e.g., mean annual temperature and annual precipitation), seasonality (e.g., annual range in temperature and precipitation) and climatic extremes (e.g., temperature of the coldest and warmest month). In addition, because elevation is closely related to annual mean temperature (Pearson coefficients were 0.92, 0.89, and 0.84 in the ITR, QTR and MKR regions, respectively), we considered elevation as a climate variable. Elevation was documented with a Garmin eTrex GPS device. We considered human land use and natural climatic variables as “basin-scale” variables comparatively, relative to the “local-scale” variables. All environmental variables are provided in the Supplementary Material Data Sheet 1.
Biotic Sampling
Benthic macroinvertebrates and diatoms were collected simultaneously from a 100 m-long reach at each sampling site in ITR in March 2013, in QTR in April 2010 and in MKR in June 2013. We collected macroinvertebrates using a Surber-net (30 × 30 cm, 250 μm mesh size) from three riffles and two pools with a total of 0.45 m2 sampling area (Chen et al., 2019). All Surber net samples were combined into one composite sample and preserved in 10% buffered formalin. In the laboratory, macroinvertebrate individuals were sorted, counted and identified to the lowest practical taxonomic level, in most case to genus (>75% of taxa, Morse et al., 1994). Presence-absence data for macroinvertebrates are provided in the Supplementary Material Data Sheet 2.
We collected diatoms from nine transects at each site. Diatoms were scraped off from one coarse substrate particle from a defined area (10.17 cm2) with a toothbrush and an area delimiter (PVC tube) at each transect. We washed and combined the nine subsamples into a single composite sample, and added distilled water to a constant volume of 500 ml. We then extracted 50 ml out of the 500 ml to a specimen bottle for taxonomic analysis and preserved the sample by adding two ml of 10% formalin. In the laboratory, a total of 500 frustules per sample were identified and counted with a light microscope (Olympus BX41TF) at 1,000 × magnification. All diatom individuals were identified to the species level (Krammer and Lange-Bertalot, 1986, 1988, 1991a,b; Krammer, 2003). Presence-absence data for diatoms are provided in the Supplementary Material Data Sheet 3.
Spatial Distance Metrics
We calculated geographical distances using straight-line distances between each pair of sites in two-dimensional space. The geographical distances were calculated using the Analysis/Proximity/Point distance tool in ArcGIS 9.3 software. However, some studies have recommended for the use of other spatial distances, such as watercourse and topographic distances instead of geographical distances in stream ecosystem (e.g., Brown and Swan, 2010; Cañedo-Argüelles et al., 2015). But, as these different distances were highly correlated (e.g., Mantel coefficients of geographical distances on watercourse distances are 0.81, 0.92, and 0.78 in the ITR, QTR, and MKR regions, respectively), in each of three basins of our study, we opted to use simple horizontal geographical distance here.
Environmental Heterogeneity
We used an analysis of homogeneity of group dispersions (PERMDISP; Anderson, 2006) to test the possible differences in the degree of environmental heterogeneity among the three river regions. We calculated mean dispersions of environmental variables across streams within a region (mean distances of sites (streams) to group (a region) centroid) as a measure of environmental variability. Prior to PERMDISP analysis, we standardized each environmental variable to mean = 0 and standard deviation = 1 using the “scale” function in R. We tested for among-group differences in the distance from the observations to their group centroid using ANOVA F-statistic with 1,000 permutations and, where significant, tested for between-group differences with Tukey's HSD test (R function Tukey-HSD). Analyses were conducted for the environment as a whole and separately for each of three environmental groups (local environmental variables, land use and climate). We conducted the PERMDISP analysis using the “betadisper” function in vegan R package (Oksanen et al., 2013; R. Core Team, 2018).
Distance-Decay Relationships
We applied distance-decay relationships (DDRs) to reveal which drivers of metacommunity assembly are most associated with macroinvertebrates and diatoms in headwater and downstream sites and the whole network. If the NPH was clearly supported, we would find significant relationship between community dissimilarity and environmental distance in both headwater and downstream sites, but significant relationship between community dissimilarity and geographical distance only in downstream sites due to mass effect (Brown and Swan, 2010). We calculated the dissimilarity index using Sørensen coefficients (Legendre and Legendre, 2012) based on presence-absence data. We also calculated the dissimilarity index using Bray-Curtis coefficients based on abundance data (see results in Table S1). Although we also separated total dissimilarities into replacement and nestedness components (Baselga, 2010), we showed only results from using total dissimilarities as replacement components were the dominant ones in our data. We calculated the environmental Euclidean distance between each pair of sites using the best subset of explanatory variables (e.g., local environmental, climatic and land use variables) selected in BIO-ENV analysis (Clarke and Ainsworth, 1993; Astorga et al., 2012). The BIO-ENV analysis provides predictors that decrease random variation in environmental distance calculation and produce the highest correlation between environmental distance matrix and community dissimilarity matrix. We conducted the BIO-ENV analysis using the vegan package (Oksanen et al., 2013). We normalized all environmental variables (except pH) using log, square-root or centered log ratio (i.e., land-use and substrata data) transformations if necessary. Prior to BIO-ENV analysis, we removed variables that were highly correlated with other variables (Pearson r > 0.8). We then used the multiple regression on distance matrices (MRM) to test the relative importance of the environmental and geographical distances on biological community dissimilarities using ecodist package (Goslee and Urban, 2007). MRM analysis is a highly useful modeling approach in analyzing community variation because it can be used to investigate multiple relationships (e.g., linear or non-linear) between distance-based matrices (Lichstein, 2007). We used a backward selection procedure to progressively eliminate non-significant (P > 0.05) matrices from the models (see Tonkin et al., 2017 for a similar approach) and tested the significance of R2 values with 10,000 permutations. Because MRM analysis may not be efficient to account for the collinearity between environmental and geographical distances, we used the linear mixed effect model (LME) to address such a potential problem (Sarremejane et al., 2017; He et al., 2020). However, the determination coefficients () obtained from LME analysis (Table S2) and the standardized coefficients obtained from MRM analysis (Table 2) are highly similar. A more detailed description of the LME analysis is provided in the Supplementary Material Text S1. Finally, to evaluate the relative importance of local-scale vs. basin-scale environmental control, we calculated the ratio between local and basin (that is, local-scale environmental/basin-scale environmental effect ratio) rather than their absolute values. Here, basin equals the sum of land use and climate .
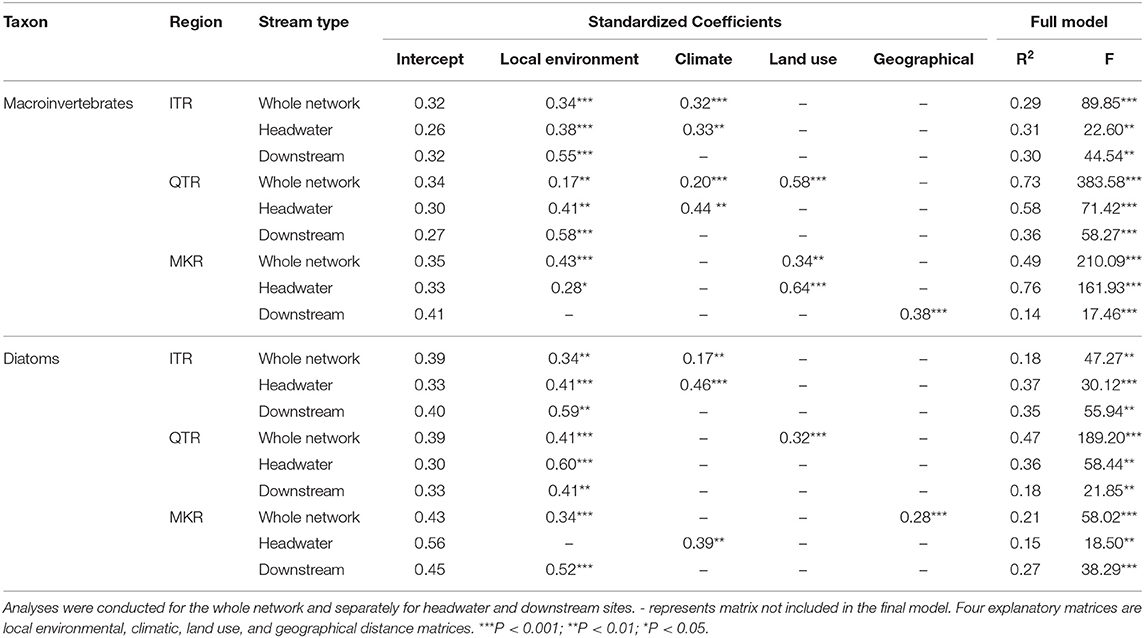
Table 2. Final modeling results of multiple regression of distance matrices (MRM) analyses between community dissimilarity and explanatory distance matrices (environmental and geographical distance) for stream macroinvertebrates and diatoms in the ITR, QTR, and MKR regions, based on 10,000 permutations.
Results
Taxa Richness
We identified a total of 219 macroinvertebrate and 206 diatom taxa from three regions (Supporting material data). Macroinvertebrate richness pattern across three basins differed notably from diatoms as ITR had the lowest total macroinvertebrate taxa richness of 60 with a mean of 17 (± 5, standard deviation) per site, whereas both QTR and MKR had two times greater total richness of 146 and 153 taxa with averages of 31 (± 21) and 40 (± 17) per site, respectively. For diatoms, the QTR had the lowest total richness of 78 with a mean of 15 (± 7) taxa per site, whereas both MKR and ITR had higher richness of 108 taxa with a mean of 19 (± 11) per site and 107 taxa with average 21 (± 10) per site, respectively.
Environmental Heterogeneity
Environmental heterogeneity varied significantly among the three regions based on PERMDISP analysis (the whole environment, local environment, land use and climate, Figure 2). The whole environmental heterogeneity, local environmental heterogeneity and land-cover heterogeneity were the highest in the QTR region, intermediate in the MKR region and the lowest in the ITR region (Figure 2). However, climatic heterogeneity was the highest in the ITR region, intermediate in the QTR region and the lowest in the MKR region (Figure 2).
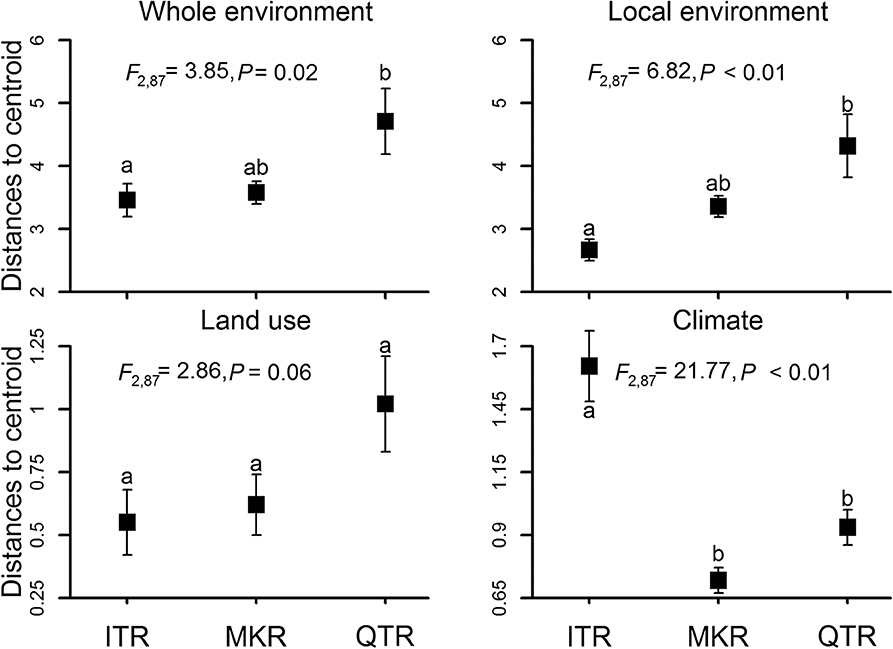
Figure 2. Average distances to centroid for sampled stream sites for the three study regions. Analyses were conducted for the environment as a whole and separately for each of three environmental groups (local environment, land use and climate) at the whole network level. Square symbols represent averages and error bars denote the standard error. Different letters represent significant differences between regions according to the Tukey's HSD test.
Distance-Decay Relationships
At the whole network level, the relationships between community dissimilarities and environmental distances were significant, but differed in their strength among regions (Table 2, Figure 3). The QTR had the highest values of the coefficients of determination (), followed by MKR and ITR (Figure 3). Geographical distance was important for diatoms only in the MKR region (Table 2). The environmental effects were generally higher in macroinvertebrates than diatoms (Figure 3), and significantly higher at the basin scale (ANOVA, F1, 6 = 14.636, P = 0.009, Table S3).
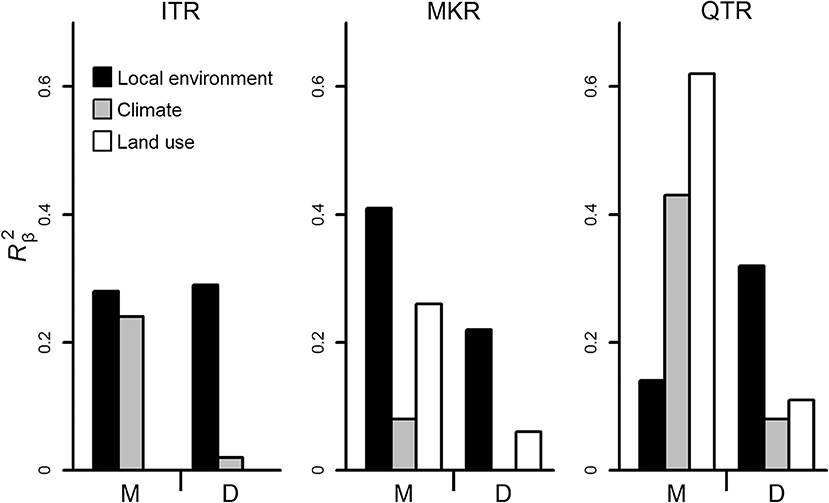
Figure 3. Strength of relationship between community dissimilarities and environmental (local environmental, climatic, and land use) distances calculated using the coefficient of determination (, y axis) for diatoms and macroinvertebrates in the three regions. Analyses were conducted at the whole network level. Abbreviations below axis are as follows. ITR, the Irtysh River in Xijiang autonomous region; QTR, the middle section of Qiantang River in Zhejiang Province; MKR, the upper section of the Mekong River in Xishuangbanna prefecture; M, Macroinvertebrates; D, Diatoms.
Environmental distances were related with community dissimilarities in both headwater and downstream sites (except the case of macroinvertebrates in MKR downstream sites, see Table 2), thus generally agreeing with NPH prediction about environmental control. However, significant relationship between geographical distance and community dissimilarity was found in downstream sites only in the MKR region and for macroinvertebrates. Thus, these results partly disagree with the general predictions of NPH as we did not find consistent support for spatial distance decay in downstream sites for both taxa groups and for all regions.
The Relative Role of Local-Scale Environmental and Basin-Scale Environmental Controls
The ratios between local-scale environmental effect and basin-scale environmental effect were consistently higher in diatom communities regardless of the region (9.7 in ITR, 1.7 in QTR, 3.8 in MKR) than in macroinvertebrate communities (1.1 in ITR, 0.1 in QTR, 1.2 in MKR, Figure 4).
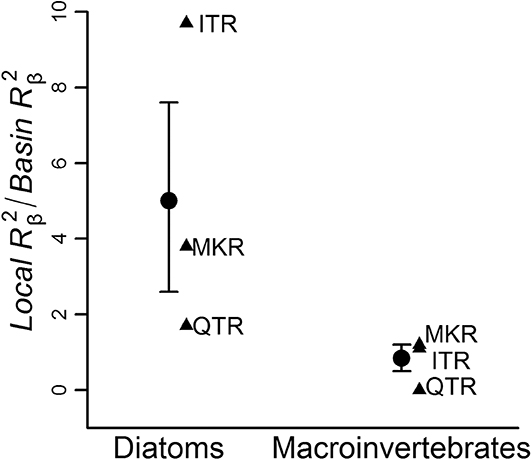
Figure 4. The ratio between local-scale environmental effect and basin-scale environmental effect (Local /Basin , on y axis) for diatom vs. macroinvertebrate communities in the three regions. Analyses were conducted at the whole network level. Triangles represent individual regions; circular symbols represent averages and error bars denote the standard error. For region abbreviations, see Figure 3.
Discussion
We found that the relative role of environmental control on community variation differed among three regions. These among-region differences were likely related to the variation in the degree of environmental heterogeneity. We further found that communities were exclusively controlled by environment in both headwater and downstream sites (except for one case), giving no consistent support for the general predictions of the NPH. Moreover, our results showed that diatom communities were more influenced by local-scale relative to basin-scale environmental filtering while the opposite was true for macroinvertebrate communities. This suggests that the difference in the ability to track environmental variation between macroinvertebrates and diatoms was most probably scale-dependent.
Comparison of Environmental Filtering Among Regions
In this study, the level of environmental control on community variation was the highest in the region with the highest environmental heterogeneity. We also found that the QTR region exhibited the highest and most significant land-cover control, whereas the ITR region exhibited the most significant climatic control. Such a finding may arise because the degree of land use heterogeneity was the highest in the QTR region, while the degree of climatic heterogeneity was the highest in the ITR region. Because the QTR region has experienced significant changes in land-use such as a dramatic decline in forests and an increase in farmland and urban land use during the last several decades (Wang et al., 2012), the QTR region covered large within-watershed land use gradients (e.g., 24–100% forested watershed land use). However, compared with the QTR and MKR regions, the ITR region covered larger spatial extent, and consequently, had higher climatic heterogeneity. Therefore, our results indicated that the importance of environmental filtering varied among regions with different level of environmental heterogeneity. Generally, heterogeneous environmental conditions offer more niche opportunities for species, thus elevating local and regional diversity if species dispersal is not limiting diversity (Heino et al., 2015b). Therefore, such environmental heterogeneity increases the role of environmental filtering (Leibold et al., 2004; Heino et al., 2015b).
Several studies have indicated only a weak influence of the level of environmental heterogeneity on environmental filtering (Landeiro et al., 2012; Grönroos et al., 2013; Bini et al., 2014; Heino et al., 2015a). However, the fact that we found a difference in the degree of environmental control among regions with different environmental heterogeneity may be related to at least two unique features of our study design: (1) In contrast to most studies that capture few environmental variables, our suite of 40 explaining variables including local environmental, climatic and land use factors (Tables S4, S5) may perhaps better reflect the true differences in environmental heterogeneity faced by the stream biota. (2) Unlike other studies that use an ecoregion (e.g., across multiple basins, Bini et al., 2014) as the metacommunity unit, we used a drainage basin as the unit of observation. In a relative sense, dispersal rates generally decrease with spatial extent (stream > basin > ecoregion). Dispersal rate among sites within a basin was probably adequate for species to track environmental variation across sites, resulting in strong environmental filtering but weak dispersal-related controls (Heino et al., 2015b,c). This was implied by the fact that the relationship between community dissimilarity and geographical distance was non-significant in most cases (Table 2), suggesting a generally weak effect of dispersal limitation but also that mass effects were not influential.
NPH Predictions
Consistent with recent stream studies (Schmera et al., 2018; Henriques-Silva et al., 2019), our results indicated that the NPH predictions cannot be regarded as general hypotheses in stream networks. We found that community dissimilarities in both headwater and downstream sites were significantly related solely to environmental distances, but not geographical distances. Therefore, general support for NPH predictions was basically lacking because mass effects were not strong enough in downstream sites. There are several reasons and evidence for a lack of mass effects on downstream metacommunities here. First, dispersal rate among sites was most probably relatively modest in our study regions due to intermediate extent, which resulted in strong environmental filtering (Ng et al., 2009; Heino et al., 2015c). Second, in half of the cases, downstream metacommunities were less connected than headwater metacommunities (Table S5), which decreases the importance of mass effects on downstream metacommunities. Third, only in half of the cases, community turnover was significantly (p < 0.001) higher at downstream sites than at headwater sites (Table S6), suggesting that beta diversity of downstream communities was only relatively weakly influenced by mass effects that should generally homogenize communities across sites (Jamoneau et al., 2018).
Environmental Control of Macroinvertebrates vs. Diatoms
Our MRM analyses showed that the level of environmental control was in general higher for macroinvertebrates than diatoms. Such a difference between macroinvertebrates and diatoms in the relative roles of environment in shaping communities across the same set of stream sites was also observed by Heino et al. (2012). Diatoms are more likely to have higher dispersal rates among sites than macroinvertebrates due their short life cycles and to being easily transported by a wide variety of vectors (e.g., wind, stream flow and animals, Kristiansen, 1996). Thus, diatoms can be often present in environmentally sub-optimal sites due to intense dispersal from environmentally suitable sites (mass effects). This may lead to a lower importance of environmental controls and a higher importance of spatial controls (Rouquette et al., 2013; Vilmi et al., 2017). Concordantly, in the MKR region, spatial control was significantly important for diatoms but not for macroinvertebrates. An alternative explanation for this pattern is that most of macroinvertebrates (e.g. aquatic insects) can often actively select environmentally suitable habitats via dispersal (Heino, 2013). Therefore, macroinvertebrates may be able to track environmental variation well-through active dispersal within our studied regions (e.g., in the ITR and QTR regions) and show stronger environmental filtering than diatoms (Farjalla et al., 2012).
However, when analyzing environmental factors more closely, we found diatom assemblages to be more related with local-scale environmental factors relative to basin-scale environmental factors while the opposite was true for macroinvertebrates. These findings indicate that diatoms are possibly better able to track local environmental variation e.g., in nutrients or water pH than macroinvertebrates, whereas macroinvertebrates are better able to track environmental variability at larger basin scale (e.g., climatic or land cover variables, physical stream variables) than diatoms. This result is congruent with earlier stream studies (Urban et al., 2006; Soininen, 2007; Liu et al., 2016). We recommend that further research aiming to compare environmental structuring of different organism groups should encompass multi-scale (e.g., local scale and basin scale) environmental variables, particularly at intermediate or large spatial extents (e.g., within a basin and across basin extents, Jyrkänkallio-Mikkola et al., 2017). Our results may thus have practical implications for stream monitoring programmes. For example, if alterations in watershed land use by agriculture or forestry are the main stressors, then macroinvertebrates might be recommended as the biological indicators. This is especially true if such land use effects modify physical structure of the streams via habitat modification or increased sediment load, for example.
Possible Caveats
Our results may have been affected also by some other factors such as biotic interactions not directly considered here. For example, we found that diatom community composition was significantly linked to macroinvertebrate community composition when correcting for both environmental and geographical distances in the QTR and MKR regions (Figure S1). This result suggests that in the QTR and MKR regions, availability of diatoms as prey may have influenced macroinvertebrate communities and grazing by macroinvertebrates may have influenced diatom communities.
A second factor may stem from seasonal and interannual biases in communities and community-environment relationships as three data sets were collected in different months. To facilitate the identification to the lowest possible level, we collected macroinvertebrates in spring season in the MKR and QTR regions as spring is the time when most macroinvertebrates are still in the larval stage but close to their maximum size. However, because of icebound waters during the spring season in the ITR region, sampling was conducted in summer season in the ITR region. Moreover, because of the large geographical extent among three regions, sampling of three regions was not possible in single year due to limited resources. However, metacommunity assembly is expected to be temporally relatively stable in perennial river systems (Sarremejane et al., 2017; Csercsa et al., 2019), such as in streams studied here. We thus believe that our main conclusions of macroinvertebrate metacommunity assembly may have been only little affected by the seasonal effects.
Conclusions
Our study suggested that environmental filtering generally overrode dispersal-related factors in stream networks and was most important in the regions characterized with high environmental heterogeneity. However, as the number of regions in our study was limited, future studies should include more regional datasets to obtain more general conclusions. Additionally, we found only weak evidence of the NPH predictions across different regions and organisms suggesting that environmental filtering prevailed throughout the river networks. Finally, we showed that diatoms and macroinvertebrates perceive their environment at different scales most probably because of their fundamental biological differences. To summarize, even if environmental filtering is generally strong on stream metacommunities, these results support a view suggesting that metacommunity assembly is relatively context-dependent, potentially related to the degree of environmental heterogeneity, biological characteristics of the focal organismal group and spatial extent.
Data Availability Statement
The original contributions presented in the study are included in the article/supplementary materials, further inquiries can be directed to the corresponding author/s.
Author Contributions
SH and BW conceived the ideas. SH, KC, and BW analyzed the data. SH, JS, and BW led the writing. All authors contributed to the writing of the paper.
Funding
This research was funded by the National Natural Science Foundation of China (NSFC, No. 41771052). The Ph.D student (SH) was funded by the China Scholarship Council (201806850078).
Conflict of Interest
The authors declare that the research was conducted in the absence of any commercial or financial relationships that could be construed as a potential conflict of interest.
Acknowledgments
We thank Dr. Vincent H. Resh from Department of Environmental Science, Policy, & Management, University of California and Dr. Christoph Matthaei from Department of Zoology, University of Otago for their valuable comments on the earlier draft. We thank many colleagues at the Laboratory of Aquatic Insects and Stream Ecology of Nanjing Agricultural University for their assistance in the field and laboratory, especially Professor Lianfang Yang, Ling Ding and Yunlei Zhou.
Supplementary Material
The Supplementary Material for this article can be found online at: https://www.frontiersin.org/articles/10.3389/fevo.2020.00141/full#supplementary-material
References
Anderson, M. J. (2006). Distance-based tests for homogeneity of multivariate dispersions. Biometrics 62, 245–253. doi: 10.1111/j.1541-0420.2005.00440.x
Astorga, A., Oksanen, J., Luoto, M., Soininen, J., Virtanen, R., and Muotka, T. (2012). Distance decay of similarity in freshwater communities: do macro- and microorganisms follow the same rules? Global Ecol. Biogeogr. 21, 365–375. doi: 10.1111/j.1466-8238.2011.00681.x
Baselga, A. (2010). Partitioning the turnover and nestedness components of beta diversity. Global Ecol. Biogeogr. 19, 134–143. doi: 10.1111/j.1466-8238.2009.00490.x
Bini, L. M., Landeiro, V. L., Padial, A. A., Siqueira, T., and Heino, J. (2014). Nutrient enrichment is related to two facets of beta diversity for stream invertebrates across the United States. Ecology 95, 1569–1578. doi: 10.1890/13-0656.1
Brown, B. L., Sokol, E. R., Skelton, J., and Tornwall, B. (2017). Making sense of metacommunities: dispelling the mythology of a metacommunity typology. Oecologia 183, 643–652. doi: 10.1007/s00442-016-3792-1
Brown, B. L., and Swan, C. M. (2010). Dendritic network structure constrains metacommunity properties in riverine ecosystems. J. Anim. Ecol. 79, 571–580. doi: 10.1111/j.1365-2656.2010.01668.x
Cañedo-Argüelles, M., Boersma, K. S., Bogan, M. T., Olden, J. D., Phillipsen, I., Schriever, T. A., et al. (2015). Dispersal strength determines meta-community structure in a dendritic riverine network. J. Biogeogr. 42, 778–790. doi: 10.1111/jbi.12457
Chen, K., Hughes, R. M., and Wang, B. (2015). Effects of fixed-count size on macroinvertebrate richness, site separation, and bioassessment of Chinese monsoonal streams. Ecol. Indic. 53, 162–170. doi: 10.1016/j.ecolind.2015.01.011
Chen, K., Sun, D., Rajper, A. R., Mulatibieke, M., Hughes, R. M., Pan, Y., et al. (2019). Concordance in biological condition and biodiversity between diatom and macroinvertebrate assemblages in Chinese arid-zone streams. Hydrobiologia 829, 245–263. doi: 10.1007/s10750-018-3836-0
Clarke, K. R., and Ainsworth, M. (1993). A Method of linking multivariate community structure to environmental variables. Mar. Ecol. Prog. Ser. 92, 205–219. doi: 10.3354/meps092205
Csercsa, A., Krasznai, E. Á., Várbíró, G., Szivák, I., Tóth, M., et al. (2019). Seasonal changes in relative contribution of environmental control and spatial structuring on different dispersal groups of stream macroinvertebrates. Hydrobiologia 828, 101–115. doi: 10.1007/s10750-018-3806-6
De Bie, T., De Meester, L., Brendonck, L., Martens, K., Goddeeris, B., Ercken, D., et al. (2012). Body size and dispersal mode as key traits determining metacommunity structure of aquatic organisms. Ecol. Lett. 15, 740–747. doi: 10.1111/j.1461-0248.2012.01794.x
Ding, N., Yang, W., Zhou, Y., Gonzalez-Bergonzoni, I., Zhang, J., Chen, K., et al. (2017). Different responses of functional traits and diversity of stream macroinvertebrates to environmental and spatial factors in the Xishuangbanna watershed of the upper Mekong River Basin, China. Sci. Total Environ. 574, 288–299. doi: 10.1016/j.scitotenv.2016.09.053
Eros, T. (2017). Scaling fish metacommunities in stream networks: synthesis and future research avenues. Commun. Ecol. 18, 72–86. doi: 10.1556/168.2017.18.1.9
Eros, T., and Lowe, W. H. (2019). The landscape ecology of rivers: from patch-based to spatial network analyses. Curr. Landsc. Ecol. Rep. 4, 103–112. doi: 10.1007/s40823-019-00044-6
Farjalla, V. F., Srivastava, D. S., Marino, N. A., Azevedo, F. D., Dib, V., Lopes, P. M., et al. (2012). Ecological determinism increases with organism size. Ecology 93, 1752–1759. doi: 10.1890/11-1144.1
Goslee, S. C., and Urban, D. L. (2007). The ecodist package for dissimilarity-based analysis of ecological data. J. Stat. Softw. 22, 1–19. doi: 10.18637/jss.v022.i07
Grönroos, M., Heino, J., Siqueira, T., Landeiro, V. L., Kotanen, J., and Bini, L. M. (2013). Metacommunity structuring in stream networks: roles of dispersal mode, distance type, and regional environmental context. Ecol. Evol. 3, 4473–4487. doi: 10.1002/ece3.834
He, S. W., Soininen, J., Deng, G. P., and Wang, B. X. (2020). Metacommunity structure of stream insects across three hierarchical spatial scales. Ecol. Evol. 10, 2874–2884. doi: 10.1002/ece3.6103
Heino, J. (2013). Environmental heterogeneity, dispersal mode, and co-occurrence in stream macroinvertebrates. Ecol. Evol. 3, 344–355. doi: 10.1002/ece3.470
Heino, J., Grönroos, M., Soininen, J., Virtanen, R., and Muotka, T. (2012). Context dependency and metacommunity structuring in boreal headwater streams. Oikos 121, 537–544. doi: 10.1111/j.1600-0706.2011.19715.x
Heino, J., Melo, A. S., and Bini, L. M. (2015b). Reconceptualising the beta diversity-environmental heterogeneity relationship in running water systems. Freshw. Biol. 60, 223–235. doi: 10.1111/fwb.12502
Heino, J., Melo, A. S., Bini, L. M., Altermatt, F., Al-Shami, S. A., Angeler, D. G., et al. (2015a). A comparative analysis reveals weak relationships between ecological factors and beta diversity of stream insect metacommunities at two spatial levels. Ecol. Evol. 5, 1235–1248. doi: 10.1002/ece3.1439
Heino, J., Melo, A. S., Siqueira, T., Soininen, J., Valanko, S., and Bini, L. M. (2015c). Metacommunity organisation, spatial extent and dispersal in aquatic systems: patterns, processes and prospects. Freshw. Biol. 60, 845–869. doi: 10.1111/fwb.12533
Heino, J., Soininen, J., Alahuhta, J., Lappalainen, J., and Virtanen, R. (2017). Metacommunity ecology meets biogeography: effects of geographical region, spatial dynamics and environmental filtering on community structure in aquatic organisms. Oecologia 183, 121–137. doi: 10.1007/s00442-016-3750-y
Henriques-Silva, R., Logez, M., Reynaud, N., Tedesco, P. A., Brosse, S., Januchowski-Hartley, S. R., et al. (2019). A comprehensive examination of the network position hypothesis across multiple river metacommunities. Ecography 42, 284–294. doi: 10.1111/ecog.03908
Jamoneau, A., Passy, S. I., Soininen, J., Leboucher, T., and Tison-Rosebery, J. (2018). Beta diversity of diatom species and ecological guilds: response to environmental and spatial mechanisms along the stream watercourse. Freshw. Biol. 63, 62–73. doi: 10.1111/fwb.12980
Johnson, R. K., Furse, M. T., Hering, D., and Sandin, L. (2007). Ecological relationships between stream communities and spatial scale: implications for designing catchment-level monitoring programs. Freshw. Biol. 52:939–958. doi: 10.1111/j.1365-2427.2006.01692.x
Jyrkänkallio-Mikkola, J., Meier, S., Heino, J., Laamanen, T., Pajunen, V., Tolonen, K. T., et al. (2017). Disentangling multi-scale environmental effects on stream microbial communities. J. Biogeogr. 44, 1512–1523. doi: 10.1111/jbi.13002
Kondolf, G. M. (1997). Application of the pebble count: notes on purpose, method, and variants. J. Am. Water Resour. Assoc. 33, 79–87. doi: 10.1111/j.1752-1688.1997.tb04084.x
Krammer, K. (2003). “Cymbopleura, delicata, navicymbula, gomphocymbellopsis, afrocymbella,” in Diatoms of Europe, Diatoms of the European Inland Waters and Comparable Habitats, vol. 4. ed H. Lange-Bertalot (Rugell: A.R.G.Gantner Verlag K.G.), 1–529.
Krammer, K., and Lange-Bertalot, H. (1986). Bacillariophyceae, Teil 1 Naviculaceae. Heidelberg: Spektrum Akademischer Verlag.
Krammer, K., and Lange-Bertalot, H. (1988). Bacillariophyceae, Teil 2 Epithemiaceae, Bacillariophyceae, Surirellaceae. Heidelberg: Spektrum Akademischer Verlag.
Krammer, K., and Lange-Bertalot, H. (1991a). Bacillariophyceae, Teil 3. Centrales, Fragilariaceae, Eunotiaceae, Achnanthaceae. Heidelberg: Spektrum Akademischer Verlag.
Krammer, K., and Lange-Bertalot, H. (1991b). Bacillariophyceae, Teil 4. Achnanthaceae, Kritische ErganzungenzuNavicula (lineolate) Und Gomphonema. Heidelberg: Spektrum Akademischer Verlag.
Kristiansen, J. (1996). Dispersal by freshwater algae—a review. Hydrobiologia 336, 151–157. doi: 10.1007/BF00010829
Landeiro, V. L., Bini, L. M., Melo, A. S., Pes, A. M. O., and Magnusson, W. E. (2012). The roles of dispersal limitation and environmental conditions in controlling caddisfly (Trichoptera) assemblages. Freshw. Biol. 57, 1554–1564. doi: 10.1111/j.1365-2427.2012.02816.x
Leibold, M. A., and Chase, J. M. (2017). Metacommunity Ecology. Princeton, NJ: Princeton University Press.
Leibold, M. A., Holyoak, M., Mouquet, N., Amarasekare, P., Chase, J. M., Hoopes, M. F., et al. (2004). The metacommunity concept: a framework for multi-scale community ecology. Ecol. Lett. 7, 601–613. doi: 10.1111/j.1461-0248.2004.00608.x
Lichstein, J. W. (2007). Multiple regression on distance matrices: a multivariate spatial analysis tool. Plant Ecol. 188, 117–131. doi: 10.1007/s11258-006-9126-3
Liu, S., Xie, G., Wang, L., Cottenie, K., Liu, D., and Wang, B. (2016). Different roles of environmental variables and spatial factors in structuring stream benthic diatom and macroinvertebrate in Yangtze River Delta, China. Ecol. Indic. 61:602–611. doi: 10.1016/j.ecolind.2015.10.011
Morse, J. C., Yang, L. F., and Tian, L. X. (1994). Aquatic Insects of China Useful for Monitoring Water Quality. Nanjing: Hohai University Press.
Ng, I. S. Y., Carr, C. M., and Cottenie, K. (2009). Hierarchical zooplankton metacommunities: distinguishing between high and limiting dispersal mechanisms. Hydrobiologia 619, 133–143. doi: 10.1007/s10750-008-9605-8
Oksanen, J., Blanchet, F. G., Kindt, R., Legendre, P., Minchin, P. R., O'hara, R. B., et al. (2013). Package ‘vegan’. Community Ecology Package, Version.
Pan, Y., Stevenson, R. J., Hill, B. H., Herlihy, A. T., and Collins, G. B. (1996). Using diatoms as indicators of ecological conditions in lotic systems: a regional assessment. J. N. Am. Benthol. Soc. 15, 481–495.
R. Core Team (2018). R: A Language and Environment for Statistical Computing. R Foundation for Statistical Computing. (Vienna). Available online at: https://www.R-project.org/
Rouquette, J. R., Dallimer, M., Armsworth, P. R., Gaston, K. J., Maltby, L., and Warren, P. H. (2013). Species turnover and geographic distance in an urban river network. Divers Distrib. 19, 1429–1439. doi: 10.1111/ddi.12120
Sarremejane, R., Cañedo-Argüelles, M., Prat, N., Mykrä, H., Muotka, T., and Bonada, N. (2017). Do metacommunities vary through time? Intermittent rivers as model systems. J. Biogeogr. 44, 2752–2763. doi: 10.1111/jbi.13077
Schmera, D., Árva, D., Boda, P., Bódis, E., Bolgovics, Á., Borics, G., et al. (2018). Does isolation influence the relative role of environmental and dispersal-related processes in stream networks? An empirical test of the network position hypothesis using multiple taxa. Freshw. Biol. 63, 74–85. doi: 10.1111/fwb.12973
Shurin, J. B., Cottenie, K., and Hillebrand, H. (2009). Spatial autocorrelation and dispersal limitation in freshwater organisms. Oecologia 159, 151–159. doi: 10.1007/s00442-008-1174-z
Soininen, J. (2007). Environmental and spatial control of freshwater diatoms - A review. Diatom Res. 22, 473–490. doi: 10.1080/0269249X.2007.970572
Soininen, J. (2014). A quantitative analysis of species sorting across organisms and ecosystems. Ecology 95, 3284–3292. doi: 10.1890/13-2228.1.sm
Song, M. Y., Leprieur, F., Thomas, A., Lek-Ang, S., Chon, T. S., and Lek, S. (2009). Impact of agricultural land use on aquatic insect assemblages in the Garonne river catchment (SW France). Aquatic Ecol. 43, 999–1009. doi: 10.1007/s10452-008-9218-3
Tonkin, J. D., Tachamo Shah, R. D., Shah, D. N., Hoppeler, F., Jähnig, S. C., and Pauls, S. U. (2017). Metacommunity structuring in Himalayan streams over large elevational gradients: the role of dispersal routes and niche characteristics. J. Biogeogr. 44, 62–74. doi: 10.1111/jbi.12895
Urban, M. C., Skelly, D. K., Burchsted, D., Price, W., and Lowry, S. (2006). Stream communities across a rural–urban landscape gradient. Divers. Distrib. 12:337–350. doi: 10.1111/j.1366-9516.2005.00226.x
Vilmi, A., Tolonen, K. T., Karjalainen, S. M., and Heino, J. (2017). Metacommunity structuring in a highly-connected aquatic system: effects of dispersal, abiotic environment and grazing pressure on microalgal guilds. Hydrobiologia 790, 125–140. doi: 10.1007/s10750-016-3024-z
Wang, B. X., Liu, D. X., Liu, S. R., Zhang, Y., Lu, D. Q., and Wang, L. Z. (2012). Impacts of urbanization on stream habitats and macroinvertebrate communities in the tributaries of Qiangtang River, China. Hydrobiologia 680, 39–51. doi: 10.1007/s10750-011-0899-6
Winegardner, A. K., Jones, B. K., Ng, I. S. Y., Siqueira, T., and Cottenie, K. (2012). The terminology of metacommunity ecology. Trends Ecol. Evol. 27, 253–254. doi: 10.1016/j.tree.2012.01.007
Keywords: environmental filtering, distance-decay, dispersal, spatial scale, environmental heterogeneity, dendritic networks
Citation: He S, Soininen J, Chen K and Wang B (2020) Environmental Factors Override Dispersal-Related Factors in Shaping Diatom and Macroinvertebrate Communities Within Stream Networks in China. Front. Ecol. Evol. 8:141. doi: 10.3389/fevo.2020.00141
Received: 20 March 2020; Accepted: 24 April 2020;
Published: 19 May 2020.
Edited by:
Miguel Cañedo-Argüelles, University of Barcelona, SpainReviewed by:
Julie Crabot, Délégation Rhône Auvergne (CNRS), FranceTibor Eros, MTA Centre for Ecological Research, Hungary
Copyright © 2020 He, Soininen, Chen and Wang. This is an open-access article distributed under the terms of the Creative Commons Attribution License (CC BY). The use, distribution or reproduction in other forums is permitted, provided the original author(s) and the copyright owner(s) are credited and that the original publication in this journal is cited, in accordance with accepted academic practice. No use, distribution or reproduction is permitted which does not comply with these terms.
*Correspondence: Beixin Wang, wangbeixin@njau.edu.cn