- 1Institut des Sciences de l'Evolution de Montpellier (Université de Montpellier, CNRS, IRD, EPHE), Montpellier, France
- 2Department of Geology and Palaeontology, Natural History Museum of Geneva, Geneva, Switzerland
Both biotic and abiotic factors likely played a role in influencing the diversification patterns of clades. Although the role of environmental forcing on the long-term evolution of biodiversity has been explored for invertebrate clades, little is known about how vertebrate groups responded to environmental changes. Among vertebrates, fishes (ray-finned fishes and elasmobranchs) have a long, rich, and complex evolutionary history comprising numerous diversification and extinction events. Yet, knowledge on the causes for the diversity fluctuations of these most speciose aquatic vertebrate clades in modern marine and continental ecosystems were restricted to qualitative interpretations. Here we use multiple regression methods to quantitatively examine the role of six abiotic parameters over the long-term variations of elasmobranch and actinopterygian genus-level diversity. We find that marine actinopterygian diversity is mainly controlled by temperature while continental fragmentation is the primary driver of the diversity fluctuations of elasmobranchs. Sea-level variations correlate positively with the diversity variations of both marine groups, whereas none of the tested proxies explains the diversity variation of freshwater ray-finned fishes. Our results indicate that such contrasting responses are mainly due to ecological and life-history trait differences between these groups.
Introduction
Understanding the relationships between biodiversity and global environmental variations through geological time represents a major focus in macroevolution and global change studies. However, this challenge has long been hampered by controversies over how much reliable the fossil record is (Erwin, 2009). It has been demonstrated that taking the fossil record at face value to estimate diversity variations produces inaccurate palaeodiversity reconstructions due to several geological and sampling biases that affect its direct reading (Raup, 1976; Peters and Foote, 2001; Smith, 2001; Peters, 2005, 2006; Benton and Emerson, 2007). Over the past decade however, a number of analytical approaches have been developed to circumvent these biases and produce more reliable estimates of deep-time diversity variations based on fossil data (Norell, 1993; Alroy et al., 2008; Alroy, 2014; Silvestro et al., 2014). Meanwhile, geochemical studies have gathered a tremendous amount of data (Prokoph et al., 2008; Veizer and Prokoph, 2015) on a number of proxies that reflect climate and environmental parameters (Cárdenas and Harries, 2010; Hannisdal and Peters, 2011; Mayhew et al., 2012). These recent advances have permitted to test the hypothesis of a long-term control of various environmental factors over the fluctuations of taxonomic richness throughout the Phanerozoic. These studies mainly focused on marine invertebrate data (Mayhew et al., 2008, 2012; Cárdenas and Harries, 2010; Ezard et al., 2011; Hannisdal and Peters, 2011; Liow et al., 2015), whereas those that explored the role of some environmental variations over marine or continental vertebrate clades are comparatively scarce and concern low-diversity tetrapod groups (Marx and Uhen, 2010; Benson and Butler, 2011; Butler et al., 2011; Martin et al., 2014; Mannion et al., 2015). Multiple lines of evidence indicate that temperature acted as a major control over the evolutionary history of clades (Aguirre and Riding, 2005; Hunt et al., 2005; Marx and Uhen, 2010; Ezard et al., 2011; Figueirido et al., 2012; Mayhew et al., 2012; Condamine et al., 2013, 2019; Martin et al., 2014; De Blasio et al., 2015; Mannion et al., 2015), but it has been demonstrated that other factors such as sea-level (Peters, 2005; Benson and Butler, 2011; Hannisdal and Peters, 2011), tectonics (Zaffos et al., 2017), and nutrient availability (Cárdenas and Harries, 2010) likely acted over the evolution of biodiversity.
We focus on elasmobranchs (sharks, skates, and rays) and actinopterygians (ray-finned fishes)—the most speciose aquatic vertebrate clades in modern marine and continental ecosystems—to test the hypothesis of a long-term control of abiotic factors over vertebrates' diversity. These clades encompass a tremendous range of ecological, biological, morphological and environmental adaptations and their evolutionary history includes three of the biggest diversification events among jawed vertebrates (Alfaro et al., 2009). Previous family-level analyses indicated similar diversification patterns for marine actinopterygians and elasmobranchs, whereas the diversity variations of freshwater actinopterygians show contrasting patterns (Guinot and Cavin, 2015). However, quantitative analyses of the determinants of the diversification patterns of these clades are lacking at lower taxonomic levels. Here we use multiple regression methods to examine the role of six abiotic parameters over the long-term diversity variations of elasmobranch, marine and freshwater ray-finned fish clades based on bias-corrected diversity data at genus level.
Methods and Data
Taxic Diversity Data
The diversity datasets used in the present work (Figures 1A,B, Supplementary Data 1) encompass all extant and extinct elasmobranch genera (541 taxa) over most of the time interval of the group (Upper Triassic to Recent) and all extinct actinopterygian genera (780) over the Upper Jurassic-Paleocene interval. Post-Paleocene phylogenetic and fossil record data for actinopterygians could not be included in the analyses for a two-fold reason: integrating extinct taxa into the still fluctuating molecular phylogenies of recent acanthomorphs alongside the necessary re-assessment of the systematic affinities of much of the enormous Eocene to Recent fossil record are tasks beyond the scope of this study.
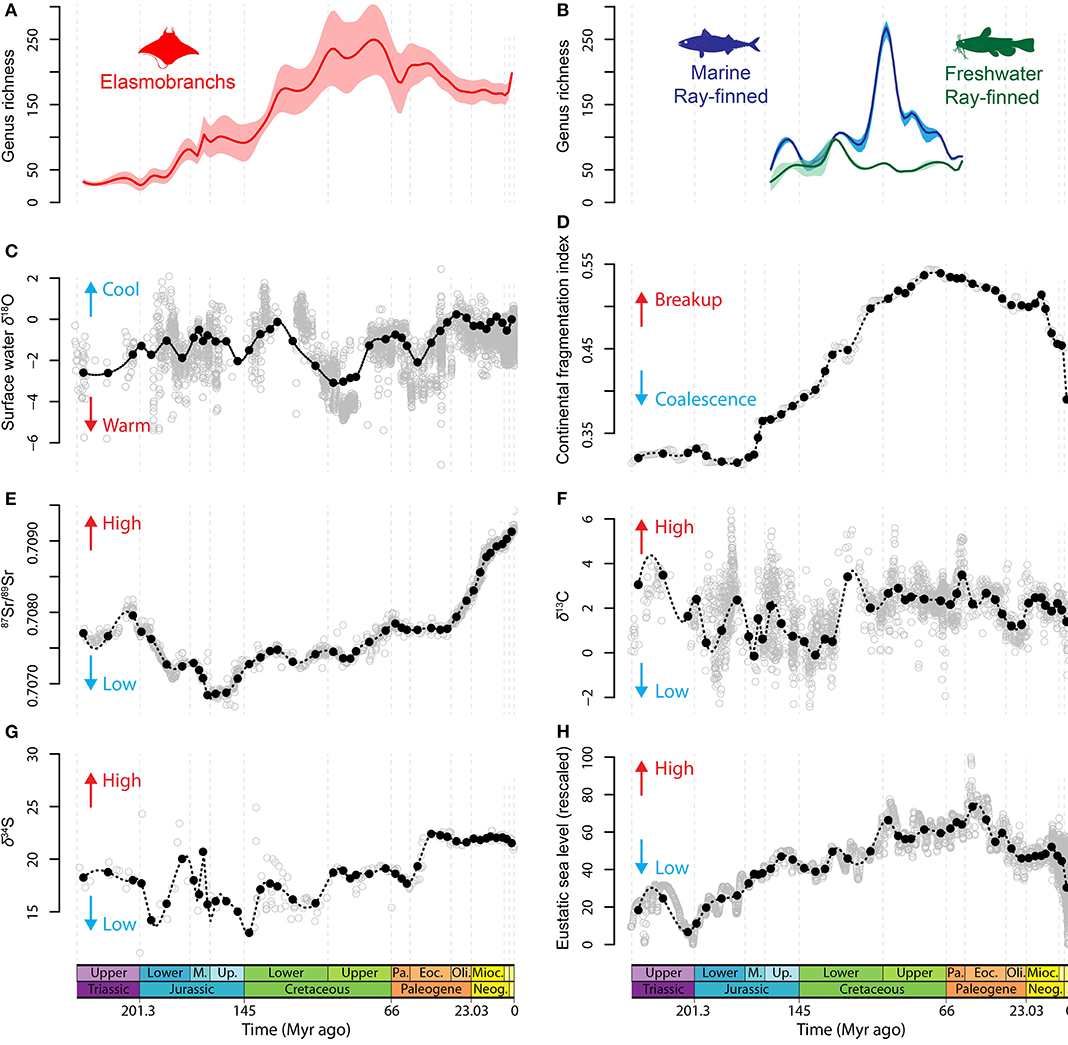
Figure 1. Global “fish” genus-level diversity and abiotic variables. (A) PDE estimates for elasmobranchs and (B) for actinopterygians. Thick curves represent the median values computed to sum up the four PDE considered here and pale-colored areas represent the range of values of all PDE. Curves were produced using the spline interpolation method based on diversity values of each time bin. (C) Oxygen isotope data, (D) continental fragmentation index, (E) strontium, (F) carbon, (G) sulfur, and (H) sea-level data. Empty gray circles represent data points that served to compute mean data points (black points) for each time bin. Curves were produced using the spline interpolation method and are provided for visual representation of the trends only. Source data are available in Supplementary Data 1.
Data were extracted from a phylogeny-based analysis of elasmobranch and actinopterygian fossil records and diversification patterns (Guinot and Cavin, 2016). This analysis produced a genus-level supertree for each clade and provided phylogenetic diversity estimates (PDE). For each timescaled phylogeny, polytomies were treated in two ways following Boyd et al. (2011): (i) resolved in chronological order [pectinate arrangement placing the taxon having the oldest First Appearance Datum (FAD) at the base of the resolved clade and the one with the youngest FAD in the most derived position] and (ii) resolved in reverse-chronological order (pectinate arrangement placing the taxon having the youngest FAD at the base of the resolved clade and the one with the oldest FAD in the most derived position). This produced two topologies (chronological and reverse-chronological polytomy resolutions) for each of the supertrees considered. Uncertainty in the age of FAD were considered in each tree topologies by randomly picking an absolute age within the FAD range of each taxon, for each 1,000,000 replicates as in Boyd et al. (2011). Two tree topologies were selected for each polytomy resolution method based on their congruence scores (Wills, 1999; Pol and Norell, 2001), which assess the fit of phylogenetic relationships with stratigraphic ranges of terminal taxa. This resulted in four phylogenetic diversity estimates (PDE) for each clade: most congruent chronological, least congruent chronological, most congruent reverse-chronological and least congruent reverse-chronological (see Guinot and Cavin, 2016). Actinopterygian PDE data were further divided into three datasets corresponding to three ecological preferences: (i) exclusively marine actinopterygian genera, (ii) exclusively freshwater actinopterygian genera, and (iii) genera from mixed environments (i.e., genera either including species from freshwater and marine environments, or including euryhaline taxa). The latter were excluded from our analyses because of equivocal environmental distributions. For each dataset, analyses were performed on each four PDE data. We also selected the median diversity value for each time bin in order to sum up all PDE in one curve (Figures 1A,B).
Selection of Explanatory Variables
Data on abiotic variables (Figures 1C–H, Supplementary Data 1) were taken from the literature and averaged for each time bin to comply with the diversity data. Two sets of data on sea level fluctuations were selected. Data from Haq et al. (1987) are based on sequence stratigraphy and span the Triassic-Recent interval. Sea level data from Miller et al. (2005) reflect eustatic changes due to variations in continental ice sheet volumes, which are recorded by δ18O from foraminifera over the Early Jurassic-Recent interval. Data of Miller et al. (2005), although more recent, do not span the entire time interval of the elasmobranch evolutionary history. We therefore created a composite sea level dataset by standardizing the original data at minimal and maximal values of 0 and 100, respectively, following Cárdenas and Harries (2010) and using the function scale () of the R-package “Scales” (Wickham, 2017). We then selected the scaled data of Miller et al. (2005) for the Aalenian-Recent interval and those of Haq et al. (1987) for the Carnian-Toarcian interval. Oxygen isotope records (expressed as δ18O values) were selected as representatives of trends in global climate change. Although climate change is multifactorial, δ18O records are considered as an inverse proxy for global climate change that mainly reflects temperature fluctuations (also global ice volume during icehouse periods) (Zachos et al., 2008). The δ18O data used here were taken from Veizer and Prokoph (2015). We selected the surface-water (mixed surface layer) data for the temperate and tropical realms excluding deep-sea and high-latitude data to preserve a homogenous signal over time bins and to comply with the dominant geographic and environmental distributions of the taxa included in our dataset. The δ13C data of Prokoph et al. (2008) were selected to represent a proxy of productivity and preservation of organic matter (Cárdenas and Harries, 2010). Proxies representing nutrient input from continental weathering (Martin, 1996; Shields, 2007) (87Sr/89Sr) and from recycling of organic material in ocean sediments (Cárdenas and Harries, 2010) (δ34S) over the Triassic-Recent interval were extracted form Prokoph et al. (2008). Missing value for two time bins (Tithonian and Hettangian) in the δ34S data were extrapolated using the spline interpolation method with the function aspline () of the R-package “akima” (Akima and Gebhardt, 2016) to perform an univariate akima interpolation at 0.5 years intervals. These were then averaged for each of the time bins used here. The index of continental fragmentation produced by Zaffos et al. (2017) that ranges from 0 (maximum tectonic plate aggregation) to 1 (complete plate fragmentation) was selected as another abiotic variable to represent temporal changes in the geographic arrangement of continental crust.
Proxies representing geological sampling were selected in order to account for the possible effect of this parameter in biasing diversity estimates. The elasmobranch and actinopterygian fossil records have been sampled since the end of the 18th century with uneven information about historic collections, which precludes a direct measure of the number of fish-bearing collections. Consequently, sampling effort is represented here by counts of fossiliferous geological stratae and we also considered counts of fossil collections, which were tested alternatively in our models. Collection or strata ages that span several time bins due to uncertainty were considered present in their whole age range. Data representing this proxy were downloaded from the Paleobiology Database (http://paleobiodb.org, accessed on July 2018) and divided into marine and continental subsets.
Generalized Least Squares
This approach is a multiple regression method that offers the possibility of comparing a single dependent variable (here the PDE) to multiple explanatory variables (proxies). Whereas ordinary least square (OLS) regressions assume no serial correlation, generalized least squares (GLS) do not assume that data series or values within data series are independent and include autoregressive models in the analyses to account for autocorrelation issues. The set of models tested here comprises all possible combinations among the six explanatory variables considered as well as a null (intercept) model, which resulted in 64 models. The marine-related explanatory variables represented by δ13C and δ34S were not considered in analyses of the freshwater actinopterygian PDE because of their irrelevance as proxies for continental macroevolutionary patterns. However, we chose to keep the 87Sr/89Sr variable as it also represents variations in weathering intensity of landmasses and orogenic uplifts (Raymo and Ruddiman, 1992; Shields, 2007). This resulted in 33 models (including the null model) for the freshwater actinopterygian analyses. All models were duplicated to include a variable representing the rock record sampling, either expressed as the global count of fossiliferous stratae or as the number of fossil collections. This was made with the aim of considering the potential increased fit of adding such variables to our models, which would suggest incomplete sampling bias correction of the diversity variables. Following the method used in some previous macroevolutionary studies (e.g., Hunt et al., 2005; Marx and Uhen, 2010), we first fitted our 128 models (66 models for the freshwater data) using OLS and then fitted autoregressive models [function ar () of the R-package “Stats”] of order 1 (first-order autoregressive covariance model) to the residuals of the OLS. We then ran our models using GLS with the correlation structure of the residuals provided for the first order of autoregressive models. We subsequently compared the fit of autoregressive orders 0 (no autocorrelation) and 1 for each model and selected the best model for each combination of variables using the modified version of Akaike's information criterion for small sample sizes (AICc). The conditional probabilities for each model (Akaike weights, wAICc) as well as the generalized coefficient of determination (R2) were computed to inform on the proportion of variance in the dependent variable that is explained by each model.
Non-normality of the residuals and heteroskedasticity (unequal variance of residuals) can cause issues in biasing estimates of the standard errors and of the variance of the coefficient estimators (incorrect testing of confidence intervals and hypothesis testing). We tested for normality and homoskedasticity of residuals using the Jarque and Bera (1980) and Breusch and Pagan (1979) tests, respectively. Most models for the elasmobranch dataset and some models in the marine and freshwater actinopterygian datasets contained non-normally distributed residuals that could not be removed by transforming the data (either Log or Square root transformation). For elasmobranch data, non-normality was avoided by deleting the data point for the Pleistocene-Recent interval and, in most instances, models that contained non-normality in all datasets were not ranked among the best fit models and did not affect the interpretation of our results. One exception is the freshwater actinopterygian analysis on the least congruent chronological PDE data, which contains non-normally distributed residuals. However, results were comparable to the same models for other freshwater actinopterygian PDE datasets with normally distributed residuals (see below). Heteroskedasticity was absent from the models of the actinopterygian datasets but present in some models of the elasmobranch dataset and we used heteroskedasticity-consistent standard error (and p-value) estimators (White, 1980) to correct estimates. We used the function vcovHC () of the R-package “Sandwich” to produce heteroskedasticity-consistent estimations of the variance-covariance matrix of coefficient estimates using the H3 type, as recommended by Long and Ervin (2000). However, such method might overcorrect for heteroskedasticity since significance is sometimes lost after H3 type correction for variables in models that contain heteroskedasticity, whereas similar homoskedastic models used for other PDE estimates provided significant correlations (see below).
Ecology Data
We compiled the main ecologies of living marine actinopterygian and elasmobranch species (Supplementary Data 2) with the aim of estimating the proportion of species living on (benthic) or close to (demersal, bathydemersal) the bottom among these two clades. Species with a range of ecologies that are not strictly benthic/demersal (e.g., benthopelagic) were not counted. Data for marine actinopterygians were extracted from FishBase (Froese and Pauly, 2019) through the R package “rfishbase” (Boettiger et al., 2012) and those for elasmobranchs were mainly taken from Ebert et al. (2013) and Last et al. (2016) with some complements from FishBase. For elasmobranchs, among a total of 1,203 species, 1,157 are marine and 1,003 marine species are benthic, demersal, or bathydemersal. Among the 14,324 strictly marine actinopterygian species, 6,560 species are benthic, demersal or bathydemersal.
Results
Results of our analyses on the median diversity data are summarized in Table 1 and those of all PDE datasets are detailed in Supplementary Data 3–5. Regardless of the taxonomic dataset considered, none of the models including the sampling bias proxies as an additional explanatory variable is ranked among the best fit models (Supplementary Data 3–6). This tends to suggest that PDE provide robust estimations of diversity and efficiently correct for sampling biases. Alternatively, the sampling biases affecting the elasmobranch and actinopterygian datasets might not be accurately represented by the available global sampling bias proxies. However, in the absence of a direct measure of the number of “fish”-bearing collections (see section Selection of Explanatory Variables), there are no other available data that quantify sampling biases.
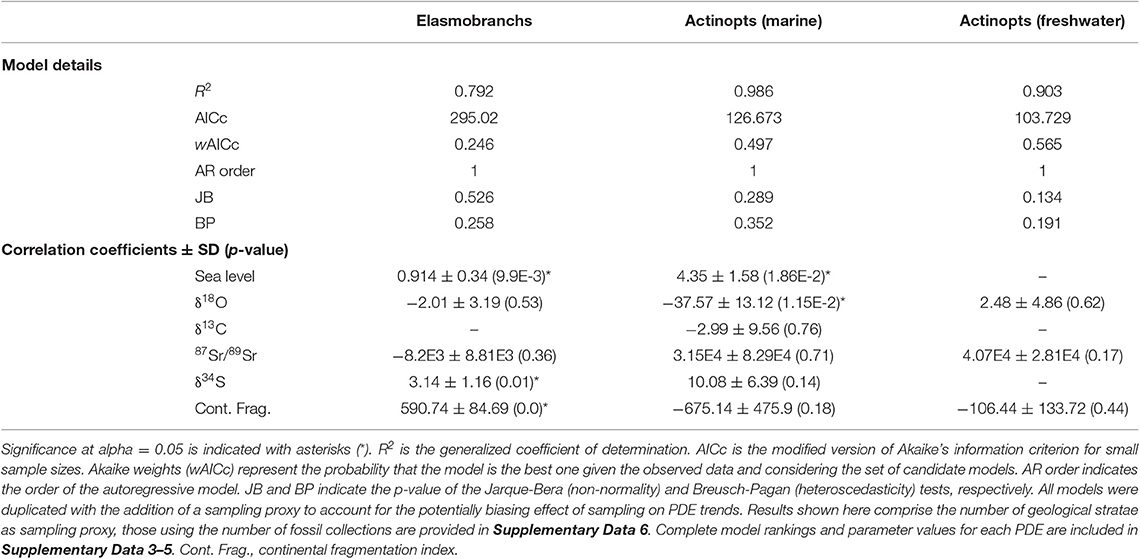
Table 1. Estimated best-fit model parameters computed with generalized least squares regression for the median genus-level PDE of the elasmobranch, marine, and freshwater actinopterygian datasets.
Results for the elasmobranch dataset indicate close ΔAICc values for the first four best fit models as well as low wAICc (Supplementary Data 3), suggesting a nearly equivalent fit. However, all four best fit models, which altogether represent a cumulative wAICc of 0.807, indicate a strong (from 587 to 597 according to models) and statistically significant (p < 9.98e−10) positive correlation coefficient for the continental fragmentation index. Sea level correlates weakly (0.909–0.92) but significantly in all first four models. Similarly, sulfur correlates moderately (3.14–3.28) in all four models with statistical significance for all of them, although significance is lost after the use of heteroskedasticity-consistent estimates for the two models that contain heteroskedasticity.
Results for the marine actinopterygian dataset indicate that the best fit model (wAICc = 0.497, R2 = 0.986) is the one that comprises all six abiotic variables. Among them, surface-water (mixed surface layer) δ18O values have a strong negative correlation coefficient (−37.57) with strong statistical support (p = 0.015). In addition, a positive correlation (4.35) is found significant (p = 0.019) for the variable representing sea level fluctuations. Similar results are found for all four PDE datasets taken independently (Supplementary Data 4). Analyses on the freshwater actinopterygian data identify the model including δ18O, continental fragmentation and 87Sr/89Sr data as explanatory variables (wAICc = 0.565, R2 = 0.90). However, none of the correlation coefficients is statistically supported, and similar results are obtained for each PDE hypothesis (Supplementary Data 5).
Our results indicate that sea-level fluctuations correlate positively with the diversity variations of both marine actinopterygians and elasmobranchs. Furthermore, elasmobranch diversity is strongly positively correlated with continental fragmentation whereas diversity fluctuations of marine actinopterygians are predominantly affected by sea surface temperature variations. It could be expected that differences between both marine clades be due to the different time interval covered by the elasmobranch (Upper Triassic-Recent) and actinopterygian (Upper Jurassic-Paleocene) datasets. However, analyses ran with the elasmobranch dataset over the Upper Jurassic-Paleocene interval still indicate that continental fragmentation has a strong and significant positive correlation coefficient in all best fit models (Supplementary Data 7).
Discussion
A positive relationship between diversity and continental flooding, through eustatic sea level change, has been observed for both marine invertebrates (Peters, 2005; Hannisdal and Peters, 2011) and marine tetrapods (Benson and Butler, 2011; Butler et al., 2011; Mannion et al., 2015). Continental flooding has been considered as a potential driver of marine diversity through a species-area relationship (Peters, 2005) by controlling the extent of epicontinental seas [although the relationship might be more complex, see Holland (2012)], which host most of the marine vertebrate and invertebrate diversity today (Tittensor et al., 2010). A previous macroevolutionary analysis on marine vertebrates indicated that sea level is positively correlated with the diversity of shallow marine tetrapods, but showed a weaker relationship with open sea taxa (Benson and Butler, 2011). It is likely that the positive correlation found with marine actinopterygians and elasmobranchs reflects the dominance of shallow water taxa in the clades analyzed here. Numerous studies have suggested or found a link between sea level (or continental flooding) and sampling bias metrics (Sepkoski, 1976; Peters and Foote, 2001; Smith, 2001; Peters, 2005, 2006; Benton and Emerson, 2007), which led to establishing the “common cause” hypothesis (Peters, 2005). However, our results indicate a lower fit for models that include a sampling proxy, suggesting that sea level has a genuine biological effect and represents an important driver of the long-term fluctuations of marine biodiversity.
Although sea level likely played a role in shaping the evolutionary history of marine fish clades, our results indicate that other abiotic variables had stronger effects on their paleobiodiversity variations. We found that marine actinopterygian diversity correlates strongly negatively with δ18O values, which are considered as an inverse proxy for global climate change that mainly reflects temperature fluctuations (also global ice volume during icehouse periods) (Zachos et al., 2008). The positive link between temperature and diversity has probably been the most widely recovered relationship in macroevolutionary studies on both marine and terrestrial groups and across many lineages (Aguirre and Riding, 2005; Hunt et al., 2005; Marx and Uhen, 2010; Ezard et al., 2011; Figueirido et al., 2012; Mayhew et al., 2012; Condamine et al., 2013, 2019; Martin et al., 2014; De Blasio et al., 2015; Mannion et al., 2015). Temperature is also recognized as acting on the spatial distribution of biodiversity in modern ecosystems through the latitudinal diversity gradient (Willig et al., 2003; Hillebrand, 2004; Tittensor et al., 2010). Although considering a direct relationship between climate and available energy might be an oversimplified view (Clarke and Gaston, 2006; Erwin, 2009), empirical data indicate that increasing energetic resources provided by warmer climates have increased mutation rates and shortened generation times (Rohde, 1992; Gillooly et al., 2002) and hence supported faster speciation and/or lower extinction rates (Allen et al., 2006). The correlation recovered here provides additional evidence for a positive effect of temperatures on clades' diversification and quantitatively confirms previous hypotheses that marine ray-finned fish diversity variations were linked with sea surface temperatures (Cavin and Forey, 2007; Cavin et al., 2007). This is exemplified by the tremendous Cenomanian and Palaeocene-Eocene actinopterygian diversifications (Guinot and Cavin, 2016), which occurred during hyperthermal events (Zachos et al., 2008; O'Brien et al., 2017). In addition, it has been demonstrated that higher seawater temperatures tend to shorten both planktonic larval and egg duration times in marine organisms (Hirst and Lopez-Urrutia, 2006; Duarte, 2007; O'Connor et al., 2007). Shorter planktonic larval and egg phases imply decreasing dispersal capabilities, which promotes isolation and speciation (Duarte, 2007; O'Connor et al., 2007). The vast majority of ray-finned fish groups include larval phase and most comprise a free/pelagic egg phase in their development (Kendall et al., 1984). This temperature-sensitive trait may partly explain the stronger effect of climate on actinopterygian diversity than on elasmobranchs, which produce less egg cases (or youngs per liters) and have no larval or free egg phases. However, a recent species-level analysis provided evidence that lamniform extinction rates were negatively correlated with temperature (Condamine et al., 2019), which indicates that this parameter also played a role in some clades of mostly pelagic elasmobranchs.
Nonetheless, our analyses show that elasmobranch diversity variations most strongly correlate with continental fragmentation, suggesting a first-order effect of this environmental parameter on elasmobranch diversification patterns. Plate tectonics has long been hypothesized to have impacted global biodiversity (Valentine and Moores, 1970) by reducing biodiversity during periods of continental coalescence and increasing biodiversity during breakup of continental masses. Under this hypothesis, continental fragmentation produces geographic barriers that lead to the evolution of new lineages via vicariance, which contributes to the global biodiversity increase. In the marine realm, continental fragmentation also produces more coastlines and neritic environments that are known to correlate with the taxic diversity of coastal groups in modern ecosystems (Tittensor et al., 2010). However, this plate tectonic regulation hypothesis lacked quantitative testing in a macroevolutionary context until a recent analysis demonstrated that the long-term trends in biodiversity of Phanerozoic skeletonized marine invertebrates are well predicted by the supercontinent coalescence-breakup cycle (Zaffos et al., 2017). The temporal similarities between the fluctuations of elasmobranch biodiversity and the continental coalescence-breakup cycles (Figures 1A,D) is exemplified by the almost continuous Jurassic-Late Cretaceous diversity increase that matches a steady rise in continental fragmentation, which reflects the breakup of Pangaea and particularly of Gondwana (Zaffos et al., 2017). This result on a marine vertebrate clade supports previous invertebrate-based evidence that the shifting distribution of continental landmasses has been a major driver of long-term global Phanerozoic biodiversity patterns (Valentine and Moores, 1970, 1972; Zaffos et al., 2017). However, we found no significant effect of continental fragmentation on the biodiversity variations of marine actinopterygians, which, added to our results for temperature, indicates contrasting responses to long-term environmental changes between these two clades. Living elasmobranch species are dominated by benthic and demersal taxa (86.7%), while these ecologies represent minor components (45.8%) of marine actinopterygian diversity (Supplementary Data 2). Fossil-based estimates of the ecological distribution of Mesozoic ray-finned fishes remain to be completed. However, the large post-Mesozoic diversification of reef-associated demersal fish lineages (Alfaro et al., 2007; Cowman and Bellwood, 2011) and the absence during the Mesozoic of typical demersal clades such as the flatfishes (Pleuronectiformes) (Friedman, 2008), or of extinct clades with similar ecology, indicate that the demersal component of ray-finned fishes was even lower during the Mesozoic than during the Cenozoic. These results are consistent with the expectation that habitat area and geographic barriers more strongly influence species richness of clades dominated by benthic taxa, which are more prone to vicariance due to limited dispersal capabilities (Hart and Pearson, 2011). Finally, we note that δ34S correlates positively with elasmobranch diversity, but statistical significance is not present in all datasets. Although regarded as a proxy for organic nutrient inputs or shelf redox conditions (Cárdenas and Harries, 2010; Mayhew et al., 2012), the ecological significance of δ34S is contentious (Cárdenas and Harries, 2010; Hannisdal and Peters, 2011) and a full understanding of the direct link between this parameter and biodiversity variations requires further study. Apart from sea level, which appears to be a common driver in the marine groups investigated here, our results indicate that different abiotic parameters played a role between the macroevolutionary history of elasmobranchs (continental fragmentation) and marine actinopterygians (climate change).
An analysis of the fish family-level diversity dynamics demonstrated that both marine actinopterygians and elasmobranchs follow a similar equilibrium model of diversity variation (Guinot and Cavin, 2015). This apparent contradiction with the present analyses may relate to the use of different taxonomic levels as it has been proposed that logistic diversity curves prevail for higher taxonomic ranks and gradually change toward an exponential distribution when lower levels are considered (Benton, 1997; Lane and Benton, 2003; Benton and Emerson, 2007). Although post-Paleocene genus-level diversity data are lacking for actinopterygians, our pre-Eocene data (Figure 1B) suggest that such contrasting taxonomic-level patterns might occur in marine ray-finned fishes. Yet, the genus-level elasmobranch diversification pattern also fits an equilibrium model (Guinot and Cavin, 2015), and our results indicate that this equilibrium model is mainly tectonically driven. This suggests that continental fragmentation probably drove higher-level taxonomic richness, which can be regarded as reflecting key morphological or physiological adaptations (Guinot and Cavin, 2015), and that diversification patterns at lower taxonomic levels are influenced by different parameters according to the ecology of the clades.
Another noticeable result is the absence of correlation between the tested variables (continental fragmentation, sea level, δ18O and 87Sr/89Sr) and the long-term freshwater ray-finned fish diversity fluctuations. This result should be parallelized with previous family-level analysis showing that the diversity dynamics of freshwater ray-finned fishes do not follow that of their marine relatives, i.e., the variation is better described with an exponential function rather than with a logistic one (Guinot and Cavin, 2015). Potential causes at the origin of this pattern are proposed and discussed by Guinot and Cavin (2015). The present study highlights the fact that continental fish diversity may be under control of abiotic parameters that are unquantifiable at the macro scale (e.g., modifications of regional hydrographic systems, orogenesis). In addition, biotic factors such as competition and predation, which are known to enhance speciation and to be more intense in the freshwater realm (Vermeij and Grosberg, 2010), may play a preponderant role in shaping continental fish diversity. It has been proposed that sea level/continental flooding may have exerted a control on the diversity variations of continental clades on the basis of the well-established species-area relationship (Barnosky et al., 2005), but none of the analyses that quantitatively tested this hypothesis provided significant results (Butler et al., 2011; Mannion et al., 2011). Our results on continental actinopterygians lend support to the absence of a long-term effect of sea level on diversity in the continental realm, although some large variations probably punctually affected diversity changes (Hallam, 1989; Hallam and Wignall, 1999).
Our analyses cover all available abiotic parameters that are so far quantifiable and proposes potential environmental drivers and their outcomes on the two most speciose vertebrate clades in today's aquatic realms. The contrasting response of different “fish” clades to extrinsic parameters indicates that biological factors such as life-history traits and ecology likely control the response of clades to environmental change. Many macroevolutionary studies have analyzed the drivers of the Phanerozoic marine invertebrate clades as a whole (Cárdenas and Harries, 2010; Mayhew et al., 2012), with no distinction between groups (taxonomic, biological, physiological, ecological). Based on our results, it is expected that results found in such Phanerozoic marine invertebrate analyses are unbalanced by dominant ecologies among marine invertebrates and that the real patterns of abiotic control on deep-time biodiversity variations are more complex than previously expected. Although this is a first step in the understanding of what shapes the evolutionary history of clades, biotic interactions including competition (Liow et al., 2015; Silvestro et al., 2015; Voje et al., 2015) and predation (Huntley and Kowalewski, 2007; Marx and Uhen, 2010) have been more scarcely tested but have been demonstrated to play a role in the diversification patterns of clades, including sharks (Condamine et al., 2019). Exploring these intrinsic biotic effects represent another challenge for future studies, as is the understanding of the long-term drivers of continental fish diversity.
Data Availability Statement
All datasets generated for this study are included in the article/Supplementary Material.
Author Contributions
GG and LC designed the study and wrote the manuscript. GG compiled the data and performed the analyses.
Funding
This paper is a contribution to the project Fish response to long-term global change supported by the Swiss National Science Foundation (200021-140827).
Conflict of Interest
The authors declare that the research was conducted in the absence of any commercial or financial relationships that could be construed as a potential conflict of interest.
Acknowledgments
We wish to thank Emmanuel Paradis and Roger Benson for helpful discussions on the methodology. Fabien Condamine is thanked for his support and fruitful discussions as well as the two reviewers for providing useful comments on an earlier version of the manuscript.
Supplementary Material
The Supplementary Material for this article can be found online at: https://www.frontiersin.org/articles/10.3389/fevo.2019.00513/full#supplementary-material
Supplementary Data 1. Abiotic and diversity data used in the analyses.
Supplementary Data 2. Ecological data for living marine actinopterygian and elasmobranch species.
Supplementary Data 3. Results of generalized least square analyses on elasmobranch data.
Supplementary Data 4. Results of generalized least square analyses on marine actinopterygian data.
Supplementary Data 5. Results of generalized least square analyses on freshwater actinopterygian data.
Supplementary Data 6. Results of generalized least square analyses on PDE data of elasmobranch, marine, and freshwater data using the number of fossil collections as proxy for sampling.
Supplementary Data 7. Results of generalized least square analyses on elasmobranch data over the Oxfordian-Thanetian interval.
References
Aguirre, J., and Riding, R. (2005). Dasycladalean algal biodiversity compared with global variations in temperature and sea level over the past 350 Myr. Palaios 20, 581–588. doi: 10.2110/palo.2004.p04-33
Akima, H., and Gebhardt, A. (2016). Akima: Interpolation of Irregularly and Regularly Spaced Data. R package version 0.6−2. Available online at: https://CRAN.R-project.org/package=akima
Alfaro, M. E., Santini, F., Brock, C., Alamillo, H., Dornburg, A., Rabosky, D. L., et al. (2009). Nine exceptional radiations plus high turnover explain species diversity in jawed vertebrates. Proc. Natl. Acad. Sci. U.S.A. 106, 13410–13414. doi: 10.1073/pnas.0811087106
Alfaro, M. E., Santini, F., and Brock, C. D. (2007). Do reefs drive diversification in marine teleosts? Evidence from pufferfishes and their allies (order Tetraodontiformes). Evolution 61–69, 2104–2126. doi: 10.1111/j.1558-5646.2007.00182.x
Allen, A. P., Gillooly, J. F., Savage, V. M., and Brown, J. H. (2006). Kinetic effects of temperature on rates of genetic divergence and speciation. Proc. Natl. Acad. Sci. U.S.A. 103, 9130–9135. doi: 10.1073/pnas.0603587103
Alroy, J. (2014). Accurate and precise estimates of origination and extinction rates. Paleobiology 40, 374–397. doi: 10.1666/13036
Alroy, J., Aberhan, M., Bottjer, D. J., Foote, M., Fursich, F. T., Harries, P. J., et al. (2008). Phanerozoic trends in the global diversity of marine invertebrates. Science 321, 97–100. doi: 10.1126/science.1156963
Barnosky, A. D., Carrasco, M. A., and Davis, E. B. (2005). The impact of the Species–Area relationship on estimates of paleodiversity. PLoS Biol. 3:e266. doi: 10.1371/journal.pbio.0030266
Benson, R. B. J., and Butler, R. J. (2011). Uncovering the diversification history of marine tetrapods: ecology influences the effect of geological sampling biases. Geol. Soc. Lond. Spec. Publ. 358, 191–208. doi: 10.1144/SP358.13
Benton, M. J. (1997). Models for the diversification of life. Trends Ecol. Evol. 12, 490–495. doi: 10.1016/S0169-5347(97)84410-2
Benton, M. J., and Emerson, B. C. (2007). How did life become so diverse? The dynamics of diversification according to the fossil record and molecular phylogenetics. Palaeontology 50, 23–40. doi: 10.1111/j.1475-4983.2006.00612.x
Boettiger, C., Lang, D. T., and Wainwright, P. C. (2012). rfishbase: exploring, manipulating and visualizing FishBase data from R. J. Fish Biol. 81, 2030–2039. doi: 10.1111/j.1095-8649.2012.03464.x
Boyd, C. A., Cleland, T. P., Marrero, N. L., and Clarke, J. A. (2011). Exploring the effects of phylogenetic uncertainty and consensus trees on stratigraphic consistency scores: a new program and a standardized method. Cladistics 27, 52–60. doi: 10.1111/j.1096-0031.2010.00320.x
Breusch, T. S., and Pagan, A. R. (1979). A simple test for heteroscedasticity and random coefficient variation. Econometrica 47, 1287–1294. doi: 10.2307/1911963
Butler, R. J., Benson, R. B. J., Carrano, M. T., Mannion, P. D., and Upchurch, P. (2011). Sea level, dinosaur diversity and sampling biases: investigating the ‘common cause’ hypothesis in the terrestrial realm. Proc. R. Soc. Lond. B Biol. Sci. 278, 1165–1170. doi: 10.1098/rspb.2010.1754
Cárdenas, A. L., and Harries, P. J. (2010). Effect of nutrient availability on marine origination rates throughout the Phanerozoic eon. Nature Geosci. 3, 430–434. doi: 10.1038/ngeo869
Cavin, L., and Forey, P. L. (2007). Using ghost lineages to identify diversification events in the fossil record. Biol. Lett. 3, 201–204. doi: 10.1098/rsbl.2006.0602
Cavin, L., Forey, P. L., and Lecuyer, C. (2007). Correlation between environment and Late Mesozoic ray-finned fish evolution. Palaeogeogr. Palaeoclimatol. Palaeoecol. 245, 353–367. doi: 10.1016/j.palaeo.2006.08.010
Clarke, A., and Gaston, K. J. (2006). Climate, energy and diversity. Proc. R. Soc. B 273, 2257–2266. doi: 10.1098/rspb.2006.3545
Condamine, F. L., Rolland, J., and Morlon, H. (2013). Macroevolutionary perspectives to environmental change. Ecol. Lett. 16(Suppl. 1), 72–85. doi: 10.1111/ele.12062
Condamine, F. L., Romieu, J., and Guinot, G. (2019). Climate cooling and clade competition likely drove the decline of lamniform sharks. Proc. Natl. Acad. Sci. U.S.A. 116:201902693. doi: 10.1073/pnas.1902693116
Cowman, P. F., and Bellwood, D. R. (2011). Coral reefs as drivers of cladogenesis: expanding coral reefs, cryptic extinction events, and the development of biodiversity hotspots. J. Evol. Biol. 24, 2543–2562. doi: 10.1111/j.1420-9101.2011.02391.x
De Blasio, F. V., Liow, L. H., Schweder, T., and De Blasio, B. F. (2015). A model for global diversity in response to temperature change over geological time scales, with reference to planktic organisms. J. Theor. Biol. 365, 445–456. doi: 10.1016/j.jtbi.2014.10.031
Duarte, C. M. (2007). Marine ecology warms up to theory. Trends Ecol. Evol. 22, 331–333. doi: 10.1016/j.tree.2007.04.001
Ebert, D. A., Fowler, S., and Compagno, L. (2013). Sharks of the World: A Fully Illustrated Guide. Plymouth: Wild Nature Press.
Erwin, D. H. (2009). Climate as a driver of evolutionary change. Current Biol. 19, R575–R583. doi: 10.1016/j.cub.2009.05.047
Ezard, T. H. G., Aze, T., Pearson, P. N., and Purvis, A. (2011). Interplay between changing climate and species' ecology drives macroevolutionary dynamics. Science 332, 349–351. doi: 10.1126/science.1203060
Figueirido, B., Janis, C. M., Pérez-Claros, J. A., Renzi, M. D., and Palmqvist, P. (2012). Cenozoic climate change influences mammalian evolutionary dynamics. Proc. Natl. Acad. Sci. U.S.A. 109, 722–727. doi: 10.1073/pnas.1110246108
Friedman, M. (2008). The evolutionary origin of flatfish asymmetry. Nature 454, 209–212. doi: 10.1038/nature07108
Froese, R., and Pauly, D. (2019). FishBase. World Wide Web electronic publication version (07/2019). Available online at: www.fishbase.org (accessed January 07, 2019).
Gillooly, J. F., Charnov, E. L., West, G. B., Savage, V. M., and Brown, J. H. (2002). Effects of size and temperature on developmental time. Nature 417, 70–73. doi: 10.1038/417070a
Guinot, G., and Cavin, L. (2015). Contrasting “Fish” diversity dynamics between marine and freshwater environments. Curr. Biol. 25, 2314–2318. doi: 10.1016/j.cub.2015.07.033
Guinot, G., and Cavin, L. (2016). ‘Fish’ (Actinopterygii and Elasmobranchii) diversification patterns through deep time. Biol Rev. 91, 950–981. doi: 10.1111/brv.12203
Hallam, A. (1989). The case for sea-level change as a dominant causal factor in mass extinction of marine invertebrates. Philos. Trans. R. Soc. B 325, 437–455. doi: 10.1098/rstb.1989.0098
Hallam, A., and Wignall, P. B. (1999). Mass extinctions and sea-level changes. Earth Sci. Rev. 48, 217–250. doi: 10.1016/S0012-8252(99)00055-0
Hannisdal, B., and Peters, S. E. (2011). Phanerozoic earth system evolution and marine biodiversity. Science 334, 1121–1124. doi: 10.1126/science.1210695
Haq, B. U., Hardenbol, J., and Vail, P. (1987). Chronology of fluctuating sea levels since the Triassic. Science 235, 1156–1167. doi: 10.1126/science.235.4793.1156
Hart, P. J. B., and Pearson, E. (2011). An application of the theory of island -biogeography to fish speciation on seamounts. Mar. Ecol. Prog. Ser. 430, 281–288. doi: 10.3354/meps08948
Hillebrand, H. (2004). On the generality of the latitudinal diversity gradient. Am. Nat. 163, 192–211. doi: 10.1086/381004
Hirst, A., and Lopez-Urrutia, A. (2006). Effects of evolution on egg development time. Mar. Ecol. Prog. Ser. 326, 29–35. doi: 10.3354/meps326029
Holland, S. M. (2012). Sea level change and the area of shallow-marine habitat: implications for marine biodiversity. Paleobiology 38, 205–217. doi: 10.1666/11030.1
Hunt, G., Cronin, T. M., and Roy, K. (2005). Species–energy relationship in the deep sea: a test using the Quaternary fossil record. Ecol. Lett. 8, 739–747. doi: 10.1111/j.1461-0248.2005.00778.x
Huntley, J. W., and Kowalewski, M. (2007). Strong coupling of predation intensity and diversity in the Phanerozoic fossil record. Proc. Natl. Acad. Sci. U.S.A. 104, 15006–15010. doi: 10.1073/pnas.0704960104
Jarque, C. M., and Bera, A. K. (1980). Efficient tests for normality, homoscedasticity and serial independence of regression residuals. Econ. Lett. 6, 255–259. doi: 10.1016/0165-1765(80)90024-5
Kendall, A. W. Jr., Ahlstrom, E. H., and Moser, H. G. (1984). “Early life history stages of fishes and their characters,” in Ontogeny and Systematics of Fishes, eds H. G. Moser, W. J. Richards, D. M. Cohen, M. P. Fahay, A. W. Jr. Kendall, and S. L. Richardson (Lawrence, KS), 11–22. Available online at: https://ci.nii.ac.jp/naid/10010414274 (accessed August 28, 2019).
Lane, A., and Benton, M. J. (2003). Taxonomic level as a determinant of the shape of the Phanerozoic marine biodiversity curve. Am. Nat 162, 265–276. doi: 10.1086/377188
Last, P., White, W., Séret, B., Naylor, G., Carvalho, M., and de Stehmann, M. (2016). Rays of the World. Clayton, MO: Csiro Publishing. doi: 10.1071/9780643109148
Liow, L. H., Reitan, T., and Harnik, P. G. (2015). Ecological interactions on macroevolutionary time scales: clams and brachiopods are more than ships that pass in the night. Ecol. Lett. 18, 1030–1039. doi: 10.1111/ele.12485
Long, J. S., and Ervin, L. H. (2000). Using heteroscedasticity consistent standard errors in the linear regression model. Am. Stat. 54, 217–224. doi: 10.1080/00031305.2000.10474549
Mannion, P. D., Benson, R. B. J., Carrano, M. T., Tennant, J. P., Judd, J., and Butler, R. J. (2015). Climate constrains the evolutionary history and biodiversity of crocodylians. Nat. Commun. 6:8438. doi: 10.1038/ncomms9438
Mannion, P. D., Upchurch, P., Carrano, M. T., and Barrett, P. M. (2011). Testing the effect of the rock record on diversity: a multidisciplinary approach to elucidating the generic richness of sauropodomorph dinosaur richness. Biol. Rev. 86, 157–181. doi: 10.1111/j.1469-185X.2010.00139.x
Martin, J. E., Amiot, R., Lécuyer, C., and Benton, M. J. (2014). Sea surface temperature contributes to marine crocodylomorph evolution. Nat. Commun. 5:4658. doi: 10.1038/ncomms5658
Martin, R. E. (1996). Secular Increase in nutrient levels through the phanerozoic: implications for productivity, biomass, and diversity of the marine biosphere. Palaios 11, 209–219. doi: 10.2307/3515230
Marx, F. G., and Uhen, M. D. (2010). Climate, critters, and cetaceans: Cenozoic drivers of the evolution of modern whales. Science 327, 993–996. doi: 10.1126/science.1185581
Mayhew, P. J., Bell, M. A., Benton, T. G., and McGowan, A. J. (2012). Biodiversity tracks temperature over time. Proc. Natl. Acad. Sci. U.S.A. 109, 15141–15145. doi: 10.1073/pnas.1200844109
Mayhew, P. J., Jenkins, G. B., and Benton, T. G. (2008). A long-term association between global temperature and biodiversity, origination and extinction in the fossil record. Proc. R. Soc. B 275, 47–53. doi: 10.1098/rspb.2007.1302
Miller, K. G., Kominz, M. A., Browning, J. V., Wright, J. D., Mountain, G. S., Katz, M. E., et al. (2005). The Phanerozoic record of global sea-level change. Science 310, 1293–1298. doi: 10.1126/science.1116412
Norell, M. A. (1993). Tree-based approaches to understanding history; comments on ranks, rules and the quality of the fossil record. Am. J. Sci. 293, 407–417. doi: 10.2475/ajs.293.A.407
O'Brien, C. L., Robinson, S. A., Pancost, R. D., Sinninghe Damsté, J. S., Schouten, S., Lunt, D. J., et al. (2017). Cretaceous sea-surface temperature evolution: constraints from TEX86 and planktonic foraminiferal oxygen isotopes. Earth-Sci. Rev. 172, 224–247. doi: 10.1016/j.earscirev.2017.07.012
O'Connor, M. I., Bruno, J. F., Gaines, S. D., Halpern, B. S., Lester, S. E., Kinlan, B. P., et al. (2007). Temperature control of larval dispersal and the implications for marine ecology, evolution, and conservation. Proc. Natl. Acad. Sci. U.S.A. 104, 1266–1271. doi: 10.1073/pnas.0603422104
Peters, S. E. (2005). Geologic constraints on the macroevolutionary history of marine animals. Proc. Natl. Acad. Sci. U.S.A. 102, 12326–12331. doi: 10.1073/pnas.0502616102
Peters, S. E. (2006). Genus extinction, origination, and the durations of sedimentary hiatuses. Paleobiology 32, 387–407. doi: 10.1666/05081.1
Peters, S. E., and Foote, M. (2001). Biodiversity in the Phanerozoic: a reinterpretation. Paleobiology 27, 583–601. doi: 10.1666/0094-8373(2001)027<0583:BITPAR>2.0.CO;2
Pol, D., and Norell, M. A. (2001). Comments on the manhattan stratigraphic measure. Cladistics 17, 285–289. doi: 10.1006/clad.2001.0166
Prokoph, A., Shields, G. A., and Veizer, J. (2008). Compilation and time-series analysis of a marine carbonate δ18O, δ13C, 87Sr/86Sr and δ34S database through earth history. Earth Sci. Rev. 87, 113–133. doi: 10.1016/j.earscirev.2007.12.003
Raup, D. M. (1976). Species diversity in the Phanerozoic: an interpretation. Paleobiology 2, 289–297. doi: 10.1017/S0094837300004929
Raymo, M. E., and Ruddiman, W. F. (1992). Tectonic forcing of late cenozoic climate. Nature 359, 117–122. doi: 10.1038/359117a0
Rohde, K. (1992). Latitudinal gradients in species diversity: the search for the primary cause. Oikos 65, 514–527. doi: 10.2307/3545569
Sepkoski, J. J. (1976). Species diversity in the Phanerozoic: species-area effects. Paleobiology 2, 298–303. doi: 10.1017/S0094837300004930
Shields, G. A. (2007). A normalised seawater strontium isotope curve: possible implications for Neoproterozoic-Cambrian weathering rates and the further oxygenation of the Earth. eEarth 2, 35–42. doi: 10.5194/ee-2-35-2007
Silvestro, D., Antonelli, A., Salamin, N., and Quental, T. B. (2015). The role of clade competition in the diversification of North American canids. Proc. Natl. Acad. Sci. U.S.A. 112, 8684–8689. doi: 10.1073/pnas.1502803112
Silvestro, D., Schnitzler, J., Liow, L. H., Antonelli, A., and Salamin, N. (2014). Bayesian estimation of speciation and extinction from incomplete fossil occurrence data. Syst. Biol. 63, 349–67. doi: 10.1093/sysbio/syu006
Smith, A. B. (2001). Large-scale heterogeneity of the fossil record: implications for Phanerozoic biodiversity studies. Philos. Trans. R. Soc. B 356, 351–367. doi: 10.1098/rstb.2000.0768
Tittensor, D. P., Mora, C., Jetz, W., Lotze, H. K., Ricard, D., Berghe, E. V., et al. (2010). Global patterns and predictors of marine biodiversity across taxa. Nature 466, 1098–1101. doi: 10.1038/nature09329
Valentine, J. W., and Moores, J. W. (1970). Plate-tectonic regulation of faunal diversity and sea level: a model. Nature 228, 657–659. doi: 10.1038/228657a0
Valentine, J. W., and Moores, J. W. (1972). Global tectonics and the fossil record. J. Geol. 80, 167–184. doi: 10.1086/627723
Veizer, J., and Prokoph, A. (2015). Temperatures and oxygen isotopic composition of Phanerozoic oceans. Earth Sci. Rev. 146, 92–104. doi: 10.1016/j.earscirev.2015.03.008
Vermeij, G. J., and Grosberg, R. K. (2010). The great divergence: when did diversity on land exceed that in the sea? Integr. Comp. Biol. 50, 675–682. doi: 10.1093/icb/icq078
Voje, K. L., Holen, Ø. H., Liow, L. H., and Stenseth, N. C. (2015). The role of biotic forces in driving macroevolution: beyond the Red Queen. Proc. R. Soc. B 282:20150186. doi: 10.1098/rspb.2015.0186
White, H. (1980). A heteroskedasticity-consistent covariance matrix estimator and a direct test for heteroskedasticity. Econometrica 48, 817–838. doi: 10.2307/1912934
Wickham, H. (2017). Scales: Scale Functions for Visualization. R package version 0.5.0. Available online at: https://CRAN.R-project.org/package=scales (accessed July 01, 2019).
Willig, M. R., Kaufman, D. M., and Stevens, R. D. (2003). Latitudinal gradients of biodiversity: pattern, process, scale, and synthesis. Annu. Rev. Ecol. Evol. Syst. 34, 273–309. doi: 10.1146/annurev.ecolsys.34.012103.144032
Wills, M. A. (1999). Congruence between phylogeny and stratigraphy: randomization tests and the gap excess ratio. Syst. Biol. 48, 559–580. doi: 10.1080/106351599260148
Zachos, J. C., Dickens, G. R., and Zeebe, R. E. (2008). An early Cenozoic perspective on greenhouse warming and carbon-cycle dynamics. Nature 451, 279–283. doi: 10.1038/nature06588
Keywords: macroevolution, abiotic factors, actinopterygians, chondrichthyans, biodiversity, climate
Citation: Guinot G and Cavin L (2020) Distinct Responses of Elasmobranchs and Ray-Finned Fishes to Long-Term Global Change. Front. Ecol. Evol. 7:513. doi: 10.3389/fevo.2019.00513
Received: 10 October 2019; Accepted: 17 December 2019;
Published: 23 January 2020.
Edited by:
Zerina Johanson, Natural History Museum, United KingdomReviewed by:
Sebastian Stumpf, University of Vienna, AustriaMichael James Benton, University of Bristol, United Kingdom
Copyright © 2020 Guinot and Cavin. This is an open-access article distributed under the terms of the Creative Commons Attribution License (CC BY). The use, distribution or reproduction in other forums is permitted, provided the original author(s) and the copyright owner(s) are credited and that the original publication in this journal is cited, in accordance with accepted academic practice. No use, distribution or reproduction is permitted which does not comply with these terms.
*Correspondence: Guillaume Guinot, Z3VpbGxhdW1lLmd1aW5vdCYjeDAwMDQwO3Vtb250cGVsbGllci5mcg==