- 1Swansea Lab for Animal Movement, Biosciences, College of Science, Swansea University, Swansea, United Kingdom
- 2Fundación Oceanogràfic de la Comunitat Valenciana, Valencia, Spain
- 3Red Sea Research Centre and Computational Biology Research Centre, King Abdullah University of Science and Technology, Thuwal, Saudi Arabia
- 4Future Ocean Cluster of Excellence, GEOMAR Helmholtz Centre for Ocean Research, Kiel, Germany
Vertebrates are recognized as sentient beings. Consequently, urgent priority is now being given to understanding the needs and maximizing the welfare of animals under human care. The general health of animals is most commonly determined by physiological indices e.g., blood sampling, but may also be assessed by documenting behavior. Physiological health assessments, although powerful, may be stressful for animals, time-consuming and costly, while assessments of behavior can also be time-consuming, subject to bias and suffer from a poorly defined link between behavior and health. However, behavior is recognized as having the potential to code for stress and well-being and could, therefore, be used as an indicator of health, particularly if the process of quantifying behavior could be objective, formalized and streamlined to be time efficient. This study used Daily Diaries (DDs) (motion-sensitive tags containing tri-axial accelerometers and magnetometers), to examine aspects of the behavior of bycaught loggerhead turtles, Caretta caretta in various states of health. Although sample size limited statistical analysis, significant behavioral differences (in terms of activity level and turn rate) were found between “healthy” turtles and those with external injuries to the flippers and carapace. Furthermore, data visualization (spherical plots) clearly showed atypical orientation behavior in individuals suffering gas emboli and intestinal gas, without complex data analysis. Consequently, we propose that the use of motion-sensitive tags could aid diagnosis and inform follow-up treatment, thus facilitating the rehabilitation process. This is particularly relevant given the numerous rehabilitation programs for bycatch sea turtles in operation. In time, tag-derived behavioral biomarkers, TDBBs for health could be established for other species with more complex behavioral repertoires such as cetaceans and pinnipeds which also require rehabilitation and release. Furthermore, motion-sensitive data from animals under human care and wild conspecifics could be compared in order to define a set of objective behavioral states (including activity levels) for numerous species housed in zoos and aquaria and/or wild species to help maximize their welfare.
Introduction
Animals are recognized as cognizant beings, with high priority now given to understanding behavioral requirements and maximizing animal welfare under human care (Hawkins, 2004; Boissy et al., 2007; Shorter et al., 2017). Generally, animal well-being is evaluated through physiological health assessments e.g., periodic blood sampling, which can cause animals distress and pain (Abou-Ismail et al., 2007; Burman et al., 2007; Scollo et al., 2014) whilst also being expensive and time consuming (Hawkins, 2004). However, animal health can also be assessed through behavior, requiring an understanding of differing behavioral states that result from factors like elevated stress, infirmity, and injury (Broom and Johnson, 1993; Rushen, 2003; Lawrence, 2008). At present, assessments of behavior can be time-consuming, subject to bias and suffer from a poorly defined link between behavior and well-being in general (Broom and Johnson, 1993; Rushen, 2003; Lawrence, 2008). Animal-attached technology may thus provide a solution to many of these issues, enabling the process of quantifying behavior to be formalized and streamlined to be time-efficient and objective (see Cooke et al., 2004; Ropert-Coudert and Wilson, 2005; Ellwood et al., 2007; Cooke, 2008; Guesgen and Bench, 2017).
The attachment of tags to animals, which started decades ago [as early as the 1960s in marine vertebrates (Kooyman, 2004)], has transformed our understanding of animal behavior and eco-physiology (Naito, 2004) and catalyzed the development of whole new disciplines such as movement ecology (Nathan et al., 2008). In particular, archival-, rather than transmission-tag technology [aka bio-logging, where multiple parameters are recorded (Ropert-Coudert and Wilson, 2005)], has demonstrated its use in helping transform our understanding of animal physiology (Block, 2005; Sherub et al., 2017), behavior (Brown et al., 2013), and ecology (Wilmers et al., 2015). Biologging also has huge potential in areas relating to animal well-being via studies on farmed animals, particularly cattle (Turner et al., 2000; Shirai and Yokoyama, 2014; Thorup et al., 2015), but also aquaculture (Andrewartha et al., 2015) and with respect to conservation (Cooke, 2008; Ropert-Coudert et al., 2009; Bograd et al., 2010; Wilson et al., 2015). Accelerometer biologgers have also proved valuable for tracking behavioral changes and the survival of various fish species (including blacktip sharks, Carcharhinus limbatus (Whitney et al., 2016); arapaima, Arapaima cf. arapaima (Lennox et al., 2018), and bonefish, Albula spp. (Brownscombe et al., 2013) post-release, following fishery-related and recreational capture. For farmers, tagging has a wide range of applications from locating animals that have escaped their paddocks and tracking resource consumption (Sikka et al., 2006), to detecting lameness (Thorup et al., 2015); activities which would usually require manpower and time. Recently biotelemetry has also gained popularity within pet caring practices with dog owners tracking their pets to know their whereabouts (Mancini et al., 2012).
Somewhat surprisingly, given the clear potential of biologging to monitor animal health, the tagging community has done relatively little work in zoos and aquaria (with the exception of some studies that have used animals under human care to help identify behaviors with a view to using loggers on wild animals (Shepard et al., 2008; Ismail et al., 2012). One notable study that does, however, report on the potential of logging technology to study the well-being of animals under human care, is that by Shorter et al. (2017), which examined the activity of a total of ca. 57 h of data derived from 5 bottlenose dolphins, Tursiops truncatus, using motion-sensing animal-attached tags (DTAGS, see Johnson and Tyack, 2003). Another study, on Koalas at a conservation center, used accelerometers in combination with electrocardiogram recorders to assess heart rate during periods of inactivity whilst animals where in the presence and absence of tourists (Ropert-Coudert et al., 2009). Otherwise the lack of tags on animals maintained in a controlled environment per se is particularly curious since tags are unlikely to be lost (Bidder et al., 2014), animals are easy to catch compared to their wild counterparts and can even be trained to participate voluntarily (Ward and Melfi, 2015; Shorter et al., 2017). Moreover, the issue of animal welfare is repeatedly raised within the context of zoos and aquaria (Hill and Broom, 2009). Indeed, many of the major issues discussed relating to animal welfare, such as the incidence of stereotypic behaviors (Mason and Rushen, 2008), stress (Wiepkema and Koolhaas, 1993), and assessing the extent to which behaviors exhibited by animals under human care conform to those of their wild conspecifics (Veasey et al., 1996), could potentially be well quantified by logger technology (Wilson et al., 2014; Pagano et al., 2017).
A decade ago, a multi-sensor archival tag, the “Daily Diary,” DD (which records tri-axial acceleration and tri-axial magnetometry, temperature and pressure), was conceived to quantify the behavior and ecology of threatened megafauna (Wilson et al., 2008). However, this tool has not been used, until now, to elucidate the link between animal behavior and health (Broom and Johnson, 1993; Rushen, 2003; Lawrence, 2008). Since behavioral state should relate to biomarkers of stress and well-being, the DD has the potential to be used to derive metrics which act as “behavioral biomarkers” of health (Broom, 1991; Lawrence, 2008) and form part of a less invasive diagnosis process (requiring no physiological samples).
The present study used DD loggers to examine aspects of the behavior of bycaught loggerhead turtles, Caretta caretta, housed in the “Arca del Mar” rehabilitation center at the Oceanogràfic aquarium, Valencia, Spain. Sea turtles being rehabilitated at the center commonly suffer bycatch-related external and/or internal injuries, including gas emboli (i.e., the formation of gas bubbles within the blood stream and tissues) and decompression sickness (Portugues et al., 2018). No animal was caught for the purpose of this study. The aim of this study was to investigate whether tag-derived “behavioral biomarkers” (TDBBs) for health could be established by monitoring changes in movement behaviors determined by multi-sensor tags and validated through conventional health assessments during sea turtle rehabilitation. We hypothesized that specific behavioral aspects would vary in accordance with a particular illness/injury, thus enabling the creation of TDBBs that could then be used to track recovery and potentially serve as diagnostic tools. This article also discusses how data from motion- and orientation-sensitive animal-attached tags might be used to derive useful metrics (such as activity level and turning rate) for assessing animal health and welfare in human controlled environments.
Method
Animals
All of the loggerhead turtles used in this study were accidentally captured (bycaught) in gillnet and trawling fisheries off the coast of eastern Spain except potentially: three that were found floating at the surface, two that were transferred from other aquariums and one that was found stranded (see Table 1). Sea turtles were brought to the facility by staff from the local strandings network; the duration from the point of accidental capture to arriving at the center was not known. All bycaught turtles from participating fishing boats were brought to the clinic even if the animal did not exhibit visible signs of disease or trauma. Sea turtles were typically brought to the center with a variety of bycatch-related external and/or internal injuries including gas emboli and decompression sickness (Garcia-Parraga et al., 2014; Portugues et al., 2018).
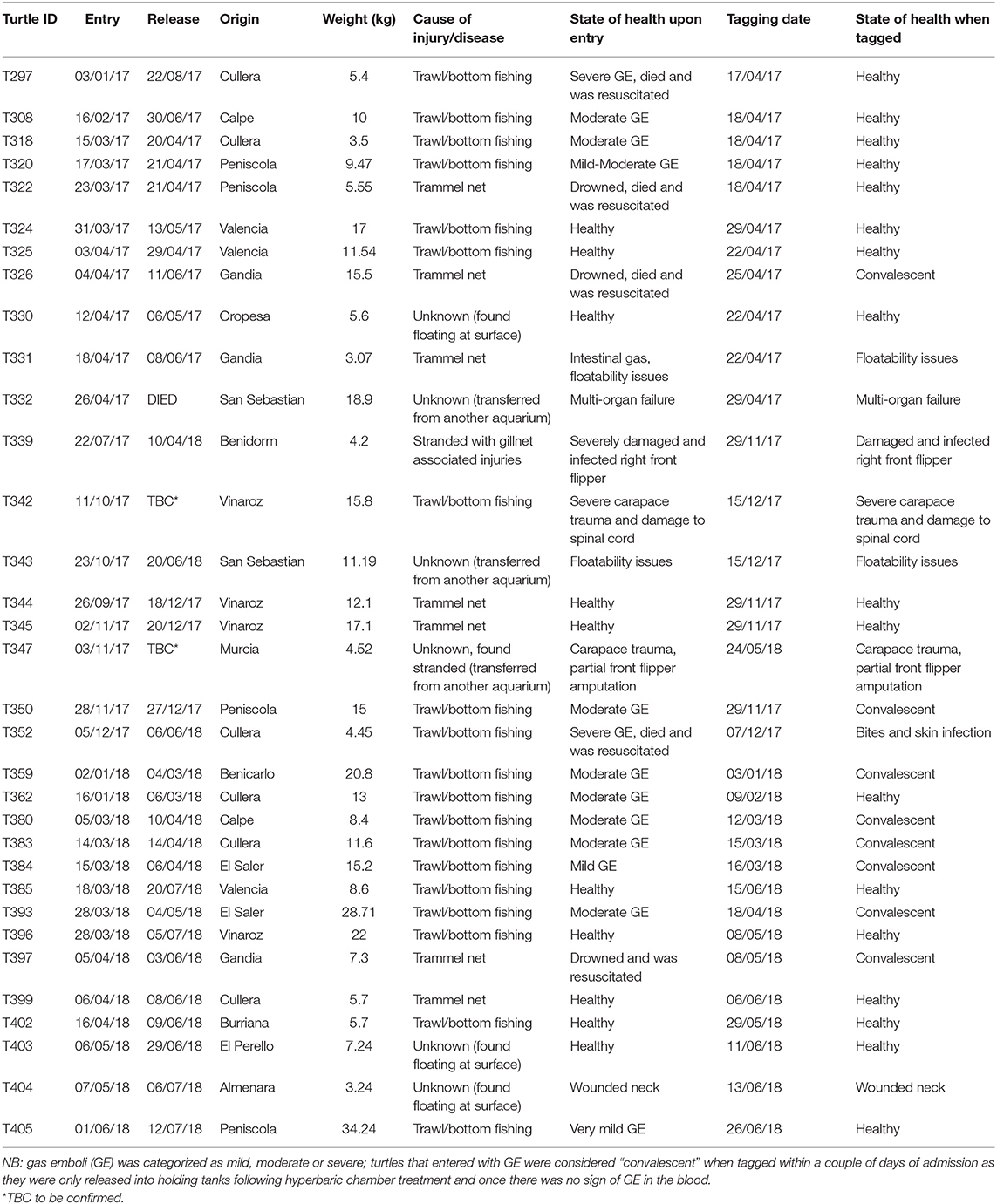
Table 1. Summary of tagged turtle data including: turtle identification number, entry to and release dates from the rehabilitation center, bycatch origin, weight (kg), cause of injury/disease (when known), and the animals' state of health upon entry, and on the date of tagging (as deduced via veterinary examination).
Veterinary Care
Clinical examination was carried out at the rehabilitation center, “Arca del Mar” which is managed by the Fundación Oceanogràfic in Valencia, Spain. The facility has a permit from the Valencian Regional Government for sea turtle rehabilitation (both bycaught and stranded) and post-mortem examination. Upon arrival, all turtles underwent a health assessment including a complete physical examination, blood sample collection and diagnostic imaging (radiographs and ultrasound). Vets used turtle entry number (a running count of the number of turtles admitted year on year) to identify individuals; for ease the same identification numbers were used in this manuscript. Turtle numbers were preceded by a T to help differentiate them from other numbers within the text. Sea turtles at the facility were housed in circular tanks, ranging from 2 to 6 m in diameter and with a water depth of 0.95 m. Two different filtration systems operated maintaining “A” and “B” tanks at temperatures of ~20°C and ~24°C, respectively, in order to acclimatize sea turtles to lower temperatures before they were released. All animals admitted were maintained at the rehabilitation center until they were deemed fit for release.
Gas Embolism and Decompression Sickness
It has recently been found that some bycaught loggerheads exhibit gas emboli within the blood stream and tissues and suffer symptoms of decompression sickness; afflicted animals have also been found to display anomalous behaviors ranging from being comatose to being hyperactive (Garcia-Parraga et al., 2014). Embolisms can lead to organ injury, impairment, and even animal mortality, especially in individuals with moderate to severe gas emboli that do not receive hyperbaric O2 treatment (Garcia-Parraga et al., 2014).
The presence and severity of gas emboli were determined by radiographs and ultrasound examination and scored on a 5-point scale: no intravascular gas detected, very mild, mild, moderate and severe (for further details see Garcia-Parraga et al., 2014; Fahlman et al., 2017). Animals with observable gas emboli received recompression therapy using pure O2 from a pressurized medical O2 cylinder. This hyperbaric oxygen treatment was administered via a custom-built hyperbaric chamber (41 × 77 cm, internal height and diameter). After recompression treatment (which was often administered overnight due to turtles arriving in the afternoon/evening), another health assessment was conducted to evaluate the resolution of gas emboli. Individuals were only placed in holding tanks once no gas emboli were detected in the blood (usually the morning after recompression treatment) and were considered to be in a state of recovery (convalescent) from that moment on. Turtles remained under daily supervision until their blood values and their feeding, swimming and diving behaviors were normal.
Tagging
Sea turtle behavior was studied by equipping animals with acceleration- and magnetic-field-measuring data-loggers [“Daily Diaries,” DD, housing dimensions 54 × 29 × 22 mm, mass 22 g, although there was some variation in size (Wilson et al., 2008)] recording at 20 Hz per channel. Devices measured both acceleration [logged with respect to gravity (~1 g), range; ± 16 g] and magnetic field intensity [recorded in Gauss (G) at 0.73 mG resolution, range; ± 0.88 G] in three orthogonal axes: heave (dorso-ventral), sway (lateral) and surge (anterior-posterior). In addition to describing behavior via body posture, body “vibrancy” (Halsey et al., 2011b) and body rotation (Williams et al., 2017), tags quantified proxies for energy expenditure (Dynamic Body Acceleration—DBA, specifically VeDBA (Halsey et al., 2011b), and the physical characteristics of the animal's environment, i.e., temperature and pressure (Wilson et al., 2008).
In order to attach the DDs, bycaught turtles were lifted out of their holding tank and placed onto a foam mat and/or into a plastic box. Tags were attached to the second central scute of the carapace with a two-part epoxy (Veneziani Subcoat S), pre-mixed in water. Animals were tagged opportunistically for deployment periods lasting just over a day, up to six consecutive days during April-May and November-May 2017–2018. When possible, turtles were tagged as soon as they were released into one of the holding tanks at the rehabilitation center. This varied according to condition; for healthy turtles and those with minor injuries, individuals could be admitted to a tank following a veterinary health assessment, whereas for turtles with gas emboli (which typically received hyperbaric treatment overnight), this was usually the day following recompression treatment. All protocols were approved by the Oceanogràfic Animal Care & Welfare Committee (OCE-16-18) and the Swansea University Animal Welfare Ethical Review Body (STU_BIOL_82015_011117151527_1). No medical procedures were conducted solely for research purposes.
Data Analysis
The data were analyzed using custom designed software “Daily Diary Multi Trace” (DDMT, http://wildbytetechnologies.com/software.html), R-Studio (version 3.6.0, R Foundation for Statistical Computing, Vienna, Austria, http://www.R-project.org/), the R packages “nlme,” [version 3.1–141 (Pinheiro and Bates, 2000; Pinheiro et al., 2015)] and “MuMIn,” [version 1.43.6 (Barton, 2019)] and Microsoft Excel (version 365). Data visualization with DDMT displayed sensor lines (tri-axial acceleration, tri-axial magnetic field intensity, pressure, temperature and derivatives—see below) on the y-axis against time on the x-axis as well as multi-dimensional plots that were used to reveal patterns in the data (Walker et al., 2016; Wilson et al., 2016; Williams et al., 2017). These took the form of spherical (tri-axial) plots where two axes (the horizontal axes) displayed two different parameters, such as time and animal body pitch, while the third, vertical, axis displayed a frequency count. This enabled the incidence of particular conditions to be examined easily. Certain parameters, such as pitch and roll, have values that describe a sphere, resulting in frequency histograms forming on the surface of a sphere.
Derivatives used for describing behaviors included the dynamic body acceleration (DBA), specifically the Vectorial Dynamic Body Acceleration (VeDBA), using methods described in Qasem et al. (2012), because DBA is a proxy for both energy expenditure in vertebrates in general (Halsey et al., 2011b) and loggerhead turtles in particular (Halsey et al., 2011a, cf. Enstipp et al., 2011), as well as being a useful general measure for activity (Gleiss et al., 2011). Another useful derivative when examining animal behavior is that of compass heading (i.e., orientation about the yaw axis or turning) (Bidder et al., 2015; Walker et al., 2015b; Williams et al., 2017). DDMT software uses calibration data to correct for iron distortions and tilt offsets prior to calculating heading on a scale of 0–360°. Any tilt of the DD causes a distortion in the compass heading values, which are corrected through the use of the static component of acceleration (due to gravity; 9.81 m/s−2), the animal's pitch and roll values, in relation to the output of the tri-axial magnetometers (Walker et al., 2016); also known as a tilt-compensated compass. For information regarding the stages and equations involved in the computation of pitch, roll and compass heading see Bidder et al. (2015) and Walker et al. (2015b). Subsequent analyses including mean VeDBA per hour and heading (specifically the number of turns per hour surpassing a threshold of 45°) were calculated using data undersampled from 20 Hz to 4 Hz; VeDBA and heading data was also smoothed over 2 s to reduce noise. VeDBA and heading were used in statistical analysis after data visualizations indicated differences in animal movement (pitch, roll and directionality) for turtles in various states of health and because the two parameters combined provided a straight-forward (and therefore easily applicable) but also reasonably comprehensive way of investigating potential differences in movement behavior.
A linear mixed-effects model (LMEM) was performed to see if turtle condition (included as a predictor) affected the relationship between the number of turns per hour (surpassing a threshold of 45°) and mean VeDBA per hour. A log transform was performed on both the dependent (VeDBA) and independent (turn rate) variables to normalize the data and turtle ID was incorporated into the model as a random effect to account for inter-individual differences (for example, turtle size and sex). Tank size was also included in the model to account for any confounding effects it might have; consequently, the model included all turtles (n = 22) tagged post July 2017 for which tank size (i.e., the available water mass) was known (see Table 1).
In order to perform the analysis, turtle condition was grouped into three categories: healthy (n = 9; used as baseline reference), external injury (n = 5; e.g., skin lesions and flipper and carapace damage) and internal injury (n = 8; e.g., intestinal gas and gas emboli). To account for diurnal changes in behavior the model contained 24 h of data per turtle with the analysis starting 1 h after each turtle had been released into a tank to allow for acclimatization post handling. The model was run using the “lme” function in R, from the “nlme” package (Pinheiro and Bates, 2000; Pinheiro et al., 2015); to allow for heterogeneity of variance between individuals (indicated by model diagnostic plots) the model was updated to include the “varIdent” function (Gałecki and Burzykowski, 2013). Akaike Information Criterion (AIC) values along with forwards stepwise selection were used to find the best fitting model and p-values were obtained via the “anova” summary. Marginal and conditional R2 values for model goodness-of-fit were calculated using the “r.squaredGLMM” function in the “MuMIn” package (Barton, 2019), (the marginal R2 indicated the variance explained by fixed factors, and conditional R2 indicated the variance explained by both fixed and random factors) (Nakagawa and Schielzeth, 2013). The magnitude of dependence in scores attributable to differences between turtles (turtle ID) was quantified via the intraclass correlation coefficient (ICC). This was estimated as proportion of variance in the dependent variable (VeDBA) resulting from turtle ID, to total variance; where was the estimated turtle variance and was the estimated residual variance (Kenny and Hoyt, 2009).
Results
Thirty-three turtles were tagged during this study; upon the date of tagging, 17 were considered healthy (based on veterinarian assessments), eight were recovering from various degrees of gas emboli (convalescent), four had external injuries (on the neck, flipper, and/or carapace), two had floatability issues and one suffered multi-organ failure of unknown causes (see Table 1 for details). Despite the relatively large sample size, the variation in condition and small number of comparable individuals for condition (especially with respect to correcting for e.g., size and sex) meant that we had little capacity to verify our results statistically; as such we were unable to link specific pathologies with movement data.
Nonetheless, statistical analysis did indicate that turtle condition [grouped into healthy (n = 9), external injury (n = 5) and internal injury (n = 8)] affected behavior, specifically the relationship between mean VeDBA and the number of 45° turns performed per hour (Table 2; Figure 1). Forwards stepwise selection and AIC values showed that the full model incorporating all covariates (turn rate, turtle condition, turtle ID and tank size) yielded the best goodness-of-fit (marginal R2 = 0.81; conditional R2 = 0.96). The number of turns per hour (that surpassed 45°) significantly affected VeDBA (LMEM: = 143.28, p < 0.001); for every 10% increase in turn rate, VeDBA increased by just over 3% [Est. = 0.33 ± 0.02 (S.E), t = 17.04, 95% CI [0.292, 0.367], p < 0.001]. The largest tank size (containing a water mass of 19,212 kg) had a significant negative effect on this relationship. External injuries had a significant positive effect on turtle activity; per 10% increase in turn rate, VeDBA increased by almost 2% [Est. = 0.18 ± 0.06 (S.E), t = 3.01, 95% CI [0.052, 0.301], p = 0.01]. Internal injuries, however, did not significantly affect the relationship between turn rate and activity [Est. = 0.07 ± 0.054 (S.E.), t = 1.52, 95% CI [−0.025, 0.157], p = 0.15]. The intraclass correlation coefficient (ICC) was high (0.98), indicating high similarity between values from the same group (n = 22).
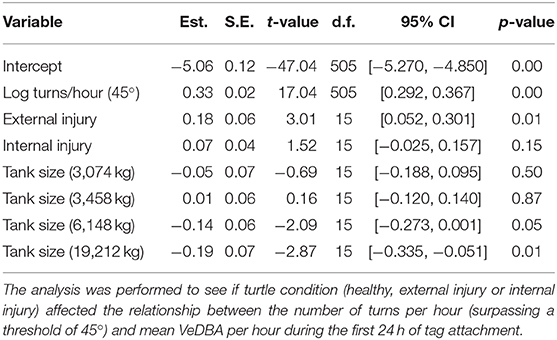
Table 2. Linear mixed-effects model (LMEM) estimates of fixed effects, p-values and 95% confidence intervals for log-transformed VeDBA.
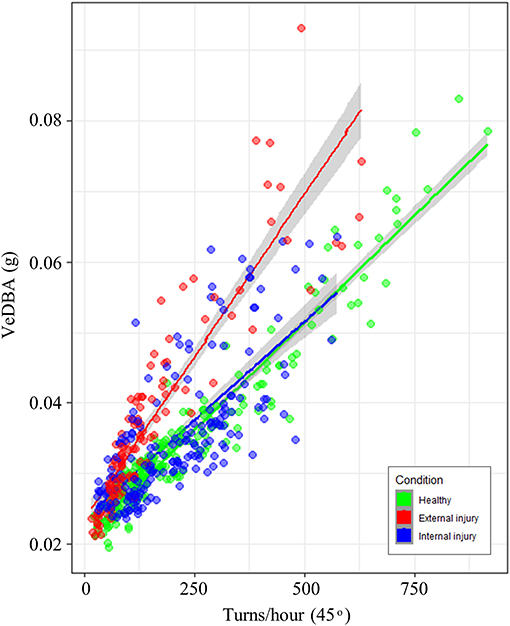
Figure 1. Relationship between the number of turns per hour that surpassed a 45° threshold and mean VeDBA (g) per hour. Data points and regression lines are colored according to turtle condition (healthy = green, external injury = red and internal injury = blue); 95% confidence intervals are indicated by the gray shading either side of regression lines. Line gradients indicate that the relationship between turning rate and VeDBA differed little between healthy turtles and those with internal injuries; turtles with external injuries however, had substantially higher VeDBA values per number of turns.
Movement Patterns for Various Conditions
Raw acceleration data showing movement patterns for turtles in varying states of health indicated differences at the individual level and in relation to condition (Figures 2, 3) although more data are needed to be able to have the statistical power to determine this. A period of initial heightened activity was apparent in all example turtles (except T332) and ranged from half an hour to 3 h (see Figure 2, individuals T402 and T384, respectively) or more (see Figure 3, individuals T350 and T359). Acceleration data together with depth (pressure) data showed that turtles generally exhibited alternating active and rest periods (with rest periods at the bottom of the tank typically lasting 10–15 min). This behavior was most clearly defined in healthy turtles. Rest periods in turtles recovering from gas emboli (T383 and T384) were less distinguishable (as the acceleration traces were not as smooth) and more erratic. Magnetometry data (indicating animal orientation), changed closely in accordance with acceleration movement in healthy example turtles and individuals recovering from gas emboli (Figure 3). The trace that differed most from the others was that of T332 with multi-organ failure (Figure 2) that died soon after tagging. The turtle remained at the surface and moved little (as indicated by the elevated depth trace remaining constant and the small spikes in the VeDBA trace compared to the other turtles, respectively; Figure 2).
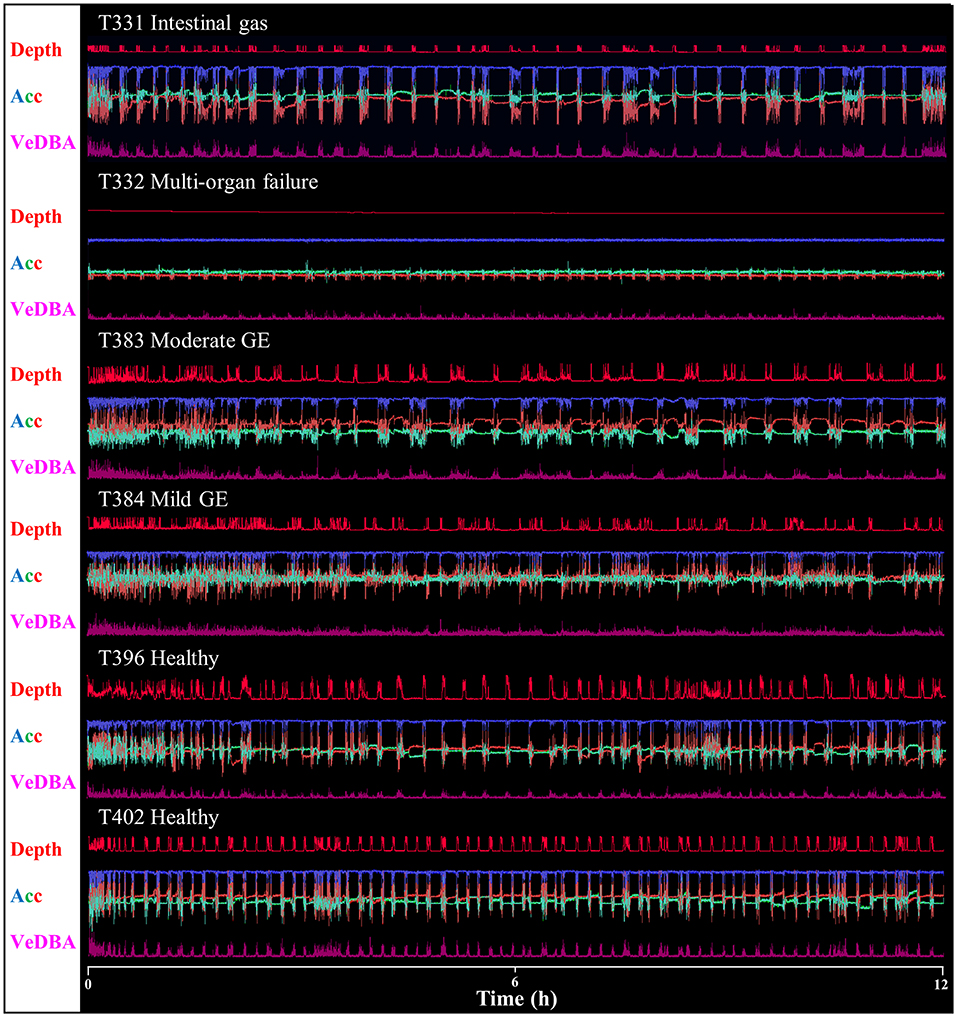
Figure 2. Daily Diary recordings for five different individuals in various conditions showing depth (dives are readily apparent), the three orthogonal acceleration channels (Acc) and a general activity metric (VeDBA – for definition see text). Scale is omitted to declutter graph, but acceleration limits are −1 – 1 g, and the depth limit is ca. 1 m). Data show 12 h from the first time a turtle was tagged. Note how traces vary with animal condition, in particular individual T332 with multi-organ failure that, unlike the other individuals, did not exhibit regular, alternating rest and dive periods. Most animals displayed increased activity at first, that decreased with time; this was most evident in animals with gas emboli (GE) where an initial period of 2–3 h of high activity was visible (cf. Figure 2).
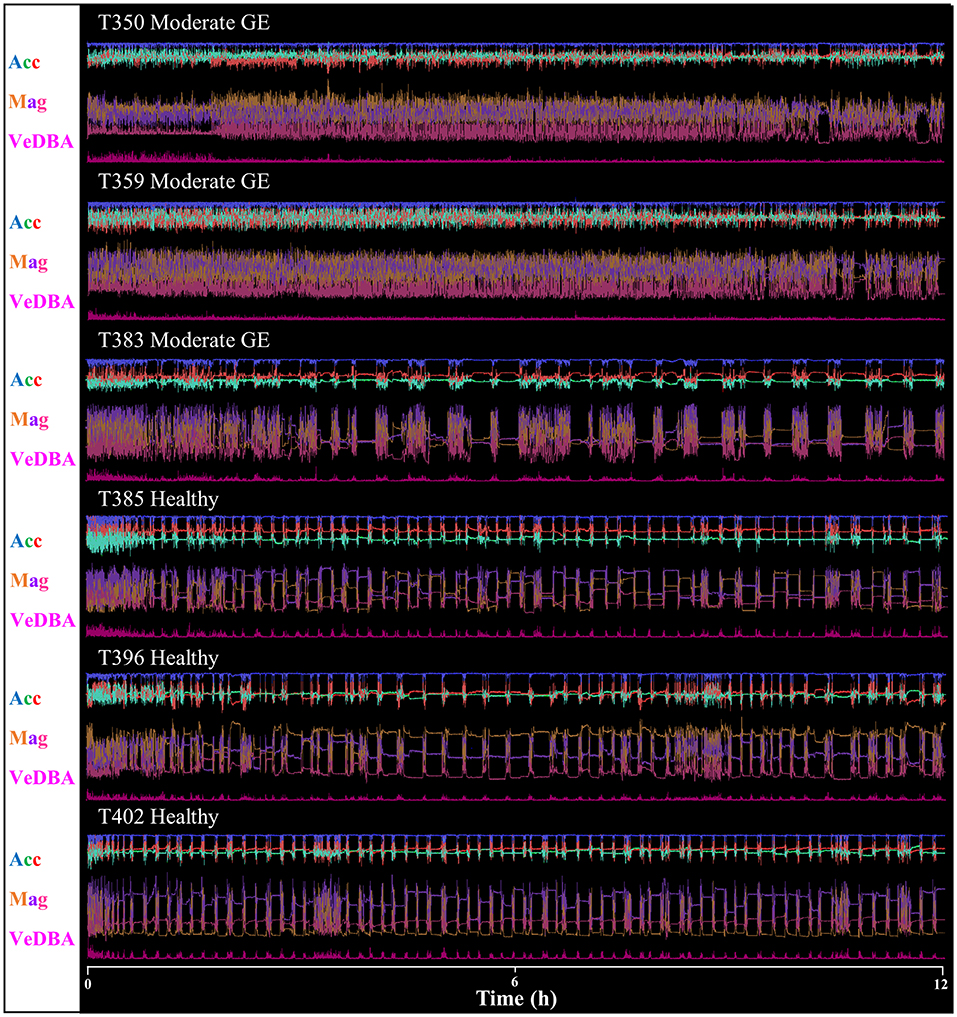
Figure 3. Daily Diary recordings for five different individuals in various conditions showing the three orthogonal acceleration channels (Acc), three magnetometry channels (Mag) and a general activity metric (VeDBA—for definition see text). Scale is omitted to declutter graph; acceleration limits are −1–1 g, magnetometry limits 0.2−0.8G). Data show 12 h from the first time a turtle was tagged. Note how traces vary between individuals that are healthy and those with gas emboli (GE); the former tended to display regular, alternating rest and dive periods, exhibited by the magnetic field data, and the latter exhibited increased activity lasting 3 h or more.
Activity Over Time
As observed previously, VeDBA (activity) in all healthy and unhealthy turtles (except T332 with multi-organ failure) was markedly raised for the first 3 to 4 h (Figure 4), particularly in animals with gas emboli (Figure 4B). After this initial period, VeDBA values tended to remain low and constant (<0.05 g) although some infirm individuals exhibited erratic periods of higher and lower VeDBAs (see Figure 4, individuals T331 and T384 in particular and Figure 5). The mean VeDBA for healthy turtles and standard deviation (Figure 4) were calculated using the seven turtles considered free of both disease and injury upon admission (despite 17 being considered healthy on the date of tagging) due to the subtle or undetectable long-term damage that gas emboli (particularly severe cases) can cause. During the first 24 h of tagging the VeDBA values of most afflicted turtles were within one standard deviation of healthy ones. However, the two rehabilitating turtles that deviated most frequently (T342 and T347) had both suffered severe carapace traumas (the latter also had a damaged fore flipper). Consistently low VeDBA values were recorded for T342, whereas for T347 they were within the healthy turtle range for the first 12 h and then rose markedly above but in parallel with a small hump in VeDBA observed in healthy turtles some 20 h post-tagging.
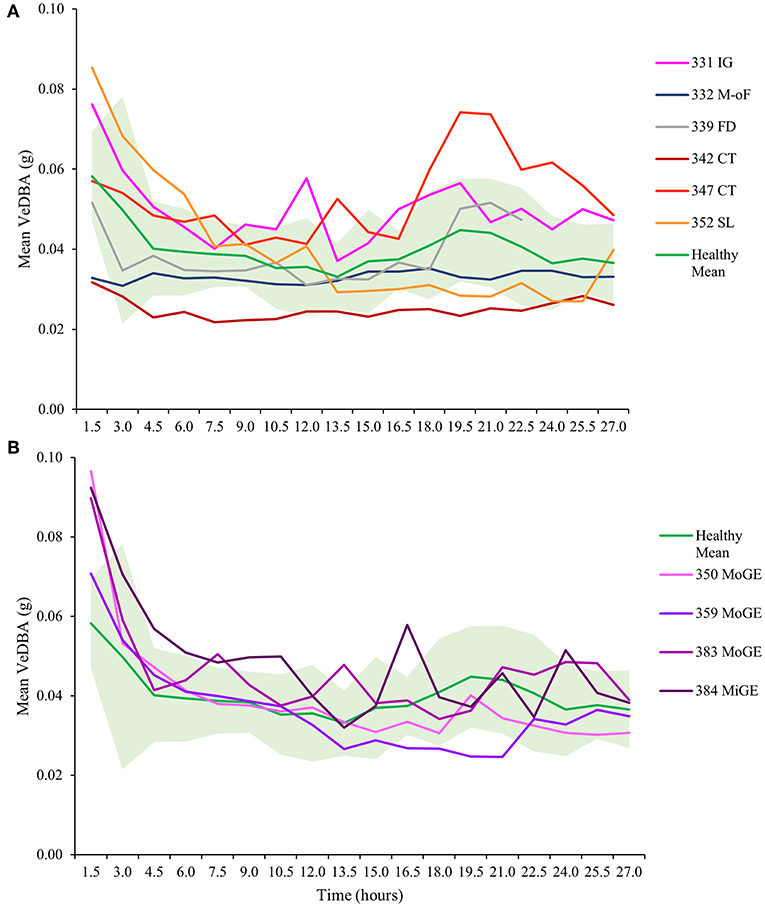
Figure 4. Mean VeDBA (calculated over hourly intervals) in turtles with (A) various diseases and injuries and (B) turtles with gas emboli (GE), from the first moment they were tagged (cf. Figures 2, 3). Turtles are identified by number (see Table 1). IG, intestinal gas; M-oF, multi-organ failure; FD, flipper damage; CT, carapace trauma; SL, skin lesions; MoGE, moderate gas emboli; MiGE, mild gas emboli. The mean VeDBA of turtles that were considered healthy upon entry (individuals T324, T325, T330, T344, T345, T385, and T396) is shown in green; the shaded light green area represents one standard deviation. Note the initially high values observed in most turtles for the first three to four hours (particularly in animals with GE).
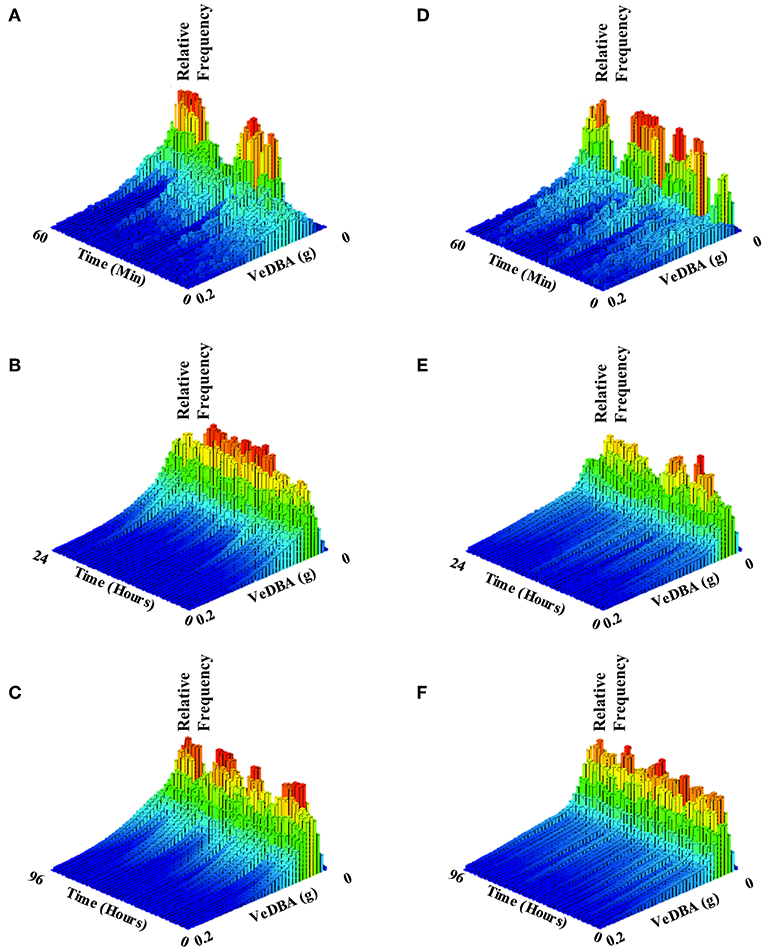
Figure 5. Histograms showing allocation of activity (VeDBA) over varying time intervals, of two example turtles: T324, which was considered healthy (figures on the left; A–C) and T331 which had intestinal gas and associated floatability issues (figures on the right; C,D,F). Histograms cover time periods of one hour (A,D), 24 h (B,E) and four days (C,F). Bar color and height indicate the relative number of observations within the time interval. Note how, in both example turtles, lower VeDBA values occurred most frequently (red bars) but that the individual with intestinal gas exhibited erratic periods of high VeDBA, showing high effort in bursts interspaced by a high frequency of rests (cf. A,D) compared to the healthy individual. As the time period over which the animals are examined increases, the healthy individual spends more time overall exhibiting greater activity (manifest by the higher VeDBA - nominally from swimming) than the unhealthy animal (cf. B,C with E,F).
Pitch, Roll, and Directionality
G-sphere visualizations of body pitch and roll [derived from the acceleration data, which showed the time allocated to different pitch and roll values [body attitude] (Wilson et al., 2016)] indicated slight differences between healthy and unhealthy rehabilitating turtles (Figure 6). Animals with serious illnesses and reduced activity generally occupied a smaller area of the g-sphere relative to healthy turtles; however, individuals recovering from gas emboli tended to occupy a slightly greater area. Magnetometry plots (m-plots, see Williams et al., 2017) showed clearer differences in movement behavior; unhealthy animals generally displayed greater variability in directionality than healthy ones (Figure 7). Variability in orientation was also observed in a turtle that underwent MRI, with rose plots indicating directionality becoming more concentrated with time post-scan (Figure 8).
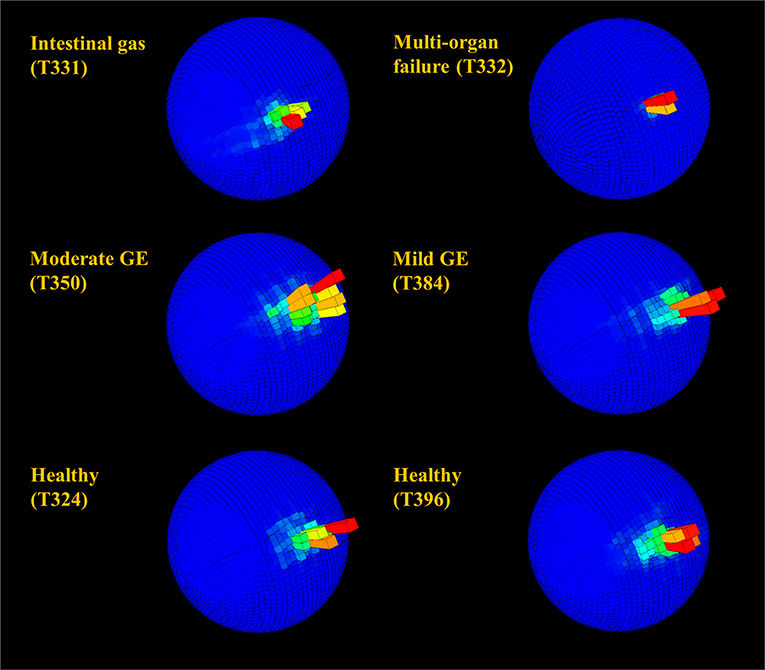
Figure 6. Spherical histograms showing relative time (bar height and color; the mode is shown in red) over 24 h allocated to body pitch and roll (see Wilson et al., 2016 for more detail) in rehabilitating turtles. Individuals in rehabilitation are identified by number (see Table 1) and suffered from various diseases including gas emboli (GE). Despite the superficial semblance of plots, very infirm individuals (in particular T332) with reduced activity generally occupied a small area of the sphere relative to healthy turtles, whereas individuals with mild to moderate GE generally occupied a slightly greater area.
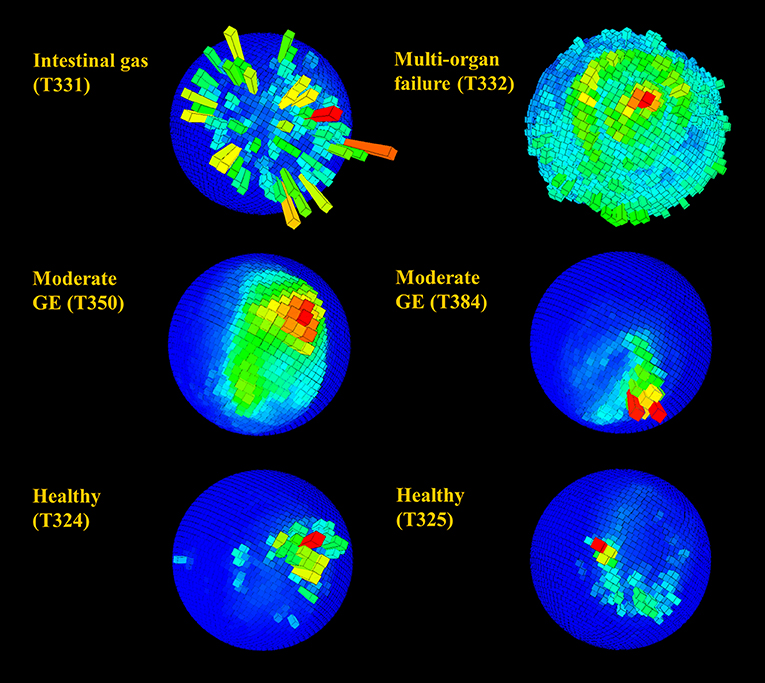
Figure 7. Spherical magnetometer histogram plot (m-plot see Williams et al., 2017) showing relative time allocation (bar height and color; the mode is shown in red) to direction in rehabilitating turtles during a 24 h period. Individuals are identified by number (see Table 1) and suffered from various diseases including gas emboli (GE). Note the generally higher directionality observed in healthy individuals and the clear lack of directionally in animals T331 and T332.
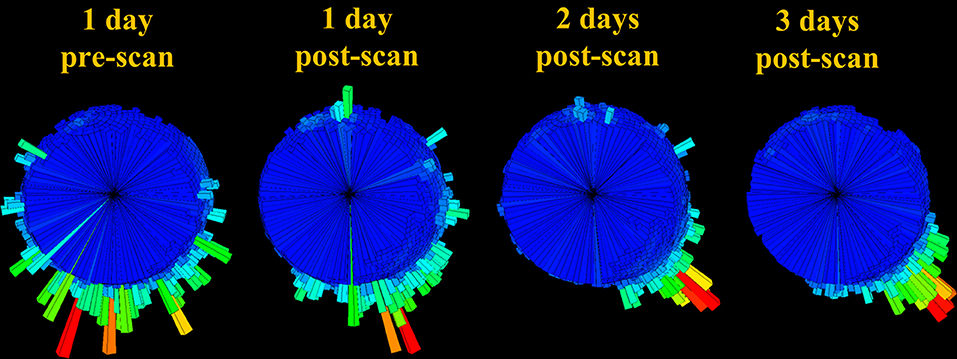
Figure 8. Body orientation histogram (rose plot) over 24 h time-periods for T383 prior to undergoing an MRI scan and for the 3 days following this. Bar length denotes length of time allocated to each direction (the mode is shown in red). Note how the appreciable variability pre-MRI scan appears to diminish with time after the scan.
Discussion
The purpose of this study was to investigate the effectiveness of animal-attached loggers to elucidate behavior in order to assess animal health in sea turtles undergoing rehabilitation. Behavior is recognized as having the potential to serve as an indicator of health (Abou-Ismail et al., 2007; Burman et al., 2007; Scollo et al., 2014; Guesgen and Bench, 2017), so movement-sensitive tags, such as the DDs used in this study, could be used to provide an objective and time-efficient way of quantifying behavior via the creation of TDBBs for health. Our statistical analysis, although with limited power, indicated that behavior (specifically the relationship between mean VeDBA and the number of 45° turns per hour) differed significantly between healthy individuals and those with external injuries (e.g., flipper damage, carapace trauma and skin lesions). Although this study focused on loggerhead turtles undergoing rehabilitation following fisheries interaction, the approach could potentially be adopted for a suite of aquatic (cf. Shorter et al., 2017), terrestrial (Mason and Rushen, 2008) or aerial species (Shepard et al., 2008). Our limited access to animals precluded us from presenting exhaustive data analyses from a suite of turtles of defined size, unknown sex and in various states of health, so by in large we present sample data as examples of the features that can be resolved using this technology and speculate how these relate to health status.
Behavior and Condition
After being released into a holding tank, bycatch turtles generally exhibited a period of elevated activity ranging from half an hour to several hours (when examined over a 24 h period). In part this was probably due to tagging occurring during the day when activity levels were higher and there was more disturbance (caused by feeding and tank cleaning). In healthy turtles, this initial increase in activity typically lasted <2 h whereas in individuals with gas emboli this was always 3 h or more. This disparity could reflect the condition of the turtle, especially given that individuals with gas emboli have been known to display abnormal behavior, ranging from hyperactive to catatonic (Garcia-Parraga et al., 2014). However, disparities may also arise from a variety of other factors: side-effects of hyperbaric treatment, stress induced by handling (Grandin, 1997; Moberg, 2000; Carere and van Oers, 2004; Waiblinger et al., 2004; Gourkow and Fraser, 2006; Hemsworth et al., 2011), tag attachment (Bridger and Booth, 2003; Geertsen et al., 2004; Vandenabeele et al., 2011; Walker et al., 2011; Thomson and Heithaus, 2014), re-entering the water after many hours on land and being released into an unknown environment (Teixeira et al., 2007; Roe et al., 2010). These factors make it difficult to know what truly “healthy” turtle behavior in a rehabilitation center looks like using tag data. Nonetheless, significant behavioral differences in relation to activity and turn rate were found between “healthy” turtles and those with external injuries (see section Metrics That Might Indicate Changing State and Behavioral Breadth). Our statistical analysis also indicated that within group values had a high similarity, thus indicating that once healthy, turtles in rehabilitation exhibited similar behavior.
Interestingly, the relationship between activity level and turning for turtles with internal injuries did not differ significantly from healthy animals. However, the internal injuries included in analysis were unlikely to affect energy expenditure and movement to the same degree as missing part of a flipper or sustaining severe carapace trauma. Most of the turtles (six out of eight) that suffered internal injuries were admitted with gas emboli and as such were only released into a tank once they had no gas bubbles left in their blood (as per the standard veterinary procedure). By this time these individuals may have recovered sufficiently to exhibit activity levels and turning rates akin to those of “healthy” turtles. Potential differences between healthy animals and those with internal damage may have also been more apparent if two turtles with severe internal complications (T331 and T332) could have been included in statistical analysis. They were excluded from the analysis because the available water mass in which they had to move was unknown and our analysis suggested that tank size significantly affected behavior.
Further behavioral comparisons of healthy turtles and individuals recovering from internal injuries such as gas emboli and intestinal gas indicated other potential differences relating to condition. Rest and active periods (typically composed of active ascents and descents interspaced with resting on the tank floor) were often less defined in convalescent turtles; not only did rest intervals appear more sporadically, but acceleration traces were noisier, probably indicating impaired movement control during recovery and/or post hyperbaric treatment. Magnetometer plots also indicated a difference between healthy rehabilitating turtles and those with gas emboli, the latter tending to display less directionality, potentially indicating impaired stability or movement control. Differences between healthy and infirm individuals with intestinal gas (T331) and multi-organ failure (T332) were even more apparent, with deviations covering almost half, or more, of the m-sphere, respectively. Indeed, the trace that differed most from the others was that of turtle T332; the animal remained at the surface and was relatively inactive for the duration of tagging (4 days). As with many animals, maintaining a very low energy state and fatigue can be indicative of serious illness and a precursor of death (Drake et al., 2003; Gailliot et al., 2006). With a sufficient sample size, a range of expected energy levels (including the duration of “rest” and “active” periods as well as changes in VeDBA over time) for a given condition could be calculated, although these would also have to take into account turtle age, size, sex, and surroundings i.e., enclosure size, enrichment and water temperature, if found to be relevant.
Metrics That Might Indicate General Activity Patterns
We suggest that it should be possible to assess health status using VeDBA as a metric of general activity, for example, the comparison of animals with gas emboli vs. healthy individuals, aside from showing different postural changes, also demonstrated different VeDBA signatures. The paddling behavior in diseased animals was more intense and prolonged than in healthy individuals. This could have been a side effect of being out of the water for a number of hours and/or hyperbaric treatment, which is thought to increase activity (Vicente Marco pers. comm.). Increased activity was also observed in the individual with intestinal gas. The link between VeDBA and physical condition was clearer in this case because the extra gas within the body caused greater buoyancy, making it more difficult to dive and requiring more vigor (Minamikawa et al., 2000). Thus, while attempting to dive underwater in order to rest on the tank floor, as is normal, individuals with overall body densities less than that of seawater must spend additional energy paddling to overcome the added buoyancy, resulting in elevated VeDBA during descent (cf. Wilson and McMahon, 2006).
A very different VeDBA signature was observed for the individual that died of multi-organ failure; our study animal never reached the bottom of the tank to rest (cf. Minamikawa et al., 2000). Instead, periods of attempted descent were interspaced with periods of rest at the surface. This pattern became clear when comparing the VeDBA trace (which was consistently low) with that of depth.
Metrics That Might Indicate Disease/Injury
We suggest that diagnostics of health could be based on multiple parameters in a disease/injury identification key that could be combined to form specific TDBBs. Thus, an indication that a turtle has problems with buoyancy could be provided by having: (i) a higher incidence of body pitched-down, (ii) a greater incidence of high VeDBA and (iii) greater amplitude in VeDBA cycles stemming from exhaustion (recovery time at the surface due to greater oxygen use while underwater). This, for example, was observed in an individual with intestinal gas which had higher buoyancy than controls and was unable to descend the water column and reach the bottom of the tank without excessive paddling. A clear signal that this was the case was given by body pitch angle since the animal spent a large proportion of the time swimming down (with the body pitched forward) against buoyancy, whereas control animals only had the body pitched forward for the short periods they spent moving from the water surface to their preferred depths.
In fact, the body attitude of the individual with intestinal gas not only differed with respect to that of the healthy animal with regard to pitch, for which a mechanistic basis can be proposed (see above), it also differed with respect to roll (as observed in magnetometer plots—cf. Williams et al., 2017), indicating apparent instability which was not the case in healthy animals. This apparent lack of control was also observed in example individuals recovering from gas emboli and with multi-organ failure. We suggest that consideration of body posture, particularly in sea turtles (and potentially other aquatic species), and derivatives of this, such as rate of change of body posture, would be a useful way of documenting deviations in health status from the “norm.”
Additionally, assessing changes in body posture before and after treatment could help to track animals through recovery and elucidate potential negative side effects of certain procedures, in particular, MRI scans, which expose animals to high magnetic fields in order to generate high quality diagnostic images (superior to those of radiographs and ultrasound) (Rübel et al., 1994; Walzer et al., 2003; Jandial et al., 2005; Thornton et al., 2005). Despite evidence that sea turtles rely on geomagnetic cues to navigate and reach specific nesting and feeding sites (Lohmann et al., 2004; Putman et al., 2011), MRIs have been widely used in anatomical examinations of the ear (Ketten and Bartol, 2005), head (Arencibia et al., 2012) and coelomic structures (Valente et al., 2006), as well as to investigate internal injuries caused by the ingestion of debris (Gasau and Ninou, 2000) and internal tumors in turtles with fibropapillomatosis (Croft et al., 2004). To date, no study has considered whether exposing turtles to intense magnetic fields could impair navigational abilities post-release. In this study, we presented information of the directionality in a single turtle (that had been admitted with gas emboli) pre- and post-MRI, which indicated increased directionality in the days following the scan. The implications of this possibly transient effect and whether this behavioral change should be attributed to recovering from gas emboli or magnetic field exposure or another factor is unknown and requires further study.
Monitoring Periods
After initial release into rescue tanks, VeDBA values from afflicted turtles during day 1 of tagging were typically within one standard deviation of healthy turtles. However, the probability of values from infirm turtles falling outside of this range would be likely to increase as a function of time and treatment; for example, sedatives would reduce activity whereas hyperbaric treatment and physiotherapy may increase it. Certain afflictions were more likely to alter behavior only in the short-term. For example, most turtles with gas emboli often did not exhibit defined active and rest periods (as observed in healthy turtles) for a few hours after release into a rescue tank. Nevertheless, veterinary diagnostics indicate that after hyperbaric treatment, turtles show full gas reabsorption. It is worth noting that in cases with severe gas emboli, bubble formation may have caused permanent damage. Observations also indicated that turtles were more active during daylight hours and therefore diel patterns should be taken into account when considering how long animals should be monitored. For many turtles, a second peak in VeDBA was observed some 20 h post-tagging, between 8:00 a.m. and 12:00 p.m. (noon), which was consistent with increasing light levels (the start of a new day) and tank cleaning and feeding (which takes place most mornings).
The infirm turtles that differed most from the general “healthy turtle” trend were T342 and T347; both had suffered traumas to the carapace and the latter also had a partially amputated flipper. The two turtles were first tagged some months after arriving at the center (T342 was tagged 2 months after arriving and T347 almost 7 months) due to treatment and/or injuries making the standard tag deployment procedure not feasible. The VeDBA values exhibited by T342 were consistently below the mean of healthy turtles whereas for T347 they were within the healthy turtles' range for the first 12 hours and then rose markedly above them. This rise, which peaked between 10:00 a.m. and 12:00 p.m., coincided with tank cleaning (which may cause animals some disturbance) and feeding. For T347, all swimming, but in particular descending to the bottom of the tank in order to eat, required exertion that was clearly greater than the norm, thus illustrating the potential for injury-specific feeding signatures as part of TDBBs relating to physical condition. The data from T342 and T347 also demonstrate that certain injuries, especially those involving flippers or carapace trauma, have long-lasting or even permanent effects on behavior. Consequently, monitoring the activity of such individuals at regular intervals over the long-term could provide a valuable tool when assessing recovery, especially if specific TDBBs defining expected improvements existed and could be used in comparison.
In future, baseline data on expected VeDBA values for a variety of conditions through time could be determined by attaching DDs to turtles in rehabilitation centers and aquaria around the world. Such collaboration would be needed to build a behavioral repository of certified healthy animals, taking into account turtle size, sex, season, water temperature, enrichment, and enclosure size/available water mass. Although we found no significant relationship between available water mass and VeDBA, our analysis included turtles in various states of health and was only based on data from the first 24 h of tagging (whilst this should encompass diel changes, it would not take into account more longitudinal trends). The collection of such baseline data is of primary importance when defining suitable lengths of time to monitor rehabilitating animals.
Metrics That Might Indicate Changing State and Behavioral Breadth
This work begins the examination of the health of managed care animals by comparing the behavior of rehabilitating turtles with various diseases and injuries. The small sample size makes this necessarily speculative at the moment, but the results provided should encourage researchers to develop a common data base, or at least to share data, in order to gain the statistical power to differentiate conditions using tag-derived metrics confidently. In addition to increasing the sample size for turtles, it would benefit zoological institutions to expand the work to other taxa (cf. Ropert-Coudert et al., 2009 and Shorter et al., 2017). It is also important for workers using DD-type tags (cf. Johnson and Tyack, 2003) incorporating inter alia sophisticated and powerful sensors such as accelerometers and magnetometers, to recognize the large number of potentially important variables that can be gleaned from such devices to aid in the discrimination of differing behavioral states. These include, but are not limited to, animal heading (Williams et al., 2017), saccadic movement (Wilson et al., 2015) and rates of change of a suite of parameters (e.g., depth, pitch, roll, yaw) over different time intervals (Wilson et al., 2018) and there is an increasing number of analytical systems available to help in this (e.g., Walker et al., 2015a; Wilson et al., 2018).
There are, however, some metrics that will be more universal than others, and an example of this is VeDBA—a powerful metric that codes for overall body activity and acts as a proxy for metabolic rate (Qasem et al., 2012). Normally, we would expect the healthiest animals to be the most active, although the particular cases of intestinal gas and gas emboli show that this is not always true. Another less frequently used but extremely useful derivative is that of animal heading (turning) (Bidder et al., 2015; Walker et al., 2015b; Williams et al., 2017). Unlike VeDBA, which is derived from acceleration data and as such is affected by currents in air and water that can distort the signal-to-noise ratio (Halsey et al., 2011b), compass heading is not. VeDBA is also of limited use when examining the behavior of slow moving, relatively inactive or gliding animals that maintain a steady velocity for extended periods, for example turtles (Wyneken, 1997; Eckert, 2002) and soaring birds (Williams et al., 2015). In such cases, using magnetometers and examining movement patterns about the yaw axis may elucidate behaviors that are not evident in acceleration data alone (Williams et al., 2017). We found that VeDBA and heading in unison showed a promising way of differentiating between healthy and infirm turtles with external injuries. This was not surprising as severe flipper damage and carapace trauma affecting the spinal cord (as observed in individuals T342 and T347) had a clearly visible impact on the swimming and maneuverability of individuals. Critically though, it is specifically the combination of parameters (here dynamic acceleration and turning rates) that demonstrates that mixed sensor outputs can be particularly useful in TDBBs for state and our work is a first step in this direction. Although tagging animals with obvious external injuries would be unnecessary for diagnostic purposes, examining their behavior over time could be beneficial in order to track recovery and determine when behavior has returned to “normal” relative to healthy individuals. In some cases, behavioral biomarkers may indicate that an animal will never be fit for re-release into the wild.
Implications for Other Species
Given the ever-growing welfare concerns for animals maintained in zoos and aquaria (Johnson and Tyack, 2003; Shepard et al., 2008; Ismail et al., 2012) and the myriad research opportunities that these venues provide, it is surprising that little quantitative information (which is readily available via biologging) exists in relation to the activity and health of most species (Flint and Bonde, 2017; Shorter et al., 2017). This is especially pertinent to managed marine vertebrates (particularly cetaceans and other pinnipeds), whose presence in aquaria is regularly scrutinized (Rose et al., 2017). As such, current health and welfare assessments and monitoring practices, which generally rely on qualitative observations (including what individuals eat and social interactions) could be greatly aided by the collection of behavior in a quantifiable manner (Shorter et al., 2017). Studies using tags on farmed animals show how advantageous this could be; for example, improved lameness detection via leg-mounted accelerometers on dairy cows (Thorup et al., 2015).
In contrast to observational monitoring, which takes time and has a large degree of subjectivity (Broom and Johnson, 1993; Rushen, 2003; Lawrence, 2008; Rose et al., 2017), biologging enables the collection of quantitative data in a fast (often at several Hertz) and unbiased manner (Block, 2005; Sherub et al., 2017). Furthermore, information is recorded in sufficient detail to; (i) develop species-specific guidelines to standardize captive assessments, (ii) determine if adequate welfare requirements are being fulfilled, i.e., by defining what constitutes “typical” or “healthy” behavior and (iii) provide guidance on whether an animal is suitable for release after rehabilitation (Rose et al., 2017). This has particularly important implications for a wide range of species (including fish, sea turtles, birds, pinnipeds and cetaceans) that are frequently injured in fishery related interactions or by marine debris (Raum-Suryan et al., 2009; Adimey et al., 2014; Gall and Thompson, 2015; Jambeck et al., 2015; Nelms et al., 2015; Stelfox et al., 2016).
Unlike the animals in human care, logging devices have been widely used with their wild counterparts (Eckert, 2002; Shorter et al., 2017). The information derived from these applications is highly valuable as understanding natural behavior and ecology is key to informing appropriate welfare standards for animals in captivity (Eckert, 2002; Shorter et al., 2017) and the wild (Rose et al., 2017). It also informs critical decisions such as whether to conserve natural habitats or recreate them artificially- these decisions usually involve vulnerable animals (for example dugongs and manatees) and can have major consequences (Rose et al., 2017).
Limitations and Perspectives
This dataset provides a small first step in demonstrating the usefulness of tags for collecting information on animals in human care. However, studies with a greater sample size and covering longer tag attachment durations are necessary to give proper statistical credibility to these initial findings. Nonetheless, significant behavioral differences (related to VeDBA verses the number of turns per hour) were found between healthy controls and turtles with external injuries to the flippers and carapace, suggesting that even with limited data, the utility of this tool is justifiable. Furthermore, comparisons between healthy individuals and those with gas emboli, intestinal gas and multi-organ failure, in the form of spherical plots revealed appreciable differences in orientation during a 24 h time window.
Although our categorization of turtle health status relied on veterinary diagnostic techniques (including blood sampling, radiographs and ultrasounds), the goal of creating illness specific TDBBs would be to limit the use of these potentially invasive and stress inducing procedures. In addition, the use of tags that transmit data would enable remote data collection in real time (Laske et al., 2014; Wilmers et al., 2015), both reducing animal handling and speeding up diagnosis/ our ability to track an animal's recovery. This would make our approach suitable to a variety of different applications in captive animal monitoring.
The financial and societal value of many species in managed care means that even limited data such as ours are appreciably better than nothing if it helps to enhance animal health and well-being. Indeed, within the zoo veterinary field, approaches are developed by slowly increasing findings from individual animals to larger numbers (Swaisgood and Shepherdson, 2005; Kuhar, 2006). Reaching the appropriate sample size required to obtain biologically or statistically significant results is a notable difficulty, often because few (if any) individuals are maintained in zoos/aquaria. Furthermore, in this circumstance, animals were tagged opportunistically as they entered the rehabilitation center with a variety of different injuries and diseases, which reduced suitable comparisons.
Conclusion
This manuscript showcases how data collected from motion- and orientation-sensitive animal-attached technology can be used to derive metrics which may aid animal health assessments and that could in time be combined to form an injury/disease identification guide. For example, data visualization showed behavioral differences between “healthy” sea turtles and individuals suffering gas emboli and intestinal gas, with the latter apparently paddling more frequently and spending more time with the body pitched downwards (presumably in order to compensate for increased buoyancy). Appropriate visualization showed such diagnostic patterns immediately without complex data analysis. We also found that VeDBA and compass heading in unison showed a promising way of differentiating between healthy and infirm turtles with external injuries to the flippers and carapace. Given this, we propose that the use of motion-sensitive tags could aid diagnosis and inform therapy, in particular cases follow-up, monitoring improvement and response to treatment. This is particularly relevant to turtles, given the numerous rehabilitation programs for bycatch sea turtles in operation. We suggest that establishing tag-derived behavioral biomarkers (TDBBs) for health in these animals based on the visualizations and metrics discussed in this paper is therefore timely and should both facilitate and improve the rehabilitation process.
Future Directions
Obvious further developments on the work presented here would be to equip more individuals with tags in order to augment sample size and validity and test the various metrics highlighted in this paper. After establishing TDBBs in loggerheads, the next step would be to trial them on other sea turtle species to gauge whether they were transferable or easily adaptable. Another line of work would be to ascertain whether TDBBs could be made specific to not just different diseases and injuries but also animal states (i.e., positive: happy or negative: sad, fearful, aggressive etc.) in order to use behavior as a measure of welfare (Benn et al., 2019). If successful, TDBBs could be adapted to fit other bycaught species in the same way that farm animal welfare assessments have been modified for zoos (Fraser, 2009; Hill and Broom, 2009) and aquaria [for example the “C-Well” welfare assessment index for dolphins in managed care (Clegg et al., 2015)]. In other words, TDBBs could be established for other species undergoing rehabilitation and release with more complex behavioral repertoires such as cetaceans and pinnipeds. Furthermore, motion-sensitive data from animals in human care and wild conspecifics could be compared in order to define a set of expected behavioral states and/or activity levels for numerous species housed in zoos/aquaria to help ensure their welfare. Finally, animal health is an increasing concern for wild populations, and appropriate validation of objective TDBBs in managed care populations could be of relevance when studying health and welfare in free-ranging animals.
Data Availability Statement
The datasets generated for this study are available on request to the corresponding author.
Ethics Statement
This study was carried out in accordance with the recommendations of the Animal Care and Welfare Committee of the Oceanogràfic Foundation (OCE-17-16 and amendment OCE-29-18). The protocol was approved by the Oceanogràfic Animal Care and Welfare Committee (OCE-16-18).
This study was also carried out in accordance with the recommendations of the Animal (Scientific Procedures) Act 1986 (Amended 2012) and the associated Code of Practice for accommodation and care of animals, Swansea University, Animal Welfare Ethical Review Body. The protocol was approved by the Swansea University, Animal Welfare Ethical Review Body (STU_BIOL_82015_011117151527_1).
Author Contributions
AA, EA, AF, CD, and RW: experimental conception and design. AA, EA, AF, VC, and JC-P: data collection. AA, EA, AF, MH, RG, and RW: data analysis. AA, EA, AF, RG, and RW: manuscript preparation. All authors contributed to manuscript revision, read and approved the submitted version.
Funding
This research contributes to the CAASE project funded by King Abdullah University of Science and Technology (KAUST) under the KAUST Sensor Initiative. The Fundación Oceanogràfic provided a student bursary for AA and paid the costs to run the rehabilitation facility ARCA were all the work was done.
Conflict of Interest
The authors declare that the research was conducted in the absence of any commercial or financial relationships that could be construed as a potential conflict of interest.
The handling editor is currently co-organizing a Research Topic with one of the authors, AF, and confirms the absence of any other collaboration.
Acknowledgments
We are grateful to Phil Hopkins for help with the tag housings. We would like to thank all the professionals at the Oceanogràfic, especially at the rehabilitation center (ARCA) taking care of the animals, for their efforts and dedication to provide excellent animal care. We are very grateful to all the fishermen contributing to the project as well as to the Valencian Government, especially to the Servicio de Vida Silvestre de la Conselleria d'Agricultura, Medi Ambient, Canvi Climàtic i Desenvolupament Rural de la Generalitat Valenciana.
References
Abou-Ismail, U., Burman, O., Nicol, C., and Mendl, M. (2007). Can sleep behaviour be used as an indicator of stress in group-housed rats (Rattus norvegicus)? Anim. Welf. 16, 185–188.
Adimey, N. M., Hudak, C. A., Powell, J. R., Bassos-Hull, K., Foley, A., Farmer, N. A., et al. (2014). Fishery gear interactions from stranded bottlenose dolphins, Florida manatees and sea turtles in Florida, USA. Mar. Pollut. Bull. 81, 103–115. doi: 10.1016/j.marpolbul.2014.02.008
Andrewartha, S., Elliott, N., McCulloch, J., and Frappell, P. (2015). Aquaculture sentinels: smart-farming with biosensor equipped stock. J. Aquac. Res. Dev. 7, 1–4. doi: 10.4172/2155-9546.1000393
Arencibia, A., Hidalgo, M. R., Vázquez, J. M., Contreras, S., Ramírez, G., and Orós, J. (2012). Sectional anatomic and magnetic resonance imaging features of the head of juvenile loggerhead sea turtles (Caretta caretta). Am. J. Vet. Res. 73, 1119–1127. doi: 10.2460/ajvr.73.8.1119
Barton, K. (2019). Package ‘MuMIn’. R package version, 1.43.6. Available online at: https://CRAN.R-project.org/package=MuMIn
Benn, A. L., McLelland, D. J., and Whittaker, A. L. (2019). A review of welfare assessment methods in reptiles, and preliminary application of the welfare quality® protocol to the pygmy blue-tongue skink, Tiliqua adelaidensis, using animal-based measures. Animals 9:27. doi: 10.3390/ani9010027
Bidder, O., Arandjelović, O., Almutairi, F., Shepard, E., Lambertucci, S. A., Qasem, L., et al. (2014). A risky business or a safe BET? A Fuzzy Set Event Tree for estimating hazard in biotelemetry studies. Anim. Behav. 93, 143–150. doi: 10.1016/j.anbehav.2014.04.025
Bidder, O., Walker, J., Jones, M., Holton, M., Urge, P., Scantlebury, D., et al. (2015). Step by step: reconstruction of terrestrial animal movement paths by dead-reckoning. Mov. Ecol. 3:23. doi: 10.1186/s40462-015-0055-4
Block, B. A. (2005). Physiological ecology in the 21st century: advancements in biologging science. Integr. Comp. Biol. 45, 305–320. doi: 10.1093/icb/45.2.305
Bograd, S. J., Block, B. A., Costa, D. P., and Godley, B. J. (2010). Biologging technologies: new tools for conservation. Introduction. Endang. Species Res. 10, 1–7. doi: 10.3354/esr00269
Boissy, A., Manteuffel, G., Jensen, M. B., Moe, R. O., Spruijt, B., Keeling, L. J., et al. (2007). Assessment of positive emotions in animals to improve their welfare. Physiol. Behav. 92, 375–397. doi: 10.1016/j.physbeh.2007.02.003
Bridger, C. J., and Booth, R. K. (2003). The effects of biotelemetry transmitter presence and attachment procedures on fish physiology and behavior. Rev. Fish. Sci. 11, 13–34. doi: 10.1080/16226510390856510
Broom, D. M. (1991). Animal welfare: concepts and measurement. J. Anim. Sci. 69, 4167–4175. doi: 10.2527/1991.69104167x
Broom, D. M., and Johnson, K. G. (1993). Stress and Animal Welfare. Springer Science & Business Media. doi: 10.1007/978-94-024-0980-2
Brown, D. D., Kays, R., Wikelski, M., Wilson, R., and Klimley, A. P. (2013). Observing the unwatchable through acceleration logging of animal behavior. Anim. Biotelem. 1:20. doi: 10.1186/2050-3385-1-20
Brownscombe, J. W., Thiem, J. D., Hatry, C., Cull, F., Haak, C. R., Danylchuk, A. J., et al. (2013). Recovery bags reduce post-release impairments in locomotory activity and behavior of bonefish (Albula spp.) following exposure to angling-related stressors. J. Exp. Mar. Biol. Ecol. 440, 207–215. doi: 10.1016/j.jembe.2012.12.004
Burman, O. H., Ilyat, A., Jones, G., and Mendl, M. (2007). Ultrasonic vocalizations as indicators of welfare for laboratory rats (Rattus norvegicus). Appl. Anim. Behav. Sci. 104, 116–129. doi: 10.1016/j.applanim.2006.04.028
Carere, C., and van Oers, K. (2004). Shy and bold great tits (Parus major): body temperature and breath rate in response to handling stress. Physiol. Behav. 82, 905–912. doi: 10.1016/S0031-9384(04)00312-9
Clegg, I., Borger-Turner, J., and Eskelinen, H. (2015). C-well: the development of a welfare assessment index for captive bottlenose dolphins (Tursiops truncatus). Anim. Welf. 24, 267–282. doi: 10.7120/09627286.24.3.267
Cooke, S. J. (2008). Biotelemetry and biologging in endangered species research and animal conservation: relevance to regional, national, and IUCN Red List threat assessments. Endang. Species Res. 4, 165–185. doi: 10.3354/esr00063
Cooke, S. J., Hinch, S. G., Wikelski, M., Andrews, R. D., Kuchel, L. J., Wolcott, T. G., et al. (2004). Biotelemetry: a mechanistic approach to ecology. Trends Ecol. Evol. 19, 334–343. doi: 10.1016/j.tree.2004.04.003
Croft, L. A., Graham, J. P., Schaf, S. A., and Jacobson, E. R. (2004). Evaluation of magnetic resonance imaging for detection of internal tumors in green turtles with cutaneous fibropapillomatosis. J. Am. Vet. Med. Assoc. 225, 1428–1435. doi: 10.2460/javma.2004.225.1428
Drake, R., Frost, J., and Collins, J. J. (2003). The symptoms of dying children. J. Pain Symp. Manage. 26, 594–603. doi: 10.1016/S0885-3924(03)00202-1
Eckert, S. A. (2002). Swim speed and movement patterns of gravid leatherback sea turtles (Dermochelys coriacea) at St Croix, US Virgin Islands. J. Exp. Biol. 205, 3689–3697.
Ellwood, S. A., Wilson, R. P., and Addison, A. C. (2007). “Technology in conservation: a boon but with small print,” in Key Topics in Conservation Biology, eds D. Macdonald and K. Service (Oxford: Blackwell Publishing), 105–119
Enstipp, M. R., Ciccione, S., Gineste, B., Milbergue, M., Ballorain, K., Ropert-Coudert, Y., et al. (2011). Energy expenditure of freely swimming adult green turtles (Chelonia mydas) and its link with body acceleration. J. Exp. Biol. 214, 4010–4020. doi: 10.1242/jeb.062943
Fahlman, A., Crespo-Picazo, J. L., Sterba-Boatwright, B., Stacy, B. A., and Garcia-Parraga, D. (2017). Defining risk variables causing gas embolism in loggerhead sea turtles (Caretta caretta) caught in trawls and gillnets. Sci. Rep. 7:2739. doi: 10.1038/s41598-017-02819-5
Flint, M., and Bonde, R. K. (2017). “Assessing welfare of individual sirenians in the wild and in captivity,” in Marine Mammal Welfare. Animal Welfare, Vol. 17, ed. A. Butterworth (Cham: Springer), 381–393. doi: 10.1007/978-3-319-46994-2_21
Fraser, D. (2009). Assessing animal welfare: different philosophies, different scientific approaches. Zoo Biol. 28, 507–518. doi: 10.1002/zoo.20253
Gailliot, M. T., Schmeichel, B. J., and Baumeister, R. F. (2006). Self-regulatory processes defend against the threat of death: Effects of self-control depletion and trait self-control on thoughts and fears of dying. J. Personal. Soc. Psychol. 91:49. doi: 10.1037/0022-3514.91.1.49
Gałecki, A., and Burzykowski, T. (eds.). (2013). “Fitting linear models with heterogeneous variance: the gls () function,” in Linear Mixed-Effects Models Using R (New York, NY: Springer), 149–158. doi: 10.1007/978-1-4614-3900-4_8
Gall, S. C., and Thompson, R. C. (2015). The impact of debris on marine life. Mar. Pollut. Bull. 92, 170–179. doi: 10.1016/j.marpolbul.2014.12.041
Garcia-Parraga, D., Crespo-Picazo, J., Bernaldo de Quiros, Y., Cervera, V., Martí-Bonmati, L., Díaz-Delgado, J., et al. (2014). Decompression sickness (‘the bends’) in sea turtles. Dis. Aquat. Organ. 111, 191–205. doi: 10.3354/dao02790
Gasau, S. P., and Ninou, F. A. (2000). Work of the foundation for the conservation and recovery of marine life. Mar. Turtle Newsl. 87, 5–7.
Geertsen, B. M., Teilmann, J., Kastelein, R., Vlemmix, H., and Miller, L. A. (2004). Behaviour and physiological effects of transmitter attachments on a captive harbour porpoise (Phocoena phocoena). J. Cetacean Res. Manage. 6, 139–146.
Gleiss, A. C., Wilson, R. P., and Shepard, E. L. (2011). Making overall dynamic body acceleration work: on the theory of acceleration as a proxy for energy expenditure. Methods Ecol. Evol. 2, 23–33. doi: 10.1111/j.2041-210X.2010.00057.x
Gourkow, N., and Fraser, D. (2006). The effect of housing and handling practices on the welfare, behaviour and selection of domestic cats (Felis sylvestris catus) by adopters in an animal shelter. Anim. Welfare 15, 371–377.
Grandin, T. (1997). Assessment of stress during handling and transport. J. Anim. Sci. 75, 249–257. doi: 10.2527/1997.751249x
Guesgen, M., and Bench, C. (2017). What can kinematics tell us about the affective states of animals? Anim. Welf. 26, 383–397. doi: 10.7120/09627286.26.4.383
Halsey, L. G., Jones, T. T., Jones, D. R., Liebsch, N., and Booth, D. T. (2011a). Measuring energy expenditure in sub-adult and hatchling sea turtles via accelerometry. PLoS ONE. 6:e22311. doi: 10.1371/journal.pone.0022311
Halsey, L. G., Shepard, E. L., and Wilson, R. P. (2011b). Assessing the development and application of the accelerometry technique for estimating energy expenditure. Comp. Biochem. Physiol. Part A 158, 305–314. doi: 10.1016/j.cbpa.2010.09.002
Hawkins, P. (2004). Bio-logging and animal welfare: practical refinements. Mem. Natl. Inst. Polar Res. 58, 58–68.
Hemsworth, P. H., Rice, M., Karlen, M. G., Calleja, L., Barnett, J. L., Nash, J., et al. (2011). Human–animal interactions at abattoirs: Relationships between handling and animal stress in sheep and cattle. Appl. Anim. Behav. Sci. 135, 24–33. doi: 10.1016/j.applanim.2011.09.007
Hill, S. P., and Broom, D. M. (2009). Measuring zoo animal welfare: theory and practice. Zoo Biol. 28, 531–544. doi: 10.1002/zoo.20276
Ismail, A., Rahman, F., Miyazaki, N., and Naito, Y. (2012). Initial application of bio-logging techniques on captive Milky Stork (Mycteria cinerea) in Malaysia. Trop. Ecol. 53, 177–181.
Jambeck, J. R., Geyer, R., Wilcox, C., Siegler, T. R., Perryman, M., Andrady, A., et al. (2015). Plastic waste inputs from land into the ocean. Science 347, 768–771. doi: 10.1126/science.1260352
Jandial, R., Greenberg, M., Aryan, H., Taylor, W., Sutherland-Smith, M., Kearns, K., et al. (2005). Lumbar diskectomy in a human-habituated western lowland gorilla. Internet J. Neurosur. 1, 1–11. doi: 10.5580/17c3
Johnson, M. P., and Tyack, P. L. (2003). A digital acoustic recording tag for measuring the response of wild marine mammals to sound. IEEE J. Ocean. Eng. 28, 3–12. doi: 10.1109/JOE.2002.808212
Kenny, D. A., and Hoyt, W. T. (2009). Multiple levels of analysis in psychotherapy research. Psychother. Res. 19, 462–468. doi: 10.1080/10503300902806681
Ketten, D. R., and Bartol, S. M. (2005). Functional Measures of Sea Turtle Hearing. Woods Hole, MA: Woods Hole Oceanographic Institution. doi: 10.21236/ADA446809
Kooyman, G. L. (2004). Genesis and evolution of bio-logging devices: 1963-2002. Mem. Natl. Inst. Polar Res. 58, 15–22.
Kuhar, C. W. (2006). In the deep end: pooling data and other statistical challenges of zoo and aquarium research. Zoo Biol. 25, 339–352. doi: 10.1002/zoo.20089
Laske, T. G., Garshelis, D. L., and Iaizzo, P. A. (2014). Big data in wildlife research: remote web-based monitoring of hibernating black bears. BMC Physiol. 14:13. doi: 10.1186/s12899-014-0013-1
Lawrence, A. B. (2008). Applied animal behaviour science: past, present and future prospects. Appl. Anim. Behav. Sci. 115, 1–24. doi: 10.1016/j.applanim.2008.06.003
Lennox, R. J., Brownscombe, J. W., Cooke, S. J., and Danylchuk, A. J. (2018). Post-release behaviour and survival of recreationally-angled arapaima (Arapaima cf. arapaima) assessed with accelerometer biologgers. Fisheries Res. 207, 197–203. doi: 10.1016/j.fishres.2018.05.007
Lohmann, K. J., Lohmann, C. M., Ehrhart, L. M., Bagley, D. A., and Swing, T. (2004). Animal behaviour: geomagnetic map used in sea-turtle navigation. Nature 428, 909–910. doi: 10.1038/428909a
Mancini, C. J., Van Der Linden, Bryan, J., and Stuart, A. (2012). “Exploring interspecies sensemaking: dog tracking semiotics and multispecies ethnography,” in Proceedings of the 2012 ACM Conference on Ubiquitous Computing (Pittsburgh, PA), 143–152. doi: 10.1145/2370216.2370239
Mason, G., and Rushen, J. (2008). Stereotypic Animal Behaviour: Fundamentals and Applications to Welfare. Wallingford: CAB International.
Minamikawa, S., Naito, Y., Sato, K., Matsuzawa, Y., Bando, T., and Sakamoto, W. (2000). Maintenance of neutral buoyancy by depth selection in the loggerhead turtle Caretta caretta. J. Exp. Biol. 203, 2967–2975.
Moberg, G. P. (2000). “Biological response to stress: implications for animal welfare,” in The Biology of Animal Stress: Basic Principles and Implications for Animal Welfare, eds G. P. Moberg and J. A. Mnech (London: CABI Publishing), 1–21. doi: 10.1079/9780851993591.0001
Nakagawa, S., and Schielzeth, H. (2013). A general and simple method for obtaining R2 from generalized linear mixed-effects models. Methods Ecol. Evol. 4, 133–142. doi: 10.1111/j.2041-210x.2012.00261.x
Nathan, R., Getz, W. M., Revilla, E., Holyoak, M., Kadmon, R., Saltz, D., et al. (2008). A movement ecology paradigm for unifying organismal movement research. Proc. Natl. Acad. Sci. U.S.A. 105, 19052–19059. doi: 10.1073/pnas.0800375105
Nelms, S. E., Duncan, E. M., Broderick, A. C., Galloway, T. S., Godfrey, M. H., Hamann, M., et al. (2015). Plastic and marine turtles: a review and call for research. ICES J. Mar. Sci. 73, 165–181. doi: 10.1093/icesjms/fsv165
Pagano, A. M., Rode, K. D., Cutting, A., Owen, M., Jensen, S., Ware, J., et al. (2017). Using tri-axial accelerometers to identify wild polar bear behaviors. Endang. Species Res. 32, 19–33. doi: 10.3354/esr00779
Pinheiro, J., Bates, D., DebRoy, S., and Sarkar, D. (2015). R Core Team: nlme: Linear and Nonlinear Mixed Effects Models. R package version 3.1-122. Available online at: http://CRAN.R-project.org/package=nlme
Pinheiro, J. C., and Bates, D. M. (2000). Mixed Effects Models in S and S-Plus. New York, NY: Springer-Verlag. doi: 10.1007/B98882
Portugues, C., Crespo-Picazo, J. L., García-Párraga, D., Altimiras, J., Lorenzo, T., Borque-Espinosa, A., et al. (2018). Impact of gas emboli and hyperbaric treatment on respiratory function of loggerhead sea turtles (Caretta caretta). Conserv. Physiol. 6:cox074. doi: 10.1093/conphys/cox074
Putman, N. F., Endres, C. S., Lohmann, C. M., and Lohmann, K. J. (2011). Longitude perception and bicoordinate magnetic maps in sea turtles. Curr. Biol. 21, 463–466. doi: 10.1016/j.cub.2011.01.057
Qasem, L., Cardew, A., Wilson, A., Griffiths, I., Halsey, L. G., Shepard, E. L., et al. (2012). Tri-axial dynamic acceleration as a proxy for animal energy expenditure; should we be summing values or calculating the vector? PLoS ONE 7:e31187. doi: 10.1371/journal.pone.0031187
Raum-Suryan, K. L., Jemison, L. A., and Pitcher, K. W. (2009). Entanglement of Steller sea lions (Eumetopias jubatus) in marine debris: Identifying causes and finding solutions. Mar. Pollut. Bull. 58, 1487–1495. doi: 10.1016/j.marpolbul.2009.06.004
Roe, J. H., Frank, M. R., Gibson, S. E., Attum, O., and Kingsbury, B. A. (2010). No place like home: an experimental comparison of reintroduction strategies using snakes. J. Appl. Ecol. 47, 1253–1261. doi: 10.1111/j.1365-2664.2010.01886.x
Ropert-Coudert, Y., Brooks, L., Yamamoto, M., and Kato, A. (2009). ECG response of koalas to tourists proximity: a preliminary study. PLoS ONE 4:e7378. doi: 10.1371/journal.pone.0007378
Ropert-Coudert, Y., and Wilson, R. P. (2005). Trends and perspectives in animal-attached remote sensing. Front. Ecol. Environ. 3, 437–444. doi: 10.1890/1540-9295(2005)003[0437:TAPIAR]2.0.CO;2
Rose, N. A., Hancock Snusz, G., Brown, D. M., and Parsons, E. (2017). Improving captive marine mammal welfare in the United States: science-based recommendations for improved regulatory requirements for captive marine mammal care. J. Int. Wildlife Law Policy 20, 38–72. doi: 10.1080/13880292.2017.1309858
Rübel, A., Kuoni, W., and Augustiny, N. (1994). Emerging techniques: CT scan and MRI in reptile medicine. Semin. Avian Exotic Pet Med. 3, 156–160.
Rushen, J. (2003). Changing concepts of farm animal welfare: bridging the gap between applied and basic research. Appl. Anim. Behav. Sci. 81, 199–214. doi: 10.1016/S0168-1591(02)00281-2
Scollo, A., Gottardo, F., Contiero, B., and Edwards, S. A. (2014). Does stocking density modify affective state in pigs as assessed by cognitive bias, behavioural and physiological parameters? Appl. Anim. Behav. Sci. 153, 26–35. doi: 10.1016/j.applanim.2014.01.006
Shepard, E. L. C., Wilson, R. P., Quintana, F., Gómez Laich, A., Liebsch, N., Albareda, D. A., et al. (2008). Identification of animal movement patterns using tri-axial accelerometry. Endang. Species Res. 10, 47–60. doi: 10.3354/esr00084
Sherub, S., Fiedler, W., Duriez, O., and Wikelski, M. (2017). Bio-logging, new technologies to study conservation physiology on the move: a case study on annual survival of Himalayan vultures. J. Comp. Physiol. A 203, 531–542. doi: 10.1007/s00359-017-1180-x
Shirai, M., and Yokoyama, S. (2014). “Grazing behavior and local management of cattle and buffaloes in rural laos,” in Integrated Studies of Social and Natural Environmental Transition in Laos, eds S. Yokoyama, K. Okamoto, C. Takenaka, and I. Hirota (Tokyo: Springer), 63–84. doi: 10.1007/978-4-431-54956-7_4
Shorter, A. K., Shao, Y., Ojeda, L., Barton, K., Rocho-Levine, J., Hoop, J., et al. (2017). A day in the life of a dolphin: using bio-logging tags for improved animal health and well-being. Mar. Mamm. Sci. 33, 785–802. doi: 10.1111/mms.12408
Sikka, P., Corke, P., Valencia, P., Crossman, C., Swain, D., and Bishop-Hurley, G. (2006). “Wireless adhoc sensor and actuator networks on the farm,” in Proceedings of the 5th ACM/IEEE International Conference on Information Processing in Sensor Networks (IPSN) (New York, NY: ACM), 492–499. doi: 10.1145/1127777.1127852
Stelfox, M., Hudgins, J., and Sweet, M. (2016). A review of ghost gear entanglement amongst marine mammals, reptiles and elasmobranchs. Mar. Pollut. Bull. 111, 6–17. doi: 10.1016/j.marpolbul.2016.06.034
Swaisgood, R. R., and Shepherdson, D. J. (2005). Scientific approaches to enrichment and stereotypies in zoo animals: what's been done and where should we go next? Zoo Biol. 24, 499–518. doi: 10.1002/zoo.20066
Teixeira, C. P., De Azevedo, C. S., Mendl, M., Cipreste, C. F., and Young, R. J. (2007). Revisiting translocation and reintroduction programmes: the importance of considering stress. Anim. Behav. 73, 1–13. doi: 10.1016/j.anbehav.2006.06.002
Thomson, J. A., and Heithaus, M. R. (2014). Animal-borne video reveals seasonal activity patterns of green sea turtles and the importance of accounting for capture stress in short-term biologging. J. Exp. Mar. Biol. Ecol. 450, 15–20. doi: 10.1016/j.jembe.2013.10.020
Thornton, S. J., Hochachka, P. W., Crocker, D. E., Costa, D. P., LeBoeuf, B. J., Spielman, D. M., et al. (2005). Stroke volume and cardiac output in juvenile elephant seals during forced dives. J. Exp. Biol. 208, 3637–3643. doi: 10.1242/jeb.01789
Thorup, V. M., Munksgaard, L., Robert, P.-E., Erhard, H., Thomsen, P., and Friggens, N. (2015). Lameness detection via leg-mounted accelerometers on dairy cows on four commercial farms. Animal 9, 1704–1712. doi: 10.1017/S1751731115000890
Turner, L., Udal, M., Larson, B., and Shearer, S. (2000). Monitoring cattle behavior and pasture use with GPS and GIS. Can. J. Anim. Sci. 80, 405–413. doi: 10.4141/A99-093
Valente, A. L. S., Cuenca, R., Zamora, M. A., Parga, M. L., Lavin, S., Alegre, F., et al. (2006). Sectional anatomic and magnetic resonance imaging features of coelomic structures of loggerhead sea turtles. Am. J. Vet. Res. 67, 1347–1353. doi: 10.2460/ajvr.67.8.1347
Vandenabeele, S., Wilson, R., and Grogan, A. (2011). Tags on seabirds: how seriously are instrument-induced behaviours considered? Anim. Welfare UFAW J. 20:559–571.
Veasey, J., Waran, N., and Young, R. (1996). On comparing the behaviour of zoo housed animals with wild conspecifics as a welfare indicator. Anim. Welf. 5, 13–24.
Waiblinger, S., Menke, C., Korff, J., and Bucher, A. (2004). Previous handling and gentle interactions affect behaviour and heart rate of dairy cows during a veterinary procedure. Appl. Anim. Behav. Sci. 85, 31–42. doi: 10.1016/j.applanim.2003.07.002
Walker, J., Borgo, R., and Jones, M. W. (2016). TimeNotes: a study on effective chart visualization and interaction techniques for time-series data. IEEE Trans. Visual. Comput. Graph. 22, 549–558. doi: 10.1109/TVCG.2015.2467751
Walker, J. S., Jones, M. W., Laramee, R. S., Bidder, O. R., Williams, H. J., Scott, R., et al. (2015a). TimeClassifier: a visual analytic system for the classification of multi-dimensional time series data. Vis. Comp. 31, 1067–1078. doi: 10.1007/s00371-015-1112-0
Walker, J. S., Jones, M. W., Laramee, R. S., Holton, M. D., Shepard, E. L., Williams, H. J., et al. (2015b). Prying into the intimate secrets of animal lives; software beyond hardware for comprehensive annotation in ‘Daily Diary’ tags. Mov. Ecol. 3:29. doi: 10.1186/s40462-015-0056-3
Walker, K. A., Trites, A. W., Haulena, M., and Weary, D. M. (2011). A review of the effects of different marking and tagging techniques on marine mammals. Wildlife Res. 39, 15–30. doi: 10.1071/WR10177
Walzer, C., Url, A., Robert, N., Kübber-Heiss, A., Nowotny, N., and Schmidt, P. (2003). Idiopathic acute onset myelopathy in cheetah (Acinonyx jubatus) cubs. J. Zoo Wildlife Med. 34, 36–46. doi: 10.1638/1042-7260(2003)34[0036:IAOMIC]2.0.CO;2
Ward, S. J., and Melfi, V. (2015). Keeper-animal interactions: differences between the behaviour of zoo animals affect stockmanship. PLoS ONE 10:e0140237. doi: 10.1371/journal.pone.0140237
Whitney, N. M., White, C. F., Gleiss, A. C., Schwieterman, G. D., Anderson, P., Hueter, R. E., et al. (2016). A novel method for determining post-release mortality, behavior, and recovery period using acceleration data loggers. Fisheries Res. 183, 210–221. doi: 10.1016/j.fishres.2016.06.003
Williams, H., Shepard, E., Duriez, O., and Lambertucci, S. A. (2015). Can accelerometry be used to distinguish between flight types in soaring birds? Anim. Biotelem. 3:45. doi: 10.1186/s40317-015-0077-0
Williams, H. J., Holton, M. D., Shepard, E. L., Largey, N., Norman, B., Ryan, P. G., et al. (2017). Identification of animal movement patterns using tri-axial magnetometry. Mov. Ecol. 5:6. doi: 10.1186/s40462-017-0097-x
Wilmers, C. C., Nickel, B., Bryce, C. M., Smith, J. A., Wheat, R. E., and Yovovich, V. (2015). The golden age of bio-logging: how animal-borne sensors are advancing the frontiers of ecology. Ecology 96, 1741–1753. doi: 10.1890/14-1401.1
Wilson, A. D., Wikelski, M., Wilson, R. P., and Cooke, S. J. (2015). Utility of biological sensor tags in animal conservation. Conserv. Biol. 29, 1065–1075. doi: 10.1111/cobi.12486
Wilson, R. P., Grundy, E., Massy, R., Soltis, J., Tysse, B., Holton, M., et al. (2014). Wild state secrets: ultra-sensitive measurement of micro-movement can reveal internal processes in animals. Front. Ecol. Environ. 12, 582–587. doi: 10.1890/140068
Wilson, R. P., Holton, M. D., di Virgilio, A., Williams, H., Shepard, E. L., Lambertucci, S., et al. (2018). Give the machine a hand: a Boolean time-based decision-tree template for rapidly finding animal behaviours in multisensor data. Methods Ecol. Evol. 9, 2206–2215. doi: 10.1111/2041-210X.13069
Wilson, R. P., Holton, M. D., Walker, J. S., Shepard, E. L., Scantlebury, D. M., Wilson, V. L., et al. (2016). A spherical-plot solution to linking acceleration metrics with animal performance, state, behaviour and lifestyle. Mov. Ecol. 4:22. doi: 10.1186/s40462-016-0088-3
Wilson, R. P., and McMahon, C. R. (2006). Measuring devices on wild animals: what constitutes acceptable practice? Front. Ecol. Environ. 4, 147–154. doi: 10.1890/1540-9295(2006)004[0147:MDOWAW]2.0.CO;2
Wilson, R. P., Shepard, E. L. C., and Liebsch, N. (2008). Prying into the intimate details of animal lives: use of a daily diary on animals. Endang. Species Res. 4, 123–137. doi: 10.3354/esr00064
Keywords: animal behavior, animal health assessment, archival tag, accelerometer, magnetometer, bycatch, rehabilitation, sea turtle
Citation: Arkwright AC, Archibald E, Fahlman A, Holton MD, Crespo-Picazo JL, Cabedo VM, Duarte CM, Scott R, Webb S, Gunner RM and Wilson RP (2020) Behavioral Biomarkers for Animal Health: A Case Study Using Animal-Attached Technology on Loggerhead Turtles. Front. Ecol. Evol. 7:504. doi: 10.3389/fevo.2019.00504
Received: 16 November 2018; Accepted: 11 December 2019;
Published: 21 January 2020.
Edited by:
Dennis Murray, Trent University, CanadaReviewed by:
Jacob Brownscombe, Dalhousie University, CanadaAdrian C. Gleiss, Murdoch University, Australia
Copyright © 2020 Arkwright, Archibald, Fahlman, Holton, Crespo-Picazo, Cabedo, Duarte, Scott, Webb, Gunner and Wilson. This is an open-access article distributed under the terms of the Creative Commons Attribution License (CC BY). The use, distribution or reproduction in other forums is permitted, provided the original author(s) and the copyright owner(s) are credited and that the original publication in this journal is cited, in accordance with accepted academic practice. No use, distribution or reproduction is permitted which does not comply with these terms.
*Correspondence: Alexandra C. Arkwright, a.arkwright@hotmail.co.uk