- 1Research on the Ecology of Carnivores and Their Prey (RECaP) Laboratory, Department of Fisheries and Wildlife, Michigan State University, East Lansing, MI, United States
- 2Center for Wildlife Management Studies, The School for Field Studies, Karatu, Tanzania
Carnivore depredation of livestock is a global problem which negatively impacts both agropastoral livelihoods and carnivore population viability. Given the gravity of this issue, research has increasingly focused on applied techniques capable of quantifying the factors that increase the risk of livestock depredation. One such technique is risk modeling. This multivariate approach is designed to produce predictions of the spatial configuration of depredation so as to prioritize interventionist activities. Thus, the efficacy of subsequent interventions is, in part, dependent upon the accuracy of the predictions deriving from the risk models. The predictability of spatial patterns in carnivore depredation of livestock is influenced by the degree of spatial autocorrelation evident in the data distributions. We conducted a multi-year assessment to quantify the degree of spatial autocorrelation within livestock depredation data. We centered our study in the Maasai steppe of Tanzania, which experiences some of the highest rates of human-carnivore conflict in the world. We applied three geostatistical measures to assess spatial clustering in data describing livestock depredation by lions (Panthera leo), leopards (Panthera pardus), spotted hyenas (Crocuta crocuta), black-backed jackals (Canis mesomelas), and cheetahs (Acinonyx jubatus) at the household (i.e., livestock enclosure) scale. Using an ordinal spatial scan statistic, a Bernoulli spatial scan statistic, and the Getis-Ord local spatial statistic, we found that the spatial patterns in carnivore depredation of livestock tended not to significantly differ from random. As the predictive ability of spatial risk models may be limited where spatial patterns of carnivore depredation of livestock do not statistically differ from random, explicitly assessing such patterns is an important component of conflict mitigation efforts. We discuss the inferences of this analysis for the optimization of interventionist activities intending to develop sustainable solutions for human-carnivore conflict.
Introduction
Large carnivore hunting and killing of domesticated livestock represents one of the most common triggers of human-carnivore conflict globally (Mizutani, 1999; Frank et al., 2005; Maggi et al., 2014). Within this context, people who have experienced livestock losses will often retaliate against those carnivores perceived to be responsible or in an effort to prevent future livestock losses (Kissui, 2008; Hazzah et al., 2009; Goldman et al., 2013; Dickman et al., 2014; Lichtenfeld et al., 2014; Kahler and Gore, 2015). Termed “livestock depredation,” this driver of conflict has been exacerbated by increasing population growth, range expansion, and meat dependency among the global human population (Naughton-Treves et al., 2003; Treves and Karanth, 2003; Ripple et al., 2014). Today, >75% of the world's large carnivore species are experiencing population declines, and retaliatory killing in response to depredation is one of the primary threats to the conservation of these species (Treves and Karanth, 2003; Linnell et al., 2012; Inskip et al., 2013; Chapron et al., 2014; Ripple et al., 2014). Given the importance of this issue, much research has been devoted to documenting the biotic and abiotic conditions that correlate with carnivore depredation of livestock (Miller, 2015; Montgomery et al., 2018a,b).
Typically, this research seeks to develop predictions capable of optimizing the implementation of interventionist activities meant to decrease carnivore attacks on livestock (Treves et al., 2011; Meena et al., 2014; Miller, 2015). There are a number of models used to predict spatial patterns in carnivore depredation of livestock, which are often referred to as risk models. These models generally fall into one of three categories including correlation modeling, spatial interpolation, and spatial associations (Miller, 2015). Correlation modeling and spatial interpolation inherently test for associations between depredation incidents and the landscapes in which they occur (Hebblewhite et al., 2005; Northrup et al., 2013). Spatial association analyses, in contrast, test for spatial autocorrelation among depredation locations independent of the landscape (Baruch-Mordo et al., 2008; Dale and Fortin, 2014; Peeters et al., 2015). Across the three categories, the models developed to predict carnivore depredation of livestock are all informed by the principles of spatial autocorrelation (Miller, 2015). Thus, if spatial patterns in depredation are spatially autocorrelated then the number of carnivore-killed livestock should exhibit clustering at close distances and dispersion with increasing distance. The calculations of clustering or dispersion are carried out via a comparison of the data to a completely spatial random pattern (Aldstadt, 2010; Chakraborty, 2011; Diggle, 2014).
As such, prior to predictive model fitting, diagnostic tests, including the calculation of spatial autocorrelation, should be assessed (Baruch-Mordo et al., 2008; Chakraborty, 2011; Miller, 2015). If tests of this type are not assessed or described in risk mapping of carnivore depredation of livestock, it is unclear whether measured spatial patterns in these data conform to the principles of spatial autocorrelation. It might be more challenging to derive applied management actions from the outputs of spatial risk models if patterns in carnivore depredation of livestock are not statistically different from random. Correspondingly, this would hamper the implementation of interventions built from those models.
Here we conducted a series of diagnostic tests, typically carried out prior to predictive spatial modeling, to determine the degree of spatial autocorrelation evident in carnivore depredation of livestock data. Our objective was to explicitly assess the assumption of spatial autocorrelation. In doing so, we hope to draw conclusions about important considerations in future depredation risk modeling studies, to increase the efficacy of the management and intervention efforts that are based on such models. As there are multiple possible approaches to testing for spatial autocorrelation within a data set, and given that these tests are rarely described in the risk mapping literature, we used a triangulation approach to further verify our results. We applied three diagnostic tests (the ordinal spatial scan statistic, the Bernoulli spatial scan statistic, and the Getis-Ord local spatial statistic) of spatial autocorrelation to our depredation data. We discuss the results of our analysis for spatial modeling of carnivore depredation data and the interventionist activities that are typically associated with this research. Spatially autocorrelated patterns of livestock depredation are used to inform predictions of future predation risk, and management efforts to reduce this risk. Therefore, the ecological inferences that derive from such analyses have important implications for the optimization of activities that are meant to alleviate conflict between humans and carnivores.
Methods
Study Area
We positioned our study in the Maasai steppe of Northern Tanzania, a 22,000 km2 landscape consisting of a complex matrix of protected areas and village lands (Figure 1). Twenty-three villages with an estimated 350,000 people largely maintaining agro-pastoral lifestyles are interspersed among Tarangire National Park (2,800 km2), Lake Manyara National Park (330 km2), and Manyara Ranch Conservancy (140 km2; Nelson, 2005; Kissui, 2008). These villages are dispersed across a mosaic of wards, a Tanzanian administrative unit consisting of multiple villages. Villages are organized within wards which are organized within districts (see Figure 2). The villages are also flanked to the northwest by the 8,290 km2 Ngorongoro Conservation Area (Figure 1). Livestock-owners keep sheep and goats (collectively referred to as shoats), cattle, and donkeys. All of these livestock are vulnerable to depredation, especially at night when they are herded into enclosures (hereafter referred to as bomas; Ogada et al., 2003, Kissui, 2008). The landscape also supports large numbers of wildlife, including a globally important population stronghold for lions (Panthera leo; see Riggio et al., 2013), as well as robust populations of leopards (Panthera pardus) and spotted hyenas (Crocuta crocuta; Bauer et al., 2004, 2015; Kissui, 2008). Within this system, and in East Africa more broadly, these three species are commonly responsible for the majority of depredation of livestock (Kolowski and Holekamp, 2006; Kissui, 2008; Linnell et al., 2012), though to a lesser extent black-backed jackals (Canis mesomelas) and cheetahs (Acionyx jubatus) also contribute (Maingi et al., under review). Due to the high spatial overlap between human communities and this sympatric suite of carnivores, the Maasai steppe experiences some of the highest rates of human-carnivore conflict triggered by livestock depredation in the world (Graham et al., 2005; Kissui, 2008; Ripple et al., 2014; Mkonyi et al., 2017a,b; Kissui et al., 2019).
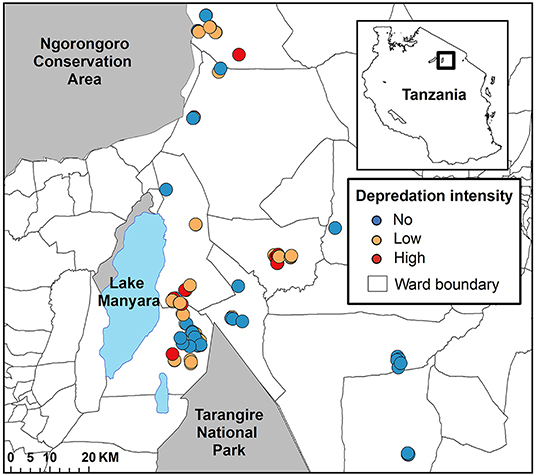
Figure 1. The spatial configuration of bomas in the Maasai steppe of Northern Tanzania. The depredation intensity of each of the 113 bomas between June 2009 and October 2013 is represented by the symbol color. Bomas that experienced no depredation are in blue, those that experienced low depredation intensity are in orange, and those that experienced high depredation intensity are in red.
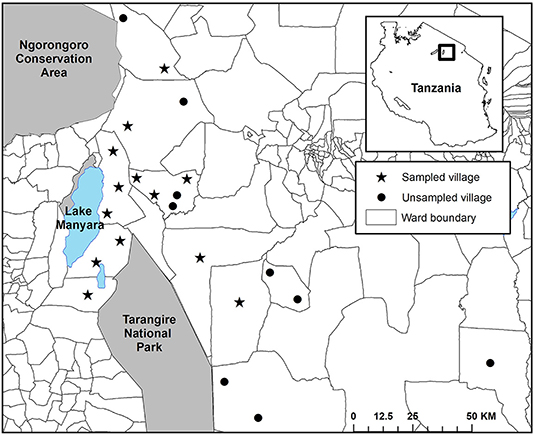
Figure 2. The spatial configuration of villages in the Maasai steppe of Northern Tanzania, categorized by study inclusion. Villages that were sampled for livestock depredation intensity are indicated with a star, those that were not sampled are indicated by a circle.
Data Collection
Between 2009 and 2013, we collected detailed records of livestock depredation events across our study area as part of the Tarangire Lion Project's long-term human-carnivore conflict monitoring program (Kissui, 2008; Kissui et al., 2019; Figure 1). We collected this data among 13 focal villages in the Maasai steppe (Emboreet, Engaruka, Esilalei, Kakoi, Lokisale, Losirwa, Makuyuni, Minjingu, Mswakini, Naiti, Olasiti, Oltukai, and Selela). These villages were distributed among nine distinct wards (Figure 2). We selected bomas for monitoring according to a stratified random sample designed to incorporate the breadth of boma structures present in our study region. We defined a depredation event as a discrete occasion where a carnivore killed or injured ≥1 head of livestock (e.g., cattle, shoats, or donkeys). We collected data on livestock depredation evens through a combined approach, wherein the entire suite of study bomas were monitored through regular revisits on a 30-days cycle and bomas were visited within 24 h of a reported depredation event. In the occasional case when extenuating circumstances made it unfeasible to conduct the standard monthly visits, we applied an additional approach to collect depredation records. In these instances, an additional interview was conducted with the boma owner as soon as possible to collect information on depredation attempts within the previous 30 days. For reported depredation events, initial reports were collected by local residents who were trained to collect detailed records of livestock depredation events. These local assistants then alerted our research team so we were able to conduct a visit to the boma to verify the depredation event via semi-structured interviews with herders or livestock owners. Notably, there is no active compensation scheme for livestock depredation in Tanzania. Thus, there is minimal incentive to report loss of livestock, and it is likely that fewer livestock depredation events were reported than occurred leading to an underestimate in the extent of depredation (Kissui, 2008). At all reported events, we collected the following information: (i) type and number of livestock attacked, (ii) GPS location of the boma, (iii) outcome of the attack (whether the livestock was injured or killed), and (iv) species of the responsible carnivore whenever possible. Identification of the carnivore species responsible for each attack was determined via direct sightings of carnivores by respondents or distinctive tracks, signs, and behavioral characteristics that are commonly known and easily differentiated among the raiding carnivores in the region. Thus, the final database for analysis consisted of multiple depredation events at the household scale (sensu Montgomery et al., 2018a). Each entry included a categorical response variable, with bomas reporting either livestock depredation event = 1 or no event = 0. In the case of the former, the entry included additional details regarding the depredation event. We combined all records by boma, resulting in a total count of depredation events for each boma during the study period. We then categorized these values into three bins, representing the intensity of livestock depredation events at each boma (No, Low, and High). We determined the break values for each category using the Jenks natural breaks method. This method, also known as the goodness of fit variance, reduces within class variance while maximizing variance between classes (Jenks, 1977).
Data Analysis
We evaluated the degree of spatial autocorrelation inherent to these data using the ordinal spatial scan statistic, the Bernoulli spatial scan statistic, and the Getis-Ord local spatial statistic. We chose these three statistical approaches given two key considerations. First, the spatial association analyses conducted had to be capable of accurately testing for spatial autocorrelation among categorical data (i.e., modeling discrete events; see Aldstadt, 2010). As there are multiple ways to do so, we chose to use three different statistical tests as a triangulation approach to verify our results. Second, there is a clear research-implementation gap that separates risk modeling for human-carnivore conflict and the development of policies designed to conserve these species (Miller, 2015; Gray et al., 2019). Thus, our secondary consideration involved the scale of inference of the statistic. We chose statistics with analytical and inferential power at fine scales, as those are the scales most relevant to the implementation of human-carnivore conflict mitigation efforts (Jarvis et al., 2015; Montgomery et al., 2018a).
Ordinal Spatial Scan Statistic
Using SaTScan ver. 9.5 (http://www.satscan.org), we applied the spatial scan statistic to evaluate spatial clustering in the intensity of carnivore depredation of livestock, modeled as an ordinal distribution (Jung et al., 2007). Spatial scan statistics detect spatial or temporal clusters with significantly high or low event occurrence. The resulting clusters are ranked according to the statistical likelihood that the observed event occurrence differs from that in the background population (Kulldorff, 1997, 1999, Fukuda et al., 2005, Riitters and Coulston, 2005). While it has been used in epidemiological studies for decades, the spatial scan statistic has only recently been applied to ecological research. Nevertheless, the statistic has been identified as having great promise for assessments of ecological data (Dale and Fortin, 2014).
Under the ordinal distribution, the probability of depredation of any given intensity (k) occurring within the scanning window (pk) is equal to the probability of depredation of the same intensity outside of the scanning window (qk).
Within this hypothesis testing framework the alternative hypothesis articulates that the detected clusters represent a set of bomas in which the probability of high intensity depredation is significantly (at the α <0.05 level) different than that outside the scanning window. At least one inequality must be strict, and the inequalities can be reversed when assessing for cold spots (Jung et al., 2007).
The test compares all categories individually, as well as in ordered groups. For example, the likelihood of bomas with no depredation can be compared to the likelihood of bomas with low depredation intensity and bomas with high depredation intensity combined. The order of the categories is maintained, and at least one category must be isolated to produce a likelihood ratio ordering (Jung et al., 2007).
Bernoulli Spatial Scan Statistic
Next, we modeled these data using the spatial scan statistic as a Bernoulli distribution (Kulldorff and Nagarwalla, 1995). The Bernoulli distribution allows for an examination of spatial patterns among two states. We first compared bomas with no depredation, to those with high depredation intensity. We then tested bomas with no depredation against those with low or high intensity. Our interest here was to compare bomas with no livestock depredation to those with livestock depredation. Under the null hypothesis in the Bernoulli model, the probability of having a boma with livestock depredation of the specified intensity is the same inside and outside the scanning window (Kulldorff and Nagarwalla, 1995; Kulldorff, 1997). As in the ordinal model, the corresponding alternative hypothesis is that the probability differs within and outside the scanning window. Such a result indicates non-random patterns in the spatial distribution of livestock depredation by carnivores (Chen et al., 2008).
For each of the spatial scan statistics (i.e., the ordinal and Bernouli models), we tested for both low and high clusters. We set the maximum cluster size to 50% of the total population (Jung et al., 2007), and the scanning windows centered on the boma locations. We evaluated the distribution of maximum likelihood under the null hypothesis using the Monte Carlo hypothesis testing set with 999 simulations (Fukuda et al., 2005; Riitters and Coulston, 2005; Jung et al., 2007). In both cases, we mapped the resulting clusters in ArcMap 10.5 (ESRI, Redlands, CA).
Getis-Ord Local Spatial Statistic
Finally, we used the Getis-Ord statistic to evaluate the presence and significance of spatial hot- and cold-spots of depredation intensity in the study area (Getis and Ord, 1992). This statistic measures the degree of association in a given variable by evaluating the level to which each point is surrounded by points with similar values of that variable (Getis and Ord, 1992; Haining, 2003; Ord and Getis, 2010; Peeters et al., 2015). More specifically, compares the concentration of values within a set distance of the point of interest (i.e., the “neighborhood”) to the concentration of values of that variable across the entire study area. Each point is spatially weighted, and the concentration is given by the sum of the values for these points (Getis and Ord, 1992; Baruch-Mordo et al., 2008; Ord and Getis, 2010; Peeters et al., 2015). Thus, this technique allows for the identification of hot spots (i.e., statistically significant clustering) or cold spots (i.e., statistically significant dispersion) in the spatial data. We defined this neighborhood as the ward (see Figure 5). Here;
is defined as:
where the expected value (assuming complete randomness) depends on the number of local neighbors:
measures the degree of association in depredation count for j points within distance d of point i within each ward (Ord and Getis, 1996; Dale and Fortin, 2014). Locations of high spatial association (hot spots) will be indicated with positive z-scores near the maximum ends of the data distribution, while locations of low spatial association (cold spots) will be indicated with low z-scores near the minimum ends of the data distribution. Z-scores >1.96 or < −1.96 indicate significant (at the α <0.05 level) hot spots and cold spots, respectively (Baruch-Mordo et al., 2008; Dale and Fortin, 2014; Meena et al., 2014). We calculated the statistic to identify clusters of bomas based on the intensity of livestock depredation. We used the “zone of indifference” spatial relationship, which is most appropriate for point data without sharp boundaries in neighborhood relationships (Getis and Aldstadt, 2010; Peeters et al., 2015).
Results
Between 2009 and 2013 we collected a total of 170 records from 113 bomas, including 119 confirmed livestock depredation events. Just under half of the bomas surveyed (44.2%, n = 50 of 113) experienced no livestock depredation activity (“no depredation”), 41.6% (n = 47) experienced 1–2 depredation events (“low intensity”), and the remaining 14.2% (n = 16) experienced three or more events (“high intensity”; Table 1). Close to 90% (n = 107) of depredation events were by spotted hyenas, with only 4.2% (n = 5) by leopards, 2.5% (n = 3) each by lions and black-backed jackals, and 0.8% (n = 1) by cheetahs. Hyenas killed livestock at low and high intensity, whereas the other species were only responsible for low intensity depredation at any given boma (Table 2).
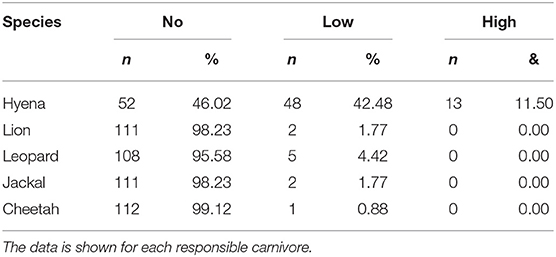
Table 2. The number and percentage of bomas experiencing no, low, and high livestock depredation intensity (see Table 1) in the Maasai steppe, Tanzania collected from 2009 to 2013.
Ordinal Spatial Scan Statistic
Via the ordinal spatial scan statistic we detected two significant clusters (Figure 3). Cluster one was the most likely cluster identified (LLR = 12.68, p < 0.001), while Cluster two (LLR = 9.56, p < 0.05) was a lower-likelihood secondary cluster, with the clusters ordered by their statistical significance (Table 3; Figure 3). Cluster one was a cold spot, in which the number of high intensity depredation bomas was lower than expected, as compared to that in the area outside the scanning window. More specifically, this cluster identified an area with a low number of bomas with low and high depredation intensity combined. Cluster two was a hot spot, identifying an area with a higher than expected number of bomas with high depredation intensity (Figure 3).
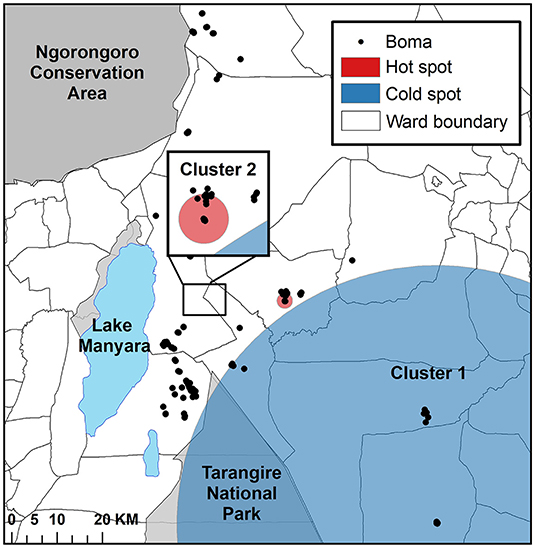
Figure 3. The results of the cluster analysis mapping the intensity of livestock depredation by carnivores in the Maasai steppe, TZ from 2009 to 2013, conducted using the spatial scan statistic under the ordinal model.
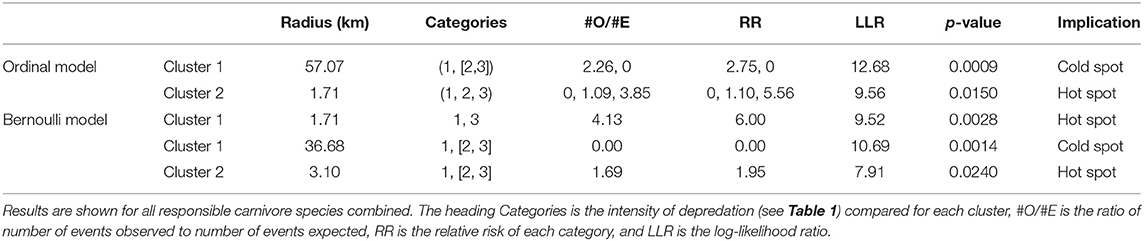
Table 3. The results of the cluster analysis for intensity of livestock depredation by carnivores in the Maasai steppe, Tanzania from 2009 to 2013, conducted using the spatial scan statistic with the ordinal and Bernoulli models.
Bernoulli Spatial Scan Statistic
The Bernoulli spatial scan statistic identified one significant cluster when comparing high depredation intensity bomas to control bomas (Figure 4A; Table 3). This cluster (LLR = 9.52, p < 0.01) was a hot spot, indicating a higher proportion of high intensity bomas inside the scanning window than outside. The second component of the statistic, which compared bomas with low and high depredation intensity combined to bomas with no depredation, revealed two significant clusters (Figure 4B; Table 3). Cluster one (LLR = 10.69, p < 0.01) was a cold spot, and Cluster two (LLR = 7.91, p < 0.05) was a hot spot.
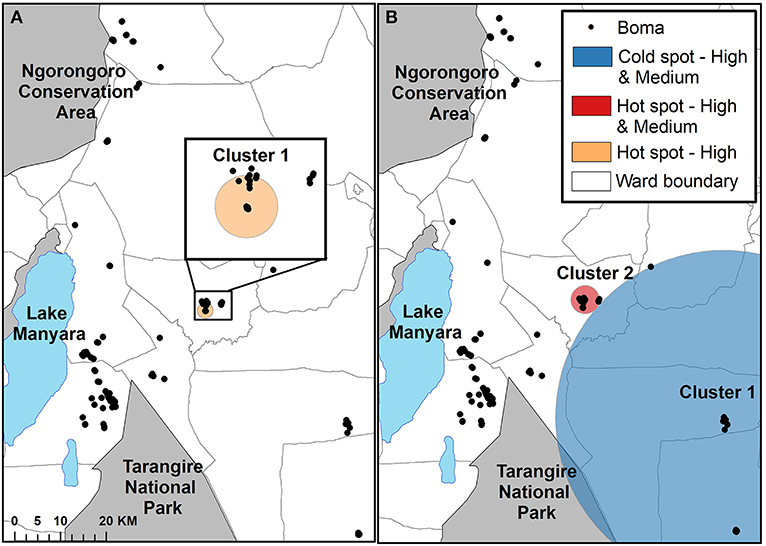
Figure 4. The results of the cluster analysis mapping the intensity of livestock depredation by carnivores in the Maasai steppe, TZ from 2009 to 2013, conducting using the spatial scan statistic under the Bernoulli model. (A) Shows the results for the comparison of high depredation intensity and no depredation. (B) Shows the results for the comparison of low and high depredation intensity combined vs. no depredation.
Getis-Ord Local Spatial Statistic
Application of the Getis-Ord statistic detected 19 bomas (16.8%) that were significantly clustered (i.e., Z-scores of ≥ 1.96). Of these bomas, 18 were tightly clustered within one ward (Figure 5). There were eight bomas with Z-scores of ≤ -1.96, indicating a significant cold spot. These bomas were dispersed in clusters of two to three bomas each, within two neighboring wards (Figure 5).
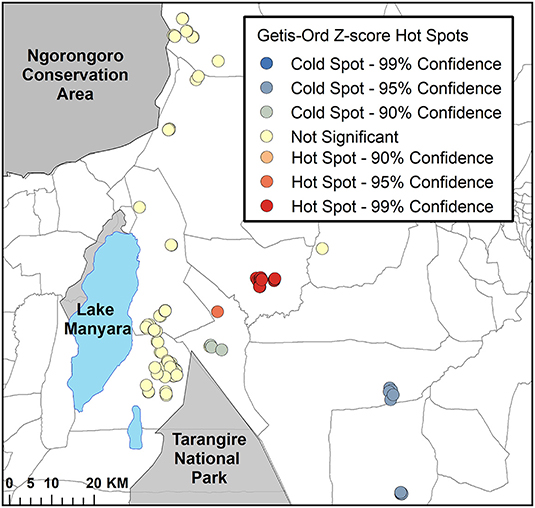
Figure 5. The results of the Getis-Ord hot spot analysis for intensity of livestock depredation in the Maasai steppe, TZ from 2009 to 2013. The bright red circles depict significant clustering of livestock depredation event hot spots (Z ≥ 1.96), and the dark blue circles depict significant cold spots (Z ≤ −1.96).
Discussion
Via the application of three different model diagnostic approaches, we detected little evidence of spatial patterning in the intensity of carnivore depredation of livestock data. All three statistical methods identified just one primary hot spot consisting of only 18 bomas (15.9% of those studied) located in a cluster north of Tarangire National Park (Figures 3–5). Thus, in terms of the intensity of livestock depredation events, the majority of our study site did not differ from a completely spatial random pattern. This result suggests that there may be some other processes, potentially ecological or methodological in form, influencing or obscuring the spatial patterns of livestock depredation in this region. Without an understanding of such processes, and incorporation of that knowledge into spatial pattern analyses of this nature, the ability to develop accurate predictive models for human-carnivore conflict will be limited. Here, we discuss the potential processes that could inform this observed spatial randomness.
We had anticipated that patterns in livestock depredation would be non-random, indicating the presence of behaviorally-grounded carnivore hunting strategies similar to those observed in wild prey predation by the same species. This assumption was supported by previous research showing that the risk of livestock depredation by hyenas increased with vegetative cover (Kolowski and Holekamp, 2006) and depredation risk from lions was significantly higher near riverine habitats (Abade et al., 2014). Nevertheless, the majority of the spatial patterns of livestock depredation events examined here exhibited spatial randomness even though extensive research has documented that large carnivores do not pursue wild prey randomly (Hopcraft et al., 2005; Hayward, 2006; Hayward et al., 2006; MacNulty et al., 2007). As an example, previous research has shown that lions preferentially hunt in areas of semi-dense vegetation and cover, such as tall grasses and open shrublands (Elliott et al., 1977; Scheel, 1992; Spong, 2002; Hopcraft et al., 2005; Fischhoff et al., 2007). This pattern is likely due to the fact that lions are primarily ambush-style predators, relying on vegetation that can hide their presence from prey species until the last possible moment while not restricting their view of potential prey individuals (Hopcraft et al., 2005; Valeix et al., 2009). Similarly, leopards prefer to hunt in areas with moderate woody plant cover, such as open mixed woodlands (Balme et al., 2007). However, substantially less is known about the behaviors of these carnivores in relation to encountering domestic prey. This is particularly true at the household scale (i.e., the scale of bomas; Montgomery et al., 2018a). It remains unclear however, the extent to which hunting behaviors of lions, leopards, or hyenas for wild prey might apply to the selection of livestock for depredation. Therefore, it is possible that the carnivores, in fact, respond to potential livestock prey in the boma randomly.
It is also likely that human presence and activity at the boma contributed to the inherent randomness in the spatial patterns in carnivore depredation of livestock. Livestock husbandry practices, the structural integrity of bomas, as well as cues of human presence including noises, lights, the presence of dogs, and many other elements can be deterrents to large carnivores (Ogada et al., 2003; Frank, 2010; Loveridge et al., 2017). Thus, humans have a capacity to intentionally or inadvertently disturb large carnivores intending to kill livestock at the boma. However, the exact combination of factors that might best deter advancing carnivores has not yet been identified. Importantly, our assessment was focused only on known attacks of livestock, not the other stages of the depredation process (Macarthur and Pianka, 1966; MacNulty et al., 2007; i.e., carnivore search and pursuit of livestock in the boma). To assess how human behavior influences the probability of attack, the rates at which carnivores encounter bomas and do not attack must be calculated. Within wild prey systems, encounter rates are one of the primary determinants of predation intensity (Hebblewhite et al., 2005; Balme et al., 2007). We identify the study of the rates at which carnivores encounter livestock at the boma and do not attack as a productive area of future research.
Finally, the spatial randomness that we observed may also be attributable, at least in part, to noise in the data collection system. Such noise would include issues in sampling, translation, misreporting of depredation events, or spatio-temporal dimensionality. For instance, we considered depredation data from 2009 to 2013, and collapsing the temporal extent of the data could have obscured fine scale temporal dynamics in the depredation patterns. Many studies have shown the importance of temporal resolution in revealing and predicting the mechanisms driving spatial patterning (Elliott et al., 1977; Van Orsdol, 1984; Stander and Albon, 1993). Examining the temporal dynamism associated with these data is part of a separate analysis in which we discovered strong effect of seasonality, with attacks being 2.84 times more likely to occur in the wet season than the dry season, aligning with a similar influence of seasonality found by Kuiper et al. (2015); Kissui et al. (2019). Additionally, our data showed substantial year-to-year variation in hyena depredation patterns (Kissui et al., 2019).
Our study emphasizes the importance of conducting model diagnostic tests of spatial autocorrelation in depredation risk models. Such tests provide the framework for meaningful application of conflict intervention efforts (Baruch-Mordo et al., 2008; Chakraborty, 2011; Miller, 2015). As the principles of spatial autocorrelation underlie the majority of risk model analyses (Chakraborty, 2011; Miller, 2015), without explicit examination of the autocorrelative patterns within the depredation datasets, the results may be misrepresentative of the processes occurring on that landscape. Spatial randomness may indicate that there is no clustering of livestock depredation events, when that result may in fact be due to the presence of other processes that exhibit spatially random patterns at the spatial or temporal scale of assessment. Consideration of such factors is essential for effective application and interpretation of livestock depredation risk models.
The outputs of these risk models are used to identify high-priority locations in which to apply conflict intervention or mitigation efforts around the world, thus informing preventative action to maximize impact and minimize cost (Marucco and Mcintire, 2010; Treves et al., 2011; Miller, 2015). Notably, the exact processes at play may differ depending on the ecological community, human culture, and environmental characteristics of the study location. However, the range of alternative spatial processes identified here are representative of the diversity of factors that should be considered within these examinations. Therefore, such diagnostic approaches can be applied to other study systems to inform subsequent examinations of biotic and abiotic correlates of carnivore depredation of livestock. Without refined understanding of the potential sources of spatial randomness, the model output may not be well-aligned with the implementation of interventions meant to reduce depredation. This misapplication of intervention efforts could result in higher livelihood costs for local communities, increased rates of retaliatory killing of carnivores, and overall increase in conflict between the two (Dickman, 2010; Inskip et al., 2013). Such concerns are not limited to the East African system in which this study is situated, but are relevant to any location experiencing human-carnivore conflict over livestock depredation. The widespread and urgent nature of this threat underscores the necessity of effective use of all available resources and tools, and livestock depredation risk models are a valuable contribution to this effort (Treves et al., 2011; Miller, 2015; Miller et al., 2015). With attention to spatial processes such as those identified here, they are more likely to provide accurate management-relevant predictions of livestock depredation, thus increasing the impact of research-informed conservation efforts and the management practices derived therein.
Data Availability Statement
The datasets generated for this study are available on request to the corresponding author.
Ethics Statement
The data examined herein was approved by the Tanzanian Committee on Science and Technology (COSTECH) and the Tanzanian Wildlife Research Institute (TAWIRI) and all requisite research permits were received. This study was carried out as part of the Tarangire Lion Project's long term monitoring program, did not comprise of any direct, or indirect interactions with wildlife, and did not collect any personal information from participating individuals. Thus, no additional ethics approvals were required.
Author Contributions
All work within this manuscript is original research carried out by the authors. BK developed the original research design and field data collection. CH prepared the data for analysis, carried out the analysis, and drafted the article. RM contributed to the spatial analysis of the data and editing. All authors contributed substantially to the writing and proofing of the article, and read and approved the final submitted version.
Funding
This work was supported by AWF's Charlotte Conservation Fellowship Program, The Lincoln Park Zoo Field Conservation Fund and WCS's Kaplan Award For Wild Cat Conservation.
Conflict of Interest
The authors declare that the research was conducted in the absence of any commercial or financial relationships that could be construed as a potential conflict of interest.
Acknowledgments
We would like to thank TAWIRI and COSTECH (TWRI/RG/ VOL.33/88/91) for providing the permits and research permission to carry out the data collection. Additionally, we thank the Tarangire Lion Project field assistants (Ifura Ukio, Dennis Minja, Peter Perkep) and the village enumerators who assisted with the collection of livestock depredation data.
References
Abade, L., Macdonald, D. W., and Dickman, A. J. (2014). Assessing the relative importance of landscape and husbandry factors in determining large carnivore depredation risk in Tanzania's Ruaha landscape. Biol. Conserv. 180, 241–248. doi: 10.1016/j.biocon.2014.10.005
Aldstadt, J. (2010). “Spatial clustering,” in: Handbook of Applied Spatial Analysis, eds M. M. Fischer and A. Getis (Berlin: Springer-Verlag), 279–300. doi: 10.1007/978-3-642-03647-7_15
Balme, G., Hunter, L., and Slotow, R. (2007). Feeding habitat selection by hunting leopards Panthera pardus in a woodland savanna: prey catchability versus abundance. Anim. Behav. 74, 589–598. doi: 10.1016/j.anbehav.2006.12.014
Baruch-Mordo, S., Breck, S. W., and Wilson, K. R. (2008). Spatiotemporal distribution of black bear-human conflicts in Colorado, USA. J. Wildl. Manage. 72, 1853–1862. doi: 10.2193/2007-442
Bauer, H., Chapron, G., Nowell, K., Henschel, P., Funston, P., Hunter, L. T. B., et al. (2015). Lion (Panthera leo) populations are declining rapidly across Africa, except in intensively managed areas. PNAS 112, 14894–14899. doi: 10.1073/pnas.1500664112
Bauer, H., Van, S., and Merwe, D. (2004). Inventory of free-ranging lions Panthera leo in Africa. Oryx 38, 26–31. doi: 10.1017/S0030605304000055
Chakraborty, J. (2011). “Revisiting Tobler's first law of geography: spatial regression models for assessing environmental justice and health risk disparities,” in: Geospatial Analysis of Environmental Health (Dordrecht: Springer Netherlands), 337–356. doi: 10.1007/978-94-007-0329-2_17
Chapron, G., Kaczensky, P., Linnell, J. D. C., von Arx, M., Huber, D., Andrén, H., et al. (2014). Recovery of large carnivores in Europe's modern human-dominated landscapes. Science. 346, 1517–1519. doi: 10.1126/science.1257553
Chen, J., Roth, R. E., Naito, A. T., Lengerich, E. J., and MacEachren, A. M. (2008). Geovisual analytics to enhance spatial scan statistic interpretation: an analysis of U.S. cervical cancer mortality. Int. J. Health Geogr. 7:57. doi: 10.1186/1476-072X-7-57
Dale, M., and Fortin, J.-M. (2014). Spatial Analysis: A Guide for Ecologists, 2nd edn. Cambridge; Cambridge University Press. doi: 10.1017/CBO9780511978913
Dickman, A. J. (2010). Complexities of conflict: the importance of considering social factors for effectively resolving human-wildlife conflict. Anim. Conserv. 13, 458–466. doi: 10.1111/j.1469-1795.2010.00368.x
Dickman, A. J., Hazzah, L., Carbone, C., and Durant, S. M. (2014). Carnivores, culture and “contagious conflict”: multiple factors influence perceived problems with carnivores in Tanzania's Ruaha landscape. Biol. Conserv. 178, 19–27. doi: 10.1016/j.biocon.2014.07.011
Diggle, P. J. (2014). Statistical Analysis of Spatial and Spatio-Temporal Point Patterns, 3rd Edn. Boca Raton, FL: Taylor & Francis. doi: 10.1201/b15326
Elliott, J. P., Cowan, I. M., and Holling, C. S. (1977). Prey capture by the African lion. Can. J. Zool. 55, 1811–1828. doi: 10.1139/z77-235
Fischhoff, I. R., Sundaresan, S. R., Cordingley, J., and Rubenstein, D. I. (2007). Habitat use and movements of plains zebra (Equus burchelli) in response to predation danger from lions. Behav. Ecol. 18, 725–729. doi: 10.1093/beheco/arm036
Frank, L. (2010). “Living with lions: lessons from Laikipia,” in Conserving Wildlife in African Landscapes: Kenya's Ewaso Ecosystem, eds N. J. Georgiadis (Washington, DC: Smithsonian Institution Scholarly Press), 73–83. doi: 10.5479/si.00810282.632.73
Frank, L., Woodroffe, R., and Ogada, M. O. (2005). “People and predators in Laikipia District, Kenya,” in People and Wildlife: Conflict or Coexistence?, eds R. Woodroffe, S. Thirgood, A. Rabinowitz (Cambridge, UK: Cambridge University Press), 286–304. doi: 10.1017/CBO9780511614774.019
Fukuda, Y., Umezaki, M., Nakamura, K., and Takano, T. (2005). Variations in societal characteristics of spatial disease clusters: examples of colon, lung and breast cancer in Japan. Int. J. Health Geogr. 4:16. doi: 10.1186/1476-072X-4-16
Getis, A., and Aldstadt, J. (2010). Constructing the Spatial Weights Matrix Using a Local Statistic. Berlin, Heidelberg: Springer. 147–163. doi: 10.1007/978-3-642-01976-0_11
Getis, A., and Ord, J. K. (1992). The analysis of spatial association by use of distance statistics. Geogr. Anal. 24, 189–206. doi: 10.1111/j.1538-4632.1992.tb00261.x
Goldman, M. J., De Pinho, J. R., and Perry, J. (2013). Beyond ritual and economics: maasai lion hunting and conservation politics. Oryx 47, 490–500. doi: 10.1017/S0030605312000907
Graham, K., Beckerman, A. P., and Thirgood, S. (2005). Human-predator-prey conflicts: ecological correlates, prey losses and patterns of management. Biol. Conserv. 122, 159–171. doi: 10.1016/j.biocon.2004.06.006
Gray, S. M., Booher, C. R., Elliott, K. C., Kramer, D. B., Waller, J. C., Millspaugh, J. J., et al. (2019). Research-implementation gap limits the actionability of human-carnivore conflict studies in East Africa. Anim. Conserv. 1–11. doi: 10.1111/acv.12520
Haining, R. (2003). Spatial Data Analysis: Theory and Practice. Cambridge; Cambridge University Press. doi: 10.1017/CBO9780511754944
Hayward, M. W. (2006). Prey preferences of the spotted hyaena (Crocuta crocuta) and degree of dietary overlap with the lion (Panthera leo). J. Zool. 270, 606–614. doi: 10.1111/j.1469-7998.2006.00183.x
Hayward, M. W., Henschel, P., O 'brien, J., Hofmeyr, M., Balme, G., Kerley, G.I.H., et al. (2006). Prey preferences of the leopard (Panthera pardus). J. Zool. 270, 298–313. doi: 10.1111/j.1469-7998.2006.00139.x
Hazzah, L., Borgerhoff Mulder, M., and Frank, L. (2009). Lions and Warriors: social factors underlying declining African lion populations and the effect of incentive-based management in Kenya. Biol. Conserv. 142, 2428–2437. doi: 10.1016/j.biocon.2009.06.006
Hebblewhite, M., Merrill, E. H., McDonald Hebblewhite, T. L., and McDonald, T. L. (2005). Spatial decomposition of predation risk using resource selection functions: an example in a wolf-elk predator-prey system. Oikos 111, 101–111. doi: 10.1111/j.0030-1299.2005.13858.x
Hopcraft, J. G. C., Sinclair, A. R. E., Packer, C., and Packert, C. (2005). Planning for success: serengeti lions seek prey accessibility rather than abundance. J. Anim. Ecol. 74, 559–566. doi: 10.1111/j.1365-2656.2005.00955.x
Inskip, C., Ridout, M., Fahad, Z., Tully, R., Barlow, A., Greenwood Barlow, C., et al. (2013). Human-tiger conflict in context: risks to lives and livelihoods in the Bangladesh sundarbans. Source Hum. Ecol. 41, 169–186. doi: 10.1007/s10745-012-9556-6
Jarvis, R. M., Borrelle, S. B., Bollard Breen, B., and Towns, D. R. (2015). Conservation, mismatch and the research-implementation gap. Pacific Conserv. Biol. 21, 105–107. doi: 10.1071/PC14912
Jenks, G. F. (1977). “Optimal data classification for choropleth maps,” Occasional Paper No. 2 (Lawrence, KS: University of Kansas).
Jung, I., Kulldorff, M., and Klassen, A. C. (2007). A spatial scan statistic for ordinal data. Stat. Med. Stat. Med. 26, 1594–1607. doi: 10.1002/sim.2607
Kahler, J. S., and Gore, M. L. (2015). Local perceptions of risk associated with poaching of wildlife implicated in human-wildlife conflicts in Namibia. Biol. Conserv. 189, 49–58. doi: 10.1016/j.biocon.2015.02.001
Kissui, B., Kiffner, C., Konig, H., and Montgomery, R. (2019). Patterns of livestock depredation and cost-effectiveness of fortified livestock enclosures in Northern Tanzania. Ecol. Evol. 9, 11420–11433. doi: 10.1002/ece3.5644
Kissui, B. M. (2008). Livestock predation by lions, leopards, spotted hyenas, and their vulnerability to retaliatory killing in the Maasai steppe, Tanzania. Anim. Conserv. 11, 422–432. doi: 10.1111/j.1469-1795.2008.00199.x
Kolowski, J. M., and Holekamp, K. E. (2006). Spatial, temporal, and physical characteristics of livestock depredations by large carnivores along a Kenyan reserve border. Biol. Conserv. 128, 529–541. doi: 10.1016/j.biocon.2005.10.021
Kuiper, T. R., Loveridge, A. J., Parker, D. M., Johnson, P. J., Hunt, J. E., Stapelkamp, B., et al. (2015). Seasonal herding practices influence predation on domestic stock by African lions along a protected area boundary. BIOC 191, 546–554. doi: 10.1016/j.biocon.2015.08.012
Kulldorff, M. (1997). A spatial scan statistic. Commun. Stat. 26, 1481–1496. doi: 10.1080/03610929708831995
Kulldorff, M. (1999). Spatial Scan Statistics: Models, Calculations, and Applications, in: Scan Statistics and Applications. Boston, MA: Birkhäuser Boston, 303–322. doi: 10.1007/978-1-4612-1578-3_14
Kulldorff, M., and Nagarwalla, N. (1995). Spatial disease clusters: detection and inference. Stat. Med. 14, 799–810. doi: 10.1002/sim.4780140809
Lichtenfeld, L. L., Trout, C., and Kisimir, E. L. (2014). Evidence-based conservation: predator-proof bomas protect livestock and lions. Biodivers. Conserv. 24, 483–491. doi: 10.1007/s10531-014-0828-x
Linnell, J. D., Odden, J., and Mertens, A. (2012). Mitigation Methods for Conflicts Associated With Carnivore Depredation on Livestock in Carnivore Ecology and Conservation: A Handbook of Techniques—Google Books. Oxford: Oxford University Press. doi: 10.1093/acprof:oso/9780199558520.003.0014
Loveridge, A. J., Kuiper, T., Parry, R. H., Sibanda, L., Hunt, J. H., Stapelkamp, B., et al. (2017). Bells, bomas and beefsteak: complex patterns of human-predator conflict at the wildlife-agropastoral interface in Zimbabwe. PeerJ 5:e2898. doi: 10.7717/peerj.2898
Macarthur, R. H., and Pianka, E. R. (1966). On optimal use of a patchy environment. Am. Nat. 100, 603–609. doi: 10.1086/282454
MacNulty, D. R., Mech, L. D., and Smith, D. W. (2007). A proposed ethogram of large-carnivore predatory behavior, exemplified by the wolf. J. Mammal. 88, 595–605. doi: 10.1644/06-MAMM-A-119R1.1
Maggi, C., Keane, A., and Cotterill, A. (2014). Assessing Landscape-Level Variations in Human Propensity to Kill Lions in Samburu, Isiolo and Laikipia Counties, Northern Kenya. London: Imperial College London.
Marucco, F., and Mcintire, E. J. B. (2010). Predicting spatio-temporal recolonization of large carnivore populations and livestock depredation risk: wolves in the Italian Alps. J. Appl. Ecol. 47, 789–798. doi: 10.1111/j.1365-2664.2010.01831.x
Meena, V., Macdonald, D. W., and Montgomery, R. A. (2014). Managing success: asiatic lion conservation, interface problems and peoples' perceptions in the Gir Protected Area. Biol. Conserv. 174, 120–126. doi: 10.1016/j.biocon.2014.03.025
Miller, J. R. B. (2015). Mapping attack hotspots to mitigate human-carnivore conflict: approaches and applicatoins of spatial predation risk modeling. Biodivers. Conserv. 24, 2887–2991. doi: 10.1007/s10531-015-0993-6
Miller, J. R. B., Jhala, Y. V., Jena, J., and Schmitz, O. J. (2015). Landscape-scale accessibility of livestock to tigers: implications of spatial grain for modeling predation risk to mitigate human-carnivore conflict. Ecol. Evol. 5, 1354–1367. doi: 10.1002/ece3.1440
Mizutani, F. (1999). Impact of leopards on a working ranch in Laikipia, Kenya. Afr. J. Ecol 37, 211–225. doi: 10.1111/aje.1999.37.2.211
Mkonyi, F. J., Estes, A. B., Msuha, M. J., Lichtenfeld, L. L., and Durant, S. M. (2017a). Local Attitudes and Perceptions Toward Large Carnivores in a Human-Dominated Landscape of Northern Tanzania. Int. J. 22, 314–330. doi: 10.1080/10871209.2017.1323356
Mkonyi, F. J., Estes, A. B., Msuha, M. J., Lichtenfeld, L. L., and Durant, S. M. (2017b). Socio-economic correlates and management implications of livestock depredation by large carnivores in the Tarangire ecosystem, northern Tanzania. Int. J. Biodivers. Sci. Ecosyst. Serv. Manage. 13, 248–263. doi: 10.1080/21513732.2017.1339734
Montgomery, R. A., Elliott, K. C., Hayward, M. W., Gray, S. M., Millspaugh, J. J., Riley, S. J., et al. (2018b). Examining evident interdisciplinarity among prides of lion researchers. Front. Ecol. Evol. 6, 1–13. doi: 10.3389/fevo.2018.00049
Montgomery, R. A., Hoffmann, C. F., Tans, E. D., and Kissui, B. (2018a). Discordant scales and the potential pitfalls for human-carnivore conflict mitigation. Biol. Conserv. 224, 170–177. doi: 10.1016/j.biocon.2018.05.018
Naughton-Treves, L., Grossberg, R., and Treves, A. (2003). Paying for tolerance: rural citizens' attitudes toward wolf depredation and compensation. Conserv. Biol. 17, 1500–1511. doi: 10.1111/j.1523-1739.2003.00060.x
Nelson, F. (2005). Wildlife Management and Village Land Tenure in Northern Tanzania. Tanzania Natural Resource Forum Occasional Paper No. 6.
Northrup, J. M., Hooten, M. B., Anderson, C. R., and Wittemyer, G. (2013). Practical guidance on characterizing availability in resource selection functions under a use-availability design. Ecology. doi: 10.1890/12-1688.1
Ogada, M. O., Woodroffe, R., Oguge, N. O., and Frank, L. G. (2003). Limiting depredation by African carnivores: the role of livestock husbandry. Conserv. Biol. 17, 1521–1530. doi: 10.1111/j.1523-1739.2003.00061.x
Ord, J. K., and Getis, A. (1996). “Local Spatial Statistics,” in Spatial Analysis: Modeling in a GIS Environment, eds P. Longley and M. Batty (New York, NY: John Wiley and Sons, Inc.). p. 261–278.
Ord, J. K., and Getis, A. (2010). Local spatial autocorrelation statistics: distributional issues and an application. Geogr. Anal. 27, 286–306. doi: 10.1111/j.1538-4632.1995.tb00912.x
Peeters, A., Zude, M., Käthner, J., Ünlü, M., Kanber, R., Hetzroni, A., et al. (2015). Getis–Ord's hot- and cold-spot statistics as a basis for multivariate spatial clustering of orchard tree data. Comput. Electron. Agric. 111, 140–150. doi: 10.1016/j.compag.2014.12.011
Riggio, J., Jacobson, A., Dollar, L., Bauer, H., Becker, M., Dickman, A., et al. (2013). The size of savannah Africa: a lion's (Panthera leo) view. Biodivers. Conserv. 22, 17–35. doi: 10.1007/s10531-012-0381-4
Riitters, K. H., and Coulston, J. W. (2005). Hot spots of perforated forest in the Eastern United States. Environ. Manage. 35, 483–492. doi: 10.1007/s00267-003-0220-1
Ripple, W. J., Estes, J. A., Beschta, R. L., Wilmers, C. C., Ritchie, E. G., Hebblewhite, M., et al. (2014). Status and ecological effects of the world's largest carnivores. Science. 343, 151–162. doi: 10.1126/science.1241484
Scheel, D. (1992). Profitability, encounter rates, and prey choice of African lions. Behav. Ecol. 4, 90–97. doi: 10.1093/beheco/4.1.90
Spong, G. (2002). Space use in lions, Panthera leo, in the selous game reserve: social and ecological factors. Behav. Ecol. Sociobiol. 52, 303–307. doi: 10.1007/s00265-002-0515-x
Stander, P. E., and Albon, S. D. (1993). Hunting success of lions in a semi-arid environment. Zool. Symp. 65, 127–143.
Treves, A., and Karanth, A. K. U. (2003). Human-carnivore conflict and perspectives on carnivore management worldwide. Conserv. Biol. 17, 1491–1499. doi: 10.1111/j.1523-1739.2003.00059.x
Treves, A., Martin, K. A., Wydeven, A. P., and Wiedenhoeft, J. E. (2011). Forecasting environmental hazards and the application of risk maps to predator attacks on livestock. BioScience 61, 451–458. doi: 10.1525/bio.2011.61.6.7
Valeix, M., Loveridge, A. J., Chamaillé-Jammes, S., Davidson, Z., Murindagomo, F., Fritz, H., et al. (2009). Behavioral adjustments of African herbivores to predation risk by lions: spatiotemporal variations influence habitat use. Ecology 90, 23–30. doi: 10.1890/08-0606.1
Keywords: human-carnivore conflict, livestock depredation, spatial autocorrelation, risk modeling, conflict intervention
Citation: Hoffmann CF, Kissui BM and Montgomery RA (2019) Spatial Pattern Analysis Reveals Randomness Among Carnivore Depredation of Livestock. Front. Ecol. Evol. 7:478. doi: 10.3389/fevo.2019.00478
Received: 30 August 2019; Accepted: 25 November 2019;
Published: 11 December 2019.
Edited by:
Tasos Hovardas, University of Cyprus, CyprusReviewed by:
Carlos Bautista, Institute of Nature Conservation, Polish Academy of Sciences, PolandDaniel M. Parker, University of Mpumalanga, South Africa
Copyright © 2019 Hoffmann, Kissui and Montgomery. This is an open-access article distributed under the terms of the Creative Commons Attribution License (CC BY). The use, distribution or reproduction in other forums is permitted, provided the original author(s) and the copyright owner(s) are credited and that the original publication in this journal is cited, in accordance with accepted academic practice. No use, distribution or reproduction is permitted which does not comply with these terms.
*Correspondence: Claire F. Hoffmann, hoffm523@msu.edu
†ORCID: Claire F. Hoffmann orcid.org/0000-0001-7312-4459