- 1Department of Biology, Environment and Climate Change Canada, Canadian Rivers Institute, University of New Brunswick, Fredericton, NB, Canada
- 2Faculty of Forestry and Environmental Management, University of New Brunswick, Fredericton, NB, Canada
- 3Great Lakes Forestry Centre, Natural Resources Canada, Marie, ON, Canada
- 4Centre for Biodiversity Genomics and Department of Integrative Biology, University of Guelph, Guelph, ON, Canada
- 5Department for Fisheries and Oceans, Gulf Fisheries Centre, Moncton, NB, Canada
An ongoing challenge for ecological studies has been the collection of data with high precision and accuracy at a suitable scale to detect and manage critical global change processes. A major hurdle has been the time-consuming and challenging process of sorting and identification of organisms, but the rapid development of DNA metabarcoding as a biodiversity observation tool provides a potential solution. As high-throughput sequencing becomes more rapid and cost-effective, a “big data” revolution is anticipated, based on higher and more accurate taxonomic resolution, more efficient detection, and greater sample processing capacity. These advances have the potential to amplify the power of ecological studies to detect change and diagnose its cause, through a methodology termed “Biomonitoring 2.0.” Despite its promise, the unfamiliar terminology and pace of development in high-throughput sequencing technologies has contributed to a growing concern that an unproven technology is supplanting tried and tested approaches, lowering trust among potential users, and reducing uptake by ecologists and environmental management practitioners. While it is reasonable to exercise caution, we argue that any criticism of new methods must also acknowledge the shortcomings and lower capacity of current observation methods. Broader understanding of the statistical properties of metabarcoding data will help ecologists to design, test and review evidence for new hypotheses. We highlight the uncertainties and challenges underlying DNA metabarcoding and traditional methods for compositional analysis, specifically comparing the interpretation of otherwise identical bulk-community samples of freshwater benthic invertebrates. We explore how taxonomic resolution, sample similarity, taxon misidentification, and taxon abundance affect the statistical properties of these samples, but recognize these issues are relevant to applications across all ecosystem types. In conclusion, metabarcoding has the capacity to improve the quality and utility of ecological data, and consequently the quality of new research and efficacy of management responses.
Introduction
Biodiversity loss and the risks it poses to ecosystem functions and services remain a major societal concern (Cardinale et al., 2012), but due to a lack of consistently-observed data, there is no consensus regarding the speed or severity of this decline (Vellend et al., 2013; Newbold et al., 2015). There are very few ecosystems in which we can quantify the magnitude of degradation, nor can we discriminate among multiple stressors, both key goals for environmental monitoring programs (Bonada et al., 2006). The power to detect change in ecological communities has been hampered by sampling costs predominantly associated with skilled human labor and travel. As a result, ecosystem monitoring programs must manage a trade-off between the scope of a study, including the phylogenetic breadth of taxon coverage and the resolution to which taxa are described (our universe of observation), and its spatial and temporal coverage (e.g., tropical forests Gardner et al., 2008; marine sediments Musco et al., 2009). A history of such trade-offs has led to entrenched practices relying on observation of a narrow range of taxa, which aim to provide a surrogate for the full biodiversity complement, yet whose taxonomic, spatial, or temporal relationships are largely undefined (Lindenmayer and Likens, 2011). Landscapes are under increasing stress from multiple drivers, and yet the troubling reality is that management decisions are informed by very limited and potentially biased information, generated by approaches that no longer reflect our understanding of how ecosystems and species interact (Woodward et al., 2013).
Fortunately, technological advances offer the opportunity to generate high-quality biodiversity data in a consistent manner, increasingly automating processing pipelines, and radically expanding the scope of ecosystem monitoring (Turner, 2014; Bush et al., 2017). One of the most promising of these is the technique of DNA metabarcoding, which supports the massively-parallelized, and hence high-throughput, taxonomic identification of organism assemblages within a biological sample. While single-specimen DNA barcoding uses short genetic sequences to identify individual taxa, often at the species-level, metabarcoding supports simultaneous identification of entire assemblages via high-throughput sequencing (Taberlet et al., 2012; Yu et al., 2012). The application of metabarcoding for ecosystem monitoring has been termed “Biomonitoring 2.0” (Baird and Hajibabaei, 2012) because it could provide a universal platform to identify any, and potentially all, phylogenetic groups occurring within an ecosystem, including many taxa currently not identifiable by expert taxonomists (e.g., streams: Sweeney et al., 2011; rainforest: Brehm et al., 2016; marine zooplankton: Zhang et al., 2018). As DNA sequencing capacity continues to increase, there is a growing interest from ecological researchers and environmental managers for guidance in how to apply these new tools, and to provide clear evidence of their value relative to existing microscopy-based methods. However, it is important to emphasize that comparisons between traditional morphological identifications and DNA sequences are far from straightforward. For example, while metabarcoding can observe the occurrence of DNA sequences within a specified environmental matrix (e.g., soil sample), it does not discriminate between intact, living organisms, and their presence as parts, ingested, or extraneous tissue. While some may see this as a challenge to be overcome, to retrofit a new method to an old system of observation, we view this as an opportunity to expand our universe of interest, and gain new insight into metacommunity assembly and structure (Bohan et al., 2017). We draw on recent research into metabarcoding of freshwater macroinvertebrates to illustrate these issues, the most widely applied non-microbial applications of DNA metabarcoding to date, but many of the analytical concepts we discuss will be common to other ecosystems and assemblages.
Aquatic researchers have long recognized the challenges of taxonomic identification and resulting limitations it imposes on the scale and scope of observational, experimental and monitoring studies (Jones, 2008). Freshwater monitoring programs rely upon a subset of taxa, primarily aquatic macroinvertebrates, fish, or algae, with little consistency across environmental agencies or regions (Friberg et al., 2011), although we acknowledge efforts in Europe to rectify these divides (Birk et al., 2012). Sparse spatial and temporal coverage and limited taxonomic resolution (e.g., Orlofske and Baird, 2013) ultimately constrains outcomes to “pass/fail” (impacted/non-impacted; Clarke et al., 2006; Strachan and Reynoldson, 2014), with causes of degradation inferred rather than supported by direct evidence. After decades of research, our ability to disentangle the influence of even the most basic drivers that impact the state of freshwater ecosystems is still limited (Woodward et al., 2013). Given the challenges faced by aquatic ecologists it is not surprising that within a decade of the first preliminary studies (Hajibabaei et al., 2011), attention is now focused on how to overcome the barriers to full-scale implementation (e.g., technological and regulatory Keck et al., 2017; Hering et al., 2018; Leese et al., 2018; Porter and Hajibabaei, 2018a). It is therefore timely to highlight how the interpretation of metabarcoding and traditional morphological identification differ, their sources of error, and sources of uncertainty.
Our Unit and Universe of Observation
The science of aquatic biomonitoring is based on the principle that site-level observations of biological assemblages integrate responses to prevailing environmental conditions over space and time, reducing the intensity of sampling required to detect stressor-related changes in the environment, and providing an immediate signal of “ecosystem health” (Friberg et al., 2011). However, consistently observing more than a narrow range of taxa within an ecological community has proved costly and impractical, with accuracy of identification often unrecorded or difficult to quantify, and varying across taxa. The observation universe is further constrained by sampling methods (e.g., mesh-size of collection nets), rather than common phylogenetic or ecological characteristics, with further downgrading or exclusion of groups that are difficult to identify (e.g., Vlek et al., 2006). Even with the best taxonomic expertise available, it is practically impossible to identify all specimens to species-level, since many early life-stages lack necessary diagnostic features (Orlofske and Baird, 2013). Species are subsequently aggregated at higher taxonomic ranks, obscuring species-level responses, constraining our knowledge of whether species' environmental preferences are conserved or variable (Macher et al., 2016; Beermann et al., 2018). In our view, the level of observation provided by direct morphological identification of biological specimens in a sample is highly variable (typically referred to as “lowest taxonomic level”), disconnected from ecological theory, and contains an unknown, yet potentially significant degree of bias (Jones, 2008; Nakov et al., 2018).
DNA metabarcoding offers the potential to reduce many of the costs involved in routine morphological identification (Ji et al., 2013), and can also generate a richer list of taxa (Sweeney et al., 2011; Gibson et al., 2015). Taxonomic assignment is continually improving as DNA-barcode reference libraries expand (e.g., Curry et al., 2018; Weigand et al., 2019), and in contrast to morphological approaches, a universe of observation defined by the DNA region and primers (see below) is less ambiguous. The opportunity this represents has triggered a wide range of metabarcoding studies in aquatic ecosystems (e.g., rivers Hajibabaei et al., 2011; wetlands Gibson et al., 2015; lakes Bista et al., 2017), and applied to describe community composition in a wide variety of taxa (e.g., worms Vivien et al., 2015; insects Emilson et al., 2017; diatoms Vasselon et al., 2017).
The Universe of Observation for Monitoring With Metabarcoding in Freshwaters
While metabarcoding offers the potential to observe a greater diversity of taxa, a crucial step for any metabarcoding study is the selection of primers used to amplify specific DNA sequence marker regions, as they determine the taxonomic groups under study and resolution of assignment (Hajibabaei et al., 2012; Gibson et al., 2014). In order to expand taxonomic coverage, it is necessary to employ a range of primers, and marker sequences (see Figure 3 in Gibson et al., 2014). The cost of sequencing additional primers can therefore limit the number of sites surveyed, but these costs may rapidly decline as automated processing becomes available. Refining primers for different taxonomic groups or species has taken considerable effort, but primers with broad coverage for invertebrates have now been established (e.g., Hajibabaei et al., 2012; Elbrecht and Leese, 2017). However, amplification bias due to variable affinity among sequence variants for amplification can distort the relationship between sample biomass and the number of sequence reads (Elbrecht and Leese, 2015; Zhang et al., 2018). Metabarcoding can therefore support a taxonomically broad universe of observation, but outputs should be treated as occurrences and do not support reliable estimation of organism biomass or abundance.
Another key issue is the distinction between bulk-community sampling and environmental DNA (eDNA). eDNA samples focus on a signal derived predominantly from traces of intracellular and extracellular DNA without attempting to isolate organisms (e.g., from water or soil; Deiner et al., 2017; Cristescu and Hebert, 2018), whereas bulk-community samples include eDNA, but target the collection of whole organisms. eDNA can be effective in detecting biological signal from the environment, but the significant spatial and temporal uncertainty of that signal clouds its application in observational studies. In addition, the ease with which trace amounts of DNA can be transported makes cross-contamination a critical issue for eDNA studies (i.e., the addition of false-positives Ficetola et al., 2015), whereas the high concentrations of template material in bulk samples mean this is less of a concern (Majaneva et al., 2018). As a result, our examples of metabarcoding below focus entirely on observations derived from unsorted bulk-community samples that are otherwise identical to traditional monitoring surveys.
Interpretation
The statistical power and precision of any ecological assessment that is based on sample assemblage composition depends upon how results are aggregated and analyzed, how misidentification (i.e., false-presences and false-absences) can obscure expectations when setting the baseline composition, limiting our ability to detect deviations from this baseline and infer that change has occurred (e.g., Clarke et al., 2002; Clarke, 2009). Although many sources of uncertainty affect our ability to infer regional and landscape-level trends from site-level observations, these are difficult to address with traditional approaches (Clarke, 2009; Carstensen and Lindegarth, 2016). To illustrate this problem, and whether metabarcoding can alleviate it, we focus on how four sources of error involved in describing freshwater biodiversity differ between morphological and metabarcoding workflows: (a) taxonomic resolution, (b) replicate similarity, (c) taxonomic misidentification, and (d) quantitative measures like abundance.
Taxonomic Resolution
Biomonitoring 2.0 (Baird and Hajibabaei, 2012) employs metabarcoding to overcome the taxonomic bottleneck of sample processing, removing a critical trade-off between sample taxonomic resolution and the number of samples that can be studied (Jones, 2008). Moreover, sample metrics derived from higher taxonomic categories, such as family- or genus-level, make a tacit assumption that species within those higher categories share similar environmental responses, and possess similar ecological functions. However, when studies are able to differentiate taxa at the species level, they may reject this assumption (e.g., nutrient and sediment sensitivity; Macher et al., 2016; Beermann et al., 2018), and this can significantly influence study outcomes (Hawkins et al., 2000; Schmidt-Kloiber and Nijboer, 2004; Sweeney et al., 2011).
Observing taxonomic assemblages at genus- or family-level masks turnover in composition, reducing our power to detect subtle changes among communities over space and time. As each species is less common than its parent taxonomic group, there will be fewer observations with which to establish reliable associations, and their inclusion could add noise to statistical models, echoing the long-running debate about the value of rare taxa in biomonitoring (Nijboer and Schmidt-Kloiber, 2004; Lavoie et al., 2009). This “noise” is not only due to the stochastic occurrence of uncommon species, but also sampling error, which can be quantified using hierarchical occupancy models (Clarke, 2009; Guillera-Arroita, 2017). We should therefore be particularly cautious about concluding how taxonomic resolution affects the strength of statistical relationships (Arscott et al., 2006; Martin et al., 2016). Instead, our current challenge is understanding when these subtle changes, previously invisible to traditional monitoring, are related to natural environmental factors or anthropogenic disturbance.
One criticism of DNA metabarcoding is that high taxonomic resolution is not valuable if those taxa cannot be linked to a binomial taxonomic name, a limitation that emerges when barcode reference libraries are incomplete (Curry et al., 2018). However, many methods of ecological assessment evaluate community level characteristics such as alpha- and beta-diversity, and therefore do not retain taxon identity, particularly at the species-level (Birk et al., 2012). For this reason, interest in taxonomy-free approaches is increasing among those studying poorly-known assemblages whose morphological identification is challenging (e.g., meiofauna or diatoms; Vasselon et al., 2017). Clearly defining the unit and universe of observation (i.e., taxonomic breadth and resolution) is fundamental to comparing such characteristics (Cordier et al., 2018; Pawlowski et al., 2018), but doing so could also improve compatibility between biogeographically separated programs (Turak et al., 2017; Bailet et al., 2019). Nonetheless, to tie DNA-based monitoring to historic surveys, and to assign ancillary information such as traits, it is still a requirement to assign taxonomic names to identified sequences (e.g., Compson et al., 2018). Based on the wealth of ecological information available that could complement DNA-based ecological studies, and the considerable body of legacy data generated by historical studies, including regulatory monitoring, increasing reference library coverage should be a priority for management agencies transitioning to DNA-based surveys (Rimet et al., 2018; Stokstad, 2018; Weigand et al., 2019).
Replicate Similarity
Depending on the scale of observation, species are rarely distributed randomly or uniformly in nature (e.g., Soininen et al., 2016). For example, the distribution of macroinvertebrate taxa in streams is notoriously dynamic, as species adjust to changes in both abiotic (e.g., flow velocity, substratum size) and biotic (e.g., fish predation, mussel aggregation) factors (Downes et al., 1993; Vaughn and Spooner, 2006). Heterogeneity may also result from stochastic processes such as dispersal and colonization (Fonseca and Hart, 2001), ephemeral resources (Lancaster and Downes, 2014), or disturbance regimes at multiple scales (Effenberger et al., 2006). Indeed, heterogeneity is so pervasive that a shift toward greater homogeneity within aquatic communities could indicate human modification of the landscape (Petsch, 2016). Given such heterogeneity, the challenge for ecological studies or biomonitoring is to detect a sufficient proportion of the community, whilst also minimizing processing costs, so that further detections are unlikely to alter the interpretation of subsequent analyses. Counting all individuals in a sample can have value, but it is prohibitive for routine observational studies, and not cost-effective for biomonitoring purposes (e.g., Vlek et al., 2006). Most studies therefore employ subsampling (i.e., identifying a subset of individuals collected from the field) to reduce the time, effort, and cost of processing macroinvertebrate samples. However, reducing the effort per sampling unit can significantly underestimate the richness per sample (Doberstein et al., 2000; Buss et al., 2014) and although subsampling is standardized by volume, time, weight, or number of individuals, it is often difficult to compare among survey methods and biomonitoring schemes (Buss et al., 2014). Although sensitivity to subsampling depends on the metric employed, subsampling can substantially increase the misclassification of site status (Clarke et al., 2006; Petkovska and Urbanič, 2010), and exaggerate the perceived rarity of many taxa, whose exclusion from analyses may further bias interpretations of condition (Schmidt-Kloiber and Nijboer, 2004).
Regardless of the sub-sampling approach, a single sample only recovers a subset of the community, particularly in heterogeneous environments. As sampling effort increases, either by area or time, more taxa are recovered until the rate of new discoveries declines (Vlek et al., 2006). The rate of accumulation depends on taxon abundance distributions, their dispersion, and ease of collection, including the effects of environment on collection efficiency (Guillera-Arroita, 2017). For example, a typical 3-min kick-sample recovered only 50% of the macroinvertebrates species, and 60% of the families, found in total from six replicate kick-samples (Furse et al., 1981). Figure 1 illustrates a similar degree of turnover also occurs among replicate samples from the same location for other standardized protocols that study aquatic benthic invertebrates.
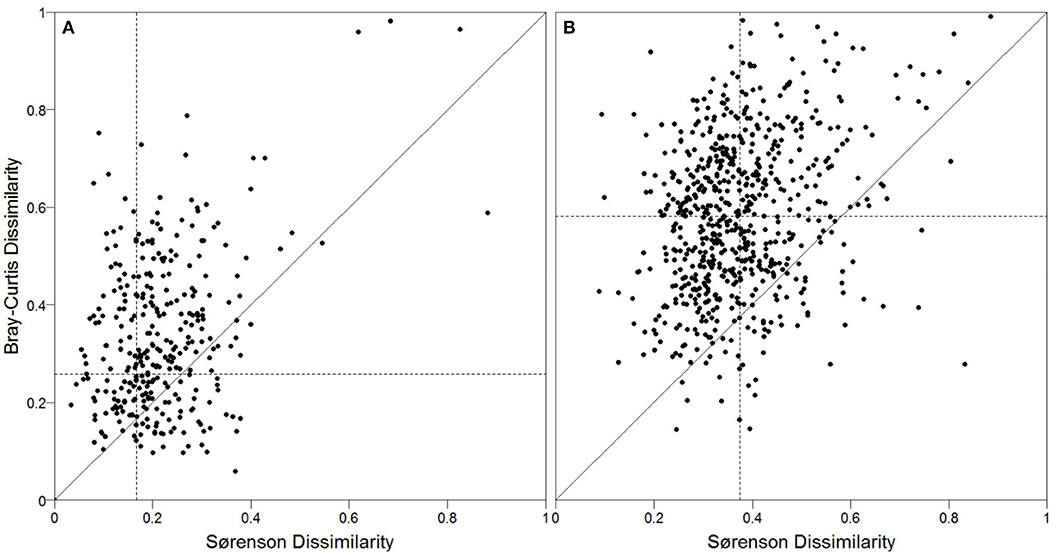
Figure 1. Dissimilarity between replicate samples (same location and time) based on presence/absence data (Sørensen), and count data (Bray-Curtis) of morphologically identified macroinvertebrate families from (A) 417 CABIN (Canadian Aquatic Biomonitoring Network; ECCC, 2018) surveys (total n = 1,656, mean richness = 16 ± 4.8), and (B) 787 surveys from the STAR-AQEM dataset (total n = 1,673) from 14 European countries (mean richness = 51 ± 18.4; Furse et al., 2006; Schmidt-Kloiber et al., 2014).
Metabarcoding can, in principle, substantially reduces detection error by identifying damaged and juvenile specimens, and because aliquots from homogenized bulk community samples are likely to be more representative than morphological subsamples. Nonetheless, successfully detecting all taxa is still conditional on which primers were selected, on the sequencing platform (Singer et al., 2019), the sequencing “effort” (checked by rarefaction of taxon richness and sequencing depth), and, particularly with bulk biological samples, the representativeness of each extraction (checked by analyzing extractions from multiple DNA aliquots). Although low-biomass, low abundance taxa are more likely to be missed (Hajibabaei et al., 2012; Elbrecht et al., 2017a), metabarcoding can detect a higher proportion of the target assemblage compared to morphologically-identified samples (i.e., faster rate of accumulation: Figure 2), thereby increasing the power of monitoring programs to detect change. Figure 2 compares the accumulation curves of macroinvertebrate families collected in the Peace-Athabasca Delta between 2011 and 2016 (updated from surveys published in Gibson et al., 2015). Note that to compare the efficiency of sampling, the metabarcoding data in Figure 2 were aggregated to an equivalent family-level taxonomy of the morphologically-identified samples, but the complete metabarcoding dataset actually observed 109 families, 263 genera, and thousands of unique sequences.
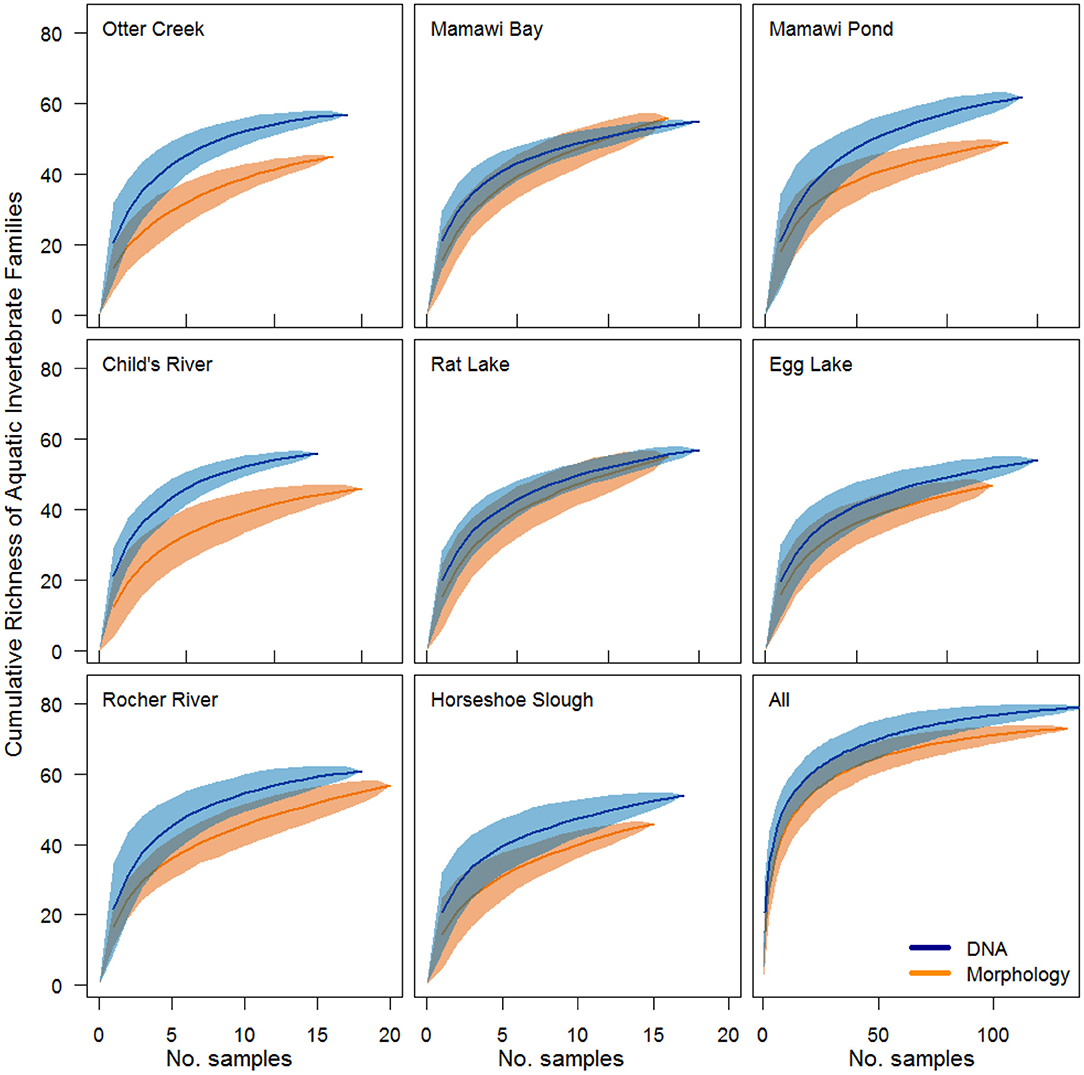
Figure 2. Accumulated richness (mean ± 95% confidence interval) of aquatic invertebrate families from 8 wetland sites in the Peace-Athabasca Delta, and for all samples combined (note different scale) using DNA metabarcoding and morphological identification. Metabarcoded sequences were aggregated and restricted to the same taxa as observed in the morphological dataset for the entire delta.
Misidentification
Morphological identification of diverse taxonomic groups, such as invertebrates, is challenging, as demonstrated by a lack of reliable species-level data generated by routine biomonitoring programs. The probability of misidentifying an individual depends on the quality of the specimen (e.g., is the specimen partial or complete? Is it mature or immature?), the availability and completeness of identification keys, and the taxonomist's experience. Early audits of the RIVPACS program showed that 8.3% of family occurrences were missed, and approximately one false presence was added in every four samples (Clarke, 2009). Similarly, an audit of a range of European programs by Haase et al. (2006) found that after accounting for misidentifications and sorting errors, samples were on average 40% dissimilar to their initial composition (based on lowest taxonomic level). Though procedures for quality control and assessment in biomonitoring programs have reduced the likelihood of misidentification (Haase et al., 2010), false positives and negatives are still common, identification errors compound the loss of taxa during sub-sampling, and misidentifications remain difficult to predict.
A major advantage of metabarcoding over traditional morphological identification is the ability to generate more accurate identifications in a consistent manner (Orlofske and Baird, 2013; Jackson et al., 2014). However, if organisms are misidentified at the time of sequence deposition, reference library sequences become associated with an incorrect taxonomic name. To minimize this challenge, the Barcode of Life Database (BOLD) stores information on voucher specimens, supporting linkage of sequences to material in curated reference specimen collections. Overall, database coverage for animals is expanding rapidly (Porter and Hajibabaei, 2018b) and is already relatively high for freshwater invertebrates (Leese et al., 2018; Weigand et al., 2019). For example, sequences exist for 95% of the genera observed in >1% of samples collected by the Canadian national biomonitoring program (Curry et al., 2018). Currently, the BOLD reference library is better suited to identifying macroinvertebrate families routinely observed in Canada, reflecting the greater effort on DNA barcode library development in that country when compared to Australia and the UK (Figure 3, Supplementary Material S1). At the time of writing, a routine Bayesian classifier (Porter and Hajibabaei, 2018c) is expected to misidentify 4.4, 6.1, and 7.7% of families within CABIN, RIVPACS, and AUSRIVAS programs, respectively. It cannot be overstated that this is a significant improvement on the documented ability of current best-available morphological identification, and is accompanied by an ability to drill down to species-level, which will only improve as DNA libraries become more complete.
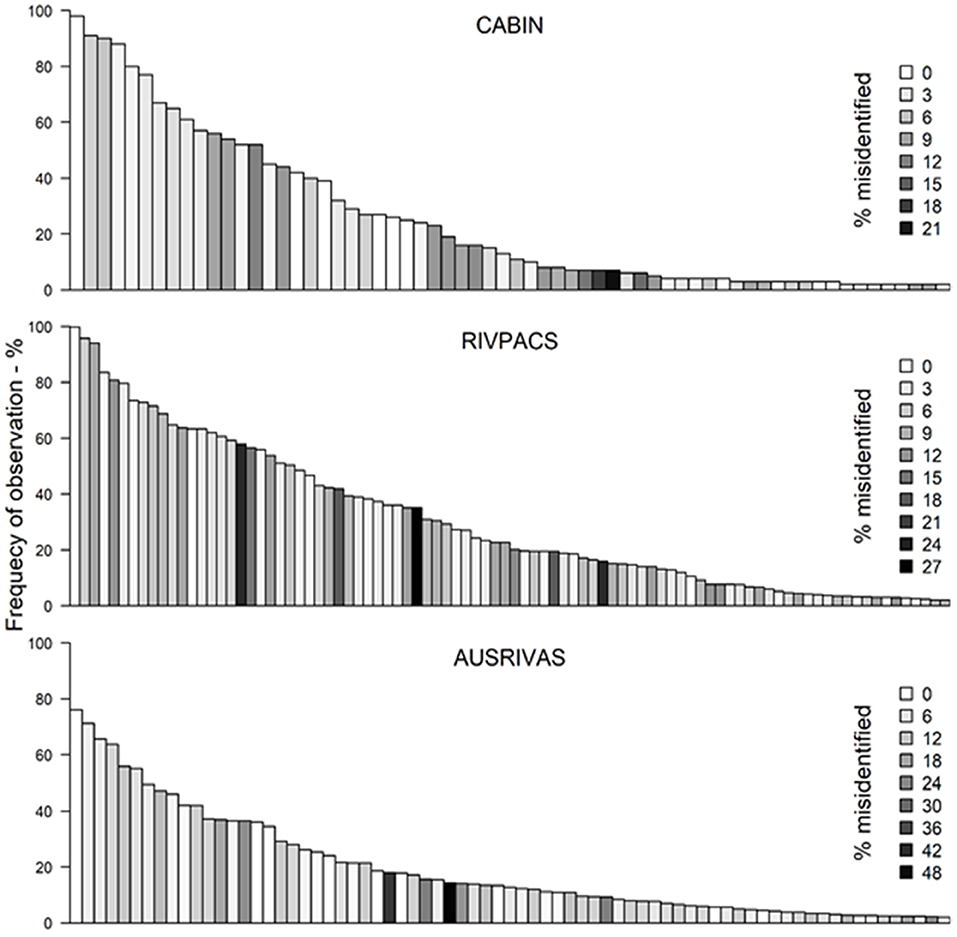
Figure 3. Families ordered by frequency of occurrence within three biomonitoring programs: the CABIN (n = 540), the UK River Invertebrate Prediction and Classification System (RIVPACS, n = 2,504), and the Australian River Assessment System (AUSRIVAS n = 1,516) from Victoria. Shading reflects the likelihood taxa could be misidentified using the CO1 RDP classifier v.3 (see Supplementary Material S1 for further details).
Quantitative Measures of Biodiversity
As stated above, DNA metabarcoding results do not currently produce a reliable signal of abundance or biomass (Elbrecht and Leese, 2015), although at the same time a bias in organism biomass can reduce the detectability of rare taxa (Elbrecht et al., 2017a). Nonetheless, it is equally misleading to suggest that current biomonitoring practices are themselves able to effectively detect differences in macroinvertebrate abundance without substantial effort. The difficulty of processing samples, coupled with species' patchy distributions, means few studies can claim to have truly quantified patterns of abundance for multispecies invertebrate assemblages (e.g., Hawkins et al., 2000).
Reliable estimates of taxon abundance or biomass can support studies of many key ecological processes, and are fundamental to detecting shifts in species dominance that are not associated with changes in composition. This is particularly true in depauperate systems, if species are pooled at higher taxonomic levels, or rare taxa are discarded (Reynoldson et al., 1997). Nonetheless, differences in the composition of diverse assemblages are often sufficient to discriminate among sites, even at relatively coarse taxonomic resolution (Thorne et al., 1999; Hawkins et al., 2000). Thus, the challenge has always been the reliable identification of those taxa. While count or relative abundance information may provide another axis for discrimination, their inherent variability exaggerates the dissimilarity among replicate samples (Figure 1), rendering baseline conditions more variable, thus reducing statistical power to detect change. These limitations are well illustrated by studies that have replaced quantitative count data with qualitative categories or occurrence data (e.g., Wright et al., 1984; Armanini et al., 2013). These approaches have proved acceptable to practitioners precisely because count data provide little or no incremental improvement to detecting differences among sites. Moreover, approaches based on occurrence data illustrate a direct pathway to implement DNA metabarcoding in routine biomonitoring programs (Beentjes et al., 2018).
Performance
Study design and interpretation should acknowledge the sources of uncertainty in both morphological and metabarcoding approaches to deliver specific goals. As they are driven by regulatory needs, most monitoring programs focus on relatively simple outcomes (e.g., local deviation from baseline; categorical quality assessment), and thus can greatly benefit from increased precision and statistical power. Recent freshwater ecosystem studies have demonstrated that metabarcoding data can support detection of ecological change at a greater level of discrimination than traditional approaches (Gibson et al., 2015; Elbrecht et al., 2017b; Emilson et al., 2017). Although regulators have thus far remained hesitant to transition to monitoring with metabarcoding, these early studies have highlighted a lack of precision and consistency in the application of existing morphological approaches, shortcomings that are too often overlooked (but see Giupponi, 2007; Clarke, 2009; Birk et al., 2012; Voulvoulis et al., 2017).
Our intent has been to explore the ability of DNA metabarcoding as an observational tool that provides consistently-observed information to answer routine questions posed by managers (e.g., Is biological composition at a site significantly different from expectations, and if so, is there evidence of impact?). Comparisons between metabarcoding and morphology-based methods have involved sorting and identification of a sample using existing taxonomic keys, followed by the reassembly of the sample for metabarcoding (but see also Hajibabaei et al., 2012; Gibson et al., 2015). These approaches have demonstrated that DNA metabarcoding recovered ~90% of the taxa identified by morphology, and all false-absences were from taxa that represented <1% of individuals. Most recently, we have also evaluated the similarity of taxa recovered by metabarcoding using paired samples (Figure 4; GRDI-Ecobiomics, 2017). DNA was extracted from unsorted bulk samples and, as for Figure 2, the data are aggregated to family-level for comparison with the resolution of routine monitoring in Canada (ECCC, 2018). The average similarity of morphological and metabarcoded samples was 73%, within the range of variation expected for replicate samples (see Figure 1; Clarke et al., 2002). Of the families observed by both methods, DNA observed 79% of the observations made by morphology, whereas morphology only matched 61% of those made by DNA. Some families also appear to be consistently under-represented or absent from this DNA dataset (Figures 4A,B, bottom-left), most likely due to a combination of gaps in the reference library (aquatic mites and oligochaetes in particular) and primer bias (Gibson et al., 2014; Elbrecht et al., 2017b). Beyond mere overlap, a better estimate of performance could be the likelihood each family was missed based on their detectability in replicate samples (Figure 4B). Both methods are likely to have missed many families at least once, but the mean and likelihood of multiple false absences was lower among metabarcoding samples than for samples identified by morphology (Supplementary Material S2). Metabarcoding therefore represents a major advance in how consistently we observe the taxonomic structure of aquatic invertebrate communities.
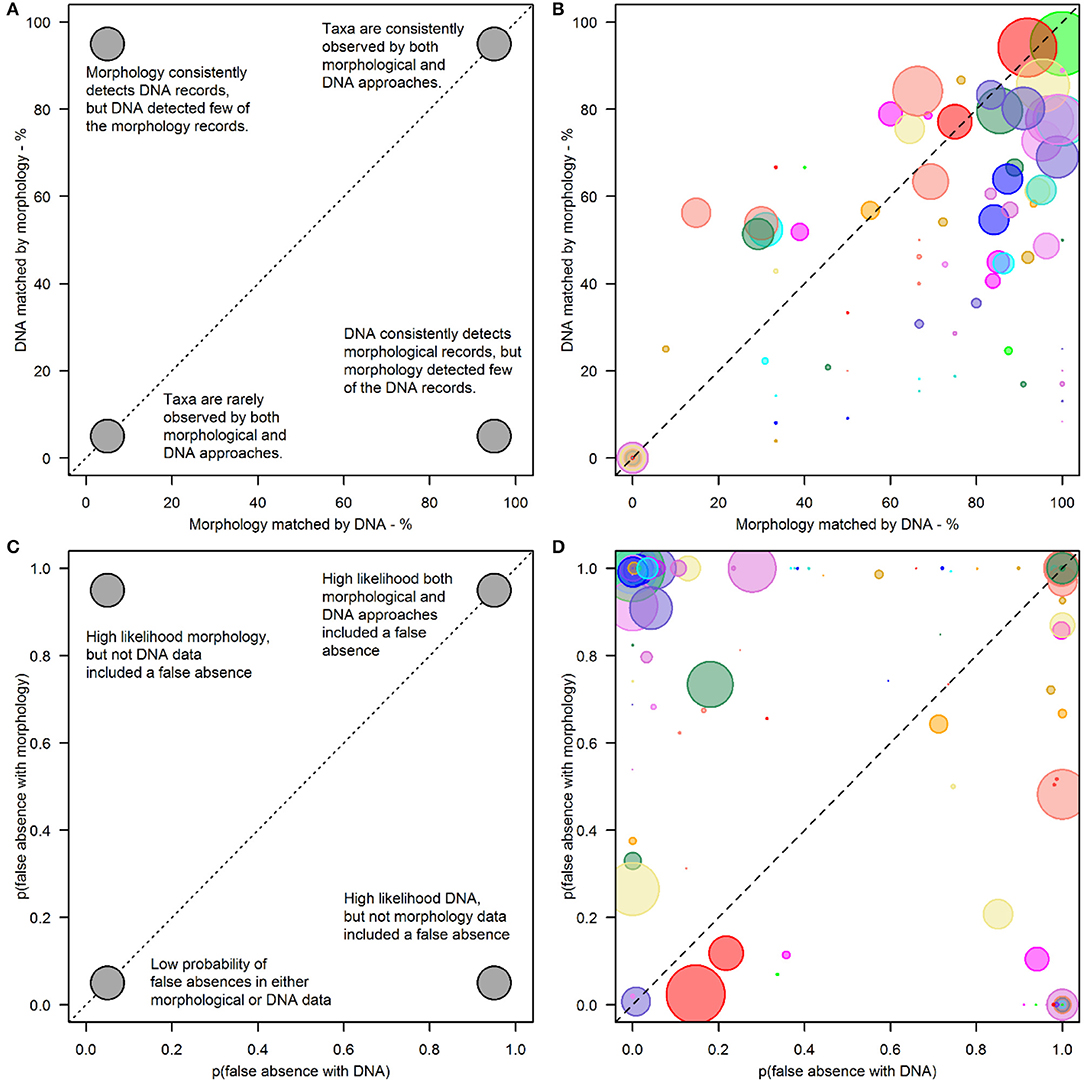
Figure 4. Comparison of macroinvertebrate families (n = 114) observed in pairs of standard 3-min river benthos kick samples (n = 141 sites). (A,B) Shows the correspondence between observations of each taxonomic family using either morphological identification or DNA metabarcoding. Points are scaled relative to the number of morphological observations. (C,D) Shows the probability that each method included at least one false absence for each taxon (see Supplementary Material S2 for code and raw data).
Conclusions
Biomonitoring 2.0 (Baird and Hajibabaei, 2012) envisaged the use of DNA metabarcoding to generate consistently-observed biodiversity data to detect environmental change efficiently and rapidly. This can be done with only minor modification of existing sample collection methods, ensuring backwards compatibility with legacy data. Finer taxonomic resolution, more efficient detection (Figure 2), and the capacity to increase spatiotemporal coverage can all increase the statistical power to detect change and diagnose its cause (Bonada et al., 2006). Finer taxonomic resolution and more samples with metabarcoding would improve the estimation of detection errors(e.g., Davis et al., 2018), and once standard operating procedures emerge, many tasks can be automated, further reducing the risk of handling errors and the costs of sequencing (Porter and Hajibabaei, 2018a). Currently, the cost of processing an invertebrate community sample (from DNA-extraction to sequencing) is approximately half the cost of morphological identification by taxonomists, but as we have stressed, the divergent properties of each approach make it misleading to base comparisons on costs alone.
We can only manage what we can measure, and at present the unknown magnitude and consequences of global biodiversity loss emphasizes the value of metabarcoding as a technique to support improved ecological observation in all field studies of multispecies assemblages. We expect the increasing numbers of metabarcoding studies, and sequences in reference libraries, will help refine the uncertainties associated with observations, and accelerate the large-scale implementation of metabarcoding (e.g., Leese et al., 2018). Metabarcoding is also being used for increasingly novel applications, such as the study of trophic interactions, either through direct analyses of gut contents, or via the reconstruction of networks of multi-trophic assemblages (Bohan et al., 2017). Other fields of research such as meta-community theory (Miller et al., 2018), and ecosystem function relationships (Vamosi et al., 2017) also benefit where previously the statistical relationships were obscured by coarse taxonomic resolution. These applications could generate substantial added value to existing or future biomonitoring programs (Compson et al., 2018).
In conclusion, ecologists in all ecosystems should be aware of the shortcomings in their data, and acknowledge it publicly if the uncertainty could alter their conclusions. Metabarcoding is now an established technique, with the capacity to improve the quality and utility of ecological data, and understanding its statistical properties will help ecologists to design, test and review evidence for new hypotheses.
Author Contributions
AB wrote the first draft and all coauthors contributed to the final edit. Data was collected by AB, ZC, WM, DB, and EE and analyzed by RS, TP, MR, NG, and MH.
Funding
This work was supported by the Ontario Genomics Institute and Genome Canada, NSERC, Environment and Climate Change Canada program funds, and the Canadian federal Genomics Research & Development Initiative.
Conflict of Interest
The authors declare that the research was conducted in the absence of any commercial or financial relationships that could be construed as a potential conflict of interest.
Acknowledgments
We thank Guy Woodward, Richard Marchant, Astrid Schmidt-Kloiber, and Daniel Hering for providing data from monitoring programs in the UK, Australia, and EU.
Supplementary Material
The Supplementary Material for this article can be found online at: https://www.frontiersin.org/articles/10.3389/fevo.2019.00434/full#supplementary-material
References
Armanini, D. G., Monk, W. A., Carter, L., Cote, D., and Baird, D. J. (2013). Towards generalised reference condition models for environmental assessment: a case study on rivers in Atlantic Canada. Environ. Monit. Assess. 185, 6247–6259. doi: 10.1007/s10661-012-3021-2
Arscott, D. B., Jackson, J. K., and Kratzer, E. B. (2006). Role of rarity and taxonomic resolution in a regional and spatial analysis of stream macroinvertebrates. J. North Am. Benthol. Soc. 25, 977–997. doi: 10.1899/0887-3593(2006)025[0977:RORATR]2.0.CO;2
Bailet, B., Bouchez, A., Franc, A., Frigerio, J.-M., Keck, F., Karjalainen, S.-M., et al. (2019). Molecular versus morphological data for benthic diatoms biomonitoring in Northern Europe freshwater and consequences for ecological status. Metabarcod. Metagenom. 3:e34002. doi: 10.3897/mbmg.3.34002
Baird, D., and Hajibabaei, M. (2012). Biomonitoring 2.0: a new paradigm in ecosystem assessment made possible by next-generation DNA sequencing. Mol. Ecol. 21, 2039–2044. doi: 10.1111/j.1365-294X.2012.05519.x
Beentjes, K. K., Speksnijder, C. L., Schilthuizen, M., Schaub, B. E. M., and van der Hoorn, B. B. (2018). The influence of macroinvertebrate abundance on the assessment of freshwater quality in The Netherlands. Metabarcod. Metagenom. 2:e26744. doi: 10.3897/mbmg.2.26744
Beermann, A. J., Zizka, V. M. A., Elbrecht, V., Baranov, V., and Leese, F. (2018). DNA metabarcoding reveals the complex and hidden responses of chironomids to multiple stressors. Environ. Sci. Eur. 30:26. doi: 10.1186/s12302-018-0157-x
Birk, S., Bonne, W., Borja, A., Brucet, S., Courrat, A., Poikane, S., et al. (2012). Three hundred ways to assess Europe's surface waters: an almost complete overview of biological methods to implement the Water Framework Directive. Ecol. Indic. 18, 31–41. doi: 10.1016/j.ecolind.2011.10.009
Bista, I., Carvalho, G. R., Walsh, K., Seymour, M., Hajibabaei, M., Lallias, D., et al. (2017). Annual time-series analysis of aqueous eDNA reveals ecologically relevant dynamics of lake ecosystem biodiversity. Nat. Commun. 8:14087. doi: 10.1038/ncomms14087
Bohan, D. A., Vacher, C., Tamaddoni-Nezhad, A., Raybould, A., Dumbrell, A. J., and Woodward, G. (2017). Next-generation global biomonitoring: large-scale, automated reconstruction of ecological networks. Trends Ecol. Evol. 32, 477–487. doi: 10.1016/j.tree.2017.03.001
Bonada, N., Prat, N., Resh, V. H., and Statzner, B. (2006). Developments in aquatic insect biomonitoring: a comparative analysis of recent approaches. Annu. Rev. Entomol. 51, 495–523. doi: 10.1146/annurev.ento.51.110104.151124
Brehm, G., Hebert, P. D. N., Colwell, R. K., Adams, M.-O., Bodner, F., Friedemann, K., et al. (2016). Turning up the heat on a hotspot: DNA barcodes reveal 80% more species of geometrid moths along an andean elevational gradient. PLoS ONE 11:e0150327. doi: 10.1371/journal.pone.0150327
Bush, A., Sollmann, R., Wilting, A., Bohmann, K., Cole, B., Balzter, H., et al. (2017). Connecting earth observation to high-throughput biodiversity data. Nat. Ecol. Evol. 1:0176. doi: 10.1038/s41559-017-0176
Buss, D. F., Carlisle, D. M., Chon, T.-S., Culp, J., Harding, J. S., Keizer-Vlek, H. E., et al. (2014). Stream biomonitoring using macroinvertebrates around the globe: a comparison of large-scale programs. Environ. Monit. Assess. 187:4132. doi: 10.1007/s10661-014-4132-8
Cardinale, B. J., Duffy, J. E., Gonzalez, A., and Hooper, D. U. (2012). Biodiversity loss and its impact on humanity. Nature 486, 59–67. doi: 10.1038/nature11148
Carstensen, J., and Lindegarth, M. (2016). Confidence in ecological indicators: a framework for quantifying uncertainty components from monitoring data. Ecol. Indic. 67, 306–317. doi: 10.1016/j.ecolind.2016.03.002
Clarke, R. (2009). Uncertainty in WFD Assessments for Rivers Based on Macroinvertebrates and RIVPACS. Integrated Catchment Science Programme Science Report: SC060044/SR4, Bristol, UK, 1–87.
Clarke, R. T., Furse, M. T., Gunn, R. J. M., Winder, J. M., and Wright, J. F. (2002). Sampling variation in macroinvertebrate data and implications for river quality indices. Freshw. Biol. 47, 1735–1751. doi: 10.1046/j.1365-2427.2002.00885.x
Clarke, R. T., Lorenz, A., Sandin, L., Schmidt-Kloiber, A., Strackbein, J., Kneebone, N. T., et al. (2006). Effects of sampling and sub-sampling variation using the STAR-AQEM sampling protocol on the precision of macroinvertebrate metrics. Hydrobiologia 566, 441–459. doi: 10.1007/s10750-006-0078-3
Compson, Z. G., Monk, W. A., Curry, C. J., Gravel, D., Bush, A. C., et al. (2018). Linking DNA metabarcoding and text mining to create network-based biomonitoring tools: a case study on boreal wetland macroinvertebrate communities. Adv. Ecol. Res. 59, 33–74. doi: 10.1016/bs.aecr.2018.09.001
Cordier, T., Forster, D., Dufresne, Y. C., Martins, I. M., Stoeck, T., et al. (2018). Supervised machine learning outperforms taxonomy-based environmental DNA metabarcoding applied to biomonitoring. Mol. Ecol. Resour. 18, 1381–1391. doi: 10.1111/1755-0998.12926
Cristescu, M. E., and Hebert, P. D. N. (2018). Uses and misuses of environmental DNA in biodiversity science and conservation. Annu. Rev. Ecol. Evol. Syst. 49, 209–230. doi: 10.1146/annurev-ecolsys-110617-062306
Curry, C. J., Gibson, J. F., Shokralla, S., Hajibabaei, M., and Baird, D. J. (2018). Identifying North American freshwater invertebrates using DNA barcodes: are existing COI sequence libraries fit for purpose? Freshw. Sci. 37, 178–189. doi: 10.1086/696613
Davis, A. J., Williams, K. E., Snow, N. P., Pepin, K. M., and Piaggio, A. J. (2018). Accounting for observation processes across multiple levels of uncertainty improves inference of species distributions and guides adaptive sampling of environmental DNA. Ecol. Evol. 8, 10879–10892. doi: 10.1002/ece3.4552
Deiner, K., Bik, H. M., Mächler, E., Seymour, M., Lacoursière-Roussel, A., Altermatt, F., et al. (2017). Environmental DNA metabarcoding: transforming how we survey animal and plant communities. Mol. Ecol. 26, 5872–5895. doi: 10.1111/mec.14350
Doberstein, C. P., Karr, J. R., and Conquest, L. L. (2000). The effect of fixed-count subsampling on macroinvertebrate biomonitoring in small streams. Freshw. Biol. 44, 355–371. doi: 10.1046/j.1365-2427.2000.00575.x
Downes, B. J., Lake, P. S., and Schreiber, E. S. G. (1993). Spatial variation in the distribution of stream invertebrates: implications of patchiness for models of community organization. Freshw. Biol. 30, 119–132. doi: 10.1111/j.1365-2427.1993.tb00793.x
ECCC (2018). CABIN Canadian Aquatic Biomonitoring Network, Environment and Climate Change Canada. Available online at: https://open.canada.ca/data/en/dataset/13564ca4-e330-40a5-9521-bfb1be767147
Effenberger, M., Sailer, G., Townsend, C. R., and Matthaei, C. D. (2006). Local disturbance history and habitat parameters influence the microdistribution of stream invertebrates. Freshw. Biol. 51, 312–332. doi: 10.1111/j.1365-2427.2005.01502.x
Elbrecht, V., and Leese, F. (2015). Can DNA-based ecosystem assessments quantify species abundance? Testing primer bias and biomass—sequence relationships with an innovative metabarcoding protocol. PLoS ONE 10:e0130324. doi: 10.1371/journal.pone.0130324
Elbrecht, V., and Leese, F. (2017). Validation and development of COI metabarcoding primers for freshwater macroinvertebrate bioassessment. Front. Environ. Sci. 5:38. doi: 10.3389/fenvs.2017.00038
Elbrecht, V., Peinert, B., and Leese, F. (2017a). Sorting things out: assessing effects of unequal specimen biomass on DNA metabarcoding. Ecol. Evol. 7, 6918–6926. doi: 10.1002/ece3.3192
Elbrecht, V., Vamos, E. E., Meissner, K., Aroviita, J., and Leese, F. (2017b). Assessing strengths and weaknesses of DNA metabarcoding-based macroinvertebrate identification for routine stream monitoring. Methods Ecol. Evol. 8, 1265–1275. doi: 10.1111/2041-210X.12789
Emilson, C. E., Thompson, D. G., Venier, L. A., Porter, T. M., Swystun, T., Chartrand, D., et al. (2017). DNA metabarcoding and morphological macroinvertebrate metrics reveal the same changes in boreal watersheds across an environmental gradient. Sci. Rep. 7:12777. doi: 10.1038/s41598-017-13157-x
Ficetola, G. F., Pansu, J., Bonin, A., Coissac, E., Giguet-Covex, C., De Barba, M., et al. (2015). Replication levels, false presences and the estimation of the presence/absence from eDNA metabarcoding data. Mol. Ecol. Resour. 15, 543–556. doi: 10.1111/1755-0998.12338
Fonseca, D. M., and Hart, D. D. (2001). Colonization history masks habitat preferences in local distributions of stream insects. Ecology 82, 2897–2910. doi: 10.1890/0012-9658(2001)082[2897:CHMHPI]2.0.CO;2
Friberg, N., Bonada, N., Bradley, D. C., Dunbar, M. J., Edwards, F. K., Grey, J., et al. (2011). “Biomonitoring of human impacts in freshwater ecosystems: the good, the bad and the ugly,” in Advances in Ecological Research, Vol. 44, ed G. Woodward (Amsterdam), 1–68.
Furse, M., Hering, D., Moog, O., Verdonschot, P., Johnson, R. K., Brabec, K., et al. (2006). “The STAR project: context, objectives and approaches,” in The Ecological Status of European Rivers: Evaluation and Intercalibration of Assessment Methods, eds M. T. Furse, D. Hering, K. Brabec, A. Buffagni, L. Sandin, and P. F. M. Verdonschot (Dordrecht: Springer Netherlands, 3–29.
Furse, M. T., Wright, J. F., Armitage, P. D., and Moss, D. (1981). An appraisal of pond-net samples for biological monitoring of lotic macro-invertebrates. Water Res. 15, 679–689. doi: 10.1016/0043-1354(81)90160-3
Gardner, T. A., Barlow, J., Araujo, I. S., Ávila-Pires, T. C., Bonaldo, A. B., Costa, J. E., et al. (2008). The cost-effectiveness of biodiversity surveys in tropical forests. Ecol. Lett. 11, 139–150. doi: 10.1111/j.1461-0248.2007.01133.x
Gibson, J., Shokralla, S., Porter, T. M., King, I., van Konynenburg, S., Janzen, D. H., et al. (2014). Simultaneous assessment of the macrobiome and microbiome in a bulk sample of tropical arthropods through DNA metasystematics. Proc. Natl. Acad. Sci. U.S.A. 111, 8007–8012. doi: 10.1073/pnas.1406468111
Gibson, J. F., Shokralla, S., Curry, C., Baird, D. J., Monk, W. A., King, I., et al. (2015). Large-scale biomonitoring of remote and threatened ecosystems via high-throughput sequencing. PLoS ONE 10:e0138432. doi: 10.1371/journal.pone.0138432
Giupponi, C. (2007). Decision support systems for implementing the european water framework directive: the MULINO approach. Environ. Model. Softw. 22, 248–258. doi: 10.1016/j.envsoft.2005.07.024
GRDI-Ecobiomics (2017). Ecobiomics: Metagenomics Based Ecosystem Biomonitoring Project, Government of Canada, Genomics R&D Initiative, Year-End Performance Report for Shared Priority Projects (2017–2018). GRDI-Ecobiomics.
Guillera-Arroita, G. (2017). Modelling of species distributions, range dynamics and communities under imperfect detection: advances, challenges and opportunities. Ecography 40, 281–295. doi: 10.1111/ecog.02445
Haase, P., Murray-Bligh, J., Lohse, S., Pauls, S., Sundermann, A., Gunn, R., et al. (2006). Assessing the impact of errors in sorting and identifying macroinvertebrate samples. Hydrobiologia 566, 505–521. doi: 10.1007/s10750-006-0075-6
Haase, P., Pauls, S. U., Schindehütte, K., and Sundermann, A. (2010). First audit of macroinvertebrate samples from an EU Water Framework Directive monitoring program: human error greatly lowers precision of assessment results. 29, 1279–1291, 13. doi: 10.1899/09-183.1
Hajibabaei, M., Shokralla, S., Zhou, X., Singer, G. A. C., and Baird, D. J. (2011). Environmental barcoding: a next-generation sequencing approach for biomonitoring applications using river benthos. PLoS ONE 6:e17497. doi: 10.1371/journal.pone.0017497
Hajibabaei, M., Spall, J. L., Shokralla, S., and van Konynenburg, S. (2012). Assessing biodiversity of a freshwater benthic macroinvertebrate community through non-destructive environmental barcoding of DNA from preservative ethanol. BMC Ecol. 12:28. doi: 10.1186/1472-6785-12-28
Hawkins, C. P., Norris, R. H., Hogue, J. N., and Feminella, J. W. (2000). Development and evaluation of predictive models for measuring the biological integrity of streams. Ecol. Appl. 10, 1456–1477. doi: 10.1890/1051-0761(2000)010[1456:DAEOPM]2.0.CO;2
Hering, D., Borja, A., Jones, J. I., Pont, D., Boets, P., Bouchez, A., et al. (2018). Implementation options for DNA-based identification into ecological status assessment under the European Water Framework Directive. Water Res. 138, 192–205. doi: 10.1016/j.watres.2018.03.003
Jackson, J. K., Battle, J. M., White, B. P., Pilgrim, E. M., Stein, E. D., Miller, P. E., et al. (2014). Cryptic biodiversity in streams: a comparison of macroinvertebrate communities based on morphological and DNA barcode identifications. Freshw. Sci. 33, 312–324. doi: 10.1086/675225
Ji, Y., Ashton, L., Pedley, S. M., Edwards, D. P., Tang, Y., Nakamura, A., et al. (2013). Reliable, verifiable and efficient monitoring of biodiversity via metabarcoding. Ecol. Lett. 16, 1245–1257. doi: 10.1111/ele.12162
Jones, F. C. (2008). Taxonomic sufficiency: The influence of taxonomic resolution on freshwater bioassessments using benthic macroinvertebrates. Environ. Rev. 16, 45–69. doi: 10.1139/A07-010
Keck, F., Vasselon, V., Tapolczai, K., Rimet, F., and Bouchez, A. (2017). Freshwater biomonitoring in the information age. Front. Ecol. Environ. 15, 266–274. doi: 10.1002/fee.1490
Lancaster, J., and Downes, B. J. (2014). Population densities and density–area relationships in a community with advective dispersal and variable mosaics of resource patches. Oecologia 176, 985–996. doi: 10.1007/s00442-014-3062-z
Lavoie, I., Dillon, P. J., and Campeau, S. (2009). The effect of excluding diatom taxa and reducing taxonomic resolution on multivariate analyses and stream bioassessment. Ecol. Indic. 9, 213–225. doi: 10.1016/j.ecolind.2008.04.003
Leese, F., Bouchez, A., Abarenkov, K., Altermatt, F., Borja, Á., Bruce, K., et al. (2018). “Chapter 2: Why we need sustainable networks bridging countries, disciplines, cultures and generations for aquatic biomonitoring 2.0: a perspective derived from the DNAqua-net COST action,” in Advances in Ecological Research, eds D. A. Bohan, A. J. Dumbrell, G. Woodward, and M. Jackson (Amsterdam: Academic Press, 63–99. doi: 10.1016/bs.aecr.2018.01.001
Lindenmayer, D. B., and Likens, G. E. (2011). Direct measurement versus surrogate indicator species for evaluating environmental change and biodiversity loss. Ecosystems 14, 47–59. doi: 10.1007/s10021-010-9394-6
Macher, J. N., Salis, R. K., Blakemore, K. S., Tollrian, R., Matthaei, C. D., and Leese, F. (2016). Multiple-stressor effects on stream invertebrates: DNA barcoding reveals contrasting responses of cryptic mayfly species. Ecol. Indic. 61, 159–169. doi: 10.1016/j.ecolind.2015.08.024
Majaneva, M., Diserud, O. H. S., Eagle, H. C., Hajibabaei, M., and Ekrem, T. (2018). Choice of DNA extraction method affects DNA metabarcoding of unsorted invertebrate bulk samples. Metabarcod. Metagenom. 2:e26664. doi: 10.3897/mbmg.2.26664
Martin, G. K., Adamowicz, S. J., and Cottenie, K. (2016). Taxonomic resolution based on DNA barcoding affects environmental signal in metacommunity structure. Freshw. Sci. 35, 701–711. doi: 10.1086/686260
Miller, E. T., Svanbäck, R., and Bohannan, B. J. M. (2018). Microbiomes as metacommunities: understanding host-associated microbes through metacommunity ecology. Trends Ecol. Evol. 33, 926–935. doi: 10.1016/j.tree.2018.09.002
Musco, L., Terlizzi, A., Licciano, M., and Giangrande, A. (2009). Taxonomic structure and the effectiveness of surrogates in environmental monitoring: a lesson from polychaetes. Mar. Ecol. Prog. Ser. 383, 199–210. doi: 10.3354/meps07989
Nakov, T., Beaulieu, J. M., and Alverson, A. J. (2018). Insights into global planktonic diatom diversity: the importance of comparisons between phylogenetically equivalent units that account for time. ISME J. 12, 2807–2810. doi: 10.1038/s41396-018-0221-y
Newbold, T., Hudson, L. N., Hill, S. L., Contu, S., Lysenko, I., and Senior, R. A. (2015). Global effects of land use on local terrestrial biodiversity. Nature 520, 45–50. doi: 10.1038/nature14324
Nijboer, R. C., and Schmidt-Kloiber, A. (2004). The effect of excluding taxa with low abundances or taxa with small distribution ranges on ecological assessment. Hydrobiologia 516, 347–363. doi: 10.1023/B:HYDR.0000025275.49062.55
Orlofske, J. M., and Baird, D. J. (2013). The tiny mayfly in the room: implications of size-dependent invertebrate taxonomic identification for biomonitoring data properties. Aquat. Ecol. 47, 481–494. doi: 10.1007/s10452-013-9460-1
Pawlowski, J., Kelly-Quinn, M., Altermatt, F., Apothéloz-Perret-Gentil, L., Beja, P., Boggero, A., et al. (2018). The future of biotic indices in the ecogenomic era: integrating (e)DNA metabarcoding in biological assessment of aquatic ecosystems. Sci. Total Environ. 637–638, 1295–1310. doi: 10.1016/j.scitotenv.2018.05.002
Petkovska, V., and Urbanič, G. (2010). Effect of fixed-fraction subsampling on macroinvertebrate bioassessment of rivers. Environ. Monitor. Assess. 169, 179–201. doi: 10.1007/s10661-009-1161-9
Petsch, D. K. (2016). Causes and consequences of biotic homogenization in freshwater ecosystems. Int. Rev. Hydrobiol. 101, 113–122. doi: 10.1002/iroh.201601850
Porter, T. M., and Hajibabaei, M. (2018a). Scaling up: a guide to high-throughput genomic approaches for biodiversity analysis. Mol. Ecol. 27, 313–338. doi: 10.1111/mec.14478
Porter, T. M., and Hajibabaei, M. (2018b). Over 2.5 million COI sequences in GenBank and growing. PLoS ONE 13:e0200177. doi: 10.1371/journal.pone.0200177
Porter, T. M., and Hajibabaei, M. (2018c). Automated high throughput animal CO1 metabarcode classification. Sci. Rep. 8:4226. doi: 10.1038/s41598-018-22505-4
Reynoldson, T. B., Norris, R. H., Resh, V. H., Day, K. E., and Rosenberg, D. M. (1997). The reference condition: a comparison of multimetric and multivariate approaches to assess water-quality impairment using benthic macroinvertebrates. J. North Am. Benthol. Soc. 16, 833–852. doi: 10.2307/1468175
Rimet, F., Abarca, N., Bouchez, A., Kusber, W.-H., Jahn, R., Kahlert, M., et al. (2018). The potential of High-Throughput Sequencing (HTS) of natural samples as a source of primary taxonomic information for reference libraries of diatom barcodes. Fottea 18, 37–54. doi: 10.5507/fot.2017.013
Schmidt-Kloiber, A., and Nijboer, R. C. (2004). The effect of taxonomic resolution on the assessment of ecological water quality classes. Hydrobiologia 516, 269–283. doi: 10.1023/B:HYDR.0000025270.10807.10
Schmidt-Kloiber, A., Strackbein, J., Vogl, R., Furse, M. T., and Hering, D. (2014). Description of the AQEM/STAR invertebrate database. Freshw. Metadata J. 2, 1–8. doi: 10.15504/fmj.2014.2
Singer, G. A. C., Fahner, N. A., Barnes, J. G., McCarthy, A., and Hajibabaei, M. (2019). Comprehensive biodiversity analysis via ultra-deep patterned flow cell technology: a case study of eDNA metabarcoding seawater. Sci. Rep. 9:5991. doi: 10.1038/s41598-019-42455-9
Soininen, J., Jamoneau, A., Rosebery, J., and Passy, S. I. (2016). Global patterns and drivers of species and trait composition in diatoms. Glob. Ecol. Biogeogr. 25, 940–950. doi: 10.1111/geb.12452
Stokstad, E. (2018). Researchers launch plan to sequence 66,000 species in the United Kingdom. Science 366. doi: 10.1126/science.aav9295.
Strachan, S. A., and Reynoldson, T. B. (2014). Performance of the standard CABIN method: comparison of BEAST models and error rates to detect simulated degradation from multiple data sets. Freshw. Sci. 33, 1225–1237. doi: 10.1086/678948
Sweeney, B. W., Battle, J. M., Jackson, J. K., and Dapkey, T. (2011). Can DNA barcodes of stream macroinvertebrates improve descriptions of community structure and water quality? J. North Am. Benthol. Soc. 30, 195–216. doi: 10.1899/10-016.1
Taberlet, P., Coissac, E., Hajibabaei, M., and Rieseberg, L. H. (2012). Environmental DNA. Mol. Ecol. 21, 1789–1793. doi: 10.1111/j.1365-294X.2012.05542.x
Thorne, R. S. J., Williams, W. P., and Cao, Y. (1999). The influence of data transformations on biological monitoring studies using macroinvertebrates. Water Res. 33, 343–350. doi: 10.1016/S0043-1354(98)00247-4
Turak, E., Harrison, I., Dudgeon, D., Abell, R., Bush, A., Darwall, W., et al. (2017). Essential biodiversity variables for measuring change in global freshwater biodiversity. Biol. Conserv. 213, 272–279. doi: 10.1016/j.biocon.2016.09.005
Vamosi, J. C., Gong, Y.-B., Adamowicz, S. J., and Packer, L. (2017). Forecasting pollination declines through DNA barcoding: the potential contributions of macroecological and macroevolutionary scales of inquiry. N. Phytol. 214, 11–18. doi: 10.1111/nph.14356
Vasselon, V., Rimet, F., Tapolczai, K., and Bouchez, A. (2017). Assessing ecological status with diatoms DNA metabarcoding: scaling-up on a WFD monitoring network (Mayotte island, France). Ecol. Indic. 82, 1–12. doi: 10.1016/j.ecolind.2017.06.024
Vaughn, C. C., and Spooner, D. E. (2006). Unionid mussels influence macroinvertebrate assemblage structure in streams. J. North Am. Benthol. Soc. 25, 691–700. doi: 10.1899/0887-3593(2006)25[691:UMIMAS]2.0.CO;2
Vellend, M., Baeten, L., Myers-Smith, I. H., Elmendorf, S. C., Beauséjour, R., Brown, C. D., et al. (2013). Global meta-analysis reveals no net change in local-scale plant biodiversity over time. Proc. Natl. Acad. Sci. U.S.A. 110, 19456–19459. doi: 10.1073/pnas.1312779110
Vivien, R., Wyler, S., Lafont, M., and Pawlowski, J. (2015). Molecular barcoding of aquatic oligochaetes: implications for biomonitoring. PLoS ONE 10:e0125485. doi: 10.1371/journal.pone.0125485
Vlek, H. E., Šporka, F., and Krno, I. J. (2006). Influence of macroinvertebrate sample size on bioassessment of streams. Hydrobiologia 566, 523–542. doi: 10.1007/s10750-006-0074-7
Voulvoulis, N., Arpon, K. D., and Giakoumis, T. (2017). The EU water framework directive: from great expectations to problems with implementation. Sci. Total Environ. 575, 358–366. doi: 10.1016/j.scitotenv.2016.09.228
Weigand, H., Beermann, A. J., Ciampor, F., Costa, F. O., Csabai, Z., Duarte, S., et al. (2019). DNA barcode reference libraries for the monitoring of aquatic biota in Europe: gap-analysis and recommendations for future work. Sci. Total Environ. 678, 499–524. doi: 10.1016/j.scitotenv.2019.04.247
Woodward, G., Gray, C., and Baird, D. J. (2013). Biomonitoring for the 21 st Century: new perspectives in an age of globalisation and emerging environmental threats. Limnetica 29, 159–174. doi: 10.23818/limn.32.14
Wright, J. F., Moss, D., Armitage, P. D., and Furse, M. T. (1984). A preliminary classification of running-water sites in Great Britain based on macro-invertebrate species and the prediction of community type using environmental data. Freshw. Biol. 14, 221–256. doi: 10.1111/j.1365-2427.1984.tb00039.x
Yu, D. W., Ji, Y., Emerson, B. C., Wang, X., Ye, C., Yang, C., et al. (2012). Biodiversity soup: metabarcoding of arthropods for rapid biodiversity assessment and biomonitoring. Methods Ecol. Evol. 3, 613–623. doi: 10.1111/j.2041-210X.2012.00198.x
Keywords: biodiversity observation, high-throughput sequencing, taxonomic resolution, community ecology, environmental genomics, freshwater, benthic macroinvertebrate
Citation: Bush A, Compson ZG, Monk WA, Porter TM, Steeves R, Emilson E, Gagne N, Hajibabaei M, Roy M and Baird DJ (2019) Studying Ecosystems With DNA Metabarcoding: Lessons From Biomonitoring of Aquatic Macroinvertebrates. Front. Ecol. Evol. 7:434. doi: 10.3389/fevo.2019.00434
Received: 29 July 2019; Accepted: 23 October 2019;
Published: 08 November 2019.
Edited by:
Alireza Tamaddoni-Nezhad, Imperial College London, United KingdomReviewed by:
Christian Henri Nozais, Université du Québec à Rimouski, CanadaRosetta Charlotte Blackman, Swiss Federal Institute of Aquatic Science and Technology, Switzerland
Copyright © 2019 Bush, Compson, Monk, Porter, Steeves, Emilson, Gagne, Hajibabaei, Roy and Baird. This is an open-access article distributed under the terms of the Creative Commons Attribution License (CC BY). The use, distribution or reproduction in other forums is permitted, provided the original author(s) and the copyright owner(s) are credited and that the original publication in this journal is cited, in accordance with accepted academic practice. No use, distribution or reproduction is permitted which does not comply with these terms.
*Correspondence: Alex Bush, alexalbush@gmail.com