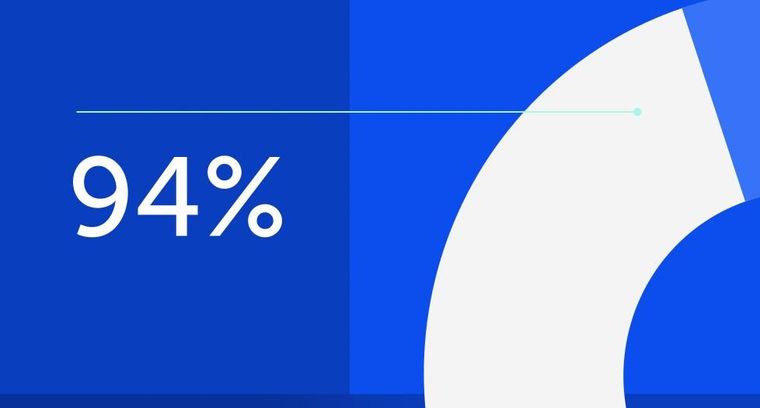
94% of researchers rate our articles as excellent or good
Learn more about the work of our research integrity team to safeguard the quality of each article we publish.
Find out more
REVIEW article
Front. Ecol. Evol., 08 November 2019
Sec. Biogeography and Macroecology
Volume 7 - 2019 | https://doi.org/10.3389/fevo.2019.00424
This article is part of the Research TopicUnifying Ecology Across Scales: Progress, Challenges and OpportunitiesView all 19 articles
Humans have observed the natural world and how people interact with it for millennia. Over the past century, synthesis and expansion of that understanding has occurred under the banner of the “new” discipline of ecology. The mechanisms considered operate in and between many different scales—from the individual and short time frames, up through populations, communities, land/seascapes and ecosystems. Whereas, some of these scales have been more readily studied than others—particularly the population to regional landscape scales—over the course of the past 20 years new unifying insights have been possible via the application of ideas from new perspectives, such as the fields of complexity and network theory. At any sufficiently large gathering (and with sufficient lubrication) discussions over whether ecologists will ever uncover unifying laws and what they may look like still persist. Any pessimism expressed tends to grow from acknowledgment that gaping holes still exist in our understanding of the natural world and its functioning, especially at the smallest and grandest scales. Conceptualization of some fundamental ideas, such as evolution, are also undergoing review as global change presents levels of directional pressure on ecosystems not previously seen in recorded history. New sensor and monitoring technologies are opening up new data streams at volumes that can seem overwhelming but also provide an opportunity for a profusion of new discoveries by marrying data across scales in volumes hitherto infeasible. As with so many aspects of science and life, now is an exciting time to be an ecologist.
Models have many roles in ecology—from explanatory (conceptual) exploration of theoretical hypotheses, to anticipatory predictions to guide short-term tactical decision making, or longer-term projections to inform strategic direction setting (FAO, 2008; Mouquet et al., 2015). While predictive capacity is important when models are being used to guide explicit decision making, models are useful conversation starters to generate interest and discussion around a topic. Indeed, it is the authors' experience that more breakthrough learnings about system function have eventuated when a model has been wrong than when it has been right (as all involved are keen to know why it was wrong, fewer people ask when a model matches observations or expectations). All of these roles continue a long tradition of synthesizing knowledge in generalisable and useful forms.
Humanity has been codifying its theories about the function of the world since the earliest story tellers and religious practices, although the discipline of ecology was not formally recognized until the late nineteenth century (Egerton, 1977) and is often still seen as a relatively “young” science. Throughout that long history and up to the present day ecologists have drawn upon observations to try to summarize the world around us and the complex interrelations contained therein (Haeckel in Egerton, 1977), to elucidate the underlying mechanisms that shape the patterns we see (Levin, 1992). In some instances, they have combined forces with mathematicians to provide a more theoretical standpoint or to create synthesis via model construction, which explicitly involves abstracting away unnecessary detail and retaining only the essentials required to produce observed patterns (Costanza and Sklar, 1985; Levin, 1992; Håkanson, 1997; Fulton et al., 2003a; Plagányi et al., 2014).
As mechanisms often occur on different scales to the patterns they shape, this makes scale the key conceptual problem in ecology (Levin, 1992). Ecological studies began at small scales (Melbourne-Thomas et al., 2017), but more recently, is grappling with more data streams, from more disciplines, new technologies and across broader scales, particularly as global change has become such a dominant consideration (Chave, 2013). As understanding has accumulated there have been numerous attempts to unify the threads into a common framework (e.g., Scheiner and Willig, 2005, 2008). These exercises have had mixed success and significant gaps remain—particularly at the largest and smallest scales. This “modelers' perspective” provides a summary motivated by the authors' experience with those gaps, assessing which have been filled and which loom largest on the horizon.
Our starting point was to reflect on our experience, its dead ends as much as its successes, the persistent trials along with more recently emerging challenges. Our observations are summarized in Table 1, which captures our collective view of the strengths, gaps, and drivers of change across the broad research domains of empirical ecological studies, theoretical and mathematical ecology, and system-level hybrid models. In pondering how scale plays into these issues we developed a set of “Stommel diagrams” (Stommel, 1963) to frame our thinking. This series of schematic diagrams (Figure 1) reflects the scales and dimensions that characterize key system components and processes (terrestrial, marine, and anthropogenic), as well as the reach of observational methods and models in representing those features. The shading on the diagrams highlights the extent of understanding drawn from historical ecological and modeling studies, with the arrows in Figures 1C,F showing the likely directions of expansion for ecological knowledge and model representations of ecological processes (some of which has already begun).
Table 1. Summary of the strengths, gaps and drivers for change in empirical and theoretical ecology and modeling as perceived by the authors.
Figure 1. Set of schematic Stommel diagrams showing: (A) key ecological processes (with the solid arrow indicating the new understanding about the scales of action of evolution and the dashed line showing the cross scale connection ecology is realizing stems from linking processes at different scales such as parasitism, ecosystem structure and function and large scale nutrient cycles); (B) marine phenomena (components and processes); (C) terrestrial phenomena (including the scale of natural disasters such as fire, flood, earthquakes, and volcanoes); (D) human dimensions (including the scale of human settlements and decision making processes and influences); (E) observational scales from illustrative major sensor platform types (noting that citizen science is significantly extending data collection beyond the scales of the platforms shown); and (F) the scales most reliably captured by models (the solid dots indicate scales well-captured by traditional approaches, the shaded area the growing extent of models, the small and large arrows the push for continuing development and the dashed line the push for coupling across scales; there may be additional model types that already sit outside the shaded domain, but it is largely indicative of the scope of scales covered). Together these diagrams create a conceptual figure highlighting the scales and disciplinary dimensions that characterize reality. The base figure for the key ecological process and marine components is redrawn and updated from Vance and Doel (2010). For the other diagrams the scales of the key phenomena and system features also drawn from Clark (1985b), Marquet et al. (1993), Peterson et al. (1998), Westley et al. (2002), Scholes et al. (2013), Kavanaugh et al. (2016), and Rose et al. (2017). Note for these other diagrams (C–F), we have chosen to draw them on a flat two dimensional space as the original Stommel diagram's third dimension may not be as relevant for these other dimensions (but there was insufficient information to reliably try to replicate this third axis for the other properties).
Modern ecological modeling (represented by the shaded area in Figure 1F) has been underpinned by both empirical studies and mathematical explorations (Table 1). The early efforts of observational, theoretical and mathematical ecology were relatively simple and often confined to “local” (small) scales (the phenomena on scales of <1–100 km and from a few months to a few years in extent shown in Figure 1). However, the strong relationship between time and space scales (the diagonal patterns seen across Figures 1A–C,F) also allowed for some researchers (e.g., Clements, 1916) to conceptualize processes on large (landscape) scales over very large time periods (the top right corner of Figure 1B). Since then there has been a coevolution of empirical and conceptual/theoretical ecology. Accumulating data streams, technological improvements (e.g., increased computing power) and a growing understanding of the interconnected and nested nature of ecological systems has seen all ecological fields, but perhaps particularly modeling, which simultaneously come under pressure to become increasingly complex while appreciating the extensive list of things they still do not know (continuously pushing out the shaded areas of understanding in Figure 1). Our experience of this pressure over the past 2 decades motivates this perspective piece, which briefly explains the historical trajectory of (theoretical and mathematical) ecology, then summarizes more recent realizations for the field (including insights from models and implications for their ongoing development) and finally concludes with our thoughts on what the future holds and what may be fruitful pathways of enquiry. While we have tried to be even handed in our discussion, much of our experience stems from marine ecosystems and we acknowledge that bias. We hope that any of our unintentional omissions are not so grievous as to detract from our intent to start a conversation about how the scientific community can go about broadening the cloth of modeling and ecology.
The broad concepts that underpin ecology have been accepted for decades—e.g., species have heterogeneous distributions contingent on interactions with their experienced abiotic and biotic environments; resources and life spans are finite and realized ecological properties are shaped by a mix of behavior and evolution (Scheiner and Willig, 2008). Nevertheless, finding a “simple” set of rules that can be used predictively to describe those concepts has proved more challenging. There have been many contenders—either for a “grand unifying” concept or at least theories that synthesize significant chunks of ecological thought, including: island biogeography theory (MacArthur and Wilson, 1967), succession theory (Pickett et al., 1987), metapopulation theory (Hanski, 1999), food web theory (Pimm, 1982), unified neutral theory of biodiversity and biogeography (Hubbell, 2001), metabolic theory of ecology (Brown et al., 2004), biodiversity via emergent neutrality (Scheffer and van Nes, 2006), theories of macroecosystem ecology (Rose et al., 2017), and the many other theories listed in publications such as Marquet et al. (2015). Indeed, so many unified theories have been proposed that there are now pushes to unify the unified theories (McGill, 2010). Of these many ecological concepts, cross-scale macroecological theories (McGill and Collins, 2003) are one of the few that explicitly address multi-scale processes—from local interactions to large scale dynamics.
Drawing on our modeling experience, our sympathies lie with those who see strength in diversity and see “a monolithic unified theory of ecology is neither feasible nor desirable” (Loreau, 2010a,b); preferring instead to deploy the wide range of competing ideas in addressing applied ecological problems such as resource management, conservation, or restoration (Palmer et al., 2008). Much as with the debates over “top-down vs. bottom-up” control of food webs (Lynam et al., 2017), “density dependent vs. density independent” control factors (Turchin, 1995), or the relative importance of birth-death vs. movement related processes (Kondoh et al., 2016), it has been our experience that reproducing real world dynamics often involves a mix of most of the concepts, with what is/isn't important changing with the system, the dominant conditions and most importantly the question being asked. Reflecting on experiences from studying multiple ecosystems has seen us realize that there are some common features (a common “skeleton” if you will), but that, unlike physics and chemistry, each ecological system will have its particular idiosyncrasies (the “flesh” of the detail). General principles and patterns do of course exist and more remain to be found, but while we wait for those theoretical principles to be tested, as scientists servicing applied management needs, we have chosen to pragmatically combine concepts and tools as needed—i.e., the hybrid approach that is beginning to gain traction in the modeling world. This approach, highlighted in Table 1 as a strong direction of future model development, appears to be the best means (in terms of practicality of implementation and level of model skill) of achieving the linking across scales shown by the dashed arrow in Figure 1F. It has certainly been the only means we have found of capturing or anticipating the emergent properties that have posed the greatest challenges to coastal resource managers. For instance, to correctly capture the responses of reef-associated fish communities to interacting environmental and fishing pressures along the northwest coast of Australia, we needed to tie mean field representations of advectable larval patches to age structured metapopulatons of settled juveniles to individual-based representations of the adult stages of key large fish species (Gray et al., 2006). Similarly, in producing models of developed coastal margins (for the purposes of envisaging potential alternative future development and management pathways) it has been necessary to combine a multitude of modeling methods to successfully represent the many ecosystem components and human uses present in these systems. For example, in a model of Patagonia developed to assist with planning for sustainable aquaculture (Fulton et al., 2018), it was necessary to couple time series (for environment drivers and economic demand) with qualitative networks (of social and ethnic aspects), statistical models (to represent the epidemiology of farmed fish), process-based analytical models (e.g., age structured metapopulation models for wild fish stocks), agent-based representations (of large marine mammals), cellular automata (for land uses such as agriculture, forestry and urban settlements) and stocks and flow representations of industrial production (e.g., mining, energy production, and manufacturing).
As the scope of the models have grown from populations to communities to ecosystems and finally socioecological systems (the central arc of points in Figure 1F) it has been necessary and important to draw upon an expanding range of disciplines—not just for directly relevant information on the processes to be considered (expanding from ecology to biology, chemistry, geology through to human oriented sciences like economics, sociology, psychology, etc.), but also for the new perspectives they bring. Without this expansion of perspective, we have found it impossible to successfully reproduce the history of exploited ecosystems (e.g., in south eastern Australia; Fulton et al., 2005, Fulton and Gorton, 2014).
The value of considering the world from new points of view is something ecology has struggled to achieve (with some notable exceptions, such as the interchange of ideas around game theory with economics; e.g., Riechert and Hammerstein, 1983; Brown, 2016). Ecological observation, particularly before the invention of modern remote or autonomous monitoring systems was logistically difficult. Technological advances have also not negated that observations can also be costly or protracted. These realities led to an accidental bias in the formative ecological studies, with the majority of the most influential tending to come from more accessible locations—i.e., terrestrial forests and grasslands (Elton, 1924), freshwater lakes (Tilman, 1977; Carpenter et al., 1987), or the marine intertidal (Paine, 1969); and were often situated in the northern hemisphere. While the importance of scale in ecology was appreciated on similar time scales in the marine (Smith, 1978; Steele, 1978) and terrestrial realms (Allen and Starr, 1982), the sharing and transference of ecological concepts between landscapes and seascapes has often lagged. Metapopulation theory, for example, began its development on land more than 30 years before it was considered in marine systems (Sale et al., 2016). There has also been a substantial separation in the focus of work done by practically focused ecologists working on resource management (e.g., for forestry, fisheries or pest control), who typically began from a population stand point (e.g., Russell, 1931; Hjort et al., 1933), and academic ecology which had a much earlier focus on complexity and community dynamics (e.g., Clements, 1916). Access bias also means that critical ecological processes that structure the harder to access marine systems may be under-appreciated (or unrealized).The hemispherical bias has meant that our understanding of southern hemisphere ecosystems (e.g., in the Southern Ocean) has faced challenges because aspects of the ecosystem functioning are substantially different (the northern hemisphere is dominated by continental land masses, the southern by ocean, this influences large scale current patterns, climate, temperature patterns, life histories, ecology (Chown et al., 2004), as well as levels of human occupation, exploitation and pollution (FAO, 2005; World Health Organization air pollution database available from https://www.who.int).
There are a few common ecological principles such as source-sink dynamics (Pulliam, 1988), that hold equally well in marine and terrestrial realms; whereas other processes are more obviously prominent in one context than another (e.g., habitat selection is clearly important in terrestrial forests or coral reefs, but its role in pelagic systems, while important, is not as well-appreciated). Given the different properties of the essentially two-dimensional nature of many terrestrial ecosystems vs. the three-dimensional reality of open ocean ecosystems, it is likely that theories developed in one perspective may not easily equate in the other (Steele, 1985). As highlighted by Webb (2012) some comparisons are straightforward—the same taxa in different environments, or the same process (e.g., predation) in different environments, consideration of community structure in similar environments (soils and marine sediments)—while others are less obvious [e.g., when taxa as different as whales and trees have a similar biogeochemical role, such as carbon storage and nutrient cycling (Ratnarajah et al., 2016)]. This need for creative equivalence has delayed appreciation of how some ecological properties do port between systems—as demonstrated by the importance of size as a structuring mechanism on land and sea (Yvon-Durocher et al., 2011) and the related fact that the biomass equivalence rule popularized by Sheldon et al. (1972) in the pelagic marine realm holds equally well in the 3D interstitial realm inhabited by soil communities, something registered by Ghilarov (1944) but not effectively recognized, even by size spectra specialists, until recently (Polishchuk and Blanchard, 2019).
Models have played a beneficial role in taking ideas between the different realms; they have also built off ecological observations and theory to provide feedback that helps advance all the disciplines. Early mathematical analyses of ecological systems established many of the basic concepts that underlie ecology (Pacala, 1994)—such as competition (Ekschmitt and Breckling, 1994)—even when empirical evidence has been hard to source (e.g., Allee effects; Courchamp et al., 2008). Anderson and May (1979), for instance, not only used models to describe phenomena such as disease outbreaks but to emphasize how these analyses could function as a test bed for theories of spatial ecology (Ferguson et al., 1997). Marine (typically fisheries inspired) modeling has played a significant role in advancing population (e.g., as summarized in Allen, 1975) and ecosystem modeling (see review in Fulton, 2010) as well as bio-economic modeling (e.g., Clark, 1985a). Marine work has also inspired inclusion of socio-cultural aspects in models (van Putten et al., 2013) and contributed to some of the earliest work on epidemiological modeling (e.g., Anderson and May, 1978).
Initial simplistic concepts such as the Lotka-Volterra representation of interactions (Volterra, 1926; Lotka, 1932) have been refined—for example via the foraging arena concept (Ahrens et al., 2012) or the explicit relation of physiological/metabolic processes to body size. Allometric approaches, now widely used to explain ecological processes on land and sea, grew from early attempts to find simple relationships using mean body size (Yodzis and Innes, 1992). Attempts to push ecological models to new scales and embed them in larger models representing entire socioecological systems (linking with social, economic and climate components) has highlighted entirely new gaps in our understanding of system connections and feedbacks (Levin et al., 2013). Examples include how human exploitation can influence evolutionary processes on ecologically relevant timeframes (Audzijonyte et al., 2013) and how changing social-ecological conditions need to be accounted for to understand and manage regional resilience of terrestrial protected areas (Cumming et al., 2015). This has helped drive the desire to share ideas from other research fields, to help close some of these gaps—for example bringing together ecologists, economists and others to capture iterative interactions between human actions and natural system responses in fisheries (e.g., Fulton et al., 2014; Weber et al., 2019), catchments (e.g., Voinov et al., 1999) and agricultural landscapes (e.g., Münier et al., 2004; Crepin and Lindahl, 2009).
Marine modeling has maintained a relatively steady focus on population modeling (as discussed in 2015 special issue of ICES Journal of Marine Science), biogeochemical modeling (e.g., Franks, 2002), multispecies or ecosystem modeling (e.g., Plagányi et al., 2014), as well as habitat, species, and community distributions (e.g., Cheung et al., 2009; Dunstan and Foster, 2011; Pitcher et al., 2018). Terrestrial modelers have similar interests and some shared methods, but have tended to have a different methodological focus to their efforts. For instance, statistical methods appear to have played a much larger role in some areas of terrestrial ecology, perhaps due to access to larger data sets than are often available in the ocean. Structural equation modeling, a multivariate technique useful for evaluating multivariate causal relationships, has been used to explore ecosystem responses (e.g., to climate change or human disturbance) and processes (Fan et al., 2016). Bayesian hierarchical models have also been used to great effect, not only to look at species distributions (e.g., Diez and Pulliam, 2007; Stewart-Koster et al., 2013), but also to look at ecological impacts and responses to human activities (such as to altered environmental flows; de Little et al., 2013) and to allow for the integration of experimental ecology and mechanistic (or process-based) modeling (e.g., Ogle, 2009). Bayesian Belief Networks (BBNs) have been used in both marine and terrestrial circumstances, but have perhaps had more attention in terrestrial systems. These models graphically represent probabilistic influence networks and correlative and causal relationships among variables and have been used to look at the implications of alternative management approaches (McCann et al., 2006). Some of the most substantial efforts in terrestrial ecological modeling have gone into the modeling of terrestrial biosphere (Fisher et al., 2014), including biogeochemical cycles, soil and vegetation (e.g., Rastetter et al., 1991), or landcover and associated properties—such as state and transition models of rangeland management (Bestelmeyer et al., 2017) and semi-mechanistic models of community-level biodiversity and its responses to climate shifts (Mokany and Ferrier, 2011). More recently there have been a growing number of efforts to breach the divide and share lessons between the terrestrial and marine domains (Milner-Gulland et al., 2010).
Looking across both land and sea over the last 20 years, as the scope and the number of scales included in ecological studies and models expanded, two particularly useful additions to the ecological lexicon were complexity theory and network theory. Complexity theory is an interdisciplinary approach that integrates concepts from a broad range of disciplines (including chaos theory, computer science, mathematics, fuzzy logic, statistical physics information theory, non-linear dynamics, evolutionary biology, cognitive psychology, behavioral economics, anthropology, and general systems theory) which attempts to explain the behavior and evolution of common properties of complex systems such as embedding, the importance of diversity and interconnections, contextual dependence, emergent properties, and distributed control. Complexity theory brings together methods to tackle the behavior of systems (e.g., ecosystems displaying non-linear and dynamically adaptive responses) that had eluded more traditional, often equilibrium, approaches (Hastings et al., 2018). We have found this to be a particularly useful framework for dealing with the hierarchy of interactions within and across the 14 orders of magnitude in spatial scales (and the similarly large number of temporal scales) that contribute to ecosystems—from the bacterial to basin and global scales (Figure 1). Metapopulation concepts took the first step, recognizing within and between patch processes (Levin, 1992), but complexity theory went further and ecosystems are now clearly seen to be complex adaptive systems characterized by: feedbacks between processes occurring at different scales, amplification of responses to minor (noisy) variations, and emergent patterns (Levin, 1998; Anand et al., 2010). A powerful example of this is ecosystem patterns that are driven by ecological interactions that are themselves mediated by metabolically determined chemical signaling (Chave, 2013). The value of complexity theory as a means of solving issues of mismatches in scales of modeling biophysical systems, anthropogenic drivers, and socio-economic dynamics has been given considerable attention in the terrestrial modeling literature. For example, Parker et al. (2008) summarized the complexity of modeling land-use systems due to direct and indirect interactions and the mismatch of scales of human actions and their impacts, specifically dealing with: harmonizing models and data sets with vastly different resolutions in space and time; using expert knowledge to constrain modeled transitions; and carefully considering the level of coupling required of the biophysical and socioeconomic components—whether it is “one-way” or “fully coupled” and whether it considers only direct or also indirect links.
Network theory (whereby key system components and their interactions are represented by a set of “nodes” connected by “links” of varying strength) has also been particularly useful for understanding ecology from local to regional scales—allowing for characterization of trophic and habitat interactions and key player identification (Jordán et al., 2006; Bascompte, 2009; Thébault and Fontaine, 2010), as well as to predict management outcomes for interacting threats (Marzloff et al., 2016b; Baker et al., 2018; Tulloch et al., 2018), or distributed patches, such as networks of aquaculture leases (Mardones et al., 2011). Network theory has also supplied science with a powerful means of integrating information sources (e.g., by allowing for explicit connection of observations from different disciplines and with traditional knowledge) to provide new insights into system functioning (e.g., Dambacher et al., 2003). Furthermore, network theory has provided the capacity to deal with multilayer networks (spanning multiple spatiotemporal scales and other forms of ecological complexity such as ontogenetic shifts). This, in turn, has enabled analysis of interactions between the processes that operate within and between scales and the evolution of networks through space and time (Pilosof et al., 2017). Network analysis is also allowing for the identification of universal properties that govern multi-scale system behavior. Gao et al. (2016) demonstrate that the resilience of multi-dimensional complex systems are strongly influenced by the topological properties of density, heterogeneity and symmetry. This capacity to highlight common properties of multi-scale behaviors, integrating ecological and management aspects and their associated feedbacks, means that network theory is helping to fill the gaps in the diagonal spine of the diagrams in Figure 1. We have found network-based representations of systems (using loop analysis) such a useful means of characterizing conceptual understanding of ecosystem form and function that it is now routinely used as the first step in our modeling work.
Whereas, finding universal (cross-scale) properties of structure and function is a comforting anchor for those trying to understand, conserve or manage the world around us, the reality is that contingent dynamics of complex systems is the recurrent theme and challenge of the new generation of ecological problems. The first step in tackling this situation has been to accept the system specificity (contextual dependence) of the outcome of some processes (e.g., evolution and food web structure; Eklöf and Stouffer, 2016) and the resulting implications for human use and management—such as the performance of ecological indicators (e.g., Dale and Beyeler, 2001; Shin et al., 2018) or natural vs. artificial marine substrata (Ferrario et al., 2016). This has also led to the realization that whereas there are core ecological properties that are universal (and codified in general modeling frameworks), system idiosyncrasies will often demand bespoke model modifications if the applied models are to faithfully capture the observed dynamics of the system in question (Fulton et al., 2004). Care must be taken to restrict this to necessary processes and guard against unnecessary over-fitting, but experience has shown that the extent to which this is an issue depends on the use of the model (whether it is for strategic or tactical questions) and the interpretation of outputs.
Another step in improving the veracity of ecological models in our rapidly changing world is to understand the limitation of the observational record. One of the most commonly discussed reasons for the patchy reliability of projections based on statistical relationships is that empirical correlations often fail when moving into conditions outside the observed range (Levin, 1992; Mokany et al., 2016). Even when using increasingly sophisticated statistical approaches to ease computation demands there is still the need to build from assumptions (or preferably robust mechanistic understanding) of the phenomena being considered (Mouquet et al., 2015). Taking a theory-based approach can extend the envelope of reliability, while simultaneously assisting in the accumulation of knowledge and the reduction of uncertainty (Thuiller et al., 2013). In principle, ecology could draw on historical analogs to inform ideas and models of future change. Unfortunately, such efforts are sparse, particularly in the marine realm, as they often draw on paleontological time frames rather than simply observations from recorded history, as the degree of future environmental change may shift ecosystems into states that are governed by previously unobserved variables and interactions. Where available, fossil records have already given insights into the changing role of functional diversity, species composition and network structure with shifting pressures on ancient ecosystems and how a weakening of functional diversity exacerbated responses to later pressures (e.g., Yasuhara et al., 2008; Mitchell et al., 2012; Dunne et al., 2014; Roopnarine and Angielczyk, 2015; Pimiento et al., 2017; Lowery et al., 2018). Work on paleontological patterns of extinction underline that areas with high intrinsic risk of extinction (due the geographic range and taxonomic identity of species found there) coincide today with areas of rapid climate shifts and elevated human impacts (Finnegan et al., 2015; Pimiento et al., 2017). Paleoecological proxies—such as the examination of pollen patterns or tree ring records (Birks and Birks, 2003), or diatoms in seabed cores (Mackay et al., 2003)—can allow for reconstruction of the dynamics of ecosystems beyond the immediate observational record on to scales of 1000s of years (in particular over the Holocene), meaning they include a greater overlap with the species alive today. Models can then be tested against these proxies to see the veracity of the model's capacity to effectively capture dynamics of these species and ecosystems beyond the modern period. Iglesias et al. (2015), for example, describe how linking sedimentary charcoal data and ecological models has been used to reconstruct past fire regimes and the implications of climate-vegetation-fire linkages and drivers at different spatial and temporal scales. While such proxy-based data sets are typically sparser than modern observations, they are a lot less sparse than the fossil record.
The field of ecology will be faced with a fast-paced race to update models and understanding in an attempt to keep pace with rapid observational corrections to prior predictions. In attempting to anticipate this modeling arms race, modelers have begun to look at the inclusion of fine scale processes that influence species' responses and adaptation. Unfortunately, this is where ecological modelers encounter a number of significant challenges. It is not a simple process to incorporate sub-grid scale processes, fine-scale behaviors and phenology into ecological models; particularly those models attempting to capture the dynamics of entire ecosystems (Griffith and Fulton, 2014). It is computationally infeasible to explicitly represent the small spatial and short time scales involved (Hastings et al., 2018). Advances can be made by clever use of statistical characterizations or abstractions which influence the mechanistic model dynamics and modify outcomes on the explicitly modeled scales (Ellis and Pantus, 2001; Moorcroft et al., 2001) or to use agent-based approaches which see the “emergence” of the desired phenomena (DeAngelis and Mooij, 2005). However, even then ecology can fall short in terms of providing suitable mechanistic understanding. Environmental data is currently too coarsely resolved to support fine-grained processes (Mouquet et al., 2015). Moreover, not all ecological processes have received equal attention—mutualism, amensalism, and facilitation have received a tiny fraction of the attention garnered by predator-prey interactions (Martorell and Freckleton, 2014); and mixotrophy, which has an important part to play in marine planktonic systems, has rarely been included in models to date (Berge et al., 2017).
Both macroecological relationships (Brown et al., 2004; Cabral et al., 2017) and trait based approaches (McGill et al., 2006) appear to have strong potential as means of connecting the arrows along the spines of Figures 1C,F—simultaneously synthesizing cross scale processes, helping bridge the divide between models of ecosystem composition and function, and delivering larger scale patterns without requiring crippling complexity, or unreasonable computational and data loads (Blanchard et al., 2017). Even then, caution is required due to the scale-dependent role of traits (Suárez-Castro et al., 2018), the significant variation that exists around macroecological relationships (Gaston and Blackburn, 1999) and knowledge that the relationships can change dynamically (Supp et al., 2012). These very factors may help explain phenotypic responses and range edge effects (Chuang and Peterson, 2016), as well as species-specific responses to processes such as ocean acidification (Vargas et al., 2017) and localized variation in adaptation and adaptive capacity (Bennett et al., 2015). Nonetheless, they are also likely to have important implications for spatiotemporal patterns in the diversity and functioning of ecosystems and our capacity to model those patterns (Mokany et al., 2016).
All of these issues act to highlight additional ecological gaps. There is still limited understanding of how ecosystem structure changes through time and space—and how the processes concur or differ between land and sea (e.g., many of the well-studied features at smaller spatial scales appear to span longer time periods on land than at sea—compare Figure 1A and Figure 1B). Nevertheless, recent advances in process-based macroecology (Cabral et al., 2017; Connolly et al., 2017) and trait-based approaches (Kiørboe et al., 2018) are making advances in terms of dynamic prediction of macroecological patterns, including across scales. Isotopic methods are helping bridge the trophic gaps at larger spatial and temporal scales (Hobson et al., 2010; Quillfeldt et al., 2010) and new metagenomic methods are being used to generate new ecosystem scale maps of active processes and biodiversity (Raes et al., 2018). As we outline above, nested network approaches are also helping to link understanding of interactions and connectivity across processes, space, and time (Pilosof et al., 2017). As an example, to support management of the deleterious impacts of crown of thorns starfish on Australia's Great Barrier Reef coral communities, ecological models are being integrated across a range of scales accounting for fine-scale Allee effects (Rogers et al., 2017) through to embedding an ecological model in a meta-community reef network model incorporating large scale processes such as cyclone and terrestrial runoff from agriculture practices (Condie et al., 2018).
Unfortunately, it remains the case that there is little empirical understanding of the true natural structure and interactions in many systems (Griffith and Fulton, 2014). Science often likes to begin from a “no effects” case and then build incrementally from there, but in ecology that is proving exceptionally difficult to do given humanity's pervasive footprint (Halpern et al., 2015). Palaeoecological studies (that aim to reconstruct ancient webs prior to human influence) are making advances (Mitchell et al., 2012; Yeakel et al., 2013), but few systems have survived a human signature (Yeakel et al., 2014). This means we are starting from partial information when trying to decipher what are healthy system structures, how humans may impact those and what to expect as we either degrade or endeavor to recover ecosystems.
The challenges above are compounded further when we move from strict ecological to socio-ecological responses. Many key processes in socioecological systems, such as those driving institutional change, have gone unrecorded and the footprint of human activities and settlements push into the combinations of time and space scales with low predictability (see the area marking out the scale of settlements in Figure 1D). This creates new challenges for ecology and modeling. For instance, the question of how to manage the novel ecosystems arising as a result of climate associated range shifts now bedevils resource managers, especially where valuable stocks move from one country's jurisdiction to another or where new interaction effects emerge (Pinksy et al., 2018). Recovering marine mammal stocks are causing significant issues in a number of marine systems. This is via predation pressure that may be beyond what the current human perturbed ecosystem states can support (Estes et al., 2009; Chasco et al., 2017), or are at least beyond what human users are used to or (in some cases) willing to accept, leading to tensions with local fisheries and other users (as reported in the popular media of Australia, Canada, USA, and Chile). Moreover, contextual dependency of complex systems also means we cannot simply rewind the clock—reintroductions fail (e.g., Barkai and McQuaid, 1988) or strong hysteresis create blockages (Marzloff et al., 2016a). Key to addressing these challenges are quantitative modeling methods that can predict the consequences for the recipient ecosystem. Fortunately, significant advances have already been made on these issues in terrestrial systems (e.g., Baker et al., 2017).
Work with more complex and dynamic systems has also allowed ecologists to appreciate that some of the ideas fundamental to ecology, such as evolution, need expansion or revision to correctly connect their expression at the scales now being observed (see the arrow pushing to smaller scales in Figure 1C). For example, 20–30 years ago it was standard practice to teach evolution as a process that was irrelevant on less than effectively geological scales (disease resistance dynamics being one of the few exceptions). Ecological modelers were comfortable ignoring evolutionary drivers (i.e., those developing models for the bottom left of the diagrams in Figure 1 typically did not bother with processes in the upper right). Suggestions that processes on ecological scales could drive observable change were strongly contested (e.g., the debate around fisheries induced evolution; Audzijonyte et al., 2013; Eikeset et al., 2016). Now, as human activity provides strong directional pressure at multiple scales, variants of Gould and Eldredge's (1977) idea of punctuated equilibrium seem to be a dynamically experienced event and there is frequent discussion of phenotypic variation, eco-evolutionary processes (Pelletier et al., 2009; Chave, 2013; Gillman and Wright, 2014; Laland et al., 2015; Watson et al., 2016; Weiss-Lehman et al., 2016), and epigenetic effects (Danchin et al., 2011; Bonduriansky, 2012; Ryu et al., 2018). Hence ecologists are realizing that their models are missing key processes. Fulton and Gorton (2014) found that to reliably reproduce recent observed ecosystem shifts in their models of south east Australia, evolution and acclimation processes (along with modification of coastlines by human activities) were needed. When those models were projected forward they led to vastly different projections of future system state than models that used only fixed parameters. In trying to validate the rate of expression of these additional approaches it was soon clear that this is one of many cases illustrating that our understanding of how the natural world operates is not as complete as we had thought. We may have had a credible grasp of the functioning of systems within certain limits and configurations, but the Anthropocene is moving our world beyond those limits, which is highlighting new or underappreciated processes and species roles (Hobbs et al., 2009). This new reality drives home that ecology lacks a universally solid foundation from which to extend existing theories and modeling approaches to easily absorb the many interacting components and scales. Moreover, for modelers it is highlighting how many of the abstractions that underpin the representation of the more complex ecological interactions and processes are unvalidated, impossible to measure directly and based on equilibrium concepts that are not compatible with the dynamically transient nature of changing ecosystems influenced by both human use and environmental shifts.
A good example of where this realization hits home is in our capacity to reliably model the changing distribution of species. There is no denying that the science has come a long way and can capture large scale observable patterns on land and in the oceans (Follows et al., 2009; Olsen et al., 2016). This has been made possible in part because modern tracking technology means we can now track animal movements to a degree unheard of only 5 years ago (Kays et al., 2015; Klein et al., 2019; Lowerre-Barbieri et al., 2019). However, research is still struggling to link physiological tolerances to multivariate habitat dependencies (Bozinovic and Naya, 2015), life-history and ecological traits (dispersal capability; Bates et al., 2014) and species co-occurrence (Cazelles et al., 2016) in order to reliably predict range shifts, habitat contraction or expansion and the role of adaptation in these processes. For example, while zooplankton assemblages in the North Atlantic have conserved their thermal niches and tracked isotherms poleward (e.g., Chust et al., 2013), spatial distributions of Southern Ocean mesozooplankton communities have not advanced polewards, despite surface layer warming in the Atlantic sector over at least the past six decades (Tarling et al., 2017), contrary to the assumptions of species distribution models (e.g., Mackey et al., 2012). In addition, global models often do not perform well for the poles in particular, but can also require tailoring (in downscaling efforts) to particular regions—where the system specificity proves particularly strong (e.g., Bryndum-Buchholz et al., 2018).
Some issues of scale mismatch and interconnection have been solved within terrestrial environments, with methods developed that scale-up individual-based models of fine-scale physiological and ecological processes and dynamics to global ecosystem scales (Moorcroft et al., 2001), including embedding fine-scale vegetation demography within Earth Systems Models (e.g., Fisher et al., 2018) to account for changing climatic conditions. Integral projection models (e.g., those developed by Coulson, 2012) actively try to address the interplay of population ecology and evolution (quantitative genetics). These models clearly show that phenotypes and life histories (and thus parameters in ecological models) will change as adaptation occurs, leading in turn to changing population dynamics (Coulson et al., 2015) and likely ecosystem interactions (Forestier et al., in press). The modeling approach is being extended to cope with novel environmental conditions by linking it with dynamic energy budget models (Smallegange et al., 2017).
Advancing ecological modeling means acknowledging the gap in understanding around cross-scale processes and interactions (represented by the dashed line in Figure 1C), which are only beginning to be appreciated (e.g., Donadi et al., 2017). Traditionally, ecological questions tackled by experimentation have gained tractability by simplifying the circumstances, focusing on single taxa or functional roles, or limited spatial scales. However, recent studies are finding multitrophic richness and abundance strongly influence ecosystem functioning (Soliveres et al., 2016; Ushio et al., 2018). This has likely meant there has been an under-appreciation of the role of diversity in ecosystem functioning (Soliveres et al., 2016), slowing the merging of the composition and functional modeling communities. Similarly, there has been an under-appreciation of the functional role of cross-scale contributions of diversity to functional resilience of ecosystems. For example, there has been much discussion around algal-coral dynamics and resilience to disturbance in tropical reefs (e.g., Hughes et al., 2007), but it wasn't until 2016 that cross-scale processes were considered explicitly (Nash et al., 2015). That study provided empirical support for resilience as a result of the combined effects of cross-scale and functional redundancy—whereby multiple species within a functional group perform a functional role at different spatial scales (cross-scale redundancy) and respond to disturbance in different ways (i.e., functional redundancy via response diversity). Appreciating this involves understanding the contributions of trait diversity (e.g., in dispersal at different life history stages), the implications for the species scale of operation, and how that affects ecosystem properties (Massol et al., 2016). Furthermore, when trying to understand the outcomes of perturbations and predict ecosystem responses there is the simultaneous need to consider the spatial and temporal dimensions of disturbances (i.e., what scales they cross) and how that interacts with these multifaceted forms of redundancy—e.g., can dispersal act as a rescue mechanism or does patch fragmentation or even a regime shift occur (Zelnik et al., 2018)? Bartlett et al. (2016), for example, found that ecosystem responses (both in terms of abundance and compositional structure) were mediated by synergistic interactions between habitat loss and fragmentation; the relative sensitivity of fauna (e.g., large-sized animals) to fragmentation (as this influenced top-down processes within the trophic webs) also played in an important role.
If the contributions of such cross-scale process are to be understood, however, there is the need to be collecting data at the appropriate scales to understand what is going on (and at scales appropriate for addressing the management needs). Unfortunately, as highlighted by a number of recent meta-analyses, many ecological studies have not included a wide enough range of scales to accurately estimate the true scales of effect for particular processes and the resulting inferred ecological-environmental relationships (Jackson and Fahrig, 2015; Martin, 2018). Coming to terms with all of this complexity and avoiding mis-steps due to misplaced focus would likely benefit from a healthier marriage of experimental, theoretical, applied and model-based studies (Essington et al., 2017; see also Table 1). This is particularly important as ecology is not so much about identifying “one true scale” of operation, but recognizing dynamic change is occurring simultaneously across multiple scales due to multiple interacting phenomena (Levin, 1992), thus requiring multiple approaches to elucidate the true dynamics. While integration of multiple lines of evidence (e.g., field studies and ecosystem modeling) is becoming more common there is still a tendency (often due to logistical constraints) for one or the other to feel an unequal partner—ultimately short changing the effective value that can be gained from an even handed combination of the constituent players; such as where field data could provide models with information on how the relationship between predators and prey varies with environmental conditions through space and time (Griffith and Fulton, 2014).
Having worked on a number of large multi-faceted projects over the last 20 years, it is a common pattern in our experience that models are seen as an integrating factor, but that time to develop and deliver that work is concertinaed in terms of time and available resources due to delays in delivery of data or logistical hiccups. This is not about laying blame, but recognizing the plans/intent vs. reality of execution. In areas with greater funding streams, later projects can alleviate this outcome by allowing time to be made up in subsequent rounds of research. This is not the case in economically constrained nations (including Australia in terms of being able to service its entire marine estate given its relatively small human population). This means modelers have to rely on rapid and easily deployable “starting steps” (e.g., loop analysis) so that they can make the most of data as it comes along and have much of the preparatory work done ahead of polishing the final product during later stages of the project work. It also means that integration of input from multiple disciplines must be a very intentional action or “later steps” will be lost. This is often the case if human dimensions are addressed sequentially after biophysical aspects have been addressed and a model includes very simplistic representations that fall short of the dynamic richness seen in the other facets of the model. If these pitfalls can be avoided via intentional efforts to integrate “early and often” then model potential is maximized (as has been the experience in oceanographic models or earth system models, e.g., Medlyn et al., 2016), and it becomes more of an issue of sharing learnings back out to the broader group interested in that system. It is often the case that a modeler more than any other ends up with the completest view of system content, structure and function.
While recognizing these shortfalls is important for going forward from an informed position, to target future steps to reduce uncertainty and improve reliability of predictions, we are not advocating a blanket increase in model complexity. There are many circumstances where building complex models is simply infeasible. Moreover, there is a significant body of work indicating that increasingly complex models are not immediately more reliable (and can often degrade in performance; Ludwig and Walters, 1985; Fulton et al., 2003a; Evans et al., 2013; Collie et al., 2016). Overly simplistic models are error prone (due to the omission of key dynamics), but highly complex models typically lead to poorer decision making—due to accumulated biases, errors and difficulty in interpreting outputs (Ludwig and Walters, 1985; Fulton et al., 2003a). A lot of intuitive insight is sacrificed when models become too complicated. Model performance is often greatest when using a minimum realistic (Butterworth and Harwood, 1991), intermediate level of complexity (Plagányi et al., 2014) that captures the essence of the main interactions (this philosophy holds even when building system models, and including or excluding components with care). In practice this means it is important to think about how we might go about capturing the essence of the cross scale connections without adding undue complexity; how to modify existing approaches or take entirely new model development directions to address the identified shortcomings of existing modeling platforms and successfully tackle cross-scale issues (Table 1).
Operational (tactical) scale models face some of the steepest hurdles when it comes to cross-scale considerations. These models need to be tractably and transparently executed on short time scales and often with limited data sets consistent with what is available to management bodies. Yet they are being increasingly asked to address ecosystem considerations (Plagányi et al., 2014, 2018), such as the implications of the exploitation of prey species for predators or competitors (e.g., Punt et al., 2016). In terrestrial systems there have been some successes in automated near real time process-based models, but the experience in the marine realm (which typically deals with substantially more trophic levels and processes) has been quite different. Growing from the concept of including only the key interactions and processes needed to reproduce the dynamics of the phenomena of interest (Levin, 1992), “Models of Intermediate Complexity for Ecosystem assessments” (MICE) have tackled this marine systems operational challenge by employing simple formulations that are statistically fit to available data, but applied across ecological-environmental-anthropogenic dimensions, to explain the dynamics of small groups of interconnected species (Plagányi et al., 2014). This approach advances tactical management efforts to incorporate and address considerations such as climate effects (Tulloch et al., 2019), moving the modeling more and more into the scales most relevant to resource management interests (Figure 1F). However, hurdles remain around how to provide guidance on ecosystem structural concerns rather than stock status. The ecological and spatiotemporal coverage of these kinds of models is also being expanded by new ways of approaching ecological modeling—such as moving away from population oriented representations to size and trait based models (Blanchard et al., 2017) or equation-free mechanistic empirical dynamic models (Sugihara et al., 2012; Yea et al., 2015; Ye and Sugihara, 2016; Ushio et al., 2018).
As ecological models mature there has been a greater focus on the inter-related topics of parameter fitting (Kramer-Schadt et al., 2007), sensitivity analysis and model uncertainty (Pantus, 2006), model-data fusion (Kuppel et al., 2014), model skill reporting (Olsen et al., 2016), and statistical ensemble modeling (Spence et al., 2018). It is now routinely expected that at least some, if not all, of these will be provided or discussed when documenting model content and outcomes. This is however raising the issue of having sufficient data available to follow through on all aspects of the model. More typically the model is fit to the limited number of available time series (usually only available for exploited or conservation species and often patchy spatially), meaning that model reliability varies across components; which may become problematic if the relative role of the more uncertain components increases under the kinds of disturbance of interest. One underutilized means of checking ecological model skill is retrospective assessment of performance, where model projections are revisited and checked against how the system actually evolved. Given the hundreds of published and applied models it is surprising how rarely this is done (often due to the dictates of shifting funding and focus which does not provide for revisiting old sites and topics). If more is to be made of this potential reservoir of model learning—whether it is used directly to improve mechanistic models or simply to help train machine learning-based emulators—then more needs to be done in terms of having standardized protocols and making models publicly available in repositories (Melbourne-Thomas et al., 2017), much like the push for large publicly available data stores for observational data (Mouquet et al., 2015).
A closer evaluation of model skill will no doubt highlight some model shortcomings. However, when addressing cross-scale issues modelers have already identified and begun to act on some pressing issues. One long-standing aspect of multi-scale effects that has been of prime concern to modelers is the issue of appropriately capturing lags and inertia in ecological and ecosystem models (Fulton et al., 2003a). This has become even more challenging as models have grown to couple physical and socioecological processes (Österblom et al., 2013; Plagányi et al., 2014; Fulton et al., 2015). Even when just constraining the focus to lags in the ecological components, success requires considerable effort. This is because the many interacting processes within ecosystems that dictate biodiversity and ecosystem function operate on and feedback across different scales; necessitating frameworks that explicitly allow for elucidation of the mechanisms and circumstances contributing to cumulative lagged responses (Essl et al., 2015). The need for clear conceptual frameworks to support clear thinking around cumulative, non-linear and interacting effects grows larger once the dimensions (and scales) of the cumulative effects are expanded. This is evident in the growing interest in a pragmatic means of assessing cumulative impacts of human activities and other stressors on ecosystems (Giakoumi et al., 2015; Holsman et al., 2017; Jones et al., 2018; Stelzenmüller et al., 2018). Despite these limitations, the importance of linking human and ecological processes to predict future dynamics has been recognized for some time—e.g., in urban and agricultural systems (Alberti, 2008)—with advances achieved using agent-based models that couple socio-demographic, ecological, and biophysical models (e.g., Filatova et al., 2013; Fulton et al., 2015).
Another aspect of models that is getting much more explicit consideration is dynamic change and variability. In the past the available data and ambient conditions most often meant simplification to single parameterizations were sufficient rather than recognizing individual-level variability (such as the pioneering work by Clark et al., 2003). The directed pressures that characterize our world and expanded available observational data sets mean we now appreciate that heterogeneity is often important and can no longer be safely abstracted away. The resulting move to represent variability and dynamic change in models has come in multiple forms—from recognition of intraspecific variation within existing modeling frameworks (Moran et al., 2006; Melbourne-Thomas et al., 2011; Fulton et al., 2019), to the explicit representation of functional influences of biodiversity, acclimation, adaptation, and eco-evolutionary feedbacks (Table 1). In their simplest forms these processes may be included via allowing for dynamic parameterisations (Jørgensen et al., 2016). In contrast, in terrestrial dynamic global vegetation models fundamental adaptive processes (e.g., acclimation, plasticity, migration, selection, and evolution), are now being accounted for to allow for an exploration of their potential to mitigate effects of climate extremes (Scheiter et al., 2013). Some modeling frameworks are also beginning to explicitly represent evolution and its implications for ecological processes (Grimm and Berger, 2016), such as predation pressure and trait expression (Forestier et al., in press; Romero-Mujalli et al., 2019). This can create new tensions in modeling—what is the effective benefit of replacing one set of fixed parameters (e.g., around growth) with others (e.g., around rates of evolution). Modelers will, most likely, need to ponder this on a case by case basis, at least until alternative means of dealing with the representation of dynamic ecological processes are more mainstream (e.g., potentially leveraging off the model morphing approaches of Gray and Wotherspoon, 2015 discussed more below).
There have also been calls to find a new form of ecosystem modeling that explicitly combines species/functional composition properties with ecosystem process representation (Griffith and Fulton, 2014; Mokany et al., 2016). The intent is to better capture how simultaneous alterations to environmental drivers and compositional diversity (e.g., via exploitation) could interact with significant consequences for ecosystem function (Loreau, 2010a; Durance et al., 2016). Existing composition- and function-based modeling approaches are individually inadequate for a number of reasons (as outlined in Mokany et al., 2016): (i) correlative compositional (biodiversity) models fail to reflect the dynamic outcome of key ecological processes; (ii) trait-based methods are hampered by insufficient information; and (iii) hybrid models that are functionally oriented (i.e., that combine models of key individual species with coarser functional groups) are incapable of tractably representing the high biodiversity present in the majority of systems (Mokany et al., 2016). How to tractably address all of these shortfalls is not yet clear as it will involve developing methods that allow for emergence of the desired phenomena, not all of which are well-understood. Some steps down this road may well have been taken—witness the number of ecological properties related to size (metabolic rate, clearance rate, swimming speed, sensory range, trophic strategy, sensory mode, body shape, and reproductive strategy), feeding mode and “jellyness” (Andersen et al., 2016). Avoiding hardwiring desired behaviors is important however. This is a hard learnt lesson by many marine trophic modelers and is why current food web models allow for the expression of shifts in dominant pathways (Shin et al., 2010). Successfully representing the interactions between system function, fine scale species composition and the implications of different forms of biodiversity within dynamic frameworks will likely involve a fresh take on ecosystem modeling and the development of customized models that integrate processes and scales relevant to both ecosystem composition and function (Mokany et al., 2016; Grimm et al., 2017). In turn, this is likely to require cross fertilization from across many modeling lineages and scientific disciplines. The value of such cross discipline inspiration is being realized in many complex system relevant domains currently, witness the burgeoning of interdisciplinary science (Nature's 2015 special volume, 525, on interdisciplinarity; McDonald et al., 2018).
As we have discussed above some of the key challenges facing modeling and ecology are embracing new modeling approaches, spanning more dimensions (covering more and more of the grid in Figure 1E) and allowing for dynamic and evolving model parameterizations and structures. Multiple nagging concerns are associated with all of these, specifically: (a) how to achieve valuable improvements without being overwhelmed with additional complexity and (b) having sufficient data at multiple scales to usefully inform and constrain the models (Mouquet et al., 2015), with the handling of uncertainty a part of this conundrum. This latter concern, of insufficient data, is not new when looking at barriers to advancing ecology—being expressed for instance by the early ecosystem modelers in the 1970s (Gurney et al., 1994; Pacala, 1994; Scheffer and Beets, 1994). In the first instance the use of cross scale models themselves will help ease the data burden. Where data are unavailable at one scale (e.g., the individual or regional scale), data can be used to assess model performance at another scale (e.g., global). This approach is being exploited in global models (e.g., see Harfoot et al., 2014 or Fisher et al., 2018).
New data sources will also help, though they will come with their own challenges. Technological advances—in terms of automation, sensor capacity, and new monitoring methods (from biochemical tracers, genomics and environmental DNA through to high resolution remote sensing)—are providing data in quantities that can overwhelm traditional methods, driving searches for new approaches that maximize utilization of these valuable resources. Learning to use this data wealth well is a key step (Durden et al., 2017), continuing the demand for the development of new methods. The ecological value of taking the time to do this cannot be overemphasized, as evidenced by the blossoming of discoveries derived from having sufficient volumes of data to deconstruct complex patterns and by marrying data across scales (Chave, 2013; Mouquet et al., 2015). Examples such as the development of close-kin mark recapture methods (Bravington et al., 2016) showcase how new data streams can revolutionize ecological data collection on large scales and provide precision far beyond what was possible for earlier generations of ecologists. The complementary use of multiple data sources—gut contents, biochemical and isotopic tracers, and DNA—are also revolutionizing our understanding of foodweb connections (Pethybridge et al., 2018; McCormack et al., 2019) and changes through time (Young et al., 2015). This kind of utilization is important, as models (conceptual or mathematical) play a key role in bringing many data streams together; the emergence of frameworks to facilitate access and synthesis (e.g., statistical methods, data portals etc.) is beneficial to ecology more broadly.
The broader range of available datasets is also expanding the scales and processes modelers can consider (pushing out the boundary of the area marked in Figure 1F). Due to data constraints models have typically been constructed and tested at levels where the most detailed information exists—an approach known as the “middle-out” (Noble, 2003) or rhomboid approach (deYoung et al., 2004). Whereas this modeling approach can be an intentional developmental decision it is also often an accident of available information—meaning models have focused on the more well-understood scales and processes and missed components at the extremes (see the location of the solid dots in Figure 1F). In the last few years, there has been a growing appreciation of the true influence of pathogens and parasites on ecosystems (e.g., Weitz et al., 2015; Guerrero and Berlanga, 2016; Jephcott et al., 2016; Mischler et al., 2016; Preston et al., 2016; Médoc et al., 2017; Trivedi et al., 2017). It is now understood that predation and parasitism have non-additive effects within food webs (Banerji et al., 2015) and that the collective actions of metabolic processes within aggregate biofilms can have a profound influence on individual, community and ecosystem properties (Guerrero and Berlanga, 2016). Fungal and microbial communities (e.g., in soils) can interactively shape ecosystems at regional to continental scales and mediate energy and material flows at multiple temporal scales (Dighton, 2016; Trivedi et al., 2017). This means that (along with other flora and fauna) microbes play essential roles within biogeochemical cycles of water, carbon, nutrients (Schmitz et al., 2010, 2018), and trace elements (Weitz et al., 2015; Preston et al., 2016); and influence fluxes of both biomass and energy (Mischler et al., 2016), ultimately influencing temporal ecosystem dynamics and disturbance, succession, and stability relevant processes (Preston et al., 2016). Appropriately recognizing the role of the smallest denizens of ecosystems, which also have the most rapid expression of adaptive capacity, will likely be extremely important as we consider the implications of global change for ecosystems.
Bringing together the disparate fields necessary to realize these and other mechanisms behind ecological feedbacks in the earth system is likely to be a key focus of the activities of Earth system groups over the next few years and of the coming UN Decade of Ocean Science. The effort to link changes in energy flow through food webs to carbon uptake and climate regulation will likely involve giving more consideration to mechanisms previously accorded marginal attention—such as the role of consumers, including top predators, in mediating productivity (Estes et al., 2011), either directly via contributions to nutrient cycles (Pastor et al., 2006; Nicol et al., 2010) and physical mixing in the ocean (Katija and Dabiri, 2009), or indirectly via the “ecology of fear” (where signs of predators in an area can modify prey behavior even in the absence of direct attacks; Wirsing and Ripple, 2011). It may even involve the exploration of small scale interactions between seemingly unconnected processes—such as the potential for fisheries (which can have cascading effects down to the plankton, Reid et al., 2000) to influence local atmospheric processes via plankton-mediated processes. Examples include dimethyl sulfide production and the influence on cloud formation (Malin et al., 1992), or the carbon cycle (Monroe et al., 2018). Model coupling—interactively joining models of different types and scales (physical, biogeochemical, trophic, habitat, human use)—will likely also be a strong feature of the coordinated work across disciplines, as already seen in some terrestrial modeling (e.g., Alberti, 2008) and in earth system modeling efforts to date (Prinn, 2012; Fisher et al., 2014, 2018). The evolution of ecosystem modeling has already been marked with a shift from one-way coupled drivers influencing small groups of species to more systematic representations of interaction networks characterized by multiple pathways and processes (Shin et al., 2010), allowing for significant growth in modeling capacity.
This increase in capacity has seen models used in an increasing number of roles—not just academic, but as decision support (Condie et al., 2005; Plagányi et al., 2014) and as a means of inputting advice to international initiatives, such as the IPCC (e.g., Hartmann et al., 2013). This has put the effective handling of uncertainty front and center. A full review of how that field is developing is beyond the scope of this paper, but it is worth noting that recognition of structural uncertainty (i.e., uncertainty pertaining to the model assumptions, formulation, and internal connections) has been a key part of these modeling efforts. The use of Bayesian approaches and model ensembles to provide ranges of possible outcomes across model types is now becoming more common (e.g., Gharbia et al., 2016). The first use of model ensembles was in economics (Bates and Granger, 1969) but has now become a staple of many fields—economics, systematics, meteorology, and climatology—and is often now used when considering shifting species distributions (e.g., Araújo and New, 2007) or terrestrial ecosystem impacts (Baker et al., 2019). Model intercomparison projects (MIPs; e.g., Warszawski et al., 2014; Lotze et al., 2019) are also bringing together the world's modeling community to share outcomes, lessons and understanding, accelerating model improvements and rigor. This kind of multi-model approach has been relatively easily accepted, as it is a logical outgrowth of the even more familiar parameter variation form of sensitivity testing and uncertainty analysis.
By comparison, the future development of multi-scale and hybrid approaches (Table 1) will require ecology to move well beyond its current “comfort zone.” Most disciplines begin with simplifying assumptions as a foundation to build from and ecology has been no exception—note the simplicity of the Lotka-Volterra assumptions for predators and prey. Indeed, the vast majority of extant ecological and ecosystem models still assume fixed parameterisations and even for those that don't (e.g., Jørgensen, 2012) they most often assume fixed functional relationships and formulations. This means, as touched on above, that more remains to be done in terms of representing appropriate levels of variability and dynamic change. Under perturbation, ecological systems can exhibit significant structural reorganization and shifts in the dominance of species and processes. Modeling techniques attempting to appropriately represent this magnitude of response may well require not just changing parameters but dynamically changing structural components (the network connections) and process expression (e.g., functional forms), and potentially even shifting model scales as the simulated systems evolve.
In terms of the approaches that can facilitate the representation of fully dynamic structural properties of models and ecosystems, both allometric food web models (Curtsdotter et al., 2017; Reum et al., 2019) and network approaches show promise. Experimentation with dynamic network approaches is allowing for the representation of dynamic restructuring behavior (Bryden et al., 2012), reflecting changes in the properties of system components (e.g., individuals or species) and the effect that has on interactions. These approaches (and other model types that allow for shifting food web links) have the potential to capture some of the ecological surprises that arise in changing ecosystems; such as context-dependent reversal of predator-prey roles, as has occurred between sprat and cod in the Baltic (Köster and Möllmann, 2000) as well as lobsters and whelks on Marcus Island in South Africa (Barkai and McQuaid, 1988).
One approach to spanning ecological scales is to explicitly link models that resolve processes and function at different resolutions (Walpole et al., 2013). A number of ecosystem models already do this to differing degrees and the approach has significant potential—at least up to a point. While it is possible to follow a common formulation regime to resolve neighboring scales, it becomes more difficult as the number of scales grows. The kinds of challenges to continuing those approaches as we move to the smallest and largest scales have already been touched on in previous sections. A promising alternative approach for tackling multiple scales and dynamic ecological phenomena is the hybrid modeling approach (Table 1). This modeling method sees modelers create ecological systems models by combining multiple modeling approaches from multiple disciplines (e.g., system models, process-based representations, Markov chains, Bayesian networks, cellular automata or other agent/individual based approaches, statistical models (including multivariate and Bayesian methods discussed previously), partial differential equation based physical or biogeochemical models, geographic information systems and approaches from artificial intelligence, or machine learning algorithms). This approach has been a very useful step toward grappling with multi-scale complexity of ecosystems or broader socioecological systems (McDonald et al., 2008; Parrott, 2011). Use of such mixed model formulations has matured over the past 20 years, where it started from the humble beginnings of using empirically derived functional forms or correlative statistical approaches to represent more poorly known system components or ecological processes (Fulton et al., 2003a). This combined approach is being advocated strongly as additional scales and processes are embraced in ecological models—for example in implementing integrated models of ecosystem composition and function (Mokany et al., 2016) or considering socioecological systems (Melbourne-Thomas et al., 2017). Taking a hybrid approach allows for the synthesis of many kinds of data from many different sources (Cressie et al., 2009), thereby fostering greater understanding (Mouquet et al., 2015) by providing an interface across disparate scales, phenomena and disciplines (Levin, 1992).
This flexible hybrid approach allows for the selective representation of each component of a system in the “currency” (spatiotemporal scales, units, complexity of detail) that best captures that component and maximizes the overall model utility (Gray and Wotherspoon, 2015). New mathematical breakthroughs have shown that it is possible to go still further, to allow for truly dynamic model structures that shift in representational form as the dominant components and processes operating in the system change (Gray and Wotherspoon, 2015). These new approaches allow sub-models to change their form (e.g., from differential equations to individual based) conditional on their own state and the states of the other system components that they are directly or indirectly interacting with. For example, moving from a population level representation to an individual-based model and back as migrating animals encounter a contaminant plume (Gray and Wotherspoon, 2012), or any other event where heterogeneity in a process may be important for the system level outcome. The results of these early efforts indicate that allowing for such switching forms is not only feasible but beneficial in terms of fidelity to observed real world dynamics and the computational efficiency. Fine scale detail is only retained when it is needed, when fine scale phenomena dominate, with the statistical summaries saving that detail in a useful state during periods when coarser representations are sufficient as dominant processes are occurring at higher scales. While the tree-based mathematics and coding languages required to develop such models are still under active development, the potential seems vast for addressing more scales while keeping model complexity tractable. In addition, marrying such methods with the growing fields of artificial intelligence and neurocomputing could see fluidly self-mutating model structures that allow ecologists to delve seamlessly into and across all the spatiotemporal scales. This push for new methods is not to say simpler models should be universally abandoned, but that new approaches should be added to the suite of tools available to expand our capacity to look at questions from all relevant angles.
All of the roles that models have traditionally had in ecology—explanatory and predictive—will be just as important going forward. The utility of models as thinking tools will be needed for interpreting new and novel situations, where the magnitude of disturbance potentially highlights how strong the links between scales can really be. In addition, it is already clear that models will be central aids for navigating adaptation and equitable, sustainable societal outcomes. In our experience, the changing tenor of the questions being asked of (socio)ecological models over the course of the last 20–30 years clearly shows that global change is already presenting decision makers with increasingly complex and fraught decisions. The volume of the safe operating space is being squeezed (Steffen et al., 2015) and models are needed to help understand how to navigate a world where transient dynamics rather than equilibrium assumptions are most relevant. Hence, we posit that some of the key challenges facing modeling and ecology involve embracing new modeling approaches and data streams, spanning more dimensions, filling the gaps at the smallest and grandest scales, and allowing for dynamic and evolving model parameterizations and structures (Table 1).
Ecology has been built by generations of scientists concerned with the dynamics of ecosystems and the mechanisms shaping the spatial and temporal patterns that characterize them (Levin, 1992). Now the scales considered are expanding, complementing traditional foci with an interest in new scales (large and small), new processes, and new analytical and modeling methods, may also require rethinking the goalposts of progress in ecological science (Currie, 2019). We've come a long way, but as we outline in the sub-sections above, there is still a long way to go, especially given growing anthropogenic pressures and the resultant rates of change in socioecological systems. We summarize gaps in fundamental ecological understanding that widen further when considering socio-ecological responses as well as emerging needs to handle uncertainty. Moreover, large gaps are evident in ecological understanding of entire fields of research, such as the influence of pathogens and parasites on ecosystems. Most of the competing extant model formulations overlap and equally explain the bulk of conditions, but the devil has been in the detail at the extremes (Fulton et al., 2003b). Unfortunately, we are increasingly living in a world where we are pushing toward extremes. Closing the gaps in future ecological work will necessitate understanding mechanisms behind ecological feedbacks in the earth system. Consequently, in the coming decades, ecology will be spending a lot of time weaving those new scales and new lessons into its lexicon of understanding.
EF conceived of the paper. All authors contributed to writing it (agreeing on content of what became Table 1, concepts included in what ultimately became Figure 1). While VT did bring in extra terrestrial content, in actuality all authors contributed to the ideas, writing and links to broader literature contained in the revised text.
The authors declare that the research was conducted in the absence of any commercial or financial relationships that could be construed as a potential conflict of interest.
Thanks are due to the many mentors and collaborators who have discussed modeling concepts and fixes with us through the years. In particular the contributions by Tony Smith, John Parslow, Carl Walters, Simon Ferrier, Karel Mokany, Asta Audzijonyte, George Branch, Doug Butterworth, and John G. Field. In particular, we would like to sincerely thank E. J. Milner-Gulland for some very insightful comments on a draft of this paper.
Ahrens, R. N. M., Walters, C. J., and Christensen, V. (2012). Foraging arena theory. Fish. Fish. 13, 41–59. doi: 10.1111/j.1467-2979.2011.00432.x
Alberti, M. (2008). Advances in Urban Ecology: Integrating Humans and Ecological Processes in Urban Ecosystems. New York, NY: Springer.
Anand, M., Gonzalez, A., Guichard, F., Kolasa, J., and Parrott, L. (2010). Ecological systems as complex systems: challenges for an emerging science. Diversity 2:395410. doi: 10.3390/d2030395
Andersen, K. H., Berge, T., Gonçalves, R. J., Hartvig, M., Heuschele, J., Hylander, S., et al. (2016). Characteristic sizes of life in the oceans, from bacteria to whales. Annu. Rev. Mar. Sci. 8, 3.1–3.25. doi: 10.1146/annurev-marine-122414-034144
Anderson, R. M., and May, R. M. (1978). Regulation and stability of host-parasite population interactions. J. Anim. Ecol. 47, 219–247. doi: 10.2307/3933
Anderson, R. M., and May, R. M. (1979). Population biology of infectious diseases: Part II. Nature 280, 455–461. doi: 10.1038/280361a0
Araújo, M. B., and New, M. (2007). Ensemble forecasting of species distributions. Trends Ecol. Evol. 22, 42–47. doi: 10.1016/j.tree.2006.09.010
Audzijonyte, A., Kuparinen, A., and Fulton, E. A. (2013). How fast is fisheries-induced evolution? Quantitative analysis of modelling and empirical studies. Evol. Appl. 6, 585–595. doi: 10.1111/eva.12044
Baker, C. M., Bode, M., Dexter, N., Lindenmayer, D. B., Foster, C., MacGregor, C., et al. (2019). A novel approach to assessing the ecosystem-wide impacts of reintroductions. Ecol. Appl. 29:e01811. doi: 10.1002/eap.1811
Baker, C. M., Gordon, A., and Bode, M. (2017). Ensemble ecosystem modeling for predicting ecosystem response to predator reintroduction. Cons. Biol. 31, 376–384. doi: 10.1111/cobi.12798
Baker, C. M., Holden, M. H., Plein, M., McCarthy, M. A., and Possingham, H. P. (2018). Informing network management using fuzzy cognitive maps. Biol. Conserv. 224, 122–128. doi: 10.1016/j.biocon.2018.05.031
Banerji, A., Duncan, A. B., Griffin, J. S., Humphries, S., Petchey, O. L., and Kaltz, O. (2015). Density- and trait-mediated effects of a parasite and a predator in a tri-trophic food web. J. Anim. Ecol. 84, 723–733. doi: 10.1111/1365-2656.12317
Barkai, A., and McQuaid, C. (1988). Predator-prey role reversal in a marine benthic ecosystem. Science 242, 62–64. doi: 10.1126/science.242.4875.62
Bartlett, L. J., Newbold, T., Purves, D. W., Tittensor, D. P., and Harfoot, M. B. J. (2016). Synergistic impacts of habitat loss and fragmentation on model ecosystems. Proc. R. Soc. B. 283:20161027. doi: 10.1098/rspb.2016.1027
Bascompte, J. (2009). Disentangling the web of life. Science 325, 416–419. doi: 10.1126/science.1170749
Bates, A. E., Pecl, G. T., Frusher, S., Hobday, A. J., Wernberg, T., Smale, D. A., et al. (2014). Defining and observing stages of climate-mediated range shifts in marine systems. Glob. Environ. Change 26, 27–38. doi: 10.1016/j.gloenvcha.2014.03.009
Bates, J. M., and Granger, C. W. (1969). The combination of forecasts. Operat. Res. Quart. 20, 451–468. doi: 10.1057/jors.1969.103
Bennett, S., Wernberg, T., Joy, B. A., de Bettignies, T., and Campbell, A. H. (2015). Central and rear-edge populations can be equally vulnerable to warming. Nat. Comm. 6:10280. doi: 10.1038/ncomms10280
Berge, T., Chakraborty, S., Hansen, P. J., and Andersen, K. H. (2017). Modeling succession of key resource-harvesting traits of mixotrophic plankton. ISME J. 11, 212–223. doi: 10.1038/ismej.2016.92
Bestelmeyer, B. T., Ash, A., Brown, J. R., Densambuu, B., Fernández-Giménez, M., Johanson, J., et al. (2017). “State and transition models: theory, applications, and challenges,” in Rangeland Systems Processes, Management and Challenges, ed D. D. Briske (Cham: Springer). doi: 10.1007/978-3-319-46709-2_9
Birks, H. H., and Birks, H. J. B. (2003). Multi-proxy studies in palaeolimnology. Veg. Hist. Archaeobot. 15, 235–251. doi: 10.1007/s00334-006-0066-6
Blanchard, J. L., Heneghan, R. F., Everett, J. D., Trebilco, R., and Richardson, A. J. (2017). From bacteria to whales: using functional size spectra to model marine ecosystems. Trends Ecol. Evol. 32, 174–186. doi: 10.1016/j.tree.2016.12.003
Bonduriansky, R. (2012). Rethinking heredity, again. Trends Ecol. Evol. 27, 330–336. doi: 10.1016/j.tree.2012.02.003
Bozinovic, F., and Naya, D. E. (2015). “Linking physiology, climate, and species distributional ranges,” in Integrative Organismal Biology, eds L. B. Martin, C. K. Ghalambor, and H. A. Woods (Hoboken, NJ: John Wiley & Sons), 277–290.
Bravington, M. V., Skaug, H. J., and Anderson, E. C. (2016). Close-kin mark-recapture. Stat. Sci. 31, 259–274. doi: 10.1214/16-STS552
Brown, J. H., Gillooly, J. F., Allen, A. P., Savage, V. M., and West, G. B. (2004). Toward a metabolic theory of ecology. Ecology 85, 1771–1789. doi: 10.1890/03-9000
Brown, J. S. (2016). Why Darwin would have loved evolutionary game theory. Proc. R. Soc. B 283:20160847. doi: 10.1098/rspb.2016.0847
Bryden, J., Funk, S., Geard, N., Bullock, S., and Jansen, V. A. A. (2012). Stability in flux: community structure in dynamic networks. J. R. Soc. Interface 8, 1031–1040. doi: 10.1098/rsif.2010.0524
Bryndum-Buchholz, A., Tittensor, D. P., Blanchard, J. L., Cheung, W. W. L., Coll, M., Galbraith, E. D., et al. (2018). 21st Century climate change impacts on marine animal biomass and ecosystem structure across ocean Basins. Glob. Change Biol. 25, 459–472. doi: 10.1111/gcb.14512
Butterworth, D. S., and Harwood, J. (eds.). (1991). Report on the Benguela Ecology Programme Workshop on Seal-Fishery Biological Interactions. Cape Town: Benguela Ecology Programme, Department Zoology, University of Cape Town.
Cabral, J. S., Valente, L., and Hartig, F. (2017). Mechanistic simulation models in macroecology and biogeography: state-of-art and prospects. Ecography 40, 267–280. doi: 10.1111/ecog.02480
Carpenter, S. R., Kitchell, J. F., Hodgson, J. R., Cochran, P. A., Elser, J. J., Elser, M. M., et al. (1987). Regulation of lake primary productivity by food web structure. Ecology 68, 1863–1876. doi: 10.2307/1939878
Cazelles, K., Aráujo, M. B., Mouquet, N., and Gravel, D. (2016). A theory for species co-occurrence in interaction networks. Theor. Ecol. 9, 39–48. doi: 10.1007/s12080-015-0281-9
Chasco, B. E., Kaplan, I. C., Thomas, A. C., Acevedo-Gutiérrez, A., Noren, D. P., Ford, M. J., et al. (2017). Competing tradeoffs between increasing marine mammal predation and fisheries harvest of Chinook salmon. Sci. Rep. 7:15439. doi: 10.1038/s41598-017-14984-8
Chave, J. (2013). The problem of pattern and scale in ecology: what have we learned in 20 years? Ecol. Lett. 16, 4–16. doi: 10.1111/ele.12048
Cheung, W. W. L., Lam, V. W. Y., Sarmiento, J. L., Kearney, K., Watson, R., and Pauly, D. (2009). Projecting global marine biodiversity impacts under climate change scenarios. Fish Fish. 10, 235–251. doi: 10.1111/j.1467-2979.2008.00315.x
Chown, S. L., Sinclair, B. J., Leinaas, H. P., and Gaston, K. J. (2004). Hemispheric asymmetries in biodiversity - a serious matter for ecology. PLoS Biol. 2:e406. doi: 10.1371/journal.pbio.0020406
Chuang, A., and Peterson, C. R. (2016). Expanding population edges: theories, traits, and trade-offs. Glob. Change Biol. 22, 494–512. doi: 10.1111/gcb.13107
Chust, G., Castellani, C., Licandro, P., Ibaibarriaga, L., Sagarminaga, Y., and Irigoien, X. (2013). Are Calanus spp. shifting poleward in the North Atlantic? A habitat modelling approach. ICES J. Mar. Sci. 71, 241–253. doi: 10.1093/icesjms/fst147
Clark, J. S., Mohan, J., Dietze, M., and Ibanez, I. (2003). Coexistence: How to identify trophic trade-offs. Ecology 84, 17–31. doi: 10.1890/0012-9658(2003)084[0017:CHTITT]2.0.CO;2
Clements, F. E. (1916). Plant Succession an Analysis of the Development of Vegetation. Washington, DC: Carnegie Institution of Washington. doi: 10.5962/bhl.title.56234
Collie, J. S., Botsford, L. W., Hastings, A., Kaplan, I. C., Largier, J. L., Livingston, P. A., et al. (2016). Ecosystem models for fisheries management: finding the sweet spot. Fish. Fish. 17, 101–125. doi: 10.1111/faf.12093
Condie, S., Plagányi, E., Morello, E., Hock, K., and Beeden, R. (2018). Great Barrier Reef recovery through multiple interventions. Cons. Biol. 32, 1356–1367. doi: 10.1111/cobi.13161
Condie, S. A., Waring, J., Mansbridge, J. V., and Cahill, M. L. (2005). Marine connectivity patterns around the Australian continent. Environ. Model. Softw. 20, 1149–1157. doi: 10.1016/j.envsoft.2004.07.005
Connolly, S. R., Keith, S. A., Colwell, R. K., and Rahbek, C. (2017). Process, mechanism, and modeling in macroecology. Trends Ecol. Evol. 32, 835–844. doi: 10.1016/j.tree.2017.08.011
Costanza, R., and Sklar, F. H. (1985). Articulation, accuracy and effectiveness of mathematical models: a review of freshwater wetland applications. Ecol. Model. 27, 45–68. doi: 10.1016/0304-3800(85)90024-9
Coulson, T. (2012). Integral projections models, their construction and use in posing hypotheses in ecology. Oikos 121, 1337–1350. doi: 10.1111/j.1600-0706.2012.00035.x
Coulson, T., Plard, F., Schindler, S., Ozgul, A., and Gaillard, J.-M. (2015). Quantitative genetics meets integral projection models: unification of widely used methods from ecology and evolution. bioRxiv [Preprint]. doi: 10.1101/026260
Courchamp, F., Berec, L., and Gascoigne, J. (2008). Allee Effects in Ecology and Conservation. New York, NY: Oxford University Press. doi: 10.1093/acprof:oso/9780198570301.001.0001
Crepin, A.-S., and Lindahl, T. (2009). Grazing games: sharing common property resources with complex dynamics. Environ. Resour. Econ. 44, 29–46. doi: 10.1007/s10640-008-9258-0
Cressie, N., Calder, C. A., Clark, J. S., Hoef, J. M. V., and Wikle, C. K. (2009). Accounting for uncertainty in ecological analysis: the strengths and limitations of hierarchical statistical modeling. Ecol. Appl. 19, 553–570. doi: 10.1890/07-0744.1
Cumming, G. S., Allen, C. R., Ban, N. C., Biggs, D., Biggs, H. C., Cumming, D. H., et al. (2015). Understanding protected area resilience: a multi-scale, social-ecological approach. Ecol. Appl. 25, 299–319. doi: 10.1890/13-2113.1
Currie, D. J. (2019). Where Newton might have taken ecology. Global Ecol. Biogeogr. 28, 18–27. doi: 10.1111/geb.12842
Curtsdotter, A., Banks, H. T., Banks, J. E., Jonsson, M., Jonsson, T., Laubmeier, A. N., et al. (2017). Ecosystem function in predator-prey food webs-confronting dynamic models with empirical data. J. Anim. Ecol. 88, 196–210. doi: 10.1111/1365-2656.12892
Dale, V. H., and Beyeler, S. C. (2001). Challenges in the development and use of ecological indicators. Ecol. Ind. 1, 3–10. doi: 10.1016/S1470-160X(01)00003-6
Dambacher, J. M., Li, H. W., and Rossignol, P. A. (2003). Qualitative predictions in model ecosystems. Ecol. Model. 161, 79–93. doi: 10.1016/S0304-3800(02)00295-8
Danchin, E., Charmantier, A., Champagne, F. A., Mesoudi, A., Pujol, B., and Blanchet, S. (2011). Beyond DNA: integrating inclusive inheritance into an extended theory of evolution. Nat. Rev. Genet. 12, 475–486. doi: 10.1038/nrg3028
de Little, S. C., Webb, J. A., Miller, K. A., Rutherfurd, I. D., and Stewardson, M. J. (2013). “Using Bayesian hierarchical models to measure and predict the effectiveness of environmental flows for ecological responses,” in 20th International Congress on Modelling and Simulation (Adelaide, SA). Available online at: www.mssanz.org.au/modsim2013
DeAngelis, D. L., and Mooij, W. M. (2005). Individual-based modeling of ecological and evolutionary processes. Annu. Rev. Ecol. Evol. Syst. 36, 147–168. doi: 10.1146/annurev.ecolsys.36.102003.152644
deYoung, B., Heath, M., Werner, F., Chai, F., Megrey, B., and Monfray, P. (2004). Challenges of modeling ocean basin ecosystems. Science 304, 1463–1466. doi: 10.1126/science.1094858
Diez, J. M., and Pulliam, H. R. (2007). Hierarchical analysis of species distributions and abundance across environmental gradients. Ecology 88, 3144–3152. doi: 10.1890/07-0047.1
Dighton, J. (2016). Fungi in Ecosystem Processes. Boca Raton, FL: CRC Press Taylor & Francis Group. doi: 10.1201/b19652
Donadi, S., Austin, Å. N., Bergström, U., Eriksson, B. K., Hansen, J. P., Jacobson, P., et al. (2017). A cross-scale trophic cascade from large predatory fish to algae in coastal ecosystems. Proc. R. Soc. B 284:20170045. doi: 10.1098/rspb.2017.0045
Dunne, J. A., Labandeira, C. C., and Williams, R. J. (2014). Highly resolved early Eocene food webs show development of modern trophic structure after the end-Cretaceous extinction. Proc. R. Soc. B 281:20133280. doi: 10.1098/rspb.2013.3280
Dunstan, P. K., and Foster, S. D. (2011). RAD biodiversity: prediction of rank abundance distributions from deep water benthic assemblages. Ecography 34:798–806. doi: 10.1111/j.1600-0587.2010.06552.x
Durance, I., Bruford, M. W., Chalmers, R., Chappell, N. A., Christie, M., Cosby, B. J., et al. (2016). The challenges of linking ecosystem services to biodiversity: lessons from a large-scale freshwater study. Adv. Ecol. Res. 54, 87–134. doi: 10.1016/bs.aecr.2015.10.003
Durden, J. M., Luo, J. Y., Alexander, H., Flanagan, A. M., and Grossmann, L. (2017). Integrating ‘big data’ into aquatic ecology: challenges and opportunities. Bull. Limnol. Oceanogr. 26, 101–108. doi: 10.1002/lob.10213
Egerton, F. N. (1977). A bibliographical guide to the history of general ecology and population ecology. Hist. Sci. 15, 189–215. doi: 10.1177/007327537701500302
Eikeset, A. M., Dunlop, E. S., Heino, M., Storvik, G., Stenseth, N. C., and Dieckmann, U. (2016). Roles of density-dependent growth and life history evolution in accounting for fisheries-induced trait changes. Proc. Natl. Acad. Sci. U.S.A. 113,15030–15035. doi: 10.1073/pnas.1525749113
Eklöf, A., and Stouffer, D. B. (2016). The phylogenetic component of food web structure and intervality. Theor. Ecol. 9, 107–115. doi: 10.1007/s12080-015-0273-9
Ekschmitt, K., and Breckling, B. (1994). Competition and coexistence: the contribution of modelling to the formation of ecological concepts. Ecol. Model. 75, 71–82. doi: 10.1016/0304-3800(94)90008-6
Ellis, N., and Pantus, F. (2001). Management Strategy Modelling: Tools to Evaluate Trawl Management Strategies With Respect to Impacts on Benthic Biota within the Great Barrier Reef Marine Park Area. CSIRO Marine Research, Cleveland, OH.
Elton, C. S. (1924). Periodic fluctuations in the numbers of animals: their causes and effects. Br. J. Exp. Biol. 2, 119–163.
Essington, T. E., Ciannelli, L., Heppell, S. S., Levin, P. S., McClanahan, T. R., Micheli, F., et al. (2017). Empiricism and modeling for marine fisheries: advancing an interdisciplinary science. Ecosystems 20, 237–244. doi: 10.1007/s10021-016-0073-0
Essl, F., Dullinger, S., Rabitsch, W., Hulme, P. E., Pyšek, P., Wilson, J. R. U., et al. (2015). Historical legacies accumulate to shape future biodiversity in an era of rapid global change. Divers. Distrib. 21, 534–547. doi: 10.1111/ddi.12312
Estes, J. A., Doak, D. F., Springer, A. M., and Williams, T. M. (2009). Causes and consequences of marine mammal population declines in southwest Alaska: a food-web perspective. Philos. Trans. R. Soc. B 364, 1647–1658. doi: 10.1098/rstb.2008.0231
Estes, J. A., Terborgh, J., Brashares, J. S., Power, M. E., Berger, J., Bond, W. J., et al. (2011). Trophic downgrading of planet earth. Science 333, 301–306. doi: 10.1126/science.1205106
Evans, M. R., Grimm, V., Johst, K., Knuuttila, T., de Langhe, R., Lessells, C. M., et al. (2013). Do simple models lead to generality in ecology? Trends Ecol. Evol. 28, 578–583. doi: 10.1016/j.tree.2013.05.022
Fan, Y., Chen, J., Shirkey, G., John, R., Wu, S. R., Park, H., et al. (2016). Applications of structural equation modeling (SEM) in ecological studies: an updated review. Ecol. Process. 5:19. doi: 10.1186/s13717-016-0063-3
FAO (2005). “Mapping global urban and rural population distributions,” in Environment and Natural Resources Worjing Paper 24, eds M. Salvatore, F. Pozzi, E. Ataman, B. Huddleston, and M. Bloise (Rome: FAO).
FAO (2008). Fisheries Management. 2. The Ecosystem Approach to Fisheries. 2.1 Best Practices in Ecosystem Modelling for Informing an Ecosystem Approach to Fisheries. Fisheries Technical Guidelines for Responsible Fisheries. Rome: FAO.
Ferguson, N. M., Anderson, R. M., and May, R. M. (1997). “Scale, persistence and synchronicity: measles as a paradigm of a spatially structured ecosystem,” in Spatial Ecology: the Role of Space in Population Dynamics and Interspecific Interactions, eds D. Tilman and P. Karieva (Princeton, NJ: Princeton University Press) 137–157.
Ferrario, F., Iveša, L., Jaklin, A., Perkol-Finkel, S., and Airoldi, L. (2016). The overlooked role of biotic factors in controlling the ecological performance of artificial marine habitats. J. App. Ecol. 53, 16–24. doi: 10.1111/1365-2664.12533
Filatova, T., Verburg, P. H., Parker, D. C., and Stannard, C. A. (2013). Spatial agent-based models for socio-ecological systems: challenges and prospects. Environ. Model. Softw. 45, 1–7. doi: 10.1016/j.envsoft.2013.03.017
Finnegan, S., Anderson, S. C., Harnik, P. G., Simpson, C., Tittensor, D. P., Byrnes, J. E., et al. (2015). Paleontological baselines for evaluating extinction risk in the modern oceans. Science 348, 567–570. doi: 10.1126/science.aaa6635
Fisher, J. B., Huntzinger, D. N., Schwalm, C. R., and Sitch, S. (2014). Modeling the terrestrial biosphere. Annu. Rev. Environ. Resour. 39, 91–123. doi: 10.1146/annurev-environ-012913-093456
Fisher, R. A., Koven, C. D., Anderegg, W. R., Christoffersen, B. O., Dietze, M. C., Farrior, C. E., et al. (2018). Vegetation demographics in earth system models: a review of progress and priorities. Glob. Change Biol. 24, 35–54. doi: 10.1111/gcb.13910
Follows, M. J., Dutkiewicz, S, Grant, S., and Chisholm, S.W. (2009). Emergent biogeography of microbial communities in a model ocean. Science 315, 1843–1846. doi: 10.1126/science.1138544
Forestier, R., Blanchard, J. L., Nash, K. L., Fulton, E. A., Johnson, C., and Audzijonyte, A. (in press). Species' size determines the adaptive response to predation fishing in an eco-evolutionary size-structured community model. J. Anim. Ecol.
Franks, P. J. S. (2002). NPZ models of plankton dynamics: their construction, coupling to physics and application. J. Oceanog. 58, 379–387. doi: 10.1023/A:1015874028196
Fulton, E. A. (2010). Approaches to end-to-end ecosystem models. J. Mar. Syst. 81, 171–183. doi: 10.1016/j.jmarsys.2009.12.012
Fulton, E. A., Boschetti, F., Sporcic, M., Jones, T., Little, L. R., Dambacher, J. M., et al. (2015). A multi-model approach to engaging stakeholder and modellers in complex environmental problems. Environ. Sci. Policy 48, 44–56. doi: 10.1016/j.envsci.2014.12.006
Fulton, E. A., Bravo, F., Bustamante, R. H., Condie, S., Dambacher, J., Gorton, R., et al. (2018). “SIMA austral systems models for integrated risk assessment of chilean aquaculture,” in SIMA Austral. FIE V008 - Integrated Ecosystem-Based Sanitary and Environmental Management System for Aquaculture, eds Steven et al. (Hobart: CSIRO), 38–64.
Fulton, E. A., Fuller, M., Smith, A. D. M., and Punt, A. (2005). Ecological Indicators of the Ecosystem Effects of Fishing: Final Report. Australian Fisheries Management Authority Report.
Fulton, E. A., and Gorton, R. (2014). Adaptive Futures for SE Australian Fisheries & Aquaculture: Climate Adaptation Simulations. CSIRO.
Fulton, E. A., Parslow, J. S., Smith, A. D. M., and Johnson, C. R. (2004). Biogeochemical marine ecosystem models II: the effect of physiological detail on model performance. Ecol. Model. 173, 371–406. doi: 10.1016/j.ecolmodel.2003.09.024
Fulton, E. A., Punt, A. E., Dichmont, C. M., Harvey, C. J., and Gorton, R. (2019). Ecosystems say good management pays off. Fish. Fish. 20, 66–96. doi: 10.1111/faf.12324
Fulton, E. A., Smith, A. D. M., and Johnson, C. R. (2003a). Effect of complexity on marine ecosystem models. Mar. Ecol. Prog. Ser. 253, 1–16. doi: 10.3354/meps253001
Fulton, E. A., Smith, A. D. M., and Johnson, C. R. (2003b). Mortality and predation in ecosystem models: is it important how these are expressed? Ecol. Model. 169, 157–178. doi: 10.1016/S0304-3800(03)00268-0
Fulton, E. A., Smith, A. D. M., Smith, D. C., and Johnson, P. (2014). An integrated approach is needed for ecosystem based fisheries management: insights from ecosystem-level management strategy evaluation. PLoS ONE 9:e84242. doi: 10.1371/journal.pone.0084242
Gao, J., Barzel, B., and Barabási, A.-L. (2016). Universal resilience patterns in complex networks. Nature 530, 307–312. doi: 10.1038/nature16948
Gaston, K. J., and Blackburn, T. M. (1999). A critique for macroecology. Oikos 84, 353–368. doi: 10.2307/3546417
Gharbia, S. S., Gill, L., Johnston, P., and Pilla, F. (2016). Multi-GCM ensembles performance for climate projection on a GIS platform. Model. Earth Syst. Environ. 2:102. doi: 10.1007/s40808-016-0154-2
Ghilarov, M. S. (1944). Correlation between size and number of soil animals. Dokl. Akad. Nauk 43, 283–285.
Giakoumi, S., Halpern, B. S., Michel, L. N., Gobert, S., Sini, M., Boudouresque, C. F., et al. (2015). Towards a framework for assessment and management of cumulative human impacts on marine food webs. Cons. Biol. 29, 1228–1234. doi: 10.1111/cobi.12468
Gillman, L. N., and Wright, S. D. (2014). Species richness and evolutionary speed: the influence of temperature, water and area. J. Biogeogr. 41, 39–51. doi: 10.1111/jbi.12173
Gould, S. J., and Eldredge, N. (1977). Punctuated equilibria: the tempo and mode of evolution reconsidered. Paleobiology 3, 115–151. doi: 10.1017/S0094837300005224
Gray, R., Fulton, E. A., Little, L. R., and Scott, R. (2006). Operating Model Specification Within an Agent Based Framework. North West Shelf Joint Environmental Management Study Technical Report. CSIRO, Hobart, TAS, 127.
Gray, R., and Wotherspoon, S. (2012). Increasing model efficiency by dynamically changing model representations. Environ. Model. Softw. 30, 115–122. doi: 10.1016/j.envsoft.2011.08.012
Gray, R., and Wotherspoon, S. (2015). Adaptive submodel selection in hybrid models. Front. Environ. Sci. 3:58. doi: 10.3389/fenvs.2015.00058
Griffith, G. P., and Fulton, E. A. (2014). New approaches to simulating the complex interaction effects of multiple human impacts on the marine environment. ICES J. Mar. Sci. 71, 764–774. doi: 10.1093/icesjms/fst196
Grimm, V., Ayllón, D., and Railsback, S. F. (2017). Next-generation individual-based models integrate biodiversity and ecosystems: yes we can, and yes we must. Ecosystems 20, 229–236. doi: 10.1007/s10021-016-0071-2
Grimm, V., and Berger, U. (2016). Structural realism, emergence, and predictions in next-generation ecological modelling: Synthesis from a special issue. Ecol. Model. 326,177–187. doi: 10.1016/j.ecolmodel.2016.01.001
Guerrero, R., and Berlanga, M. (2016). From the cell to the ecosystem: the physiological evolution of symbiosis. Evol. Biol. 43, 543–552. doi: 10.1007/s11692-015-9360-5
Gurney, W., Ross, A. H., and Broekhuisen, N. (1994). “Coupling the dynamics of species and materials,” in Linking Species and Ecosystems, eds J. Lawton and C. G. Jones (Berlin: Springer-Verlag), 176–193. doi: 10.1007/978-1-4615-1773-3_18
Håkanson, L. (1997). Modelling of radiocesium in lakes-on predictive power and lessons for the future. Stud. Environ. Sci. 68,3–45. doi: 10.1016/S0166-1116(09)70081-0
Halpern, B. S., Frazier, M., Potapenko, J., Casey, K. S., Koenig, K., Longo, C., et al. (2015). Spatial and temporal changes in cumulative human impacts on the world's ocean. Nat. Commun. 6:7615. doi: 10.1038/ncomms8615
Harfoot, M. B., Newbold, T., Tittensor, D. P., Emmott, S., Hutton, J., Lyutsarev, V., et al. (2014). Emergent global patterns of ecosystem structure and function from a mechanistic general ecosystem model. PLoS Biol. 12:e1001841. doi: 10.1371/journal.pbio.1001841
Hartmann, D. L., Klein Tank, A. M. G., Rusticucci, M., Alexander, L. V., Brönnimann, S., Charabi, Y., et al. (2013). “Observations: atmosphere and surface,” in Climate Change 2013: The Physical Science Basis, Contribution of Working Group I to the Fifth Assessment Report of the Intergovernmental Panel on Climate Change, eds T. F. Stocker, D. Qin, G.-K. Plattner, M. Tignor, S. K. Allen, J. Boschung, A. Nauels, Y. Xia, V. Bex, and P. M. Midgley (Cambridge, UK; New York, NY: Cambridge University Press), 159–254.
Hastings, A., Abbott, K. C., Cuddington, K., Francis, T., Gellner, G., Lai, Y.-C., et al. (2018). Transient phenomena in ecology. Science 361:eaat6412. doi: 10.1126/science.aat6412
Hobbs, R. J., Higgs, E., and Harris, J. A. (2009). Novel ecosystems: implications for conservation and restoration. Trends Ecol. Evol. 24, 599–605. doi: 10.1016/j.tree.2009.05.012
Hobson, K. A., Barnett-Johnson, R., and Cerling, T. (2010). “Using isoscapes to track animal migration,” in Isoscapes: Understanding Movement, Pattern, and Process on Earth Through Isotope Mapping, eds J. B. West, G. J. Bowen, T. E. Dawson, K. P. Tu (Dordrecht: Springer), 273–297. doi: 10.1007/978-90-481-3354-3_13
Holsman, K., Samhouri, J., Cook, G., Hazen, E., Olsen, E., Dillard, M., et al. (2017). An ecosystem-based approach to marine risk assessment. Ecosyst. Health Sustain. 3:e01256. doi: 10.1002/ehs2.1256
Hubbell, S. (2001). The Unified Neutral Theory of Biodiversity and Biogeography (MPB-32). Princeton, NJ: Princeton University Press.
Hughes, T. P., Rodrigues, M. J., Bellwood, D. R., Ceccarelli, D., Hoegh-Guldberg, O., McCook, L., et al. (2007). Phase shifts, herbivory, and the resilience of coral reefs to climate change. Curr. Biol. 17, 360–365. doi: 10.1016/j.cub.2006.12.049
Iglesias, V., Yospin, G., and Whitlock, C. (2015). Reconstruction of fire regimes through integrated paleoecological proxy data and ecological modeling. Front. Plant Sci. 5:785. doi: 10.3389/fpls.2014.00785
Jackson, H. B., and Fahrig, L. (2015). Are ecologists conducting research at the optimal scale? Global Ecol. Biogeogr. 24, 52–63. doi: 10.1111/geb.12233
Jephcott, T. G., Sime-Ngando, T., Gleason, F. H., and Macarthur, D. J. (2016). Host-parasite interactions in food webs: diversity, stability, and coevolution. Food Webs 6, 1–8. doi: 10.1016/j.fooweb.2015.12.001
Jones, A. R., Doubleday, Z. A., Prowse, T. A. A., Wiltshire, K. H., Deveney, M. R., Ward, T., et al. (2018). Capturing expert uncertainty in spatial cumulative impact assessments. Sci. Rep. 8:1469. doi: 10.1038/s41598-018-19354-6
Jordán, F., Liu, W.-C., and Davis, A. J. (2006). Topological keystone species: measures of positional importance in food webs. Oikos 112, 535–546. doi: 10.1111/j.0030-1299.2006.13724.x
Jørgensen, S. E. (2012). Structurally dynamic models of lakes. Int. J. Design Nat. Ecodyn. 7, 117–139. doi: 10.2495/DNE-V7-N2-117-139
Jørgensen, S. E., Nielsen, S. N., and Fath, B. D. (2016). Recent progress in systems ecology. Ecol. Model. 319, 112–118. doi: 10.1016/j.ecolmodel.2015.08.007
Katija, K., and Dabiri, J. O. (2009). A viscosity-enhanced mechanism for biogenic cean mixing. Nature 460, 624–627. doi: 10.1038/nature08207
Kavanaugh, M. T., Oliver, M. J., Chavez, F. P., Letelier, R. M., Muller-Karger, F. E., and Doney, S. C. (2016). Seascapes as a new vernacular for pelagic ocean monitoring, management and conservation. ICES J. Mar. Sci. 73, 1839–1850. doi: 10.1093/icesjms/fsw086
Kays, R., Crofoot, M. C., Jetz, W., and Wikelski, M. (2015). Terrestrial animal tracking as an eye on life and planet. Science 348:aaa2478. doi: 10.1126/science.aaa2478
Kiørboe, T., Visser, A., and Andersen, K. H. (2018). A trait-based approach to ocean ecology. ICES J. Mar. Sci. 75, 1849–1863. doi: 10.1093/icesjms/fsy090
Klein, K., Sommer, B., Nim, H. T., Flack, A., Safi, K., Nagy, M., et al. (2019). Fly with the flock: immersive solutions for animal movement visualization and analytics. J. R. Soc. Interface 16:20180794. doi: 10.1098/rsif.2018.0794
Kondoh, M., Mougi, A., Ushimaru, A., and Nakata, K. (2016). Adaptive movement and food-chain dynamics: towards food-web theory without birth-death processes. Theor. Ecol. 9, 15–25. doi: 10.1007/s12080-015-0266-8
Köster, F. W., and Möllmann, C. (2000). Trophodynamic control by cupeid predators on recruitment success in Baltic cod? ICES J. Mar. Sci. 57, 310–323. doi: 10.1006/jmsc.1999.0528
Kramer-Schadt, S., Revilla, E., Wiegand, T., and Grimm, V. (2007). Patterns for parameters in simulation models. Ecol. Model. 204, 553–556. doi: 10.1016/j.ecolmodel.2007.01.018
Kuppel, S., Peylin, P., Maignan, F., Chevallier, F., Kiely, G., Montagnani, L., et al. (2014). Model-data fusion across ecosystems: from multisite optimizations to global simulations. Geosci. Model Dev. 7, 2581–2597. doi: 10.5194/gmd-7-2581-2014
Laland, K. N., Uller, T., Feldman, M. W., Sterelny, K., Müller, G. B., Moczek, A., et al. (2015). The extended evolutionary synthesis: its structure, assumptions and predictions. Proc. R. Soc. B 282, 20151019. doi: 10.1098/rspb.2015.1019
Levin, S. A. (1992). The problem of pattern and scale in ecology. Ecology 73, 1943–1967. doi: 10.2307/1941447
Levin, S. A. (1998). Ecosystems and the biosphere as complex adaptive systems. Ecosystems 1, 431–436. doi: 10.1007/s100219900037
Levin, S. A., Xepapadeas, T., Crépin, A.-S., Norberg, J., De Zeeuw, A., Folke, C., et al. (2013). Social-ecological systems as complex adaptive systems: modelling and policy implications. Environ. Dev. Econ. 18, 111–132. doi: 10.1017/S1355770X12000460
Loreau, M. (2010a). Linking biodiversity and ecosystems: towards a unifying ecological theory. Philos. Trans. R. Soc. B. 365, 49–60. doi: 10.1098/rstb.2009.0155
Loreau, M. (2010b). From Populations to Ecosystems Theoretical Foundations for a New Ecological Synthesis. Princeton, NJ: Princeton University Press. doi: 10.1515/9781400834167
Lotka, A. J. (1932). The growth of mixed populations: two species competing for a common food supply. J. Washington Acad. Sci. 22, 461–469.
Lotze, H. K., Tittensor, D. P., Bryndum-Buchholz, A., Eddy, T. D., Cheung, W. W. L., Galbraith, E. D., et al. (2019). Global ensemble projections reveal trophic amplification of ocean biomass declines with climate change. Proc. Nat. Acad. Sci. U.S.A. 116, 12907–12912. doi: 10.1073/pnas.1900194116
Lowerre-Barbieri, S. K., Kays, R., Thorson, J. T., and Wikelski, M. (2019). The ocean's movescape: fisheries management in the bio-logging decade (2018-2028). ICES J. Mar. Sci. 76, 477–488. doi: 10.1093/icesjms/fsy211
Lowery, C. M., Bralower, T. J., Owens, J. D., Rodríguez-Tovar, F. J., Jones, H., Smit, J., et al. (2018). Rapid recovery of life at ground zero of the end-Cretaceous mass extinction. Nature 558, 288–292. doi: 10.1038/s41586-018-0163-6
Ludwig, D., and Walters, C. J. (1985). Are age-structured models appropriate for catch-effort data? Can. J. Fish. Aquat. Sci. 42, 1066–1072. doi: 10.1139/f85-132
Lynam, C. P., Llope, M., Möllmann, C., Helaouët, P., Bayliss-Brown, G. A., and Stenseth, N. C. (2017). Interaction between top-down and bottom-up control in marine food webs. Proc. Natl. Acad. Sci. U.S.A. 114, 1952–1957. doi: 10.1073/pnas.1621037114
MacArthur, R. H., and Wilson, E. (1967). The Theory of Island Biogeography. Princeton, NJ: Princeton University Press.
Mackay, A., Jones, V. J., and Battarbee, R. W. (2003). “Approaches to holocene climate reconstruction using diatoms,” in Global Change in the Holocene, eds A. Mackay, R. W. Battarbee, H. J. B. Birks, and F. Oldfield (London: Arnold), 294–309.
Mackey, A. P., Atkinson, A., Hill, S. L., Ward, P., Cunningham, N. J., Johnston, N. M., et al. (2012). Antarctic macrozooplankton of the southwest Atlantic sector and Bellingshausen Sea Baseline historical distributions (Discovery Investigations, 1928-1935) related to temperature and food, with projections for subsequent ocean warming. Deep-Sea Res. II 59–60, 130–146. doi: 10.1016/j.dsr2.2011.08.011
Malin, G., Turner, S. M., and Liss, P. S. (1992). Sulfur: the plankton/climate connection. J. Phycol. 28, 590–597. doi: 10.1111/j.0022-3646.1992.00590.x
Mardones, F. O., Perez, A. M., Valdes-Donoso, P., and Carpenter, T. E. (2011). Farm-level reproduction number during an epidemic of infectious salmon anemia virus in southern Chile in 2007-2009. Prevent. Vet. Med. 102, 175–184. doi: 10.1016/j.prevetmed.2011.07.005
Marquet, P. A., Allen, A. P., Brown, J. H., Dunne, J. A., Enquist, B. J., Gillooly, J. F., et al. (2015). On theory in ecology. Bioscience 64, 701–710. doi: 10.1093/biosci/biu098
Marquet, P. A., Fortin, M. J., Pineda, J., Wallin, D. O., Clark, J., Wu, Y., et al. (1993). “Ecological and evolutionary consequences of patchiness: a marine-terrestrial perspective,” in Patch Dynamics, Vol. 96, eds S. A. Levin, T. M. Powell, and J.W. Steele (Berlin, Heidelberg: Springer) doi: 10.1007/978-3-642-50155-5_19
Martin, A. E. (2018). The spatial scale of a species' response to the landscape context depends on which biological response you measure. Curr. Land. Ecol. Rep. 3, 23–33. doi: 10.1007/s40823-018-0030-z
Martorell, C., and Freckleton, R. P. (2014). Testing the roles of competition, facilitation and stochasticity on community structure in a species-rich assemblage. J. Ecol. 102, 74–85. doi: 10.1111/1365-2745.12173
Marzloff, M. P., Little, L. R., and Johnson, C. R. (2016a). Building resilience against climate-driven shifts in a temperate reef system: staying away from context-dependent ecological thresholds. Ecosystems 19, 1–15. doi: 10.1007/s10021-015-9913-6
Marzloff, M. P., Melbourne-Thomas, J., Hamon, K. G., Hoshino, E., Jennings, S., van Putten, I. E., et al. (2016b). Modelling marine community responses to climate-driven species redistribution to guide monitoring and adaptive ecosystem-based management. Glob. Change Biol. 22, 2462–2474. doi: 10.1111/gcb.13285
Massol, F., Altermatt, F., Gounand, I., Gravel, D., Leibold, M. A., and Mouquet, N. (2016). How life-history traits affect ecosystem properties: effects of dispersal in meta-ecosystems. Oikos 126, 532–546. doi: 10.1111/oik.03893
McCann, R. K., Marcot, B. G., and Ellis, R. (2006). Bayesian belief networks: applications in ecology and natural resource management. Can. J. For. Res. 36, 3053–3062. doi: 10.1139/x06-238
McCormack, S. A., Trebilco, R., Melbourne-Thomas, J., Blanchard, J. L., Fulton, E. A., and Constable, A. (2019). Using stable isotope data to advance marine food web modelling. Rev. Fish Biol. Fish 29, 277–296. doi: 10.1007/s11160-019-09552-4
McDonald, A. D., Little, L. R., Gray, R., Fulton, E., Sainsbury, K. J., and Lyne, V. D. (2008). An agent-based modelling approach to evaluation of multiple-use management strategies for coastal marine ecosystems. Math. Comput. Sim. 78, 401–411. doi: 10.1016/j.matcom.2008.01.039
McDonald, K. S., Hobday, A. J., Fulton, E. A., and Thompson, P. A. (2018). Interdisciplinary knowledge exchange across scales in a globally changing marine environment. Glob Change Biol. 24, 3039–3054. doi: 10.1111/gcb.14168
McGill, B., and Collins, C. (2003). A unified theory for macroecology based on spatial patterns of abundance. Evol. Ecol. Res. 5, 469–492.
McGill, B. J. (2010). Towards a unification of unified theories of biodiversity. Ecol. Lett. 13, 627–642. doi: 10.1111/j.1461-0248.2010.01449.x
McGill, B. J., Enquist, B. J., Weiher, E., and Westoby, M. (2006). Rebuilding community ecology from functional traits. Trends Ecol. Evol. 21, 178–185. doi: 10.1016/j.tree.2006.02.002
Medlyn, B. E., De Kauwe, M. G., Zaehle, S., Walker, A. P., Duursma, R. A., Luus, K., et al. (2016). Using models to guide field experiments: a priori predictions for the CO2 response of a nutrient- and water-limited native Eucalypt woodland. Glob. Change Biol. 22, 2834–2851. doi: 10.1111/gcb.13268
Médoc, V., Firmat, C., Sheath, D. J., Pegg, J., Andreou, D., and Britton, J. R. (2017). Parasites and biological invasions: predicting ecological alterations at levels from individual hosts to whole networks. Adv. Ecol. Res. 57, 1–54. doi: 10.1016/bs.aecr.2016.10.003
Melbourne-Thomas, J., Constable, A. J., Fulton, E. A., Corney, S. P., Trebilco, R., Hobday, A. J., et al. (2017). Integrated modelling to support decision-making for marine social-ecological systems in Australia. ICES J. Mar. Sci. 74, 2298–2308. doi: 10.1093/icesjms/fsx078
Melbourne-Thomas, J., Johnson, C. R., Fung, T., Seymour, R. M., Chérubin, L. M., Arias-González, J. E., et al. (2011). Regional-scale scenario modeling for coral reefs: a decision support tool to inform management of a complex system. Ecol. Appl. 21, 1380–1398. doi: 10.1890/09-1564.1
Milner-Gulland, E. J., Arroyo, B., Bellard, C., Blanchard, J., Bunnefeld, N., Delibes-Mateos, M., et al. (2010). New directions in management strategy evaluation through crossfertilization between fisheries science and terrestrial conservation. Biol. Lett. 6, 719–722. doi: 10.1098/rsbl.2010.0588
Mischler, J., Johnson, P. T. J., McKenzie, V. J., and Townsend, A. R. (2016). Parasite infection alters nitrogen cycling at the ecosystem scale. J. Anim. Ecol. 85, 817–828. doi: 10.1111/1365-2656.12505
Mitchell, J. S., Roopnarine, P. D., and Angielczyk, K. D. (2012). Late Cretaceous restructuring of terrestrial communities facilitated the end-Cretaceous mass extinction in North America. Proc. Natl. Acad. Sci. U.S.A. 109, 18857–18861. doi: 10.1073/pnas.1202196109
Mokany, K., and Ferrier, S. (2011). Predicting impacts of climate change on biodiversity: a role for semi-mechanistic community-level modelling. Divers. Distrib. 17, 374–380. doi: 10.1111/j.1472-4642.2010.00735.x
Mokany, K., Ferrier, S., Connolly, S. R., Dunstan, P. K., Fulton, E. A., Harfoot, M. B., et al. (2016). Integrating modelling of biodiversity composition and ecosystem function. Oikos 125, 10–19. doi: 10.1111/oik.02792
Monroe, J. G., Markman, D. W., Beck, W. S., Felton, A. J., Vahsen, M. L., and Pressler, Y. (2018). Ecoevolutionary dynamics of carbon cycling in the Anthropocene. Trends Ecol. Evol. 33, 213–225. doi: 10.1016/j.tree.2017.12.006
Moorcroft, P. R., Hurtt, G. C, and Pacala, S. W. (2001). A method for scaling vegetation dynamics: the ecosystem demography model. Ecol. Monogr. 71, 557–585. doi: 10.1890/0012-9615(2001)071<0557:AMFSVD>2.0.CO;2
Moran, E. V., Hartig, F., and Bell, D. M. (2006). Intraspecific trait variation across scales: implications for understanding global change responses. Glob. Change Biol. 22, 137–150. doi: 10.1111/gcb.13000
Mouquet, N., Lagadeuc, Y., Devictor, V., Doyen, L., Duputié, A., Eveillard, D., et al. (2015). Predictive ecology in a changing world. J. App. Ecol. 52, 1293–1310. doi: 10.1111/1365-2664.12482
Münier, B., Birr-Pedersen, K., and Schou, J. S. (2004). Combined ecological and economic modelling in agricultural land use scenarios. Ecol. Model. 174, 5–18. doi: 10.1016/j.ecolmodel.2003.12.040
Nash, K. L., Graham, N. A. J., Jennings, S., Wilson, S. K., and Bellwood, D. R. (2015). Herbivore cross-scale redundancy supports response diversity and promotes coral reef resilience. J. Appl. Ecol. 53, 646–655. doi: 10.1111/1365-2664.12430
Nicol, S., Bowie, A., Jarman, S., Lannuzel, D., Meiners, K. M., and Van Der Merwe, P. (2010). Southern Ocean iron fertilization by baleen whales and Antarctic krill. Fish Fish. 11, 203–209. doi: 10.1111/j.1467-2979.2010.00356.x
Noble, D. (2003). The future: putting Humpty-Dumpty together again. Biochem. Soc. Trans. 31, 156–158. doi: 10.1042/bst0310156
Ogle, K. (2009). Hierarchical bayesian statistics: merging experimental and modeling approaches in ecology. Ecol. Appl. 19, 577–581. doi: 10.1890/08-0560.1
Olsen, E., Fay, G., Gaichas, S., Gamble, R., Lucey, S., and Link, J. S. (2016). Ecosystem model skill assessment. Yes we can! PLoS ONE 11:e0146467. doi: 10.1371/journal.pone.0146467
Österblom, H., Merrie, A., Metian, M., Boonstra, W. J., Blenckner, T., Watson, J. R., Folke, C., et al. (2013). Modeling social-ecological scenarios in marine systems. Bioscience 63, 735–744. doi: 10.1093/bioscience/63.9.735
Pacala, S. W. (1994). “An ecologist's encounter with some models in the social sciences,” in Integrated Regional Models, eds P. M. Groffman and G. Likens (London: Chapman & Hall), 35–49. doi: 10.1007/978-1-4684-6447-4_3
Paine, R. T. (1969). A note on trophic complexity and community stability. Am. Nat. 103, 91–93. doi: 10.1086/282586
Palmer, M. A., Ambrose, R. F., and Poff, N. L. (2008). Ecological theory and community restoration ecology. Restor. Ecol. 5, 291–300. doi: 10.1046/j.1526-100X.1997.00543.x
Pantus, F. J. (2006). Sensitivity analysis for complex ecosystem models (Ph.D. thesis). Brisbane, QLD: University of Queensland.
Parker, D. C., Hessl, A., and Davis, S. C. (2008). Complexity, land-use modeling, and the human dimension: Fundamental challenges for mapping unknown outcome spaces. Geoforum 39, 789–804. doi: 10.1016/j.geoforum.2007.05.005
Parrott, L. (2011). Hybrid modelling of complex ecological systems for decision support: recent successes and future perspectives. Ecol. Inform. 6, 44–49. doi: 10.1016/j.ecoinf.2010.07.001
Pastor, J., Cohen, Y., and Hobbs, N. (2006). “The roles of large herbivores in ecosystem nutrient cycles,” in Large Herbivore Ecology, Ecosystem Dynamics and Conservation, eds K. Danell, R. Bergström, P. Duncan, and J. Pastor (Cambridge: Cambridge University Press), 289–325. doi: 10.1017/CBO9780511617461.012
Pelletier, F., Garant, D., and Hendry, A. P. (2009). Eco-evolutionary dynamics. Philos. Trans. R. Soc. Lond. B. 364, 1483–1489. doi: 10.1098/rstb.2009.0027
Peterson, G., Allen, C., and Holling, C. (1998). Ecological eesilience, biodiversity, and scale. Ecosystems 1:6. doi: 10.1007/s100219900002
Pethybridge, H. R., Choy, C. A., Polovina, J. J., and Fulton, E. A. (2018). Improving marine ecosystem models with biochemical tracers. Annu. Rev. Mar. Sci. 10, 199–228. doi: 10.1146/annurev-marine-121916-063256
Pickett, S. T. A., Collins, S. L., and Armesto, J. J. (1987). Models, mechanisms and pathways of succession. Bot. Rev. 53, 335–371. doi: 10.1007/BF02858321
Pilosof, S., Porter, M. A., Pascual, M., and Kéfi, S. (2017). The multilayer nature of ecological networks. Nat. Ecol. Evol. 1, 1–9. doi: 10.1038/s41559-017-0101
Pimiento, C., Griffin, J. N., Clements, C. F., Silvestro, D., Varela, S., Uhen, M. D., et al. (2017). The Pliocene marine megafauna extinction and its impact on functional diversity. Nat. Ecol. Evol, 1, 1100–1106. doi: 10.1038/s41559-017-0223-6
Pinksy, M., Reygondeau, G., Caddell, R., Palacios-Abrantes, J., Spijkers, J., and Cheung, W. (2018). Preparing ocean governance for species on the move. Science 360, 1189–1191. doi: 10.1126/science.aat2360
Pitcher, C. R., Rochester, W., Dunning, M., Courtney, T., Broadhurst, M., Noell, C., et al. (2018). Putting Potential Environmental Risk of Australia's Trawl Fisheries In Landscape Perspective: Exposure of Seabed Assemblages to Trawling, and Inclusion in Closures and Reserves. CSIRO Oceans & Atmosphere, Brisbane, QLD.
Plagányi, É. E., Deng, R., Campbell, R., Dennis, D., Hutton, T., Haywood, M., et al. (2018). Evaluating an empirical harvest control rule for the Torres Strait Panulirus ornatus tropical rock lobster fishery. Bull. Mar. Sci. 94:1095–1120. doi: 10.5343/bms.2017.1101
Plagányi, É. E., Punt, A. E., Hillary, R., Morello, E. B., Thébaud, O., Hutton, T., et al. (2014). Multispecies fisheries management and conservation: tactical applications using models of intermediate complexity. Fish. Fish. 15, 1–22. doi: 10.1111/j.1467-2979.2012.00488.x
Polishchuk, L. V., and Blanchard, J. L. (2019). Uniting discoveries of abundance-size distributions from soils and seas. Trends Ecol. Evol. 34, 2–5. doi: 10.1016/j.tree.2018.10.007
Preston, D. L., Mischler, J. A., Townsend, A. R., and Johnson, P. T. J. (2016). Disease ecology meets ecosystem science. Ecosystems 19, 737–748. doi: 10.1007/s10021-016-9965-2
Prinn, R. G. (2012). Development and application of earth system models. Proc. Natl. Acad. Sci. U.S.A. 110, 3673–3680. doi: 10.1073/pnas.1107470109
Pulliam, H. R. (1988). Sources, sinks, and population regulation. Am. Nat. 132, 652–661. doi: 10.1086/284880
Punt, A. E., MacCall, A. D., Essington, T. E., Francis, T. B., Hurtado-Ferro, F., Johnson, K. F., et al. (2016). Exploring the implications of the harvest control rule for Pacificsardine, accounting for predator dynamics: a MICE model. Ecol. Model. 337, 79–95. doi: 10.1016/j.ecolmodel.2016.06.004
Quillfeldt, P., Masello, J. F., McGill, R. A. R., Adams, M., and Furness, R. W. (2010). Moving polewards in winter: a recent change in the migratory strategy of a pelagic seabird? Front. Zool. 7:15. doi: 10.1186/1742-9994-7-15
Raes, E. J., Bodrossy, L., van de Kamp, J., Bissett, A., Ostrowski, M., Brown, M. V., et al. (2018). Oceanographic boundaries constrain microbial diversity gradients in the South Pacific Ocean. Proc. Natl. Acad. Sci. U.S.A. 115, E8266–E8275. doi: 10.1073/pnas.1719335115
Rastetter, E. B., Ryan, M. G., Shaver, G. R., Melillo, J. M., Nadelhoffer, K. J., Hobbie, J. E., et al. (1991). A general biogeochemical model describing the responses of the C and N cycles in terrestrial ecosystems to changes in CO2, climate, and N deposition. Tree Phys. 9, 101–126. doi: 10.1093/treephys/9.1-2.101
Ratnarajah, L., Melbourne-Thomas, J., Marzloff, M. P., Lannuzel, D., Meiners, K. M., Chever, F., et al. (2016). A preliminary model of iron fertilisation by baleen whales and Antarctic krill in the Southern Ocean: sensitivity of primary productivity estimates to parameter uncertainty. Ecol. Model. 320, 203–212. doi: 10.1016/j.ecolmodel.2015.10.007
Reid, P. C., Battle, E. J. V., Batten, S. D., and Brander, K. M. (2000). Impacts of fisheries on plankton community structure. ICES J. Mar. Sci. 57, 495–502. doi: 10.1006/jmsc.2000.0740
Reum, J. C. P., Blanchard, J. L., Holsman, K. K., Aydin, K., and Punt, A. E. (2019). Species-specific ontogenetic diet shifts attenuate trophic cascades and lengthen food chains in exploited ecosystems. Oikos 128, 1051–1064. doi: 10.1111/oik.05630
Riechert, S. E., and Hammerstein, P. (1983). Game theory in the ecological context. Ann. Rev. Ecol. Syst. 14, 377–409. doi: 10.1146/annurev.es.14.110183.002113
Rogers, J., Babcock, R., and Plagányi, É. (2017). Aggregation, Allee effects and critical thresholds for the management of the crown of thorns starfish Acanthaster planci. Mar. Ecol. Prog. Ser. 578, 99–114. doi: 10.3354/meps12252
Romero-Mujalli, D., Jeltsch, F., and Tiedemann, R. (2019). Individual-based modeling of eco-evolutionary dynamics: state of the art and future directions. Reg. Environ. Change 19, 1–12. doi: 10.1007/s10113-018-1406-7
Roopnarine, P. D., and Angielczyk, K. D. (2015). Community stability and selective extinction during the Permian-Triassic mass extinction. Science 350, 90–93. doi: 10.1126/science.aab1371
Rose, K. C., Graves, R. A., Hansen, W. D., Harvey, B. J., Qiu, J., Wood, S. A., et al. (2017). Historical foundations and future directions in macrosystems ecology. Ecol. Lett. 20, 147–157. doi: 10.1111/ele.12717
Russell, E. S. (1931). Some theoretical considerations on the ‘overfishing’ problem. ICES J. Mar. Sci. 6, 1–20. doi: 10.1093/icesjms/6.1.3
Ryu, T., Veilleux, H. D., Donelso, J. M., Munday, P. L., and Ravasi, T. (2018). The epigenetic landscape of transgenerational acclimation to ocean warming. Nat. Clim. Change 8, 504–509. doi: 10.1038/s41558-018-0159-0
Sale, P. F., Hanski, I., and Kritzer, J. P. (2016). “The merging of metapopulation theory and marine ecology: establishing the historical context,” in Marine Metapopulations, eds J. P. Kritzer and P. F. Sale (New York, NY: Elsevier), 3–28. doi: 10.1016/B978-012088781-1/50004-2
Scheffer, M., and Beets, J. (1994). Ecological models and the pitfalls of causality. Hydrobiologia 275, 115–124. doi: 10.1007/BF00026704
Scheffer, M., and van Nes, E. H. (2006). Self-organized similarity, the evolutionary emergence of groups of similar species. Proc. Natl. Acad. Sci. U.S.A. 103, 6230–6235. doi: 10.1073/pnas.0508024103
Scheiner, S. M., and Willig, M. R. (2005). Developing unified theories in ecology as exemplified with diversity gradients. Am. Nat. 166, 458–469. doi: 10.1086/444402
Scheiner, S. M., and Willig, M. R. (2008). A general theory of ecology. Theor. Ecol. 1, 21–28. doi: 10.1007/s12080-007-0002-0
Scheiter, S., Langan, L., and Higgins, S. I. (2013). Next-generation dynamic global vegetation models: learning from community ecology. N. Phytol. 198, 957–969. doi: 10.1111/nph.12210
Schmitz, O. J., Hawlena, D., and Trussell, G. C. (2010). Predator control of ecosystem nutrient dynamics. Ecol. Lett. 13, 1199–1209. doi: 10.1111/j.1461-0248.2010.01511.x
Schmitz, O. J., Wilmers, C. C., Leroux, S. J., Doughty, C. E., Atwood, T. B., Galetti, M., et al. (2018). Animals and the zoogeochemistry of the carbon cycle. Science 362:eaar3213. doi: 10.1126/science.aar3213
Scholes, R. J., Reyers, B., Biggs, R., Spierenburg, M. J., and Duriappah, A. (2013). Multi-scale and cross-scale assessments of social-ecological systems and their ecosystem services. Curr. Opin. Environ. Sustain. 5:16–25. doi: 10.1016/j.cosust.2013.01.004
Sheldon, R. W., Prakash, A., and Sutcliffe, W. H. Jr. (1972). The size distribution of particles in the ocean. Limnol. Oceanogr. 17, 327–340. doi: 10.4319/lo.1972.17.3.0327
Shin, Y.-J., Houle, J. E., Akoglue, E., Blanchard, J. L., Bundy, A., Coll, M., et al. (2018). The specificity of marine ecological indicators to fishing in the face of environmental change: a multi-model evaluation. Ecol. Ind. 89, 317–326. doi: 10.1016/j.ecolind.2018.01.010
Shin, Y.-J., Travers, M., and Maury, O. (2010). Coupling low and high trophic levels models: towards a pathways-orientated approach for end-to-end models. Prog. Oceanogr. 84, 105–112. doi: 10.1016/j.pocean.2009.09.012
Smallegange, I. M., Caswell, H., Toorians, M. E. M., and de Roos, A. M. (2017). Mechanistic description of population dynamics using dynamic energy budget theory incorporated into integral projection models. Methods Ecol. Evol. 8, 146–154. doi: 10.1111/2041-210X.12675
Smith, P. E. (1978). Biological effects of ocean variability: time and space scales of biological response. J. Cons. Int. Mer. 173, 117–127.
Soliveres, S., van der Plas, F., Manning, P., Prati, D., Gossner, M. M., Renner, S. C.E., et al. (2016). Biodiversity at multiple trophic levels is needed for ecosystem multifunctionality. Nature 536, 456–459. doi: 10.1038/nature19092
Spence, M. A., Blanchard, J. L., Rossberg, A. G., Heath, M. R., Heymans, J. J., Mackinson, S., et al. (2018). A general framework for combining ecosystem models. Fish. Fish. 19, 1031–1042. doi: 10.1111/faf.12310
Steele, J. H. (1978). “Some comments on plankton patches,” in Spatial Pattern in Plankton Communities, ed J. H. Steele (New York, NY: Plenum Press), 11–20. doi: 10.1007/978-1-4899-2195-6_1
Steele, J. H. (1985). A comparison of terrestrial and marine ecological systems. Nature 313, 355–358. doi: 10.1038/313355a0
Steffen, W., Richardson, K., Rockström, J., Cornell, S. E., Fetzer, I., Bennett, E. M., et al. (2015). Planetary boundaries: Guiding human development on a changing planet. Science 347:1259855. doi: 10.1126/science.1259855
Stelzenmüller, V., Coll, M., Mazaris, A. D., Giakoumi, S., Katsanevakis, S., Portman, M. E., et al. (2018). A risk-based approach to cumulative effect assessments for marine management. Sci. Tot. Environ. 612, 1132–1140. doi: 10.1016/j.scitotenv.2017.08.289
Stewart-Koster, B., Boone, E. L., Kennard, M. J., Sheldon, F., Bunn, S. E., and Olden, J. D. (2013). Incorporating ecological principles into statistical models for the prediction of species' distribution and abundance. Ecography 36, 342–353. doi: 10.1111/j.1600-0587.2012.07764.x
Stommel, H. (1963). Varieties of oceanographic experience: The ocean can be investigated as a hydrodynamical phenmonen as well as explored geographically. Science 139, 572–576. doi: 10.1126/science.139.3555.572
Suárez-Castro, A. F., Simmonds, J. S., Mitchell, M. G. E., Maron, M., and Rhodes, J. R. (2018). The scale-dependent role of biological traits in landscape ecology: a review. Curr. Land. Ecol. Rep. 3, 12–22. doi: 10.1007/s40823-018-0031-y
Sugihara, G., May, R., Ye, H., Hsieh, C. H., Deyle, E., Fogarty, M., et al. (2012). Detecting causality in complex ecosystems. Science 338, 496–500. doi: 10.1126/science.1227079
Supp, S. R., Xiao, X., Ernest, S. K. M., and White, E. P. (2012). An experimental test of the response of macroecological patterns to altered species interactions. Ecology 93, 2505–2511. doi: 10.1890/12-0370.1
Tarling, G. A., Ward, P., and Thorpe, S. E. (2017). Spatial distributions of Southern Ocean mesozooplankton communities have been resilient to long-term surface warming. Glob. Change Biol. 34, 117–111. doi: 10.1111/gcb.13834
Thébault, E., and Fontaine, C. (2010). Stability of ecological communities and the architecture of mutualistic and trophic networks. Science 329, 853–856. doi: 10.1126/science.1188321
Thuiller, W., Münkemüller, T., Lavergne, S., Mouillot, D., Mouquet, N., Schiffers, K., et al. (2013). A road map for integrating eco-evolutionary processes into biodiversity models. Ecol. Lett. 16, 94–105. doi: 10.1111/ele.12104
Tilman, D. (1977). Resource competition between plankton algae: an experimental and theoretical approach. Ecology 58, 338–348. doi: 10.2307/1935608
Trivedi, P., Delgado-Baquerizo, M., Trivedi, C., Hamonts, K., Anderson, I. C., and Singh, B. K. (2017). Keystone microbial taxa regulate the invasion of a fungal pathogen in agro-ecosystems. Soil Biol. Biochem. 111, 10–14. doi: 10.1016/j.soilbio.2017.03.013
Tulloch, A. I. T., Chadès, I., and Lindenmayer, D. B. (2018). Species co-occurrence analysis predicts management outcomes for multiple threats. Nat. Ecol. Evol. 2, 465–474. doi: 10.1038/s41559-017-0457-3
Tulloch, V. J. D., Plagányi, E., Brown, C., Matear, R., and Richardson, A. J. (2019). Future recovery of baleen whales is imperiled by climate change. Glob. Change Biol. 25, 1263–1281. doi: 10.1111/gcb.14573
Turchin, P. (1995). “Population regulation: old arguments and a new synthesis,” in Population Dynamics: New Approaches and Synthesis, ed N. Cappuccino and P. W. Price (San Diego, CA: Academic Press), 19–40. doi: 10.1016/B978-012159270-7/50003-8
Ushio, M., Hsieh, C.-,h., Masuda, R., Deyle, E. R., Ye, H., Chang, C.-W., et al. (2018). Fluctuating interaction network and time-varying stability of a natural fish community. Nature 554, 360–363. doi: 10.1038/nature25504
van Putten, I. E., Gorton, R. J., Fulton, E. A., and Thebaud, O. (2013). The role of behavioural flexibility in a whole of ecosystem model. ICES J. Mar. Sci. 70, 150–163. doi: 10.1093/icesjms/fss175
Vance, T. C., and Doel, R. E. (2010). Graphical methods and cold war scientific practice: The Stommel diagram's intriguing journey from the physical to the biological environmental sciences. Hist Stud. Nat. Sci. 40, 1–47. doi: 10.1525/hsns.2010.40.1.1
Vargas, C. A., Lagos, N. A., Lardies, M. A., Duarte, C., Manríquez, P. H., Aguilera, V. M., et al. (2017). Species-specific responses to ocean acidification should account for local adaptation and adaptive plasticity. Nat. Ecol. Evol. 1:0084. doi: 10.1038/s41559-017-0084
Voinov, A., Costanza, R., Wainger, L., Boumans, R., Villa, F., Maxwell, T., et al. (1999). Patuxent landscape model: integrated ecological economic modeling of a watershed. Environ. Model. Softw. 14, 473–491. doi: 10.1016/S1364-8152(98)00092-9
Volterra, V. (1926). “Variations and fluctuations of the numbers of individuals in animal species living together,” in Animal Ecology, ed R.N. Chapman (New York, NY: McGraw Hill) 31–113.
Walpole, J., Papin, J. A., and Peirce, S. M. (2013). Multiscale computational models of complex biological systems. Annu. Rev. Biomed. Eng. 15, 137–154. doi: 10.1146/annurev-bioeng-071811-150104
Warszawski, L., Frieler, K., Huber, V., Piontek, F., Serdeczny, O., and Schewe, J. (2014). The Inter-Sectoral Impact Model Intercomparison Project (ISI-MIP): Project framework. Proc. Natl. Acad. Sci. U.S.A. 111, 3228–3232. doi: 10.1073/pnas.1312330110
Watson, R. A., Mills, R., Buckley, C. L., Kouvaris, K., Jackson, A., Powers, S. T., et al. (2016). Evolutionary connectionism: algorithmic principles underlying the evolution of biological organisation in evo-devo, evo-eco and evolutionary transitions. Evol. Biol. 43, 553–581. doi: 10.1007/s11692-015-9358-z
Webb, T. J. (2012). Marine and terrestrial ecology: unifying concepts, revealing differences. Trends Ecol. Evol. 27, 535–541. doi: 10.1016/j.tree.2012.06.002
Weber, C. T., Borit, M., and Aschan, M. (2019). An interdisciplinary insight into the human dimension in fisheries models. A systematic literature review in a european union context. Front. Mar. Sci. 6:223. doi: 10.3389/fmars.2019.00369
Weiss-Lehman, C., Hufbauer, R. A., and Melbourne, B. A. (2016). Rapid trait evolution drives increased speed and variance in experimental range expansions. Nat. Commun. 8:14303. doi: 10.1038/ncomms14303
Weitz, J. S., Stock, C. A., Wilhelm, S. W., Bourouiba, L., Coleman, M. L., Buchan, A., et al. (2015). A multitrophic model to quantify the effects of marine viruses on microbial food webs and ecosystem processes. ISME J. 9, 1352–1364. doi: 10.1038/ismej.2014.220
Westley, F., Carpenter, S., Brock, W. A., Holling, C. S., and Gunderson, L. H. (2002). “Why systems of people and nature are not just social and ecological systems,” in Panarchy: Understanding Transformations in Human and Natural Systems, eds L. Gunderson and C. S. Holling (London: Island Press), 103–119.
Wirsing, A. J., and Ripple, W. J. (2011). A comparison of shark and wolf research reveals similar behavioral responses by prey. Front. Ecol. Environ. 9, 335–341. doi: 10.1890/090226
Yasuhara, M., Cronin, T. M., deMenocal, P. B., Okahashi, H., and Linsley, B. K. (2008). Abrupt climate change and collapse of deep-sea ecosystems. Proc. Natl. Acad. Sci. U.S.A. 105, 1556–1560. doi: 10.1073/pnas.0705486105
Ye, H., and Sugihara, G. (2016). Information leverage in interconnected ecosystems: overcoming the curse of dimensionality. Science 353, 922–925. doi: 10.1126/science.aag0863
Yea, H., Beamishb, R. J., Glaser, S. M., Grant, S. C. H., Hsieh, C., Richards, L. J., et al. (2015). Equation-free mechanistic ecosystem forecasting using empirical dynamic modelling. Proc. Natl. Acad. Sci. U.SA. 112, E1569–E1576. doi: 10.1073/pnas.1417063112
Yeakel, J. D., Guimarães, P. R. Jr., Bocherens, H., and Koch, P. L. (2013). The impact of climate change on the structure of Pleistocene food webs across the mammoth steppe. Proc. R. Soc. B 280:20130239. doi: 10.1098/rspb.2013.0239
Yeakel, J. D., Pires, M. M., Rudolfe, L., Dominy, N. G., Koch, P. L., Guimarães, P. R. Jr., and Gross, T. (2014). Collapse of an ecological network in Ancient Egypt. Proc. Natl. Acad. Sci. U.S.A. 111, 14472–14477. doi: 10.1073/pnas.1408471111
Yodzis, P., and Innes, S. (1992). Body size and consumer-resource dynamics. Am. Nat. 139, 1151–1175. doi: 10.1086/285380
Young, J. W., Hunt, B. P. V., Cook, T. R., Llopiz, J. K., Hazen, E. L., Pethybridge, H. R., et al. (2015). The trophodynamics of marine top predators: current knowledge, recent advances and challenges. Deep Sea Res. II 113, 170–187. doi: 10.1016/j.dsr2.2014.05.015
Yvon-Durocher, G., Reiss, J., Blanchard, J., Ebenman, B., Perkins, D. M., Reuman, D. C., et al. (2011). Across ecosystem comparisons of size structure: methods, approaches and prospects. Oikos 120, 550–563. doi: 10.1111/j.1600-0706.2010.18863.x
Keywords: ecology, scale, modeling, anthropocene, challenges
Citation: Fulton EA, Blanchard JL, Melbourne-Thomas J, Plagányi ÉE and Tulloch VJD (2019) Where the Ecological Gaps Remain, a Modelers' Perspective. Front. Ecol. Evol. 7:424. doi: 10.3389/fevo.2019.00424
Received: 11 February 2019; Accepted: 21 October 2019;
Published: 08 November 2019.
Edited by:
Diego Barneche, University of Exeter, United KingdomReviewed by:
Michael C. Dietze, Boston University, United StatesCopyright © 2019 Fulton, Blanchard, Melbourne-Thomas, Plagányi and Tulloch. This is an open-access article distributed under the terms of the Creative Commons Attribution License (CC BY). The use, distribution or reproduction in other forums is permitted, provided the original author(s) and the copyright owner(s) are credited and that the original publication in this journal is cited, in accordance with accepted academic practice. No use, distribution or reproduction is permitted which does not comply with these terms.
*Correspondence: Elizabeth A. Fulton, YmV0aC5mdWx0b25AY3Npcm8uYXU=
Disclaimer: All claims expressed in this article are solely those of the authors and do not necessarily represent those of their affiliated organizations, or those of the publisher, the editors and the reviewers. Any product that may be evaluated in this article or claim that may be made by its manufacturer is not guaranteed or endorsed by the publisher.
Research integrity at Frontiers
Learn more about the work of our research integrity team to safeguard the quality of each article we publish.