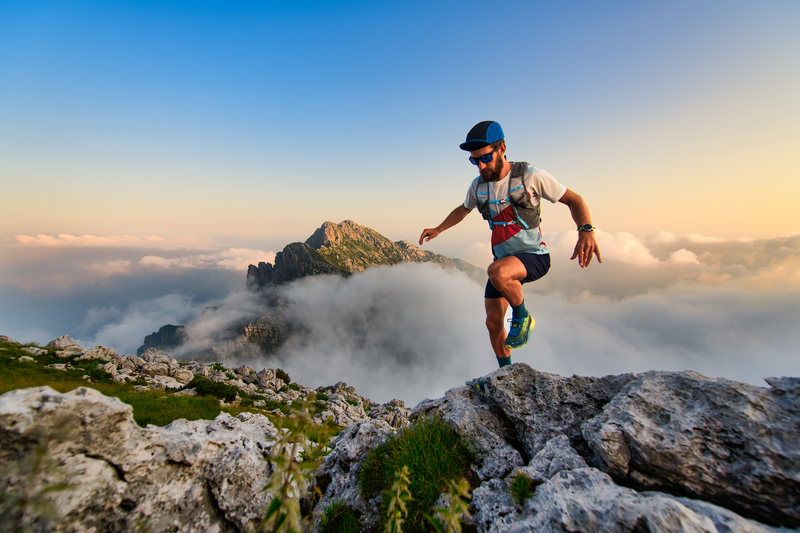
95% of researchers rate our articles as excellent or good
Learn more about the work of our research integrity team to safeguard the quality of each article we publish.
Find out more
ORIGINAL RESEARCH article
Front. Ecol. Evol. , 29 October 2019
Sec. Biogeography and Macroecology
Volume 7 - 2019 | https://doi.org/10.3389/fevo.2019.00407
This article is part of the Research Topic Operationalizing the Concepts of Resilience and Resistance for Managing Ecosystems and Species at Risk View all 13 articles
Screening is a strategy for detecting undesirable change prior to manifestation of symptoms or adverse effects. Although the well-recognized utility of screening makes it commonplace in medicine, it has yet to be implemented in ecosystem management. Ecosystem management is in an era of diagnosis and treatment of undesirable change, and as a result, remains more reactive than proactive and unable to effectively deal with today's plethora of non-stationary conditions. In this paper, we introduce spatial imaging-based screening to ecology. We link advancements in spatial resilience theory, data, and technological and computational capabilities and power to detect regime shifts (i.e., vegetation state transitions) that are known to be detrimental to human well-being and ecosystem service delivery. With a state-of-the-art landcover dataset and freely available, cloud-based, geospatial computing platform, we screen for spatial signals of the three most iconic vegetation transitions studied in western USA rangelands: (1) erosion and desertification; (2) woody encroachment; and (3) annual exotic grass invasion. For a series of locations that differ in ecological complexity and geographic extent, we answer the following questions: (1) Which regime shift is expected or of greatest concern? (2) Can we detect a signal associated with the expected regime shift? (3) If detected, is the signal transient or persistent over time? (4) If detected and persistent, is the transition signal stationary or non-stationary over time? (5) What other signals do we detect? Our approach reveals a powerful and flexible methodology, whereby professionals can use spatial imaging to verify the occurrence of alternative vegetation regimes, image the spatial boundaries separating regimes, track the magnitude and direction of regime shift signals, differentiate persistent and stationary transition signals that warrant continued screening from more concerning persistent and non-stationary transition signals, and leverage disciplinary strength and resources for more targeted diagnostic testing (e.g., inventory and monitoring) and treatment (e.g., management) of regime shifts. While the rapid screening approach used here can continue to be implemented and refined for rangelands, it has broader implications and can be adapted to other ecological systems to revolutionize the information space needed to better manage critical transitions in nature.
Screening is widely applied to the early detection of undesirable change. Pioneering approaches to screening in medicine made it possible to detect diseases before consequences to health were realized by the individual or confirmed through practitioner diagnosis (Morabia and Zhang, 2004). The use of spatial medical imaging in early screening for a variety of diseases is now commonplace. It is important to differentiate between screening, which indicates the potential presence of a disease, ideally before the emergence of signs and/or symptoms, and diagnostic testing, which confirms the presence of a disease following the emergence of characteristics signs and/or symptoms or screening-based detection (Gilbert et al., 2001). Screening generates information that can be used to proactively diagnose and treat a disease, and thereby, avoid or mitigate its detrimental effects (Morris, 1994; Saunders et al., 2001).
Despite its clear utility in medicine, screening for undesirable ecological change (i.e., regime shifts; state transitions) has yet to be implemented in ecosystem management. Ecosystem management to date has largely focused on diagnosing (e.g., monitoring and inventorying) and treating (e.g., managing) regime shifts (Figure 1). It is not surprising then that ecosystem management as a whole has remained more reactive than proactive in an era of global change, uncertainty, and surprise. Even though ecology has long sought objective early warning indicators of regime shifts, in the language of medicine, most applications of early warning indicators are diagnostic, in that they are predicated on the detection of the signs and/or symptoms of change that has already begun to occur (Biggs et al., 2009; Dakos et al., 2015). Furthermore, many early warning indicators of ecological regime shifts require extensive data and a priori understanding of focal systems and/or the disturbances they experience (Gsell et al., 2016). This means that such applications may be incapable of objectively representing the risk of undesirable regime shifts prior to the manifestation of their symptoms, which limits preventative management efforts and leaves professionals reliant upon reactive strategies that lag behind transition signals.
Figure 1. In medicine, screening is used for early disease detection, prior to the emergence of disease signs and/or symptoms. Technological and theoretical barriers have prevented the implementation of screening methods in ecology, so the discipline has been largely reactive and incapable of dealing with large-scale undesirable changes that affect human well-being. Now, critical theory–data–technology linkages enable imaging and screening for ecological regime shifts.
The study of regime shifts in ecology is rooted in resilience thinking (Folke et al., 2010), where resilience is defined as the capacity of a system to absorb disturbance without transitioning to an alternative regime, whether the present or alternative regime is desirable or undesirable from a human perspective. Beyond its utility as a metaphor, the quantification of resilience has long been heralded for its potential to enhance the ability of management to prevent undesirable regime shifts; however, resilience quantification has proven notoriously elusive (Angeler and Allen, 2016). Among the major advances toward resilience quantification and operationalization in ecological management is Carpenter et al.'s (2001) recommendation to consider the “resilience of what to what” at the outset of any resilience-based assessment. In other words, identifying the focal system and disturbance(s) affecting it is fundamental for managing for resilience and avoiding undesirable regime shifts.
The “resilience of what to what” has factored into theoretical and quantitative advances in spatial resilience—an extension of resilience that can be defined simply as the contribution of spatial attributes to feedbacks that generate resilience (Allen et al., 2016). This includes, but is not limited to, the spatial arrangement of and interactions among internal system components, spatial variation in internal system phases (e.g., successional stages), and the system's spatial context (Cumming, 2011a,b). When disturbances exceed system resilience, regime shifts occur with spatial-temporal order (Sundstrom et al., 2017; Roberts et al., 2019). Through the lens of landscape ecology, one example of a spatial regime shift playing out over time is the spread of an initially rare invasive species patch through an initially abundant background landcover matrix. With some degree of spatial-temporal order, propagation of the invasive species fragments and reduces cover of the background matrix until the invasive species is so abundant and connected that it becomes the new background matrix, with the former matrix persisting only in isolated patches (Zurlini et al., 2014). When framed in succession theory, regime shifts may occur when alteration of historical disturbance regimes pushes systems into earlier or later successional stages. For example, increased fire frequency and severity may shift forests to woodlands and woodlands to grasslands, just as decreased fire frequency and severity may cause shifts in the opposite direction (Twidwell et al., 2013a; Fuhlendorf et al., 2017). There is certainly promise in enhancing understanding of systems and the disturbances that affect them, as well as in examining the spatial attributes of resilience and regime shifts from the perspective of different ecological subdisciplines; however, in returning to the language of medicine, these advances and explorations are more closely aligned with diagnosis than screening.
The development of approaches for screening for environmental change could contribute to improved decision-making in and effectiveness of ecological management. Undesirable regime shifts in ecological systems are often hysteretic (Scheffer et al., 2001), meaning that restoration is less feasible and more costly than if actions are put in place to avoid them from occurring in the first place (Holling and Meffe, 1996). Screening could be used to identify core areas of desirable regimes not yet experiencing regime shifts, which may be prioritized for preventative management that builds resilience of desirable regimes (Chapin et al., 2010). In the same way, areas in which screening indicates regime shift imminence may be prioritized for intensive management aimed at halting or reversing the regime shift. Finally, areas in which screening indicates that regime shifts have already occurred—particularly at large scales—or areas in which it is infeasible to halt regime shift advances, may explore avenues for transformation in law, policy, and governance to better deal the realities of the new regime (Chaffin et al., 2016; Garmestani et al., 2019).
Instead of replacing diagnostic approaches to regime shift detection, screening complements them and maximizes their utility. The absence of screening in ecosystem management up to this point in time is at least partly the result of data and computation limitations (Guttal and Jayaprakash, 2009); however, advances in technology and monitoring continue to make more accurate data available at greater spatial and temporal resolutions (i.e., grains) and extents (i.e., areas) (Pimm et al., 2015; Jones et al., 2018; Xie et al., 2019), and advances in geospatial cloud-computing enable efficient analysis of such data (Yang et al., 2011). At the same time, metrics are being developed in resilience science to quantify spatial contexts and signals that correspond with changes in ecological resilience, the collapse of ecological regimes, and their displacement by novel ecosystem states (Cline et al., 2014; Kéfi et al., 2014; Allen et al., 2016; Roberts et al., 2018a). Now, for the first time, critical components of theory, data, and technology are converging in linkages that make it plausible to screen for and image ecological regime shifts.
In this study, we introduce the practice of screening for the early detection of undesirable regime shifts in ecological systems, using rangeland systems of the Western United States as test cases. Rangelands are idealized systems for studying regime shifts, as different rangeland vegetation regimes (i.e., alternative stable states) are more-or-less desirable for certain suites of ecosystem services and are therefore the basis of ecosystem management (Westoby et al., 1989). Although not completely irreversible, undesirable regime shifts present considerable restoration challenges (Twidwell et al., 2013b), making it advisable to avoid shifts in the first place and to consider how screening might help do so. With a spatial informatics approach that links resilience theory with a state-of-the-art landcover dataset and a powerful cloud-computing platform, we screened for the following set of iconic rangeland vegetation transitions in landscapes of the western United States: (1) erosion and desertification; (2) woody plant encroachment; and (3) exotic annual grass invasion. In adherence to the First Law of Geography (Tobler, 1970), these regime shifts tend to manifest as spatially contagious processes, meaning that the likelihood that a location will experience a regime shift increases with geographic proximity to other locations that have experienced the shift. Simply put, spatial context is a critical determinant of a location's spatial resilience, and conversely, its spatial vulnerability to change.
During screening, we answer five questions: (1) Which regime shift is expected or of greatest concern? (2) Can we detect a signal associated with the expected regime shift? (3) If detected, is the signal transient or persistent over time? (4) If detected and persistent, is the transition signal stationary or non-stationary (i.e., moving) over time? (5) What other signals do we detect while screening? We then discuss the potential of our approach to detect vegetation transition signals and to characterize them according to their degrees of persistence and non-stationarity with little-to-no a priori understanding of focal system state, feedbacks, disturbances, or alternative stable states, and thereby, to contribute to more preventative and efficient management of rangelands and other ecological systems.
We used a state-of-the-art rangeland landcover raster dataset and a powerful, freely-accessible, cloud-based, geospatial computing platform to rapidly screen for spatial regime shift (i.e., vegetation transition) signals in five rangeland-dominated landscapes of the western United States from 2000 to 2017. The landcover dataset contains yearly, 30 m resolution, continuous percent cover estimates for the following four plant functional groups and three abiotic landcover components (hereafter referred to collectively as functional groups) in western United States rangelands from 1984 to2018: annual forbs and grasses, bare ground, litter, perennial forbs and grasses, rocks, shrubs, and trees (Jones et al., 2018), with rangelands delineated according to Reeves and Mitchell (2011). We used spatial covariance between functional group combinations as a screening metric. In rangelands, bare ground is representative of a state transition to an unvegetated state; however, litter has no basis as an alternative state and rock is stationary over the scale of our analysis. Therefore, neither percent litter nor percent rock were used for screening in this study, which reduced the number of contrasting functional groups to 5, and the number of unique pairwise functional group combinations to 10.
Our approach to regime shift screening emerges from resilience theory, which posits that a system exists in one, not multiple, regimes (i.e., stability domains; basins of attraction) at a time (Holling, 1973; Folke et al., 2010) and that transitions from one ecological regime to another exhibit spatial order at one or more scales of organization (Allen et al., 2016; Roberts et al., 2019). Given that alternative ecological regimes cannot occupy the same space over time, the simultaneous existence of multiple alternative regimes means that every regime is neighbored in space by an alternative regime(s)—each with its own reinforcing feedbacks and structures.
As a screening metric, we used a moving window algorithm to calculate spatial covariance between rangeland functional groups, where strong negative spatial covariance provides a geographic transition signal of regime boundaries that can be tracked through time. More generally, covariance is useful for highlighting boundaries and other correlations in data that averaging-based methods tend to smooth over (Frasinski et al., 1989; van der Heijden, 1995; Ando, 2000), and for decades, has been utilized to quantify spatial and temporal dynamics of ecological systems (Kershaw, 1961; Goodall, 1965; Noy-Meir and Anderson, 1971; Greig-Smith, 1983; Dale and Blundon, 1991; Wagner, 2003; Houlahan et al., 2018). Our selection of spatial covariance as a screening metric builds on the efforts of Pielou (1961), Goodall (1965), and other pioneers of methods for quantifying the spatial arrangement of ecological entities in relation to one-another. Functional groups that do not coexist should exhibit negative spatial covariance at their boundaries because of spatial irregularities (i.e., asymmetries, Norberg and Cumming, 2008) in their relational organization (i.e., tendency to separate from one another in space). In addition to quantifying the spatial arrangement of functional groups in relation to one another, spatial covariance is multivariate, and multivariate metrics tend to outperform univariate metrics in the detection of regime shifts in complex systems (Spanbauer et al., 2014; Roberts et al., 2018a). When computed in a moving window algorithm, spatial covariance also incorporates spatial context—an important external element of spatial resilience (Zurlini et al., 2006, 2014; Cumming, 2011a,b; Allen et al., 2016). Therefore, spatial covariance acts as an edge-detection technique, and importantly, one closely aligned with spatial resilience theory.
In the computation of spatial covariance, the more two entities are negatively associated with one another in space (i.e., one increases while the other decreases in a given area), the more strongly negative is their spatial covariance, whereas the more two entities are positively associated with one another in space (i.e., they increase or decrease together over the given neighborhood), the more strongly positive is their spatial covariance (Wagner, 2003). Spatial covariance of 0 means that no spatial relationship exists between two entities. In other words, spatial covariance provides a measure of the degree of coexistence between two entities. In the case of functional groups, more strongly negative spatial covariance provides a signal that emerges from the inability of two functional groups to coexist in a given space. For example, in Figure 2, strong negative spatial covariance mirrors the visual boundary between adjacent perennial grassland and tree regimes. With movement away from the boundary into either the core of grassland or forest regimes, perennial–tree spatial covariance increases from <-200 toward 0. The lack of trees within the core of the grassland regime results in the absence of a spatial relationship between grasses and trees in that area, just as the lack of grass within the core of the forest regime results in the absence of a spatial relationship between grasses and trees in that area.
Figure 2. (A) Landscape with visually apparent grass–tree boundaries and an example area (red box) for spatial covariance computation, and (B) The same landscape with perennial–tree spatial covariance mapped in the example area, where negative spatial covariance provides a regime shift signal associated with a lack of coexistence between perennials and trees (i.e., spatial regime boundary).
To rapidly compute spatial covariance between pairwise functional group combinations at relatively high spatial resolutions and over relatively broad geographic extents, we used Google Earth Engine—a powerful, freely-available, cloud-based geospatial computing platform (Gorelick et al., 2017). Within Google Earth Engine, we applied the covariance reducer function (Pébay, 2008) in a moving window (i.e., neighborhood) analysis to compute spatial covariance over four geographic neighborhood sizes: 3 by 3 pixels, 9 by 9 pixels, 27 by 27 pixels, and 113 by 113 pixels. In the moving window analysis, all kernels were weighted uniformly with values of 1 (i.e., no distance decay within neighborhoods). Analyses were conducted at a spatial grain of 30 m for the 3 by 3 pixel (0.81 hectares), 9 by 9 pixel (7.29 hectares), and 27 by 27 pixel (65.61 hectares) neighborhoods, and at a spatial grain of 60 m for the 113 by 113 pixel (4,596.84 hectares) neighborhood. Larger neighborhoods incorporate more spatial context into map pixel spatial covariance outputs. For each map pixel in each year, the raw output of Google Earth Engine's covariance function is a spatial variance–covariance matrix, in which matrix diagonals are spatial variance values (i.e., the spatial associations of functional groups with themselves) and the top and bottom matrix triangles are spatial covariance values (i.e., the spatial associations of functional groups with one another). Because the top and bottom spatial variance–covariance matrix triangles contain identical values, we extracted and mapped values from the top triangle of each pixel's matrix in five focal landscapes between 2000 and 2017. We exported spatial covariance maps of all 10 functional group combinations in all years as raster images in GeoTIFF format for processing and visualization in ArcMap (Esri, 2016) and R (R Core Team, 2018), with the lattice (Sarkar, 2008), latticeExtra (Sarkar and Andrews, 2016), sp (Bivand et al., 2013), raster (Hijmans, 2018), RColorBrewer (Neuwirth, 2014), rasterVis (Lamigueiro and Hijmans, 2018), rgdal (Bivand et al., 2018), and stringr (Wickham, 2018) packages for R.
We screened for regime shifts by asking and answering five questions: (1) Which regime shift is expected or of greatest concern? (2) Can we detect a signal associated with the expected regime shift? (3) If detected, is the signal transient or persistent over time? (4) If detected and persistent, is the transition signal stationary or non-stationary (i.e., moving) over time? (5) What other signals do we detect while screening? The spatially explicit answers to these questions—each derived through spatial imaging—are useful for flagging locations for continued screening, as well as diagnostic testing and treatment by local experts, stakeholders, managers, and scientists. Whether a spatial transition signal is present vs. absent, persistent vs. transient, and non-stationary vs. stationary informs the screening–diagnosis–treatment decision (Figure 3).
Figure 3. Workflow for screening for vegetation transitions in ecological systems, according to spatial signal presence vs. absence, persistence vs. transience, and non-stationarity vs. stationarity over time. The yellow-color in box number 6 represents transition signals that are particularly concerning because of their temporal presence, persistence, and non-stationarity.
Of the greatest concern are regime shift signals that are present, persistent, and non-stationary (i.e., moving). If signals are not persistent (i.e., transient), then they cannot be associated with regime shifts. This may be the case in instances where vegetation recovers in the wake of a disturbance. Another possibility is that vegetation signals persist through time but are spatially stationarity (i.e., do not spread), which is likely to occur along the geographic boundaries of opposing spatial regimes. Such places are also unlikely to be flagged as areas of concern during screening. Areas that are likely to be flagged as areas of concern are those that display spatially persistent and dynamic vegetation transition signals over time (i.e., non-stationarity). Such areas, where one spatial regime is actively displacing another, should be targeted for in-depth diagnosis of the change occurring (e.g., through remotely sensed imagery, monitoring, inventory, and local expert opinion) and/or treatment (e.g., management for preventing, halting, mitigating the effects of, or adapting to regime shift). Areas ahead of persistent and non-stationary transition signals (i.e., areas where change has not yet occurred but is likely to in the future) should be targeted for preventative management action, as they provide opportunities to anchor conservation efforts and build resilience against approaching regime shifts. The speed and degree of non-stationarity provide varying temporal windows for such vulnerability assessments and responses. Importantly, the targeting of areas for preventative management is predicated on the expectation that management is capable of preventing a given regime shift, which is not always true, particularly when dealing with broad-scale regime shift drivers (e.g., changing climatic conditions make the persistence of an established plant community below a given elevation unlikely, regardless of management) (Wonkka et al., 2019). For this reason, it is important to link screening results to management through a formal diagnosis of the regime shift and its likely causes. If management is incapable of preventing an approaching regime shift, then management resources and efforts may be more effectively devoted to transforming the system to mitigate the negative effects of the regime shift (Chapin et al., 2010; Chaffin et al., 2016; Garmestani et al., 2019).
We possessed varying degrees of knowledge about potential drivers of the spatial transition signals detected through screening in each of the focal landscapes. For illustrative purposes, we moved from screening to diagnosis by simply examining aerial imagery of focal landscapes and by speculating about potential spatial transition signal drivers. Further diagnosis could have been applied via on-site field inventory, monitoring, and analysis. In practice, there is a clear distinction between screening and diagnostic testing, so we avoid blurring screening and diagnosis here except where a focal example required further investigation and explanation.
We screened for signals of the following three iconic rangeland vegetation transitions: (1) erosion and desertification; (2) woody encroachment; and (3) exotic annual grass invasion. Erosion and desertification involve shifts from vegetated to non-vegetated (i.e., bare ground) states; woody encroachment is a shift from grass-to-woody plant dominance resulting from the displacement of herbaceous perennial vegetation to shrubs or tree dominance; and exotic annual grass invasion is a shift from herbaceous perennials, shrubs, or trees to annual grasses. All of these spatial regime shifts, and the mechanisms by which they occur, are dependent on local contexts and differ across western USA rangelands. We therefore chose multiple study sites spanning the American Southwest, the Southern and Northern Great Plains, and the Great Basin to screen for spatial vegetation transition signals from 2000 to 2017. Each focal landscape is dominated by rangeland but possesses a unique environmental setting, species assemblage, disturbance history, and set of alternative regimes to which its rangelands may shift. Below, we overview briefly each of the three types of vegetation transitions and the focal landscape(s) we selected for screening.
Erosion and desertification are problematic phenomena that threaten human livelihoods in dryland rangelands worldwide and are therefore actively managed against (Bestelmeyer et al., 2015). In the United States, substantial investments are allocated each year to reducing erosion in order to avoid catastrophes like the Dust Bowl that accompanied the historic drought of the 1930s (Egan, 2006). We screened two landscapes with supposed heightened vulnerabilities to erosion and desertification: the Sandhills of north-central Nebraska and a cropped valley of the Mojave Desert of southwestern Nevada.
Large-scale erosion is a constant subject of concern in the Nebraska Sandhills—an ecoregion with sandy soils that are stabilized by perennial vegetation—particularly during wildfire and drought (Arterburn et al., 2018). At a smaller scale, blowouts (i.e., de-vegetated sand pits) are a common landscape feature throughout the Sandhills, but the spread of blowouts to neighboring grass-dominated areas is actively managed against, especially under dry conditions (Schmeisser McKean et al., 2015). Using spatial covariance between bare ground and perennial forbs and grasses (hereafter perennials) functional groups in a moving window algorithm over a 27 by 27 pixel neighborhood, we screened for large-scale erosion and desertification in a Sandhills landscape centered around 42.8033°N and −100.0414°W in the years leading up to and following 2012, a year of extreme drought and in which the Region 24 Complex Wildfire occurred. At a smaller scale, we also used bare ground–perennial spatial covariance in a moving window algorithm over a 3 by 3 pixel neighborhood to screen for increased erosion in the vicinity of a randomly selected blowout centered around 42.7294°N and −100.0502°W within the Region 24 Complex Wildfire perimeter over the same set of years.
Despite arid conditions, portions of the Mojave Desert support irrigated rowcrop agriculture; however, tilled soils are easily eroded and carried by wind into neighboring, non-tilled shrublands (Okin et al., 2001). Using spatial covariance between annual forbs and grasses (hereafter annuals) and bare ground in a moving window algorithm over a 9 by 9 pixel neighborhood, we screened for transition signals associated with erosion of croplands in a network of center-pivot irrigated rowcrop fields in southwestern Nevada centered around 37.7605°N and −118.0783°W.
Woody plant encroachment threatens grasslands and savannas worldwide (Lasslop et al., 2016). In many systems, the dramatic shift in fire management associated with European colonization has facilitated the spread of woody plants into grasslands (Bowman et al., 2011). This class of regime shift demonstrates how disturbance regime alteration—in this case, severe reduction or elimination of historical disturbances—permits systems to advance to later successional trajectories with a high degree of spatial-temporal order. In other words, this regime shift is associated with lags in response to human-induced land management (Streit Krug et al., 2017). Herbaceous perennial species and woody plants tend not to coexist on a large scale in grasslands; therefore, woody encroachment results in a shift from herbaceous-to-woody plant dominance. We screened for transition signals associated with the encroachment of three woody species in two landscapes of the North American Great Plains: mesquite (Prosopis spp.) and ashe juniper (Juniperus ashei) in rangelands near the City of Breckenridge in north-central Texas and eastern redcedar (Juniperus virginiana) in rangelands of the Loess Canyons of west-central Nebraska.
Increases in mesquite cover have become a serious rangeland management challenge throughout much of northern Texas, with evidence that significant increases in mesquite cover can occur in treated (e.g., root-plowed) and untreated areas (Ansley et al., 2001). Using spatial covariance between perennials and trees in a moving window algorithm over a 27 by 27 pixel neighborhood, we screened for woody plant encroachment in a set of north-central Texas properties, near the City of Breckenridge, centered around 32.8635°N and −98.9537°W.
The Loess Canyons landscape is located in southwest Nebraska, south of the Platte River, in the area centered around 40.9339°N and −100.5338°W. Steep hills and canyons are grazed for cattle production, but in recent decades, have rapidly experienced increases in eastern redcedar cover (Roberts et al., 2018b). We used spatial covariance between perennials and trees in a moving window algorithm over a 113 by 113 pixel neighborhood to screen for transition signals associated with shifts from grass-to-woody plant dominance in the Loess Canyons between 2000 and 2017.
Over the past several decades, the exotic annual cheatgrass (Bromus tectorum) has rapidly invaded rangelands of the western United States, many of which were historically dominated by fire-intolerant shrubs and herbaceous perennial species. One of the major consequences of cheatgrass invasion has been fire regime alteration (D'Antonio and Vitousek, 1992; Balch et al., 2013; Chambers et al., 2019) and the emergence of a self-perpetuating annual grass/fire cycle. Cheatgrass changes fine fuel bed characteristics, resulting in larger and more frequent fires than would have occurred in uninvaded rangelands where fire was rare and spatially discontinuous. Reseeding is a commonly implemented management action for shifting vegetative dominance back from cheatgrass to perennials and to avoid further loss of sagebrush (Artemisia spp.) dominated areas to cheatgrass (Chambers et al., 2014).
In a southeastern Oregon landscape centered around 42.3662°N and −117.8300°W, we used spatial covariance between annuals and shrubs in a moving window algorithm over a 27 by 27 pixel neighborhood to screen for transition signals associated with cheatgrass invasion and management. In this case, management included herbicide treatment to control brush and reseeding to an introduced perennial bunchgrass (crested wheatgrass; Agropyron cristatum) in the late 1960s (Heady and Bartolome, 1977). The management unit and surrounding landscape burned in the 2012 Long Draw wildfire.
In the Nebraska Sandhills ecoregion, screening returned no evidence of large-scale erosion/desertification following the Region 24 Complex wildfire (Figures 4A–D). Although a stark signal that corresponded with the wildfire perimeter occurred in 2012 (Figure 4C), it vanished the following year and was therefore considered transient over time. From a practitioner's standpoint, we would conclude that no large-scale erosion or desertification is occurring, and therefore, the location could be flagged for continued screening, but neither additional diagnosis (e.g., intensive field monitoring) nor expensive treatment (e.g., reseeding) are warranted. This conclusion is supported by a previous study that found rapid re-establishment and recovery of Sandhills prairie following the historic drought and wildfire (Arterburn et al., 2018).
Figure 4. Screening and spatial imaging for vegetation transitions following wildfire in the Sandhills of Nebraska, USA, during a severe drought. (A–D) Large-scale imaging at the extent of a 2012 wildfire perimeter; (E–H) local-scale imaging at the extent of a single blowout within the 2012 wildfire perimeter. Shown here are perennial–bare ground spatial covariance images, where more strongly negative spatial covariance values indicate increasing spatial incompatibility of the two functional groups at the relative scale of analysis.
An alternative explanation for the lack of a persistent, large-scale transition signal in the Sandhills landscape could be that erosion and destabilization are occurring at a finer scale of analysis but are not yet evident at the scale of the entire wildfire. When reviewing localized spatial signals, we detected a strong, persistent, and largely stationary bare ground–perennial signal that corresponded with a blowout within the wildfire perimeter (Figures 4E–H). The only notable change in the spatial transition signal representing the boundary between prairie and the blowout was in 2012 (Figure 4G), when the signal disappeared entirely from the blowout and moved to the wildfire perimeter. However, in the years following the fire, the bare ground–perennial signal associated with the fire perimeter rapidly faded and the signal associated with the perimeter of the blowout reappeared. Thus, from 2007 to 2017, the blowout's spatial transition signal was present and persistent, but it failed to exhibit non-stationary by spreading into surrounding areas—before, during, or after the wildfire—in a manner that led to the expansion of the blowout and displacement of perennial grassland. From a practitioner's standpoint, we would once again conclude that no additional diagnostic monitoring or treatment is warranted for this screening result. For illustrative purposes, we only analyzed a single, randomly-selected blowout for spatial transition signals of erosion and expansion; however, our approach could be used by a resource professional to monitor the entire networks of blowouts.
While screening for potential erosion and destabilization of the Sandhills prairie ecosystem, we also screened for spatial transition signals from other functional group combinations that might not have been expected (Supplementary Figure 1). At the large-scale, we detected a strong, persistent, and non-stationary spatial transition signal associated with perennial–tree spatial covariance over a 27 by 27 pixel neighborhood (Figure 5). This spatial transition signal corresponded geographically with the Niobrara River valley forest corridor. In the 5 years preceding the 2012 wildfire, the transition signal was present, persistent, and stationary (Figure 5A). However, there was a drastic shift in the spatial order of the signal following the wildfire that carried over into subsequent years (Figure 5B). This is indicative of a rapid and drastic collapse of a major portion of the riverine forest corridor, which included a mix of coniferous (ponderosa pine and eastern redcedar) and deciduous species. Local experts could use this information to focus their attention on the most pertinent regime shift occurring in this landscape, thereby avoiding overtreatment and potential misuse of funds because of a signal that will likely regain its intensity (recover) without human intervention, and hold in-depth discussions about the next steps needed to diagnosis the specifics of the regime shift and whether management intervention is necessary.
Figure 5. Screening for additional vegetation transitions in the Nebraska Sandhills revealed (A) a present, persistent, and stationary large-scale perennial–tree spatial covariance signal from 2007 to 2011 and (B) a present, persistent, and non-stationary perennial–tree spatial covariance signal from 2012 to 2017, following the wildfire-induced collapse of the riparian forest corridor. More strongly negative spatial covariance values indicate increasing spatial incompatibility of the two functional groups at the relative scale of analysis Below, the outline of groups of pixels with perennial–tree spatial covariance of <100 in 2007, 2011, 2012, and 2017.
Persistent spatial transition signals associated with the individual perimeters of a network of center-pivot irrigated rowcrop fields were apparent in the Mojave (Figure 6). These persistent spatial transition signals remained stationary for many of the individual fields between 2003 and 2017 (Figure 6A), but non-stationarity was detected at other locations, where erosion is likely occurring and contributing to the desertification of adjacent lands. For example, in Figure 6B, the eastward bleeding of the spatial transition signal over time provides evidence of erosion and desertification. We did not detect any additional persistent spatial transition signals.
Figure 6. Screening for spatial transition signals associated with erosion and desertification in a network of center pivot-irrigated rowcrop fields in the Mojave Desert every other year from 2003 to 2017, where more strongly negative spatial covariance values indicate increasing spatial incompatibility of the two functional groups at the relative scale of analysis, with examples of: (A) present, persistent, and stationary boundaries (i.e., no erosion/desertification); and (B) present, persistent, and non-stationary boundaries (i.e., erosion and desertification).
Brush management for mesquite often results in a patch-work of properties with hard, stationary boundaries, where one property is dominated by perennial grass vegetation and the adjacent property is dominated by a mesquite shrubland. We were able to detect a transition signal for this type of boundary near Breckenridge, Texas (Figure 7A). Prior to 2008, a persistent and stationary transition signal was observed on an east–west line bisecting the landscape. We confirmed this to be two pastures separated by a fence-line post-hoc. The spatial transition signal became non-stationary in 2012. Aerial imagery for the years 2008, 2012, and 2017 revealed an increase in mesquite density and cover in the southwestern pasture over time that corresponded with the spatial transition signal, with the key implication being that an undesirable vegetation regime shift was detected and began to spread to areas previously dominated by perennial vegetation. Local in-depth diagnosis is warranted to determine why management is no longer holding the line, whether management has been discontinued, and how this regime shift can be prevented from continuing to expand into the surrounding rangeland landscape.
Figure 7. Screening for spatial transition signals associated with woody plant encroachment near Breckenridge, Texas, where more strongly negative spatial covariance values indicate increasing spatial incompatibility of the two functional groups at the relative scale of analysis, with: (A) a present, persistent, and non-stationary perennial–tree spatial transition signal associated with woody encroachment from 2008 to 2016 and (B) the emergence of a present, persistent, and non-stationary bare ground–perennial spatial transition signal associated with energy and road development from 2008 to 2011.
While screening for the expected and concerning spatial transition signal, we noted a secondary signal in the spatial covariance between bare ground and perennial functional groups over a 9 by 9 pixel neighborhood (Figure 7B). This signal appeared from 2011 to 2014 and exhibited markedly different patterns than in previous years. Further diagnostic investigation using aerial imagery over the same time period revealed that the spatial signal was associated with energy development and associated road infrastructure. Although the signal was the outcome of a purposeful, small-scale vegetation transition, this example shows how this rapid screening technique can image and track both known and unknown types of regime shifts occurring in rangelands, irrespective of whether shifts are of human or non-human origin.
In the Loess Canyons ecoregion, we detected a strong, persistent, and directionally non-stationary spatial transition signal indicating a shift from grass-to-woody plant dominance (Figure 8A). A peak in the intensity of the spatial transition signal occurred from 2010 to 2011 and was followed by a brief interruption from 2012 to 2014 (Figure 8B), after which directional change resumed and then plateaued from 2015 to 2017 (Figure 8C). The interruption of the spatial transition signal was likely the result of a severe drought in 2012 that differentially affected perennials and trees and briefly masked spatial associations driven by grass–tree interactions (i.e., strong negative spatial covariance), and the stabilization of the signal from 2015 to 2017 is presumably the result of large-scale, coordinated eastern redcedar management by a local prescribed burn association. No other persistent spatial transition signals were detected.
Figure 8. Screening for spatial transition signals associated with woody plant encroachment in the Loess Canyons ecoregion of Nebraska, where more strongly negative spatial covariance values indicate increasing spatial incompatibility of the two functional groups at the relative scale of analysis, with: (A) a present, persistent, and non-stationary perennial–tree spatial transition signal from 2000 and 2011; (B) a brief interruption of the transition signal from 2012 to 2014 following historic drought; and (C) an increased and then management-driven stabilization of the transition signal from 2015 to 2017.
We detected a persistent spatial transition signal with a geometry that corresponded to the boundary of a site with a history of wildfire and management against cheatgrass invasion (1960s herbicide treatment and reseeding to crested wheatgrass) in southeastern Oregon (Figure 9). Multiple transition signals were evident in the year preceding wildfire (2011), weakened in the year post-fire (2013) when vegetation was absent, and subsequently re-emerged or disappeared with vegetation recovery. The shrub–annual transition signal varied in intensity yet retained its overall spatial structure over time (Figure 9A). The emergence and disappearance of additional, localized shrub–annual transition signals in the vicinity of the original transition signal make it difficult to ascertain the degree to which the regime shift is spreading over time. It is likely the disappearance of these local signals corresponds with a loss of sagebrush and increase in annuals in the untreated landscape adjacent to the management unit following the 2012 wildfire. Similar overarching patterns emerged in the bare ground–annual (Figure 9B) and perennial–annual (Figure 9C) transition signals. The spatial covariance between perennials and annuals at the management unit border was slightly positive, which may be the result of cheatgrass replacing bare ground between bunchgrasses but not immediately replacing the bunchgrasses. Indeed, the strong negative spatial covariance between annuals and bare ground likely stems from the loss of bare ground in plant interspaces that accompanies transitions to cheatgrass. Therefore, these spatial transition signals may be collectively reflecting differences in the ability to resist cheatgrass invasion between the management unit dominated by perennial bunchgrasses and surrounding untreated lands. Additional on-site inventory and monitoring is needed to confirm the degree to which management has been successful at stemming cheatgrass conversion at this site and if regime shifts are occurring and spreading in the adjacent area. Analysis of temporal trends in data (e.g., detection of boom–bust cycles in the growth, spread, and interaction of annuals with other functional groups) may provide additional insights.
Figure 9. Screening for spatial transition signals associated with exotic annual grass invasion in a southeastern Oregon landscape that experienced a 2012 wildfire, where more strongly negative spatial covariance values indicate increasing spatial incompatibility of the two functional groups at the relative scale of analysis. From 2000 to 2017, matching transition signals between (A) shrubs and annuals and (B) bare ground and annuals were present and relatively persistent and stationary. Over the same set of years, (C) a similarly-patterned perennial–annual spatial covariance signal (albeit slightly positive) between perennials and annuals was also evident. The management boundary is associated with the application of herbicide and reseeding with crested wheatgrass (Agropyron cristatum) in the 1960s (Heady and Bartolome, 1977).
This paper explores the potential to image and screen for rangeland vegetation transitions. For a diverse set of rangeland-dominated landscapes of the western United States, we implemented a workflow (Figure 3) that: (1) identified the regime shift of greatest concern or that was most expected; (2) detected the presence of spatially explicit signals that were potential regime shift candidates; (3) differentiated transient signals of vegetation response from more persistent signals of vegetation transition over time; (4) determined whether persistent transition signals were stationary or non-stationary over time, and therefore, transitioning in space; and (5) repeat the process to screen for additional transitions in rangeland vegetation that were or lesser concern, not expected, or unknown a priori. This flexible methodology allows professionals to use spatial imaging to image spatial regime boundaries, track the magnitude and direction of regime shift signals, differentiate persistent and stationary transition signals from more-concerning persistent and non-stationary transition signals, and leverage disciplinary strength and resources for targeted diagnostic testing (e.g., inventory and monitoring) and treatment (e.g., management) of regime shifts. Because ecological systems experience frequent disturbances and are subject to external forcing, screening for regime shifts—according to signal presence, persistence, and non-stationary—may help differentiate between temporary aberrations in conditions and major, possibly permanent, shifts.
Imaging and screening for regime shifts in ecology follows a similar logic to the mission of screening in medicine (Morris, 1994; Saunders et al., 2001), in that the consequences of such shifts are often so severe that it is in humanity's best interest to prevent the emergence of detrimental regime shifts or to treat them at the earliest possible point of detection. Many consequences of spatial regime shifts are unable to be predicted until after the shift occurs. Cheatgrass invasion and regional dominance led to surprising changes in wildfire behavior and occurrence, heightened exposure of urban populations to smoke and air pollution, and cascading impacts to endemic wildlife (D'Antonio and Vitousek, 1992; Balch et al., 2013; Chambers et al., 2014). Juniper displacement of prairie ecosystems in the Great Plains is now linked to concerning impacts on water resources (Zou et al., 2018), public school funding (Lally et al., 2016), wildfire suppression potential, and collapses in pastoral agricultural revenue and rural livelihoods (Twidwell et al., 2013a). Erosion and desertification has been a notorious regime shift that has been actively avoided since the tragedy of the Dust Bowl (Wallace and Silcox, 1936), which was driven in part by human conversion of rangeland to cropland.
Spatial metrics derived from resilience theory, next-generation data products, technological capabilities, and computational power have all advanced to the point where spatial signals of regime shifts can be imaged and tracked at geographic and temporal extents and resolutions that were previously infeasible. In the past, the computation and application of resilience theory metrics across large geographic extents and through time was logistically infeasible due both to data limitations and extreme computational requirements. Advances in geospatial cloud-computing have overcome such computational hurdles and have also contributed to recent advances in landcover data (Xie et al., 2019), including the data used in this study—continuous (i.e., not categorical) percent cover estimates for major functional groups at high spatiotemporal resolution (i.e., 30 m and yearly) and extent (i.e., western United States and multi-decadal) (Jones et al., 2018). Additional theory–data–technology linkages are foundational for the continued testing and application of resilience theory at multiple scales in ecological systems.
Moving forward, it is important to continue advancing regime shift screening with a guiding understanding of its strengths and limitations, which reflect those of screening in general. Regime shift screening may be most useful in circumstances where regime shifts have strongly negative consequences for people and the environment (Scheffer et al., 2001), when the scale(s) of policies match the scale(s) at which regime shifts are occurring (Cumming et al., 2006), and when there are established pathways between screening, diagnosis, and treatment. Alternatively, a general limitation of screening that should be addressed in future research endeavors is the susceptibility of screening to false positives. Here, the precautionary principle should be applied and candidate locations should be flagged for continued screening, diagnosis, and treatment, as it is arguably better to erroneously flag undesirable transition signals than to fail to detect them and be surprised by them. Additionally, the implementation of Holling and Allen (2002) adaptive inference could help minimize type II error (i.e., false negatives) during the initial screening stage and then subsequently reduce type I (i.e., false positives) through continued screening, diagnostic testing, and treatment. In diagnostic tests for regime shifts, spatial transition signals could also be paired with other sources of information (e.g., proportional cover of functional groups, Jones et al., 2018, vegetation inventory data, and local expert knowledge) to confirm the presence or absence of specific regime shifts. Regime shift screening may also benefit from comparisons with existing screening approaches, such as those based in medicine (Morabia and Zhang, 2004), environmental toxicity (Kramer et al., 2009), crop drought-tolerance (Tuberosa, 2012), and wildlife disease (Grogan et al., 2014). Such comparisons may also involve exploration of alternative screening metrics. We selected spatial covariance as a screening metric because of its alignment with ecology (Pielou, 1961; Goodall, 1965; Greig-Smith, 1983), complex systems theory (Norberg and Cumming, 2008), and spatial resilience theory (Cumming, 2011a,b; Allen et al., 2016). Although the primary focus of this study is the overall regime shift methodology, future studies should formally compare spatial covariance to alternative screening and diagnosis metrics with similar backings in resilience and complex systems theory. Such comparisons will be critical for extending and refining our approach to regime shift screening.
Using spatial imaging to screen for ecological regime shifts notably diverges from the existing and prevailing application of early indicators of regime shifts in ecology. Our approach to regime shift screening is not diagnostic and requires little-to-no a priori understanding of focal systems, their characteristic feedbacks, the disturbances they experience, or the alternative regimes to which they may shift. Many early warning indicators are largely diagnostic in their approach to undesirable transitions, as they focus on detecting change in its early stages (Biggs et al., 2009; Dakos et al., 2015) and require extensive understanding of the focal system and its characteristic disturbances (Gsell et al., 2016). This has contributed to the tendency of ecosystem management to remain reactive instead of proactive in the present era of global change. Importantly, we do not disregard or devalue diagnostic approaches or place-based information, but instead emphasize the power of applying screening beforehand, at the front-end of the scientific process, as part of the overall mission of translating science to the general citizenship (Figure 1). Screening simply informs where to continue screening, diagnosing (e.g., inventorying), and treating (e.g., restoring and monitoring) at different points in time. Given the inherent spatial order of regime shifts, screening results can be used to prioritize locations in proximity to those exhibiting persistent and non-stationary transition signals for preventative management that builds resilience against the impending regime shift (Holling and Meffe, 1996; Chapin et al., 2010). Such locations have not yet experienced regime shifts, but are likely to in the future, and can therefore serve as areas for anchoring conservation efforts and building resilience against approaching regime shifts. Other locations in close proximity to regime shift signals may be prioritized for intensive management aimed at halting regime shift advance, while still other locations where regime shifts are already occurring at broad scales may be slated for adaptation-based management under the new regime (Chaffin et al., 2016; Garmestani et al., 2019). Local and expert sources of knowledge, as well as perspectives from social and political science, are essential for effective diagnosis and treatment of regime shifts, in order to better inform how screening results can be used to support decision-making and management.
Screening is widely applied to the early detection of undesirable change, and despite its utility in other fields (e.g., medicine) screening has not yet been introduced to ecological management. Meanwhile, under the increasing pressures of global change, ecological systems continue to experience shifts to alternative and often undesirable regimes (i.e., states). We developed a workflow for regime shift screening (Figure 3), which we used to screen for three of the most concerning transitions in rangelands: (1) erosion and desertification; (2) woody encroachment; and (3) annual exotic grass invasion. We screened for these transitions in an array of rangeland-dominated landscapes of the western United States—from the Mojave Desert to the Great Plains. Screening returned no evidence of regional-scale erosion/desertification in the Nebraska Sandhills following wildfire but did detect erosion in the vicinity of irrigated rowcrop fields in the Mojave. Screening also indicated local-to-regional-scale woody plant encroachment in several Great Plains landscapes and annual grass invasion in southeastern Oregon. While the screening approach outlined in this study is relatively new and unknown, it is well-grounded in the theories of ecology, complex systems, and spatial resilience, and as such, holds promise for the early detection of ecological regime shifts, particularly when effectively linked with strategies for regime shift diagnosis and treatment.
Data are available for visualization and analysis via the Rangeland Analysis Platform (https://rangelands.app/rap).
DU conducted the analysis and took the lead on writing. DT and DU conceived the idea. All authors contributed to writing and analytical design and development.
This work was funded by the National Science Foundation (OIA-1920938), United States Department of Agriculture – Natural Resources Conservation Service and Pheasants Forever (PG18-62799-01 and SGI 2.0-19-06), and the University of Nebraska Agricultural Research Division. This work was made possible by the NRCS Working Lands for Wildlife in support of sage-grouse and prairie chicken conservation and the USDA Conservation Effects Assessment Project.
The authors declare that the research was conducted in the absence of any commercial or financial relationships that could be construed as a potential conflict of interest.
The Supplementary Material for this article can be found online at: https://www.frontiersin.org/articles/10.3389/fevo.2019.00407/full#supplementary-material
Allen, C. R., Angeler, D. G., Cumming, G. S., Folke, C., Twidwell, D., and Uden, D. R. (2016). Quantifying spatial resilience. J. Appl. Ecol. 53, 625–635. doi: 10.1111/1365-2664.12634
Ando, S. (2000). Image field categorization and edge/corner detection from gradient covariance. IEEE Trans. Pattern Anal. Mach. Intell. 22, 179–190. doi: 10.1109/34.825756
Angeler, D. G., and Allen, C. R. (2016). Editorial: quantifying resilience. J. Appl. Ecol. 53, 617–624. doi: 10.1111/1365-2664.12649
Ansley, R. J., Ben Wu, X., and Kramp, B. A. (2001). Observation: long-term increases in mesquite canopy cover in a north Texas savanna. J. Range Manage. 54, 171–176. doi: 10.2307/4003179
Arterburn, J. R., Twidwell, D., Schacht, W. H., Wonkka, C. L., and Wedin, D. A. (2018). Resilience of Sandhills grassland to wildfire during drought. Rangeland Ecol. Manage. 71, 53–57. doi: 10.1016/j.rama.2017.07.010
Balch, J. K., Bradley, B. A., D'Antonio, C. M., and Gómez-Dans, J. (2013). Introduced annual grass increases regional fire activity across the arid western USA (1980–2009). Glob. Change Biol. 19, 173–183. doi: 10.1111/gcb.12046
Bestelmeyer, B. T., Okin, G. S., Duniway, M. C., Archer, S. R., Sayre, N. F., Williamson, J. C., et al. (2015). Desertification, land use, and the transformation of global drylands. Front. Ecol. Environ. 13, 28–36. doi: 10.1890/140162
Biggs, R., Carpenter, S. R., and Brock, W. A. (2009). Turning back from the brink: detecting an impending regime shift in time to avert it. Proc. Natl. Acad. Sci. U.S.A. 106, 826–831. doi: 10.1073/pnas.0811729106
Bivand, R. S., Keitt, T., and Rowlingson, B. (2018). rgdal: Bindings for the ‘Geospatial' Data Extraction Library. R package version 1.3-6. Available online at: https://CRAN.R-project.org/package=rgdal
Bivand, R. S., Pebesma, E., and Gomez-Rubio, V. (2013). Applied Spatial Data Analysis With R, 2nd Edn. New York, NY: Springer, 405. doi: 10.1007/978-1-4614-7618-4
Bowman, D. M. J. S., Balch, J., Artaxo, P., Bond, W. J., Cochrane, M. A., D'Antonio, C. M., et al. (2011). The human dimension of fire regimes on Earth. J. Biogeogr. 38, 2223–2236. doi: 10.1111/j.1365-2699.2011.02595.x
Carpenter, S., Walker, B., Anderies, J. M., and Able, N. (2001). From metaphor to measurement: the resilience of what to what? Ecosystems 4, 765–781. doi: 10.1007/s10021-001-0045-9
Chaffin, B. C., Garmestani, A. S., Gunderson, L. H., Benson, M. H., Angeler, D. G., Arnold, C. A., et al. (2016). Transformative environmental governance. Annu. Rev. Environ. Resour. 41, 399–423. doi: 10.1146/annurev-environ-110615-085817
Chambers, J. C., Bradley, B. A., Brown, C. S., D'Antonio, C., Germino, M. J., Grace, J. B., et al. (2014). Resilience to stress and disturbance, and resistance to Bromus tectorum L. invasions in cold desert shrublands of western North America. Ecosystems 17, 360–375. doi: 10.1007/s10021-013-9725-5
Chambers, J. C., Brooks, M. L., Germino, M. J., Maestas, J. D., Board, D. I., Jones, M. O., et al. (2019). Operationalizing resilience and resistance concepts to address invasive grass/fire cycles. Front. Ecol. Evol. 7:185. doi: 10.3389/fevo.2019.00185
Chapin, F. S. I. I. I., Carpenter, S. R., Kofinas, G. P., Folke, C., Abel, N., Clark, W. C., et al. (2010). Ecosystem stewardship: sustainability strategies for a rapidly changing planet. Trends Ecol. Evol. 25, 241–249. doi: 10.1016/j.tree.2009.10.008
Cline, T. J., Seekell, D. A., Carpenter, S. R., Pace, M. L., Hodgson, J. R., Kitchell, J. F., et al. (2014). Early warning of regime shifts: evaluation of spatial indicators from a whole-ecosystem experiment. Ecosphere 5:102. doi: 10.1890/ES13-00398.1
Cumming, G. S. (2011a). Spatial resilience: integrating landscape ecology, resilience, and sustainability. Landsc. Ecol. 26, 899–909. doi: 10.1007/s10980-011-9623-1
Cumming, G. S., Cumming, D. H. M., and Redman, C. L. (2006). Scale mismatches in social–ecological systems: causes, consequences, and solutions. Ecol. Soc. 11:14. doi: 10.5751/ES-01569-110114
Dakos, V., Carpenter, S. R., van Nes, E. H., and Scheffer, M. (2015). Resilience indicators: prospects and limitations for early warnings of regime shifts. Philos. Trans. R. Soc. B 370:20130263. doi: 10.1098/rstb.2013.0263
Dale, M. R. T., and Blundon, D. J. (1991). Quadrat covariance analysis and the scales of interspecific association during primary succession. J. Veg. Sci. 2, 103–112. doi: 10.2307/3235902
D'Antonio, C. M., and Vitousek, P. M. (1992). Biological invasions by exotic grasses, the grass/fire cycle, and global change. Annu. Rev. Ecol. Syst. 23, 63–87. doi: 10.1146/annurev.es.23.110192.000431
Egan, T. (2006). The Worst Hard Time: The Untold Story of Those Who Survived the Great American Dust Bowl. Boston, MA: Houghton Mifflin Company, 340.
Esri (2016). ArcGIS Desktop. Version 10.4.1. Available online at: https://www.esri.com/en-us/home
Folke, C., Carpenter, S. R., Walker, B., Scheffer, M., Chapin, T., and Rockström, J. (2010). Resilience thinking: integrating resilience, adaptability, and transformability. Ecol. Soc. 15:20. doi: 10.5751/ES-03610-150420
Frasinski, L. J., Codling, K., and Hatherly, P. A. (1989). Covariance mapping: a correlation method applied to multiphoton multiple ionization. Science 246, 1029–1031. doi: 10.1126/science.246.4933.1029
Fuhlendorf, S. D., Flynn, R. W., McGranahan, D. A., and Twidwell, D. (2017). “Heterogeneity as the basis for rangeland management,” in Rangeland Systems, ed D. D. Briske (Cham: Springer), 169–196. doi: 10.1007/978-3-319-46709-2_5
Garmestani, A. S., Ruhl, J. B., Chaffin, B. C., Craig, R. K. H. F, van Rijswick, M. W., et al. (2019). Untapped capacity for resilience in environmental law. Proc. Natl. Acad. Sci. U.S.A. 116, 19899–19904. doi: 10.1073/pnas.1906247116
Gilbert, R., Logan, S., Moyer, V. A., and Elliot, E. J. (2001). Assessing diagnostic and screening tests. West. J. Med. 174, 405–409. doi: 10.1136/ewjm.174.6.405
Goodall, D. W. (1965). Plot-less tests of interspecific association. J. Ecol. 53, 197–210. doi: 10.2307/2257577
Gorelick, N., Hancher, M., Dixon, M., Illyushchenko, S., Thau, D., and Moore, R. (2017). Google Earth Engine: planetary-scale geospatial analysis for everyone. Remote Sens. Environ. 202, 18–27. doi: 10.1016/j.rse.2017.06.031
Greig-Smith, P. (1983). Quantitative Plant Ecology, 3rd Edn. Berkeley, CA: University of California Press. 359.
Grogan, L. F., Berger, L., Rose, K., Grillo, V., Cashins, S. D., and Skerratt, L. F. (2014). Surveillance for emerging biodiversity diseases of wildlife. PLoS Pathog. 10:e1004015. doi: 10.1371/journal.ppat.1004015
Gsell, A. S., Scharfenberger, U., Özkundakci, D., Walters, A., Hansson, L. A., Janssen, A. B. G., et al. (2016). Evaluating early-warning indicators of critical transitions in natural aquatic systems. Proc. Natl. Acad. Sci. U.S.A. 113, E8089–E8095. doi: 10.1073/pnas.1608242113
Guttal, V., and Jayaprakash, C. (2009). Spatial variance and spatial skewness: leading indicators of regime shifts in spatial ecological systems. Theor. Ecol. 2, 3–12. doi: 10.1007/s12080-008-0033-1
Heady, H. F., and Bartolome, J. (1977). The Vale Rangeland Rehabilitation Program: The Desert Repaired in Southeastern Oregon. USDA Forest Service Resource Bulletin PNW-70. Portland, OR: Pacific Northwest Forest and Range Experiment Station, Forest Service, U.S. Department of Agriculture. 139.
Hijmans, R. J. (2018). raster: Geographic Data Analysis and Modeling. R package version 2.7-15. Available online at: https://CRAN.R-project.org/package=raster
Holling, C. S. (1973). Resilience and stability of ecological systems. Annu. Rev. Ecol. Syst. 4, 1–23. doi: 10.1146/annurev.es.04.110173.000245
Holling, C. S., and Allen, C. R. (2002). Adaptive inference for distinguishing credible from incredible patterns in nature. Ecosystems 5, 319–328. doi: 10.1007/s10021-001-0076-2
Holling, C. S., and Meffe, G. K. (1996). Command and control and the pathology of natural resource management. Conserv. Biol. 10, 328–337. doi: 10.1046/j.1523-1739.1996.10020328.x
Houlahan, J. E., Currie, D. J., Cottenie, K., Cumming, G. S., Findlay, C. S., Fuhlendorf, S. D., et al. (2018). Negative relationships between species richness and temporal variability are common but weak in natural systems. Ecology 99, 2592–2604. doi: 10.1002/ecy.2514
Jones, M. O., Allred, B. W., Naugle, D. E., Maestas, J. D., Donnelly, P., Metz, L. J., et al. (2018). Innovation in rangeland monitoring: annual, 30 m, plant functional type percent cover maps for US rangelands, 1984–2017. Ecosphere 9:e02430. doi: 10.1002/ecs2.2430
Kéfi, S., Guttal, V., Brock, W. A., Carpenter, S. R., Ellison, A. M., Livina, V. N., et al. (2014). Early warning signals of ecological transitions: methods for spatial patterns. PLoS ONE 9:e92097. doi: 10.1371/journal.pone.0092097
Kershaw, K. A. (1961). Association and co-variance analysis of plant communities. J. Ecol. 49, 643–654. doi: 10.2307/2257229
Kramer, M. G., Firestone, M., Kavlock, R., and Zenick, H. (2009). The future of toxicity testing for environmental contaminants. Environ. Health Perspect. 117:A283. doi: 10.1289/ehp.12891
Lally, D., Bielski, C., Schick, B., Westerhold, C., Zahn, A., Allen, C., et al. (2016). Eastern Redcedar Invasions Threatens Funding for Nebraska's Public Schools. University of Nebraska–Lincoln Beefwatch. Available online at: https://newsroom.unl.edu/announce/beef/5577/31325
Lamigueiro, O. P., and Hijmans, R. J. (2018). rasterVis. R package version 0.45. Available online at: https://CRAN.R-project.org/package=rasterVis
Lasslop, G., Brovkin, V., Reick, C. H., Bathiany, S., and Kloster, S. (2016). Multiple stable states of tree cover in a global land surface model due to fire–vegetation feedback. Geophys. Res. Lett. 43, 6324–6331. doi: 10.1002/2016GL069365
Morabia, A., and Zhang, F. F. (2004). History of medical screening: from concepts to action. Postgrad. Med. J. 80, 463–469. doi: 10.1136/pgmj.2003.018226
Neuwirth, E. (2014). RColorBrewer: ColorBrewer Palettes. R package version 1.1-2. Available online at: https://CRAN.R-project.org/package=RColorBrewer
Norberg, J., and Cumming, G. S. (eds.). (2008). Complexity Theory for a Sustainable Future. New York, NY: Columbia University Press, 315.
Noy-Meir, I., and Anderson, D. J. (1971). “Multiple pattern analysis, or multiscale ordination: towards a vegetation hologram?” in Statistical Ecology, eds G. P. Patil, C. Pielou, and W. E. Waters (University Park, PA: Pennsylvania State University Press, 207–231.
Okin, G. S., Murray, B., and Schlesinger, W. H. (2001). Degradation of sandy arid shrubland environments: observations, process modeling, and management implications. J. Arid Environ. 47, 123–144. doi: 10.1006/jare.2000.0711
Pébay, P. (2008). Formulas for Robust, One-Pass Parallel Computation of Covariances and Arbitrary-Order Statistical Moments. Sandia Report SAND2008-6212. Springfield, VA: Sandia National Laboratories. United States Department of Commerce. 16. doi: 10.2172/1028931
Pielou, E. C. (1961). Segregation and symmetry in two-species populations as studied by nearest neighbour relationships. J. Ecol. 49, 255–269. doi: 10.2307/2257260
Pimm, S. L., Alibhai, S., Bergl, R., Dehgan, A., Giri, C., Jewell, Z., et al. (2015). Emerging technologies to conserve biodiversity. Trends Ecol. Evol. 30, 685–696. doi: 10.1016/j.tree.2015.08.008
R Core Team (2018). R: A Language and Environment for Statistical Computing. Vienna: R Foundation for Statistical Computing. Available online at: https://www.R-project.org/
Reeves, M. C., and Mitchell, J. E. (2011). Extent of coterminous US rangelands: quantifying implications of differing agency perspectives. Rangeland Ecol. Manage. 64, 585–597. doi: 10.2111/REM-D-11-00035.1
Roberts, C. P., Allen, C. R., Angeler, D. G., and Twidwell, D. (2019). Shifting avian spatial regimes in a changing climate. Nat. Clim. Chang. 9, 562–568. doi: 10.1038/s41558-019-0517-6
Roberts, C. P., Twidwell, D., Burnett, J. L., Donovan, V. M., Wonkka, C. L., Bielski, C. L., et al. (2018a). Early warnings for state transitions. Rangeland Ecol. Manage. 71, 659–670. doi: 10.1016/j.rama.2018.04.012
Roberts, C. P., Uden, D. R., Allen, C. R., and Twidwell, D. (2018b). Doublethink and scale mismatch polarize policies for an invasive tree. PLoS ONE 13:e0189733. doi: 10.1371/journal.pone.0189733
Sarkar, D. (2008). Lattice: Multivariate Data Visualization With R. New York, NY: Springer. 265. doi: 10.1007/978-0-387-75969-2
Sarkar, D., and Andrews, F. (2016). latticeExtra: Extra Graphical Utilities Based on Lattice. R package version 0.6-28. Available online at: https://CRAN.R-project.org/package=latticeExtra
Saunders, D. L., Olive, D. M., Wallace, S. B., Lacy, D., Leyba, R., and Kendig, N. E. (2001). Tuberculosis screening in the federal prison system: an opportunity to treat and prevent tuberculosis in foreign-born populations. Public Health Rep. 116, 210–218. doi: 10.1016/S0033-3549(04)50036-5
Scheffer, M., Carpenter, S., Foley, J. A., Folke, C., and Walker, B. (2001). Catastrophic shifts in ecosystems. Nature 413, 591–596. doi: 10.1038/35098000
Schmeisser McKean, R. L., Goble, R. J., Mason, J. B., Swinehart, J. B., and Loope, D. B. (2015). Temporal and spatial variability in dune reactivation across the Nebraska Sand Hills, USA. Holocene 25, 523–535. doi: 10.1177/0959683614561889
Spanbauer, T. L., Allen, C. R., Angeler, D. G., Eason, T., Fritz, S. C., Garmestani, A. S., et al. (2014). Prolonged instability prior to a regime shift. PLoS ONE 9:e108936. doi: 10.1371/journal.pone.0108936
Streit Krug, A., Uden, D. R., Allen, C. R., and Twidwell, D. (2017). Culturally induced range infilling of eastern redcedar: a problem in ecology, an ecological problem, or both? Ecol. Soc. 22:46. doi: 10.5751/ES-09357-220246
Sundstrom, S. M., Eason, T., Nelson, R. J., Angeler, D. G., Barichievy, C., Garmestani, A. S., et al. (2017). Detecting spatial regimes in ecosystems. Ecol. Lett. 20, 19–32. doi: 10.1111/ele.12709
Tobler, W. R. (1970). A computer movie simulating urban growth in the Detroit region. Econ. Geogr. 46, 234–240. doi: 10.2307/143141
Tuberosa, R. (2012). Phenotyping for drought tolerance of crops in the genomics era. Front. Physiol. 3:347. doi: 10.3389/fphys.2012.00347
Twidwell, D., Fuhlendorf, S. D., Taylor, C. A. Jr., and Rogers, W. E. (2013a). Refining thresholds in coupled fire–vegetation models to improve management of encroaching woody plants in grasslands. J. Appl. Ecol. 50, 603–613. doi: 10.1111/1365-2664.12063
Twidwell, D., Rogers, W. E., Fuhlendorf, S. D., Wonkka, C. L., Engle, D. M., Weir, J. R., et al. (2013b). The rising Great Plains fire campaign: citizens' response to woody plant encroachment. Front. Ecol. Environ. 11:e64–e71. doi: 10.1890/130015
van der Heijden, F. (1995). Edge and line feature extraction based on covariance models. IEEE Trans. Pattern Anal. Mach. Intell. 17, 16–33. doi: 10.1109/34.368155
Wagner, H. (2003). Spatial covariance in plant communities: integrating ordination, geostatistics, and variance testing. Ecology 84, 1045–1057. doi: 10.1890/0012-9658(2003)084[1045:SCIPCI]2.0.CO;2
Wallace, H. A., and Silcox, F. A. (1936). The Western Range: Letter From the Secretary of Agriculture. Transmitting in Response to Senate Resolution No. 289. A Report on the Western Range—A Great but Neglected Natural Resource. Washington, DC: United States Government Printing Office. 620.
Westoby, M., Walker, B., and Noy-Meir, I. (1989). Opportunistic management for rangelands not at equilibrium. J. Range Manage. 42, 266–274. doi: 10.2307/3899492
Wickham, H. (2018). stringr: Simple, Consistent Wrappers for Common String Operations. R package version 1.3.1. Available online at: https://CRAN.R-project.org/package=stringr
Wonkka, C. L., Twidwell, D., Allred, B. W., Bielski, C. H., Donovan, V. M., Roberts, C. P., et al. (2019). Rangeland vulnerability to state transition under global climate change. Clim. Change 153, 59–78. doi: 10.1007/s10584-018-02365-7
Xie, Z., Phinn, S. R., Game, E. T., Pannell, D. J., Hobbs, R. J., Briggs, P. R., et al. (2019). Using Landsat observations (1988–2017) and Google Earth Engine to detect vegetation cover changes in rangelands – a firs step toward identifying degraded lands for conservation. Remote Sens. Environ. 232:111317. doi: 10.1016/j.rse.2019.111317
Yang, C., Goodchild, M., Huang, Q., Nebert, D., Raskin, R., Xu, Y., et al. (2011). Spatial cloud computing: how can the geospatial sciences use and help shape cloud computing? Int. J. Digital Earth 4, 305–329. doi: 10.1080/17538947.2011.587547
Zou, C. B., Twidwell, D., Bielski, C. H., Fogarty, D. T., Mittelstet, A. R., Starks, P. J., et al. (2018). Impact of eastern redcedar proliferation on water resources in the Great Plains USA—current state of knowledge. Water 10:1768. doi: 10.3390/w10121768
Zurlini, G., Jones, K. B., Riitters, K. H., Li, B. L., and Petrosillo, I. (2014). Early warning signals of regime shifts from cross-scale connectivity of land-cover patterns. Ecol. Indic. 45, 549–560. doi: 10.1016/j.ecolind.2014.05.018
Keywords: diagnosis, early warning indicator, Google Earth Engine, proactive management, rangeland analysis platform, resilience, spatial resilience, treatment
Citation: Uden DR, Twidwell D, Allen CR, Jones MO, Naugle DE, Maestas JD and Allred BW (2019) Spatial Imaging and Screening for Regime Shifts. Front. Ecol. Evol. 7:407. doi: 10.3389/fevo.2019.00407
Received: 15 March 2019; Accepted: 09 October 2019;
Published: 29 October 2019.
Edited by:
Charles K. Lee, University of Waikato, New ZealandReviewed by:
Kiri Joy Wallace, University of Waikato, New ZealandCopyright © 2019 Uden, Twidwell, Allen, Jones, Naugle, Maestas and Allred. This is an open-access article distributed under the terms of the Creative Commons Attribution License (CC BY). The use, distribution or reproduction in other forums is permitted, provided the original author(s) and the copyright owner(s) are credited and that the original publication in this journal is cited, in accordance with accepted academic practice. No use, distribution or reproduction is permitted which does not comply with these terms.
*Correspondence: Daniel R. Uden, ZHVkZW4yQHVubC5lZHU=
Disclaimer: All claims expressed in this article are solely those of the authors and do not necessarily represent those of their affiliated organizations, or those of the publisher, the editors and the reviewers. Any product that may be evaluated in this article or claim that may be made by its manufacturer is not guaranteed or endorsed by the publisher.
Research integrity at Frontiers
Learn more about the work of our research integrity team to safeguard the quality of each article we publish.