- School of Biology and Environmental Science, Earth Institute, University College Dublin, Dublin, Ireland
Complex regimes of stress arise when multiple stressors combine simultaneously, with varying degrees of temporal separation or variation in their sequential order. A manipulative field experiment was run to test whether doses of two stressors (Copper and Biocide) varied in their effects on marine epifauna and ecosystem functioning depending on their sequence, timing and delay before sampling. Our key finding was that time-lags between stressors led to longer-lasting effects. We also found that the sequential order of two stressors influenced effects on measures of ecosystem-level processes: for community respiration (CR) the metal-first sequence of stressors had a negative effect; for clearance rates the biocide-first sequence had the greater effect. Effects of stressors delivered simultaneously on CR and clearance rates were short-lived. Intra-individual effects on cellular viability did not correspond with effects on ecosystem-level variables. Results show that current frameworks for understanding and managing the effects of multiple stressors can be improved by incorporating temporal variation in both cause and effect.
Highlights
- We tested how doses of two stressors varied in their effects on marine epifauna and ecosystem functioning depending on their sequence, timing, and delay before sampling.
- We found that time-lags between stressors led to greater effects on measures of ecosystem-level processes and that the sequential order of two stressors also influenced those effects.
- We also found that intra-individual effects on cellular viability did not correspond with effects on ecosystem-level variables.
Introduction
Humans exert many pressures on ecosystems, with great potential to alter their biodiversity, functioning and capacity to provide ecosystem services (Vitousek, 1997; Assessment, 2005; Crowe et al., 2015). Research in the past has tended to focus on the effects of individual stressors in isolation (Halpern et al., 2008) but progress has been made on understanding how ecosystems are being affected by combinations of both cumulative individual and multiple stressors (Paine et al., 1998; Folt et al., 1999; Crain et al., 2008; Darling and Cote, 2008; Strain et al., 2014; Piggott et al., 2015; Côté et al., 2016; Brooks and Crowe, 2018). That said, our capacity to predict the combined effects of multiple local stressors in the future is limited by our lack of understanding of the potential of climatic change to modify interactions between them. For example, climatic change is expected to increase rainfall and storminess (IPCC, 2012), which is likely to amplify the intensity or change the temporal regimes of localized stressors (e.g., pollution, wave action, nutrient loading, freshwater influx, etc.) (Schiedek et al., 2007).
The potential importance of changing temporal regimes of disturbance has been recognized and explored (Benedetti-Cecchi, 2003; Lyons et al., 2015). Adams (2005) highlighted the importance of considering “time-lags” of both cause and effect in determining the impacts of stressors, where a time-lag of cause is delay in the arrival of stressors and time-lag of effect is temporal variation in the manifestation of an effect. It may be, for example, that where there is a delay between the arrival of one stress event and the arrival of the next, a system may be able to recover between stress events leading to a reduction in the overall effect relative to that caused by simultaneous events. Alternatively, if the system is repeatedly affected by separate stress events, it may be unable to recover between events and thereby be more severely affected. Experimental studies (e.g., Bertocci et al., 2005; Bulleri et al., 2014) have predominantly focused on cumulative effects of individual stressors where, for example, systems near the edge of their tolerance to a first stressor can have a much lower threshold to withstand the effects of a second stress event (Hewitt et al., 2016). However, little has been done to extend these ideas and experimental tests on temporal regimes of stressors to situations involving combinations of stressors or to explore the relative importance of cumulative individual vs. multiple stressors (but see Molinos and Donohue, 2010).
Stressor identity and the response of the system to the first stressor can be a determinant of how the same system responds to either a second occurrence of the same stressor or the arrival of a new stressor into the system. For example, repeated hurricane events over a given period had less impact than repeated hurricane events followed by chronic algal blooms due to overfishing in coral reefs (Hughes and Connell, 1999). The importance of the sequence in which different stressors arrive in a system was highlighted more than a decade ago (Breitburg et al., 1998; Paine et al., 1998) and appropriate experimental tests have been designed (e.g., Giller et al., 2004). Yet, because of the complexity and size of these designs, few studies have dealt with the sequential order of stressors. Nonetheless, there have been some notable exceptions in both conceptual (Vinebrooke et al., 2004) and empirical terms (Fukami, 2001; Nystrom and Folke, 2001; Macinnis-Ng and Ralph, 2004; Flöder and Hillebrand, 2012; Cheng et al., 2015). While most of these studies manipulated the sequential order of stressors, none explicitly tested the role of time-lags between those stressors and in most cases responses were only measured at the individual level of biological organization for one or two species.
Multiple lines of evidence should be used to determine effects of multiple stressors (Adams, 2005). This is important because utilizing a suite of techniques across varying levels of biological organization (Attrill and Depledge, 1997; Martinez-Crego et al., 2010) allows for testing of multiple hypotheses (Underwood and Peterson, 1988; Mayer-Pinto et al., 2010), increasing the potential to understand the effects of both cumulative and multiple stressors on ecosystem processes and functioning. For example, in sedimentary systems, O'Meara et al. (2017) showed that as the number of stressors increase, the ability to predict the role of NH4+ flux in governing ecosystem function is compromised due to a breakdown in networked processes.
Similarly, sampling regimes need to be designed specifically to characterize variation in response with time after application of the stressor/stressors (Adams, 2005; Martinez-Crego et al., 2010). Indeed, such sampling regimes have long been established but are not always used (Adams, 2005). That said, there have been some more recent long term studies that show that the responses of systems to the effects of stressors may vary temporally over longer time periods (e.g., Godbold and Solan, 2013; Hewitt et al., 2016).
Mussels and other reef-forming bivalves are among the most important habitat-forming and habitat-modifying species in aquatic systems, as they provide living space and favorable conditions for other species, facilitating a high biodiversity of associated species (Seed, 1996; Norling and Kautsky, 2007). They have a major influence on nutrient assimilation and recycling, energy flow and benthic-pelagic coupling due to their filtration activities (Kautsky and Evans, 1987; Newell, 2004). They are also an important food source for other invertebrates (e.g., crabs, sea-stars) and a range of bird species (e.g., oyster-catchers, gulls) and underpin both aquatic and terrestrial food webs (Paine, 1966; Prins et al., 1997; Norling and Kautsky, 2007). Given this importance, changes to ecosystem-level processes of bivalve-dominated assemblages caused by temporal and sequential regimes of multiple stressors have potentially broad implications for both aquatic and terrestrial systems alike.
Field experiments that deal with complex temporal regimes of stressors across multiple levels of biological organization are rare, due to logistical constraints. Here we present a fully factorial field-based experiment, using our field-based dosing system at Malahide Marina on the east coast of Ireland (Browne et al., 2016), which aims to (a) test if ecosystem properties are affected more by the cumulative effects (repeated doses) of single stressors or the combined effects of multiple stressors, (b) test if variable time-lags between doses of stressors affect the outcome, (c) characterize variation in responses with time after application of stressors, (d) determine if the sequential order of two stressors influences their combined effects, and finally (e) characterize variation in responses across different levels of biological organization.
Materials and Methods
Study Site and Experimental System
The model system for the current study was the epifaunal assemblage dominated by common mussels (Mytilus edulis), naturally occurring on the floating pontoons at Malahide marina, which is located in North county Dublin on the East coast of Ireland (Lat: 53° 27′ 12″ Long: 06° 9′ 5″). The assemblage included taxa such as macro-algae (e.g., Fucus vesiculosus, Enteromorpha intestinalis), ascidians (e.g., Didemnum maculosum, Ciona intestinalis), anthozoans (e.g., Actinia equina, Metridium senile), barnacles (e.g., Semibalanus balanoides, Balanus balanus) as well as mobile taxa such as gastropods (e.g., Patella vulgata, Rissoa sarsi), isopods (e.g., Idotea granulosa, Jaera albifrons), amphipods, nematodes, juvenile, and small crabs (e.g., Carcinus maenas, Porcellana platycheles), and a wide range of both sessile (e.g., Pomaterceros lamarcki), and mobile polychaetes (e.g., Cirratulus cirratus, Hedinista diversicolor). In total 64 species were identified in mussel dominated assemblages along the pontoons, however, the richness and abundance of species in each core did not vary significantly among plots along the length of the pontoons (Brooks et al., unpublished data, but also see Browne et al., 2016). The tidal range is 2–4 m and the salinity range is 18–34. When selecting experimental plots along the pontoons, a clear 13 cm wide corer marked to a depth of 15 cm was used to standardize the size of assemblages, only assemblages that were 15 cm in depth were selected and the surrounding assemblage (outside the core) was cleared using a scraper to a distance of 10 cm. The experiment ran from 22nd October until 10th December 2012 (for a total of 8 weeks).
Experimental Design
The experimental designs are described here as three subsets of treatments run simultaneously to meet the overall objectives of the experiment. Each subset of the experiment involved three factors: Treatment, Time-lag, and Sampling. The first two subsets were designed to address objectives (a), (b), and (c), comparing cumulative effects of the same stressor with effects of multiple stressors. In the first subset, metal (copper) was the primary stressor (i.e., the only stressor in the cumulative stressor regimes and the first one applied in the multiple stressor regimes). The factor “Treatment” had four levels; Metal-Metal (MM, double dose of metal), Metal-Biocide (MB, multiple stressor), No Pump was the un-manipulated control and Pump was the procedural control for pumping (i.e., the delivery method of the stressors). In the second subset, biocide was the primary stressor. The factor “Treatment” again had four levels; Biocide-Biocide (BB, double dose of biocide), Biocide-Metal (BM, multiple stressor), No Pump and Pump were the controls.
The third subset of treatments tested the effect of varying the sequential order of stressors—objective (d). The factor “Treatment” again had four levels: MB (multiple stressor with metal first), BM (multiple stressor with biocide first), No Pump, and Pump were the controls.
For all three subsets of the experiment, the factor “Time-lag” had three levels; None (no time-lag between stress events), Short (1 week between stress events) and Long (3 weeks between stress events) and the factor “Sampling” had two levels: Early (plots were sampled the day after final dosing) and Late (plots were sampled 2 weeks after final dosing). Time-lags between stressors and sampling regimes were determined based on evidence of sub-lethal effects, both in terms of duration and intensity of the individual stressors and in terms of the manifestation of effects over a given time period (see below for detailed rationale). All combinations of all treatments were independently replicated (n = 3).
To address objective (e), a range of response variables were measured for each subset of treatments and the variation in outcomes were assessed.
Rationale for Doses of Stressors, Time Lags, and Sampling Times
The two stressors tested in the experiment were copper (Metal) and chlorpyrifos (Biocide). Anthropogenic inputs of copper come from sources such as agriculture, sewage effluent, storm-water runoff (both agricultural and urbanized areas), mining (Makepeace et al., 1995; Srinivasan and Swain, 2007) and as a constituent of anti-fouling paints (Lewis, 1998). Copper's negative effects on a wide range of species are well-established (e.g., Johnston and Keough, 2000; Cartwright et al., 2006; Mayor et al., 2009). Organophosphates such as chlorpyrifos are widely used in both agriculture and marine settings (e.g., aquaculture and marinas) as non-species-specific biocides to control pests of crops, fouling assemblages, and parasites of fish (Lewis, 1998; Firbank et al., 2008).
The concentration of copper used was 420 μg l−1 and the concentration of chlorpyrifos was 230 μg l−1. This concentration is comparable to concentrations used in other studies (Pipe et al., 1999; Curtis et al., 2000; Roberts et al., 2006) and is representative of the mean value of copper found in stormwater throughout Europe (Bryan and Langston, 1992; Makepeace et al., 1995). The concentration of chlorpyrifos was 230 μgl−1 and this concentration has been shown to have sub-lethal impacts in similar systems (Guzzella et al., 1997; Serrano et al., 1997; Kim et al., 2004; US Environmental Protection Agency, 2009).
Time-lags between stressors and sampling regimes were determined based on evidence of sub-lethal effects, both in terms of duration and intensity of the individual stressors and in terms of the manifestation of effects over a given time period (Serrano et al., 1997; Pipe et al., 1999; Adams, 2005; Wu et al., 2005; Ashauer et al., 2006). Based on these studies, cellular effects of these particular stressors would be expected to be detectable within hours of exposure, whereas effects at higher levels of biological organization would be manifested at a scale of days to weeks. Information on likely recovery times between stress events was more difficult to obtain, but the short time lag was set with the expectation that recovery may be limited between stress events and the longer time lag was set with the expectation that some recovery would have taken place between stress events. This was based on empirical evidence which show expected induction/recovery times for both stressors (i.e., copper and chlorpyrifos). For example, induction of a biomarker response to both stressors is expected to occur within hours to a day, however those effects may take days to a couple of weeks to be manifested at a community or ecosystem-level; in addition, recovery is often correlated with duration of exposure (Adams, 2005; Wu et al., 2005; see Figure 1 for conceptual framework). Hence, a dosing and sampling schedule was conceived that would incorporate those expectations (Table 1).
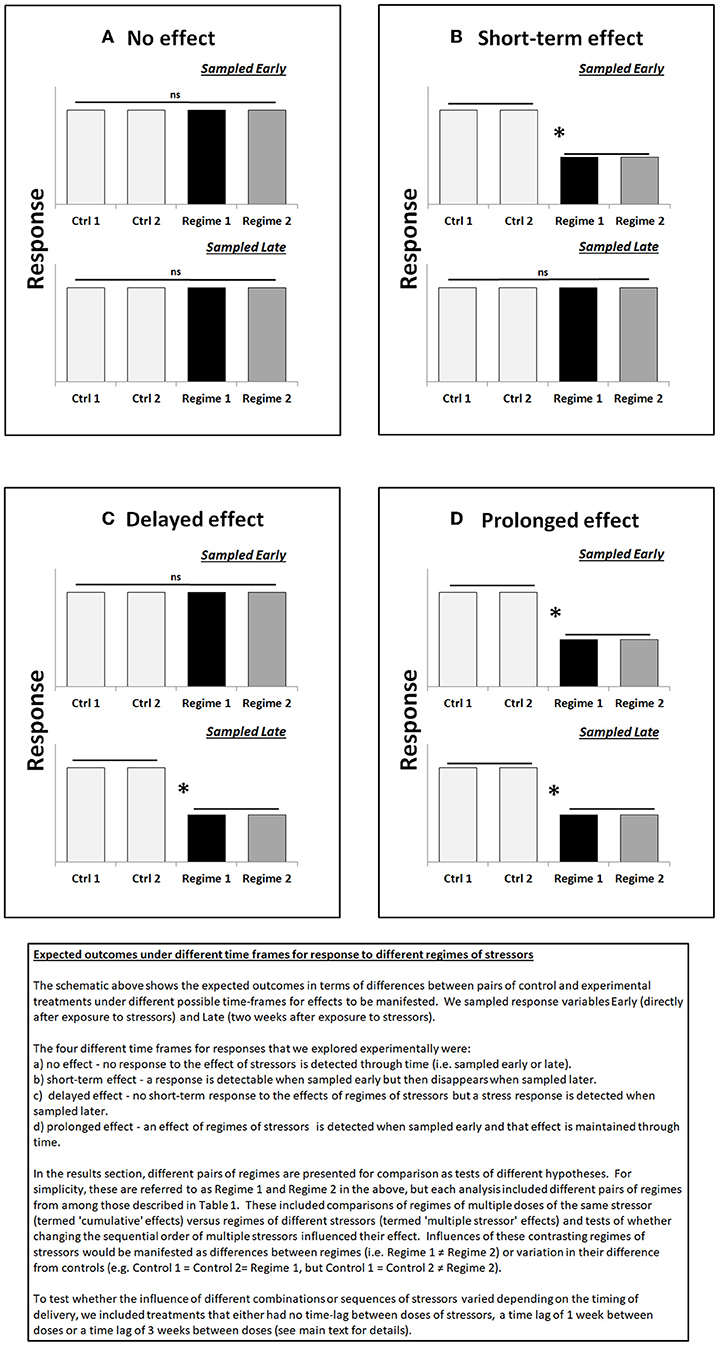
Figure 1. Overview of expected outcomes in time frames for response to different regimes of stressors tested experimentally during the study. (A) No effect. (B) Short-term effect. (C) Delayed effect. (D) Prolonged effect. Asterisks indicate significant differences between individual and/or groups of treatments.
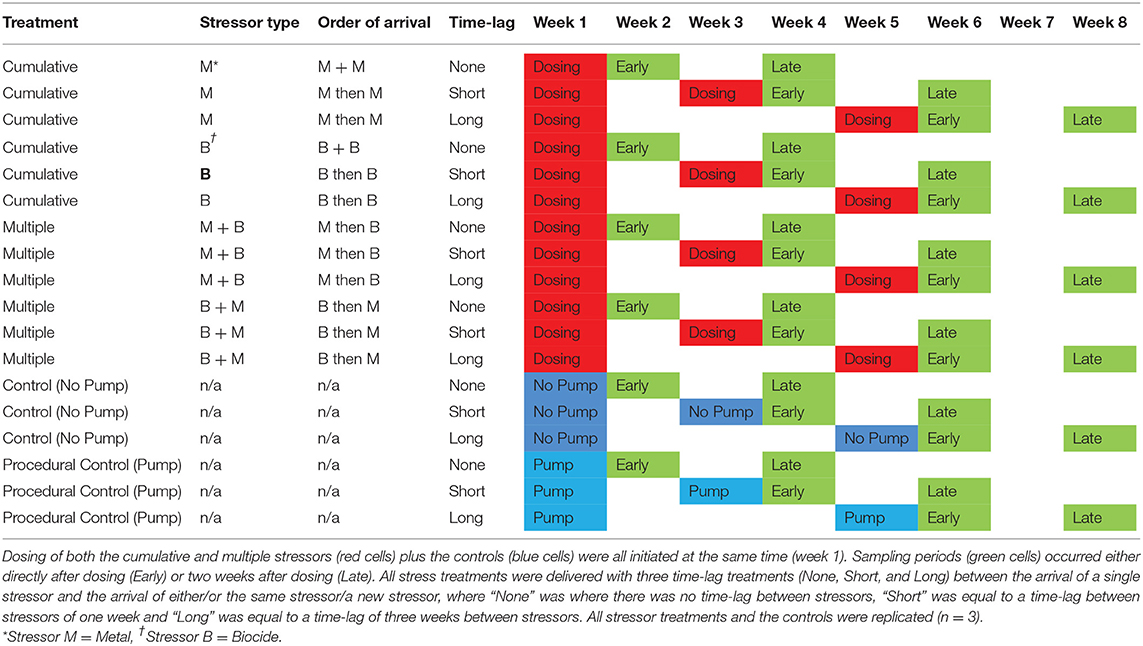
Table 1. Dosing and sampling schedule implemented over the duration of the experiment, which ran for a total of 8 weeks at Malahide.
Experimental Procedures
Experimental treatments were delivered using a programmable dosing system (Browne et al., 2016). Contaminants were fully mixed with seawater in 10 640 L containers and were delivered to experimental plots via a pump placed in each tank, controlled by digital timers. Liquid pumped from each container was split via a manifold into 10 separate 100 m lengths of tubing, each of which delivered stressors to individual plots (see Supplementary Material). Pilot studies had previously shown that 1 m was the minimum distance between plots to ensure independence (Browne et al., 2016). Plots in the current study were separated by 1.5 m. Each of the 18 treatments was randomly assigned to six replicate plots in the array. Three of the replicate plots were sampled directly after the final dosing of the assigned regime (“Sampled Early”) and the other three plots were sampled 2 weeks after final dosing (“Sampled Late”; see Table 1).
On the dates specified by the dosing and sampling schedule (Table 1), we quantified changes in the functioning of assemblages across different levels of biological organization (viability of haemocytes of mussels, community respiration, and the clearance rates of mussel assemblages).
On the day after final dosing, three mussels were collected from each plot for analysis of cellular viability. Cellular viability was estimated using the Neutral Red assay, which provides a quantitative estimation of the number of viable cells in a culture and tests the ability of viable cells to incorporate and bind neutral red dye in the lysosomes (see Brown et al., 2004). From each mussel an aliquot of haemolymph (50 μl) was pipetted into each of two wells of microtitre plates and agitated using a plate shaker (1,400 rpm for 60 s). To allow cells to adhere at the bottom of the wells plates were then left for 50 min to incubate. After the incubation period, the plates were washed with phosphate buffer solution to remove excess cells. Neutral red dye (0.4%) was then added. To prevent photolysis of cells, plates were then incubated in the dark for 3 h. Subsequently wells were then washed with phosphate buffer solution before an acidified solution of 1% acetic acid/20% ethanol was added to resolubilize the dye. Plates were analyzed using a spectrophotometer and absorbance was read at 550 nm. The total protein in the haemolymph was determined and results were presented as optical density per gram of haemocyte protein.
Community respiration (CR) was assessed as oxygen uptake in the dark by placing an opaque purpose built chamber (see Supplementary Material) over the assemblage and using a hand-held probe (HQ20 Hach Lange Ltd. portable LDO™, Loveland, USA) to measure changes in the concentration of dissolved oxygen (mg O2 L−1) over a period of time. To ensure that measurements were taken at the correct time points, a linearity test was undertaken: dissolved oxygen concentration was measured every 2 min over an hour to identify the period during which there was a linear decrease of oxygen concentration in the water. On the basis of this test, oxygen measurements to estimate CR were taken after 10 and 20 min. The initial 10 min as defined by the linearity test has been shown to allow for acclimatization of the assemblages and to ensure photosynthesis had ceased after covering with an opaque chamber (Noël et al., 2010). The chamber was also fitted with a pump to ensure water circulation and homogenization of oxygen concentration throughout the solution. Rates of oxygen uptake were estimated using the equation; CR = Δ [O2] dark/Δt dark, where CR is Community respiration, Δ [O2] dark is the difference in dissolved oxygen concentration between measurements taken, respectively, at the beginning and end of the dark period and Δt dark is the time difference between these measurements. CR was derived on a per hour basis for each individual plot and expressed as mg O2 L−1 h−1 (Nielsen, 2001; Noël et al., 2010).
Clearance rates of micro-algae from the water were measured in-situ using the same purpose-built chamber. Five milliliter of a solution containing microalgae (Isocrysis galbana) at a concentration of 12–15,000 cells per 0.5 ml−1 was injected into the chamber and a circulation pump was used to ensure that they remained suspended. Twenty milliliter samples of seawater were taken from the chamber at three time intervals: 0 (T0), 15 (T1), and 30 (T2) min. The numbers of particles retained in samples were counted using flow-cytometry and clearance rates calculated as the change in concentration per unit time using the following equation; Clearance rate = V (loge C1 – loge C2)/t, where V is the volume of water in the chamber and C1 and C2 are the algal concentration at the beginning and end of the time interval (t) (Coughlan, 1969; Canty et al., 2007).
Data Analysis
Data were analyzed with two series of analyses of variance (ANOVAs), structured to include tests for differences between the controls and different subsets of the stressor treatments to address the objectives of the study. The first series of analyses tested for differences in the outcome based on whether the assemblage was exposed to cumulative regimes (repeated doses) of the same stressor and/or comparable combinations of multiple stressors. Separate analyses were done for each of the first two subsets of treatments described above and for each of the three variables measured (i.e., cellular viability, community respiration and clearance rate). The second series of analyses involved the same factors as the first, but the objective was to test whether the sequential order of the multiple stressor treatments caused differences in the outcome. The final analyses were based on the third subset of treatments described above and again a separate analysis was done for each of the variables measured. In all analyses, all three factors (Treatment, Time-lag, and Sampling) were fixed and orthogonal and n was 3. In all analyses the factor “Treatment” had four levels, the factor “Time-lag” had three levels and the factor “Sampling” had two levels.
A supplementary analysis was completed to test for possible confounding effects due to variation in the timing of different treatments across the duration of the experiment (see below for details).
All computations were done using the software package WinGmav5 (Underwood et al., 1998). Cochran's test was used to test for homogeneity of variances. When necessary data were transformed (e.g., log transformed; Underwood, 1996). Significant results of ANOVAs were further tested using the SNK (Student Newman Keuls) post-hoc tests, to determine which means differed from each other.
Testing for Differences in Controls Over Time
Different time lags and delays in sampling led to samples being collected at different times throughout the 8 week period. At each time of sampling experimental treatments, un-manipulated controls and procedural controls were also sampled, ensuring valid comparisons at each time. We recognize, however, that assemblages and the influence of treatments on assemblages and ecosystem processes may have changed during the 8 week period of the experiment, potentially confounding comparisons between the effects of different stress regimes relative to controls. It was not logistically feasible to run all treatments such that all regimes could be initiated and sampled at the same times, which would have enabled this concern to be fully addressed. However, we did sample controls (i.e., natural assemblages—No Pump) at each of the sampling times, enabling us to assess whether the ecosystem processes of the natural assemblage varied during the 8 week period which was incorporated into each analysis. A separate analysis was done to test for variation in response in these control plots. It involved the factors “Control type” and “Sampling Event,” the factor Control type had two levels (No Pump and Pump) and was included to test if there were any artifacts in the experimental design pertaining to the pumping of the dosing system both within or between the natural mussels/assemblages (No Pump) and the mussels/assemblages which were exposed to pumped seawater (Pump). The factor “Sampling Event” had six levels (i.e., six different sampling events) and was included to test if there was any temporal variation in ecosystem processes over the duration of the experiment. Both factors were fixed and orthogonal and all controls were replicated (n = 3).
Results
Cellular Viability
When copper was the primary stressor, there was no effect of stressor treatments on the cellular viability of mussels that had no time-lag between stressors and were sampled early (i.e., directly after dosing) (Figure 2A). However, when mussels were sampled 2 weeks after final dosing, both stressor treatments reduced cellular viability in comparison with the controls (Figure 2B, Table 2a, SNK: MM = MB > No Pump = Pump, P < 0.05). The same outcome was observed when a short time-lag was introduced between the stressors, but the effect was only apparent when assemblages were sampled 2 weeks after dosing. When a long time-lag of 3 weeks between stressors was introduced, both stressor treatments at both sampling points had an effect on the cellular viability of the dosed mussels in comparison with the controls, but again there were no differences between the cumulative or the multiple stressor treatments (Figures 2A,B, Table 2a, SNK: MM = MB > No Pump = Pump, P < 0.05). Overall the analysis showed a significant difference between the controls and the two stressor treatments such that when there was a time-lag between stressors there was a greater effect than when mussels were exposed to the stressors together (Figures 2A,B, Table 2a, SNK: Long = Short > None, P < 0.01).
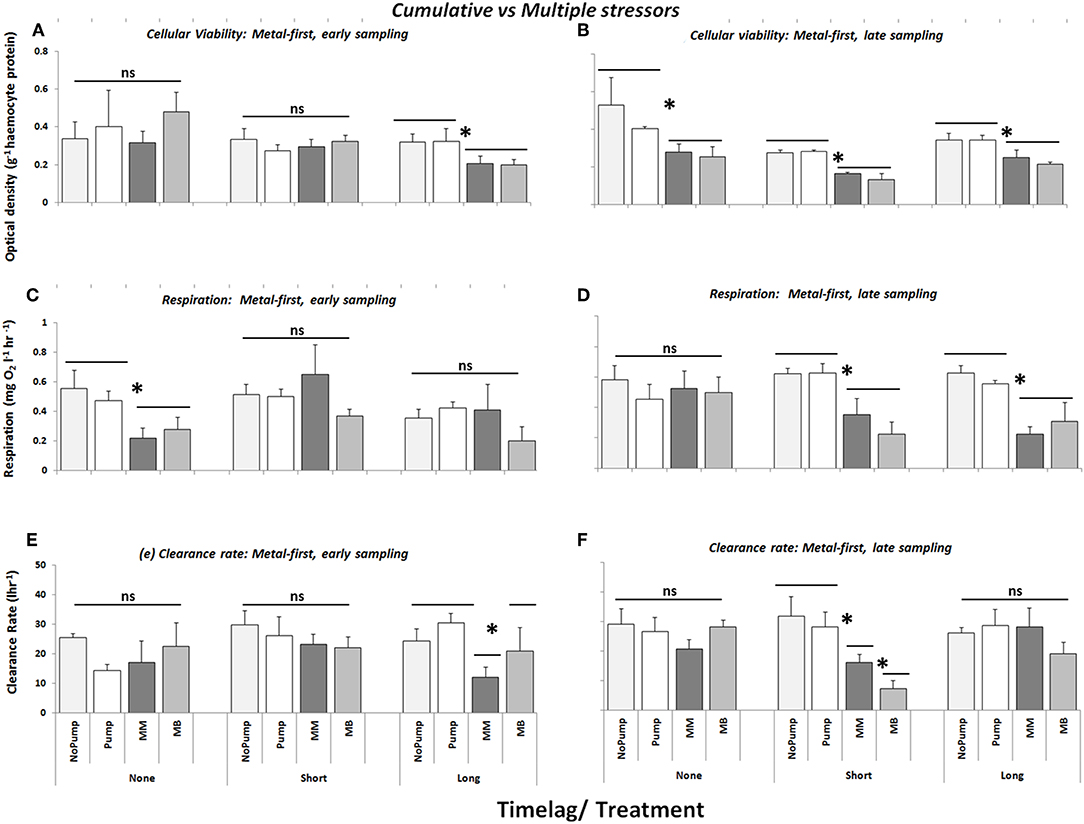
Figure 2. Cellular viability (optical density g−1 haemocyte protein), respiration (mg O2 l−1 hour−1) and clearance rate (l/hour) of mussels/mussel assemblages at Malahide marina with metal as the primary contaminant in cumulative (MM) and multiple stressor treatments (MB), M = Copper and B = Biocide. Also shown are the control treatments: “No Pump” (un-manipulated control) and “Pump” (procedural control). All stress treatments were delivered with three time-lag treatments (None, Short, and Long) between the arrival of the first stress event and the second stress event and were sampled directly after dosing (Early–A,C,E) and 2 weeks after dosing (Late–B,D,F) (n = 3). Asterisks indicate significant differences between individual and/or groups of treatments.
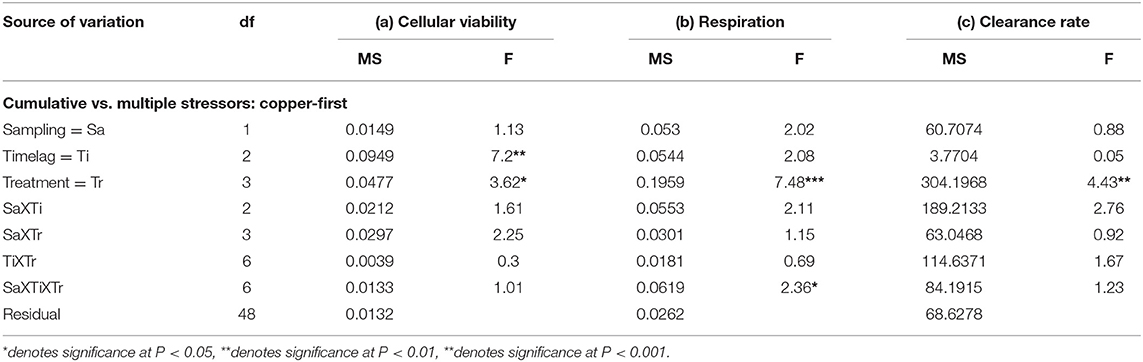
Table 2. Analyses of the (a) cellular viability (optical density g−1 haemocyte protein), (b) community respiration (mg O2 l−1 h−1), and (c) clearance rates (l/h) of mussels/mussel assemblages at Malahide marina comparing cumulative and multiple stressor treatments with copper as the primary stressor, corresponding to the first and second subsets of treatments described in the Materials and Methods (n = 3 plots; for all variables: data are untransformed; Cochran's test: ns).
When biocide was the primary stressor, a long time-lag between stressors caused greater effects on cellular viability than a short time-lag or no time-lag (Figures 3A,B, see Table 3a, SNK: Long > Short = None, P < 0.01). When mussels were sampled early after dosing, only the multiple stressor treatment (BM) had an effect on cellular viability in comparison with the cumulative stressor and the controls (Figures 3A,B, see Table 3a, SNK: BM > BB = No Pump = Pump, P < 0.05). When sampled 2 weeks after dosing both stressors treatments had an effect, which was significantly different from that of the controls but the stressor treatments did not differ from each other (Figure 3B, see Table 3a, SNK: BM = BB > No Pump = Pump, P < 0.01).
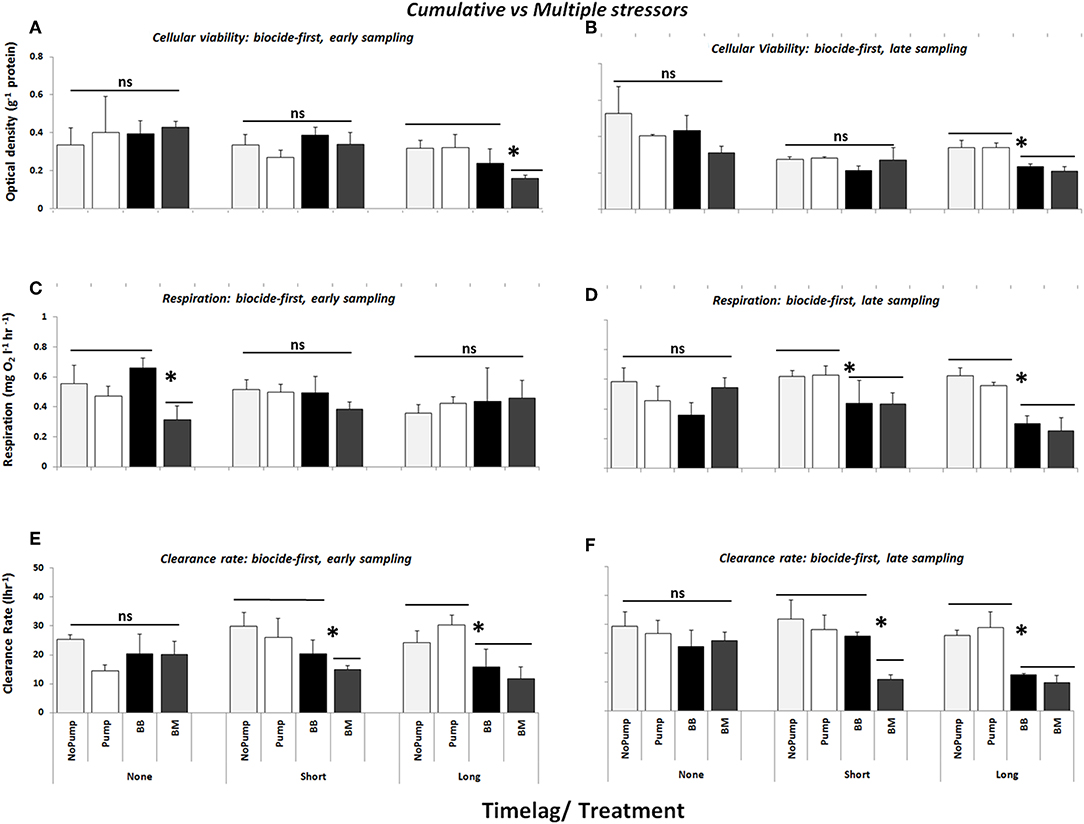
Figure 3. Cellular viability (optical density g−1 haemocyte protein), respiration (mg O2 l−1 hour−1) and clearance rate (l/hour) of mussels/mussel assemblages at Malahide marina with biocide as the primary contaminant in cumulative (BB) and multiple stressor treatments (BM), M = Copper and B = Biocide. Also shown are the control treatments: “No Pump” (un-manipulated control) and “Pump” (procedural control). All stress treatments were delivered with three time-lag treatments (None, Short, and Long) between the arrival of the first stress event and the second stress event and were sampled directly after dosing (Early–A,C,E) and 2 weeks after dosing (Late–B,D,F) (n = 3). Asterisks indicate significant differences between individual and/or groups of treatments.
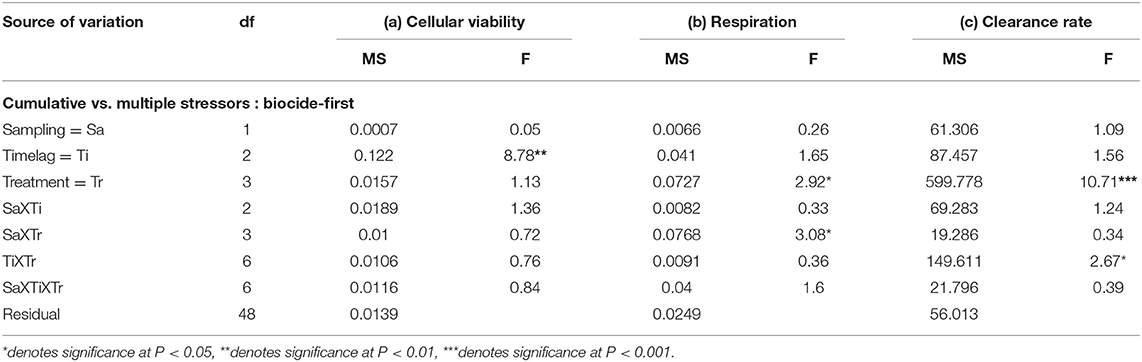
Table 3. Analyses of the (a) cellular viability (optical density g−1 haemocyte protein), (b) community respiration (mg O2 l−1 h−1), and (c) clearance rates (l/h) of mussels/mussel assemblages at Malahide marina comparing cumulative and multiple stressor treatments with biocide as the primary stressor, corresponding to the first and second subsets of treatments described in the Materials and Methods (n = 3 plots; for all variables: data are untransformed; Cochran's test: ns).
No significant differences in cellular viability were caused by varying the sequential order of stressors (Figures 4A,B, Table 4a). Instead, these analyses confirmed the importance of time-lags in enhancing the effects of multiple stressors on cellular viability (Figures 4A,B; Table 4a, SNK: Long = Short > None, P < 0.01).
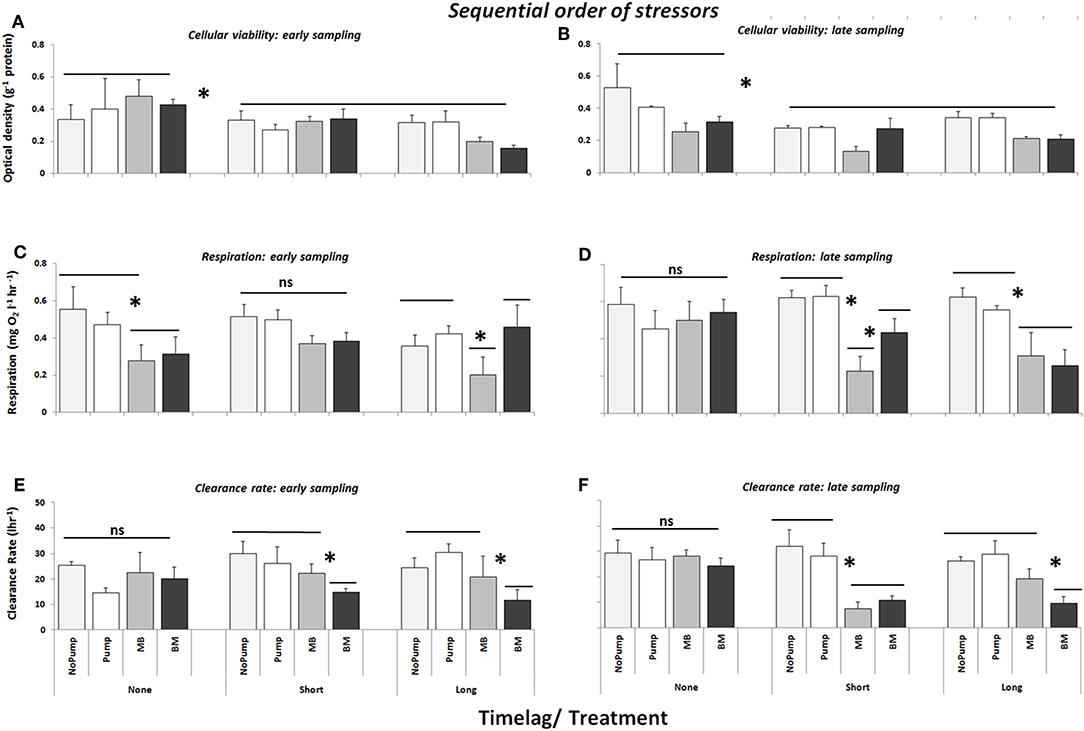
Figure 4. Cellular viability (optical density g−1 haemocyte protein), respiration (mg O2 l−1 hour−1) and clearance rate (l/hour) of mussels/mussel assemblages at Malahide marina testing the sequential order of arrival of multiple stressors (i.e., MB vs. BM, where B = Biocide and M = Copper). Also shown are the control treatments: “No Pump” (an un-manipulated control) and “Pump” (a procedural control). All treatments were subject to three time-lag treatments (None, Short and Long) between the arrival of the first stress event and the second stress event and were sampled directly after dosing (Early–A,C,E) and 2 weeks after dosing (Late–B,D,F) (n = 3). Asterisks indicate significant differences between individual and/or groups of treatments.
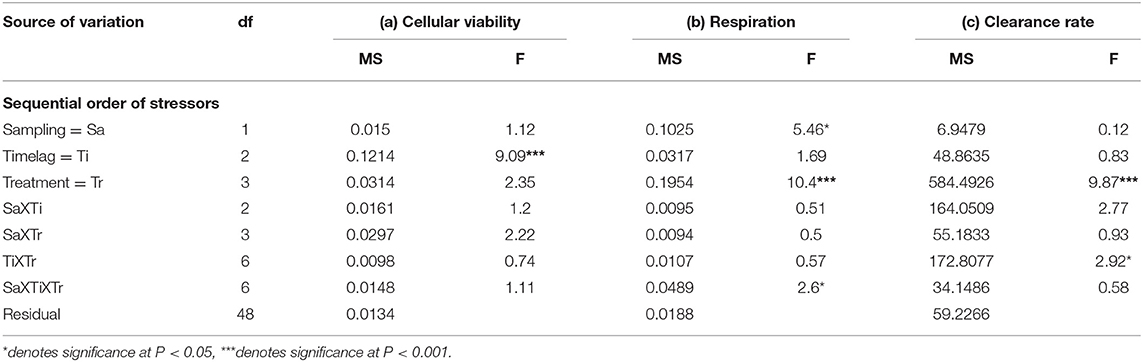
Table 4. Analyses of the (a) cellular viability (optical density g−1 haemocyte protein), (b) community respiration (mg O2 l−1 h−1), and (c) clearance rates (l/h) of mussels/mussel assemblages at Malahide marina testing the influence of the sequential order of arrival of multiple stressors—the third subset of treatments described in the Materials and Methods (n = 3 plots; data are untransformed; Cochran's test: ns).
Respiration
When copper was applied first, the community respiration of assemblages exposed to both stressor combinations with no time-lag between each dose and sampled directly after dosing (Early) was significantly greater than that in the control treatments (Figure 2C, Table 2b, SNK: MM = MB > No Pump = Pump, P < 0.05). However, when these assemblages were sampled 2 weeks later (Late), that effect had disappeared (Figure 2D). Assemblages for which there was a short time-lag between stressors and had significantly reduced community respiration when sampled late than when sampled early (Figures 2C,D, Table 2b, SNK: Short: Late > Early, P < 0.05) but there was no difference between the stressor treatments (Figures 2C,D, Table 2b, SNK: Short: MB = BM > No Pump = Pump, P < 0.05). The same effect occurred when a long time-lag was introduced between the stressors: there was no effect when sampled directly after dosing (Figure 2C, Table 2b, SNK: Long: Late > Early, P < 0.05) but an effect was manifested 2 weeks after dosing (Figure 2D, Table 2b, SNK: Long: MB = BM > No Pump = Pump, P < 0.05).
When biocide was the primary stressor, community respiration was significantly less under cumulative doses of the same stressor (BB) than under multiple stressors or controls, but this difference was again dependent on the sampling time (see Figures 3C,D). On average across all time-lag treatments sampled 2 weeks (Late) after final dosing, the community respiration of mussel assemblages was significantly reduced by the cumulative biocide treatment (Figure 3D, Table 3b, SNK: BB: Late > Early, P < 0.05) in comparison with the controls and the multiple stressor treatment.
The effect of the sequential order of delivery of stressors varied depending on the time-lag between stressors and when they were sampled. For example, there was an effect on the community respiration rates of mussel assemblages exposed to both stressor treatments when there was no time-lag between stressors and when community respiration was sampled directly after dosing, regardless of sequential order (Figure 4C, Table 4b, SNK: None: Early > Late, P < 0.05) but that effect had disappeared 2 weeks later (Figure 4D, Table 4b, SNK: Early: MB = BM > Pump = No Pump, P < 0.05). When a short time-lag was introduced between treatments both stressor treatments had an effect in the short term (i.e., when sampled early) in comparison to the controls, but there was no difference between them (Figure 4C, Table 4b, SNK: Early: MB = BM > Pump = No Pump, P < 0.05). On the other hand, when sampled 2 weeks after dosing (Late), again they were both different from the controls but the effect was greater when metal were delivered first than when biocides were delivered first (Figure 3D, Table 4b, SNK: Late: MB > BM > Pump = No Pump, P < 0.01). Furthermore, when a long time-lag was introduced between treatments, there was only an effect in the short term when metal were delivered first (Figure 4C, Table 4b, SNK: Early: MB > BM = Pump = No Pump, P < 0.01) but when assemblages were sampled 2 weeks after dosing (Late) the effect was manifested in both stressor treatments (Figure 4D, Table 4b, SNK: Late: MB > BM > Pump = No Pump, P < 0.01).
Clearance Rates
With metal as the primary stressor, there was no effect on clearance rate when there was no time-lag between stressors. An effect was detected, however, when a short time-lag was introduced, but this effect only occurred when the assemblages were sampled 2 weeks after the last dosing, at which point both stressor treatments differed from the control treatments, but the multiple stressor treatment had a greater effect than the cumulative stressor treatment (Figures 2E,F, Table 2c, SNK: Short, Late: MB > MM > No Pump = Pump, P < 0.05). When there was a long (3 weeks) time-lag between stressors, an effect of the cumulative stressor treatment was only detected in the short-term, 1 day after dosing (Figure 2E, Table 2c, SNK: Long, Early: MM > MB = No Pump = Pump, P < 0.05).
For mussel assemblages exposed first to biocide (BB and BM), an effect on clearance was only detected for those assemblages that were exposed to the multiple stressor treatment (BM) and subject to a time-lag (either “Short” or “Long”) (Figures 3E,F, Table 3c, SNK: BM: Short = Long > None, P < 0.05). For example, when mussel assemblages were exposed to a short time-lag there was a greater reduction in the clearance rates of the assemblages exposed to the multiple stressor treatment, which in turn differed from both the cumulative stressor and the control treatments (Figures 3E,F, Table 3c, SNK: Short: BM > BB = NP = P, P < 0.01). Concomitantly, when there was a long time-lag between stress events there were no differences between the clearance rates of mussels subjected to different sets of stressors, but both stressor treatments did again differ from the controls (Figures 3E,F, Table 3c, SNK: Long: BM = BB > NP = P, P < 0.01).
The effect of sequential order of stressors on clearance rates was dependent on whether there was a time-lag between the individual stressors. When there was a short time-lag between treatments, there was no difference between the stressor treatments but both were significantly different from the controls (Figures 4E,F, Table 4c, SNK: Short: BM = MB > NP = P, P < 0.01). Conversely, when there was a long time-lag, clearance rates in assemblages which were exposed to biocide before metal (BM), were more strongly affected (i.e., had a more greatly reduced clearance rate) than those exposed to copper before biocide (MB) and to the controls (Figures 4E,F, Table 4c, SNK: Long: BM > MB = NP = P, P < 0.01). Overall when biocides were introduced first (BM) there was only a significant reduction of the clearance rate of the assemblages when there was a time-lag between the stressors (Figures 4E,F, Table 4c, SNK: BM: Short = Long > None, P < 0.01).
Variation in Controls During the Experimental Period
In terms of the controls, there were no differences in the cellular viability of mussels either between or within both the procedural and the un-manipulated in the controls over the duration of the experiment (Figure 5A, Table 5a). The respiration of mussel assemblages was not different between the control types (i.e., the procedural and the un-manipulated controls) but there were differences between the sampling events (Figure 5B, Table 5b), however the post-hoc analysis was unable to unravel which means were different. Additionally, there were no differences in the clearance rates of mussel assemblages in both control types nor were any differences between sampling events over the duration of the experiment (Figure 5C, Table 5c).
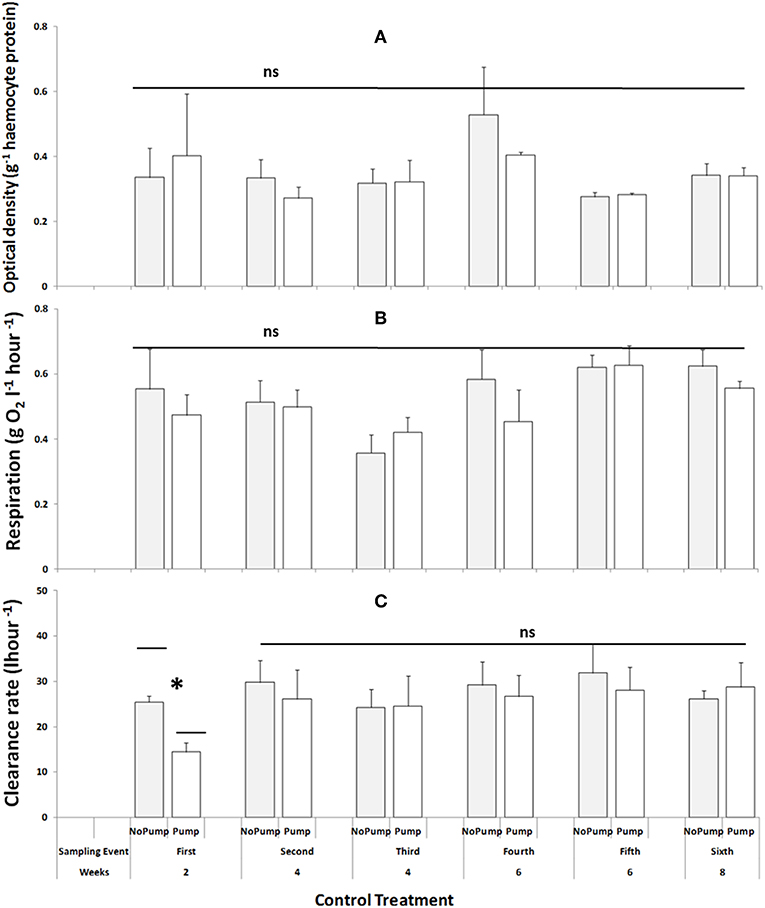
Figure 5. Control treatments sampled over the duration of the experiment at Malahide marina for all three response variables; (A) Cellular viability (optical density g−1 haemocyte protein) of mussels, (B) Respiration (mg O2 l−1 hour−1) and (C) Clearance rate (l/hour) data of mussel assemblages. Data shown includes both control treatments: “No Pump” (an un-manipulated control) and “Pump” (a procedural control). All treatments were subject to six sampling events over the duration of the experiment, which ran for a total of eight weeks (n = 3 plots; data are untransformed; Cochran's test: ns). Asterisks indicate significant differences between individual and/or groups of treatments.
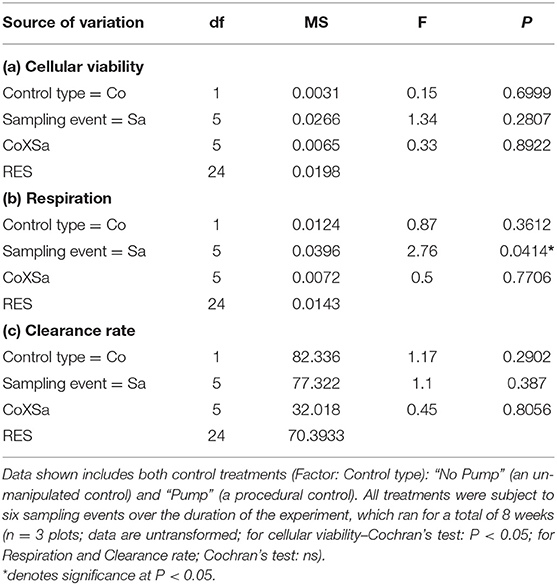
Table 5. Analysis of control treatments sampled over the duration of the experiment for (a) cellular viability (optical density g−1 haemocyte protein) data, (b) Community respiration (mg O2 l−1 h−1) data, and (c) Clearance rate (l/h).
Discussion
A key finding from this study was that, in contrast to current expectations, we found clear evidence that time-lags between stressors often increased their effects. For example, here we found that mussels exposed to stressors with short or long time-lags between stress events had decreased cellular viability in comparison to when there was no time-lag between stressors. The influence of time-lags on the effects of stressors also occurred for both measures of ecosystem functioning but how that was manifested varied between the measures and was dependent on the identity of the primary stressor: for community respiration, time-lags between stressors enhanced their effect when copper was the primary stressor but not when biocide was the primary stressor. In contrast, time-lags between stress events only enhanced the effect of stressors on clearance rate when biocide was the primary stressor.
The regime of stressors/disturbances has long been seen to be a key determinant of their impact on a given system (Bender et al., 1984; Sousa, 1984; Benedetti-Cecchi et al., 2000; Benedetti-Cecchi, 2003; Bertocci et al., 2005). Our results for effects of cumulative (or repeated) occurrences of a single stressor support that view but also highlight that the regimes of multiple stressors or time-lags between stress events are also important. Others have also reported that temporal patterns of multiple stressors can alter their effects (Hughes and Connell, 1999; Molinos and Donohue, 2010; Bennett et al., 2015) but none have shown that time-lags between stressors can increase their effect, as revealed in the current study. Given that, the intensity and duration of stress events is becoming all the more relevant as we are faced with a future of unpredictability, both in terms of the temporal variability in extreme climatic events (Easterling et al., 2000; IPCC, 2012) and in terms of how ecosystems might respond out of proportion to the duration or intensity of those extreme events (Easterling et al., 2000; Jentsch et al., 2007; Walter et al., 2013), it is important that this be taken into consideration.
In fact, temporal gaps in disturbance regimes of stressors have been identified as one possible pathway for the development of cross-tolerance (positive or negative) and stress-hardening in organisms (Vinebrooke et al., 2004; Kültz, 2005; Todgham et al., 2005). If there is interdependence between either biochemical or physiological processes within an organism, organisms can use the same processes to deal with subsequent stressor events (stress-hardening) or deal with different stressors (cross-tolerance), but again the likelihood of these tolerances developing may be dependent on the status of the organism, such as level of recovery and/or synthesis of stress-proteins. For example, Todgham et al. (2005) showed that cross-tolerance only occurred between thermal and hyperosmotic stress in tide-pool sculpins (fish), when either sufficient time between stressors had passed to allow for recovery or when they were pre-exposed to either stressor. Similar mechanisms have been reported in plants after short duration stress events (e.g., drought, frost, nutrient deficiency, etc.) where exposure to subsequent stress invokes a “memory” of that first event, thus allowing the plant to respond more rapidly using the same mechanisms as used previously (Walter et al., 2011, 2013; Kreyling et al., 2012a,b). In the current study, the findings were generally consistent with negative cross-tolerance between stressors (Lloret et al., 2004; Mueller et al., 2005; Kreyling et al., 2011), in that time-lags had a tendency to increase the effect of certain stressors.
It is possible that in the current study the duration of exposure to each particular stress event and/or the time-lag between stress events was not sufficiently long to allow development of tolerance or hardening to the next stress event. Indeed, other studies have shown that the long-term effects of climate driven multiple stressors may vary depending on season (Godbold and Solan, 2013) and although this was not tested in the current study, it may have been that the responses observed could have been influenced by seasonal variation. However, the time-lags of both cause and effect we used were based on evidence of sub-lethal effects, both in terms of duration and intensity of the individual stressors and manifestation of effects over a given time period (see Table 1 and rationale in section Materials and Methods). Other studies have shown effects using similar concentrations and even shorter exposure times (Curtis et al., 2000; Roberts et al., 2006). The sampling regimes were based on evidence from the literature that led us to expect cellular responses to occur within hours to a day after exposure and ecosystem-level responses to be manifested after hours to days to weeks (Adams, 2005; Wu et al., 2005; Supplementary Material). In fact our results here show that effects across all variables can be transient: in some cases, an effect was detected the day after final exposure which was no longer detectable 2 weeks later. Exposure to stressors is often an intermittent event (Ward, 1987) as stressors can arrive into a system episodically (Breitburg et al., 1998; Paine et al., 1998; Hughes and Connell, 1999; Adams, 2005). With climatic change (Easterling et al., 2000; IPCC, 2012), the temporal patterns of terrestrial run-off are likely to change, with increased rainfall and storminess, thereby potentially changing the intensity and sequence of arrival of local stressors (such as those tested in the current study) into aquatic systems (Adams, 2005; Schiedek et al., 2007), with consequences that require further research of the kind reported here.
Our findings clearly show the importance of the sequential order of multiple stressors in determining the strength of their effect on the functioning of mussel assemblages. The sequence of stressors had no effect at a cellular-level (Figures 3A,B), but there was a strong influence of the identity of the first stressor on outcomes in terms of each measure of ecosystem functioning. For example, community respiration was most affected when copper was applied first, but clearance rate was more greatly reduced when biocides were applied first. That unique link between each functional measure and stressor identity is most likely due to the mode of action of each individual stressor. For example, for copper its toxicity is related to its capacity to reduce oxygen binding to haemolymph cells (thereby inducing hypoxia) and to increase permeability of cellular membranes in invertebrate species (Pipe et al., 1999; Taylor and Anstiss, 1999). On the other hand, the biocide used (chlorpyrifos) enters marine organisms through external membranes during feeding and respiration (Serrano et al., 1997) and its direct mode of action is to affect nerve synapses, which can result in loss of muscle function (Key and Fulton, 1993; Kim et al., 2004). Loss of muscle function would impair the ability of an organism to feed in which may explain the reduced clearance rates of the affected assemblages in the current study. Indeed, similar results were also reported for the effects of multiple chemical stressors on a sea-grass species (Zostera capricorni) in Australia (Macinnis-Ng and Ralph, 2004). In contrast to our results they found that double doses of each individual stressor had a greater effect than sequential doses of different stressors. (Nystrom and Folke, 2001), on the other hand, found that individual stressors (heat and copper) had less impact alone than when they were combined in a particular sequence on a coral species. Similar effects have been shown for combinations of biotic and abiotic stressors, such as grazing and pH (Flöder and Hillebrand, 2012) or predation and invasion (Fukami, 2001). Flöder and Hillebrand (2012) found that certain sequences of two stressors (grazing and pH) had greater effects on the stability of phytoplankton communities but that effect was dependent on both the species richness of the assemblage and on whether the most dominant species was present in the mixtures, concepts that have also been explored elsewhere (e.g., Blake and Duffy, 2010; O'Connor and Donohue, 2013).
While most empirical studies on the sequential order of stressors have been conducted in aquatic settings, the sequential arrival of stressors in terrestrial ecosystems can also be a potential determinant in terms of compounding the effects of single disturbances over a temporal period (Dale et al., 2001). In grasslands, exposure to drought before frost is less detrimental than the reverse sequence, because drought leads to a higher rate of synthesized sugars thus reducing frost effects (Kreyling et al., 2012a). Furthermore, synthesis of heat-shock proteins in plants exposed to heat stress first can reduce the effects of subsequent exposure to heavy metals (Sabehat et al., 1998). Another study revealed that tomato fruits exposed to moderately high temperatures first (38°C) can withstand lower temperatures (2°C) and not develop chilling injuries but this does not occur when the sequence is reversed (Lurie and Klein, 1991).
In the current study we found evidence of variation in response to different stressors across the levels of biological organization we measured. In general the effects at the cellular level could not be used to predict effects at the ecosystem level (community respiration and clearance rate). This is somewhat counter-intuitive given that this assay is used as a biomarker of effect, with the expectation that it is an early warning system on the basis that the effect is first developed at the cellular level but then is translated or propagated up to higher levels of organization (Widdows and Donkin, 1992; Coles et al., 1995; Pipe et al., 1999). These findings highlight the importance of using multi-functional measures across different levels of biological organization in order to qualify the effects of stressors, and are in agreement with other authors (Attrill and Depledge, 1997; Adams, 2005; Smith et al., 2009; Martinez-Crego et al., 2010). In fact a long-term grassland experiment (covering 5 years in total) looking at the effects of repeated drought events, measured 32 parameters, which were categorized into five key ecosystems functions and concluded that without evaluating different functional measures across various parameters there is increased potential to under represent the actual effects of a stressor or disturbance (Jentsch et al., 2011). Indeed, a focus on individual response variables as independent measures of ecosystem function can prove to be misleading, in terms of identifying causative drivers of change in ecosystem processes (Hooper and Vitousek, 1998; Bracken and Stachowicz, 2006) such that deploying resources to reduce effects could be misguided and ineffective (Adams, 2005).
Conclusion
Multiple stressors affect many, if not all, terrestrial and aquatic systems to some extent (Harley et al., 2006; Darling and Cote, 2008; Halpern et al., 2008; Piggott et al., 2015). Quantifying the way in which ecosystems act in response to a multitude of anthropogenic stressors is an important priority for those concerned with preserving biodiversity, ecosystem functioning, and the services that ecosystems provide to humanity (Adger, 2006; Crain et al., 2008; Crowe et al., 2015). The effects of multiple stressors can be extremely variable (Strain et al., 2014; Piggott et al., 2015; Côté et al., 2016; Brooks and Crowe, 2018) and that variability can be due to range of interacting ecological and environmental factors, such as the identity of the stressors (Crain et al., 2008), their spatial extent (Russell et al., 2009) or the identity of the species/assemblage/ecosystem being affected (Vinebrooke et al., 2004; Breitburg and Riedel, 2005; Lyons et al., 2015; Jackson et al., 2016; Brooks and Crowe, 2018). Whilst this experiment was only conducted across relatively small temporal and spatial scales it is the first experiment of that we know to test the effects of sequential and short-term temporal variability of dosing by two stressors across different levels of biological organization on naturally occurring assemblages in the field. Our findings highlight the importance of including the potential effects of the relative timing and sequential order of cumulative and multiple stressors into management strategies. Although the management of cumulative and multiple stressors is already complex, the inclusion of these concepts may aid in reducing misdiagnosis of effects of multiple stressors. Understanding which combinations, sequences and time lags of stressors will have the greater impact could provide managers with a basis for decisions that could control and/or modify the sequence of arrival of multiple stressors to minimize the net impact on the system, which is crucial for the effective management, restoration and preservation of ecosystems (Burton and Johnston, 2010).
Author Contributions
PB and TC conceived and designed the study and gave final approval for publication. PB carried out the experiment and statistical analyses and wrote the first draft of the manuscript. TC contributed greatly to the final version.
Funding
PB was supported by the UCD Graduate Research Education Programme in Sustainable Development (funded by IRCSET and IRCHSS).
Conflict of Interest
The authors declare that the research was conducted in the absence of any commercial or financial relationships that could be construed as a potential conflict of interest.
Acknowledgments
We thank Damian Offer and staff at Malahide Marina for permission to do field experiments within the marina. For lab and field assistance, thanks to Jen Coughlan and members of the MarBEE group.
Supplementary Material
The Supplementary Material for this article can be found online at: https://www.frontiersin.org/articles/10.3389/fevo.2019.00387/full#supplementary-material
References
Adams, S. M. (2005). Assessing cause and effect of multiple stressors on marine systems. Mar. Pollut. Bull. 51, 649–657. doi: 10.1016/j.marpolbul.2004.11.040
Adger, W. N. (2006). Vulnerability. Glob. Environ. Change 16, 268–281. doi: 10.1016/j.gloenvcha.2006.02.006
Ashauer, R., Boxall, A., and Brown, C. (2006). Predicting effects on aquatic organisms from fluctuating or pulsed exposure to pesticides. Environ. Toxicol. Chem. 25, 1899–1912. doi: 10.1897/05-393R.1
Assessment, M. E. (2005). Ecosystems and Human Well-Being: Wetland and Water. Synthesis. Available online at: http://www.millenniumassessment.org/proxy/ (accessed May 10, 2016).
Attrill, M. J., and Depledge, M. H. (1997). Community and population indicators of ecosystem health: targeting links between levels of biological organisation. Aqua. Toxicol. 38, 183–197. doi: 10.1016/S0166-445X(96)00839-9
Bender, E. A., Case, T. J., and Gilpin, M. E. (1984). Perturbation experiments in community ecology: theory and practice. Ecology 65, 1–13. doi: 10.2307/1939452
Benedetti-Cecchi, L. (2003). The importance of the variance around the mean effect size of ecological processes. Ecology 84, 2335–2346. doi: 10.1890/02-8011
Benedetti-Cecchi, L., Bulleri, F., and Cinelli, F. (2000). The interplay of physical and biological factors in maintaining mid-shore and low-shore assemblages on rocky coasts in the north-west Mediterranean. Oecologia 123, 406–417. doi: 10.1007/s004420051028
Bennett, S., Wernberg, T., de Bettignies, T., Kendrick, G. A., Anderson, R. J., Bolton, J. J., et al. (2015). Canopy interactions and physical stress gradients in subtidal communities. Ecol. Lett. 18, 677–686. doi: 10.1111/ele.12446
Bertocci, I., Maggi, E., Vaselli, S., and Benedetti-Cecchi, L. (2005). Contrasting effects of mean intensity and temporal variation of disturbance on a rocky seashore. Ecology 86, 2061–2067. doi: 10.1890/04-1698
Blake, R. E., and Duffy, J. E. (2010). Grazer diversity affects resistance to multiple stressors in an experimental seagrass ecosystem. Oikos 119, 1625–1635. doi: 10.1111/j.1600-0706.2010.18419.x
Bracken, M. E., and Stachowicz, J. J. (2006). Seaweed diversity enhances nitrogen uptake via complementary use of nitrate and ammonium. Ecology 87, 2397–2403. doi: 10.1890/0012-9658(2006)87[2397:SDENUV]2.0.CO;2
Breitburg, D. L., Baxter, J. W., Hatfield, C. A., Howarth, R. W., Jones, C. G., Lovett, G. M., et al. (1998). “Understanding effects of multiple stressors: ideas and challenges,” in Successes, Limitations, and Frontiers in Ecosystem Science, eds M. L. Pace and P. M. Groffman (New York, NY: Springer, 416–431.
Breitburg, D. L., and Riedel, G. F. (2005). Multiple Stressors in Marine Systems. Marine Conservation Biology: The Science of Maintaining the Sea's Biodiversity. Washington: Island Press.
Brooks, P. R., and Crowe, T. P. (2018). Density and biotic interactions modify the combined effects of global and local stressors. Oikos. 127, 1746–1758. doi: 10.1111/oik.04459
Brown, R., Galloway, T., Lowe, D., Browne, M. A., Dissanayake, A., Jones, M., et al. (2004). Differential sensitivity of three marine invertebrates to copper assessed using multiple biomarkers. Aquat. Toxicol. 66, 267–278. doi: 10.1016/j.aquatox.2003.10.001
Browne, M. A., Brooks, P. R., Clough, R., Fisher, A. S., Mayer Pinto, M., and Crowe, T. P. (2016). Simulating regimes of chemical disturbance and testing impacts in the ecosystem using a novel programmable dosing system. Method. Ecol. Evol. 7, 609–618. doi: 10.1111/2041-210X.12521
Bryan, G., and Langston, W. (1992). Bioavailability, accumulation and effects of heavy metals in sediments with special reference to United Kingdom estuaries: a review. Environ. Pollut. 76, 89–131. doi: 10.1016/0269-7491(92)90099-V
Bulleri, F., Xiao, S., Maggi, E., and Benedetti Cecchi, L. (2014). Intensity and temporal variability as components of stress gradients: implications for the balance between competition and facilitation. Oikos 123, 47–55. doi: 10.1111/j.1600-0706.2013.00604.x
Burton, G. A., and Johnston, E. L. (2010). Assessing contaminated sediments in the context of multiple stressors. Environ. Toxicol. Chem. 29, 2625–2643. doi: 10.1002/etc.332
Côté, I. M., Darling, E. S., and Brown, C. J. (2016). Interactions among ecosystem stressors and their importance in conservation. Proc. Biol. Sci. 283:20152592. doi: 10.1098/rspb.2015.2592
Canty, M., Hagger, J., Moore, R., Cooper, L., and Galloway, T. (2007). Sublethal impact of short term exposure to the organophosphate pesticide azamethiphos in the marine mollusc Mytilus edulis. Mar. Pollut. Bull. 54, 396–402. doi: 10.1016/j.marpolbul.2006.11.013
Cartwright, S. R., Coleman, R. A., and Browne, M. A. (2006). Ecologically relevant effects of pulse application of copper on the limpet Patella vulgata. Mar. Ecol. Progr. 326, 187–194. doi: 10.3354/meps326187
Cheng, B. S., Bible, J. M., Chang, A. L., Ferner, M. C., Wasson, K., Zabin, C. J., et al. (2015). Testing local and global stressor impacts on a coastal foundation species using an ecologically realistic framework. Glob. Change Biol. 21:2488–2499. doi: 10.1111/gcb.12895
Coles, J. A., Farley, S. R., and Pipe, R. K. (1995). Alteration of the immune response of the common marine mussel Mytilus edulis resulting from exposure to cadmium. Dis. Aquat. Org. 22, 59–65. doi: 10.3354/dao022059
Coughlan, J. (1969). The estimation of filtering rate from the clearance of suspensions. Mar. Biol. 2, 356–358. doi: 10.1007/BF00355716
Crain, C. M., Kroeker, K., and Halpern, B. S. (2008). Interactive and cumulative effects of multiple human stressors in marine systems. Ecol. Lett. 11, 1304–1315. doi: 10.1111/j.1461-0248.2008.01253.x
Crowe, T. P., Frid, C. L., and Austen, M. (2015). Marine Ecosystems: Human Impacts on Biodiversity, Functioning and Services. Cambridge: Cambridge University Press.
Curtis, T. M., Williamson, R., and Depledge, M. H. (2000). Simultaneous, long-term monitoring of valve and cardiac activity in the blue mussel Mytilus edulis exposed to copper. Mar. Biol. 136, 837–846. doi: 10.1007/s002270000297
Dale, V. H., Joyce, L. A., McNulty, S., Neilson, R. P., Ayres, M. P., Flannigan, M. D., et al. (2001). climate change and forest disturbances: climate change can affect forests by altering the frequency, intensity, duration, and timing of fire, drought, introduced species, insect and pathogen outbreaks, hurricanes, windstorms, ice storms, or landslides. BioScience 51, 723–734. doi: 10.1641/0006-3568(2001)051[0723:CCAFD]2.0.CO;2
Darling, E. S., and Cote, I. M. (2008). Quantifying the evidence for ecological synergies. Ecol. Lett. 11, 1278–1286. doi: 10.1111/j.1461-0248.2008.01243.x
Easterling, D. R., Meehl, G. A., Parmesan, C., Changnon, S. A., Karl, T. R., and Mearns, L. O. (2000). Climate extremes: observations, modeling, and impacts. Science 289, 2068–2074. doi: 10.1126/science.289.5487.2068
Firbank, L. G., Petit, S., Smart, S., Blain, A., and Fuller, R. J. (2008). Assessing the impacts of agricultural intensification on biodiversity: a British perspective. Philos. Trans. R. Soc. B 363, 777–787. doi: 10.1098/rstb.2007.2183
Flöder, S., and Hillebrand, H. (2012). Species traits and species diversity affect community stability in a multiple stressor framework. Aquat. Biol. 17, 197–209. doi: 10.3354/ab00479
Folt, C., Chen, C., Moore, M., and Burnaford, J. (1999). Synergism and antagonism among multiple stressors. Limnol. Oceanogr. 44, 864–877. doi: 10.4319/lo.1999.44.3_part_2.0864
Fukami, T. (2001). Sequence effects of disturbance on community structure. Oikos 92, 215–224. doi: 10.1034/j.1600-0706.2001.920203.x
Giller, P. S., Hillebrand, H., Berninger, U. G., Gessner, M. O., Hawkins, S., Inchausti, P., et al. (2004). Biodiversity effects on ecosystem functioning: emerging issues and their experimental test in aquatic environments. Oikos 104, 423–436. doi: 10.1111/j.0030-1299.2004.13253.x
Godbold, J. A., and Solan, M. (2013). Long-term effects of warming and ocean acidification are modified by seasonal variation in species responses and environmental conditions. Philos. Trans. R. Soc. B 368:20130186. doi: 10.1098/rstb.2013.0186
Guzzella, L., Gronda, A., and Colombo, L. (1997). Acute toxicity of organophosphorus insecticides to marine invertebrates. Bull. Environ. Contamin. Toxicol. 59, 313–320. doi: 10.1007/s001289900481
Halpern, B. S., Walbridge, S., Selkoe, K. A., Kappel, C. V., Micheli, F., D'Agrosa, C., et al. (2008). A global map of human impact on marine ecosystems. Science 319, 948–952. doi: 10.1126/science.1149345
Harley, C. D., Randall Hughes, A., Hultgren, K. M., Miner, B. G., Sorte, C. J., Thornber, C. S., et al. (2006). The impacts of climate change in coastal marine systems. Ecol. Lett. 9, 228–241. doi: 10.1111/j.1461-0248.2005.00871.x
Hewitt, J., Ellis, J. I., and Thrush, S. F. (2016). Multiple stressors, threshold effects and the implications of climate change impacts on marine coastal ecosystems. Global Change Biol. 22, 2665–2675. doi: 10.1111/gcb.13176
Hooper, D. U., and Vitousek, P. M. (1998). Effects of plant composition and diversity on nutrient cycling. Ecol. Monogr. 68, 121–149. doi: 10.1890/0012-9615(1998)068[0121:EOPCAD]2.0.CO;2
Hughes, T., and Connell, J. (1999). Multiple stressors on coral reefs: a long-term perspective. Limnol. Oceanogr. 44, 932–940. doi: 10.4319/lo.1999.44.3_part_2.0932
IPCC (2012). Managing the Risks of Extreme Events and Disasters to Advance Climate Change Adaptation: Special Report of the Intergovernmental Panel on Climate Change. Cambridge: Cambridge University Press
Jackson, M. C., Loewen, C. J., Vinebrooke, R. D., and Chimimba, C. T. (2016). Net effects of multiple stressors in freshwater ecosystems: a meta-analysis. Glob. Change Biol. 22, 180–189. doi: 10.1111/gcb.13028
Jentsch, A., Kreyling, J., and Beierkuhnlein, C. (2007). A new generation of climate-change experiments: events, not trends. Front. Ecol. Environ. 5, 365–374. doi: 10.1890/1540-9295(2007)5[365:ANGOCE]2.0.CO;2
Jentsch, A., Kreyling, J., Elmer, M., Gellesch, E., Glaser, B., Grant, K., et al. (2011). Climate extremes initiate ecosystem-regulating functions while maintaining productivity. J. Ecol. 99, 689–702. doi: 10.1111/j.1365-2745.2011.01817.x
Johnston, E. L., and Keough, M. J. (2000). Field assessment of effects of timing and frequency of copper pulses on settlement of sessile marine invertebrates. Mar. Biol. 137, 1017–1029. doi: 10.1007/s002270000420
Kautsky, N., and Evans, S. (1987). Role of biodeposition by Mytilus-edulis in the circulation of matter and nutrients in a baltic coastal ecosystem. Mar. Ecol. Progr. 38, 201–212. doi: 10.3354/meps038201
Key, P. B., and Fulton, M. H. (1993). Lethal and sublethal effects of chlorpyrifos exposure on adult and larval stages of the grass shrimp, Palaemonetes pugio. J. Environ. Sci. Health 28, 621–640. doi: 10.1080/03601239309372844
Kim, W. S., Yoon, S. J., and Yang, D. B. (2004). Effects of chlorpyrifos on the endogenous rhythm of the Manila clam, Ruditapes philippinarum (Bivalvia: Veneridae). Mar. Pollut. Bull. 48, 182–187. doi: 10.1016/j.marpolbul.2003.09.005
Kreyling, J., Jurasinski, G., Grant, K., Retzer, V., Jentsch, A., and Beierkuhnlein, C. (2011). Winter warming pulses affect the development of planted temperate grassland and dwarf-shrub heath communities. Plant Ecol. Div. 4, 13–21. doi: 10.1080/17550874.2011.558125
Kreyling, J., Thiel, D., Nagy, L., Jentsch, A., Huber, G., Konnert, M., et al. (2012a). Late frost sensitivity of juvenile Fagus sylvatica L. differs between southern Germany and Bulgaria and depends on preceding air temperature. Euro. J. Forest Res. 131, 717–725. doi: 10.1007/s10342-011-0544-y
Kreyling, J., Thiel, D., Simmnacher, K., Willner, E., Jentsch, A., and Beierkuhnlein, C. (2012b). Geographic origin and past climatic experience influence the response to late spring frost in four common grass species in central Europe. Ecography 35, 268–275. doi: 10.1111/j.1600-0587.2011.07173.x
Kültz, D. (2005). Molecular and evolutionary basis of the cellular stress response. Annu. Rev. Physiol. 67, 225–257. doi: 10.1146/annurev.physiol.67.040403.103635
Lewis, J. (1998). Marine biofouling and its prevention on underwater surfaces. Mater. Forum 22, 41–61.
Lloret, F., Siscart, D., and Dalmases, C. (2004). Canopy recovery after drought dieback in holm-oak Mediterranean forests of Catalonia NE Spain. Glob. Change Biol. 10, 2092–2099. doi: 10.1111/j.1365-2486.2004.00870.x
Lurie, S., and Klein, J. D. (1991). Acquisition of low-temperature tolerance in tomatoes by exposure to high-temperature stress. J. Am. Soc. Horti. Sci. 116, 1007–1012. doi: 10.21273/JASHS.116.6.1007
Lyons, D., Benedetti-Cecchi, L., Frid, C., and Vinebrooke, R. (2015). “Modifiers of impacts on marine ecosystems: disturbance regimes, multiple stressors and receiving environments,” in Marine Ecosystems: Human Impacts on Biodiversity, Functioning and Services, eds T. Crowe and C. Frid (Cambridge University Press), 73–92.
Macinnis-Ng, C. M., and Ralph, P. J. (2004). In situ impact of multiple pulses of metal and herbicide on the seagrass, Zostera capricorni. Aquat. Toxicol. 67, 227–237. doi: 10.1016/j.aquatox.2004.01.012
Makepeace, D. K., Smith, D. W., and Stanley, S. J. (1995). Urban stormwater quality: summary of contaminant data. Crit. Rev. Environ. Sci. Technol. 25, 93–139. doi: 10.1080/10643389509388476
Martinez-Crego, B., Alcoverro, T., and Romero, J. (2010). Biotic indices for assessing the status of coastal waters: a review of strengths and weaknesses. J. Environ. Monit. 12, 1013–1028. doi: 10.1039/b920937a
Mayer-Pinto, M., Underwood, A., Tolhurst, T., and Coleman, R. (2010). Effects of metals on aquatic assemblages: what do we really know? J. Exp. Mar. Biol. Ecol. 391, 1–9. doi: 10.1016/j.jembe.2010.06.013
Mayor, D. J., Solan, M., McMillan, H., Killham, K., and Paton, G. I. (2009). Effects of copper and the sea lice treatment Slice(R) on nutrient release from marine sediments. Mar. Pollut. Bull. 58, 552–558. doi: 10.1016/j.marpolbul.2008.11.015
Molinos, J. G., and Donohue, I. (2010). Interactions among temporal patterns determine the effects of multiple stressors. Ecol. Appl. 20, 1794–1800. doi: 10.1890/10-0018.1
Mueller, R. C., Scudder, C. M., Porter, M. E., Trotter, R. T., Gehring, C. A., and Whitham, T. G. (2005). Differential tree mortality in response to severe drought: evidence for long-term vegetation shifts. J. Ecol. 93, 1085–1093. doi: 10.1111/j.1365-2745.2005.01042.x
Newell, R. I. (2004). Ecosystem influences of natural and cultivated populations of suspension-feeding bivalve molluscs: a review. J. Shellfish Res. 23, 51–62.
Nielsen, K. J. (2001). Bottom-up and top-down forces in tide pools: test of a food chain model in an intertidal community. Ecol. Monogr. 71, 187–217. doi: 10.1890/0012-9615(2001)071[0187:BUATDF]2.0.CO;2
Noël, L. M.-L., Griffin, J. N., Thompson, R. C., Hawkins, S. J., Burrows, M. T., Crowe, T. P., et al. (2010). Assessment of a field incubation method estimating primary productivity in rockpool communities. Estuar. Coast. Shelf Sci. 88, 153–159. doi: 10.1016/j.ecss.2010.03.005
Norling, P., and Kautsky, N. (2007). Structural and functional effects of Mytilus edulis on diversity of associated species and ecosystem functioning. Mar. Ecol. Progr. 351, 163–175. doi: 10.3354/meps07033
Nystrom, M., and Folke, C. (2001). Spatial resilience of coral reefs. Ecosystems 4, 406–417. doi: 10.1007/s10021-001-0019-y
O'Connor, N. E., and Donohue, I. (2013). Environmental context determines multi-trophic effects of consumer species loss. Glob. Change Biol. 19, 431–440. doi: 10.1111/gcb.12061
O'Meara, T. A., Hillman, J. R., and Thrush, S. F. (2017) Rising tides, cumulative impacts cascading changes to estuarine ecosystem functions. Sci. Rep. 7:10218. doi: 10.1038/s41598-017-11058-7
Paine, R. T. (1966). Food web complexity and species diversity. Am. Nat. 100, 65–75. doi: 10.1086/282400
Paine, R. T., Tegner, M. J., and Johnson, E. A. (1998). Compounded perturbations yield ecological surprises. Ecosystems 1, 535–545. doi: 10.1007/s100219900049
Piggott, J. J., Townsend, C. R., and Matthaei, C. D. (2015). Reconceptualizing synergism and antagonism among multiple stressors. Ecol. Evol. 5, 1538–1547. doi: 10.1002/ece3.1465
Pipe, R., Coles, J., Carissan, F., and Ramanathan, K. (1999). Copper induced immunomodulation in the marine mussel, Mytilus edulis. Aquat. Toxicol. 46, 43–54. doi: 10.1016/S0166-445X(98)00114-3
Prins, T. C., Smaal, A. C., and Dame, R. F. (1997). A review of the feedbacks between bivalve grazing and ecosystem processes. Aquat. Ecol. 31, 349–359. doi: 10.1023/A:1009924624259
Roberts, D. A., Poore, A. G. B., and Johnston, E. L. (2006). Ecological consequences of copper contamination in macroalgae: effects on epifauna and associated herbivores. Environ. Toxicol. Chem. 25, 2470–2479. doi: 10.1897/05-661R.1
Russell, B. D., Thompson, J. A. I., Falkenberg, L. J., and Connell, S. D. (2009). Synergistic effects of climate change and local stressors: CO2 and nutrient-driven change in subtidal rocky habitats. Glob. Change Biol. 15, 2153–2162. doi: 10.1111/j.1365-2486.2009.01886.x
Sabehat, A., Weiss, D., and Lurie, S. (1998). Heat-shock proteins and cross-tolerance in plants. Physiol. Plant. 103, 437–441. doi: 10.1034/j.1399-3054.1998.1030317.x
Schiedek, D., Sundelin, B., Readman, J. W., and Macdonald, R. W. (2007). Interactions between climate change and contaminants. Mar. Pollut. Bull. 54, 1845–1856. doi: 10.1016/j.marpolbul.2007.09.020
Seed, R. (1996). Patterns of biodiversity in the macro-invertebrate fauna associated with mussel patches on rocky shores. J. Mar. Biol. Assoc. UK. 76, 203–210. doi: 10.1017/S0025315400029131
Serrano, R., Lopez, F., Hernandez, F., and Pena, J. (1997). Bioconcentration of chlorpyrifos, chlorfenvinphos, and methidathion in Mytilus galloprovincialis. Bull. Environ. Contamin. Toxicol. 59, 968–975. doi: 10.1007/s001289900577
Smith, M. D., Knapp, A. K., and Collins, S. L. (2009). A framework for assessing ecosystem dynamics in response to chronic resource alterations induced by global change. Ecology 90, 3279–3289. doi: 10.1890/08-1815.1
Sousa, W. P. (1984). The role of disturbance in natural communities. Ann. Rev. Ecol. Syst. 15, 353–391. doi: 10.1146/annurev.es.15.110184.002033
Srinivasan, M., and Swain, G. W. (2007). Managing the use of copper-based antifouling paints. Environ. Manag. 39, 423–441. doi: 10.1007/s00267-005-0030-8
Strain, E. M. A., Thomson, R. J., Micheli, F., Mancuso, F. P., and Airoldi, L. (2014). Identifying the interacting roles of stressors in driving the global loss of canopy-forming to mat-forming algae in marine ecosystems. Glob. Change Biol. 20, 3300–3312. doi: 10.1111/gcb.12619
Taylor, H., and Anstiss, J. M. (1999). Copper and haemocyanin dynamics in aquatic invertebrates. Mar. Freshw. Res. 50, 907–931. doi: 10.1071/MF99117
Todgham, A. E., Schulte, P. M., and Iwama, G. K. (2005). Cross-tolerance in the tidepool sculpin: the role of heat shock proteins. Physiol. Biochem. Zool. 78, 133–144. doi: 10.1086/425205
Underwood, A., Chapman, M., Richards, S., and Sage, M. (1998). GMAV5 for Windows. Sydney: Institute of Marine Ecology; University of Sydney
Underwood, A., and Peterson, C. (1988). Towards an ecological framework for investigating pollution. Mar. Ecol. Prog. Ser. 46, 227–234. doi: 10.3354/meps046227
Underwood, A. J. (1996). Experiments in Ecology: Their Logical Design and Interpretation Using Analysis of Variance. Cambridge: Cambridge University Press.
US Environmental Protection Agency (2009). Office of Pesticide Programs. Aquatic Life Benchmarks. Available online at: http://www.epa.gov/oppefed1/ecorisk_ders/aquatic_life_benchmark.htm (accessed March 3, 2014).
Vinebrooke, R. D., Cottingham, K. L., Norberg, J., Scheffer, M., Dodson, S. I., Maberly, S. C., et al. (2004). Impacts of multiple stressors on biodiversity and ecosystem functioning: the role of species co-tolerance. Oikos 104, 451–457. doi: 10.1111/j.0030-1299.2004.13255.x
Vitousek, P. M. (1997). Human domination of Earth's ecosystems. Science 278, 21–32. doi: 10.1126/science.277.5325.494
Walter, J., Jentsch, A., Beierkuhnlein, C., and Kreyling, J. (2013). Ecological stress memory and cross stress tolerance in plants in the face of climate extremes. Environ. Exp. Botany. 94, 3–8. doi: 10.1016/j.envexpbot.2012.02.009
Walter, J., Nagy, L., Hein, R., Rascher, U., Beierkuhnlein, C., Willner, E., et al. (2011). Do plants remember drought? Hints towards a drought-memory in grasses. Environ. Exp. Botany 71, 34–40. doi: 10.1016/j.envexpbot.2010.10.020
Ward, T. J. (1987). Temporal variation of metals in the seagrass Posidonia australis and its potential as a sentinel accumulator near a lead smelter. Mar. Biol. 95, 315–321. doi: 10.1007/BF00409019
Widdows, J., and Donkin, P. (1992). Mussels and environmental contaminants: bioaccumulation and physiological aspects. Mussel Mytilus Ecol. Physiol. Genet. Cult. 25, 383–424.
Keywords: multiple stressors, time-lags, sequential order, assemblages, ecosystems, ecosystem functioning
Citation: Brooks PR and Crowe TP (2019) Combined Effects of Multiple Stressors: New Insights Into the Influence of Timing and Sequence. Front. Ecol. Evol. 7:387. doi: 10.3389/fevo.2019.00387
Received: 15 October 2018; Accepted: 25 September 2019;
Published: 16 October 2019.
Edited by:
Samuel A. Cushman, United States Forest Service (USDA), United StatesReviewed by:
Attila D. Sándor, University of Agricultural Sciences and Veterinary Medicine of Cluj-Napoca, RomaniaJasmin Godbold, University of Southampton, United Kingdom
Copyright © 2019 Brooks and Crowe. This is an open-access article distributed under the terms of the Creative Commons Attribution License (CC BY). The use, distribution or reproduction in other forums is permitted, provided the original author(s) and the copyright owner(s) are credited and that the original publication in this journal is cited, in accordance with accepted academic practice. No use, distribution or reproduction is permitted which does not comply with these terms.
*Correspondence: Paul R. Brooks, cGF1bC5icm9va3NAdWNkLmll
†ORCID: Paul R. Brooks orcid.org/0000-0002-8105-0813