- 1Department of Civil and Environmental Engineering, Universidad de los Andes, Bogota, Colombia
- 2Ecoinformatics and Biodiversity, Department of Bioscience, Aarhus University, Aarhus, Denmark
- 3Department of Plant Biology, Faculty of Biology, Universidad de Barcelona, Barcelona, Spain
- 4Center for Conservation and Sustainable Development, Missouri Botanical Garden, St. Louis, MO, United States
Understanding the main relationships between the current macroclimate and broad spatial patterns of plant diversity is a priority in biogeography, and although there is an important body of studies on the topic worldwide, tropical mountains remain underrepresented. Because understanding primary drivers of diversity patterns in the Andean páramo is still in its infancy, we focused on evaluating the role of the current macroclimate in form of three complementary hypotheses, energy, seasonality and harshness, in explaining local variation of plant species richness. We relied on 1,559 vegetation plots that offered a fine-scale outlook on real species assemblages due to community rules and species' interactions with their surrounding environment, including climate. Generalized Least Squares (GLS) regression models provided insight on the significance of the different hypotheses in explaining local plant richness, but only the energy and seasonality hypotheses received partial support. The best model was then combined with spatial interpolation Kriging modeling techniques to project species richness for a standardized 25 m2 plot throughout the entire páramo biogeographical province. We highlighted a North-South increase in richness with several species-rich areas, potential local biodiversity hotspots, independent of the general gradient: the Amotape-Huancabamba zone, Sangay and Cotacachi areas, and eastern Venezuelan Andes. Our endeavor to finely map local richness is the first effort predicting macroecological patterns in the emblematic Andean páramo and contributes novel biogeographical knowledge useful to further support in-depth research and conservation focus in the northern Andes.
Introduction
Understanding spatial patterns of biodiversity across dimensions, scales, and areas of the world remains one of the greatest challenges in biogeography (Lomolino and Heaney, 2004; Kennedy and Norman, 2005). With 15% of the world's total plant richness, the Tropical Andes is a main biodiversity hotspot on earth (Myers et al., 2000; Barthlott et al., 2007), and home to the Andean páramo, a high mountain biogeographical province (Morrone, 2014) known as the most phytodiverse tropical high mountain system worldwide (Sklenár et al., 2014). The páramo includes all natural and semi-natural ecosystems located above the montane treeline (~3,000–5,000 m) in the northern tropical Andes of Venezuela, Colombia, Ecuador, and northern Peru (Luteyn, 1999) and presents an astonishing phytodiversity of almost 500 genera and 5,000 plant species, 60–80% of which are endemic (Londoño et al., 2014; Rangel-Churio, 2015). It is mostly thanks to the recent orogeny of the northern tropical Andes, geographic proximity to the Amazonian and Chocó-Pacific biomes, and relatively stable climatic conditions though time that the páramo evolved to become the world's coldest and fastest evolving biodiversity hotspot (Madriñan et al., 2013; Anthelme et al., 2014). The páramo, with a spatial distribution over 20 degrees latitude and 2,000 m elevation, constitutes an excellent biogeographical model system to represent tropical mountains worldwide (Anthelme and Peyre, 2019).
Macroecological research on broad-scale spatial patterns of species richness in tropical mountain regions and in the páramo is critically lacking to date. Previous studies have either focused on limited taxonomic groups (e.g., for plants: Izco et al., 2007; Nürk et al., 2013; for animals: Jacobsen, 2003; Sites et al., 2003; Moret, 2009) or reduced geographic areas (Sklenár and Jørgensen, 1999; Sklenář and Ramsay, 2001; but see Cuesta et al., 2017 for a regional approach, although with sparsely distributed data). When focusing on plant species richness, a decreasing tendency has been highlighted along the elevational gradient of certain páramos (Sklenár and Jørgensen, 1999; Sklenář and Ramsay, 2001) and latitudinal gradients remain generally understudied. Several authors have also discussed potential drivers of local species richness and found that human disturbance and several environmental factors, such as annual precipitation and scree, condition plant richness in the high tropical Andes (Sklenář and Ramsay, 2001; Vásquez et al., 2015; Cuesta et al., 2017).
Many hypotheses have been proposed to explain variation in species richness, and the environment, especially the contemporary climate, has held a prevailing place as potential driver (Hawkins et al., 2003; Willig et al., 2003; Field et al., 2009). Other simultaneously-acting hypotheses have also been advanced to play a significant role, among which, other environmental factors such as edaphic conditions, historical climate (Francis and Currie, 2003; Araújo et al., 2008), biome age and area (Rahbek, 1995; Fine, 2015), species diversification rates and historical processes (Ricklefs, 2006; Jetz and Fine, 2012), functional diversity and biotic interactions (Cavieres et al., 2014; Mod et al., 2015). Because of the broad extent of the páramo biogeographical province and the general paucity of fine data to represent potential causal factors associated with richness variation, our exploratory study focused on understanding the role of the contemporary macroclimate, with available data for the entire study area (Karger et al., 2017), as richness driver at the local scale. To do so, three complementary climatic hypotheses were set to represent the overall macroclimate: energy, seasonality, and climatic harshness (Willig et al., 2003; Kreft and Jetz, 2007; Tello and Stevens, 2010; but see Mittelbach et al., 2007).
(1) Energy: Plant species richness is generally found to be conditioned by two forms of environmental energy: kinetic—related to temperature—and potential (or chemical)—the result of photosynthesis constrained by climatic conditions, in form of temperature and water availability (Hawkins et al., 2003; Currie et al., 2004; Allen et al., 2007). Multiple mechanisms have linked both forms of energy to species richness (Currie et al., 2004; Evans et al., 2005). For example, kinetic energy has been proposed to increase biological rates, e.g., mutation, metabolism and generation times, resulting in accelerated speciation processes (Gillooly et al., 2001, 2005). Similarly, high potential energy, hence substantial productivity, can sustain larger population sizes, which can in turn reduce extinction risk and increase speciation probability (Srivastava and Lawton, 1998). In addition, high potential energy has often been correlated to carrying capacity for species, whereby more species can co-exist in highly productive environments under warm and humid climates (Fine, 2015). According to the energy hypothesis, areas with high energy concentration would support more plant diversity.
(2) Seasonality: the variation in environmental conditions at multi-temporal scales has been proposed to drive species richness patterns by influencing the co-existence and accumulation of species (Jablonski et al., 2006; Fine, 2015; Pfeifer et al., 2018). For instance, climatic stability over centuries and millennia can decrease the likelihood of extinctions and promote the saturation of niche spaces, resulting in important species richness (Ordonez and Svenning, 2017; Fordham et al., 2018). In contrast, short-term seasonality, i.e., seasonality at the intra-annual and inter-annual scale, has been suggested as a source of climatic instability that can lead to wide population fluctuations and even increase extinction risk during time of low population size (Inchausti and Halley, 2003; Somveille et al., 2015; Rajakaruna and Lewis, 2018). Alternatively, species may adapt to high seasonality by developing broad niches, hence broad geographic distributions with few dispersal and ecological barriers and little population subdivision. This in turn would reduce the likelihood of speciation events and lead to species-poor communities with little fine partitioning of their niche space (Pianka, 1966; MacArthur et al., 1972). Therefore, the seasonality hypothesis would state that short-term climatically unstable areas would possess lower species richness than stable ones.
(3) Harshness: the climate extremes might physiologically limit species in their growth and reproduction, hence in their spatial distribution (Francis and Currie, 2003; Currie et al., 2004; Evans et al., 2005; Smith, 2011). While most hypotheses about environmental conditions typically use average conditions, the harshness hypothesis is correlated to the energy hypothesis but suggests that it is the most unsuitable conditions that occur at a site that limit species distributions, hence community composition and diversity (e.g., Knapp et al., 2002; Miriti et al., 2007; Thibault and Brown, 2008). If measures of climatic tolerance are similar for most species, then harsh environments would be tolerated by fewer species and diversity would be lower, as illustrated by the relatively limited species richness at high latitudes due to freezing tolerance (Hawkins et al., 2003). Indeed, although the average annual temperature can be included in the physiological tolerance range of a species in cold regions, the minimum yearly temperature might limit the species' distribution range and contribute to a diversity decrease at the ecosystem level. A similar mechanism might occur in regions where water availability is limiting during at least part of the year and excludes species that cannot tolerate droughts (Miriti et al., 2007). Although resistance to extreme climatic events is evolution and species-dependent, the combination of cold and dry conditions is generally believed to be harsh for most taxa, as a result, we expected under the harshness hypothesis for lower species richness to be found in páramo with harsh climatic conditions.
Mapping fine-scale richness across broad areas has proved very useful guiding conservation and management planning, for example through the identification of local biodiversity hotspots (Myers et al., 2000), however these efforts remain rare to date (Beck et al., 2012; Divíšek and Chytrý, 2018; Večera et al., 2019). Most macroecological studies rely on coarse grain biological data, i.e., grid cell, with correlation analyses and predictions made based on checklist occurrence data or predicted occurrences stacked together (e.g., D'Amen et al., 2015; Ulloa Ulloa et al., 2017). In these cases, local species lists are usually not obtained from real biotic communities responding to ecological assembly rules, but by co-occurrences driven by individualistic observation or probability of presence in a given cell. On the contrary, vegetation data accounts for this issue as it provides fine resolution information on actual species assemblages in direct interaction with the surrounding environment, and as a result, a new body of biogeographical studies is starting to explore predicting spatial richness patterns from this type of data (Divíšek and Chytrý, 2018; Večera et al., 2019). As vegetation databases encompassing tropical mountain areas our flourishing worldwide, including in the páramo (Peyre et al., 2015; Bruelheide et al., 2019), new approaches to macroecological research are becoming accessible for these regions. By correlating local richness with the macroclimate in the páramo, we expected the resulting fine-scale map to highlight a general decrease richness pattern with increasing latitude (Hawkins et al., 2003; Willig et al., 2003), even though it has not yet been confirmed for tropical mountain areas (Bjorholm et al., 2005), as well as decreasing richness with elevation as previously found in Ecuadorian páramos (Sklenář and Ramsay, 2001; McCain and Grytnes, 2010). Finally and related to our climatic hypotheses, we believed local hotspots would be highlighted in climatically clement areas, for example in the western Colombian cordillera and the central eastern Ecuadorian cordillera (Herzog et al., 2011; Rangel-Churio, 2015).
The overarching objective of this study was to understand how the contemporary macroclimate influences local plant richness in the páramo and map fine-scale richness predictions using climatic drivers throughout the biogeographical province. Specifically, the respective roles of energy, seasonality, and harshness were studied as three complementary hypotheses, and local plant richness was mapped to reveal general spatial patterns and biodiversity hotspots in the high northern Andes.
Methods
All statistical analyses were conducted in R 3.3.1 (R Core Team, 2013).
Study Area and Vegetation Data
The study area encompassed the Andean páramo biogeographical province, including the high-elevation northern Andes and the Sierra Nevada de Santa Marta, but excluding other extra-Andean areas with similar altitudinal and/or environmental conditions, such as Amazonian volcanoes or Central American mountains (Figure 1). It was therefore delimited in the north by the Sierra Nevada of Santa Marta (11°N, Colombia) and in the south by the Huancabamba depression (6°S, Peru), which is usually considered a main biogeographical barrier for high-elevation plant taxa (Weigend, 2002). The easternmost páramos were located in the Cordillera de Merida (72°W, Venezuela), whereas the westernmost ones were the Piura páramos (80°W, Peru).
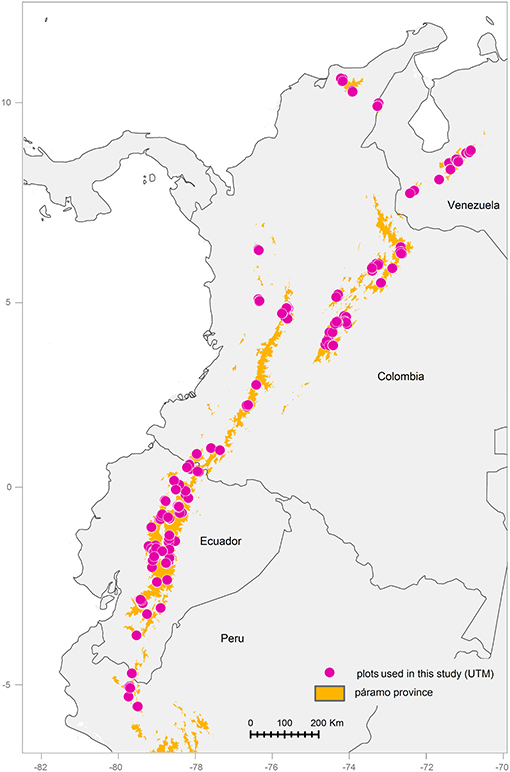
Figure 1. Distribution of the Andean páramo biogeographical province (elevation above 3,000 m) and the vegetation plots used in this study (1 Km UTM coordinates).
For floristic data, all vegetation plots contained in VegPáramo, the database for páramo flora and vegetation that compiles data from almost 40 different sources, were downloaded (Peyre et al., 2015). These plots were sampled with the phytosociological method, which involves estimating species cover in a categorical scale (from + to 5) within defined plots of a varying area set for different vegetation physiognomies (Braun-Blanquet, 1951; Figure 1 and Figure S1A). In order to estimate plant richness within each plot, the species relative cover values were transformed into presence-absence data. To standardize the taxonomy across the heterogeneous dataset, synonymy was checked, and updated using the VegPáramo taxon list, which contains updated information for about 15,000 northern Andean plant species. For dubious taxa such as orchids, the Plant List (www.plantlist.org) and Tropicos (www.tropicos.org) databases were also consulted. Non-vascular plants were then removed from the dataset, as well as unidentified species. Finally, infra-specific taxa, i.e., subspecies, varieties and forms, were elevated, and combined to the species level. Regarding the plot data, outliers to the study area and plots with a coarse georeferencing precision (>1 km) were removed from the dataset. Because this study focused on spatial patterns of fine-scale species richness over a broad area, plots whose descriptive structure and composition explicitly referred to azonal or ecotone vegetation were eliminated, according to the plot's original authors. Finally, the few plots with unusual areas (< 1 m2 and > 100 m2) were removed, for a final dataset containing 1,559 vegetation plots and 1,169 species, spread over the páramo biogeographical province and presenting considerable local-plot richness variation (Figure S1B and Table S1).
Climatic Data
Disentangling the effects of the three climatic hypotheses is challenging due to the necessary correlation between climatic predictors and consequent overlapping of the hypotheses. For energy, we included mean annual temperature (bio1), annual precipitation (bio12), actual evapotranspiration (AET), and mean monthly soil water stress coefficient defined as the fraction (percentage) of soil water content available for evapotranspiration(swcfr). For seasonality, we considered mean diurnal temperature range (bio2) for daily variation, temperature seasonality (bio 4) and precipitation seasonality (bio15) for monthly variation, as well as inter-annual temperature variation (bio20) and inter-annual precipitation variation (bio21) for yearly variation. The latter two variables were calculated as the yearly standard deviation of bio1 and bio12, respectively, during the period 1979–2013. Finally to represent harshness, we used minimum temperature of the coldest month (bio6), precipitation of the driest month (bio14), and precipitation of the warmest quarter (bio18) that we supposed woud condition richness. The bioclimatic variables (bio), except for bio20 and 21, were downloaded as means for the period 1979–2013, from the (CHELSA Project 1.1, 2017), Climatologies at High resolution for the Earth's Land Surface Areas (http://chelsa-climate.org), which is known for enhancing bioclimatic data quality in tropical areas (Karger et al., 2017). Additionally, the AET and swcfr variables were obtained from the CGIAR-CSI, Consultative Group on International Agricultural Research Consortium for Spatial Information databases (www.cgiar-csi.org; Zomer et al., 2008; Trabucco and Zomer, 2010) and all variables were obtained as raster data at a resolution of 30 arc-s (~1 km). All variables presented a certain amount of multicollinearity, some of them very high as illustrated by high Pearson correlation values (Figure S2). Nonetheless, because these variables were used in different models, or altogether but with a robust variable selection process, we considered this approach valid and took the multicollinearity information into account in our discussion.
Drivers of Richness
For these analyses, Generalized Least Squares (GLS) models were used because they efficiently account for the correlation structure in residuals (fitted with the gls function of the nlme R package; Beale et al., 2010). Through testing several correlation structures, the quadratic spatial correlation with a nugget effect was finally selected and used in all following models, based on its performance given by the AIC and spatial autocorrelation in residuals (function corRatio in the nlme R package). For each model carried out, all variables were subjected to the forward selection process proposed by Blanchet et al. (2008) to reduce collinearity and identify the most significant predictors. The procedure included successively adding a variable (from 1 to n) to the model and validate it based on variables' p-values (significance level set at 0.05) and model performance (function ordiR2step in the R package vegan, Oksanen et al., 2012). To compare the performance of the obtained models, a pseudo-R2 was calculated, based on the squared correlation coefficient between observed and predicted richness. Moreover, models were evaluated according to their AIC and ΔAIC (difference between the selected and the best performing model), considering that any ΔAIC < 2 between models would imply a similar support from the data (Burnham and Anderson, 2002).
Due to the large variation of plot areas in the dataset, characteristic of phytosociological sampling (Braun-Blanquet, 1951; Figure S1A), a preliminary analysis was conducted to determine a potential correlation between plot area and local plant richness (Lomolino, 2000). To do so, a regression between plot area in its logarithmic form and richness was conducted, model PlotArea, and showed a significant correlation between the two factors (pseudoR2 = 0.128, p-value< 0.001, Table 1 and Figure 2B). Therefore, plot area was considered a significant predictor of local plant richness and included in its logarithmic form in all the following regression analyses.
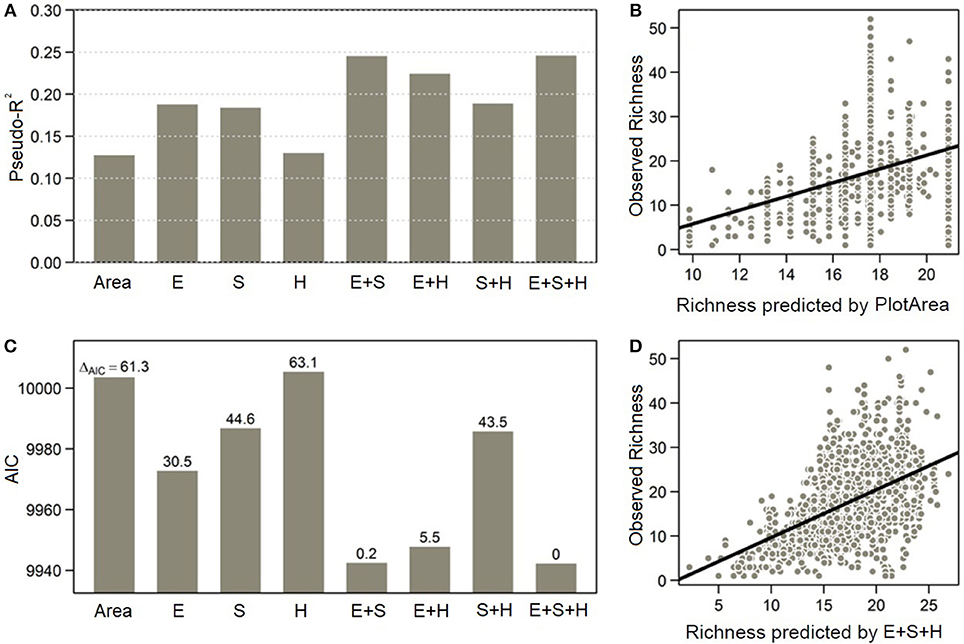
Figure 2. Richness-climate relationships captured by the energy, seasonality, and harshness model combinations: (A) Performance of all models according to the pseudo-R2 value (squared correlation coefficient between observed and predicted richness); (B) Relationship between observed and predicted richness by the model PlotArea; (C) Performance of all models according to the AIC and ΔAIC values; and (D) Relationship between observed and predicted richness by the environmental model GLS:E+S+H.
Eight regression models using different sets of climatic variables and log(area) were built. Three models included all the predictors associated with only one hypothesis: energy (Energy), seasonality (Seasonality), and harshness (Harshness). Three additional models incorporated all possible combinations of predictors from two hypotheses (models E+S, E+H, or S+H). Finally, a complete climatic model considered all variables representing the three hypotheses (E+S+H).
Regional Fine-Scale Richness Patterns
To explore spatial patterns of fine-scale plant richness in the páramo province, three predictive maps were built based on local richness for a standardized by logarithm size plot of 25 m2 to make predictions comparable despite the varying plot size. The following techniques aimed at optimizing the predictions for under-sampled areas by relying not only on climatic data but also on spatial complexity. The first interpolation technique used was a spatial Ordinary Kriging (OK) model, which estimated local species richness in non-sampled areas based solely on the distance between said area and its nearest sampled points (Banerjee et al., 2003). Second, the best performing GLS model previously obtained was used alone to predict local richness. Last, both the OK and GLS models were combined into a Universal Kriging (UK) model, which is a commonly used approach to enhance interpolation estimates and account for both climatic gradients and underlying spatial trends (Kreft and Jetz, 2007).
Results
Climatic models varied in their explaining power (Table 1). The energy (Energy) and seasonality (Seasonality) models presented a pseudo-R2 of almost 0.19, corresponding to an explaining power of almost 19 percent of the variation in local richness, while plot area alone showed a pseudo-R2 around 0.13 only (model PlotArea). On the contrary, the harshness model (Harshness), which only retained the plot area variable of all factors, behaved unsurprisingly similarly to model PlotArea. Among all models, the best performing and statistically comparable models with a ΔAIC < 2 were models E+S and E+S+H. These two models presented a pseudo-R2 close to 0.25, explaining almost 25 percent of local richness variation and showed the lowest AIC value at 9943 (Figure 2). Both models included the same significant predictors, except for one variable present only in model E+S+H, which is precipitation of the driest month (bio14), a harshness variable strongly correlated with annual precipitation (bio12) (Figure S2). They also showed similar tendencies regarding the correlation of predictors with local richness, as detailed below.
First considering the energy variables included in models E+S and E+S+H, species richness was correlated positively to annual temperature (bio1) and negatively with soil water stress coefficient (swcfr). Moreover, richness was negatively correlated to annual precipitation (bio12). Other models showed similar results for the correlation between richness and the predictors: swcfr (in models Energy and E+H) and bio12 (in model E+H), whereas bio1 was not included in any other model. Second regarding seasonality predictors, models E+S, S+H, and E+S+H retained precipitation seasonality (bio15) and found it negatively correlated to richness. Models Seasonality and S+H both contained temperature seasonality (bio4), a variable positively correlated to richness, and in the former case, mean diurnal temperature range (bio2) was also retained and positively correlated to richness. Last for harshness, precipitation of the driest month (bio14) was the only predictor included in models E+S+H, E+H, and S+H and showed a positive correlation with richness in the first two models and a negative one in the latter. Nonetheless, this variable was not even found significant in the model Harshness. In general, models included more precipitation-related variables, except for model Seasonality, which included a majority of temperature-related predictors.
All models used to create interpolated maps of local plant richness across the páramo province predicted similar spatial patterns (Figure 3 and Figure S3). The best performing GLS model selected to predict richness was model E+S+H because of its good pseudo-R2 and AIC values and consideration of a harshness variable. Both the OK and E+S+H models predicted a variation of 7–35 species per 25 m2 plot (Figures 3A,B and Figure S3) and by contrast, the UK model predicted 4–42 species, a fitter value-range to the observed 1–52 species present in the plot data (Figure 3C and Figure S1B). According to the UK model predictions, a general decrease of species richness was projected from south to north, dividing the locally more diverse páramos south of the equator and the less diverse páramos in the north (Figure 3D). Overall, Colombian páramos were found generally poorer than páramos in other countries. No clear elevational gradient was observed at this scale, assuming high elevations are located in the central part of mountain ranges, while low elevations are located at the borders. Several punctual areas with high local species richness (> 35 species per 25 m2 plot) could be observed, for example surrounding the Amotape-Huancabamba zone (~Lat. 5°S; Long. 79.5°W), the Sangay (~Lat. 2°S; Long. 78.5°W), and Cotacachi (~Lat. 0°; Long. 78°W) massifs in Ecuador, as well as the Lara páramos in Venezuela (~Lat. 9°N; Long. 71°W).
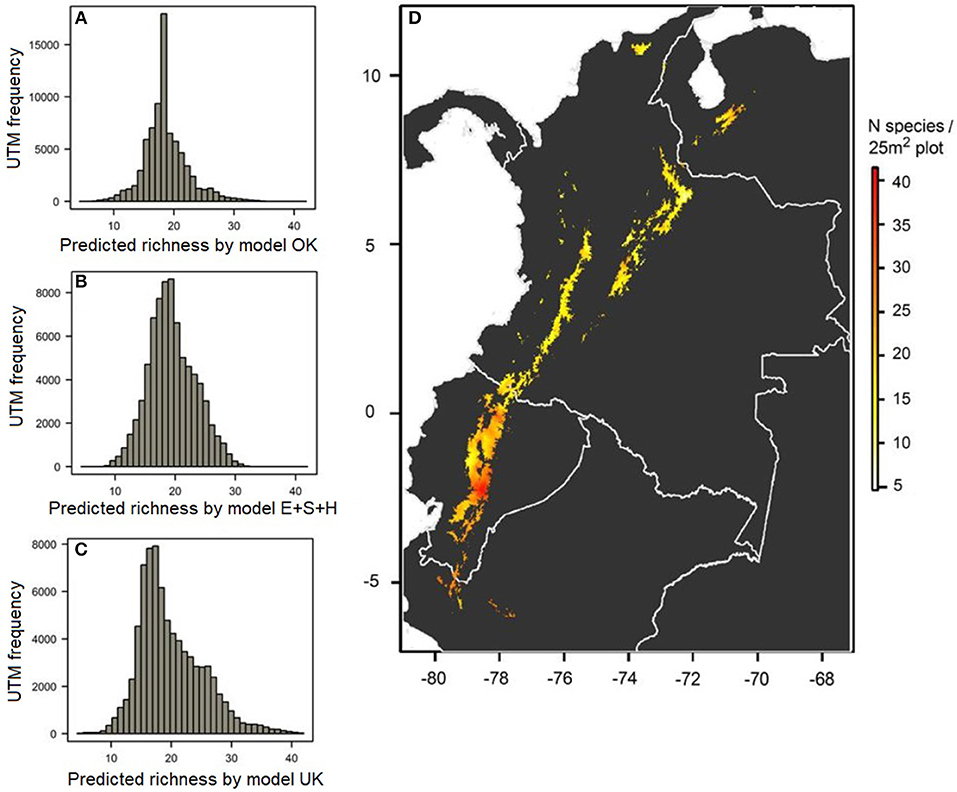
Figure 3. Fine-scale richness predictions for a standardized 25 m2 plot across the páramo biogeographical province. Histograms of frequencies of predicted local richness values by: the model OK (A), the best performing environmental model GLS: E+S+H (B), and the model UK (C); and (D) local richness map as expected by the model UK throughout the páramo biogeographical province, shown at a 30 arc-s (~1 km) resolution.
Discussion
Our study is pioneer in studying macroclimatic drivers of plant richness and conducting macroecological analyses over the entire páramo biogeographical province and based on substantial vegetation data. It also represents one of the first few efforts worldwide to propose fine-resolution richness mapping based on vegetation data, hence correlating real species assemblages in their complexity of interactions and community constraints with macroclimate features. The results obtained shed light on the role of macroclimate in explaining local variation in local richness and provided a richness fine-scale map with potential future uses in research and biodiversity management, for example through the identification of local biodiversity hotspots.
Climatic Drivers of Richness
The macroclimate was found to play a significant but rather small part in explaining variation of local plant richness, 25 percent at best (models E+S and E+S+H) and 13 percent more than plot area alone (PlotArea). Several but not all of the significant correlations found between environmental variables and plant richness agreed with the originally set expectations. Therefore, our findings should be interpreted carefully as potential influences between variables and not as direct cause-consequence relationships.
Regarding energy, we observed that local richness increased with annual temperature (bio1), which supports the hypothesis that high kinetic energy can increase biological rates, hence promoting population differentiation, speciation and therefore greater diversity, although said effects are hardly visible at the plot scale (Gillooly et al., 2001; Hawkins et al., 2003; Evans et al., 2005). Agreeing with this finding was the negative relation found between soil water stress (swcfr) and local richness. This is a commonly encountered correlation in ecology, and unlike temperature that usually enhances net primary productivity, soil water stress tends to reduce it, and therefore influence plant diversity through the ecosystem's productivity (Srivastava and Lawton, 1998; Currie et al., 2004). Nonetheless, our results showed that local richness decreased with annual precipitation (bio12), which in turn contradicts the hypothesis that high potential energy in form of water availability would increase the accumulation of species and saturation of habitats, resulting in higher species richness (Hawkins et al., 2003; McCain and Grytnes, 2010; Fine, 2015). The precipitation-richness relationship is often difficult to isolate from other factors' influence because mixed effects usually occur, for instance winds are important in conditioning local precipitation trends (McCain and Grytnes, 2010). We propose several potential explanations for this unexpected result and recommend further study to evaluate them. First, because most páramos are already humid ecosystems, i.e., receiving between 2,000 and 4,000 mm rain/year (Luteyn, 1999; Rangel-Churio, 2006), we could advance the intermediate-stress hypothesis, which forecasts highest richness at moderate intensities of environmental stress (Grime, 1979). Traditionally, this hypothesis has been more documented along aridity gradients with drought stresses (e.g., Armas et al., 2011; Butterfield et al., 2016), however, humidity gradients with wet stresses are being increasingly studied (e.g., Kramer and Boyer, 1995; Knapp et al., 2008). Most páramos receive sufficient water to function adequately, and only a few endure temporal droughts, for example the rain-shadow deserts of Mount Chimborazo (~Lat. 1.5°S; Long. 78.8°W) and Piedras blancas (~Lat. 8.8°N; Long. 70.8°W) (Sklen and Lægaard, 2003; Stansell et al., 2005). Nonetheless, many páramos face a very important up to extreme water intake, which could result in a temporal or permanent wet stress, for example in the pluvial páramos of the Western Colombian Cordillera that receive more than 6,000 mm/year (Rangel-Churio, 2006). This situation could result in less species able to tolerate the very humid environmental conditions and anoxic soils, and limit the overall species saturation of páramo ecosystems (Kammer and Möhl, 2002). Second, important precipitation could indirectly imply denser fog and cloud cover, known as horizontal precipitation, which constitutes the main water intake in most páramos (Buytaert et al., 2006; Sklenár et al., 2008) but which is very difficult to account for in models. Fog and cloud cover benefit ecosystems by providing substantial amounts of humidity for plants, protecting organisms from the damaging solar radiation and buffering extreme temperatures (Luteyn, 1999; Sklenár et al., 2008). Nonetheless, too much intercepted water around the leaves could also limit evapotranspiration and photosynthetic processes (Smith and McClean, 1989; Asbjornsen et al., 2011), resulting in less productivity and as a result lower richness. Last, precipitation might be correlated to the abundance of growth forms in a plot, which by occupying different fractions of the available space might allow more or less functional hence taxonomic diversity to co-occur locally (Wilson et al., 2012). An extreme example would be how in very humid páramos are usually associated with vegetation dominated by bamboos of the genus Chusquea, which tend to prevail and leave little space for other plant species to grow, resulting in turn in relatively low richness (Luteyn, 1999; Cleef et al., 2005; Cuello and Cleef, 2009). Downscaling our results to the páramo vegetation type or phytogeographical unit (e.g., Peyre et al., 2018) might provide clearer insight on the validity of these hypotheses (Divíšek and Chytrý, 2018).
For the seasonality hypothesis, our main results highlighted the negative correlation between richness and precipitation seasonality (bio15). This finding agrees with our original hypothesis stating that in climatically stable páramos, where seasonality is limited, species would tend to accumulate and narrow-niche species would be favored. Previous studies have agreed with this hypothesis for animals (Somveille et al., 2015; Pfeifer et al., 2018) and also plants (Morueta-Holme et al., 2013), because short-term climatic stability means less migrations and important adaptations, i.e., broad-niche species, resulting in relatively species-poor ecosystems (Pianka, 1966; MacArthur et al., 1972). However, in lesser means, our results contradict the seasonality hypothesis. The positive correlation between richness and temperature seasonality (bio4) and mean diurnal temperature range (bio2), observed in all models except the best performing E+S and E+S+H, suggest that short-term temperature variability at the day and season levels increase richness. In light of these unexpected results, we recommend further studies to focus on the effect of temperature seasonality on richness in more local conditions, for example looking at how temperature variability affects specific phenological plant responses (e.g., Pfitsch, 1988; Fagua and Gonzalez, 2007), which in turn could condition communities' composition and diversity. Finally, our study gave little support to the harshness hypothesis, with precipitation of the driest month (bio14) the only harshness variable retained in our models, moreover strongly correlated with bio12. This predictor showed a mostly positive relationship with richness, which was expected given that precipitation under extreme stress could be an important conditioner of plant survival and growth, hence of community diversity (Sklenár and Jørgensen, 1999; Sklenár and Balslev, 2005). However, this correlation was inconclusive because it was found negative in model S+H.
To conclude, our results did not support completely any of our original hypotheses on the roles of the contemporary macroclimate in form of energy, seasonality and harshness in conditioning local plant richness in the páramo. We found that the highest plant richness could exist under relatively warm conditions, with little soil water stress, where humidity was not too important, i.e., in our opinion in semi-dry climates, and under stable humidity conditions but varying temperatures. Therefore, our energy and climatic seasonality hypotheses were partly sustained, whereas harshness was not, at least at this study scale, this perhaps due to the strong overlap between the energy and harshness predictors. As a result, we suppose that including more specific bioclimatic factors representative of harshness and less correlated to energy factors, e.g., number of freezing days, could have better disentangled the harshness effects on local plant richness, however this information remains unavailable for the extent of our study.
Regional Fine-Scale Richness Patterns
Our predicted map of fine-scale richness for a standardized 25 m2 páramo plot showed important variation throughout the biogeographical province. The results forecasted a general south-north richness decrease with a marked contrast between northern and southern latitudes, which was unexpected in our original hypothesis expecting a double-ended decreasing richness gradient with latitude (Hawkins et al., 2003; Willig et al., 2003). Another unexpected finding was the overall low richness predicted for Colombia at the plot level, whereas the country is usually considered the most diverse páramo country in terms of national richness (Rangel-Churio, 2006, 2015). One potential explanation for these results might be the heterogeneity in phytosociological sampling approaches between vegetations schools in the different countries, a problem commonly encountered when using multi-sources vegetation databases (Michalcová et al., 2011). Because it is difficult to correctly evaluate, we recommend considering this potential bias carefully. Our results also predicted no-clear patterns of richness with elevation at this scale, and highlighted particularly rich páramo areas, i.e., > 35 species (per 25 m2 plot), and poor ones, i.e., < 10 species, independent of latitude and elevation.
Among the species rich areas identified were the Amotape-Huancabamba zone (~Lat. 5°S; Long. 79.5°W), the Sangay (~Lat. 2°S; Long. 78.5°W) and Cotacachi (~Lat. 0°; Long. 78°W) massifs in Ecuador, as well as the Lara páramos (~Lat. 9°N; Long. 71°W). This can be due to the geographic location of these mountain ranges, either (i) near lowland renown hotspots such as Amazonian and Chocó-Pacific biomes, in the case of Sangay and Cotacachi, respectively (Barthlott et al., 2007), or (ii) at geographic extremities of the páramo province, which promotes interchanging species with the immediate adjacent regions, such as the Llanos Occidentales in Lara and the Puna in the Amotape-Huancabamba zone (Weigend, 2002). Following that pattern, we expected the Colombian western cordillera to show high local biodiversity, but found the opposite. This might be explained by our previous interpretations on the extreme precipitation-richness relationship or by a sampling bias affecting the richness-precipitation relationship found. Previous studies had already mentioned the ecological importance of the Amotape-Huancabamba zone or easternmost Venezuelan páramos and emphasized their species richness, ecosystem diversity, endemism, and good conservation state (Lozano et al., 2009; Cuello et al., 2010). These areas remain relatively pristine thanks to direct conservation measures, for example representation in the Podocarpus and Guaramacal National Parks, as well as indirect ones, such as difficult access. Even though management efforts are in place, we believe there is a need for further research on the páramo's biogeography and global threats to reinforce preservation strategies, for example anticipating climate change (Mace et al., 2000). Although our study is exploratory and focused on the local scale, we encourage further studies to focus on these predicted species-rich areas with potential for revealing biodiversity hotspots at a broader-scale within the páramo biogeographical province. When doing so, it would be important to focus on species richness at a larger scale but also quantify ecological value in complementary ways, for example correlating richness patterns with patterns of endemism (Sklenár and Jørgensen, 1999).
Study Limitations and Future Recommendations
Our study has certain drawbacks that should be considered when interpreting the obtained results. First, the 1 km resolution of our climatic data might be considered coarse and it is possible that we did not detect valuable information due to the fine Andean topographic heterogeneity. Although we acknowledge that the fine micro- and meso-climate might play a crucial part in driving local species richness, perhaps more than the macroclimate (Anthelme and Lavergne, 2018; Graae et al., 2018), obtaining the corresponding data for the extent of our study remains considerably challenging. Second, some areas were rather under-sampled, such as the Colombian western cordillera, and it is possible that because of the resulting under-representation, their specific trends might have been overlooked in our results, making spatial interpolations from the closest sampled areas, even though useful, potentially dubious (Divíšek and Chytrý, 2018; Večera et al., 2019). We recommend additional sampling in these areas that are now more easily accessible thanks to the ended armed conflict in Colombia and in urgent need of further data exploration and associated environmental legislation (Negret et al., 2017). Last, our best performing regression models, E+S and E+S+H, only accounted for 25 percent of local species richness variation. As a result, while our results are bringing novel insight on the significant predictors of páramo plant richness, their explaining power remains relatively low and therefore, our interpretations should be handled accordingly. We believe important to focus future endeavors on additional potential richness drivers in the páramo such as the broad contemporary environment, past climate variations, disturbance variables, biome area, and biotic interactions for example (Sklenář and Ramsay, 2001; Cavieres et al., 2014; Vásquez et al., 2015). Finally, we consider that our results, despite the previously established drawbacks, advance important theories on páramo biogeography and the relevant but weak relationship between local plant richness and the macroclimate. They are also meaningful and able to guide further research and management focus on specific páramo areas identified as local biodiversity hotspots, and we believe it would be useful as a future perspective to downscale the results and focus on the specific vegetation type of phytogeographical units to identify not only geographic hotspots but habitat hotspots (Divíšek and Chytrý, 2018) with direct implications in ecosystem and biodiversity management planning.
Data Availability Statement
The data used in this study are archived and freely available on the open-access VegPáramo database webpage (www.vegparamo.com) and in Table S1.
Author Contributions
GP, HB, XF, and JT study conception and design and critical revision. GP and JT acquisition of data, analysis interpretation of data, and drafting of manuscript.
Conflict of Interest
The authors declare that the research was conducted in the absence of any commercial or financial relationships that could be construed as a potential conflict of interest.
Acknowledgments
We thank the Agency for Administration of University and Research Grants (AGAUR) from the Generalitat de Catalonia (grant FI-DGR 2011) and the University of the Andes (grant FAPA- PR.3.2016.3708) for their financial support.
Supplementary Material
The Supplementary Material for this article can be found online at: https://www.frontiersin.org/articles/10.3389/fevo.2019.00377/full#supplementary-material
Table S1. Final vegetation dataset used for the analyses and containing plot geographic information and species contents.
Figure S1. Variation in area and richness across the páramo vegetation plot data: (A) Histogram of frequencies of plot area; (B) Histogram of frequencies of local species richness at plot level.
Figure S2. Correlation matrix showing the univariate relationships among each environmental predictor for variable selection in the GLS environmental models. The color scale reflects Pearson's correlation coefficients and the “X” marks correlations that were not statistically significant. Variables for hypotheses: Energy –bio1, annual mean temperature; bio 12, annual precipitation; swcfr, mean monthly soil water stress coefficient; AET, actual evapo-transpiration; Seasonality–bio2, Mean diurnal temperature range; bio4, temperature seasonality; bio15, precipitation seasonality; bio20, inter-annual temperature variation (σ; 1979–2013); bio21, inter-annual precipitation variation (σ; 1979–2013); Harshness–bio6, minimum temperature of coldest month; bio14, precipitation of driest month; bio18, precipitation of warmest quarter.
Figure S3. Geographic patterns of local species richness values (for a 25 m2 plot) throughout the páramo biogeographical province as predicted by the models OK (A) and E+S+H (B).
References
Allen, A. P., Gillooly, J. F., and Brown, J. H. (2007). “Recasting the species-energy hypothesis: the different roles of kinetic and potential energy in regulating biodiversity” in Scaling Biodiversity, eds D. Storch, P. A. Marquet, and J. H. Brown (Cambridge: Cambridge University Press), 283–299. doi: 10.1017/CBO9780511814938.016
Anthelme, F., Jacobsen, D., Macek, P., Meneses, R. I., Moret, P., Beck, S., et al. (2014). Biodiversity patterns and continental insularity in the tropical High Andes. Arc. Antarc. Alp. Res. 46, 811–828. doi: 10.1657/1938-4246-46.4.811
Anthelme, F., and Lavergne, S. (2018). Alpine and arctic plant communities: a worldwide perspective. Perspect. Plant Ecol. Syst. 30, 1–5. doi: 10.1016/j.ppees.2017.12.002
Anthelme, F., and Peyre, G. (2019). “Biogeography of South American highlands,” in Reference Module in Earth Systems and Environmental Sciences, Encyclopedia of the World's Biomes, eds S. A. Elias, D. H. M. Alderton, V. Bliznak, J. K. Cochran, D. A. DellaSala, F. Funiciello, M. I. Goldstein, D. Gröcke, K. Lajtha, S. J. Marshall, P. Mineau, E. Wohl, and X. J. Yang (Amsterdam: Elsevier), 12. doi: 10.1016/B978-0-12-409548-9.11811-1
Araújo, M. B., Nogués-Bravo, D., Diniz-Filho, J. A. F., Haywood, A. M., Valdes, P. J., and Rahbek, C. (2008). Quaternary climate changes explain diversity among reptiles and amphibians. Ecography 31, 8–15. doi: 10.1111/j.2007.0906-7590.05318.x
Armas, C., Rodríguez-Echeverría, S., and Pugnaire, F. I. (2011). A field test of the stress-gradient hypothesis along an aridity gradient. J. Veg. Sci. 22, 818–827. doi: 10.1111/j.1654-1103.2011.01301.x
Asbjornsen, H., Goldsmith, G. R., Alvarado-Barrientos, M. S., Rebel, K., van Osch, F. P., Rietkerk, M., et al. (2011). Ecohydrological advances and applications in plant–water relations research: a review. J. Plant Ecol. 4, 3–22. doi: 10.1093/jpe/rtr005
Banerjee, S., Gelfand, A. E., and Sirmans, C. F. (2003). Directional rates of change under spatial process models. J. Am. Stat. Ass. 98, 946–954. doi: 10.1198/C16214503000000909
Barthlott, W., Hostert, A., Kier, G., Küper, W., Kreft, H., Mutke, J., et al. (2007). Geographic patterns of vascular plant diversity at continental to global scales. Erdkunde 61, 305–315. doi: 10.3112/erdkunde.2007.04.01
Beale, C. M., Lennon, J. J., Yearsley, J. M., Brewer, M. J., and Elston, D. A. (2010). Regression analysis of spatial data. Ecol. Lett. 13, 246–264. doi: 10.1111/j.1461-0248.2009.01422.x
Beck, J., Ballesteros-Mejia, L., Buchmann, C. M., Dengler, J., Fritz, S. A., Gruber, B., et al. (2012). What's on the horizon for macroecology? Ecography 35, 673–683. doi: 10.1111/j.1600-0587.2012.07364.x
Bjorholm, S., Svenning, J.-C., Skov, F., and Balslev, H. (2005). Environmental and spatial controls of palm (Arecaceae) species richness across the Americas. Glob. Ecol. Biogeogr. 14, 423–429. doi: 10.1111/j.1466-822x.2005.00167.x
Blanchet, F. G., Legendre, P., and Borcard, D. (2008). Forward selection of explanatory variables. Ecology 89, 2623–2632. doi: 10.1890/07-0986.1
Braun-Blanquet, J. (1951). Pflanzensoziologie. Grundzfige der Vegetationskunde, 2nd ed. Vienna: Springer.
Bruelheide, H., Dengler, J., Jiménez-Alfaro, B., Purschke, O., Hennekens, S. M., Chytrý, M., et al. (2019). sPlot–A new tool for global vegetation analyses. J. Veg. Sci. 30, 161–186. doi: 10.1111/jvs.12710
Burnham, K. P., and Anderson, D. R. (2002). Model Selection and Multimodel Inference: A Practical Information-Theoretic Approach, 2nd edn. New York, NY: Springer-Verlag.
Butterfield, B. J., Bradford, J. B., Armas, C., Prieto, I., and Pugnaire, F. I. (2016). Does the stress-gradient hypothesis hold water? Disentangling spatial and temporal variation in plant effects on soil moisture in dryland systems. Func. Ecol. 30, 10–19. doi: 10.1111/1365-2435.12592
Buytaert, W., Célleri, R., De Bièvre, B., Cisneros, F., Wyseure, G., Deckers, J., et al. (2006). Human impact on the hydrology of the Andean páramos. Earth-Sci. Rev. 79, 53–72. doi: 10.1016/j.earscirev.2006.06.002
Cavieres, L. A., Brooker, R. W., Butterfield, B. J., Cook, B. J., Kikvidze, Z., Lortie, C. J., et al. (2014). Facilitative plant interactions and climate simultaneously drive alpine plant diversity. Ecol. Lett.. 17, 193–202. doi: 10.1111/ele.12217
CHELSA Project 1.1 (2017). Available online at: www.chelsa-climate.org
Cleef, A. M., Rangel-Churio, J. O., Salamanca, S., Ariza, C., and van Reenen, G. B. A. (2005). “La vegetación del páramo del Macizo de Tatamá, Cordillera Occidental, Colombia” in La Cordillera Occidental Colombiana Transecto Tatama. Studies on Tropical Andean Ecosystems, Vol. 6, eds T. Van der Hammen, J. O. Rangel-Churio, and A. M. Cleef (Berlin; Stuttgart: J. Cramer, 377–468.
Cuello, N., Cleef, A. M., and Aymard, G. (2010). Phytogeography of the vascular páramo flora of Ramal de Guaramacal (Andes, Venezuela) and its ties to other páramo floras. An. Jard. Bot. Madrid 67, 177–193. doi: 10.3989/ajbm.2253
Cuello, N. L., and Cleef, A. M. (2009). The páramo vegetation of Ramal de Guaramacal, Trujillo State, Venezuela. 1. Zonal communities. Phytocoenologia 39, 295–329. doi: 10.1127/0340-269X/2009/0039-0295
Cuesta, F., Muriel, P., Llambí, L. D., Halloy, S., Aguirre, N., Beck, S., et al. (2017). Latitudinal and altitudinal patterns of plant community diversity on mountain summits across the tropical Andes. Ecography 40, 1381–1394. doi: 10.1111/ecog.02567
Currie, D. J., Mittelbach, G. G., Cornell, H. V., Field, R., Guégan, J.-F., Hawkins, B. A., et al. (2004). Predictions and tests of climate-based hypotheses of broad-scale variation in taxonomic richness. Ecol. Lett. 7, 1121–1134. doi: 10.1111/j.1461-0248.2004.00671.x
D'Amen, M., Pradervand, J. N., and Guisan, A. (2015). Predicting richness and composition in mountain insect communities at high resolution: a new test of the SESAM framework. Glob. Ecol. Biogeogr. 24, 1443–1453. doi: 10.1111/geb.12357
Divíšek, J., and Chytrý, M. (2018). High-resolution and large-extent mapping of plant species richness using vegetation-plot databases. Ecol. Indic. 89, 840–851. doi: 10.1016/j.ecolind.2017.11.005
Evans, K. L., Warren, P. H., and Gaston, K. J. (2005). Species-energy relationships at the macroecological scale: a review of the mechanisms. Biol. Rev. 80, 1–25. doi: 10.1017/S1464793104006517
Fagua, J. C., and Gonzalez, V. H. (2007). Growth rates, reproductive phenology, and pollination ecology of Espeletia grandiflora (Asteraceae), a giant Andean caulescent rosette. Plant Biol. 9, 127–135. doi: 10.1055/s-2006-924544
Field, R., Hawkins, B. A., Cornell, H. V., Currie, D. J., Diniz-Filho, J. A. F., Guégan, J.-F., et al. (2009). Spatial species-richness gradients across scales: a meta-analysis. J. Biogeogr. 36, 132–147. doi: 10.1111/j.1365-2699.2008.01963.x
Fine, P. V. (2015). Ecological and evolutionary drivers of geographic variation in species diversity. Annu. Rev. Ecol. Evol. Syst. 46, 369–392. doi: 10.1146/annurev-ecolsys-112414-054102
Fordham, D. A., Saltré, F., Brown, S. C., Mellin, C., and Wigley, T. M. (2018). Why decadal to century timescale palaeoclimate data are needed to explain present-day patterns of biological diversity and change. Glob. Chang. Biol. 24, 1371–1381. doi: 10.1111/gcb.13932
Francis, A. P., and Currie, D. J. (2003). A globally consistent richness-climate relationship for angiosperms. Am. Nat. 161, 523–536. doi: 10.1086/368223
Gillooly, J. F., Allen, A. P., West, G. B., and Brown, J. H. (2005). The rate of DNA evolution: effects of body size and temperature on the molecular clock. Proc. Nat. Acad. Sci. U.S.A. 102, 140–145. doi: 10.1073/pnas.0407735101
Gillooly, J. F., Brown, J. H., West, G. B., Savage, V. M., and Charnov, E. L. (2001). Effects of size and temperature on metabolic rate. Science 293, 2248–2251. doi: 10.1126/science.1061967
Graae, B. J., Vandvik, V., Armbruster, W. S., Eiserhardt, W. L., Svenning, J. C., Hylander, K., et al. (2018). Stay or go–how topographic complexity influences alpine plant population and community responses to climate change. Perspect. Plant. Ecol. Syst. 30, 41–50. doi: 10.1016/j.ppees.2017.09.008
Hawkins, B. A., Field, R., Cornell, H. V., Currie, D. J., Guégan, J.-F., Kaufman, D. M., et al. (2003). Energy, water, and broad-scale geographic patterns of species richness. Ecology 84, 3105–3117. doi: 10.1890/03-8006
Herzog, S. K., Martínez, R., Jørgensen, P. M., and Tiessen, H. (2011). “Climate change and biodiversity in the tropical Andes,” in Inter-American Institute for Global Change Research (IAI) and Scientific Committee on Problems of the Environment (SCOPE) (São José dos Campos), 348.
Inchausti, P., and Halley, J. (2003). On the relation between temporal variability and persistence time in animal populations. J. Anim. Ecol. 72, 899–908. doi: 10.1046/j.1365-2656.2003.00767.x
Izco, J., Pulgar, I., Aguirre, Z., and Santin, F. (2007). Estudio florístico de los páramos de pajonal meridionales de Ecuador. Rev. Per. Biol. 14, 237–246. doi: 10.15381/rpb.v14i2.1783
Jablonski, D., Roy, K., and Valentine, J. W. (2006). Out of the tropics: evolutionary dynamics of the latitudinal diversity gradient. Science 314, 102–106. doi: 10.1126/science.1130880
Jacobsen, D. (2003). Altitudinal changes in diversity of macroinvertebrates from small streams in the Ecuadorian Andes. Arch. Hydrobiol. 158, 145–167. doi: 10.1127/0003-9136/2003/0158-0145
Jetz, W., and Fine, P. V. (2012). Global gradients in vertebrate diversity predicted by historical area-productivity dynamics and contemporary environment. PLoS Biol. 10:e1001292. doi: 10.1371/journal.pbio.1001292
Kammer, P. M., and Möhl, A. (2002). Factors controlling species richness in alpine plant communities: an assessment of the importance of stress and disturbance. Arc. Antarc. Alp. Res. 34, 398–407. doi: 10.1080/15230430.2002.12003510
Karger, D. N., Conrad, O., Böhner, J., Kawohl, T., Kreft, H., Soria-Auza, R. W., et al. (2017). Climatologies at high resolution for the earth's land surface areas. Sci. Data 4:170122. doi: 10.1038/sdata.2017.122
Kennedy, D., and Norman, C. (2005). What don't we know? Science 309:75. doi: 10.1126/science.309.5731.75
Knapp, A. K., Beier, C., Briske, D. D., Classen, A. T., Luo, Y., and Reichstein, M. (2008). Consequences of more extreme precipitation regimes for terrestrial ecosystems. AIBS Bull. 58, 811–821. doi: 10.1641/B580908
Knapp, A. K., Fay, P. A., Blair, J. M., Collins, S. L., Smith, M. D., Carlisle, J. D., et al. (2002). Rainfall variability, carbon cycling, and plant species diversity in a mesic grassland. Science 298, 2202–2205. doi: 10.1126/science.1076347
Kramer, P. J., and Boyer, J. S. (1995). Water Relations of Plants and Soils. San Diego, CA: Academic press.
Kreft, H., and Jetz, W. (2007). Global patterns and determinants of vascular plant diversity. Proc. Natl. Acad. Sci. U.S.A. 104, 5925–5930. doi: 10.1073/pnas.0608361104
Lomolino, M. V. (2000). Ecology's most general, yet protean pattern: the species-area relationship. J. Biogeogr. 27, 17–26. doi: 10.1046/j.1365-2699.2000.00377.x
Lomolino, M. V., and Heaney, L. R. (2004). Frontiers of Biogeography: New Directions in the Geography of Nature. Sunderland: Sinauer Associates.
Londoño, C., Cleef, A., and Madriñán, S. (2014). Angiosperm flora and biogeography of the páramo region of Colombia, Northern Andes. Flora 209, 81–87. doi: 10.1016/j.flora.2013.11.006
Lozano, P., Cleef, A. M., and Bussmann, R. (2009). Phytogeography of the vascular páramo flora of Podocarpus National Park, South Ecuador. Arnaldoa 16, 69–85.
Luteyn, J. (1999). Páramos: A Checklist of Plant Diversity, Geographic Distribution, and Botanical Literature. Bronx, NY: New York Botanical Garden Press.
MacArthur, R. H., Diamond, J. M., and Karr, J. R. (1972). Density compensation in island faunas. Ecology 53, 330–342. doi: 10.2307/1934090
Mace, G. M., Balmford, A., Boitani, L., Cowlishaw, G., Dobson, A. P., Faith, D. P., et al. (2000). It's time to work together and stop duplicating conservation efforts. Nature 405 :393. doi: 10.1038/35013247
Madriñan, S., Cortés, A. J., and Richardson, J. E. (2013). Páramo is the world's fastest evolving and coolest biodiversity hotspot. Front. Genet. 4, 1–6. doi: 10.3389/fgene.2013.00192
McCain, C. M., and Grytnes, J. A. (2010). “Elevational gradients in species richness,” in Encyclopedia of Life Sciences (ELS) (Chichester: John Wiley and Sons), 10. doi: 10.1002/9780470015902.a0022548
Michalcová, D., Lvončík, S., Chytrý, M., and Hájek, O. (2011). Bias in vegetation databases? A comparison of stratified-random and preferential sampling. J. Veg. Sci. 22, 281–291. doi: 10.1111/j.1654-1103.2010.01249.x
Miriti, M. N., Rodríguez-Buriticá, S., Wright, S. J., and Howe, H. F. (2007). Episodic death across species of desert shrubs. Ecology 88, 32–36. doi: 10.1890/0012-9658(2007)88[32:EDASOD]2.0.CO;2
Mittelbach, G. G., Schemske, D. W., Cornell, H. V., Allen, A. P., Brown, J. M., Bush, M. B., et al. (2007). Evolution and the latitudinal diversity gradient: speciation, extinction and biogeography. Ecol. Lett. 10, 315–331. doi: 10.1111/j.1461-0248.2007.01020.x
Mod, H. K., le Roux, P. C., Guisan, A., and Luoto, M. (2015). Biotic interactions boost spatial models of species richness. Ecography 38, 913–921. doi: 10.1111/ecog.01129
Moret, P. (2009). Altitudinal distribution, diversity and endemicity of Carabidae (Coleoptera) in the páramos of Ecuadorian Andes. Ann. Soc. Entomol. Fr. 45, 500–510. doi: 10.1080/00379271.2009.10697632
Morrone, J. J. (2014). Biogeographical regionalisation of the Neotropical region. Zootaxa 3782, 1–110. doi: 10.11646/zootaxa.3782.1.1
Morueta-Holme, N., Enquist, B. J., McGill, B. J., Boyle, B., Jørgensen, P. M., Ott, J. E., et al. (2013). Habitat area and climate stability determine geographical variation in plant species range sizes. Ecol. Lett. 16, 1446–1454. doi: 10.1111/ele.12184
Myers, N., Mittermeier, R. A., Mittermeier, C. G., da Fonseca, G. A., and Kent, J. (2000). Biodiversity hotspots for conservation priorities. Nature 403, 853–858. doi: 10.1038/35002501
Negret, P. J., Allan, J., Braczkowski, A., Maron, M., and Watson, J. E. (2017). Need for conservation planning in postconflict Colombia. Conserv. Biol. 31, 499–500. doi: 10.1111/cobi.12935
Nürk, N. M., Scheriau, C., and Madriñan, S. (2013). Explosive radiation in high Andean Hypericum-rates of diversification among New World lineages. Front. Gen. 4:175. doi: 10.3389/fgene.2013.00175
Oksanen, J., Blanchet, F. G., Kindt, R., Legendre, P., Minchin, P. R., O'Hara, R. B., et al. (2012). Vegan: Community Ecology Package - R Package Version 2.0-4. Available onlline at: http://CRAN.R-project.org/package=vegan
Ordonez, A., and Svenning, J. C. (2017). Consistent role of Quaternary climate change in shaping current plant functional diversity patterns across European plant orders. Sci. Rep. 7:42988. doi: 10.1038/srep42988
Peyre, G., Balslev, H., and Font, X. (2018). Phytoregionalisation of the Andean páramo. PeerJ. 6:e4786. doi: 10.7717/peerj.4786
Peyre, G., Balslev, H., Martí, D., Sklenár, P., Ramsay, P., Lozano, P., et al. (2015). VegPáramo, a flora and vegetation database for the Andean páramo. Phytocoenologia 45, 195–201. doi: 10.1127/phyto/2015/0045
Pfeifer, R., Stadler, J., and Roland, B. (2018). Is the seasonal variation of abundance and species richness in birds explained by energy availability? Acta Ornithol. 52, 167–179. doi: 10.3161/00016454AO2017.52.2.005
Pfitsch, W. A. (1988). Microenvironment and the distribution of two species of Draba (Brassicaceae) in a Venezuelan paramo. Arct. Antarct. Alp. Res. 20, 333–341. doi: 10.2307/1551265
Pianka, E. R. (1966). Latitudinal gradients in species diversity: a review of concepts. Am. Nat. 100, 33–46. doi: 10.1086/282398
R Core Team 3.3.1 (2013). R: A Language and Environment for Statistical Computing. R Foundation for Statistical Computing, Vienna, Austria. URL Available online at: http://www.R-project.org/
Rahbek, C. (1995). The elevational gradient of species richness – a uniform pattern. Ecography 18, 200–205. doi: 10.1111/j.1600-0587.1995.tb00341.x
Rajakaruna, H., and Lewis, M. (2018). Do yearly temperature cycles reduce species richness? Insights from calanoid copepods. Theor. Ecol. 11, 39–53. doi: 10.1007/s12080-017-0347-y
Rangel-Churio, J. O. (2006). “The Biodiversity of the Colombian Páramo and Its Relation to Anthropogenic” in Land Use Change and Mountain Biodiversity 103, Chapter 8, eds E. M. Spehn, M. Liberman, and C. Körner (Boca Raton, FL: CRC Press), 103–118. doi: 10.1201/9781420002874-8
Rangel-Churio, J. O. (2015). La biodiversidad de Colombia: significado y distribución regional. Rev. Acad. Colomb. Cienc. Exactas Fis. Nat. 39, 176–200. doi: 10.18257/raccefyn.136
Ricklefs, R. E. (2006). Evolutionary diversification and the origin of the diversity–environment relationship. Ecology 87. doi: 10.1890/0012-9658(2006)87[3:EDATOO]2.0.CO;2
Sites, R. W., Willig, M. R., and Linit, M. J. (2003). Macroecology of aquatic insects: a quantitative analysis of taxonomic richness and composition in the Andes mountains of northern Ecuador. Biotropica 35, 226–239. doi: 10.1111/j.1744-7429.2003.tb00282.x
Sklen,ár, P., and Lægaard, S. (2003). Rain-shadow in the high Andes of Ecuador evidenced by paramo vegetation. Arct. Antarct. Alp. Res. 35, 8–17. doi: 10.1657/1523-0430(2003)035[0008:RSITHA]2.0.CO;2
Sklenár, P., and Balslev, H. (2005). Superpáramo plant species diversity and phytogeography in Ecuador. Flora 200, 416–433. doi: 10.1016/j.flora.2004.12.006
Sklenár, P., Bendix, J., and Balslev, H. (2008). Cloud frequency correlates to plant species composition in the high Andes of Ecuador. Basic Appl. Ecol. 9, 504–513. doi: 10.1016/j.baae.2007.09.007
Sklenár, P., Hedberg, I., and Cleef, A. M. (2014). Island biogeography of tropical alpine floras. J. Biogeogr. 41, 287–297. doi: 10.1111/jbi.12212
Sklenár, P., and Jørgensen, P. (1999). Distribution patterns of páramo plants in Ecuador. J. Biogeogr. 26, 681–691. doi: 10.1046/j.1365-2699.1999.00324.x
Sklenář, P., and Ramsay, P. M. (2001). Diversity of zonal páramo plant communities in Ecuador. Div. Distrib. 7, 113–124. doi: 10.1046/j.1472-4642.2001.00101.x
Smith, M. D. (2011). The ecological role of climate extremes: current understanding and future prospects. J. Ecol. 99, 651–655. doi: 10.1111/j.1365-2745.2011.01833.x
Smith, W. K., and McClean, T. M. (1989). Adaptive relationship between leaf water repellency, stomatal distribution, and gas exchange. Am. J. Bot. 76, 465–469. doi: 10.1002/j.1537-2197.1989.tb11335.x
Somveille, M., Rodrigues, A. S., and Manica, A. (2015). Why do birds migrate? A macroecological perspective. Glob. Ecol. Biogeogr. 24, 664–674. doi: 10.1111/geb.12298
Srivastava, D. S., and Lawton, J. H. (1998). Why more productive sites have more species: an experimental test of theory using tree-hole communities. Am. Nat. 152, 510–529. doi: 10.1086/286187
Stansell, N. D., Abbott, M. B., Polissar, P. J., Wolfe, A. P., Bezada, M., and Rull, V. (2005). Late Quaternary deglacial history of the Mérida Andes, Venezuela. J. Quat. Sci. 20, 801–812. doi: 10.1002/jqs.973
Tello, J. S., and Stevens, R. D. (2010). Multiple environmental determinants of regional species richness and effects of geographic range size. Ecogr. 33, 796–808. doi: 10.1111/j.1600-0587.2009.05991.x
Thibault, K. M., and Brown, J. H. (2008). Impact of an extreme climatic event on community assembly. Proc. Nat. Acad. Sci. U.S.A. 105, 3410–3415. doi: 10.1073/pnas.0712282105
Trabucco, A., and Zomer, R. J. (2010). Global Soil Water Balance Geospatial Database. CGIAR Consortium for Spatial Information. Available online at: http://cgiar-csi.org
Ulloa Ulloa, C., Acevedo-Rodríguez, P., Beck, S., Belgrano, M. J., Bernal, R., Berry, P. E., et al. (2017). An integrated assessment of the vascular plant species of the Americas. Science 358, 1614–1617. doi: 10.1126/science.aao0398
Vásquez, D. L., Balslev, H., and Sklenár, P. (2015). Human impact on tropical-alpine plant diversity in the northern Andes. Biodivers. Conserv. 24, 2673–2683. doi: 10.1007/s10531-015-0954-0
Večera, M., Divíšek, J., Lenoir, J., Jiménez-Alfaro, B., Biurrun, I., Knollová, I., et al. (2019). Alpha diversity of vascular plants in European forests. J. Biogeogr. 46, 1–17. doi: 10.1111/jbi.13624
Weigend, M. (2002). Observations on the biogeography of the Amotape-Huancabamba zone in northern Peru. Bot. Rev. 68, 38–54. doi: 10.1663/0006-8101(2002)068[0038:OOTBOT]2.0.CO;2
Willig, M. R., Kaufman, D. M., and Stevens, R. D. (2003). Latitudinal gradients of biodiversity: pattern, process, scale, and synthesis. Ann. Rev. Ecol. Evol. Syst. 34, 273–309. doi: 10.1146/annurev.ecolsys.34.012103.144032
Wilson, J. B., Peet, R. K., Dengler, J., and Pärtel, M. (2012). Plant species richness: the world records. J. Veg. Sci. 23, 796–802. doi: 10.1111/j.1654-1103.2012.01400.x
Keywords: contemporary macroclimate, High Andes, Kriging model, plant diversity, regression model, richness patterns
Citation: Peyre G, Balslev H, Font X and Tello JS (2019) Fine-Scale Plant Richness Mapping of the Andean Páramo According to Macroclimate. Front. Ecol. Evol. 7:377. doi: 10.3389/fevo.2019.00377
Received: 14 June 2019; Accepted: 19 September 2019;
Published: 15 October 2019.
Edited by:
Anouschka R. Hof, Wageningen University & Research, NetherlandsReviewed by:
John Arvid Grytnes, University of Bergen, NorwayMichael Kessler, University of Zurich, Switzerland
Copyright © 2019 Peyre, Balslev, Font and Tello. This is an open-access article distributed under the terms of the Creative Commons Attribution License (CC BY). The use, distribution or reproduction in other forums is permitted, provided the original author(s) and the copyright owner(s) are credited and that the original publication in this journal is cited, in accordance with accepted academic practice. No use, distribution or reproduction is permitted which does not comply with these terms.
*Correspondence: Gwendolyn Peyre, gf.peyre@uniandes.edu.co