- Centre for Biodiversity Genomics, Department of Integrative Biology, University of Guelph, Guelph, ON, Canada
DNA-based methodology has proven to be a vital tool for ecosystem assessment and monitoring. Increasingly, high-throughput approaches such as DNA metabarcoding are being used to address more complex questions, including ecological network analyses through machine learning. Despite the technological advances which allow for such questions to be posed, there remains inherent limitations in studies utilizing DNA metabarcoding, referring to environmental sample type targeted, geographical coverage and lack of standardized field and laboratory procedures. Additionally, DNA reference databases are lacking information from taxa, resulting in unidentified sequences, and underrepresentation of some taxa. These issues need to be addressed to enable a more representative approach to ecosystem monitoring to allow for detection and monitoring of global ecosystem change.
To better determine the global effects that the changing climate and anthropogenic damage have on the planets' ecosystems requires a more complete understanding of the global biodiversity than currently exists. However, this has been extremely difficult to ascertain and standardize due to the large number of taxa and the diversity of different geographic localities. More confounding is the reality that these natural and man-made changes are increasingly reshaping the global biodiversity and the associated ecosystem processes and services they provide (Díaz et al., 2015; Bohan et al., 2017). Unfortunately, to date, scientists studying the connections between biodiversity and ecosystem change in specific ecosystems have been poorly equipped to measure these relationships, and have tended to rely on the taxonomic identity and biomonitoring indicators collected from other, and perhaps distant areas, which may or may not be appropriate or accurate choices (Bohan et al., 2017).
DNA metabarcoding utilizes bulk samples such as soil, water, and benthos to extract DNA (termed environmental DNA, eDNA) and generate sequence data for standard taxonomic marker genes (e.g., DNA barcodes) via high-throughput sequencing (Porter and Hajibabaei, 2018b). By streamlining and scaling-up biodiversity data generated, DNA metabarcoding provides the ability to increase the amounts of assessment of the status of biodiversity associated with ecosystem change that can occur across a wide range of global ecosystems (Ruppert et al., 2019). The approach is cost-effective, easy to implement, and provides a robust and comprehensive dataset of taxa from environmental samples, making DNA metabarcoding an important tool of choice for future fundamental research and large-scale biodiversity monitoring programs (Zinger et al., 2019). Moreover, DNA metabarcoding provides an important component to be used with the ecological network analyses and machine learning algorithms that are rapidly advancing to enhance the capacity to detect global ecosystem change through biodiversity assessment (Bohan et al., 2017; Cordier et al., 2019). The complex relationships between changes in nodes and links, and their impact on ecosystem functions should be understood at the network level if we are to develop more robust biomonitoring (Bohan et al., 2017). That said, there are still various barriers that need to be overcome in order to accurately and effectively detect such global ecosystem change, regardless of how quickly these technologies and analyses advance.
DNA metabarcoding has been used to assess eukaryotic and prokaryotic communities, to answer ecological questions such as identifying soil microbiome communities associated with nitrogen-fixing tree species in secondary tropical forests (McGee et al., 2019), assessing bioindicators of river health through macroinvertebrate biomonitoring (Hajibabaei et al., 2011; Dowle et al., 2016) and investigating the effects of oil spills on coastal biodiversity (Xie et al., 2018). Robust experimental design is vital to ensure reproducibility and the ability to draw sound ecological conclusions from the data (Fahner et al., 2018; Zinger et al., 2019). Type I and Type II errors are common with DNA-based biomonitoring, and to overcome this, firstly the sampling design needs to be effective at capturing the full taxonomic diversity or the ecological processes being investigated (Zinger et al., 2019). Secondly, the laboratory and bioinformatic workflow should be optimized to reduce sampling, extraction, amplification, or sequencing bias (Fahner et al., 2018; Ruppert et al., 2019; Zinger et al., 2019). For detecting biodiversity changes, both the taxonomic reference database (for taxonomic annotation of sequences), and environmental sample type (as a proxy for biodiversity) need to be efficient and suitable for detection of target taxa (Ruppert et al., 2019). Geographic variability of environmental sample types also needs to be taken into consideration, to provide the most inclusive representation of taxa, which is vital for detecting biodiversity change within different ecosystems.
Ecological network analyses are becoming an increasingly popular approach to study how ecosystems respond to change and the functional implications of these responses. Typically, network analyses are able to link together species indicators, gathered via DNA metabarcoding methods and others, and functions/interactions to represent a totality of nodes as an ecosystem model (Bohan et al., 2017; Laroche et al., 2018). Network structures can elucidate environmental shifts from stable ecosystem states (Beisner et al., 2003; Bohan et al., 2017; Derocles et al., 2018) through changes that occur in species composition and manifest in an ecological network. These ecological network analyses can potentially explain and possibly predict why stable states in ecology can persist over a period of time (Carpenter et al., 2001; Scheffer et al., 2001; Beisner et al., 2003; Bohan et al., 2017), in order to aid advancements in global biomonitoring. Network analyses, combined with machine learning algorithms, provide a standardized and sensitive method at a high resolution to foster a general understanding of the current state of ecosystem function across the globe (Vacher et al., 2016; Bohan et al., 2017; Derocles et al., 2018).
However, even if we advance the technologies behind these network and machine learning methods, the reference databases for taxonomic identification, sample type, and geographical location remain as the most influential limitations to advancing an understanding of detecting global ecosystem change. Next-generation biomonitoring involves the isolation of DNA from samples including freshwater (Valentini et al., 2016; Muha et al., 2017; Harper et al., 2019), salt/brackish water (Lobo et al., 2017; Aylagas et al., 2018; Hansen et al., 2018), benthos (Hajibabaei et al., 2011; Turner et al., 2015; Aylagas et al., 2016; Robinson et al., 2019; Salonen et al., 2019), soil (Andersen et al., 2012; Yoccoz et al., 2012; Fahner et al., 2016; McGee et al., 2019), permafrost (Bellemain et al., 2013; Zielińska et al., 2017; Zimmermann et al., 2017), passive biomass collection efforts such as malaise traps (Morinière et al., 2016; Adamowicz et al., 2019), and more recently air (Kraaijeveld et al., 2015; Ferguson et al., 2019). Within these different types of environmental samples, there are taxa which are either unique to a particular sample type or can be detected across a breadth of environments, which ultimately influences the ecological questions that can be addressed with each type of environmental sample (Ruppert et al., 2019). In a brief, robust Web of Knowledge hit search from the last 5 years (2015–2019), using various search terms to show where various sample types are popularly collected, or sample type (i.e., water, soil, benthos), suggested that samples may be substantially lacking in various geographical regions. Overall, tropic* returned the greatest number of searches for environmental DNA/eDNA/metabarcoding studies (n = 319), followed by Arctic/Antarctic/polar (n = 262), and then temperate (n = 188; Table 1). What this brief hit search does not highlight is the lack of geographic coverage within some geographic regions. For example, despite temperate returning the fewest searches for environmental DNA/eDNA/metabarcoding studies, the range of sample localities is vaster than for both the tropics and arctic/Antarctic/polar regions. Studies returned for the temperate region include localities such as Asia, United Kingdom, Canada and France, whereas for Antarctic for example, the studies are concentrated around remote field stations on the Antarctic peninsula. In terms of sample type, soil environmental DNA/eDNA studies return more searches in temperate locations, whereas permafrost and benthos/sediment return a greater percentage of searches from arctic/Antarctic/polar regions (Table 2; Figure 1). Water, river/stream/pond/lake and seawater/marine return relatively even percentage of searches across the three geographic regions (Table 2; Figure 1).
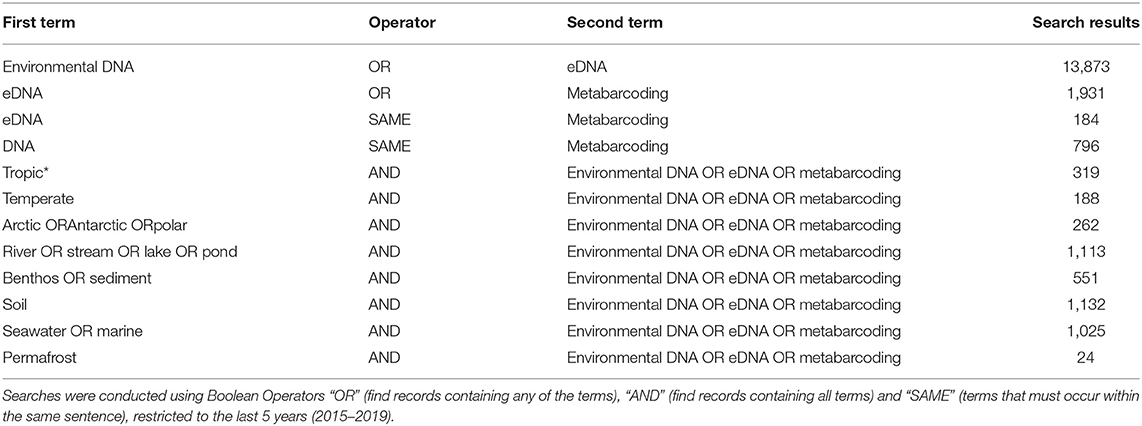
Table 1. Results of Web of Knowledge searches returned for different environmental DNA/eDNA/metabarcoding, search terms (found anywhere in the article), associated with different sample types (water, river/stream/lake/pond, benthos/sediment, soil, seawater/marine, and permafrost), or geographic region (tropic*, temperate, Arctic/Antarctic/polar).
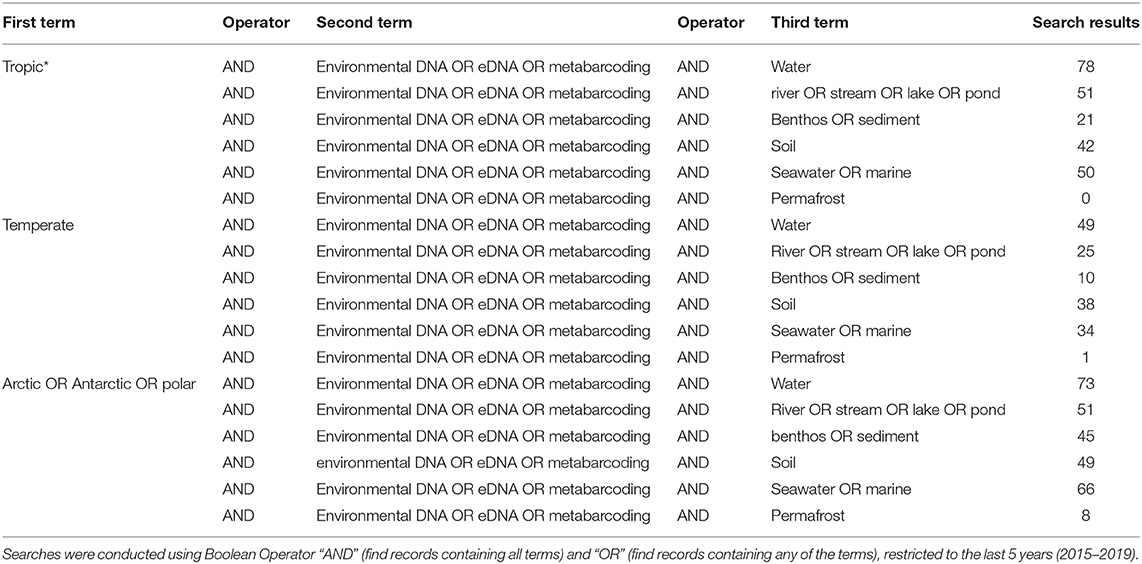
Table 2. Results of Web of Knowledge searches returned for different environmental DNA/eDNA/metabarcoding, search terms (found anywhere in the article), associated with different sample types (water, river/stream/lake/pond, benthos/sediment, soil, seawater/marine, and permafrost) for each geographic region (tropic*, temperate, Arctic/Antarctic/polar).
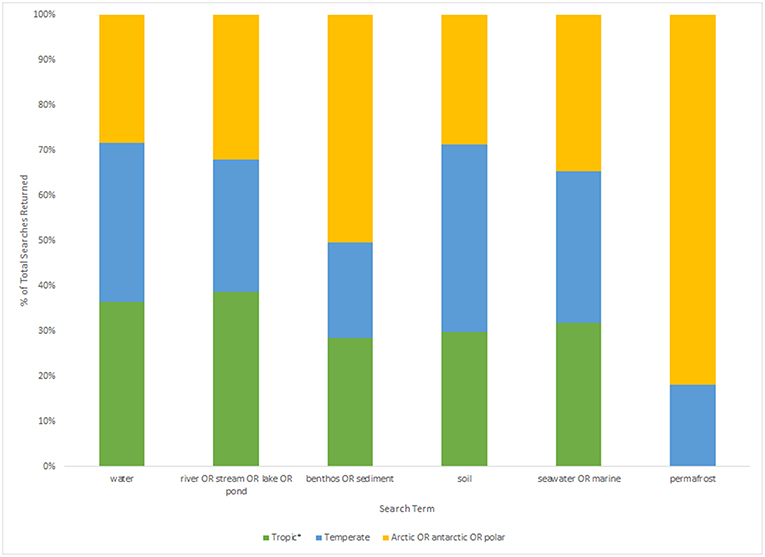
Figure 1. Histogram displaying the percentage of total results returned (tropic*: n = 242, temperate: n = 157, arctic/Antarctic/polar: n = 292; based on Table 2) for each sample type search term (water, river/stream/lake/pond, benthos/sediment, soil, seawater/marine, and permafrost) for each geographic region.
Often, one type of environmental sample is collected in an attempt to answer broad ecological questions regarding an ecosystem, such as a watershed (Dickie et al., 2018). However, this is problematic and can lead to bias in terms of taxa recovered (Baird and Hajibabaei, 2012; Taberlet et al., 2018). In addition to the geographic location of sample collection, sample type is a large bottleneck in terms of taxa recovered (Figure 2). For example, recent studies have found that eDNA samples from freshwater are a poor substitute for bulk-benthos samples for assessing macroinvertebrate community assemblages (Macher et al., 2018; Hajibabaei et al., 2019). Furthermore, the terminology surrounding the types of environmental sample is inconsistent across the literature, with variations of “eDNA” and “bulk-tissue DNA” used interchangeably (Dickie et al., 2018). Often aquatic-based DNA monitoring samples are referred to as “eDNA” (e.g., Valentini et al., 2016; Deiner et al., 2017), whereas sediment/benthos or soil samples are termed “bulk-tissue DNA” (Hatzenbuhler et al., 2017; Hajibabaei et al., 2019; Harper et al., 2019), despite these types of sample all referring to DNA which is isolated from an environmental sample (Dickie et al., 2018). This lack of consistency is particularly challenging when attempting to amalgamate literature and compare studies from different research groups and for effectively communicating results of DNA-based studies to non-specialists. Going forward, it would be greatly beneficial to have a consistent and shared ontology across the environmental DNA and metabarcoding community in terms of environmental sample type. Although eDNA could provide an all-encompassing term for analysis of DNA from environmental samples, it is important to provide complementary information about sample type (e.g., soil, water, and benthos) and technology used for detection in all scientific/technical communication. To fully investigate the current uses of DNA-based terminology, an in-depth review would be necessary, which is beyond the scope of this paper. Ultimately, different types of environmental samples, with their varying associated terminologies, are likely to reflect specific communities of taxa based on factors such as life histories, season and geographic location (Thomsen and Willerslev, 2015; Dickie et al., 2018), and if global ecological questions are to be addressed using next-generation biomonitoring, sample design will need to incorporate the processing of multiple sample types for accurate assessments of biodiversity.
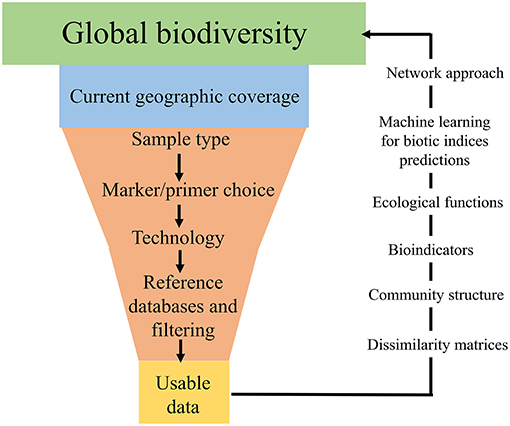
Figure 2. Infographic displaying the “bottlenecks” associated with global DNA metabarcoding data generation.
In addition, there is a substantial degree of variation within metabarcoding as to the sequencing technology implemented for data generation (Bleidorn, 2016; Evans et al., 2016; Elbrecht and Steinke, 2019; Singer et al., 2019; Zinger et al., 2019). As of 2015, there were 13 different PCR-based NGS technologies (Pavan-Kumar et al., 2015), with Illumina® MiSeq currently the prominent NGS platform for processing biomonitoring data (Bleidorn, 2017). In terms of sequencing, different environmental sample types require varying degrees of sequencing breadth and depth (Porter and Hajibabaei, 2018b; Singer et al., 2019). Tropical forest soils are considered to be one of the most diverse ecosystems on the planet, in comparison to alpine mountain lakes, which have vastly different biological richness (Schluter and Pennell, 2017; Dumbrell, 2019). For example, two separate studies looking at microbial community structure in tropical soils and alpine lakes, produced a large difference in sequence reads for the two environments (tropical soil 16s: 1.3 million; alpine lake: 184,273; Filker et al., 2016; Dopheide et al., 2019). In addition, detection of whole communities as opposed to fewer taxa will require a greater sequencing depth (Porter and Hajibabaei, 2018b). Similar to environmental sample type, the sequencing process of DNA-based biomonitoring is often referred to as “NGS,” “High-throughput sequencing (HTS),” and “Second-generation sequencing (2GS)” (Dickie et al., 2018; Divoll et al., 2018; Zinger et al., 2019); this varying use of terminology again adds another level of inconsistency to DNA-based biomonitoring. Referring to a consistent term for this sequencing technique, similar to the ontology discussed for sample terminology, would be beneficial. As many companies, such as illumina®, which produce sequencing equipment, often refer to this sequencing technology as “next-generation sequencing,” therefore it would be logical to maintain consistency with this term (von Bubnoff, 2008; Quail et al., 2012). As with sample terminology, it is necessary to provide complementary information regarding the technological processes (i.e., high-throughput targeted sequencing). Since January 2016, there have been a few publications referring to the use of Illumina®'s newest high-capacity platform, NovaSeq (Singer et al., 2019) in metabarcoding studies, which have highlighted the higher performance of this new technology in comparison to both the HiSeq and MiSeq, with NovaSeq detecting 40% more metazoan families in metabarcoded sea water samples in comparison to the MiSeq (Singer et al., 2019). The implementation of new technology brings to light the need for evaluating available technologies to address biomonitoring needs for a given system with the main limitation being the taxonomic coverage achieved per sample (Divoll et al., 2018). For example, MiSeq may provide optimal solution to tackle biodiversity in freshwater systems or specific taxonomic assemblages whereas NovaSeq would be a better platform for more complex situations such as oceanic samples. Suboptimal use of data generation platforms could lead to misrepresentation of taxonomic information and can be problematic when considering the implications of this on the ecological conclusions already having been drawn from metabarcoding-based biomonitoring data (Zinger et al., 2019).
Environmental sample choice and implementation of different sequencing platforms are not the only sources of taxa detection bias (Figure 2). There are numerous bioinformatic pipelines for processing samples, which vary greatly across studies (Alberdi et al., 2018) and appropriate clustering/filtering thresholds can lead to mis-classification and thus bias in the taxa detected (Hajibabaei et al., 2016; Alberdi et al., 2018; Zinger et al., 2019). In addition, the most prominent bottleneck in terms of recovering present taxa in an environmental sample is incomplete DNA reference databases (Figure 2; Zaiko et al., 2015; Elbrecht et al., 2017; Stat et al., 2017). Commonly used, both the BOLD (Barcode of Life Datasystem) and GenBank databases regularly lack reference sequences and/or have conflicting taxonomic assignments for the species (Ammon et al., 2018). Reference database incompletion causes inability to identify all DNA sequences in a sample and means some taxonomic groups are underrepresented (Creer et al., 2010; Ratnasingham and Hebert, 2013; Porter and Hajibabaei, 2018a), which highlights the current substantial gap in global biodiversity knowledge (Zaiko et al., 2015). If DNA-based biomonitoring is to be an effective, reliable tool for assessing biodiversity on a global scale, efforts need to be primarily concentrated toward better curation and updating of DNA reference records, as well as continued barcoding of taxonomically identified specimens to improve the quality and quantity of information in DNA databases (Hajibabaei et al., 2016; Elbrecht et al., 2017; Stat et al., 2017; Zinger et al., 2019).
In essence, what will dominate the database in terms of sequence data for various biota will be based on what has been collected from the temperate areas more so than the tropics and polar regions. Thus, for example, how will soil scientists (and others) be able to effectively identify organisms in their soil samples based on databases from other regions? More importantly, some of these areas that need to be further sampled are those that are experiencing drastic intensities of climate pattern changes. This also describes the need for more seasonal studies over periods of time to assess the variability in climate patterns across the globe. If we are to detect ecosystem change globally, more comprehensive work involving biomonitoring and DNA metabarcoding/eDNA will be needed to generate consensus data, generate the metadata, and start analyzing trends across the globe.
With advancing technologies and methodologies such as implementing machine learning and neural networks pertaining to ecological status and modeling, as has been described elsewhere (Díaz et al., 2015; Bohan et al., 2017; Derocles et al., 2018), we still need to increase the information in a database to identify particular organisms of interest and from more geographical locations across the globe, for biomonitoring, and more robust experimental designs rather than straight survey-based approaches to draw sound ecological conclusions (Zinger et al., 2019). Yet, if sample types are inherently variable due to geographical location and/or sample type across the globe, how can we ever expect these taxonomic databases to accurately reflect a global perspective of ecosystem, in order to effectively and accurately detect global ecosystem change. By collecting samples from more geographical locations where the representation is lacking, collecting a wider array of sample types, and constructing the replicated ecological networks of ecological interactions, together, will provide useful standards of global ecosystem information, dramatically enhancing the ability to assess the taxa within global ecosystems, and understanding how these respond to climate change and other forms of ecosystem damage. We propose that combining the use of these technologies would greatly enhance the capacity to better predict how various ecosystems respond to environmental change at local, regional and global levels.
Author Contributions
MH, KM, and CR conceived the idea and co-wrote the manuscript. CR conducted the literature search.
Funding
Funding was provided from Government of Canada through Canada First Excellence Research project, Food from Thought and Genome Canada & Ontario Genomics Institute project STREAM.
Conflict of Interest Statement
The authors declare that the research was conducted in the absence of any commercial or financial relationships that could be construed as a potential conflict of interest.
References
Adamowicz, S. J., Boatwright, J. S., Chain, F., Fisher, B. L., Hogg, I. D., Leese, F., et al. (2019). Trends in DNA barcoding and metabarcoding. Genome 62, v–viii. doi: 10.1139/gen-2019-0054
Alberdi, A., Aizpurua, O., Gilbert, M. T. P., and Bohmann, K. (2018). Scrutinizing key steps for reliable metabarcoding of environmental samples. Methods Ecol. Evol. 9, 134–147. doi: 10.1111/2041-210X.12849
Ammon, U., von Wood, S. A., Laroche, O., Zaiko, A., Tait, L., Lavery, S., et al. (2018). Combining morpho-taxonomy and metabarcoding enhances the detection of non-indigenous marine pests in biofouling communities. Sci. Rep. 8:16290. doi: 10.1038/s41598-018-34541-1
Andersen, K., Bird, K. L., Rasmussen, M., Haile, J., Breuning-Madsen, H., Kjær, K. H., et al. (2012). Meta-barcoding of ‘dirt’ DNA from soil reflects vertebrate biodiversity. Mol. Ecol. 21, 1966–1979. doi: 10.1111/j.1365-294X.2011.05261.x
Aylagas, E., Borja, Á., Muxika, I., and Rodríguez-Ezpeleta, N. (2018). Adapting metabarcoding-based benthic biomonitoring into routine marine ecological status assessment networks. Ecol. Indic. 95, 194–202. doi: 10.1016/j.ecolind.2018.07.044
Aylagas, E., Mendibil, I., Borja, Á., and Rodríguez-Ezpeleta, N. (2016). Marine sediment sample pre-processing for macroinvertebrates metabarcoding: mechanical enrichment and homogenization. Front. Mar. Sci. 3, 1–12. doi: 10.3389/fmars.2016.00203
Baird, D. J., and Hajibabaei, M. (2012). Biomonitoring 2.0: a new paradigm in ecosystem assessment made possible by next-generation DNA sequencing. Mol. Ecol. 21, 2039–2044. doi: 10.1111/j.1365-294X.2012.05519.x
Beisner, B. E., Haydon, D. T., and Cuddington, K. (2003). Alternative stable states in ecology. Front. Ecol. Environ. 1, 376–382. doi: 10.1890/1540-9295(2003)001[0376:ASSIE]2.0.CO;2
Bellemain, E., Davey, M. L., Kauserud, H., Epp, L. S., Boessenkool, S., Coissac, E., et al. (2013). Fungal palaeodiversity revealed using high-throughput metabarcoding of ancient DNA from arctic permafrost. Environ. Microbiol. 15, 1176–1189. doi: 10.1111/1462-2920.12020
Bleidorn, C. (2016). Third generation sequencing: technology and its potential impact on evolutionary biodiversity research. System. Biodivers. 14, 1–8. doi: 10.1080/14772000.2015.1099575
Bleidorn, C. (2017). “Sequencing Techniques,” in Phylogenomics: An Introduction, ed C. Bleidorn (Cham: Springer International Publishing), 43–60. doi: 10.1007/978-3-319-54064-1_3
Bohan, D. A., Vacher, C., Tamaddoni-Nezhad, A., Raybould, A., Dumbrell, A. J., and Woodward, G. (2017). Next-generation global biomonitoring: large-scale, automated reconstruction of ecological networks. Trends Ecol. Evol. 32, 477–487. doi: 10.1016/j.tree.2017.03.001
Carpenter, S. R., Cole, J. J., Hodgson, J. R., Kitchell, J. F., Pace, M. L., Bade, D., et al. (2001). Trophic cascades, nutrients, and lake productivity: whole-lake experiments. Ecol. Monogr. 71, 163–186. doi: 10.1890/0012-9615(2001)071[0163:TCNALP]2.0.CO;2
Cordier, T., Lanzén, A., Apothéloz-Perret-Gentil, L., Stoeck, T., and Pawlowski, J. (2019). Embracing environmental genomics and machine learning for routine biomonitoring. Trends Microbiol. 27, 387–397. doi: 10.1016/j.tim.2018.10.012
Creer, S., Fonseca, V. G., Porazinska, D. L., Giblin-Davis, R. M., Sung, W., Power, D. M., et al. (2010). Ultrasequencing of the meiofaunal biosphere: practice, pitfalls and promises. Mol. Ecol. 19, 4–20. doi: 10.1111/j.1365-294X.2009.04473.x
Deiner, K., Bik, H. M., Mächler, E., Seymour, M., Lacoursière-Roussel, A., Altermatt, F., et al. (2017). Environmental DNA metabarcoding: transforming how we survey animal and plant communities. Mol. Ecol. 26, 5872–5895. doi: 10.1111/mec.14350
Derocles, S. A. P., Bohan, D. A., Dumbrell, A. J., Kitson, J. J. N., Massol, F., Pauvert, C., et al. (2018). “Chapter one - biomonitoring for the 21st century: integrating next-generation sequencing into ecological network analysis,” in Advances in Ecological Research Next Generation Biomonitoring: Part 1., eds D. A. Bohan, A. J. Dumbrell, G. Woodward, and M. Jackson (Cambridge, MA: Academic Press), 1–62. doi: 10.1016/bs.aecr.2017.12.001
Díaz, S., Demissew, S., Carabias, J., Joly, C., Lonsdale, M., Ash, N., et al. (2015). The IPBES Conceptual Framework — connecting nature and people. Curr. Opin. Environ. Sust. 14, 1–16. doi: 10.1016/j.cosust.2014.11.002
Dickie, I. A., Boyer, S., Buckley, H. L., Duncan, R. P., Gardner, P. P., Hogg, I. D., et al. (2018). Towards robust and repeatable sampling methods in eDNA-based studies. Mol. Ecol. Resour. 18, 940–952. doi: 10.1111/1755-0998.12907
Divoll, T. J., Brown, V. A., Kinne, J., McCracken, G. F., and O'Keefe, J. M. (2018). Disparities in second-generation DNA metabarcoding results exposed with accessible and repeatable workflows. Mol. Ecol. Resour. 18, 590–601. doi: 10.1111/1755-0998.12770
Dopheide, A., Xie, D., Buckley, T. R., Drummond, A. J., and Newcomb, R. D. (2019). Impacts of DNA extraction and PCR on DNA metabarcoding estimates of soil biodiversity. Methods Ecol. Evol. 10, 120–133. doi: 10.1111/2041-210X.13086
Dowle, E. J., Pochon, X., Banks, J. C., Shearer, K., and Wood, S. A. (2016). Targeted gene enrichment and high-throughput sequencing for environmental biomonitoring: a case study using freshwater macroinvertebrates. Mol. Ecol. Resour. 16, 1240–1254. doi: 10.1111/1755-0998.12488
Dumbrell, A. J. (2019). Size matters in regulating the biodiversity of tropical forest soils. Mol. Ecol. 28, 525–527. doi: 10.1111/mec.14996
Elbrecht, V., and Steinke, D. (2019). Scaling up DNA metabarcoding for freshwater macrozoobenthos monitoring. Freshw. Biol. 64, 380–387. doi: 10.7287/peerj.preprints.3456v4
Elbrecht, V., Vamos, E. E., Meissner, K., Aroviita, J., and Leese, F. (2017). Assessing strengths and weaknesses of DNA metabarcoding-based macroinvertebrate identification for routine stream monitoring. Methods Ecol. Evol. 8, 1265–1275. doi: 10.1111/2041-210X.12789
Evans, D. M., Kitson, J. J. N., Lunt, D. H., Straw, N. A., and Pocock, M. J. O. (2016). Merging DNA metabarcoding and ecological network analysis to understand and build resilient terrestrial ecosystems. Funct. Ecol. 30, 1904–1916. doi: 10.1111/1365-2435.12659
Fahner, N. A., McCarthy, A., Barnes, J. G., Singer, G., and Hajibabaei, M. (2018). Experimental design considerations for assessing marine biodiversity using environmental DNA 6:e26814v1. doi: 10.7287/peerj.preprints.26814v1
Fahner, N. A., Shokralla, S., Baird, D. J., and Hajibabaei, M. (2016). Large-scale monitoring of plants through environmental DNA metabarcoding of soil: recovery, resolution, and annotation of four DNA markers. PLoS ONE 11:e0157505. doi: 10.1371/journal.pone.0157505
Ferguson, R. M. W., Garcia-Alcega, S., Coulon, F., Dumbrell, A. J., Whitby, C., and Colbeck, I. (2019). Bioaerosol biomonitoring: sampling optimization for molecular microbial ecology. Mol. Ecol. Resour. 19, 672–690. doi: 10.1111/1755-0998.13002
Filker, S., Sommaruga, R., Vila, I., and Stoeck, T. (2016). Microbial eukaryote plankton communities of high-mountain lakes from three continents exhibit strong biogeographic patterns. Mol. Ecol. 25, 2286–2301. doi: 10.1111/mec.13633
Hajibabaei, M., Baird Donald, J., Fahner Nicole, A., Beiko, R., and Brain Golding, G. (2016). A new way to contemplate Darwin's tangled bank: how DNA barcodes are reconnecting biodiversity science and biomonitoring. Philos. Trans. R. Soc. B Biol. Sci. 371:20150330. doi: 10.1098/rstb.2015.0330
Hajibabaei, M., Porter, T. M., Robinson, C. V., Baird, D. J., Shokralla, S., and Wright, M. (2019). Watered-down biodiversity? A comparison of metabarcoding results from DNA extracted from matched water and bulk tissue biomonitoring samples. Sci. Rep. bioRxiv [preprint]. doi: 10.1101/575928
Hajibabaei, M., Shokralla, S., Zhou, X., Singer, G. A. C., and Baird, D. J. (2011). Environmental barcoding: a next-generation sequencing approach for biomonitoring applications using river benthos. PLoS ONE 6:e17497. doi: 10.1371/journal.pone.0017497
Hansen, B. K., Bekkevold, D., Clausen, L. W., and Nielsen, E. E. (2018). The sceptical optimist: challenges and perspectives for the application of environmental DNA in marine fisheries. Fish Fisheries 19, 751–768. doi: 10.1111/faf.12286
Harper, L. R., Buxton, A. S., Rees, H. C., Bruce, K., Brys, R., Halfmaerten, D., et al. (2019). Prospects and challenges of environmental DNA (eDNA) monitoring in freshwater ponds. Hydrobiologia 826, 25–41. doi: 10.1007/s10750-018-3750-5
Hatzenbuhler, C., Kelly, J. R., Martinson, J., Okum, S., and Pilgrim, E. (2017). Sensitivity and accuracy of high-throughput metabarcoding methods for early detection of invasive fish species. Sci. Rep. 7:46393. doi: 10.1038/srep46393
Kraaijeveld, K., Weger, L. A., de, García, M. V., Buermans, H., Frank, J., Hiemstra, P. S., et al. (2015). Efficient and sensitive identification and quantification of airborne pollen using next-generation DNA sequencing. Mol. Ecol. Resour. 15, 8–16. doi: 10.1111/1755-0998.12288
Laroche, O., Pochon, X., Tremblay, L. A., Ellis, J. I., Lear, G., and Wood, S. A. (2018). Incorporating molecular-based functional and co-occurrence network properties into benthic marine impact assessments. FEMS Microbiol. Ecol. 94:fiy167. doi: 10.1093/femsec/fiy167
Lobo, J., Shokralla, S., Costa, M. H., Hajibabaei, M., and Costa, F. O. (2017). DNA metabarcoding for high-throughput monitoring of estuarine macrobenthic communities. Sci. Rep. 7:15618. doi: 10.1038/s41598-017-15823-6
Macher, J.-N., Vivancos, A., Piggott, J. J., Centeno, F. C., Matthaei, C. D., and Leese, F. (2018). Comparison of environmental DNA and bulk-sample metabarcoding using highly degenerate cytochrome c oxidase I primers. Mol. Ecol. Resour. 18, 1456–1468. doi: 10.1111/1755-0998.12940
McGee, K. M., Eaton, W. D., Shokralla, S., and Hajibabaei, M. (2019). Determinants of soil bacterial and fungal community composition toward carbon-use efficiency across primary and secondary forests in a costa rican conservation area. Microb. Ecol. 77, 148–167. doi: 10.1007/s00248-018-1206-0
Morinière, J., Araujo, B. C., de Lam, A. W., Hausmann, A., Balke, M., Schmidt, S., et al. (2016). Species identification in malaise trap samples by DNA barcoding based on NGS technologies and a scoring matrix. PLoS ONE 11:e0155497. doi: 10.1371/journal.pone.0155497
Muha, T. P., Rodríguez-Rey, M., Rolla, M., and Tricarico, E. (2017). Using environmental DNA to improve species distribution models for freshwater invaders. Front. Ecol. Evol. 5, 1–7. doi: 10.3389/fevo.2017.00158
Pavan-Kumar, P., Gireesh-Babu, A., and Lakra, W. (2015). DNA Metabarcoding: a new approach forrapid biodiversity assessment. J. Cell Sci. Mol. Biol. 2:111.
Porter, T. M., and Hajibabaei, M. (2018a). Over 2.5 million COI sequences in GenBank and growing. PLoS ONE 13:e0200177. doi: 10.1371/journal.pone.0200177
Porter, T. M., and Hajibabaei, M. (2018b). Scaling up: a guide to high-throughput genomic approaches for biodiversity analysis. Mol. Ecol. 27, 313–338. doi: 10.1111/mec.14478
Quail, M. A., Smith, M., Coupland, P., Otto, T. D., Harris, S. R., Connor, T. R., et al. (2012). A tale of three next generation sequencing platforms: comparison of Ion Torrent, Pacific Biosciences and Illumina MiSeq sequencers. BMC Genomics 13:341. doi: 10.1186/1471-2164-13-341
Ratnasingham, S., and Hebert, P. D. N. (2013). A DNA-based registry for all animal species: the barcode index number (BIN) system. PLoS ONE 8:e66213. doi: 10.1371/journal.pone.0066213
Robinson, C. V., Leaniz, C. G., and de Consuegra, S. (2019). Effect of artificial barriers on the distribution of the invasive signal crayfish and Chinese mitten crab. Sci. Rep. 9:7230. doi: 10.1038/s41598-019-43570-3
Ruppert, K. M., Kline, R. J., and Rahman, M. S. (2019). Past, present, and future perspectives of environmental DNA (eDNA) metabarcoding: a systematic review in methods, monitoring, and applications of global eDNA. Global Ecol. Conser. 17:e00547. doi: 10.1016/j.gecco.2019.e00547
Salonen, I. S., Chronopoulou, P.-M., Leskinen, E., and Koho, K. A. (2019). Metabarcoding successfully tracks temporal changes in eukaryotic communities in coastal sediments. FEMS Microbiol. Ecol. 95:fiy226. doi: 10.1093/femsec/fiy226
Scheffer, M., Carpenter, S., Foley, J. A., Folke, C., and Walker, B. (2001). Catastrophic shifts in ecosystems. Nature 413, 591–596. doi: 10.1038/35098000
Schluter, D., and Pennell, M. W. (2017). Speciation gradients and the distribution of biodiversity. Nature 546, 48–55. doi: 10.1038/nature22897
Singer, G. A. C., Fahner, N., Barnes, J., McCarthy, A., and Hajibabaei, M. (2019). Comprehensive biodiversity analysis via ultra-deep patterned flow cell technology: a case study of eDNA metabarcoding seawater. 9:5991. doi: 10.1038/s41598-019-42455-9
Stat, M., Huggett, M. J., Bernasconi, R., DiBattista, J. D., Berry, T. E., Newman, S. J., et al. (2017). Ecosystem biomonitoring with eDNA: metabarcoding across the tree of life in a tropical marine environment. Sci. Rep. 7:12240. doi: 10.1038/s41598-017-12501-5
Taberlet, P., Bonin, A., Zinger, L., and Coissac, E. (2018). Environmental DNA: For Biodiversity Research and Monitoring. New York, NY: Oxford University Press. doi: 10.1093/oso/9780198767220.001.0001
Thomsen, P. F., and Willerslev, E. (2015). Environmental DNA – An emerging tool in conservation for monitoring past and present biodiversity. Biol. Conserv. 183, 4–18. doi: 10.1016/j.biocon.2014.11.019
Turner, C. R., Uy, K. L., and Everhart, R. C. (2015). Fish environmental DNA is more concentrated in aquatic sediments than surface water. Biol. Conserv. 183, 93–102. doi: 10.1016/j.biocon.2014.11.017
Vacher, C., Tamaddoni-Nezhad, A., Kamenova, S., Peyrard, N., Moalic, Y., Sabbadin, R., et al. (2016). “Chapter 1 - learning ecological networks from next-generation sequencing data,” in Advances in Ecological Research Ecosystem Services: From Biodiversity to Society, Part 2., eds G. Woodward and D. A. Bohan (Cambridge, MA: Academic Press), 1–39. doi: 10.1016/bs.aecr.2015.10.004
Valentini, A., Taberlet, P., Miaud, C., Civade, R., Herder, J., Thomsen, P. F., et al. (2016). Next-generation monitoring of aquatic biodiversity using environmental DNA metabarcoding. Mol. Ecol. 25, 929–942. doi: 10.1111/mec.13428
von Bubnoff, A. (2008). Next-generation sequencing: the race is on. Cell 132, 721–723. doi: 10.1016/j.cell.2008.02.028
Xie, Y., Zhang, X., Yang, J., Kim, S., Hong, S., Giesy, J. P., et al. (2018). eDNA-based bioassessment of coastal sediments impacted by an oil spill. Environ. Pollut. 238, 739–748. doi: 10.1016/j.envpol.2018.02.081
Yoccoz, N. G., Bråthen, K. A., Gielly, L., Haile, J., Edwards, M. E., Goslar, T., et al. (2012). DNA from soil mirrors plant taxonomic and growth form diversity. Mol. Ecol. 21, 3647–3655. doi: 10.1111/j.1365-294X.2012.05545.x
Zaiko, A., Samuiloviene, A., Ardura, A., and Garcia-Vazquez, E. (2015). Metabarcoding approach for non-indigenous species surveillance in marine coastal waters. Mar. Pollut. Bull. 100, 53–59. doi: 10.1016/j.marpolbul.2015.09.030
Zielińska, S., Kidawa, D., Stempniewicz, L., Łoś, M., and Łoś, J. M. (2017). Environmental DNA as a valuable and unique source of information about ecological networks in Arctic terrestrial ecosystems. Environ. Rev. 25, 282–291. doi: 10.1139/er-2016-0060
Zimmermann, H. H., Raschke, E., Epp, L. S., Stoof-Leichsenring, K. R., Schwamborn, G., Schirrmeister, L., et al. (2017). Sedimentary ancient DNA and pollen reveal the composition of plant organic matter in Late Quaternary permafrost sediments of the Buor Khaya Peninsula (North-Eastern Siberia). Biogeosciences 14, 575–596. doi: 10.5194/bg-14-575-2017
Keywords: biomonitoring, DNA metabarcoding, next-generation sequencing, biodiversity, global, ecosystem, biomes
Citation: McGee KM, Robinson CV and Hajibabaei M (2019) Gaps in DNA-Based Biomonitoring Across the Globe. Front. Ecol. Evol. 7:337. doi: 10.3389/fevo.2019.00337
Received: 04 June 2019; Accepted: 23 August 2019;
Published: 06 September 2019.
Edited by:
David Andrew Bohan, INRA Centre Dijon Bourgogne Franche-Comté, FranceReviewed by:
Florian Leese, University of Duisburg-Essen, GermanyXavier Pochon, Cawthron Institute, New Zealand
Tristan Cordier, Université de Genève, Switzerland
Copyright © 2019 McGee, Robinson and Hajibabaei. This is an open-access article distributed under the terms of the Creative Commons Attribution License (CC BY). The use, distribution or reproduction in other forums is permitted, provided the original author(s) and the copyright owner(s) are credited and that the original publication in this journal is cited, in accordance with accepted academic practice. No use, distribution or reproduction is permitted which does not comply with these terms.
*Correspondence: Mehrdad Hajibabaei, aGFqaWJhYmFlaSYjeDAwMDQwO2dtYWlsLmNvbQ==