- 1Department of Biology and Wildlife, University of Alaska Fairbanks, Fairbanks, AK, United States
- 2Department of Mathematics and Statistics, University of Alaska Fairbanks, Fairbanks, AK, United States
- 3Department of Statistics, University of British Columbia, Vancouver, BC, Canada
- 4Institute for the Oceans and Fisheries, University of British Columbia, Vancouver, BC, Canada
- 5Alaska Department of Fish and Game, Fairbanks, AK, United States
- 6United States Fish and Wildlife Service, Juneau, AK, United States
- 7Institute of Arctic Biology, University of Alaska Fairbanks, Fairbanks, AK, United States
Long distance migration can increase lifetime fitness, but can be costly, incurring increased energetic expenses and higher mortality risks. Stopover and other en route behaviors allow animals to rest and replenish energy stores and avoid or mitigate other hazards during migration. Some animals, such as soaring birds, can subsidize the energetic costs of migration by extracting energy from flowing air. However, it is unclear how these energy sources affect or interact with behavioral processes and stopover in long-distance soaring migrants. To understand these behaviors and the effects of processes that might enhance use of flight subsidies, we developed a flexible mechanistic model to predict how flight subsidies drive migrant behavior and movement processes. The novel modeling framework incorporated time-varying parameters informed by environmental covariates to characterize a continuous range of behaviors during migration. This model framework was fit to GPS satellite telemetry data collected from a large soaring and opportunist foraging bird, the golden eagle (Aquila chrysaetos), during migration in western North America. Fitted dynamic model parameters revealed a clear circadian rhythm in eagle movement and behavior, which was directly related to thermal uplift. Behavioral budgets were complex, however, with evidence for a joint migrating/foraging behavior, resembling a slower paced fly-and-forage migration, which could facilitate efficient refueling while still ensuring migration progress. In previous work, ecological and foraging conditions are usually considered to be the key aspects of stopover location quality, but taxa, such as the golden eagle, that can tap energy sources from moving fluids to drive migratory locomotion may pace migration based on both foraging opportunities and available flight subsidies.
Introduction
Long-distance migration can relax competition and permit use of seasonally available resources, helping many animals maximize lifetime fitness (Newton, 2008; Avgar et al., 2014). Those benefits, however, come at substantial costs, including greater vulnerability to predators, uncertain conditions, mechanical wear, elevated energy expenditure, and time (Alerstam and Hedenström, 1998; Clark and Butler, 1999; Hedenström, 2008; Newton, 2008; Avgar et al., 2014). As many migrant species cannot store sufficient energy for nonstop, long-distance migration, stopover evolved as a behavior for strategically resting and refueling en route (Gill, 2007).
Migrant species are adapted for utilizing either soaring or flapping flight, and the different flight modes translate into stopover strategy (Hedenström, 1993; Gill, 2007). Generally, soaring flight is favorable for larger birds and flapping flight for smaller birds, though the partitioning of time for each flight mode during migration is dependent on the tradeoff between time and energy (Hedenström, 1993; Duerr et al., 2015; Katzner et al., 2015; Miller et al., 2016). In theory, a time-minimizing migrator would be expected to fly with greater directional persistence and stronger directional bias than would an energy-expenditure minimizer. Such net energy maximizers would be expected to take advantage of en route foraging opportunities and may divert or delay to replenish energy reserves. (Note that “energy minimization” has been used to describe this strategy e.g., Alerstam, 2011; Miller et al., 2016, but we use “net energy maximization” for clarity.) If time is less important, a net energy maximizer is less restricted and can spend additional time seeking an energetically superior path; the emergent path would then be more tortuous with less directional bias toward the final destination at any given point along the route. Time minimization and net energy maximization strategies are not mutually exclusive, however, and the emergent strategy and behaviors in any given migrating individual lies along a continuum (Alerstam, 2011; Miller et al., 2016).
Obligate soaring migrants must also consider routes based on their energy landscape (Shamoun-Baranes et al., 2010), the energetic constraints of movement over space (Shepard et al., 2013), which also contributes to a migrant's location along the behavioral continuum. While soaring migrants can stopover, their energy landscape is more complex. Meteorological conditions are at least as important as foraging resources for soaring migrants, which can be extremely dynamic and subsidize the energetic cost of flight directly via uplift (Pennycuick, 1971; Alerstam, 1979; Spaar and Bruderer, 1997; Gill, 2007; Duerr et al., 2012; Murgatroyd et al., 2018).
The flight performance of soaring migrants relative to subsidies provided by meteorological conditions has been well documented (Pennycuick, 1971; Alerstam, 1979; Spaar and Bruderer, 1997), establishing a clear link between diurnal migrant behavior and development of the atmospheric boundary layer. Two primary forms of uplift arise by (1) wind interacting with topography to form upslope wind or mountain waves (air currents forming standing waves established on the lee side of mountains; hereafter orographic uplift) and (2) solar heating of the earth's surface to generate thermal uplift. Other forms arise from turbulent eddies over small landscape features and ocean waves modifying the air. The dynamic nature of atmospherically-driven flight subsidies requires detailed movement data as well as carefully designed analytical techniques to investigate certain mechanisms hidden in those data.
Our understanding of migratory processes has advanced enormously in the past 30 years, as animal tracking technology developed from a novelty of coarse observation to a core method for observing animal behavior and movement in incredible detail (Luschi et al., 1998; Sawyer et al., 2005; Bridge et al., 2011; Katzner et al., 2015; Hooten et al., 2017). Global Positioning System (GPS) telemetry, in particular, allows remote observation of animal relocations across a broad spatiotemporal scale. GPS transmitters are now light and reliable enough to study the complete migrations of many large soaring migrants, including golden eagles Aquila chrysaetos, which often rely on flight subsidies during migration (Katzner et al., 2015). Golden eagles and other large soaring birds have been used as model systems for phenomenologically evaluating questions about migratory flight performance and migration strategies (sensu Duerr et al., 2012; Lanzone et al., 2012; Katzner et al., 2015; Vansteelant et al., 2015; Miller et al., 2016; Shamoun-Baranes et al., 2016; Rus et al., 2017). For example, Lanzone et al. (2012) and Katzner et al. (2015) found that golden eagles use both thermal and orographic uplift to subsidize migratory flight, although thermal soaring was often more efficient in long distance, directed flight (Duerr et al., 2012). While these studies have contributed to our understanding of soaring migration and have laid a foundation for more detailed approaches, they relate meteorology to derived movement metrics, rather than incorporate them into process-based models that mechanistically predict movement, and ignore the temporal dependence between serially observed locations (i.e., autocorrelation). Not accounting for such autocorrelation imparts bias on certain estimated parameters (e.g., variances) thereby affecting inference through, for example, underestimating uncertainty. Consequently, the links between resources distributed over the landscape, such as flight subsidies, and behavioral budgets, including stopover behavior, during migrations of soaring birds remain unclear.
Unlike previous approaches, process-based, mechanistic movement models allow explicit inference of the underlying mechanisms driving movement (e.g., changes in behavior) that may not be available from conventional phenomenological analytical approaches (Turchin, 1998; Nathan et al., 2008; Hooten et al., 2017). While it is impossible to understand fully the intricacies in animal movement, we can pose mathematical models (e.g., correlated random walks) to approximate the movement process (Kareiva and Shigesada, 1983; Turchin, 1998). We can then fit these models statistically to observed data to estimate parameters describing behavior and its relationship with dynamic environmental features that moving animals experience (Blackwell, 1997, 2003; Morales et al., 2004; Breed et al., 2017; Hooten et al., 2017). Many of the recently developed mechanistic movement models are built in a discrete state-switching framework, where animals switch between discrete behavioral states (see Hooten et al., 2017, and references cited therein). Choosing both the biologically relevant and quantitatively supported number of states, as well as interpreting the identified states in a biological context, remains challenging (Patterson et al., 2017; Pohle et al., 2017). Often, this challenge leads researchers to artificially limit the number of states and/or collapse two or more states into one biologically interpretable state. For example Pirotta et al. (2018), presented a model with five discrete kinds of avian flight, but the complexity of the model made interpreting those states difficult and poorly matched classifications manually identified by an expert.
In many cases, a more natural approach to modeling an animal's movement process is along a dynamic continuum, rather than as switching between discrete behavioral states (Breed et al., 2012; Auger-Méthé et al., 2017; Jonsen et al., 2019). Modeling along a continuum may be an especially useful approach for understanding movement behavior in soaring birds, considering the dynamic nature of atmospheric processes that influence movements. Here, we developed and applied a flexible mechanistic movement model based on a correlated random walk with time-varying parameters. This novel model was fit to movement data collected via GPS telemetry to understand how individuals in a population of long-distance soaring migrants use flight subsidies and budget stopover and migration behavior. Specifically, we were interested in identifying which flight subsidies influence stopover and migratory behavior and how the effect of key subsides and behaviors varied between spring and fall migrations. Our approach resembled continuous-time correlated random walks (Johnson et al., 2008; Blackwell et al., 2015; Gurarie et al., 2017; Michelot and Blackwell, 2019), but was easily implemented and yielded a relatively small number of dynamic parameters that could be directly interpreted biologically. A set of candidate models could be ranked, with model selection approaches, providing inference on how behavioral budgets and meteorological variables interacted to give rise to the observed migration paths. Modeling the effects of dynamic wind and uplift variables as time-varying movement behaviors of migratory golden eagles further allowed new details to emerge without imposing artificially discrete states.
Methods
Model System
The golden eagle is a large, soaring raptor, distributed across the Holarctic (Watson, 2010). Golden eagles are predatory and opportunistic, utilizing many taxa for food resources, ranging from small mammals and birds to ungulates, often scavenging carrion (Kochert et al., 2002; Watson, 2010). While many populations are classified as partial migrants, most individuals that summer and breed above approximately 55°N in North America are considered true long-distance migrants (Kochert et al., 2002; Watson, 2010). The population we observed in this study migrates over the mountainous regions of western North America between a breeding range primarily in southcentral Alaska, USA and a broad overwintering range in western North America that ranges from the southwestern US to central British Columbia and Alberta, Canada (Bedrosian et al., 2018).
Data Collection
We captured golden eagles with a remote-fired net launcher, placed over carrion bait near Gunsight Mountain, Alaska (61.67°N 147.35°W). Captures occurred between mid-March and mid-April 2014-2016. Fifty-three adult and sub-adult eagles were equipped with 45-g back pack solar-powered Argos/GPS platform transmitter terminals (PTTs; Microwave Telemetry, Inc., Columbia, MD, USA). Eagles were sexed molecularly and aged by plumage.
PTTs were programmed to record GPS locations on duty cycles, ranging from 8 to 14 fixes per day during migration, depending on year of deployment. PTTs deployed in 2014 were set to record 13 locations at 1-h intervals centered around solar noon plus a location at midnight local time. PTTs deployed in 2015 were programmed to record 8 locations with 1-h intervals centered around solar noon, and PTTs deployed in 2016 took eight fixes daily at regular 3-h time intervals. Note that the PTTs deployed in 2015 did not record locations overnight. Poor battery voltage from September to March often resulted in PTTs failing to take all programmed fixes, so the resulting GPS tracks had missing observations during these periods. Tags lasted multiple seasons, and in fact many are still deployed and transmitting at this writing. We chose to limit this analysis to the migrations that occurred in 2016. The spring and fall migratory pathways of the 2016 migration from 26 tags were available and suitable for analysis in that year: 11 deployed in 2014, 7 deployed 2015, and 8 deployed 2016. Tracks were suitable for analysis based on having few missing data, with no more than a few days of consecutive missing locations.
Movement data were managed in the online repository Movebank (https://www.movebank.org/), and we used the Track Annotation Service (Dodge et al., 2013) to extract flight subsidy (wind and uplift) data, specific to each PTT location and time of recording that location, along eagle tracks. The Track Annotation Service derives uplift variables from elevation models and weather and atmospheric reanalyses (Bohrer et al., 2012). We followed the Movebank recommendations for interpolation methods; details are below.
Movement Model
We developed a correlated random walk (CRW) movement model to reveal how changes in behavior give rise to the movement paths of migrating eagles. We chose to use a dynamic, time-varying correlation parameter, which represents behavior as a continuum rather than discrete categories, to capture complex behavioral patterns that could occur on multiple temporal and spatial scales (Breed et al., 2012; Auger-Méthé et al., 2017; Jonsen et al., 2019). We believe this approach can offer substantial flexibility, as a continuous range of behaviors is more realistic and, as we show, more naturally allows modeling behavior as a function of covariates.
The basic form of the model was a first-difference CRW presented by Auger-Méthé et al. (2017), which can take the form:
where
Here, Δti = ti − ti−1 represents the time interval between Cartesian coordinate vectors xi and xi−1 for the observed locations of the animal at times ti and ti−1. Incorporating autocorrelation in behavior, γi constitutes a random walk, such that
γi correlates displacements (or “steps”) and can be interpreted to understand the type of movement, and thus behavior, of migrating individuals: estimates of γi closer to one indicate directionally-persistent, larger-scale migratory movement, while estimates of γi closer to zero indicate more-tortuous, smaller-scale stopover movement (Breed et al., 2012; Auger-Méthé et al., 2017). Scaling γi by and the variance components by allows us to accommodate unequal time intervals (Auger-Méthé et al., 2017), which can arise from a PTT's pre-programmed duty cycles and/or missed location attempts. This assumes that over longer time intervals an animal is likely to move greater distances and that the previous step will have less influence on the current step. Notably, in introducing Δti, this CRW essentially becomes a correlated velocity model presented in terms of displacement vectors (xi−1 − xi−2) (Johnson et al., 2008; Blackwell et al., 2015; Gurarie et al., 2017), most closely resembling the autocorrelated velocity model presented by Gurarie et al. (2017). Because location error of GPS data is negligible compared to the movement of most large vertebrates (Hooten et al., 2017), we did not incorporate an observation equation to handle location error. While a covariance parameter could be added to the model, we chose to fix covariance to zero (equation 2), which assumes that movement in the x and y dimensions are independent. This assumption has been suggested to be potentially problematic (Dunn and Gipson, 1977; Blackwell, 1997); however, it is common and has been shown to draw reasonable inference from real data, as well as recover known parameters from simulated data (Breed et al., 2012, 2017; Auger-Méthé et al., 2017; Jonsen et al., 2019). To support this, we compared results from the model assuming zero covariance to one fit assuming equal variance in x and y—like estimating covariance, this ensures invariance under linear transformation of the coordinate system—to illustrate that inference remains unaffected by this assumption (Appendix 1).
Extending this CRW to introduce environmental covariates, we first made the assumption that an individual's behavior can be adequately explained by the previous behavior plus some effects of environmental conditions and random noise. This modeling approach and philosophy aligns with the movement ecology paradigm presented by Nathan et al. (2008): An animal's movement path is influenced by its internal state and the environmental conditions it experiences. We modified the behavioral (or internal state) process—previously described above as a pure random walk in one dimension (Equation 3)—similar to a linear model with a logit link function. The logit link constrains γi ∈ [0, 1] and allowed us to model it as a linear combination of continuously-distributed random variables (Jonsen et al., 2019). These variables were different meteorological conditions affecting flight subsidies. Now,
where
and is the row vector of environmental covariates associated with xi. Each element of the vector β is an estimated parameter representing the magnitude and direction of the effect of its respective covariate on the correlation parameter γi in addition to the effect of γi−1. Note that including γi−1 here preserves explicit serial correlation in the behavioral process so that any additional environmental effect is not overestimated. is only used to estimate γi; any behavioral interpretations are made in terms of γi.
Model Fitting
Subsetting Tracks
Of the 26 eagles producing suitable data in 2016, we fit the model to 15 spring and 16 fall adult golden eagle migration tracks recorded by 18 adult males and 8 adult females in 2016. This included both spring and fall migrations for five individuals. In reporting the results, we assumed any individual random effects of including both migrations for these few individuals to be negligible, which seems reasonable given fitted parameters presented in Table S1 in Appendix. The model was fit only to the migratory periods, plus two fixes prior to departure to ensure valid parameter estimates at the onset of migration. Data were constrained to migratory periods under the following rules: The first migration step was identified as the first directed movement away from what was judged to be an individual's summer (or winter) range with no subsequent return to that range, and the final migration step was defined as the step terminating in the apparent winter (or summer) range. This assignment was usually straightforward; however, in some cases there were apparent pre-migration staging areas. These were not considered part of migration and excluded from the analysis here; movement data from these individuals collected during the breeding and overwintering periods are neither presented nor analyzed here.
Environmental Covariates
Golden eagles can switch between using thermal and orographic uplift as flight subsidies (Lanzone et al., 2012; Katzner et al., 2015), so we included both variables as covariates affecting the correlation parameter in the behavioral process of the CRW (equation 5). Thermal uplift ztu and orographic uplift zou are measured in m/s with ztu, zou ∈ [0, ∞). Thermal uplift was bilinearly interpolated from European Center for Medium-Range Weather Forecasts (ECMWF) reanalyses, and orographic uplift from the nearest neighbor (grid cell) by pairing National Center for Environmental Predictions (NCEP) North American Regional Reanalysis (NARR) data with the Advanced Spaceborne Thermal Emission Reflection Radiometer (ASTER) Global Digital Elevation Model (GDEM; Brandes and Ombalski, 2004; Bohrer et al., 2012). We also introduced wind as a covariate in the behavioral process, as it can influence eagle flight as well as the flight and energy landscape of many birds during migration (Shamoun-Baranes et al., 2017). Wind data were bilinearly interpolated from the NCEP NARR u (easterly/zonal) and v (northerly/meridional) components of wind predicted 30 m above ground in m/s, from which we calculated the wind support ztw, such that ztw ∈ (−∞, ∞) (Safi et al., 2013), where positive values correspond to tailwind and negative values headwind. The bearings used to calculate each ztw, i were the compass bearings required to arrive at xi+1 from xi.
We included a time of day interaction in the model because of clear diurnal effects. This also helped reduce zero inflation, particularly for thermal uplift, which often decays to zero after sunset due to heat flux and atmospheric boundary layer dynamics. To introduce the interaction, we used a dummy variable z0, such that z0, i = 0 when ti fell after sunset but before sunrise and z0, i = 1 when ti fell after sunrise but before sunset. This assumed behavior was not dependent on the covariates at night—the combination of covariates becomes zero when z0, i = 0—which is sensible given observed diurnal behavioral cycles. Sunrise and sunset times local to each GPS point were calculated in R with the “sunriset” function in the package “maptools” (Bivand and Lewin-Koh, 2016; R Core Team, 2016). Writing out the matrix operation in Equation (5), the final overall formulation of the behavioral process for the full model was:
Prior to fitting the model, we followed Gelman et al. (2008) and log-transformed the uplift covariates and standardized variance to 0.25. We used a shifted log-transformation (Fox and Weisberg, 2019); adding one to the covariates prior to the log-transformation preserved zeros (i.e., zeros mapped to zero under the transformation). The distribution of raw wind support data appeared Gaussian, so it was only centered and standardized.
Parameter Estimation and Model Selection
We fit our correlated random walk (CRW) in a Bayesian framework. Because the model has explicit serially correlated parameters, we used Hamiltonian Monte Carlo (HMC) over more conventional Markov-chain Monte Carlo (MCMC; e.g., Metropolis steps) to sample efficiently from a posterior with such correlation.
Gelman et al. (2008) suggested Cauchy priors for logistic regression parameters; however, Ghosh et al. (2015) found that sampling from the posterior can be inefficient due to the fat tails of the Cauchy distribution. We thus chose Studentt priors centered on zero (μ = 0 and σ = 2.5) with five degrees of freedom as weakly informative priors for the covariate parameters. Weakly informative normal priors were placed on the variance parameters of the model.
We implemented HMC with R and Stan through the package “rstan” (R Core Team, 2016; Stan Development Team, 2016). Working R and Stan code, including details on prior choice, and example data are provided as Supplementary Material, as well as supplementary tables and figures (Appendices 1–3). The model was fit to each track independently with five chains of 300,000 HMC iterations, including a 200,000 iteration warm-up phase, and retaining every tenth sample. Convergence to the posterior distribution was checked with trace plots, effective sample sizes, posterior plots of parameters, and Gelman diagnostics () for each model fit.
We compared candidate models with leave-one-out cross-validation approximated by Pareto-smoothed importance sampling (PSIS-LOO) in R with the package “loo” (Vehtari et al., 2016, 2017). The candidate models included possible combinations of environmental covariates plus a null CRW model without covariates. To limit model complexity and because we were interested in competing hypotheses about key predictors of behavior, we chose not to include interactions beyond time-of-day. We ranked the models by the expected log pointwise predictive density (elpd; i.e., out-of-sample predictive accuracy) transformed onto the deviance scale (looic; Vehtari et al., 2017), which created a measure on the same scale as common information criterion (e.g., AIC) and allowed applying the rules of more traditional information-theoretic model selection (e.g., Burnham and Anderson, 2004). The model with the lowest looic was considered the best fit to the data, but if other models were within two looic of the top model, each, including the top model, were considered equally supported by the data.
To understand how the predictive ability of the full model varied among tracks, we also computed a pointwise average of the elpd for each track (Vehtari et al., 2017). Normalizing by the sample size allowed comparing the out of sample predictive ability of the full model across individual migration tracks (Table S1 in Appendix). The elpd (and looic), being sums, are otherwise dependent on the sample size for each model fit.
Results
Model Performance and Diagnostics
We fit eight candidate formulations of our CRW model to 31 migration tracks, equating to 248 total model fits. Chain mixing, Gelman diagnostics () close to one, and large effective sample sizes for all parameters indicated convergence to the posterior for most model fits. Posteriors of parameters appeared symmetric, also indicating the model was well behaved (Figure S1 in Appendix). Across all migration tracks, the full model showed strong evidence of convergence, but for five tracks, we did not consider the null model converged to the posterior (e.g., ). The five migrations for which the null model did not converge were not included from formal model selection.
Behavior During Migration
Median (interquartile range) departure and arrival dates were 5 March (4.5d) and 27 March (6.4d) in the spring and 29 September (11.7d) and 16 November (15.5d) in the fall. On average, eagles encountered similar orographic uplift in spring and fall but more intense thermal uplift and tailwind in the spring (Table 1).
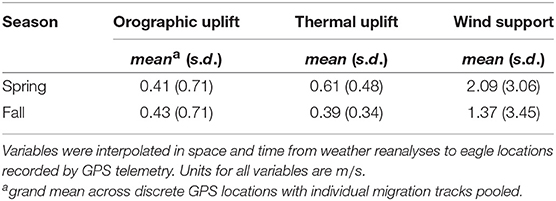
Table 1. Summary statistics of flight subsidies encountered by migrating golden eagles that summer in Alaska.
The model revealed that eagles changed their behavior on multiple scales. First, there were very strong daily rhythms in behavior during migration, with birds migrating or moving more slowly and tortuously during the day and stopping at night (Figures 1, 2). Explicitly including a time-of-day interaction could cause a daily rhythm to emerge as an artifact of model specification. However, accounting for serial correlation in behavior (Equation 5) limited that possibility. Additionally, prolonged periods of movement without an apparent daily rhythm suggest that, where daily rhythms are observed they are not a product of model specification (Figure 2). Second, there was some evidence of stopover-like behavior, but with individuals continually moving along the migration route while exhibiting less directional persistence in movement (Figure 3). The continuation along the migration route while in a stopover-like state is highlighted by track segments extended over space associated with low and intermediate estimates of γi (blue/purple in Figure 3).
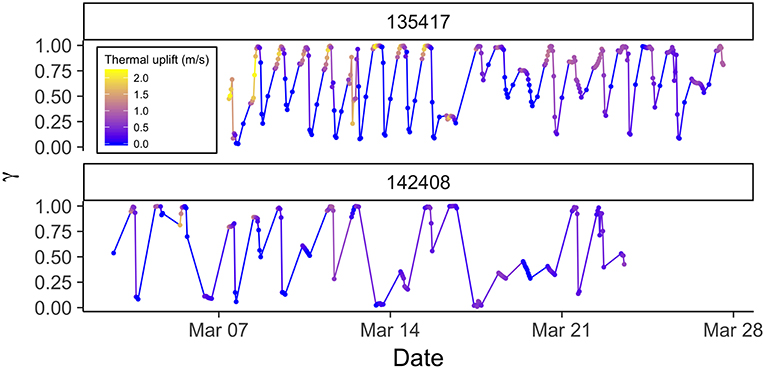
Figure 1. Time series of behavior parameter γ from correlated random walk model with full behavioral process (orographic uplift, thermal uplift, and wind support as predictors) for two golden eagles during spring migration with PTTs reporting on different duty cycles. Upper panel is 13 hourly centered on solar noon plus one at midnight, and the lower panel is 8 hourly centered on solar noon. γ close to one reflect movements associated with migratory behavior, and γ close to zero stopover behavior. Points are times of observations, and lines are linear interpolations between points. Hue indicates intensity of thermal uplift, with yellow indicating greater and blue lower. Note the daily rhythm in behavior associated with intense thermal uplift, stopover periods of one or more days, and the intermediate periods suggesting fly-and-forage.
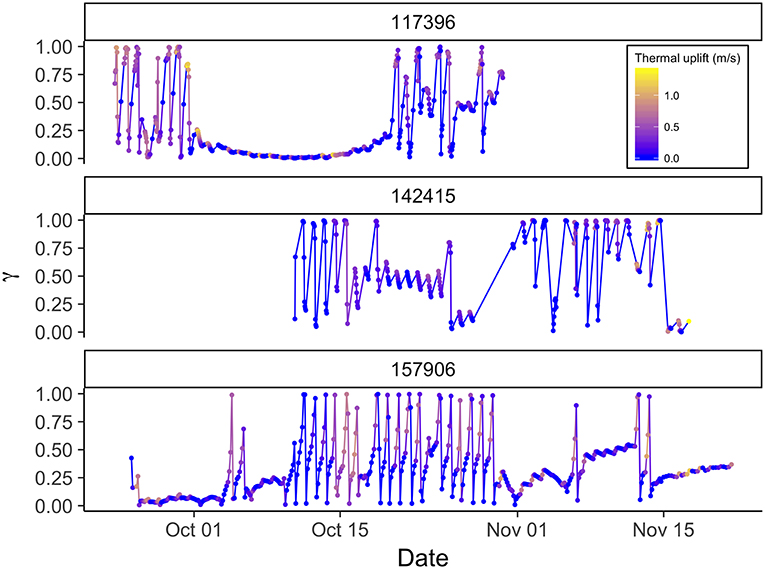
Figure 2. Time series of behavior parameter γ from correlated random walk model with full behavioral process (orographic uplift, thermal uplift, and wind support as predictors) for three golden eagles during fall migration with PTTs reporting on different duty cycles. Upper panel is 13 hourly centered on solar noon plus one at midnight, middle panel is 8 hourly centered on solar noon, and lower panel is fixed 3-h interval. γ close to one reflect movements associated with migratory behavior, and γ close to zero stopover behavior. Points are times of observations, and lines are linear interpolations between points. Hue indicates intensity of thermal uplift, with yellow indicating greater thermal uplift and blue lower. Note the daily rhythm in behavior and extended stopovers as well as periods intermediate values suggesting fly-and-forage.
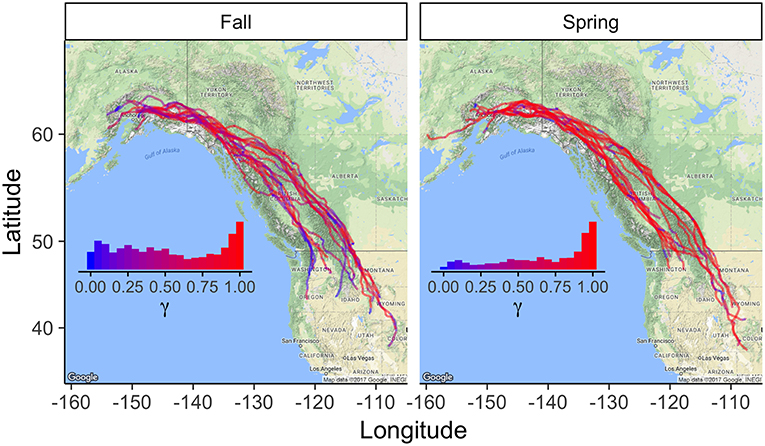
Figure 3. Golden eagle migration trajectories (N = 15 spring and N = 16 fall). Hue indicates value of behavioral parameter γ estimated with the correlated random walk model with full behavioral process, including orographic uplift, thermal uplift, and wind support as predictors. Insets show the relative frequencies of estimates of γ assigned to the displacements between observed daytime GPS locations. γ close to one reflect movements associated with migratory behavior, and γ close to zero stopover behavior. Daily rhythms, revealed in Figures 1, 2, are not apparent here because the birds moved so little at night.
There was also a clear effect of season on movement patterns and behavior. Spring was characterized by straighter, more direct trajectories and punctuated by slower, more tortuous, stopover-like movement; whereas, fall movements were much more tortuous overall and regular patterns in changes in movement rate and/or tortuosity less clear (Figures 1–3). The distributions of estimated γ values also clearly indicate that daytime movements were most frequently directed migratory moves in the spring; whereas, in the fall, the bimodal distribution indicates more equivalent partitioning between directed migratory moves and slower stopover type movement, with significant time spent exhibiting behaviors associated with intermediate tortuosity and movement rate (Figure 3).
Environmental Covariates
While there were differences in some environmental covariates between spring and fall (Table 1), parameter estimates from the full model (all covariates) indicate that there was little to no difference in effect of flight subsidies (i.e., wind and uplift) on behavior between spring and fall (Figure 4, Table S1 in Appendix). Including environmental covariates in the behavioral process, though, improved model fit for almost all fitted migrations (Table 2). Positive coefficients on the thermal uplift covariate indicate that increasing thermal uplift resulted in more highly-correlated displacements, or migratory movements. Despite that, there were some migration bouts not associated with great thermal uplift (Figures 1, 2). Coefficients close to zero for orographic uplift and wind support indicate that, in general, they were not strong drivers of directionally-persistent movements.
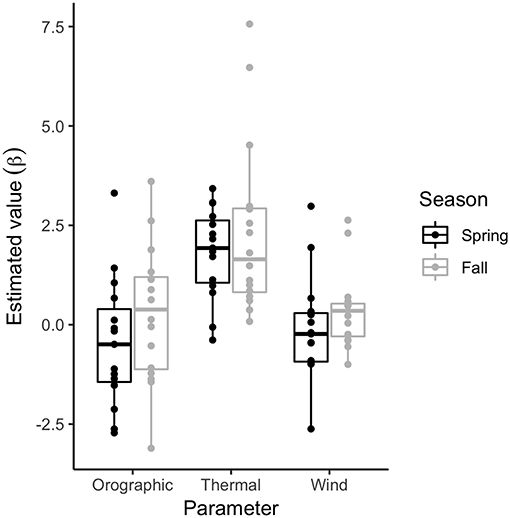
Figure 4. Point estimates of environmental covariate effect parameters (βou, βtu, βtw) on golden eagle behavior and movements during migration (N = 15 spring and N = 16 fall). Estimates are from the correlated random walk model with full behavioral process, including orographic uplift, thermal uplift, and wind support as predictors.
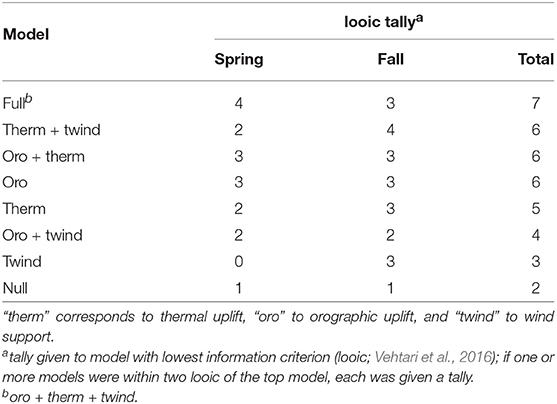
Table 2. Number of golden eagle migration tracks recorded by GPS transmitters that each candidate formulation of the behavioral process in the correlated random walk model fit the best, according to approximate leave-one-out cross-validation (Table S1).
Based on the model selection, the best-fitting formulation of the environmental drivers of the behavioral process was variable across individuals. However, in almost all cases, some form of flight subsidy was used and there was little difference between the spring and fall seasons in the pattern of subsidy use (Table 2). The high variability across individuals (Table S1 in Appendix) was likely due to differing weather patterns and thus subsidy sources encountered and/or used by each eagle as migrations were not synchronous (in time or space) across individuals. In addition, inter-individual variation was much larger than any difference attributable to demographic variables; we found no evidence that difference in sex or age explained patterns of flight subsidy use during migration. Note, though, that all eagles included in this analysis were in adult plumage, so strong age effects would not necessarily be expected.
Comparing the pointwise elpd across tracks revealed that the out of sample predictive ability of the full model varied among individuals (Table S1 in Appendix). It also showed that predictive ability was greater for fall migrations than spring.
Discussion
Here, we develop and demonstrate how dynamic parameter CRW models fit to GPS data reveal the effects of variable flight subsides available along migration routes. Use of these subsidies gives rise to diverse patterns in the movement of a long-distance soaring migrant. Behavioral changes occur continuously as available subsidies shift over time and space. These key driving mechanisms underlie emergent movement paths, yet such processes are often hidden in the discrete satellite observations available. Our mechanistic modeling approach allowed linking of dynamic meteorology to changes in behavior, and those changes in behavior to the observed movement paths, revealing time series of behaviors more complex than individuals simply apportioning time between migration and stopover.
Model Performance
Incorporating time-varying parameters into movement models has been a relatively infrequently utilized approach (Breed et al., 2012; Auger-Méthé et al., 2017; Jonsen et al., 2019). Here we provide a case study for its utility and developed the approach for achieving practical biological inference about movement processes. Modeling the serial correlation in movement as a function of environmental covariates (equation 4), allowed simultaneous inference of behavior and the effect of environmental covariates on behavior from animal trajectories with regular and irregular duty cycles and containing missing observations. While other methods exist to handle missing data, the behavioral patterns we found would be more difficult to reveal with a state-switching movement model (e.g., hidden Markov models (HMMs); Michelot et al., 2016) because each step would be forced into a discrete behavioral state from a set of usually 2–3 discrete states. Moreover, although hidden-state models have been introduced that have more than five discrete states (e.g., McClintock et al., 2012), these states can require ancillary data streams (e.g., accelerometry) to discriminate and remain extremely difficult to employ and interpret in practice (Patterson et al., 2017; Pohle et al., 2017). Finally, as HMMs include greater numbers of potential states, they tend to fit better than models with fewer states as judged by classical model selection approaches, such as AIC, even when additional states are neither biologically meaningful nor sensible (Pohle et al., 2017). Implementing models with dynamic parameters that can be interpreted along a behavioral continuum seems a more natural approach for many animal movement questions.
Effects of Tag Programming
While our CRW model revealed the same trends across duty cycles and was generally robust to the different duty cycles (Figures 1, 2), the most detail in daily behavioral rhythms was revealed in tracks with a fixed 3-hr time interval (lower panel in Figure 2), as it provided data throughout the 24-h day at regular intervals. The other duty cycles were initially chosen to minimize the risk of battery depletion overnight. Although generally robust, duty cycles did affect model fitting. HMC permitted Bayesian inference rather efficiently for our model, considering elevated correlation in the posterior of parameters due to the model formulation. Model fits typically took no more than a few hours, though tracks with much more than several hundred locations sometimes took longer. Preliminary fitting of our model with Stan and Template Model Builder (TMB; following Auger-Méthé et al., 2017) suggested that Maximum Likelihood estimation (when fit with TMB) tends to fail frequently when tag programming results in uneven temporal coverage of each day (e.g., our 2015 duty cycle), while Bayesian inference still provided sensible parameter estimates in most cases. Although the model presented herein and the model presented by Auger-Méthé et al. (2017) can make up for irregular time intervals between observations, they do have limitations. Breed et al. (2011) offer an in-depth discussion of tag programming and its effects on model fitting and inference.
Flight Subsidies as Drivers Migration of Behavior
Thermal uplift is a flight subsidy dependent on daily atmospheric boundary layer dynamics, and it was clearly an important driver of the daily rhythm in eagle movement (Figure 4, Table S1 in Appendix). Intense thermal uplift was often associated with the peaks in daily migration bouts (Figure 1). The larger magnitude of the thermal uplift effect, relative to orographic uplift, was somewhat surprising, as many individuals in our sample followed the Rocky Mountains, a large potential source of orographic uplift. Golden eagles are known to use orographic uplift as a flight subsidy while migrating through the Appalachian Mountains in eastern North America (Katzner et al., 2015). Much of the Appalachians, however, is characterized by long, unbroken, linearly-oriented ridges. Wind blowing over these ridges produces long stretches of predictable orographic uplift (Rus et al., 2017). The Rocky Mountains, by contrast, are far more rugged and nonuniform, and conditions that might produce suitable upslope winds and mountain waves, as well as strong tailwinds, likely also generate violent turbulence and could impede efficient migratory flight. Soaring raptors have been shown to use small-scale turbulence to achieve subsidized flight (Allen et al., 1996; Mallon et al., 2016); however, unpredictable, nonstationary violent turbulence, which can occur in large, high-elevation mountain ranges (Ralph et al., 1997), could produce unfavorable migratory conditions. The large effect of thermal uplift, thus, could indicate that the Rocky Mountains, a spine that spans almost the entire migration corridor for this population, as well as some areas further west (Bedrosian et al., 2018), serves as a network of thermal streets for migrating eagles (Pennycuick, 1998). More explicitly, intense sun on south facing slopes would be expected to generate linear series of thermals that birds could glide between during both spring and fall migration. It is important to keep in mind that the migrants could capitalize on fine-scale, localized features of certain flight subsidies, like orographic uplift and tailwind, that may not have been captured by the interpolated meteorological data used in our analyses. However, model selection for models including those variables did indicate they explained some variance in eagle movement, which we discuss further below.
Despite meteorological conditions along migration paths that differed between spring and fall and a stark difference between behavioral budgets, our results showed no clear difference in the use of flight subsidies between the spring and fall seasons (Figure 4). This finding contrasts with season-specific effects of flight subsidies on golden eagle migration shown phenomenologically in eastern North America, where thermal uplift was shown to be the key subsidy in migratory performance during spring, while wind with some additional support from thermal uplift is most important in the fall (Duerr et al., 2015; Rus et al., 2017). Although our results indicate that eagles use similar flight subsidizing strategies in both seasons, consistent with the differences from the eastern population, the actual behaviors performed during spring and fall migrations differed considerably. In spring, eagles used subsidies to drive a migration that allows timely arrival on the breeding grounds, consistent with a time minimization strategy. In the fall, flight was subsidized to minimize net energy use, which emerged as a much more diverse behavioral repertoire during a slower fall migration (Figure 3; Miller et al., 2016). The more rapid and direct flight punctuated by bouts of tortuous, stopover-like movement in the spring (Figure 3), suggest eagles pause, refuel, and/or perhaps wait for better migration conditions. This suggests eagles may employ, at least in part, a net energy maximization strategy (Hedenström, 1993; Miller et al., 2016), despite the need for timely arrival on the breeding grounds to avoid fitness costs (Both and Visser, 2001).
The behavioral time series of spring migrations showed some evidence of individuals responding less to thermal uplift as latitude increased (Figure 1). This likely corresponded to a general decay in thermal uplift as individuals migrated northward (Supplementary Material, Figure S2 in Appendix). Reduced thermal uplift availability would be expected at higher latitudes due to the larger amounts of remaining spring snowpack and lower solar angles. Thus, golden eagles, and likely other soaring birds, migrating to high latitudes may need to budget behaviors carefully between time minimization and net energy maximization during spring migration to best take advantage of the reduced flight subsidy from thermal uplift and mitigate the greater energy demands of flight at higher latitudes.
While our results show that thermal uplift is the most important flight subsidy for the majority of migrating eagles sampled, the model selection indicated orographic uplift and wind support improved out of sample predictive accuracy and explained some variance in eagle movement. Additionally, variability in top models across individuals (Table 2, Table S1 in Appendix) suggests among-individual variance in flight-subsidizing strategy. Although some of this variability can be attributed to real individual differences in behavioral strategy, it is at least as likely that individuals encountered different subsidies en route and used the subsidies they had available, as migrations across our sample were not synchronous. Given that orographic uplift and wind support parameter estimates were negative or close to zero for many individuals (Figure 4, Table S1 in Appendix), those covariates likely predicted the periods of slower, more tortuous movements (i.e., γi closer to zero). Wind support occurred in top models for more individuals in the fall (Table 2, Table S1 in Appendix), which is consistent with findings from others (McIntyre et al., 2008; Rus et al., 2017) and suggests it may be important during southbound migrations. Additionally, although there was variance among the types and combinations of subsidies used, the null model (without flight subsidies) was the best fitting model for very few tracks (Table 2, Table S1 in Appendix), evidence that weather and flight subsidies are of importance to migrating golden eagles, and likely also to the migrations of similar soaring species. Lastly, we found that the full model had, on average, better predictive ability in fall than spring (Table S1 in Appendix), suggesting that the weather variables explained more of the variance within movement paths in fall compared to spring; during spring migration, other internal state variables associated with greater time limitation that were not explicitly accounted for in the models were likely responsible for this seasonal difference.
Daily Rhythm and Migratory Pace
The full movement model revealed two clear, nested behavioral patterns in the long-distance migrations of golden eagles. First, there was a daily rhythm where inferred directed migratory movements (i.e., γi close to one) occurred most frequently around midday or early afternoon (Figures 1, 2). Mechanistic models of animal movement have revealed diel behavioral rhythms in other taxa (Jonsen et al., 2006). The basic aspects of daily rhythms in vertebrate behavior have hormone controls (Cassone, 1990), but the benefits can include balancing migration progress and foraging bouts (Newton, 2008). Soaring migrants also benefit by synchronizing diel movement patterns with diel atmospheric cycles. That is, consistent with our results, diurnal soaring migrants express a general circadian behavioral rhythm, where flight performance and behavior is strongly tied to thermal development of the planetary boundary layer to take best advantage of atmospherically generated flight subsides (Kerlinger et al., 1985; Leshem and Yom-Tov, 1989; Spaar and Bruderer, 1996, 1997; Mateos-Rodríguez and Liechti, 2012).
The second behavioral pattern revealed was a general stopover pattern, whereby eagles changed behavior for one to several days while en route (Figures 1–3). These changes were consistent with searching movements (i.e., γ intermediate or close to zero), possibly representing foraging behavior. In terms of soaring raptors, however, very few reports of movement patterns and behavior during stopovers have been published. Stopover segments have been previously identified by speed or some other metric calculated from tracks, then excluded from subsequent analyses (e.g., Katzner et al., 2015; Vansteelant et al., 2015); occasionally, authors noted apparent enhanced tortuosity but explored it no further (e.g., Vansteelant et al., 2017). On occasions where stopover behavior was considered, classifications based on stay duration and travel distance or speed with hard, often arbitrarily chosen cutoffs between migrating and stopover segments were used (Chevallier et al., 2011; Katzner et al., 2012; Duerr et al., 2015; Miller et al., 2016). In contrast, our modeling framework aligned with the movement ecology paradigm (Nathan et al., 2008); it used the observed data—GPS locations, rather than a derived metric—and a theoretical movement process to infer behavior from movement patterns along tracks on a spectrum ranging from stopped to rapid, directionally-persistent movement.
Our analyses, however, showed that eagles still tended to continue along their migration route during periods of movement most resembling stopover, but with reduced movement rate and directional persistence (Figures 1–3). This pattern suggests a joint migration/opportunistic foraging behavior that resembles fly-and-forage migration (Strandberg and Alerstam, 2007; Åkesson et al., 2012; Klaassen et al., 2017), which is consistent with observations of en route hunting behavior of golden eagles by Dekker (1985). Such behavior could be used to maintain balance between time expenses and energy intake, as it allows simultaneous migration progress and foraging.
This pattern does not fall very well within the “stopover” paradigm (Gill, 2007; Newton, 2008), however, as true stops during the migrations we observed were rare, except for expected nightly stops. Rather, migrants seemed to change their pace—either by slowing down, moving more tortuously, or both—but still generally moved toward their migratory destination (Figures 1–3). Thus, instead of a discrete behavioral framework, whereby migrants switch between two migratory phases (migration and stopover) with very different movement and behavioral properties, we propose that, for certain taxa, a continuous alternative framework “migratory pacing” may be more appropriate and a natural way to interpret en route migratory behavior and movement dynamics. Such taxa would include some and perhaps many soaring migrants, as well some migrating species in other fluid environments such as fishes and marine mammals. Soaring birds, even when energy reserves are relatively depleted, likely can still make steady progress toward a migratory goal when flight subsidies are available. Flapping migrants, on the other hand, would not be able to achieve this as readily, due in part to the greater energy demands for sustained flight, and would require more regular refueling stopovers where migration progress is temporarily completely arrested. Both opportunism in foraging and use of energetic subsidies are likely key characters of fly-and-forage behavior and the ability to change pace of migration without actually stopping, as they relax the need for individuals to stopover in specific, food-rich habitats, which are required by most migrants with less flexibility in food and that lack the morphological specialization to maximally exploit the energetic subsidies available in moving fluids (Gill, 2007; Piersma, 2007).
Our model results revealed seasonal variability in migratory pacing by golden eagles. The tendency for eagles to exhibit movements matching fly-and-forage behavior, and pace their migrations more slowly was most apparent during fall migration. In contrast, spring migration was usually composed of much more punctuated events of slower-paced movements but these were still extended over space (Figure 3), indicating the eagles pace their migration and employ a mixed behavioral strategy to some extent in spring as well. During spring, hibernating mammalian prey would be minimally available, leaving carrion, along with a few non-migratory and -hibernating species (e.g., ptarmigan Lagopus spp. and hare Lepus spp.), as major food sources, which could help explain the more punctuated bouts of slower-pacing. Alternatively, individuals could have been slowed by poor weather conditions (Rus et al., 2017). Scavenging large ungulate carcasses would be extremely rewarding in terms of energy accumulation. Much of the carrion we used successfully to capture eagles was large ungulate (e.g., moose Alces alces), strongly suggesting that the population we sampled uses carcasses during migration. The bimodal distribution for the behavioral parameter γ in fall shows that eagles tended to budget daytime behaviors approximately equally between rapid, directed and slower-paced movements (Figure 3); the high frequency and range of intermediate values are, again, evidence for the more complex fly-and-forage and pacing dynamic, rather than eagles simply switching between stopover and migration. This behavioral complexity might be biologically important, allowing eagles to arrive on winter home ranges in better condition compared to migration strategies that do not incorporate en route foraging opportunity. In contrast to fall, daytime movements in the spring were typically faster-paced (i.e., larger-scale and directionally-persistent; Figure 3), consistent with a time minimization strategy, where eagles need to partition time more in favor of migration progress to ensure timely arrival on breeding grounds (Hedenström, 1993; Alerstam, 2011; Miller et al., 2016). We thus see in eagles, and propose more generally, that such pacing varies between and within seasons along the continuum between time minimization and net energy maximization strategies (Alerstam, 2011; Miller et al., 2016). A migrant's pace would be expected to depend upon their energetic demands, energetic subsidies available from the environment, and the importance of arriving at the migration terminus in a timely fashion (Nathan et al., 2008).
Implications and Conclusions
We developed and applied a movement model with time-varying parameters to help reveal the mechanisms underlying the migration of a long-distance soaring migrant that relies on incredibly dynamic flight subsidies. We found that variation in flight subsidies gives rise to changes in migrant behavior with thermal uplift seemingly most important. While these findings might be expected given previous phenomenological analyses (e.g., Duerr et al., 2012; Lanzone et al., 2012; Katzner et al., 2015; Vansteelant et al., 2015; Miller et al., 2016; Shamoun-Baranes et al., 2016; Rus et al., 2017), we were able to show how meteorology is a mechanism influencing changes in movement patterns and thus behavior.
In the behavioral budgets of migrating golden eagles, we identified an expected daily rhythm, as well as evidence for behavioral dynamics that would allow nearly simultaneous foraging and migration, which is greater complexity than the traditional stopover paradigm allows. Migratory pacing, facilitated by fly-and-forage behavior, expands the traditional notion of stopover, whereby a bird migrates until resting and refueling is required, at which point it stops for a brief period in specific habitat suitable for efficient foraging (Gill, 2007; Newton, 2008). This advance was enabled by incorporating time-varying parameters into the movement model, which revealed new behavioral patterns during migration of long-distance soaring migrants. While time-varying, dynamic parameters have been infrequently employed in movement modeling (Breed et al., 2012; Jonsen et al., 2013; Auger-Méthé et al., 2017), we have shown it is a promising approach that can overcome certain limitations in discrete state-switching models and help provide novel insight into animal behavior.
This approach also has potential for further development and for revealing additional new patterns in soaring bird movement; it has already been shown to help provide new insight for other taxa as well (Jonsen et al., 2019). Although we demonstrated the approach for several individual eagles, applying our methods across a larger sample and across more years will increase the inferential strength of our results. For example, previous work found effects of wind support and orographic uplift (e.g., Katzner et al., 2015; Vansteelant et al., 2015), where we, in accounting for an eagles' underlying movement process and the inherent autocorrelation in that process, found that those meteorological variables may be of less importance, at least compared to thermal uplift. It remains unclear though, whether these are system-specific findings or a more general result. Additionally, the model we present has potential to help assess effects of habitat on the movement decisions of soaring birds and other species. One potential avenue for such would be incorporating the model into a resource selection framework (e.g., step selection function). Furthermore, given the movement process is parameterized in terms of coordinate vectors, the position likelihood could be straightforwardly extended to include the z axis to investigate questions regarding flight height of soaring birds or dive depth of marine species, assuming data of acceptable temporal resolution and location error are, or become, available.
Data Availability
All movement data used for this manuscript are managed in the online repository Movebank (https://www.movebank.org/; IDs 17680093 and 19389828). The data contain information considered sensitive by the State of Alaska, but they could be made available at the discretion of the Alaska Department of Fish & Game and U.S. Fish & Wildlife Service. Code to fit the movement model to data and example data are provided as Supplementary Material.
Ethics Statement
Field procedures were conducted following the ADF&G Animal Care & Use Committee protocol #2013-036 and University of Alaska Fairbanks Institutional Animal Care & Use Committee protocol #859448.
Author Contributions
JE and GB conceived the ideas of the research presented herein. JE, MA-M, and GB designed analytical methods. TB, CB, and SL designed the field methodology. TB, CB, SL, and JE collected the data. JE analyzed the data, and led the manuscript. All authors contributed to drafts and editing of the manuscript and provided final approval for publication.
Conflict of Interest Statement
The authors declare that the research was conducted in the absence of any commercial or financial relationships that could be construed as a potential conflict of interest.
Acknowledgments
We thank M. Kohan, B. Robinson, T. & D. Hawkins and many others for support in the field and J. Liguori and N. Paprocki for help aging eagles. We also thank T. Avgar, P. Doak, T. Katzner, K. Kielland, C. McIntyre, A. Scharf, and P. Smouse for helpful comments on drafts of the manuscript. Funding was provided by the Alaska Department of Fish & Game (ADF&G) through the federal State Wildlife Grant Program, and the U.S. Fish & Wildlife Service (USFWS) provided PTTs and data. JE was supported by the Calvin J. Lensink Fund during part of the project. MA-M acknowledges the support of the Natural Sciences and Engineering Research Council of Canada. The findings and conclusions of this paper are those of the authors and do not necessarily represent the views of the USFWS. This manuscript has been released as a pre-print at Eisaguirre et al. (2019).
Supplementary Material
The Supplementary Material for this article can be found online at: https://www.frontiersin.org/articles/10.3389/fevo.2019.00317/full#supplementary-material
References
Åkesson, S., Klaassen, R., Holmgren, J., Fox, J. W., and Hedenström, A. (2012). Migration routes and strategies in a highly aerial migrant, the common swift Apus apus, revealed by light-level geolocators. PLoS ONE 7:e41195. doi: 10.1371/journal.pone.0041195
Alerstam, T. (1979). Optimal use of wind by migrating birds: combined drift and overcompensation. J. Theor. Biol. 79, 341–353. doi: 10.1016/0022-5193(79)90351-5
Alerstam, T. (2011). Optimal bird migration revisited. J. Ornithol. 152, 5–23. doi: 10.1007/s10336-011-0694-1
Alerstam, T., and Hedenström, A. (1998). The development of bird migration theory. J. Avian Biol. 29, 343–369. doi: 10.2307/3677155
Allen, P. E., Goodrich, L. J., and Bildstein, K. L. (1996). Within- and among-year effects of cold fronts on migrating raptors at Hawk Mountain, Pennsylvania, 1934-1991. Auk 113, 329–338. doi: 10.2307/4088899
Auger-Méthé, M., Albertsen, C. M., Jonsen, I. D., Derocher, A. E., Lidgard, D. C., Studholme, K. R., et al. (2017). Spatiotemporal modelling of marine movement data using Template Model Builder (TMB). Mar. Ecol. Prog. Ser. 565, 237–249. doi: 10.3354/meps12019
Avgar, T., Street, G., and Fryxell, J. M. (2014). On the adaptive benefits of mammal migration. Can. J. Zool. 92, 481–490. doi: 10.1139/cjz-2013-0076
Bedrosian, B. E., Domenech, R., Shreading, A., Hayes, M. M., Booms, T. L., and Barger, C. R. (2018). Migration corridors of adult Golden Eagles originating in northwestern North America. PLoS ONE 13:e0205204. doi: 10.1371/journal.pone.0205204
Bivand, R., and Lewin-Koh, N. (2016). maptools: Tools for Reading and Handling Spatial Objects, Version 0.8-39.
Blackwell, P. G. (1997). Random diffusion models for animal movement. Ecol. Model. 100, 87–102. doi: 10.1016/S0304-3800(97)00153-1
Blackwell, P. G. (2003). Bayesian inference for Markov processes with diffusion and discrete components. Biometrika 90, 613–627. doi: 10.1093/biomet/90.3.613
Blackwell, P. G., Niu, M., Lambert, M. S., and Lapoint, S. D. (2015). Exact Bayesian inference for animal movement in continuous time. Methods Ecol. Evol. 7, 184–195. doi: 10.1111/2041-210X.12460
Bohrer, G., Brandes, D., Mandel, J. T., Bildstein, K. L., Miller, T. A., Lanzone, M., et al. (2012). Estimating updraft velocity components over large spatial scales: contrasting migration strategies of golden eagles and turkey vultures. Ecol. Lett. 15, 96–103. doi: 10.1111/j.1461-0248.2011.01713.x
Both, C., and Visser, M. E. (2001). Adjustment to climate change is constrained by arrival date in a long-distance migrant bird. Nature 411, 296–298. doi: 10.1038/35077063
Brandes, D., and Ombalski, D. W. (2004). Modeling raptor migration pathways using a fluid-flow analogy. J. Rapt. Res. 38, 195–207.
Breed, G. A., Costa, D. P., Goebel, M. E., and Robinson, P. W. (2011). Electronic tracking tag programming is critical to data collection for behavioral time-series analysis. Ecosphere 2:10. doi: 10.1890/ES10-00021.1
Breed, G. A., Costa, D. P., Jonsen, I. D., Robinson, P. W., and Mills-Flemming, J. (2012). State-space methods for more completely capturing behavioral dynamics from animal tracks. Ecol. Model. 235–236, 49–58. doi: 10.1016/j.ecolmodel.2012.03.021
Breed, G. A., Golson, E. A., and Tinker, M. T. (2017). Predicting animal home-range structure and transitions using a multistate Ornstein-Uhlenbeck biased random walk. Ecology 98, 32–47. doi: 10.1002/ecy.1615
Bridge, E. S., Thorup, K., Bowlin, M. S., Chilson, P. B., Diehl, R. H., Fléron, R. W., et al. (2011). Technology on the Move: recent and Forthcoming Innovations for Tracking Migratory Birds. BioScience 61, 689–698. doi: 10.1525/bio.2011.61.9.7
Burnham, K. P., and Anderson, R. (2004). Multimodel inference: understanding AIC and BIC in model selection. Sociol. Methods Res. 33, 261–304. doi: 10.1177/0049124104268644
Cassone, V. M. (1990). Effects of melatonin on vertebrate circadian systems. Trends Neurosci. 13, 457–464. doi: 10.1016/0166-2236(90)90099-V
Chevallier, D., Le Maho, Y., Brossault, P., Baillon, F., and Massemin, S. (2011). The use of stopover sites by Black Storks (Ciconia nigra) migrating between West Europe and West Africa as revealed by satellite telemetry. J. Ornithol. 152, 1–13. doi: 10.1007/s10336-010-0536-6
Clark, C. W., and Butler, R. W. (1999). Fitness components of avian migration: a dynamic model of Western Sandpiper migration. Evol. Ecol. Res. 1, 443–453.
Dekker, D. (1985). Hunting behavior of golden eagles, Aquila chrysaetos, migrating in southwestern Alberta. Can. Field-Natur. 99, 383–385.
Dodge, S., Bohrer, G., Weinzierl, R., Davidson, S. C., Kays, R., Douglas, D., et al. (2013). The environmental-data automated track annotation (Env-DATA) system: linking animal tracks with environmental data. Movem. Ecol. 1:3. doi: 10.1186/2051-3933-1-3
Duerr, A. E., Miller, T. A., Lanzone, M., Brandes, D., Cooper, J., O'Malley, K., et al. (2012). Testing an emerging paradigm in migration ecology shows surprising differences in efficiency between flight modes. PLoS ONE 7:e35548. doi: 10.1371/journal.pone.0035548
Duerr, A. E., Miller, T. A., Lanzone, M., Brandes, D., Cooper, J., O'Malley, K., et al. (2015). Flight response of slope-soaring birds to seasonal variation in thermal generation. Funct. Ecol. 29, 779–790. doi: 10.1111/1365-2435.12381
Dunn, J. E., and Gipson, P. S. (1977). Analysis of radio telemetry data in studies of home range. Biometrics 33, 85–101. doi: 10.2307/2529305
Eisaguirre, J. M., Auger-Méthé, M., Barger, C. P., Lewis, S. B., Booms, T. L., and Breed, G. A. (2019). Environmentally-driven dynamic parameters in mechanistic movement models reveal complex migratory pacing in a soaring bird. bioRxiv [Preprint]. doi: 10.1101/465427
Fox, J., and Weisberg, S. (2019). An R Companion to Applied Regression, 3rd Edn. Thousand Oaks, CA: SAGE Publications Inc.
Gelman, A., Jakulin, A., Pittau, M. G., and Su, Y. S. (2008). A weakly informative default prior distribution for logistic and other regression models. Ann. Appl. Stat. 2, 1360–1383. doi: 10.1214/08-AOAS191
Ghosh, J., Li, Y., and Mitra, R. (2015). On the use of Cauchy prior distributions for Bayesian logistic regression. ArXiv 1507.07170.
Gurarie, E., Fleming, C. H., Fagan, W. F., Laidre, K. L., Hernández-Pliego, J., and Ovaskainen, O. (2017). Correlated velocity models as a fundamental unit of animal movement: synthesis and applications. Movem. Ecol. 5, 1–18. doi: 10.1186/s40462-017-0103-3
Hedenström, A. (1993). Migration by soaring or flapping flight in birds: the relative importance of energy cost and speed. Philos. Trans. R. Soc. B Biol. Sci. 342, 353–361. doi: 10.1098/rstb.1993.0164
Hedenström, A. (2008). Adaptations to migration in birds: behavioural strategies, morphology and scaling effects. Philos. Trans. R. Soc. B 363, 287–299. doi: 10.1098/rstb.2007.2140
Hooten, M. B., Johnson, D. S., McClintock, B. T., and Morales, J. M. (2017). Animal Movement: Statistical Models for Telemetry Data. New York, NY: CRC Press.
Johnson, D. S., London, J. M., Lea, M.-A., and Durban, J. W. (2008). Continuous-time correlated random walk model for animal telemetry data. Ecology 89, 1208–1215. doi: 10.1890/07-1032.1
Jonsen, I. D., Basson, M., Bestley, S., Bravington, M. V., Patterson, T. A., Pedersen, M. W., et al. (2013). State-space models for bio-loggers: a methodological road map. Deep-Sea Res. II Top. Stud. Oceanogr. 88–89, 34–46. doi: 10.1016/j.dsr2.2012.07.008
Jonsen, I. D., McMahon, C. R., Patterson, T. A., Auger-Méthé, M., Harcourt, R., Hindell, M. A., et al. (2019). Movement responses to environment: fast inference of variation among southern elephant seals with a mixed effects model. Ecology 100, 1–8. doi: 10.1002/ecy.2566
Jonsen, I. D., Myers, R. A., and James, M. C. (2006). Robust hierarchical state-space models reveal diel variation in travel rates of migrating leatherback turtles. J. Anim. Ecol. 75, 1046–1057. doi: 10.1111/j.1365-2656.2006.01129.x
Kareiva, P. M., and Shigesada, N. (1983). Analyzing insect movement as a correlated random walk. Oecologia 56, 234–238. doi: 10.1007/BF00379695
Katzner, T. E., Brandes, D., Miller, T., Lanzone, M., Maisonneuve, C., Tremblay, J. A., et al. (2012). Topography drives migratory flight altitude of golden eagles: implications for on-shore wind energy development. J. Appl. Ecol. 49, 1178–1186. doi: 10.1111/j.1365-2664.2012.02185.x
Katzner, T. E., Turk, P. J., Duerr, A. E., Miller, T. A., Lanzone, M. J., Cooper, J. L., et al. (2015). Use of multiple modes of flight subsidy by a soaring terrestrial bird, the golden eagle Aquila chrysaetos, when on migration. J. R. Soc. Interface 12:20150530. doi: 10.1098/rsif.2015.0530
Kerlinger, P., Bingman, V., and Able, K. P. (1985). Comparative flight behavior of migrating hawks studied with tracking radar during autumn in Central New York. Can. J. Zool. 63, 755–761. doi: 10.1139/z85-110
Klaassen, R. H., Schlaich, A. E., Bouten, W., and Koks, B. J. (2017). Migrating Montagu's harriers frequently interrupt daily flights in both Europe and Africa. J. Avian Biol. 48, 180–190. doi: 10.1111/jav.01362
Kochert, M. N., Steenhof, K., McIntyre, C. L., and Craig, E. H. (2002). “Golden Eagle (Aquila chrysaetos),” in The Birds of North America, ed A. Poole (Ithaca, NY: Cornell Lab of Ornithology). Available online at: https://birdsna.org/Species-Account/bna/species/684/articles/introduction
Lanzone, M. J., Miller, T. A., Turk, P., Brandes, D., Halverson, C., Maisonneuve, C., et al. (2012). Flight responses by a migratory soaring raptor to changing meteorological conditions. Biol. Lett. 8, 710–713. doi: 10.1098/rsbl.2012.0359
Leshem, Y., and Yom-Tov, Y. (1989). The use of thermals by soaring migrants. Ibis 183, 667–674. doi: 10.1111/j.1474-919X.1996.tb04768.x
Luschi, P., Hays, G. C., Del Seppia, C., Marsh, R., and Papi, F. (1998). The navigational feats of green sea turtles migrating from Ascension Island investigated by satellite telemetry. Proc. R. Soc. Lond. Ser. B Biol. Sci. 265, 2279–2284. doi: 10.1098/rspb.1998.0571
Mallon, J. M., Bildstein, K. L., and Katzner, T. E. (2016). In-flight turbulence benefits soaring birds. Auk 133, 79–85. doi: 10.1642/AUK-15-114.1
Mateos-Rodríguez, M., and Liechti, F. (2012). How do diurnal long-distance migrants select flight altitude in relation to wind? Behav. Ecol. 23, 403–409. doi: 10.1093/beheco/arr204
McClintock, B. T., King, R., Thomas, L., Matthiopoulos, J., McConnell, B. J., and Morales, J. M. (2012). A general discrete-time modeling framework for animal movement using multistate random walks. Ecol. Monogr. 82, 335–349. doi: 10.1890/11-0326.1
McIntyre, C. L., Douglas, D. C., and Collopy, M. W. (2008). Movements of Golden Eagles (Aquila chrysaetos) from interior Alaska during their first year of independence. Auk 125, 214–224. doi: 10.1525/auk.2008.125.1.214
Michelot, T., and Blackwell, P. G. (2019). State-switching continious-time correlated random walks. Methods Ecol. Evol. 10, 637–649. doi: 10.1111/2041-210X.13154
Michelot, T., Langrock, R., and Patterson, T. A. (2016). moveHMM: an R package for the statistical modelling of animal movement data using hidden Markov models. Methods Ecol. Evol. 7, 1308–1315. doi: 10.1111/2041-210X.12578
Miller, T. A., Brooks, R. P., Lanzone, M. J., Brandes, D., Cooper, J., Tremblay, J. A., et al. (2016). Limitations and mechanisms influencing the migratory performance of soaring birds. Ibis 158, 116–134. doi: 10.1111/ibi.12331
Morales, J. M., Haydon, D. T., Frair, J., Holsinger, K. E., and Fryxell, J. M. (2004). Extracting more out of relocation data: building movement models as mixtures of random walks. Ecology 85, 2436–2445. doi: 10.1890/03-0269
Murgatroyd, M., Photopoulou, T., Underhill, L. G., Bouten, W., and Amar, A. (2018). Where eagles soar: fine-resolution tracking reveals the spatiotemporal use of differential soaring modes in a large raptor. Ecol. Evol. 8, 6788–6799. doi: 10.1002/ece3.4189
Nathan, R., Getz, W. M., Revilla, E., Holyoak, M., Kadmon, R., Saltz, D., et al. (2008). A movement ecology paradigm for unifying organismal movement research. Proc. Natl. Acad. Sci. U.S.A. 105, 19052–19059. doi: 10.1073/pnas.0800375105
Patterson, T. A., Parton, A., Langrock, R., Blackwell, P. G., Thomas, L., and King, R. (2017). Statistical modelling of individual animal movement: an overview of key methods and a discussion of practical challenges. AStA Adv. Stat. Anal. 101, 399–438. doi: 10.1007/s10182-017-0302-7
Pennycuick, C. (1998). Field observations of thermals and thermal streets, and the theory of cross-country soaring flight. J. Avian Biol. 29, 33–43. doi: 10.2307/3677338
Pennycuick, C. J. (1971). Soaring behavior and performance of some East African birds, observed from a motor-glider. Ibis 114, 178–218. doi: 10.1111/j.1474-919X.1972.tb02603.x
Piersma, T. (2007). Using the power of comparison to explain habitat use and migration strategies of shorebirds worldwide. J. Ornithol. 148(Suppl. 1):S45–S59. doi: 10.1007/s10336-007-0240-3
Pirotta, E., Katzner, T., Miller, T. A., Duerr, A. E., Braham, M. A., and New, L. (2018). State-space modelling of the flight behaviour of a soaring bird provides new insights to migratory strategies. Funct. Ecol. 32, 2205–2215. doi: 10.1111/1365-2435.13180
Pohle, J., Langrock, R., van Beest, F. M., and Schmidt, N. M. (2017). Selecting the number of states in hidden Markov models: pragmatic solutions illustrated using animal movement. J. Agricult. Biol. Environ. Stat. 22, 270–293. doi: 10.1007/s13253-017-0283-8
Ralph, F. M., Neiman, P. J., and Levinson, D. (1997). Lidar observations of a breaking mountain wave associated with extreme turbulence. Geophys. Res. Lett. 24, 663–666. doi: 10.1029/97GL00349
Rus, A. I., Duerr, A. E., Miller, T. A., Belthoff, J. R., and Katzner, T. E. (2017). Counterintuitive roles of experience and weather on migratory performance. Auk 134, 485–497. doi: 10.1642/AUK-16-147.1
Safi, K., Kranstauber, B., Weinzierl, R., Griffin, L., Rees, E. C., Cabot, D., et al. (2013). Flying with the wind: scale dependency of speed and direction measurements in modelling wind support in avian flight. Movem. Ecol. 1:4. doi: 10.1186/2051-3933-1-4
Sawyer, H., Lindzey, F., and McWhirter, D. (2005). Mule deer and pronghorn migration in western Wyoming. Wildl. Soc. Bull. 33, 1266–1273. doi: 10.2193/0091-7648(2005)33[1266:MDAPMI]2.0.CO;2
Shamoun-Baranes, J., Bouten, W., Loon, E. E. V., Meijer, C., Camphuysen, C. J., and Shamoun-baranes, J. (2016). Flap or soar? How a flight generalist responds to its aerial environment. Philos. Trans. R. Soc. B 371:20150395. doi: 10.1098/rstb.2015.0395
Shamoun-Baranes, J., Bouten, W., and Van Loon, E. E. (2010). Integrating meteorology into research on migration. Integr. Compar. Biol. 50, 280–292. doi: 10.1093/icb/icq011
Shamoun-Baranes, J., Liechti, F., and Vansteelant, W. M. (2017). Atmospheric conditions create freeways, detours and tailbacks for migrating birds. J. Compar. Physiol. A 203, 509–529. doi: 10.1007/s00359-017-1181-9
Shepard, E. L. C., Wilson, R. P., Rees, W. G., Grundy, E., Lambertucci, S. A., and Vosper, S. B. (2013). Energy landscapes shape animal movement ecology. Am. Nat. 182, 298–312. doi: 10.1086/671257
Spaar, R., and Bruderer, B. (1996). Soaring migration of Steppe Eagles Aquila nipalensis in Southern Israel: flight behaviour under various wind and thermal conditions. J. Avian Biol. 27, 289–301. doi: 10.2307/3677260
Spaar, R., and Bruderer, B. (1997). Optimal flight behavior of soaring migrants: a case study of migrating steppe buzzards, Buteo buteo vulpinus. Behav. Ecol. 8, 288–297. doi: 10.1093/beheco/8.3.288
Stan Development Team (2016). RStan: The R Interface to Stan, Version 2.10.1. Stan Development Team.
Strandberg, R., and Alerstam, T. (2007). The strategy of fly-and-forage migration, illustrated for the osprey (Pandion haliaetus). Behav. Ecol. Sociobiol. 61, 1865–1875. doi: 10.1007/s00265-007-0426-y
Turchin, P. (1998). Quantitative Analysis of Movement: Measuring and Modeling Population Redistribution in Animals and Plants. Sunderland, MA: Sinauer Associates.
Vansteelant, W. M. G., Bouten, W., Klaassen, R. H. G., Koks, B. J., Schlaich, A. E., Diermen, J. V., et al. (2015). Regional and seasonal flight speeds of soaring migrants and the role of weather conditions at hourly and daily scales. J. Avian Biol. 46, 25–39. doi: 10.1111/jav.00457
Vansteelant, W. M. G., Shamoun-Baranes, J., McLaren, J., van Diermen, J., and Bouten, W. (2017). Soaring across continents: decision-making of a soaring migrant under changing atmospheric conditions along an entire flyway. J. Avian Biol. 48, 887–896. doi: 10.1111/jav.01298
Vehtari, A., Gelman, A., and Gabry, J. (2016). loo: efficient leave-one-out cross-validation and WAIC for Bayesian models. R package version 1.0.0.
Keywords: Bayesian, correlated random walk, golden eagle, movement ecology, soaring flight
Citation: Eisaguirre JM, Auger-Méthé M, Barger CP, Lewis SB, Booms TL and Breed GA (2019) Dynamic-Parameter Movement Models Reveal Drivers of Migratory Pace in a Soaring Bird. Front. Ecol. Evol. 7:317. doi: 10.3389/fevo.2019.00317
Received: 11 March 2019; Accepted: 07 August 2019;
Published: 27 August 2019.
Edited by:
Frants Havmand Jensen, Woods Hole Oceanographic Institution, United StatesReviewed by:
Anne K. Scharf, Max Planck Institute of Ornithology, GermanyPeter E. Smouse, Rutgers University, The State University of New Jersey, United States
Copyright © 2019 Eisaguirre, Auger-Méthé, Barger, Lewis, Booms and Breed. This is an open-access article distributed under the terms of the Creative Commons Attribution License (CC BY). The use, distribution or reproduction in other forums is permitted, provided the original author(s) and the copyright owner(s) are credited and that the original publication in this journal is cited, in accordance with accepted academic practice. No use, distribution or reproduction is permitted which does not comply with these terms.
*Correspondence: Joseph M. Eisaguirre, am1laXNhZ3VpcnJlQGFsYXNrYS5lZHU=