- 1Sackler Institute for Comparative Genomics, American Museum of Natural History, New York, NY, United States
- 2Systematic Entomology Laboratory, USDA, National Museum of Natural History, Washington, DC, United States
Interpretations and analytical practices surrounding DNA barcoding are examined using a compilation of 3,756 papers (as of December 31, 2018) with “DNA Barcode” in the abstract published since 2004. By examining the rise of DNA barcoding in natural history and biodiversity science over this period, we hope to detect the extent to which its purposes, premises, rationale and application have evolved. The number of studies involving identification, taxonomic decisions and the discovery of cryptic species has grown rapidly and appears to have driven much of the publication activity of DNA barcode studies overall. Forensic studies and papers on biological conservation involving DNA barcodes have loosely tracked the ensemble number of studies but appear to have risen sharply in 2017. Although analytical paradigms have diversified, particularly following the growing availability of tools in BoLD, neighbor-joining and graphic (tree-based) criteria for species delimitation remain preeminent. We conclude that the practices and paradigms of DNA barcoding data are likely to persist and, in groups such as Lepidoptera, remain a widely used tool in taxonomic science.
The doing is often more important than the outcome.
—Arthur Ashe
Introduction
Widely heralded as a revolutionary taxonomic discovery tool, DNA barcoding represents perhaps the most reliable framework available for organizing specimens and specimen-based data for systematic research. Arranging specimens by barcode haplotype early in the study process allows for efficient inspection of material, and facilitates the organization and management of a wealth of character data and life history information, depending on how much is available for the barcoded specimens. While DNA sequences have been used to identify specimens or parts of specimens since the 1980's, their use as a broader natural history tool was not formalized until 2003. Three organizational meetings sponsored by the Sloan Foundation at the Banbury Center at Cold Spring Harbor and seminal publications that year (Hebert et al., 2003a,b; Stoeckle, 2003) christened DNA barcoding and launched the program that would globalize its application. Since then, over 3,700 peer-reviewed papers have been published with “DNA barcoding” in their title. These studies range from taxonomic works in which DNA barcodes are used to elucidate cryptic species, to surveys of environmental samples (e.g., marine sediments, ocean water) that feature estimates of phyletic diversity and regional comparisons of genetic variation, and finally to forensic and conservation applications. Many of the early papers can be characterized as proof-of-concept studies in which the utility of the COI barcoding region was being tested for particular taxonomic groups or in different study designs. To the extent controversy emerged around barcode data, it was generally associated with the taxonomic interpretation and applicability of their analyses. These included the uniformity and generalizability of criteria for circumscribing species, the phylogenetic implications of dendrograms, and the proliferation of informal specific epithets in reference to species that were discovered through DNA barcodes but which remained undescribed. Many of these concerns were mitigated by increasingly sophisticated treatments that incorporated barcodes with morphological, behavioral and ecological data under the rubric of integrative taxonomy and, for groups such as Lepidoptera in which extensive taxonomic coverage has been achieved (Hajibabaei et al., 2006; Hausmann et al., 2016; Zahiri et al., 2017), barcode data have become commonplace if not critical to taxonomic revisionary works.
As a paradigm, DNA barcoding engendered a democratization of molecular data (or at least metadata) by automating analytical steps that might otherwise have deterred may some practicing taxonomists. This quickened the pace of alpha taxonomy by enabling the rapid and unambiguous discovery of new species in many groups. One possible drawback has been that in coopting the terminology of phylogenetics, DNA barcode endeavors may have inadvertently broadened the meaning of or even re-branded terminology in a manner inconsistent with its formal interpretation. Taxonomic papers incorporating DNA barcode data routinely present metrics or tree graphics as self-evident while conflating descriptions with diagnoses or barcode trees with phylogenies. Semantics aside, we wished to understand whether such usage reflected a manifestation of some trend in how systematics is perceived by the scientific community at large.
The rapid growth of the DNA barcode paradigm thus invites an examination of how, during a 15-year period, its ontology and application developed with respect to technological, analytical, and terminological preferences that had until only recently fallen exclusively within the purview of molecular systematists. Our purpose here is to examine the development of DNA barcoding through a coarse examination of search terms and explore whether they reflect trends in how DNA barcoding practices may have evolved to accommodate analytical and practical considerations. To the extent they have not, we highlight those considerations at the empirical intersection of DNA barcoding, taxonomy and phylogenetics that are not simply semantic.
A Conceptual Framework for Examining the Ontology of DNA Barcoding
For clarity and transparency both, it is necessary to establish a conceptual framework on which to arrange this discussion. DNA barcoding intersects with systematics most conspicuously at the level of alpha taxonomy, that is in the discovery, diagnosis, and description of new species. “Description” and “diagnosis” are formal terms defined in nomenclatural codes (e.g., ICZN) that govern the naming of species and other taxa and the means of tracking and stabilizing taxonomic nomenclature. They represent components of taxonomic refinement and formalized nomenclatural change, and correspond to the character-based empirical work of substantiating named groups as historical or natural entities. It is generally understood that taxonomic rank does not of itself confer natural comparability: Any rank above species is a function of convention and discretion as well as actual data, and as long as monophyletic groups are recognized the fact that families or tribes are not uniformly or evolutionarily equivalent does not hamper studies unless they make the mistake of treating such groups, e.g., by inferring evolutionary trends from numbers of genera, families, etc. A named species, on the other hand, is a different sort of construct that may correspond to a range of biological entities consistent with historical, reproductive, or genetic criteria. Biological or historical comparability is perhaps more easily justified for species than for higher taxa because their identity as species can at least be tested by universal criteria, namely the establishment of diagnostic characters. At supra-specific taxonomic levels, in contrast, common ancestry is depicted hierarchically and articulated with reference to apomorphy, and independently derived diagnostic characters recognized as synapomorphies provide evidence both for a given species' inclusion in a given group and for that group's monophyly.
However, the usage of monophyly has been broadened to include its graphic depiction on trees, just as the traditional use of “phylogeny” as an abstract term for evolutionary history has been expanded and pluralized to include any tree-like graphics (“phylogenies”). At least one general consequence of this usage bears directly on the practice of DNA barcoding: the perception that species be legitimately represented and expected to appear as monophyletic. Whether one disputes this on the grounds that individual organisms are not related hierarchically even if mitochondria are (Doyle, 1995), or on the grounds that species often appear paraphyletic (Funk and Omland, 2003), the disconnection between the graphic representation of a monophyletic group and the characters underlying it is amplified when trees are treated as arbiters of species boundaries. When phylogenetics began to enjoy popularity, it was because there was consensus that empirical phylogenetic considerations were important to classification and evolutionary biology, but there remained strong methodological debates to the point where trees were judged less by what they said than how they were generated. The opposite experience seems to characterize DNA barcoding as a field. How barcode data—or any sequence data—are analyzed to generate trees bears directly on how those trees may be interpreted and on the scope of how DNA barcode data are ultimately used.
The ~3,700 DNA barcoding studies published over the past 15 years represent a prodigious record of peer-reviewed research, notwithstanding the variance in their intent or in the analyses and interpretations espoused. By examining the cohort of natural history and biodiversity science that incorporated DNA barcodes over this period, we explored the extent to which their purposes, premises, rationale and application have evolved.
3756 Barcoding Papers Since 2004
We compiled a glossary of terms used in DNA barcoding from our knowledge of the literature. We attempted to be as inclusive as possible with these terms and even included some from the literature on species boundaries and, speciation mechanisms. We next used the PubMed at NCBI (https://www.ncbi.nlm.nih.gov/pubmed/) to search for peer-reviewed papers with abstracts published since 2003. We used December 31, 2018 as a cutoff for inclusion in our database. In all, we compiled the abstracts from the 3,756 peer-reviewed papers with “DNA Barcode” as a query (Figure 1A), and used the resulting database (Supplementary Folder 1) to track the usage of specific terms as described below. Perhaps naïvely, all papers retrieved by the search are assumed to have been peer-reviewed as they are included in the PubMed database. Papers were cataloged by year from 2005 to 2018 since only a few papers appeared in 2003 and 2004. Hence, we combine 2003, 2004, and 2005 into a single data point. Abstracts from each of the papers were compiled in text files by year. Word searches were done in BBedit, an efficient textline editor, that retrieves the number and location of search terms. The location of the search term hit allowed us to eliminate duplicate hits in single papers. The number of hits for each search term (or combination of terms) were compiled in excel spreadsheets. Each of the terms in the glossary (Table 1) were searched and tabulated. Figure 1 provides more detail on the search strategies for the terms we used for generating graphs. For example, the raw number of hits for the general category “Neighbor Joining” was a combination of searches for “neighbor joining” plus “NJ.”
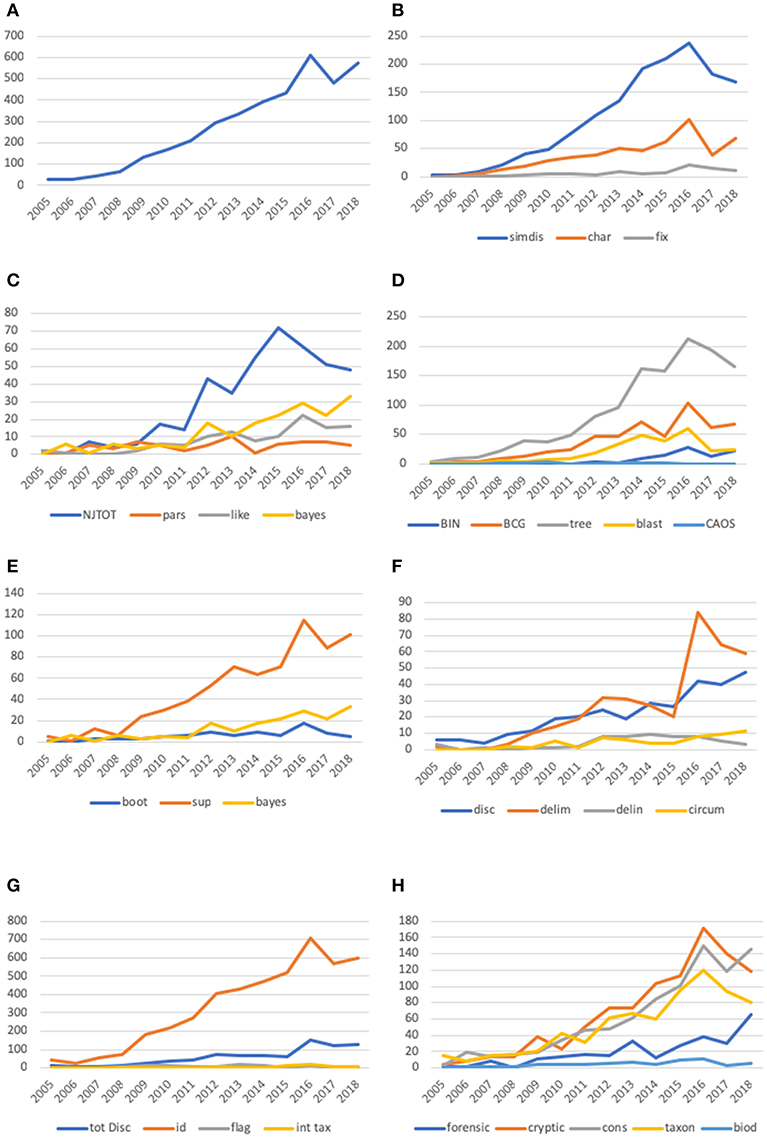
Figure 1. Line plots of number of “hits” for keywords in the DNA barcode vocabulary subcategories established in the text. In all graphs the number of citations is given on the Y-axis and year is given on the X-axis. We also computed relative percentage of citations per year and these results are shown in Supplemental Figure 1. (A) Graph of the occurrence of scientific papers with the search word “DNA barcoding” in the title from 2003 to 2018. The “blip” in number of papers in 2016 that disrupts an otherwise smooth increase in number of papers by year might represent an increase in reports for the several international meetings that occurred in 2015. (B) The results of this analysis compare character based approaches to similarity/distance approaches. For this analysis we also use fixation as a character based term and show its usage in the graph. Search terms: “similarity” and “distance” combined into “simdis” and “character” and “fixation” combined into “char.” We show the usage of “fixation” alone to demonstrate that this term is rarely used. (C) The results of this analysis compare the three major criteria for phylogenetic analysis—distance, parsimony and likelihood. Search terms: “NJ” and “neighbor joining” combined into “NJTOT,” “parsimony” listed as “pars,” likelihood listed as “like.” Bayesian phylogenetic inference methods have also been used and these are listed under “bayes.” (D) This figure shows comparison of the usage of terms that imply an examination of the robustness of the DNA barcode analysis. Such measures of robustness can be metrics such as bootstrap, or posterior probabilities such as in Bayesian phylogenetic inference. We also Search terms: “bootstrap” listed as “boot,” “support” listed as sup, statistic, bayes. (E) The figure compares various methods of treating DNA barcode data. We include tree to demonstrate the use of tree relative to these other approaches. Search terms: barcode index “number” and “BIN” combined into “BIN,” “barcode gap” listed as “BCG,” “tree” listed as “tree,” “blast” listed as “blast” and “character aggregation organization system” and “CAOS” combined into “CAOS.” (F) This figure shows the usage of species discovery vocabulary in DNA barcoding. As we point out in the text, species description is a technical term used in taxonomy, while other terms like circumscription, delimitation and delineation are terms used by biologists studying speciation and species boundaries. Search terms: “species discovery” listed as “disc,” “species delimitation” listed as “delim,” “species delineation” listed as “delin” and “species circumscription” listed as “circum.” (G) This figure compares the usage of “species discovery” terms with “specimen identification.” We also compare the usage of “flagging” listed as “flag” and “integrative taxonomy” listed as “inttax.” Search terms: “species discovery”or “totdisc” is the sum of counts for “species discovery,” “species delineation,” “species delimitation” and “species circumscription.” (H) This figure compares the focus of papers in five areas that are generally listed by DNA barcode studies. DNA barcoding has been used in forensic studies, biodiversity studies, taxonomy, cryptic species studies and conservation biology. Search terms: “forensic” listed as “forensic.” “cryptic” listed as “cryptic,” “conservation” listed as “cons,” “taxonomy” listed as “taxon” and “biodiversity” listed as “biod”.
An eclectic lexicon has grown around DNA barcoding, comprising a range of terms from taxonomy, phylogenetic and molecular systematics, and population genetics as well as a smattering of neologisms. The database we developed was queried for 29 terms based on our own extensive reading of the barcode literature. These terms span a range of purposes and methods, which we grouped according to (1) general disciplines (conservation/conservation biology/conservation genetics, forensic, taxonomy/systematics/integrative taxonomy, phylogeography); (2) biological terms (character, crypsis/cryptic species, fixation/fixed character, population); (3) graphic terms (clade, cluster, tree); (4) tree-building methods (Bayesian, likelihood, neighbor-joining, parsimony); (5) general purpose operational terms (diagnosis, species circumscription/delimitation/delineation, species description, species discovery, specimen identification/determination, flag); and finally (6) tools and metrics (barcode gap, BIN, BLAST, bootstrap, phylogenetic support). The queried terms comprise a combination of rudimentary verbiage commonly used in systematics and molecular evolution, with that specific to DNA barcoding. Neither their groupings nor the underlying terms are mutually exclusive, but we have tried to arrange the terms as coherently as possible. We did not account for context or whether the terms were used correctly or with approbation. In some cases, to facilitate broader comparisons we combined counts for intrinsically related terms such as similarity/distance, or terms used interchangeably such as species delimitation, circumscription and delineation. These are detailed in Figure 1, Table 1, and in Supplementary File 1.
Inevitably, this exercise is influenced by our own perspective which favors an integrative taxonomic approach to corroborating the results of barcode analyses with other observations. It is our impression that this perspective is reasonably widespread. In general, we prefer to think of DNA barcode variation as having the potential to reveal corroborating patterns in morphology and behavior than as necessary or sufficient requirements for discovering species or as means of generating universal distance thresholds as criteria for demarcating them. Our choice of queried terms also, therefore, reflects the distinction between indirect or tree-based interpretations that rely on inspecting dendrograms, and direct analyses of diagnostic characters. To the extent that trends may be evinced from our seemingly chimeric exploration of language, we hope that occasional inventories such as this serve to take stock of and even illuminate the direction of a field regardless of perspective.
We present the results in two ways: (1) in the form of raw counts by year to track raw usage (Figure 1; search terms themselves in Supplementary File 1) and; (2) as scaled percentages of the occurrence of all terms per year (Supplementary File 1). Although crude, this approach affords context for cross-comparison of year-to-year usage; we suspect more complex analysis of data such as these would simply obfuscate any observable trends.
Trends in DNA Barcoding Based on Its Vocabulary
Characters, Distance Measures, and Tree-Building Functions
An important comparison concerns the use of direct character information, which corresponds to the empirical treatment of observable data, vs. lumped (phenetic) summaries in the form of similarity or distance measures. By compressing character state information into a single measure of genetic similarity, distance measures mask changes in specific loci. As such, they do not enable one to discriminate homologous character state changes, much the way a mathematical average hides partitioned variation. For this reason, such methods have been eschewed in phylogenetic reconstruction for several decades and represent perhaps the most contentious points of discussion surrounding DNA barcodes.
The explosion of DNA barcode data and distance-based dendrograms did occasion certain remedial presentations (e.g., Prendini, 2005) of such methodological issues that had been debated and largely settled in the early decades of phylogenetic systematics. From our perspective, tree-building methods in the context of DNA barcoding are not, as they are in systematics, at issue on the grounds of their legitimacy as phylogenetic inference tools, if only because most studies suggest that COI analyzed in isolation is a fundamentally insufficient source of decisive phylogenetic information. Rather, distance methods fall short specifically in the realm of identification and diagnosis. The practical implications are (1) that above the level of very closely related species, the COI gene typically realizes its greatest contribution to phylogenetic matrices that include a combination of other organellar and nuclear genes (Cameron et al., 2007; Leavitt et al., 2013) and (2) that no level of parameterization can compensate for the levels of saturation that inevitably appear in datasets with distantly related species or particularly in datasets with more terminals than characters. The immediate concern for the purposes of DNA barcoding is not that COI is necessarily inadequate as a sole phylogenetic marker, but that the ability of any data analyzed via distance is equally impeded in serving the goals of DNA barcoding as it is in phylogeny reconstruction. This is a function of the incompatibility of distance data with the transmission of diagnostic information. Simply put, a properly rooted parsimoniously optimized tree represents the most efficient summary possible of the available data, and enables the direct diagnosis of would-be species based on observable character state changes. This is a matter of mathematics, not opinion (Farris, 1980). The ostensible advantage of Neighbor-joining is its computational ease and straightforward presentation (a single tree is generated). Interpretive issues may arise only if such analyses are accepted as decisive without further exploration.
Figure 1B compares the occurrence of the search terms “character” and “similarity+distance” and suggests a consistent preference for Neighbor-joining (NJ) a tree-building algorithm. This is of course at least in part a function of the tools available in BoLD (Ratnasingham and Hebert, 2007), and we do not suggest that these analyses are all interpreted identically or for the same purposes. Two empirically linked search terms “fixed” and “character” align with diagnostic approaches and track their usage (Figure 1B).
Explicit mention of other methods of sequence analysis, Neighbor-joining (NJ), parsimony or “maximum parsimony” (MP), maximum likelihood (ML), and Bayesian (Figure 1C), appear erratically prior to 2008. Since then, the mentions of ML and Bayesian analysis have risen but not approached those of NJ, with parsimony (MP) appearing least frequently. This result is not surprising given the initial availability of NJ as the prima facie tool in the Barcode of Life Database (BoLD) system.
Visualization and Interpretation of Trees
In our reading of the barcode literature we noted many cases where taxonomic decisions were based either directly on distance measures (e.g., the barcode gap, discussed below) or on trees generated by such measures, but effectively decoupled from justification or discussion of those methods. Following Goldstein and DeSalle (2011), we distinguish the strictly graphic, tree-based approaches from tree-independent approaches, among which we further differentiate distance-based (e.g., BIN, barcode gap, BLAST searches) from diagnostic (e.g., CAOS; Figure 1D). Despite occasional papers in which barcode NJ trees are referred to as phylogenies, many authors have been careful to stress the utility of DNA barcoding for identification and discovery, and not as explicit phylogenetic statements. To be clear, tree-based approaches are valuable both as inferential tools for visualizing prospective species delimitation, and as provisional road maps of where to direct further research in delimiting species boundaries.
The interpretation of a barcode tree as a visual first pass for demarcating species vs. a phylogeny properly focuses attention on the integrity of the species themselves rather than the groups to which they belong (see Introduction), and perhaps for this reason—as well as the nature of variation within the COI gene, the often high number of individual sequences under analysis, and the types of analysis employed—measures of nodal support tend to find limited relevance in typical barcode analyses. Measures of nodal support have been presented with increasing frequency among DNA barcoding studies (Figure 1E), but in our survey the search terms reflecting such use (bootstrap, Bayes and statistic) appear less than a fifth as frequently as the term “support” itself.
Tree graphics and BLAST searches have each been used steadily since the inception of DNA barcoding Figure 1D. The term “barcode gap” (BCG), first coined in 2005 (Meyer and Paulay, 2005 and reiterated by Wiemers and Fiedler, 2007), appears steadily after 2009 and is the most frequently used of the terms referring to tree-independent analytics. The most recently minted tree-independent approach (BIN; Ratnasingham and Hebert, 2013), is unique to DNA barcoding and its use has increased slightly since its introduction in 2010. In our survey there appears to be a preference for tree-based approaches accompanying the preference for NJ trees, and limited growth in the use of tree-independent terms (even distance-based ones) after 2015. Diagnostic algorithms (e.g., CAOS, Sarkar et al., 2008) appear rarely, consistent with the infrequent reliance on character-based tree-independent approaches relative to BIN, BLAST, and BCG. Table 2 summarizes the intersection between tree- and character-based (diagnostic) methods.
Specimen Identification and Species Delimitation
At the inception of DNA barcoding, two of its most frequently stressed benefits were specimen identification (or determination) and species discovery (Figure 1F). Specimen identification has been used interchangeably with “species identification” in some publications, as have a number of terms related to identification and discovery. DeSalle (2006) used the term “identification” only in the context of assigning taxonomic information. Although in the present paper we refer to this as “determination” (of specimens, not species), the published usage is too broad in intent to be parsed with any great deal of precision. Since the power of DNA barcoding resides in the coverage of the available database, the conclusion that a given species is new to science for example, is a function of whether a queried sequence corresponds to those from authoritatively identified specimens. The discovery of species new to science is thus a function of failure to assign a valid name to a given sequence under the assumption that identical (or highly similar) available sequences represent conspecific individuals. As such, “discovery” has for some authors been more controversial than identification (Matz and Nielsen, 2005), and that controversy may easily be amplified by the use of barcoding to estimate species richness in bulk samples (Andersen et al., 2012; Shokralla et al., 2012; Kress et al., 2015; Sickel et al., 2015). Specimen identification, particularly for thoroughly studied and well-sampled groups, holds broader appeal, particularly outside the academic community.
Incorporating DNA barcoding with taxonomy has been discussed and widely adopted as a form of integrative taxonomy, which simply refers to simultaneous analysis of disparate sources of data (Figure 1G). DNA barcodes are among the more readily got and appealing forms of data that may be used to flag specimens as warranting taxonomic attention (Goldstein and DeSalle, 2011). Based on their occurrences summarized in Figure 1F, “integrative taxonomy” and “flag” are not often used explicitly in connection with species “discovery.” This may suggest a disconnect between the appeal of species discovery in the abstract and its actual undertaking. If so, it highlights the important point that cryptic species discovered from DNA barcodes are not always accompanied by taxonomic revisionary work.
Since its inception, DNA barcoding has been bolstered by its utility for discovering cryptic species specifically as well as in taxonomic revision, forensics, conservation and biodiversity studies generally. Recognizing the potential bearing of cryptic species on each of these fields, Figure 1H illustrates that the study of cryptic species has consistently played a focal role in a range of fields over the 15-year period we examined, with explicit mention of conservation and taxonomy appearing with less frequent emphasis, followed by “forensic” and “biodiversity.”
Meaning
Examinations of word usage are productive only to the degree that common ground in both meaning and intent is well-understood, and inferences from any compendium of word usage are only as good as the precision with which the search terms were originally used. Loose usage of terms like “diagnosis” or “tree” seem inevitable as barcoding tools become increasingly accessible. As genomic data are generated with increasing ease, it remains to be seen whether the enthusiasm for DNA as it is currently practiced will transition to the larger endeavor of archiving accessible genomic data.
The most obvious and important result of the exercises performed here is that distance or phenetic approaches have prevailed in DNA barcoding practices for reasons that appear to be more practical than scientific. Conflating distance data with diagnoses and algorithms with tree graphics are not uncommon mistakes in the taxonomic literature. Although the use of NJ trees or distances to diagnose species appears in the literature, we would argue that doing so obviates the real diagnostic value of barcode data that would meet the requirements of diagnoses set forth in the ICZN and elsewhere.
Distance-based methods have a well-established place in population genetics, where they play important roles in evaluating raw divergence among related individuals or populations. In the context of phylogenetic inference, however, clustering operations based on phenetic similarity have for several decades been rejected by systematists for empirical and statistical reasons, not the least of which is that since they combine available character data into a single ensemble metric, they cannot test or summarize specific character homologies that would otherwise contribute to a diagnosis (Ferguson, 2002; DeSalle, 2007; Little and Stevenson, 2007). Distance metrics are nevertheless easy to calculate and methods such as NJ generate dendrograms with a seeming minimum of ambiguity. The development of DNA barcode databases hinged on the ease of NJ precisely because of this computational ease, because any lack of decisiveness among the data is not transparent in seemingly unambiguous single tree that obtains from every NJ analysis.
There exists quite a bit of variation in the handling of dendrograms (distance based figures) generated by DNA barcodes for purposes following the organization of specimens. Many draw empirical conclusions directly from a given NJ tree instead of using it recursively to examine/interpret other characters or pieces of information. But how researchers use the tree to summarize variation and evaluate actual support for would-be relationships varies considerably. Phenetic trees, rapidly generated as they are, risk yielding spurious representations of data, and represent liabilities to the extent that apparent tree structure is uncorroborated.
Clustering algorithms and dendrograms are used throughout biology for purposes ranging from ecological community analysis to visualizing gene expression data. The use of trees in phylogenetic science is distinguished from other applications by the implied superposition of a temporal dimension that enables testing hypotheses of character evolution. At its simplest, this is achieved by establishing polarity, or the direction of character state change, through the operation of rooting, followed by optimization of hypothetical character states at nodes. Regardless of whether scientists imagine distance-generated trees to be “phylogenies,” neither of these operations is possible on such trees without violating the fundamental assumptions of rooting and optimization. A raw dendrogram, however it is generated, is simply a form of metadata that summarizes similarity using a given metric or optimality criterion; it cannot by itself serve to “diagnose” anything with reference to observable character states much less evaluate synapomorphy, establish monophyly, or test ideas of character evolution.
To the credit DNA barcoding's architects, it has been stressed that barcode trees are not intended to serve as phylogenies, and as the menu of tools available on BOLD has expanded to include features that enable proper diagnoses, it is our hope that the number of taxonomic papers perpetuating that error will one day subside. Our purpose is not to belabor this any further, but to stress that despite their computational ease, NJ trees render barcode data under-utilized.
Discussion
Inevitably, whenever a new tool is developed that expedites a set of tasks, the training required prior to that development becomes at least partly obsolete, and it becomes easy to overlook standards—obsolete or not—that went along with it. In this case those standards range from matters as straightforward as species diagnosis to the more nuanced interpretation of molecular phylogenetic trees. It has at times appeared as though the antiquated view of systematics as an exercise in naming things, rather than an empirical endeavor to reconcile classifications with evolutionary hypotheses, has persisted. Graphic summary statements of phylogenetic data are rarely as decisive as they appear when stripped of their analytical details, and from the taxonomy-as-nomenclature perspective, systematics is seen as a pedantic holdover of Victorian pseudo-science, its practices the relics of a bygone era, and the very existence of undescribed species or unstable classification the function of some intrinsic psycho-intellectual flaw known collectively as the “taxonomic impediment” rather than a reflection of the raw magnitude of biodiversity. Similar brands of taxonomic naïvete have manifested elsewhere, as in recent debates over wisdom of taxonomic descriptions using photographs as “types.” (Garraffoni and Freitas, 2017; see also Amorim et al., 2016, Ceríaco et al., 2016, Pape, 2016, Santos et al., 2016). Although hailed as a possible solution to the taxonomic impediment, DNA barcoding performed uncritically risks the encumbrance of subsequent efforts and defeats its own purpose.
It seems generally accepted that, with exceptions in various groups ranging from genera to families, conventional barcode analyses work quite well in circumscribing potentially recognizable species that can be further corroborated with other characters. Why then be concerned about using distance measures as arbiters of identity? Although this paper is no place to resurrect a discussion on species concepts, there is nothing mysterious about the fact that barcode analyses tend to predict species that are ultimately recognizable by other means—certainly the rigorous evaluation of candidate loci undertaken before settling on COI has resolved that much. But it is important to separate the statement that NJ analyses “work” to identify species from the supposition that they allow us to infer anything about species in the abstract. The premise of the claim that NJ works to identify species united by some abstracted metaphysical property is that the species criterion is unspecified. This is not mere sophistry: Without establishing or allowing for an independent criterion for corroboration, there can be no means of evaluating what works and what does not because the claim is fundamentally unfalsifiable. If we adopt the perspective that species—whatever evolutionary concepts to which they may or may not conform—can be palatably recognized by congruent character data, then accepting provisional clusters as working hypotheses subject to further corroboration is quite reasonable. In other words, the fact that a very high proportion of diagnosable species are captured by NJ analyses is encouraging, but not sufficient. We maintain simply that even a small a small percentage of species overlooked or misdiagnosed warrant acknowledgment and the arbitrariness of inferring a universal distance measure is unnecessary when the means exist for quantifying diagnostic features directly.
DNA barcoding represents a tool with a range of empirical uses as broad as the array of taxa and available specimens with accompanying barcodes. Although these empirical uses do not extend to rigorous phylogenetic testing, barcode data realize their greatest potential throughout the recursive process of taxonomic investigation. In our view, the coupling of DNA barcoding with distance methods rendered its potential as a taxonomic tool under-realized. Although we actively embrace DNA barcoding in our own taxonomic research and as a near-universal advance for taxonomic research in general, we reject the premise that DNA barcoding serves to repair some inherent flaw in the practice of systematics. We view the taxonomic impediment not as a manifestation of human-induced shortcomings but as a reflection of the magnitude of global species richness.
We hope to have distinguished methodological issues from semantic ones, by pointing out, for example, the percent differences are by definition mathematically non-diagnostic. But our primary is not to redress common practices, but to suggest that more could be gained from additional analyses that would serve the formal taxonomic goals of diagnosis. It is not our intent to cast a pall over the use of barcode data to uncover diversity at fine scales, but to articulate how those data may continue to be enhanced. We stress the importance of not over-stating the implications of a word survey; our hope is merely to have provided a crude calibration of how quickly we might reasonably expect to see significant shifts in how barcode data are analyzed. A conclusion of this exercise is that researchers are more likely to follow the examples of their peers and use the tools most readily available than they are to ponder the minutiae of evolutionary analyses.
Author Contributions
Both authors listed have made a substantial, direct and intellectual contribution to the work, and approved it for publication.
Disclaimer
The authors are solely responsible for the writing of this paper.
Conflict of Interest Statement
The authors declare that the research was conducted in the absence of any commercial or financial relationships that could be construed as a potential conflict of interest.
The handling editor and reviewer, RH, declared their involvement as co-editors in the Research Topic, and confirm the absence of any other collaboration.
Acknowledgments
RD acknowledges the Institute for Comparative Genomics at the AMNH (ICG-AMNH) and the Lewis and Dorothy Cullman Program in Molecular Systematics and the Korein Family for continued support. Mention of trade names or commercial products in this publication is solely for the purpose of providing specific information and does not imply recommendation or endorsement by the USDA; USDA is an equal opportunity provider and employer.
Supplementary Material
The Supplementary Material for this article can be found online at: https://www.frontiersin.org/articles/10.3389/fevo.2019.00302/full#supplementary-material
References
Amorim, D. S., Santos, C. M., Krell, F. T., Dubois, A., Nihei, S. S., Oliveira, O. M., et al. (2016). Timeless standards for species delimitation. Zootaxa 4137, 121–128. doi: 10.11646/zootaxa.4137.1.9
Andersen, K., Bird, K. L., Rasmussen, M., Haile, J., Breuning-Madsen, H., Kjaer, K. H., et al. (2012). Meta-barcoding of ‘dirt'DNA from soil reflects vertebrate biodiversity. Mol. Ecol. 21, 1966–1979. doi: 10.1111/j.1365-294X.2011.05261.x
Avise, J. C., Arnold, J., Ball, R. M., Bermingham, E., Lamb, T., Neigel, J. E., et al. (1987). Bridge between population, genetics and systematics. Ann. Rev. Ecol. Syst. 18, 489–522. doi: 10.1146/annurev.es.18.110187.002421
Brower, A. V. Z. (1999). Delimitation of phylogenetic species with DNA sequences: a critique of Davis and Nixon's population aggregation analysis. Syst. Biol. 48, 199–213. doi: 10.1080/106351599260535
Cameron, S. L., Lambkin, C. L., Barker, S. C., and Whiting, M. F. (2007). A mitochondrial genome phylogeny of Diptera: Whole genome sequence data accurately resolve relationships over broad timescales with high precision. Syst. Entomol. 32, 40–59. doi: 10.1111/j.1365-3113.2006.00355.x
Ceríaco, L. M., Gutiérrez, E. E., and Dubois, A. (2016). Photography-based taxonomy is inadequate, unnecessary, and potentially harmful for biological sciences. Zootaxa 4196, 435–445. doi: 10.11646/zootaxa.4196.3.9
Cheng, L., Connor, T. R., Sirén, J., Aanensen, D. M., and Corander, J. (2013). Hierarchical and spatially explicit clustering of DNA sequences with BAPS software. Mol. Biol. Evol. 30, 1224–1228. doi: 10.1093/molbev/mst028
Davis, J. I., and Nixon, K. C. (1992). Populations, genetic variation, and the delimitation of phylogenetic species. Syst. Biol. 41, 421–435. doi: 10.1093/sysbio/41.4.421
De Queiroz, K. (2007). Species concepts and species delimitation. Syst. Biol. 56, 879–886. doi: 10.1080/10635150701701083
DeSalle, R. (2006). Species discovery versus species identification in DNA barcoding efforts: response to Rubinoff. Conserv. Biol. 20, 1545–1547. doi: 10.1111/j.1523-1739.2006.00543.x
DeSalle, R. (2007). Phenetic and DNA taxonomy; a comment on Waugh. Bioessays 29, 1289–1290. doi: 10.1002/bies.20667
Doyle, J. J. (1995). The irrelevance of allele tree topologies for species delimitation, and a non-topological alternative. Syst. Bot. 20, 574–588.
Drummond, A. J., and Rambaut, A. (2007). BEAST: bayesian evolutionary analysis by sampling trees. BMC Evol. Biol. 7, 214–217. doi: 10.1186/1471-2148-7-214
Farris, J. S. (1980). The efficient diagnoses of the phylogenetic system. Syst. Zool. 29, 386–401. doi: 10.2307/2992344
Felsenstein, J. (1985). Confidence limits on phylogenies: an approach using the bootstrap. Evolution 39, 783–791.
Ferguson, J. W. H. (2002). On the use of genetic divergence for identifying species. Biol. J. Linn. Soc. 75, 509–516. doi: 10.1046/j.1095-8312.2002.00042.x
Fujita, M. K., Leaché, A. D., Burbrink, F. T., McGuire, J. A., and Moritz, C. (2012). Coalescent-based species delimitation in an integrative taxonomy. Trends Ecol. Evol. 27, 480–488. doi: 10.1016/j.tree.2012.04.012
Funk, D. J., and Omland, K. E. (2003). Species-level paraphyly and polyphyly: Frequency, causes, and consequences, with insights from animal mitochondrial DNA. Annu. Rev. Ecol. Evol. Syst. 34, 397–423. doi: 10.1146/annurev.ecolsys.34.011802.132421
Garraffoni, A. R., and Freitas, A. V. (2017). Photos belong in the taxonomic Code. Science 355, 805–805. doi: 10.1126/science.aam7686
Goldstein, P. Z., and DeSalle, R. (2011). Integrating DNA barcode data with taxonomic practice: Determination, discovery, and description. Bioessays 33, 135–147. doi: 10.1002/bies.201000036
Hajibabaei, M., Janzen, D. H., Burns, J. M., Hallwachs, W., and Hebert, P. D. (2006). DNA barcodes distinguish species of tropical Lepidoptera. Proc Natl Acad Sci U.S.A. 103, 968–971. doi: 10.1073/pnas.0510466103
Hausmann, A., Miller, S. E., Holloway, J. D., deWaard, J. R., Pollock, D., Prosser, S. W., et al. (2016). Calibrating the taxonomy of a megadiverse insect family: 3000 DNA barcodes from geometrid type specimens (Lepidoptera, Geometridae). Genome 59, 671–684. doi: 10.1139/gen-2015-0197
Hebert, P. D., Cywinska, A., Ball, S. L., and deWaard, J. R. (2003a). Biological identifications through DNA barcodes. Proceedings of the Royal Society of London. Series B: Biological Sciences 270, 313–321. doi: 10.1098/rspb.2002.2218
Hebert, P. D., Ratnasingham, S., and deWaard, J. R. (2003b). Barcoding animal life: cytochrome c oxidase subunit 1 divergences among closely related species. Proc. R. Soc. Lond. Ser. B Biol. Sci. 270, S96–S99. doi: 10.1098/rsbl.2003.0025
Johnson, M., Zaretskaya, I., Raytselis, Y., Merezhuk, Y., McGinnis, S., and Madden, T. L. (2008). NCBI BLAST: a better web interface. Nucleic Acids Res. 36, W5–W9. doi: 10.1093/nar/gkn201
Jombart, T., Devillard, S., and Balloux, F. (2010). Discriminant analysis of principal components: a new method for the analysis of genetically structured populations. BMC Genet. 11:94. doi: 10.1186/1471-2156-11-94
Jörger, K. M., and Schrödl, M. (2013). How to describe a cryptic species? Practical challenges of molecular taxonomy. Front. Zool. 10:59. doi: 10.1186/1742-9994-10-59
Kress, W. J., García-Robledo, C., Uriarte, M., and Erickson, D. L. (2015). DNA barcodes for ecology, evolution, and conservation. Trends Ecol. Evol. 30, 25–35. doi: 10.1016/j.tree.2014.10.008
Leavitt, J. R., Hiatt, K. D., Whiting, M. F., and Song, H. (2013). Searching for the optimal data partition- ing strategy in mitochondrial phylogenomics: a phylogeny of Acridoidea (Insecta: Orthoptera: Caelifera) as a case study. Mol. Phylogenet. Evol. 67, 494–508. doi: 10.1016/j.ympev.2013.02.019
Little, D. P., and Stevenson, D. W. (2007). A comparison of algorithms for the identification of specimens using DNA barcodes: examples from gymnosperms. Cladistics 23, 1–21. doi: 10.1111/j.1096-0031.2006.00126.x
Matz, M. V., and Nielsen, R. (2005). A likelihood ratio test for species membership based on DNA sequence data. Philos. Trans. R. Soc. Lond. B Biol. Sci. 360, 1969–1974. doi: 10.1098/rstb.2005.1728
Meyer, C. P., and Paulay, G. (2005). DNA barcoding: error rates based on comprehensive sampling. PLoS Biol. 3:e422. doi: 10.1371/journal.pbio.0030422
Monaghan, M. T., Wild, R., Elliot, M., Fujisawa, T., Balke, M., Inward, D. J., et al. (2009). Accelerated species inventory on Madagascar using coalescent-based models of species delineation. Syst. Biol. 58, 298–311. doi: 10.1093/sysbio/syp027
Prendini, L. (2005). Comment on “Identifying spiders through DNA barcodes”. Can. J. Zool. 83, 498–504. doi: 10.1139/z05-025
Pritchard, J. K., Wen, W., and Falush, D. (2003). STRUCTURE. Documentation for Structure Software: Version 2. Available online at: http://pritch.bsd.uchicago.edu
Puillandre, N., Lambert, A., Brouillet, S., and Achaz, G. (2012). ABGD, Automatic Barcode Gap Discovery for primary species delimitation. Mol. Ecol. 21, 1864–1877. doi: 10.1111/j.1365-294X.2011.05239.x
Ratnasingham, S., and Hebert, P. D. (2013). A DNA-based registry for all animal species: the Barcode Index Number (BIN) system. PloS ONE 8:e66213. doi: 10.1371/journal.pone.0066213
Ratnasingham, S., and Hebert, P. D. N. (2007). BOLD: The Barcode of Life Data System (http://www.barcodinglife.org). Molecular ecology notes 7, 355–364. doi: 10.1111/j.1471-8286.2007.01678.x
Ronquist, F., and Huelsenbeck, J. P. (2003). Mrbayes 3: Bayesian phylogenetic inference under mixed models. Bioinformatics 19, 1572–1574. doi: 10.1093/bioinformatics/btg180
Rubinoff, D. (2006a). Utility of mitochondrial DNA barcodes in species conservation. Conserv. Biol. 20:1026–1033. doi: 10.1111/j.1523-1739.2006.00372.x
Rubinoff, D. (2006b). Barcodes, integrated. DNA barcoding evolves into the familiar. Conserv. Biol. 20, 1548–1549. doi: 10.1111/j.1523-1739.2006.00542.x
Santos, C. M. D., Amorim, D. S., Klassa, B., Fachin, D. A., Nihei, S. S., De Carvalho, C. J. B., et al. (2016). On typeless species and the perils of fast taxonomy. Syst. Entomol. 41, 511–515. doi: 10.1111/syen.12180
Sarkar, I. N., Planet, P. J., and Desalle, R. (2008). CAOS software for use in character-based DNA barcoding. Mol. Ecol. Resour. 8, 1256–1259. doi: 10.1111/j.1755-0998.2008.02235.x
Shokralla, S., Spall, J. L., Gibson, J. F., and Hajibabaei, M. (2012). Next-generation sequencing technologies for environmental DNA research. Mol. Ecol. 21, 1794–1805. doi: 10.1111/j.1365-294X.2012.05538.x
Sickel, W., Ankenbrand, M. J., Grimmer, G., Holzschuh, A., Härtel, S., Lanzen, J., et al. (2015). Increased efficiency in identifying mixed pollen samples by meta-barcoding with a dual-indexing approach. BMC Ecol. 15:20. doi: 10.1186/s12898-015-0051-y
Stoeckle, M. (2003). Taxonomy, DNA, and the bar code of life. Bioscience 53, 796–797. doi: 10.1641/0006-3568(2003)053[0796:TDATBC]2.0.CO;2
Wiemers, M., and Fiedler, K. (2007). Does the DNA barcoding gap exist?–a case study in blue butterflies (Lepidoptera: Lycaenidae). Front. Zool. 4:8. doi: 10.1186/1742-9994-4-8
Yang, Z., and Rannala, B. (2010). Bayesian species delimitation using multilocus sequence data. Proc. Natl Acad Sci. U.S.A. 107, 9264–9269. doi: 10.1073/pnas.0913022107
Zahiri, R., Lafontaine, J. D., Schmidt, B. C., deWaard, J. R., Zakharov, E. V., and Hebert, P. D. N. (2017). Probing planetary biodiversity with DNA barcodes: The Noctuoidea of North America. PLoS ONE 12:e0178548. doi: 10.1371/journal.pone.0178548
Keywords: DNA barcode, phylogenetics, diagnosis, species delimitation, specimen identification
Citation: DeSalle R and Goldstein P (2019) Review and Interpretation of Trends in DNA Barcoding. Front. Ecol. Evol. 7:302. doi: 10.3389/fevo.2019.00302
Received: 15 March 2019; Accepted: 26 July 2019;
Published: 10 September 2019.
Edited by:
David S. Thaler, Universität Basel, SwitzerlandReviewed by:
Mark Stoeckle, The Rockefeller University, United StatesRodney L. Honeycutt, Pepperdine University, United States
Copyright © 2019 DeSalle and Goldstein. This is an open-access article distributed under the terms of the Creative Commons Attribution License (CC BY). The use, distribution or reproduction in other forums is permitted, provided the original author(s) and the copyright owner(s) are credited and that the original publication in this journal is cited, in accordance with accepted academic practice. No use, distribution or reproduction is permitted which does not comply with these terms.
*Correspondence: Rob DeSalle, ZGVzYWxsZUBhbW5oLm9yZw==