- 1UFZ–Helmholtz Centre for Environmental Research, Leipzig, Germany
- 2School of Mathematics, Computer Science, Institute of Environmental Systems Research, Osnabrück University, Osnabrück, Germany
- 3iDiv–German Centre for Integrative Biodiversity Research, Leipzig, Germany
One major interest in soil systems ecology is to maintain ecosystem functions. As soil is exposed to disturbances of different spatial configurations, identifying disturbance characteristics that still allow for maintaining functions is crucial. In macro-ecology, the influence of fragmentation on ecosystems is continuously debated, especially in terms of extinction thresholds on the landscape scale. Whether this influence is positive or negative depends on the considered type of fragmentation: habitat fragmentation often promotes population extinction, whereas spatially fragmented disturbances reduce extinction probability in many cases. In this study, we make use of these concepts to analyze how spatial disturbance characteristics determine functional resilience on the microscale. We used the numerical model eColony considering bacterial growth, substrate consumption, and dispersal for analyzing the dynamic response of biodegradation as an exemplary important microbial ecosystem function to disturbance events. We systematically varied the frequency of the disturbance events, and the size and fragmentation of the disturbed area. We found that the influence of the disturbance size on functional recovery depends on the spatial fragmentation of the disturbance, indicating that to some extent disturbance size can be compensated for by the spatial configuration of the disturbed area. In general, biodegradation performance decreases as the disturbed area increases in size, and becomes more contiguous. However, if a disturbance is highly fragmented, an increase in disturbance size has no influence on biodegradation performance unless the disturbance is critically large. In this case, the functional performance decreases dramatically. Under recurrent disturbances, this critical disturbance size is shifted toward lower values depending on the disturbance frequency. Our results indicate the importance of spatial disturbance characteristics for functional resilience of soil microbial ecosystems. Critical values for disturbance size and degree of fragmentation emerge from an interplay between both characteristics. Consequently, these characteristics which are widely discussed on the landscape scale need to be equally considered on smaller scales when assessing functional resilience of soil ecosystems.
Introduction
Soil ecosystems are complex and exhibit a particularly high spatial heterogeneity, for instance due to soil particles of different sizes resulting in a specific pore size distribution (Bartoli et al., 1991; Paz Ferreiro and Vidal Vázquez, 2010). Disturbance events such as drought events or the release of toxic chemicals are often modulated by this soil texture. Moreover, this pore size network is in general not static but changes dynamically due to environmental and anthropogenic influences. In consequence, for determining the impact of disturbance events in soil ecosystems; we need to explicitly consider the spatial characteristics of such events.
This is especially important for understanding key factors that stabilize soil ecosystem functions. These functions underlie ecosystem services and thus determine several aspects of human well-being (Biggs et al., 2012). In the face of climate change, disturbance events rise in frequency, and intensity affecting ecosystems, and the contributing functions (IPCC, 2013; Mora et al., 2013; Seidl et al., 2017). Ecosystems are able to cope with such disturbances to a certain extent and maintain their functions. This ability is called “functional resilience” (Biggs et al., 2012), and can be further classified into “functional recovery” (i.e., the ability to recover a function after a disturbance), and “functional resistance” (i.e., the ability to stay essentially unchanged despite disturbances; Grimm and Wissel, 1997).
One important function of microbial ecosystems in soil is the bacterial degradation of organic substances. With this function, soil microbes contribute to nutrient cycling, and long-term carbon storage (Schmidt et al., 2011; Wiesmeier et al., 2019). Moreover, the ability to degrade organic contaminants in the face of increasing pollution of soil ecosystems is essential for soil quality as well as for all living organisms. The impact on plants, animals, and humans are drastic and long-lasting, as such pollutants have toxic effects and are often highly persistent (Harms and Bosma, 1997; Jeon and Madsen, 2013).
In this study, we transfer ideas and concepts from macro-ecology in a top-down approach to understand spatial dynamics in microscale soil systems. The importance of spatial structure on population dynamics is widely discussed in several studies and different fields of ecology (Fahrig, 2002; Haddad et al., 2015; e.g., Selwood et al., 2015). In landscape ecology, for instance, habitat fragmentation is known to be a key factor for population viability as highly fragmented habitats were shown to decrease population size, and increase extinction probability (Bascompte and Sole, 1996; Hanski and Ovaskainen, 2000; Heinz et al., 2005; Oliver et al., 2015). For example, Oliver et al. (2015) showed that reducing habitat fragmentation increases the persistence probability of drought-sensitive butterflies. This relationship can be explained by edge-effects: Largely connected habitats ensure that many individuals are placed in the core of habitat patches, where they are protected against predators, and disturbances. On the other hand, if habitats are highly fragmented, more individuals reside in the vicinity of patch boundaries, begin exposed to predators or disturbances and, thus, population viability decreases.
This situation, however, changes for spatial fragmentation of disturbances. Highly fragmented disturbances often decrease the population extinction probability compared to less fragmented disturbances (Johst and Drechsler, 2003; Kallimanis et al., 2005; Liao et al., 2015). For instance, Liao et al. (2015) identified spatial autocorrelation of recurrent disturbances as key for the population extinction probability of locally dispersing species. Using a spatially explicit model, they showed an increase in extinction probability with increasing spatial correlation of the disturbance. The more edges occur between disturbed and undisturbed areas, the faster surviving individuals can disperse into, and re-colonize the disturbed areas. If a disturbance occurs in a clustered way, it takes longer to re-colonize the areas in the core of the disturbance because the distance from the undisturbed area is longer. Thus, dispersal is a key process for recovery from spatially structured disturbances. Various studies have analyzed the importance of dispersal in disturbed environments and confirmed the relevance of this process for population recovery or biodiversity (Banitz et al., 2008; Liao et al., 2016; König et al., 2017).
In earlier studies, we already identified spatial processes (bacterial dispersal and substrate diffusion) as key for functional recovery after a single spatially structured disturbance (König et al., 2017) and showed that thresholds for functional collapses depend on disturbance frequency and fragmentation in microscale systems (König et al., 2018).
The aim of the present study is to understand the impact of disturbance size and fragmentation on the resilience of biodegradation. We used the spatially explicit model eColony (Banitz et al., 2011b; König et al., 2017) for simulating biodegradation dynamics in response to disturbance events varying in size, fragmentation, and frequency. We analyzed the functional recovery after single disturbances as well as the functional long-term resistance under recurrent disturbances, defined as the ability to maintain the level of biodegradation dynamics essentially unchanged despite recurrent disturbance events.
With our simulations, we derive (i) the combined effects of disturbance size and fragmentation on the functional resilience of soil microbial ecosystems, (ii) how disturbance frequency influences these effects, and (iii) possible indicators based on spatial metrics for predicting functional resilience.
Materials and Methods
Simulation Model
We used the spatially explicit ecological model eColony which simulates bacterial growth, dispersal and substrate consumption (representing biodegradation of organic compounds) as well as substrate supply and diffusion with a set of reaction-diffusion equations. It was developed by König et al. (2017) for assessing the effects of spatially distributed disturbance events on biodegradation dynamics. The model is an extension of an established earlier version (Banitz et al., 2011a,b) and is parameterized by laboratory experiments (Banitz et al., 2012). Here, it operates on a two dimensional domain of 128 ×128 mm with reflective boundaries consisting of rectangular habitats of 1 mm2 size each. The model equations are solved using a finite difference method (Kreft et al., 1998; Banitz et al., 2011a). The programming language used for implementation is Delphi.
Within each simulation step of 1 min the following processes are considered: substrate consumption by bacteria, bacterial growth, bacterial dispersal, substrate diffusion, and substrate input. An area-wide permanent substrate supply is implemented representing a gradual dissolution from a substrate pool (Keymer et al., 2006; Centler et al., 2011) where dissolved substrate may accumulate within a habitat up to a maximum substrate concentration Cs, max. Thus, substrate is refilled in each time step depending on the difference between maximum concentration Cs, max and current substrate concentration Cs, multiplied by the input rate parameter λ (cf. Equation 2).
Equations
The dynamics of bacterial growth and substrate degradation are described using the following reaction-diffusion equations:
where Cx and Cs are the concentrations of bacteria (gx mm−2) and substrate (gs mm−2), Dx and Ds are the diffusion coefficients of bacteria and substrate (mm2 h−1), q is the specific substrate uptake rate of bacteria (gs h−1), which is calculated according to with qmax as maximum specific uptake rate (gs h−1), and Ks as half-saturation constant (gs mm−2). YG is the growth yield coefficient (gx ), a is the specific maintenance rate (h−1), d is the specific dispersal rate, expressed as biomass decrease (h−1), and λ the substrate input rate parameter (h−1). Note that both Dx and d vary depending on substrate availability (Banitz et al., 2011a). An overview of used parameter values is given in Table 1.
Disturbance Scenarios
We calculated a spatially homogeneous undisturbed steady state of the bacterial biomass such that the incoming substrate exactly matches the maintenance and used this as a reference (Table 1). The substrate that the bacteria consume in this undisturbed reference state, is sufficient for survival, but not for reproduction or dispersal. Pulse disturbance events were then introduced as singular drastic shocks reducing bacterial biomass within a certain, randomly distributed disturbance area. In each disturbed habitat, the bacterial biomass is reduced by multiplying it with a factor of 10−9. Bacteria in the undisturbed area are not affected by the disturbance events. Adaptive processes in reaction to the disturbance were not considered.
For generating the random patterns of disturbed area, we used the midpoint displacement algorithm for creating fractal landscapes with fragmentation parameter H and proportion p (Saupe, 1988; With et al., 1997). We systematically varied the fragmentation parameter H between 0 and 1 in steps of 0.1 for representing a span between highly fragmented and not fragmented patterns, and we varied the proportion p between 0.1 and 0.9 in steps of 0.1 corresponding to a disturbed area of 10 to 90% of the simulation area which defines the disturbance size. Exemplary disturbance patterns are shown in Figure 1.
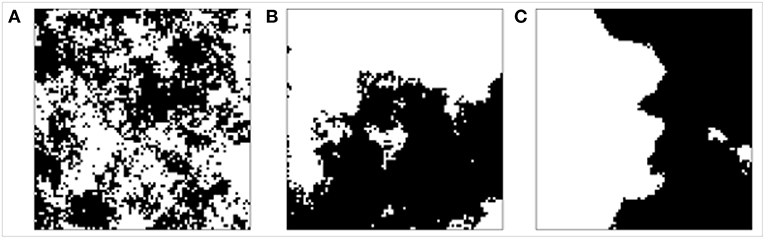
Figure 1. Examples of disturbance patterns with fragmentation parameter 0 (A), 0.5 (B), and 1 (C) and disturbance size of 50% (black: disturbed area, white: undisturbed area).
We considered two types of disturbance events: (i) single disturbance events and (ii) recurrent disturbance events. For single disturbance events, we performed 130 independent simulation runs for each combination of values for H and p over a simulation time of 500 h. Each run had a different random explicit spatial configuration of the disturbance event. For recurrent disturbance events, we performed 50 independent simulation runs for each combination of values for H and p with disturbance intervals of 10, 25, 50, and 100 h over a simulation time of 4,000 h. Each new disturbance event within one simulation run had a different random disturbance pattern. Thus, we generated for each run a distinct set of disturbance patterns with the same spatial characteristics of size p and fragmentation parameter H, but different explicit spatial realizations.
Analysis
We defined the recovery time after single disturbance events as the time needed for recovering 95% of the biodegradation performance of the undisturbed reference state. Further, we assessed the general influence of the spatial configuration of the different explicit patterns of single disturbance events on the recovery time. For this end, we calculated the mean distance between habitats in the disturbed and the respective nearest habitat in the undisturbed area Δ using
with DA the set of disturbed and UA the set of undisturbed habitats, |DA| the number of disturbed habitats, k and l Cartesian coordinates of undisturbed habitats, and i and j Cartesian coordinates of disturbed habitats.
For assessing the functional long-term resistance under recurrent disturbance events, we calculated the total amount of degraded substrate within a simulation time of 4,000 h, and compared this to the amount of substrate which is degraded in the undisturbed reference state during 4,000 h. Additionally, we defined a functional collapse when the biodegradation performance in all grid cells fell below a threshold of 5% of the biodegradation performance of the undisturbed reference state, and monitored the time span until this collapse occurred.
Results
Single Disturbance Events
After single disturbance events with disturbance size of 50%, the biodegradation performance recovers slower with decreasing degree of fragmentation of the disturbance pattern (Figure 2). However, to which extent the disturbance fragmentation has an influence on recovery depends on the size of the disturbance. If the area of the disturbance is very small (10%), functional recovery time is always short, independent of the disturbance fragmentation (Figure 3). However, already with a disturbance size of 20%, a clearly relevant influence of the degree of fragmentation is observable. The more clustered the disturbance pattern, the slower is the functional recovery. The relative impact is highest for disturbance sizes of 40%, where the recovery time is up to 80 times higher in less fragmented disturbance scenarios. If the disturbance area has a size of around 70 to 80%, an increase in fragmentation can speed up recovery by up to ~226 h, which is the highest observed absolute impact. If the disturbance size is very large (90%), the biodegradation performance recovers slowly in all cases, and the recovery time between scenarios with highest and lowest fragmentation differs only by a factor of 3. However, the system still needs up to 207 h longer to recover the pre-disturbance biodegradation performance with decreasing disturbance fragmentation.
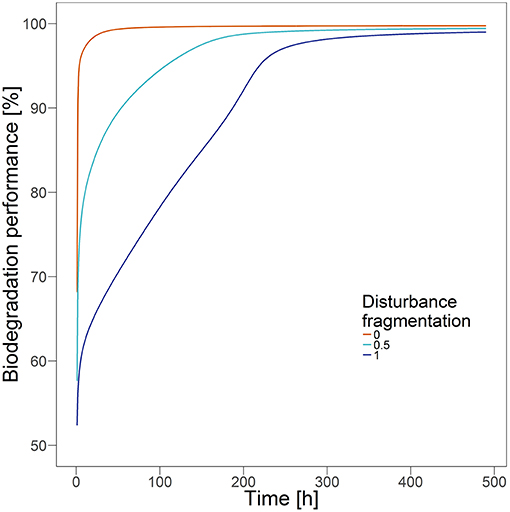
Figure 2. Biodegradation performance over time after single disturbance events with fragmentation parameter of 0, 0.5, and 1 and disturbance size of 50%.
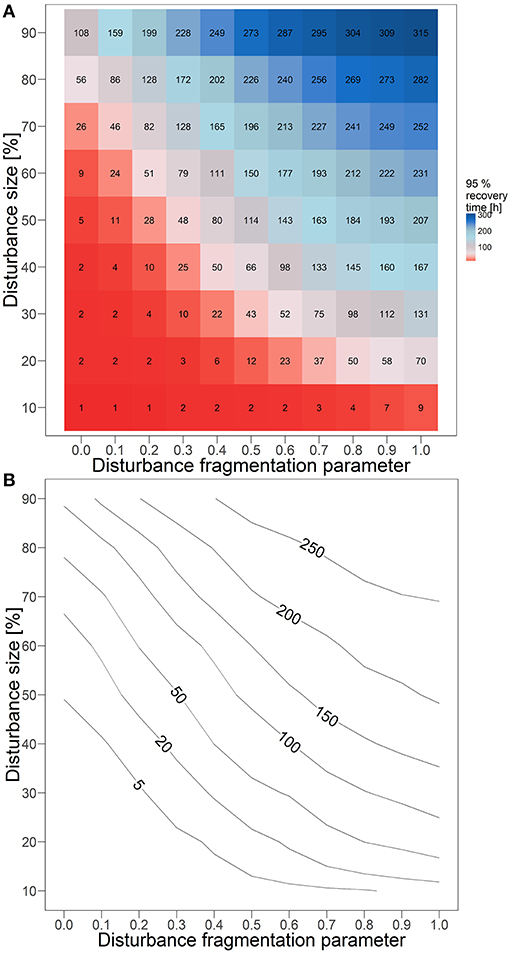
Figure 3. Time (in hours) for recovering 95% of biodegradation performance after disturbance events of different sizes and fragmentations presented (A) with boxes show mean value of 130 independent runs and (B) corresponding contour lines.
To some extent, a high fragmentation compensates for a large disturbance size. For instance, after highly fragmented, but very large disturbances (e.g., H = 0, p = 0.9), the mean recovery time (108 h) is similar to the mean recovery time of moderately fragmented, but much smaller disturbances (e.g., 114 h, in case of H = 0.5, p = 0.5). This comparable functional recovery time under high fragmentation is achieved although a larger area was disturbed, and thus more bacterial biomass was removed from the system in total. Moreover, if the disturbance is highly fragmented (H = 0), recovery is fast independently of the disturbance size, except for very large disturbances of 80 or 90%. However, the relative impact of the disturbance size on the recovery time in these scenarios is already high for 70% disturbed area, with a difference of 25 h compared to the recovery time after disturbances with 10% size. The compensatory relationship of disturbance size and fragmentation degree results in similar recovery times of the biodegradation over a wide range of different combinations of these disturbance characteristics (Figure 3B). For example, medium recovery times of around 100 h can be found in scenarios of almost all disturbance sizes (30–90%) when the degree of fragmentation is chosen accordingly.
The influence of the disturbance fragmentation is also observable in the relation between recovery time and mean distance Δ between disturbed and nearest undisturbed habitats (Figure 4). Generally, Δ is negatively correlated with the degree of fragmentation of the disturbance pattern. Therefore, the recovery time increases strongly with increasing mean distance until a certain threshold distance is exceeded. Beyond this threshold, the increasing trend of the recovery time gets weaker, and approaches a certain saturation level. For disturbance sizes of 10% we observed a different trend: at very short mean distances the functional recovery is very fast and the recovery time remains short for larger mean distances until a certain mean distance is reached beyond which recovery time starts to increase.
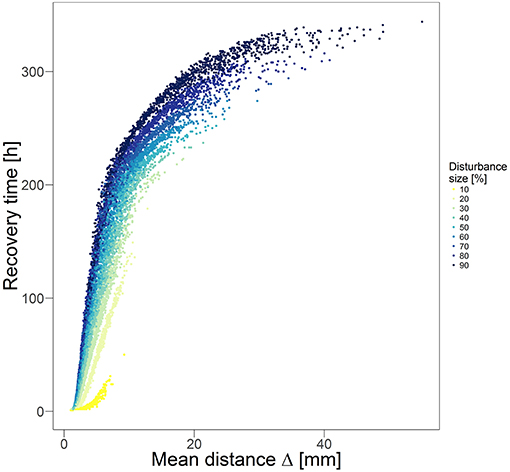
Figure 4. Time for recovering 95% of biodegradation performance after disturbance events of different sizes and fragmentations over respective mean distance Δ between disturbed and nearest undisturbed habitats. Each dot indicates recovery time of one independent simulation (12,870 simulations in total). Different colors indicate different disturbance sizes (cf. legend).
Recurrent Disturbance Events
Under recurrent disturbance events, we observed that the dependence of functional long-term resistance on disturbance size and fragmentation (Figure 5) follows a similar trend to how functional recovery after a single disturbance event depends on these disturbance characteristics (cf. Figure 3). In general, an increase in fragmentation and a decrease in size of the recurrent disturbances enhance functional resistance in terms of biodegradation performance. However, the relevance of both characteristics additionally depends on the disturbance frequency. Under short disturbance return intervals of 10 h, the disturbance size is most important. If the disturbance size is 50% or larger, a functional collapse typically occurs quite fast (Figure 5A, see also Table S1). If the disturbance size is smaller (30 or 40%), a collapse within 4,000 h can be prevented by a higher fragmentation. For very small disturbances (10 or 20%), the function does not collapse within the simulation time of 4,000 h. However, the long-term biodegradation performance under these disturbances reaches at most 80% of the undisturbed reference scenario for highly fragmented disturbance areas (H = 0) and decreases to much lower values as the fragmentation degree decreases (H = 1.0). With increasing disturbance return interval, the critical disturbance size causing a functional collapse shifts to larger values (Figures 5B–D). Moreover, the disturbance fragmentation becomes more important. For disturbance return intervals of 25 h, a high disturbance fragmentation hinders a functional collapse within 4,000 h even for disturbance sizes of up to 70% (Figure 5B). With disturbance sizes of 30% and lower, no functional collapses occur within 4,000 h independently of the degree of fragmentation. However, the long-term biodegradation performance is strongly correlated with the spatial disturbance fragmentation, with higher fragmentation enabling a better biodegradation performance. We even observed levels of biodegradation performance close to the undisturbed reference state for combinations of the smallest disturbance size and highest degree of fragmentation (lower left corner in Figure 5B). For disturbance return intervals of 50 h, a functional collapse gets again less likely (Figure 5C). Depending on the disturbance fragmentation, the biodegradation performance is quite high for disturbance sizes of up to 30%. Functional collapses occur rarely and only if the disturbance size is above 50%. For the highest spatial fragmentation of the disturbances, we even observed no functional collapses for all tested disturbance sizes, although the biodegradation performance level differs strongly between sizes. For disturbance return intervals of 100 h, functional collapses within 4,000 h occur only under disturbances with a size of 90% and low levels of fragmentation (Figure 5D). The long-term biodegradation performance shows a similar behavior as the recovery times for single disturbance events (cf. Figure 3) with the same interrelated dependence on both disturbance size and spatial fragmentation. Thus, biodegradation performance is quite high for combinations of low disturbance sizes and high spatial fragmentation. In scenarios with a disturbance size of 10%, the biodegradation performance is close to the undisturbed reference state and almost insensitive to the spatial disturbance fragmentation. The effect of the disturbance return time is also visible when comparing the contour lines of the subplots (Figures 5A–D). An increase in the return time causes a shift of the resistance measure “degraded substrate” to higher disturbance sizes, but increases also the relevance of the degree of fragmentation indicated by a higher slope of the contour lines in less frequent disturbance scenarios. For instance, with a return time of 10 h, a substrate degradation of 90% is reached only when the disturbance size is very small (10–20%) while with a return time of 100 h similar substrate degradation is observed for a broad range of disturbance sizes (30–80%) and all fragmentation degrees.
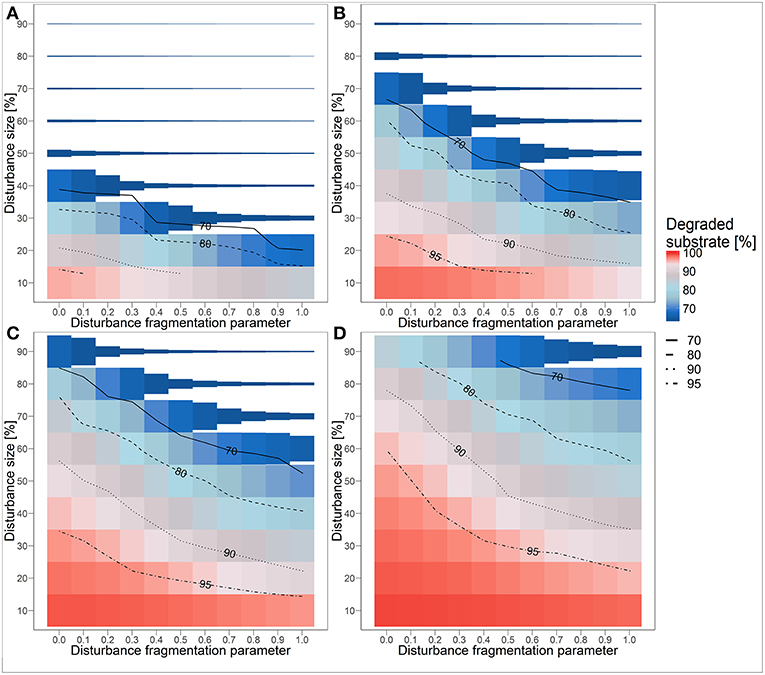
Figure 5. Functional resistance under recurrent disturbance events with a disturbance return interval of (A) 10 h, (B) 25 h, (C) 50 h, and (D) 100 h. Colors represent substrate degradation within 4,000 h of simulation time in relation to the undisturbed reference degradation performance (indicated by 100%). Height of boxes indicates time to collapse relative to maximum simulation time of 4,000 h.
Here, the mean distance Δ between disturbed and nearest undisturbed habitats once more serves as a proxy for describing the compensatory relationship of disturbance size and fragmentation degree (Figure 6). With increasing Δ, substrate degradation decreases in all scenarios, again, until a certain threshold is reached beyond which the performance does not further decrease. This threshold Δ depends on the return time of the disturbance, featuring smaller values for more frequent recurrent disturbances.
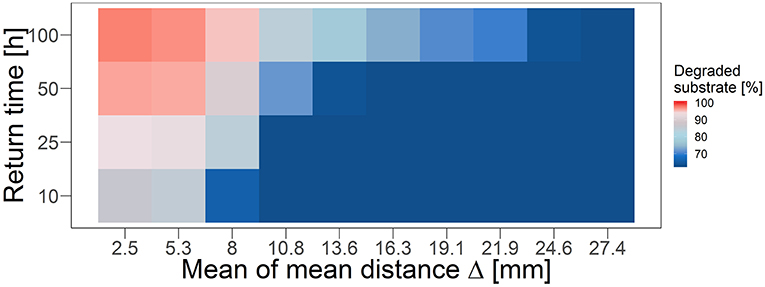
Figure 6. Functional resistance under recurrent disturbance events with different return intervals over mean of mean distance Δ of a number of disturbance patterns equivalent to the number of applied disturbance events with respective disturbance size and fragmentation. Colors represent substrate degradation within 4,000 h of simulation time in relation to the undisturbed reference degradation performance (indicated by 100%). Note that Δ is not normally distributed and thus the amount of data points underlying each box can differ.
Discussion
Our study indicates that functional resilience (recovery and long-term resistance) of microbial ecosystems in soil in terms of biodegradation performance depends on the spatial characteristics of the disturbance regime. It emerges from the interplay of size, spatial fragmentation and frequency of the disturbances. The mean distance between disturbed und undisturbed habitats turned out to be a critical factor for recovery dynamics. It depends on both disturbance size and degree of spatial fragmentation of the disturbance. The dependence on spatial distance indicates that functional recovery is mainly driven by recolonization of disturbed habitats from undisturbed habitats as opposed to re-growth in the disturbance affected habitats. A high spatial fragmentation of the disturbances leads to many interfaces and short mean distances between disturbed and undisturbed habitats. In consequence, bacterial dispersal from undisturbed to disturbed habitats is facilitated. Due to this recolonization, the bacteria can consume unused substrate that accumulate due to the removal of bacteria by the disturbance event, and biodegradation performance increases. This important role of the spatial process of bacterial dispersal for functional recovery has been highlighted and mechanistically explained before (König et al., 2017).
For larger disturbance sizes, the mean distance between disturbed and the nearest undisturbed habitat becomes longer as the disturbance's patch size increases and features more interior habitats far away from undisturbed habitats. This leads, in combination with more bacterial biomass being affected by larger disturbances, to an increase in recovery time. However, we found that a high disturbance size can to a considerable extent be compensated for by a high degree of spatial fragmentation of the disturbances (Figure 3). This indicates that the ability to recolonize disturbed areas by bacterial dispersal is as important for functional stability as the amount of affected bacterial biomass. This is in line with classical metapopulation theory showing that good dispersal conditions and connectivity alone cannot assure a high viability of the metapopulation. Additionally, a minimum size of the local subpopulations is needed to make sure that the number of dispersing individuals, and colonizers is sufficient (Frank and Wissel, 1998; Hanski and Ovaskainen, 2000; Frank, 2005).
For recurrent disturbances, a functional collapse can occur. If disturbed areas do not recover sufficiently between two disturbance events, the effects of recurrent, randomly distributed disturbances accumulate, and eventually the function deteriorates across the whole simulation area after some time. That the cumulative effect of recurrent disturbances can result in a functional collapse was also shown in an experimental study assessing how drying-rewetting cycle frequencies influence microbial activity (Ho et al., 2015). Similar to our findings, an increase in disturbance frequency increased the probability of functional collapse. In a microcosm experiment with cyanobacteria, Veraart et al. observed patterns of “critical slowing down” resulting in population collapse after several disturbances due to slower recovery with increasing disturbances (Veraart et al., 2011). Another experimental study also finds indications for cumulative effects, by assessing the recovery time of an aquatic microbial community with increasing number of disturbances (Garnier et al., 2017). Similarly, Jurburg et al. (2017) observed multiplicative effects of various temperature shocks on the recovery of soil microbial communities. In our study, such collapses become more likely with increasing disturbance size (Figure 5). However, they can be mitigated to a certain extent by a high spatial fragmentation of the disturbance, as then the function recovers faster between two disturbance events. The reason is the faster recolonization in such scenarios, which was also shown in experimental studies analyzing how recolonization from disturbed to undisturbed habitats can improve resistance under recurrent disturbances (Altermatt et al., 2011; Baho et al., 2012). Nonetheless, there is a critical disturbance size above which the functional resistance is low, and functional collapse is inevitable, independent of the disturbances' spatial fragmentation. It depends on the frequency of the recurrent disturbances and can be, again, described with a mean distance Δ combining the effects of size and fragmentation of the disturbance (cf. Figure 6). A low Δ allows for faster recovery between two disturbance events, but if the return interval between two disturbance events is not long enough for sufficient re-colonization, the system cannot further buffer the recurrent disturbance events. This threshold Δ decreases with increasing frequency and indicates the scenarios in which the disturbance size dominates functional resistance. In general, functional resistance under disturbances recurring with a return interval of 100 h is largely comparable to the functional recovery after single events (cf. Figures 3, 5D). Hence, we can qualitatively estimate the functional response to infrequent recurrent disturbance events based on the functional recovery behavior after single disturbances. However, as both depend on the specific spatial characteristics of the disturbances, it is most important to identify these characteristics for assessing how the function responds to recurrent disturbance events. More generally, our findings highlight the need to analyze resilience as a function of spatial structure. This corresponds to other recent attempts to quantify resilience of microbial ecosystems (Dai et al., 2013; Renslow et al., 2016). For instance, Renslow et al. (2016) tested recovery distance as spatial measure instead of recovery time. With population profiles, they showed that the recovery of a population depended on the distance to the disturbance at different time points during and after a disturbance event. In an earlier study, we used a similar approach by analyzing the dynamics of biodegradation after a disturbance within a spatial transect with increasing distance to the undisturbed area (König et al., 2017).
Our ecological model very generally formulates processes of dispersal and growth for simulating soil bacterial population dynamics and their response to disturbance events in a microscale system. It captures the complexity of natural soil microbial ecosystems in a simplified way. However, the spatially explicit simulations of bacterial growth, dispersal and substrate supply, diffusion and biodegradation under recurrent disturbances reflect processes that are highly important and widespread in these systems, and are also in line with comparable modeling approaches (e.g., Resat et al., 2012; Kaiser et al., 2014; Ebrahimi and Or, 2016; Vallespir Lowery and Ursell, 2019). Therefore, we consider our general findings qualitatively valid for natural systems. Their quantitative implications may considerably depend on context and additional factors. The observed sensitivity of biodegradation performance to the spatial characteristics of the disturbances may be especially relevant regarding different soil structures. In soil, the spatial structure in terms of pore size distribution influences the affected area of spatial disturbance types such as drought events, the release of toxic chemicals or salinity events. Thus, functional resilience to recurrent disturbances will likely vary with soil type. Rather homogeneous soils with well-mixed particles may thus lead to high fragmentation of disturbances and functional resilience. Soils with different particles aggregating in different areas, on the other hand, may form a pore network where disturbances occur in larger patches, resulting in lower functional resilience. This conclusion is underpinned by a recent review in which pore connectivity is identified as a key indicator for assessing soil functions (Rabot et al., 2018).
These implications on how spatial disturbance characteristics may alter functional resilience apply to disturbances affecting only part of the soil volume considered. For disturbance events affecting the whole system homogeneously, like temperature fluctuations, the specific soil structure may be less relevant.
To further understand the roles of the explicit environmental structure and the disturbance type for functional resilience of microbial ecosystems, more experimental studies are needed. One promising approach to evaluate structural effects in microbial systems is the use of microfluidics or micromodels, especially in combination with the increasing resolution of imaging techniques (Connell et al., 2013; Aleklett et al., 2018; Baveye et al., 2018; Toju et al., 2018). They allow for the observation of microbial dynamics in different spatially structured setups mimicking various soil pore structures, and also the application of disturbances like drought or heat events. Recent attempts in combining experimental microfluidics with computational modeling, for instance, to investigate spatial organization of bacterial colony growth (Wilmoth et al., 2018), or to predict chemotactic behavior (Brumley et al., 2019) show that this combination is especially promising for elucidating mechanisms behind microbial dynamics. However, it remains a challenge to analyze the resilience of microbial ecosystem functions in addition to their population dynamics, and how the two are related to each other. In our study, we tested widely discussed concepts from macro-ecology, and observed “edge-effects” caused by disturbance fragmentation, which have commonly been observed in various studies on different scales (e.g., Kanavillil et al., 2014; Oliver et al., 2015; Brinck et al., 2017). We show a positive effect of disturbance spatial fragmentation on functional stability: higher disturbance fragmentation improves both functional recovery and functional long-term resistance. Consequently, these edge-effects actually have positive consequences for functional stability as they facilitate re-colonization of disturbed areas. Different microbial studies indicate an enhancement of microbial community stability by spatial aggregation, for instance, due to higher species richness in the center of biofilms (Kanavillil et al., 2014), faster horizontal gene transfer (Madsen et al., 2012), cooperation between neighboring bacterial cells (Pande et al., 2015), or simply a higher cell density (Butler et al., 2010). We conclude that a larger disturbance area can be compensated for by a high disturbance fragmentation, which increases the activity of the surviving bacteria. A recent experimental study using microfluidics showed that spatially structured environments can increase population stability when exposed to antibiotic pulses, likely due to emerging metabolic gradients increasing metabolic interactions (Dal Co et al., 2019). Thus, fragmentation (of disturbances) is not necessarily negative but can even increase functional resilience in small scale systems. Therefore, a possible effective management strategy for enhancing functional resilience of soil ecosystems is to alter the soil pore size distribution by increasing its fragmentation affecting the possible disturbance area or stimulate microbial dispersal ability. In an earlier study, we tested variations in bacterial growth rate and dispersal ability under recurrent, but spatially invariant disturbances and showed that these bacterial properties affect functional resilience only under moderate, but not under extreme disturbance regimes (König et al., 2018). Therefore, we suppose that also in the given context of disturbance regimes differing in size, fragmentation and return time, combinations of intermediate values of these three disturbance characteristics respond most sensitive to of microbial properties. Under extreme disturbance regimes, functional resilience is likely to be less sensitive to these properties and more constrained by the disturbance characteristics. However, if disturbance effects are too harsh (i.e., too large in size and/or too frequent), there is probably no further benefit provided by increasing fragmentation or bacterial traits.
We conclude that one should be careful when postulating a direct relationship between certain disturbance characteristics and functional or structural attributes of an ecosystem, as they may be completely decoupled or even opposed to each other.
Finally, using spatial metrics that integrate more than one spatial characteristic (e.g., disturbance size and fragmentation), such as the mean distance of disturbed to the next undisturbed habitat, proofed to be helpful in predicting functional resilience in microscale systems (e.g., in soils).
Data Availability
The datasets generated for this study are available on request to the corresponding author.
Author Contributions
SK and TB developed the model. MK and A-LF designed the study supervised by SK and KF. MK, A-LF, and SK performed the simulations and analyzed the data. SK wrote the manuscript. TB, FC, and MT provided critical feedback and contributed to the preparation of the manuscript. All authors approved its submission.
Funding
This study was funded by the Helmholtz Association contributing to the integrated project Controlling Chemicals Fate (CCF) of the research topic Chemicals in the Environment (CITE). SK was funded by the German Federal Ministry of Education and Research in the project BonaRes (Module B): BonaRes Center for Soil Research (grant 031B0511). FC was funded by the German Federal Ministry of Education and Research (e:Bio project McBiogas, FKZ 031A317).
Conflict of Interest Statement
The authors declare that the research was conducted in the absence of any commercial or financial relationships that could be construed as a potential conflict of interest.
Supplementary Material
The Supplementary Material for this article can be found online at: https://www.frontiersin.org/articles/10.3389/fevo.2019.00290/full#supplementary-material
References
Aleklett, K., Kiers, E. T., Ohlsson, P., Shimizu, T. S., Caldas, V. E., and Hammer, E. C. (2018). Build your own soil: exploring microfluidics to create microbial habitat structures. ISME J. 12, 312–319. doi: 10.1038/ismej.2017.184
Altermatt, F., Bieger, A., Carrara, F., Rinaldo, A., and Holyoak, M. (2011). Effects of connectivity and recurrent local disturbances on community structure and population density in experimental metacommunities. PLoS ONE 6:e19525. doi: 10.1371/journal.pone.0019525
Baho, D. L., Peter, H., and Tranvik, L. J. (2012). Resistance and resilience of microbial communities–temporal and spatial insurance against perturbations. Environ. Microbiol. 14, 2283–2292. doi: 10.1111/j.1462-2920.2012.02754.x
Banitz, T., Fetzer, I., Johst, K., Wick, L. Y., Harms, H., and Frank, K. (2011a). Assessing biodegradation benefits from dispersal networks. Ecol. Model. 222, 2552–2560. doi: 10.1016/j.ecolmodel.2010.07.005
Banitz, T., Huth, A., Grimm, V., and Johst, K. (2008). Clumped versus scattered: how does the spatial correlation of disturbance events affect biodiversity? Theor. Ecol. 1, 231–240. doi: 10.1007/s12080-008-0023-3
Banitz, T., Johst, K., Wick, L. Y., Fetzer, I., Harms, H., and Frank, K. (2012). The relevance of conditional dispersal for bacterial colony growth and biodegradation. Microb. Ecol. 63, 339–347. doi: 10.1007/s00248-011-9927-3
Banitz, T., Wick, L. Y., Fetzer, I., Frank, K., Harms, H., and Johst, K. (2011b). Dispersal networks for enhancing bacterial degradation in heterogeneous environments. Environ. Pollut. 159, 2781–2788. doi: 10.1016/j.envpol.2011.05.008
Bartoli, F., Philippy, R., Doirisse, M., Niquet, S., and Dubuit, M. (1991). Structure and self-similarity in silty and sandy soils: the fractal approach. J. Soil Sci. 42, 167–185. doi: 10.1111/j.1365-2389.1991.tb00399.x
Bascompte, J., and Sole, R. V. (1996). Habitat fragmentation and extinction thresholds in spatially explicit models. J. Anim. Ecol. 65, 465–473. doi: 10.2307/5781
Baveye, P. C., Otten, W., Kravchenko, A., Balseiro-Romero, M., Beckers, É., Chalhoub, M., et al. (2018). Emergent properties of microbial activity in heterogeneous soil microenvironments: different research approaches are slowly converging, yet major challenges remain. Front. Microbiol. 9:1929. doi: 10.3389/fmicb.2018.01929
Biggs, R., Schlüter, M., Biggs, D., Bohensky, E. L., Burnsilver, S., Cundill, G., et al. (2012). Toward principles for enhancing the resilience of ecosystem services. Annu. Rev. Environ. Resour. 37, 421–448. doi: 10.1146/annurev-environ-051211-123836
Brinck, K., Fischer, R., Groeneveld, J., Lehmann, S., Dantas De Paula, M., Pütz, S., et al. (2017). High resolution analysis of tropical forest fragmentation and its impact on the global carbon cycle. Nat. Commun. 8:14855. doi: 10.1038/ncomms14855
Brumley, D. R., Carrara, F., Hein, A. M., Yawata, Y., Levin, S. A., and Stocker, R. (2019). Bacteria push the limits of chemotactic precision to navigate dynamic chemical gradients. Proc. Natl. Acad. Sci. U.S.A. 116, 10792–10797. doi: 10.1073/pnas.1816621116
Butler, M. T., Wang, Q., and Harshey, R. M. (2010). Cell density and mobility protect swarming bacteria against antibiotics. Proc. Natl. Acad. Sci. U.S.A. 107, 3776–3781. doi: 10.1073/pnas.0910934107
Centler, F., Fetzer, I., and Thullner, M. (2011). Modeling population patterns of chemotactic bacteria in homogeneous porous media. J. Theor. Biol. 287, 82–91. doi: 10.1016/j.jtbi.2011.07.024
Connell, J. L., Ritschdorff, E. T., Whiteley, M., and Shear, J. B. (2013). 3D printing of microscopic bacterial communities. Proc. Natl. Acad. Sci. U.S.A. 110, 18380–18385. doi: 10.1073/pnas.1309729110
Dai, L., Korolev, K. S., and Gore, J. (2013). Slower recovery in space before collapse of connected populations. Nature 496, 355–358. doi: 10.1038/nature12071
Dal Co, A., Van Vliet, S., and Ackermann, M. (2019). Emergent microscale gradients give rise to metabolic cross-feeding and antibiotic tolerance in clonal bacterial populations. bioRxiv [Preprint]. doi: 10.1101/534149.
Ebrahimi, A., and Or, D. (2016). Microbial community dynamics in soil aggregates shape biogeochemical gas fluxes from soil profiles - upscaling an aggregate biophysical model. Glob. Chang. Biol. 22, 3141–3156. doi: 10.1111/gcb.13345
Fahrig, L. (2002). Effect of habitat fragmentation on the extinction threshold: a synthesis. Ecol. Appl. 12, 346–353. doi: 10.1890/1051-0761(2002)012[0346:EOHFOT]2.0.CO;2
Frank, K. (2005). Metapopulation persistence in heterogeneous landscapes: lessons about the effect of stochasticity. Am. Nat. 165, 374–388. doi: 10.1086/428293
Frank, K., and Wissel, C. (1998). Spatial aspects of metapopulation survival – from model results to rules of thumb for landscape management. Landsc. Ecol. 13, 363–379. doi: 10.1023/A:1008054906030
Garnier, A., Pennekamp, F., Lemoine, M., and Petchey, O. L. (2017). Temporal scale dependent interactions between multiple environmental disturbances in microcosm ecosystems. Glob. Chang. Biol. 23, 5237–5248. doi: 10.1111/gcb.13786
Grimm, V., and Wissel, C. (1997). Babel, or the ecological stability discussions: an inventory and analysis of terminology and a guide for avoiding confusion. Oecologia 109, 323–334. doi: 10.1007/s004420050090
Haddad, N. M., Brudvig, L. A., Clobert, J., Davies, K. F., Gonzalez, A., Holt, R. D., et al. (2015). Habitat fragmentation and its lasting impact on Earth's ecosystems. Sci. Adv. 1:e1500052. doi: 10.1126/sciadv.1500052
Hanski, I., and Ovaskainen, O. (2000). The metapopulation capacity of a fragmented landscape. Nature 404, 755–758. doi: 10.1038/35008063
Harms, H., and Bosma, T. N. P. (1997). Mass transfer limitation of microbial growth and pollutant degradation. J. Ind. Microbiol. Biotechnol. 18, 97–105. doi: 10.1038/sj.jim.2900259
Heinz, S., Conradt, L., Wissel, C., and Frank, A. K. (2005). Dispersal behaviour in fragmented landscapes: deriving a practical formula for patch accessibility. Landsc. Ecol. 20, 83–99. doi: 10.1007/s10980-004-0678-0
Ho, A., Van Den Brink, E., Reim, A., Krause, S. M., and Bodelier, P. L. (2015). Recurrence and frequency of disturbance have cumulative effect on methanotrophic activity, abundance, and community structure. Front. Microbiol. 6:1493. doi: 10.3389/fmicb.2015.01493
IPCC (2013). "Climate change 2013: the physical science basis,” in Contribution of Working Group I to the Fifth Assessment Report of the Intergovernmental Panel on Climate Change, eds T. F. Stocker, D. Qin, G.-K. Plattner, M. Tignor, S.K. Allen, J. Boschung, A. Nauels, Y. Xia, V. Bex, and P. M. Midgley (Cambridge; New York, NY: IPCC).
Jeon, C. O., and Madsen, E. L. (2013). In situ microbial metabolism of aromatic-hydrocarbon environmental pollutants. Curr. Opin. Biotechnol. 24, 474–481. doi: 10.1016/j.copbio.2012.09.001
Johst, K., and Drechsler, M. (2003). Are spatially correlated or uncorrelated disturbance regimes better for the survival of species? Oikos 103, 449–456. doi: 10.1034/j.1600-0706.2003.12770.x
Jurburg, S. D., Nunes, I., Brejnrod, A., Jacquiod, S., Priemé, A., Sørensen, S. J., et al. (2017). Legacy effects on the recovery of soil bacterial communities from extreme temperature perturbation. Front. Microbiol. 8:1832. doi: 10.3389/fmicb.2017.01832
Kaiser, C., Franklin, O., Dieckmann, U., and Richter, A. (2014). Microbial community dynamics alleviate stoichiometric constraints during litter decay. Ecol. Lett. 17, 680–690. doi: 10.1111/ele.12269
Kallimanis, A. S., Kunin, W. E., Halley, J. M., and Sgardelis, S. P. (2005). Metapopulation extinction risk under spatially autocorrelated disturbance. Conserv. Biol. 19, 534–546. doi: 10.1111/j.1523-1739.2005.00418.x
Kanavillil, N., Balika, D., and Kurissery, S. (2014). Edge effect: a catalyst of spatial heterogeneity in natural biofilms. Hydrobiologia 744, 77–90. doi: 10.1007/s10750-014-2058-3
Keymer, J. E., Galajda, P., Muldoon, C., Park, S., and Austin, R. H. (2006). Bacterial metapopulations in nanofabricated landscapes. Proc. Natl. Acad. Sci. U.S.A. 103, 17290–17295. doi: 10.1073/pnas.0607971103
König, S., Worrich, A., Banitz, T., Centler, F., Harms, H., Kästner, M., et al. (2018). Spatiotemporal disturbance characteristics determine functional stability and collapse risk of simulated microbial ecosystems. Sci. Rep. 8:9488. doi: 10.1038/s41598-018-27785-4
König, S., Worrich, A., Centler, F., Wick, L. Y., Miltner, A., Kästner, M., et al. (2017). Modelling functional resilience of microbial ecosystems: analysis of governing processes. Environ. Model. Softw. 89, 31–39. doi: 10.1016/j.envsoft.2016.11.025
Kreft, J.-U., Booth, G., and Wimpenny, J. W. (1998). BacSim, a simulator for individual-based modelling of bacterial colony growth. Microbiology 144, 3275–3287. doi: 10.1099/00221287-144-12-3275
Liao, J., Ying, Z., Hiebeler, D. E., Wang, Y., Takada, T., and Nijs, I. (2015). Species extinction thresholds in the face of spatially correlated periodic disturbance. Sci. Rep. 5:15455. doi: 10.1038/srep15455
Liao, J., Ying, Z., Woolnough, D. A., Miller, A. D., Li, Z., and Nijs, I. (2016). Coexistence of species with different dispersal across landscapes: a critical role of spatial correlation in disturbance. Proc. Biol. Sci. 283:20160537. doi: 10.1098/rspb.2016.0537
Madsen, J. S., Burmølle, M., Hansen, L. H., and Sørensen, S. J. (2012). The interconnection between biofilm formation and horizontal gene transfer. FEMS Immunol. Med. Microbiol. 65, 183–195. doi: 10.1111/j.1574-695X.2012.00960.x
Mora, C., Frazier, A. G., Longman, R. J., Dacks, R. S., Walton, M. M., Tong, E. J., et al. (2013). The projected timing of climate departure from recent variability. Nature 502, 183–187. doi: 10.1038/nature12540
Oliver, T. H., Marshall, H. H., Morecroft, M. D., Brereton, T., Prudhomme, C., and Huntingford, C. (2015). Interacting effects of climate change and habitat fragmentation on drought-sensitive butterflies. Nat. Clim. Change 5, 941–945. doi: 10.1038/nclimate2746
Pande, S., Shitut, S., Freund, L., Westermann, M., Bertels, F., Colesie, C., et al. (2015). Metabolic cross-feeding via intercellular nanotubes among bacteria. Nat. Commun. 6:6238. doi: 10.1038/ncomms7238
Paz Ferreiro, J., and Vidal Vázquez, E. (2010). Multifractal analysis of Hg pore size distributions in soils with contrasting structural stability. Geoderma 160, 64–73. doi: 10.1016/j.geoderma.2009.11.019
Rabot, E., Wiesmeier, M., Schlüter, S., and Vogel, H. J. (2018). Soil structure as an indicator of soil functions: a review. Geoderma 314, 122–137. doi: 10.1016/j.geoderma.2017.11.009
Renslow, R. S., Lindemann, S. R., and Song, H. S. (2016). A generalized spatial measure for resilience of microbial systems. Front. Microbiol. 7:443. doi: 10.3389/fmicb.2016.00443
Resat, H., Bailey, V., Mccue, L. A., and Konopka, A. (2012). Modeling microbial dynamics in heterogeneous environments: growth on soil carbon sources. Microb. Ecol. 63, 883–897. doi: 10.1007/s00248-011-9965-x
Saupe, D. (1988). “Algorithms for random fractals,” in The Science of Fractal Images, eds H.-O. Peitgen and D. Saupe (New York, NY: Springer).
Schmidt, M. W., Torn, M. S., Abiven, S., Dittmar, T., Guggenberger, G., Janssens, I. A., et al. (2011). Persistence of soil organic matter as an ecosystem property. Nature 478, 49–56. doi: 10.1038/nature10386
Seidl, R., Thom, D., Kautz, M., Martin-Benito, D., Peltoniemi, M., Vacchiano, G., et al. (2017). Forest disturbances under climate change. Nat. Clim. Chang. 7, 395–402. doi: 10.1038/nclimate3303
Selwood, K. E., Mcgeoch, M. A., and Nally, R. (2015). The effects of climate change and land-use change on demographic rates and population viability. Biol. Rev. 90, 837–853. doi: 10.1111/brv.12136
Toju, H., Peay, K. G., Yamamichi, M., Narisawa, K., Hiruma, K., Naito, K., et al. (2018). Core microbiomes for sustainable agroecosystems. Nat. Plants 4, 247–257. doi: 10.1038/s41477-018-0139-4
Vallespir Lowery, N., and Ursell, T. (2019). Structured environments fundamentally alter dynamics and stability of ecological communities. Proc. Natl. Acad. Sci. U.S.A. 116, 379–388. doi: 10.1073/pnas.1811887116
Veraart, A. J., Faassen, E. J., Dakos, V., Van Nes, E. H., Lürling, M., and Scheffer, M. (2011). Recovery rates reflect distance to a tipping point in a living system. Nature 481, 357–359. doi: 10.1038/nature10723
Wiesmeier, M., Urbanski, L., Hobley, E., Lang, B., Von Lützow, M., Marin-Spiotta, E., et al. (2019). Soil organic carbon storage as a key function of soils - a review of drivers and indicators at various scales. Geoderma 333, 149–162. doi: 10.1016/j.geoderma.2018.07.026
Wilmoth, J. L., Doak, P. W., Timm, A., Halsted, M., Anderson, J. D., Ginovart, M., et al. (2018). A Microfluidics and agent-based modeling framework for investigating spatial organization in bacterial colonies: the case of pseudomonas aeruginosa and H1-type VI secretion interactions. Front. Microbiol. 9:33. doi: 10.3389/fmicb.2018.00033
With, K. A., Gardner, R. H., and Turner, M. G. (1997). Landscape connectivity and population distributions in heterogeneous environments. Oikos 78, 151–169. doi: 10.2307/3545811
Worrich, A., König, S., Banitz, T., Centler, F., Frank, K., Thullner, M., et al. (2016). Bacterial dispersal promotes biodegradation in heterogeneous systems exposed to osmotic stress. Front. Microbiol. 7:1214. doi: 10.3389/fmicb.2016.01214
Keywords: heterogeneity, simulation models, biodegradation, stability, resilience, microscale
Citation: König S, Köhnke MC, Firle A-L, Banitz T, Centler F, Frank K and Thullner M (2019) Disturbance Size Can Be Compensated for by Spatial Fragmentation in Soil Microbial Ecosystems. Front. Ecol. Evol. 7:290. doi: 10.3389/fevo.2019.00290
Received: 30 April 2019; Accepted: 22 July 2019;
Published: 02 August 2019.
Edited by:
Ali Ebrahimi, Massachusetts Institute of Technology, United StatesReviewed by:
Jinyun Tang, Lawrence Berkeley National Laboratory, United StatesSilke Langenheder, Uppsala University, Sweden
Copyright © 2019 König, Köhnke, Firle, Banitz, Centler, Frank and Thullner. This is an open-access article distributed under the terms of the Creative Commons Attribution License (CC BY). The use, distribution or reproduction in other forums is permitted, provided the original author(s) and the copyright owner(s) are credited and that the original publication in this journal is cited, in accordance with accepted academic practice. No use, distribution or reproduction is permitted which does not comply with these terms.
*Correspondence: Sara König, c2FyYS5rb2VuaWcmI3gwMDA0MDt1ZnouZGU=