- 1Biodiversity Research Centre and Department of Zoology, University of British Columbia, Vancouver, BC, Canada
- 2Department of Aquatic Ecology, Eawag: Swiss Federal Institute of Aquatic Science and Technology, Dübendorf, Switzerland
- 3Department of Evolutionary Biology and Environmental Studies, University of Zurich, Zurich, Switzerland
- 4Department of Fish Ecology and Evolution, Eawag: Swiss Federal Institute of Aquatic Science and Technology, Kastanienbaum, Switzerland
- 5Aquatic Ecology and Evolution, Institute of Ecology and Evolution, University of Bern, Bern, Switzerland
- 6Department of Biology, Quebec Centre for Biodiversity Science, McGill University, Montreal, QC, Canada
The persistence of ecological systems in changing environments requires energy, materials, and information. Although the importance of information to ecological function has been widely recognized, the fundamental principles of ecological science as commonly expressed do not reflect this central role of information processing. We articulate five fundamental principles of ecology that integrate information with energy and material constraints across scales of organization in living systems. We show how these principles outline new theoretical and empirical research challenges, and offer one novel attempt to incorporate them in a theoretical model. To provide adequate background for the principles, we review major concepts and identify common themes and key differences in information theories spanning physics, biology and semiotics. We structured our review around a series of questions about the role information may play in ecological systems: (i) what is information? (ii) how is information related to uncertainty? (iii) what is information processing? (iv) does information processing link ecological systems across scales? We highlight two aspects of information that capture its dual roles: syntactic information defining the processes that encode, filter and process information stored in biological structure and semiotic information associated with structures and their context. We argue that the principles of information in living systems promote a unified approach to understanding living systems in terms of first principles of biology and physics, and promote much needed theoretical and empirical advances in ecological research to unify understanding across disciplines and scales.
Principles of Ecology, Revisited
Information is fundamental to life and living systems, from subcellular processes to the biosphere (Gatlin, 1972; Davies and Walker, 2016; Tkačik and Bialek, 2016). Information is contained in the improbable organization and configuration of flows of matter and energy arising from activities and interactions of assemblages of atoms, molecules, cells or organisms (MacArthur, 1955; Ulanowicz et al., 2009; Frank, 2012; Kempes et al., 2017). All these structures—their composition and configuration—can be described using information measures, but some structures also carry, or encode, information that is interpreted and processed (Patten, 1959; Odum, 1988; Ulanowicz et al., 2006; Harte, 2011; Kempes et al., 2017). Information processing affects population dynamics (Donaldson-Matasci et al., 2010; Battesti et al., 2015; Fronhofer et al., 2017; Gil et al., 2018) and evolutionary processes (Ulanowicz, 1997; Giraldeau and Caraco, 2000; Dall et al., 2005; Frank, 2008; Ulanowicz et al., 2009; Schmidt et al., 2010; Wagner, 2017), and is part of every aspect of ecological change and human interactions with rest of the biosphere (Munday et al., 2009; van der Sluijs et al., 2010; Rossi-Santos, 2015; Altermatt and Ebert, 2016; Goldstein and Kopin, 2017; Gordon et al., 2018). Therefore, ecological science has much to gain by incorporating the fundamentals of information theories and information processing to understand and describe our changing world (Wicken, 1987; Schneider and Kay, 1994; Holt, 2007; Farnsworth, 2013).
Despite evidence that information plays fundamental roles in ecological systems, information does not yet feature prominently in the ecological principles at the center of our textbooks and theories (Appendix 1). This omission is problematic and isolating for ecology from other scientific disciplines (Schneider and Kay, 1994). Advances in other realms of science including physics, molecular biology and astrobiology increasingly recognize information, energy and material as the joint pillars of living systems (Frank, 2012; Woods and Wilson, 2013; Davies and Walker, 2016; Walker et al., 2016; Kempes et al., 2017; Wagner, 2017). Ecology, however, tends to emphasize organisms as fundamental units, and traits, environmental conditions and contingencies as fundamental concepts (Appendix 1). In expressions of ecological principles, energy and materials play important roles in explaining resource supply or environmental conditions, and information is rarely mentioned. Information processing is included by acknowledging evolution as an ecological principle (Appendix 1), but evolution is only one of many examples of information processing in ecological systems.
The costs of omitting something as fundamental as information from our general, mainstream scientific models are great. For example, ecologists have failed to anticipate the effects of environmental change on population dynamics mediated by disruptions in information processing. Studies showing that environmental change (e.g., ocean acidification) alters organisms' abilities to sense their environment and to act appropriately on environmental information such as cues for the seasonal events they use to find food or mates (Munday et al., 2009; Martín and López, 2013; Gordon et al., 2018) are often presented as surprising. A greater acceptance of the role information flow plays in population dynamics might lead to ecological theory to accommodate these effects, and deepen our understanding of the ecological consequences of disruptions of information (Schneider and Kay, 1994; Gil et al., 2018).
Another blind spot in ecological understanding concerns the roles of information flow in networks and organization. Social information (Gil et al., 2018), social learning systems (Aplin et al., 2015) and other forms of information can be central to the organization and stability of symbioses (Davy et al., 2012), social groups (Flack et al., 2006), and other living systems. The stabilizing and organizing consequences of information networks (MacArthur, 1955; Jorgensen et al., 2000; Ulanowicz et al., 2009; Babikova et al., 2013; Sentis et al., 2015; Lee et al., 2016) would be missed from a perspective of ecology that exclusively focuses on energy and material relations among individual organisms, or the interaction between an organism and its environment. Human activities may destroy the integrity of information networks and their adaptive capacities before they are ever known to science. Finally, biodiversity is a form of information; it is information stored in genes, morphologies, traits and behaviors that reflect the ecological and evolutionary history of life on earth. Ecological science is still ill-equipped to fully understand the consequences of this information (biodiversity) loss for future information processing, and flows of energy and materials. While concepts relating biodiversity and ecosystem functions have been developed (Loreau), they have not yet been grounded in the more general relationships between information and energy flows, though similar efforts have been made in information theories (Schneider and Kay, 1994; Ulanowicz et al., 2009; Coscieme et al., 2013; Norton and Ulanowicz, 2017). Thus, though it is well accepted that biodiversity is being lost at an alarming rate on the planetary scale, we do not have first principles to guide our understanding of the consequences of this information crisis.
We aim to fill the gap between information theories and modern ecological thinking by articulating principles for ecological systems that are consistent with our understanding of the role played by information in the structure and function of living systems (Box 1). We seek principles that are consistent with broader scientific knowledge and might, with additional theoretical development, allow unification of ecological theories and concepts that share a conceptual foundation (Margalef, 1963; Schneider and Kay, 1994; Jorgensen et al., 2000; Scheiner and Willig, 2008; Patten et al., 2011; Marquet et al., 2014; Patten, 2014).
Box 1. Five principles that integrate information in ecological understanding.
Drawing upon multiple research themes with domains spanning physics, information theory, ecology, evolution and semiotics, we identified a set of principles that integrate information and information processing into our understanding of ecological systems. These principles rest on the success of many decades of scientific knowledge development, but also represent the first step from this point forward to a more unified understanding of ecological systems. This is not an exhaustive set of principles for ecology, we have not considered principles of energy and matter other than how they interact with information. The principles presented here address two main concepts: the fundamental nature of information and its dual relationship with thermodynamic entropy and uncertainty (Information is Fundamental to Living Systems), and the multiscale causes and consequences of information processing (How is Information a Dynamic Part of Living Systems?). We use the term living system to include any energy-matter-fluxing life form, or aggregate of life forms, so it includes the smallest living organism up to the entire biosphere. It does not assume a priori an organism-centered, perspective on ecological systems that is typical of modern Darwinian approaches, but our concept of living systems is also not incompatible with assuming organisms are the fundamental unit of living system. These principles are not themselves a framework or research guide, but rather the minimum set of statements about nature that are the basis for theory and knowledge gain. We illustrate how a canonical model of eco-evolutionary dynamics can be modified to be consistent with these 5 principles (Box 5). Development of theories founded on these principles should lead to the development of hypotheses for how ecological systems across scales of organization grow, change and persist.
Principle 1: Information is a fundamental feature of living systems, and therefore also of all ecological systems. Syntactic and semiotic information (Table 1) constitute the two fundamental forms of information, and each is essential to the structure and function of living systems, from molecular systems to the biosphere. Consequently, some information within ecological systems is directly related to energetics in terms of thermodynamic entropy (Uncertainty and Entropy), while other aspects of information define sign systems that interact with energetics to produce life processes (Figure 1A).
Principle 2: Syntactic and semiotic information interact in feedbacks, with energetic processes and material cycles, to influence structure, function and organization in ecological systems. Ecological systems use semiotic information to structure how they expend energy for work (e.g., growth, reproduction, and consumption). (Figure 1) ecological processes are partly responsible for syntactic information (nonrandom distribution of matter on the Earth's surface). For example, reproduction represents the replication and transmission of information as encoded genetically within and across generations. Reproduction requires energy and resources, which are allocated to somatic growth or reproductive effort by information processes within the organism or between the organism and its environment.
Principle 3: Information processing requires energy and materials, therefore supply of energy and materials and thermodynamic constraints can limit information processing. The infrastructure for storing, transmitting, receiving and using information requires energy and materials (Figure 2), consequently the supply and physical constraints on the efficiency of energy and material systems may affect how much and how quickly information may be processed. These constraints are expected to lead to evolution of information systems that balance energetic and material efficiency, stability and durability with information processing capacity and reliability.
Principle 4: Information processing allows components of living systems to measure the environment and their own state and to measure the relationship between their state and past and expected environments. Subsets of information processing systems (cells, organs, individuals, etc.) receive and use cues and signals in the context of their environment (Figure 2). Organisms use evolved information processing systems to relate measurements of their current environment to expectations of their future environment [anticipation sensu (Rosen, 1985)]. This measurement combined with processing permits goal directed agency in living systems.
Principle 5: Information processing systems are linked within and across scales of biological organization. Strong positive feedbacks in information processing can define or reinforce levels of organization—from a cell to an individual to symbioses all the way to an ecosystem and the biosphere (Figures 1, 2). Information stored at higher order levels of organization, such as social groups, communities or ecosystems, can be used by lower level systems, such as individual organisms and cells. In this way, information processing occurs across scales of space and time, and can create and maintain physical or energetic structures.
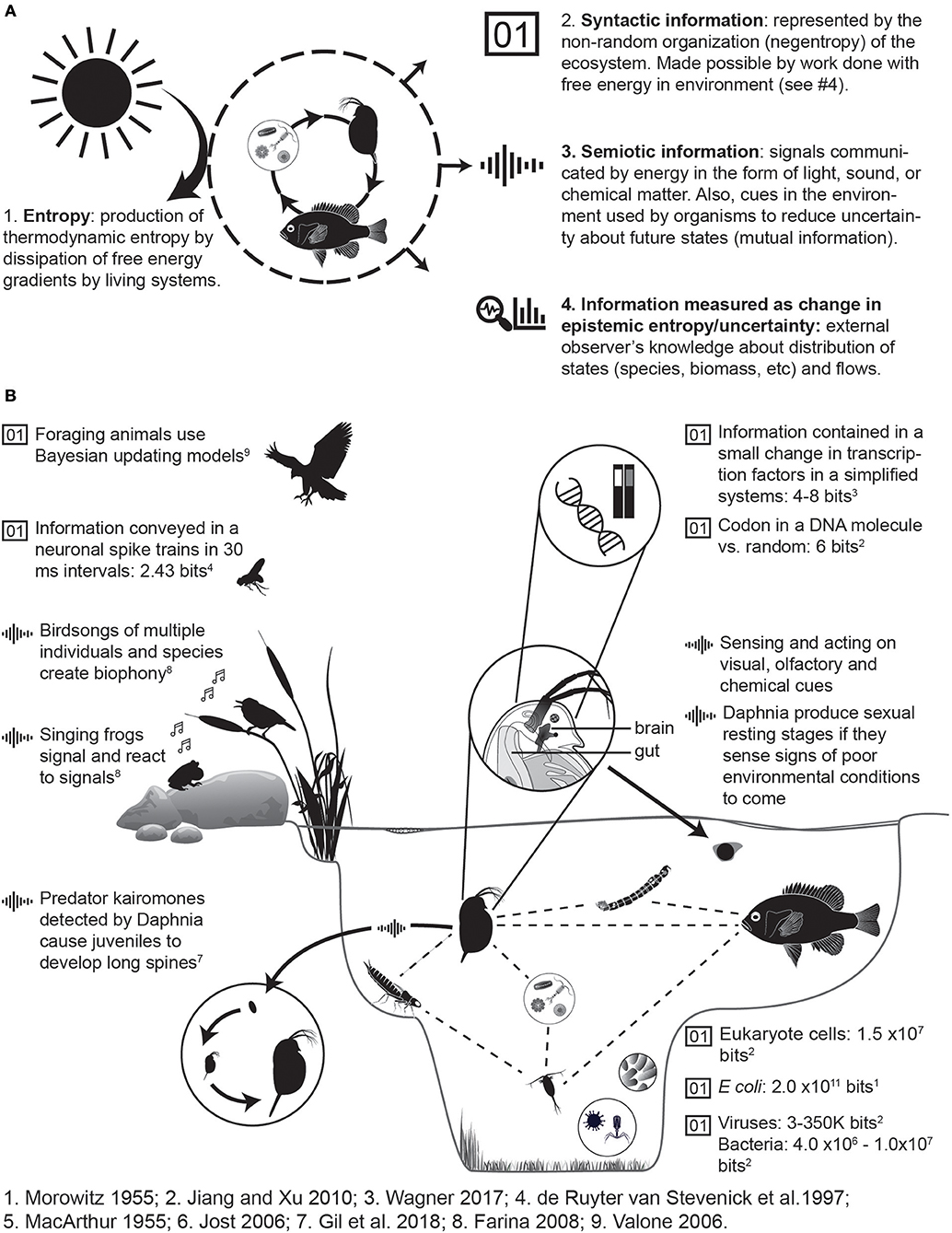
Figure 1. Information takes a variety of forms in ecological systems. (A) In an ecological system such as a simple aquatic food chain (center circle), information is present as latent information, semiotic information, and information change as states change. This living system dissipates energy, and therefore has entropy. (B) In an example aquatic system, information has been measured and reported at many levels of ecological scale, from transcription binding factors to food webs. Examples of syntactic information () contained in structures such as genes, cells, viruses, networks, and communities. Information is also contained in differences or changes in structure. Semiotic information (
), such as frog calls, kairomones from dragonfly larvae to daphnia, or use of cues and signals among organisms mediates ecological and evolutionary processes. Information can be measured using theory and equations in Box 4.
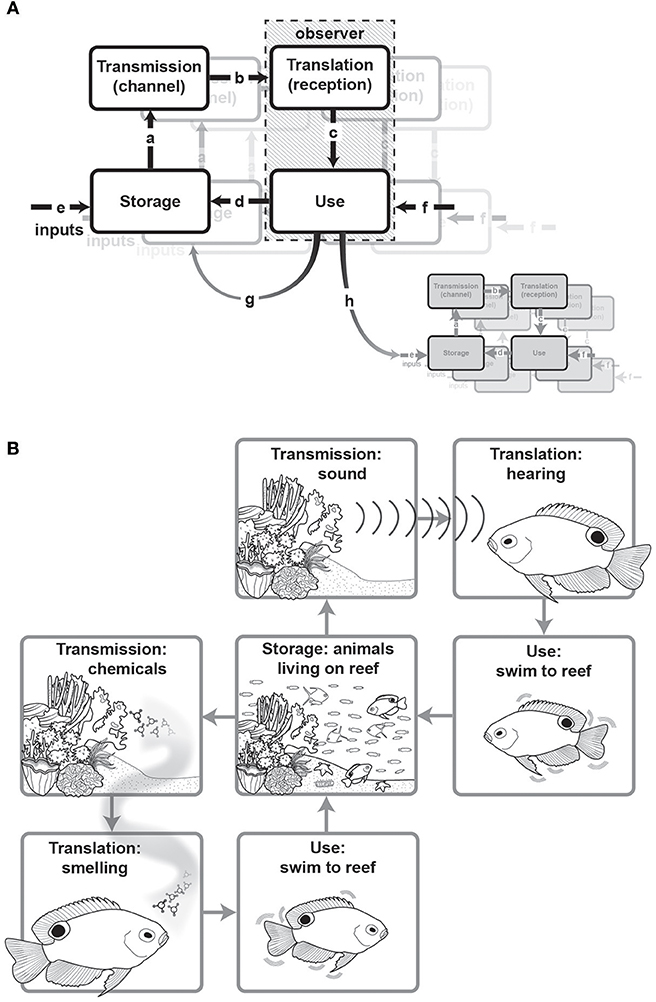
Figure 2. Four steps of information processing. (A) Information processing can occur within a closed feedback loop (a -> b -> c-> d), or by accepting information from outside the loop (e -> a-> b -> c…) and can lead to information that is used by the processing systems outside the focal system (e.g., c -> h or c->g). Information processing requires an observer, which often constitutes the reception and use of information. Inputs to any processing system may be information instantiated in chemical, electrical, energetic, or material structures. (B) Coral reef fish use olfactory (Osterhinchus doederleini) (Gerlach et al., 2007) and auditory (Gordon et al., 2018) information to relocate their home reef. The information stored in the sound or chemical patterns in the water are received by fish sensory systems—themselves information systems—and used to guide behavioral responses such as swimming to the reef and remaining there, and the recruitment of fish to these reefs contributes to structure at population and community levels.
Here, we review major concepts in the information theories that lead to these ecological principles. We structured our review around a series of questions about the role information may play in ecological systems: (i) what is information? (ii) how is information related to uncertainty? (iii) what is information processing? (iv) how does information processing link ecological systems across scales? Answers to these questions draw upon literatures as diverse as thermodynamics to cybernetics, statistics to evolution, behavioral ecology to semiotics (Boxes 2–4). As a consequence of seeking common ground and intellectual themes across such diverse literatures, we take care to define terms and introduce concepts that might be elemental in one literature but foreign to another (Table 1). We then consider briefly how ecological science might proceed to test, refine and build upon these principles This review and synthesis is intended to explain and justify our proposed set of fundamental principles for ecology (Box 1), and provide common conceptual ground for further scientific exploration of the role information plays in ecological systems.
Box 2. Timeline of selected milestones of information science.
Concepts of thermodynamic entropy and surprise emerged in the Twentieth century, setting the stage for later developments in concepts of entropy and information. But it was with Schroedinger's argument that information is central to life, followed closely by Shannon's insight that information can be considered as a quantifiable capacity for communication, that initiated a scientific revolution around information. Information has emerged in ecological thinking across scales since Shannon's work and we highlight some of these contributions to illustrate the breadth and progression of these concepts.
Box 3. Information theory and statistical inference.
For many ecologists and evolutionary biologists, the most common application of information theory is probably as a tool for statistical inference. The Akaike Information Criterion (AIC; Akaike, 1974), Bayesian Information Criteria (BIC; Schwarz, 1978), and related measures have become so familiar to ecologists as model selection procedures (Burnham and Anderson, 2002) that the philosophical and conceptual underpinnings are rarely given a second thought. For example, we use AIC to compare the relative performance of a candidate set of models, where we measure performance as the balance between fit (likelihood, L, of the data given the model) and the number of parameters k (AIC = −2 ln(L) + 2k). Rather remarkably, the AIC computed for a model is proportional to the amount of information lost—measured as the Kullbeck-Leibler divergence, DKL—in using a given statistical model p to approximate the true model that actually generated the data p*. For discrete distributions the divergence is equal to
Even though we do not know the true model, the AIC values from several candidate models can be compared. Similarly maximum entropy methods (Jaynes, 1957), which make use of information theoretic principles have been widely used to fit Species Distribution Models to location data (Phillips et al., 2006; Phillips and Dudik, 2008) and in the Maximum Entropy Theory of Ecology to estimate the parameters of macroecological distributions (Harte et al., 2008; Harte, 2011).
In one sense, the use of information theoretic principles in model selection and estimation is deeply related to the quantities we discuss throughout the manuscript: it is the structure inherent in ecological systems (i.e., their information content) that allows us to make inferences about the processes that might have generated the data we observe and predictions about that which we have not. On the other hand, such inference requires both an observer (i.e., us) and a theory about how the world works (i.e., a mathematical model) and in this article, we are concerned primarily with how information structures ecological systems, even when no one is looking.
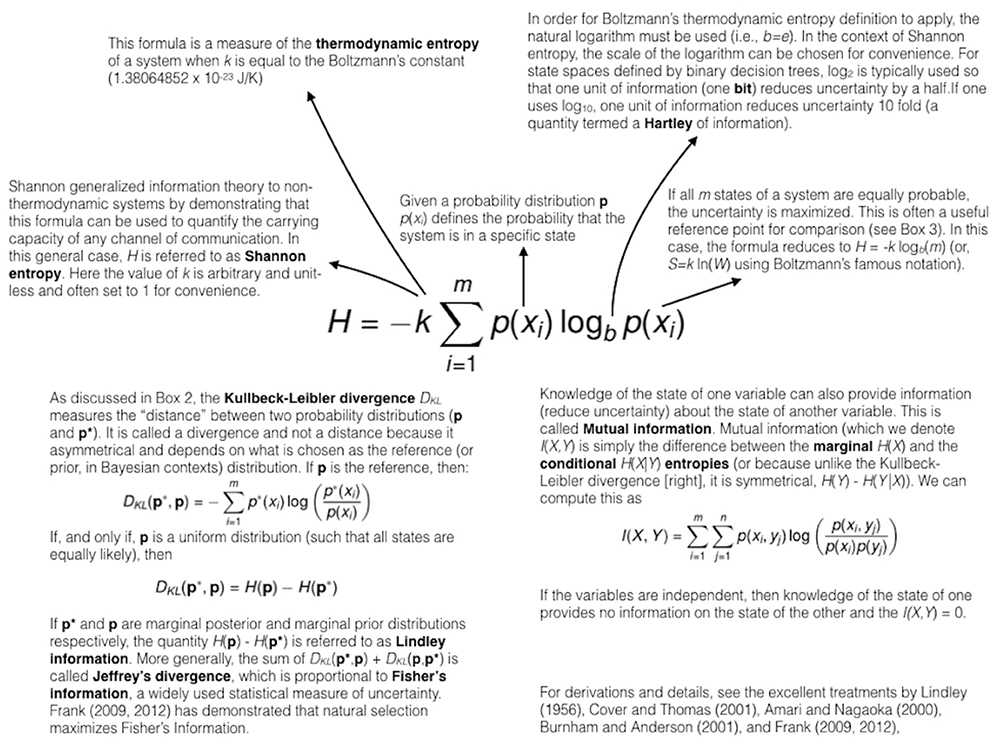
Box 4. Relationships between common information terms and concepts through a single information definition.
Information is Fundamental to Living Systems
What Is Information?
A basic definition of information is the difference between a set of realized events relative to the possible sets of those events (Table 1; Figure 1 and Box 3). Defining “what is possible” is not always easy, and entails a judgment by an observer. Sometimes “possible” is considered a random state, other times, it is considered a perfectly ordered state, or a known state (see Reference States for more explanation of reference states). The set of differences that constitutes information contains consequences of historical events that shaped the arrangement of elements in a living system. For example, the distribution, arrangement and structure of nucleic acids in a DNA molecule differs from a randomly assembled set (or any other arrangement) of the same nucleic acids. The difference between the arrangement of nucleic acids in the DNA strand and a random assemblage of the same set of nucleic acids reflects the recent history of those molecules and their translation within the ribosome, as well as the longer-term history of evolutionary processes that resulted in that particular allele's structure. We can also consider the information in the difference between two DNA molecules, identical except for a single nucleic acid. Again, the difference between the two DNA molecules reflects their shared (or different) histories of evolution and recent synthesis. But the consequence of the small differences for subsequent protein synthesis and biological function may be great. These DNA molecules, and their differences, contain syntactic information (Table 1). Syntactic information exists in any spatial or temporal arrangement of events or objects, including the species or functional diversity of a set of interacting species (Pielou, 1967; Jost, 2006), the notes and rhythms in a bird's song (Farina and Belgrano, 2006; Sánchez-García et al., 2017), or temporal pattern of sunrise and sunset (Edgar et al., 2012;Kinmonth-Schultz et al., 2013).
Information contained in structure, reflecting the structure's history, can (but does not need to) represent signs or symbols that convey meaning as interpreted by an observer (semantic information, Table 1). Semiotic information is the content and the quality of semantic information as it is carried by signs (Sebeok and Umiker-Sebeok, 1992; Kull, 1999; Dall et al., 2005; Barbieri, 2008; Schmidt et al., 2010). Semiotic information is central to interactions among molecules (proteins, enzymes, etc.), cells, physiological systems and organisms, and has long been recognized as important to ecological and evolutionary dynamics (von Uexkull, 1992). General sign theory was developed by Pierce (Atkin, 2006), and emphasized the triad of signs, objects and interpretants, highlighting how meaning in information requires not only an object and a sign that may represent it, but also an interpretation of that sign that associates the sign with the object (Atkin, 2006). Biosemiotic theories of ecology (Farina, 2008, 2011; Hoffmeyer, 2009) build on Peirce's triadic theory of signs (Atkin, 2006). The interpretant is often associated with an organism in which case this topic is the purview of the fields of behavioral ecology (e.g., evolution of signals and communication), chemical ecology and cognitive ecology. Biosemiotics, therefore, brings to ecology an understanding of information as signification and representation in ecological interactions, and its consequences are often considered in terms of fitness. For example, predators associate coloration with toxicity and avoid eating prey that exhibit these signs (Stevens and Ruxton, 2011). Another example is phenological cues associated with day length that many taxa use as signs of future favorable environmental conditions; these environmental cues have meaning because of regular variation in the environment (Helm et al., 2013).
Semiotic information plays an important role in ecological systems. In birdsong, the temporal structure of sounds carries information (and energy) imparted to it by the singing individual. The information may or may not be a reliable cue of its state; the bird's song also signifies semantic information to other birds that interpret the song. The information in a bird's song may even signify its fitness and an expectation of its own future—its likelihood of finding a mate or defending its territory, perhaps. The pattern of sounds in birdsong has meaning to other organisms, and that meaning depends on the receiver—e.g., the species or individual—and the context (Farina and Belgrano, 2006; Pijanowski et al., 2011). Even the aggregate biophony—the collective sound that vocalizing animals create in an environment—of a landscape's acoustic diversity presents a community-level semiotic context for the actions and ecology of any individual bird (Farina, 2008; Pijanowski et al., 2011) (Figure 1B). Semiotic information is important, even when signs are interpreted by biological systems that are not organisms. In the DNA example, the interpretant may be associated with a ribosome or protein, as the “observer” of the sign. The syntactic information of a gene is received by ribosomes' structure, and ribosomes encode and transcribe the information in a gene if the information matches what the ribosomes can interpret.
These two concepts—syntactic and semiotic—capture the dual nature of information (Figure 1). On the one hand, information is defined as signals sent and received by the individuals participating in the system. Their use of semiotic information drives ecological and evolutionary dynamics within the system (Patten, 1959; Gatlin, 1972; Giraldeau and Caraco, 2000; Ulanowicz et al., 2009; Gil et al., 2018) (Figure 1). On the other hand, information influences dynamics in ways not driven by individual organisms or mediated by communication; some forms of information stored in biological structures have energetic value (Parrondo et al., 2015), decay (Tkačik and Bialek, 2016), and constrain future possible states of a system (Shannon, 1948; Ulanowicz et al., 2009; Davies and Walker, 2016). The difference between a set of realized events relative to the possible sets of those events can be framed and measured in both senses we describe here. An ecological science that fully includes information will need to integrate syntactic and semiotic information (Bridging Syntactic and Semiotic Information; Box 1).
For many ecologists, the vast and varied literature on information, the related concepts of entropy, and their role in living systems is uncharted territory. This literature dates back at least a century in ecology and complex systems science (Box 2). It is a rich literature in which terms such as information and entropy are used with a plurality of definitions, meanings, and contexts (Wicken, 1987; Schneider and Kay, 1994; Gatenby and Frieden, 2006). To facilitate progress toward an ecological science that more effectively integrates information, we highlight some of the major concepts of information and their relationships to each other (Table 1). We present a brief review of thermodynamic entropy and information, and then proceed with greater emphasis on information theoretic concepts, though we highlight the continued need to bridge these gaps.
How Is Information Measured?
In ecology, as in any science, measurements are central to how we learn about our subjects, and how we visualize and operationalize theories. Measuring information and information flow in living systems is done in several ways (Figure 1). To understand which information measures or concepts are appropriate for a given context, and how metrics may or may not be comparable, we consider how information concepts are related to entropy, energy, cues and communication (Table 1).
Information can be measured in the context of information theory as the reduction in uncertainty of a system when comparing two (realized vs. possible) states of that system (Box 3, Figure 1, Table 1). We can measure information by comparing the entropy or uncertainty of an observed state relative to another state or states—a previous observation, a theoretical possible state (or set of states) such as a random state or thermodynamically dead or maximally entropic state (Box 4). In a thermodynamic context, information can be measured as negative entropy (Table 1), taking a value explicitly convertible or comparable to energy (Gatenby and Frieden, 2006; Schneider, 2010; Kempes et al., 2017). The unit of the measured information (bit, Hartley, etc.) depends on the configuration of the equation in Box 4, the reference states used or implied (Reference States), the base of the logarithm and the value of k. In the context of biosemiotics, information is measured in a different way, typically by comparing the results of the information for fitness. For example, syntactic information in the DNA and birdsong examples can be quantified using a Shannon index for allele diversity, or spectral analysis on bird song, and the semiotic information in these structures can be quantified in terms of the fitness of individuals who carry the allele or can act on the message in the song to find a fit mate (Figure 1). Measurements of information—by a scientist, or an organism or other participant in the ecological system—therefore involve assumptions or specifications about signs, observers and reference states that require consideration of fundamental aspects of the system, such as entropy (Erill, 2012).
Uncertainty and Entropy
Information is most generally considered to be a reduction in uncertainty. Uncertainty is sometimes used as a measure or synonym of entropy. There are two distinct uses of the term “entropy” in the context of information—one associated with information theory (likened to uncertainty), and another associated with thermodynamics (Table 1). Both entropy concepts can be expressed with the same equation (Box 3), though the terms and their relationships are interpreted differently depending on whether the context is thermodynamics or information theory (Table 1). Though the same word “entropy” is used for these two entropy concepts, they are actually distinct ideas and are not fully and directly interchangeable or convertible (Wicken, 1987).
Thermodynamic entropy
Living systems take energy and process it to produce more organized systems with lower entropy (Schrodinger, 1944; Schneider and Kay, 1994; Jorgensen et al., 2000) (Table 1). Understanding ecological systems in terms of thermodynamic entropy has played an important role in theories of ecosystem services, human-nature interactions, and systems ecology (Odum, 1988; Jorgensen et al., 2000; Coscieme et al., 2013). The reduction in entropy associated with processing energy has been related to information (Patten, 1959; Margalef, 1985). This form of information is explicitly related to the energy required to obtain or produce the information, in a famous solution to the physics problem of Maxwell's daemon (Wicken, 1987; Parrondo et al., 2015). The thermodynamic concept of entropy and information predates, and has been distinct from, the information theoretic concepts (Wicken, 1987) (Table 1). Work focused on understanding ecological systems in terms of thermodynamics of non-equilibrium (living) systems has moved away from using information in this context and instead emphasizes self-organization and entropy reduction (Wicken, 1987; Schneider and Kay, 1994) (Table 1).
Information theoretic entropy
Information has also been defined relative to a concept of entropy in information theory (Table 1, Box 4). This entropy is the information theoretic entropy introduced by Shannon when the parameter k = 1 (Box 4) (Shannon, 1948). The Shannon equation can be used to estimate information by calculating the entropy (H) of two states of a system (an observed state 1 and a reference state R), and taking the difference in entropies:
Uncertainty is maximized, and information is minimized, when the probability of the observed state of a system may be drawn from a uniform distribution of possibilities (one in which any state of the system is equally probable).
Though Shannon introduced his concept of syntactic information using the term entropy, and the use of the term entropy in this context does still persist (Jost, 2006; Sherwin et al., 2017; Gaggiotti et al., 2018), information theoretic entropy is not explicitly related to thermodynamic entropy (see Wicken, 1987 for an explanation of some of the core differences). Instead, information theoretic entropy is often related to the concept of equiprobability or disorder (without any explicit thermodynamic value to disorder). However, somewhat conversely to the trajectory of terminology in thermodynamics, the term entropy in information theory has in many cases been replaced by synonyms uncertainty (Dall et al., 2005; Gatenby and Frieden, 2006), indeterminacy (Ulanowicz et al., 2009), or complexity (Wicken, 1987).
Reference States
Information is a reduction in uncertainty between two states of the same system (Box 4). Central to this concept is the question, which two states are being compared? These two states may be defined in several ways. How they are defined influences how the observer interprets the information measured—how it may be related to energy, entropy and how it may be compared to other estimates of information.
There are absolute reference states. For a change in thermodynamic entropy, one reference state is thermodynamic zero (no entropy) (Jorgensen et al., 2000; Kempes et al., 2017). In early efforts, several researchers estimated the information content in cells based on the number of binary steps required to construct a biological structure from an unstructured arrangement of elements. They then verified these estimates using energetic methods, assuming that the information content of the structure was convertible to energy content via its inverse relationship to thermodynamic entropy. For example, Morowitz's estimate of 2 × 1011 bits in an Escherichia coli cell generally matched estimates based on calorimetric analyses (Morowitz, 1955). For non-thermodynamic entropy, maximum entropy is another absolute reference state. Full information may also be an absolute reference state. Full information is a known set of all possible states of a system and their probability of occurrence (Parrondo et al., 2015), but this one requires knowing the system. Pioneering attempts to integrate information theory into ecology drew on this idea of quantifying information by estimating all binary differences in a realized biological or ecosystem structure relative to all possible differences (Margalef, 1957, 1985; Patten, 1959). However, it was recognized relatively early on that knowing all possible states of an ecosystem was prohibitively difficult (Patten and Odum, 1981), and that thermodynamic zero is not always the most relevant reference state for comparing information between different life forms far from equilibrium. Approaches to estimate information in energetic terms had to make assumptions about these probabilities (Patten, 1959).
When observers (scientists, or participants in systems) define reference states other than absolute reference states, the accuracy of an absolute estimate of information depends on how well observers know (have a model) of the set of possible events and their relative probabilities—the set of possible nucleic acids, species, or letters in an alphabet. For entropy defined in terms of any value of k other than the Boltzmann constant in Equation 1 (Box 4), there is no explicit reference state to ground comparisons of states of a system. Any reference state can be specified by the recipient or user of the information (Boxes 3, 4). The reference state could be another known state of the system. For example, Jiang and Xu (2010) used the Shannon entropy index and data on genome size to estimate the information content of viruses and a variety of prokaryote and eukaryotic cells by estimating all the binary differences between their DNA structure (b = 2, Box 4), minus “junk DNA,” and the reference state of a completely disordered arrangement of base pairs but respecting the known probabilities of occurrence. They calculated information values ranging between 3 K and 340 K bits in viruses, 3.2 × 106 to 1.2 × 107 bits in bacteria taxa, and 1.5 × 107 to 8.4 × 108 bits in eukaryotes (Figure 1). Foraging animals use internally defined reference states based on their recent experiences of their environment and foraging activities when they use Bayesian updating processes to forage or choose mates in a dynamic environment (Dall et al., 2005; Valone, 2006). Erill (2012) argued that evolution acts as the informed observer for genetic information processes underlying evolution.
Examples of Information Measures in Ecological Studies
The earliest major effort to take an information theoretic perspective on ecological systems was Robert MacArthur's 1955 paper on diversity and stability (MacArthur, 1955). He introduced the Shannon entropy index to ecology to quantify uncertainty in how energy might flow through a community based on the number of possible energy flow pathways. In this example, MacArthur was exploring the idea that uncertainty is an attribute of the ecological system, in this case, a food web. Within a food web, he considered a probabilistic “indeterminacy” regarding the pathway in the network that energy might travel between two nodes. He used the Shannon index to model p(xi) (the central term, Box 4) as the proportion of energy flow through a particular node in the food web (not the proportion of individuals comprising that node, as we do today when we apply the Shannon index to describe diversity in ecological communities). His reference state was a uniform distribution (maximum uncertainty). The advantage of using the concept of entropy is that he did not need to know the exact energy flow pathways at any given time, or even which is most probable. He simply needed to know that the energy flow pathways are indeterminate; that there is an entropy of the network. Stability in a food web energy-flow network arises from indeterminacy in energy flow (Ulanowicz, 2001), or in other words, from uncertainty within the system about which pathway energy will flow through. This approach considers indeterminacy is an attribute of the network, not an attribute of an observer's (the scientist's) knowledge of the network.
Since this initial pioneering use of information theory to gain new insight in ecology, the Shannon index has been used extensively to estimate diversity in ecological systems (Pielou, 1967; Jost, 2006). The conventional definition of Shannon diversity (H') based on individuals of different species in a species assemblage is attributed to (Margalef, 1957, 1961; Ulanowicz, 2002). Estimates of diversity using Shannon or Simpson indices are examples of the use of an information theoretic measure of diversity, and these are applied to genetic systems or species assemblages (Jost, 2006; Sherwin et al., 2017; Gaggiotti et al., 2018). In fact, the development of metrics to measure diversity as information using entropy-based measures (Hill numbers) has played a key role in unifying understanding of diversity across ecological and evolutionary paradigms (Gaggiotti et al., 2018). Recent developments in the theory of using information metrics for diversity estimates of biological systems allow comparison of diversity across levels of an ecological system (e.g., genetic diversity and species diversity for a single species assemblage) (Gaggiotti et al., 2018).
Structural information has been an influential concept in community, ecosystem, and systems ecology (Patten, 1959; Margalef, 1985; Ulanowicz, 1997), in molecular biology (Gatenby and Frieden, 2006; Erill, 2012; Sherwin et al., 2017), and biodiversity science (MacArthur, 1955; Jost, 2006; Sherwin et al., 2017). For example, research on non-equilibrium thermodynamics has developed an understanding of ecosystem services, and even information in human societies (Odum, 1988) that is explicitly related to thermodynamic entropy and its related information (Jorgensen et al., 2000). Extensive work has employed structural information concepts and theory to understand macrostates in biological and ecological systems (Sole et al., 1996; Harte, 2011; Seoane and Solé, 2018).
Semiotic information is typically quantified in terms of the outcomes of situations in which living systems are exposed to sources of information that vary in their semantic content and context. These procedures are common in chemical ecology, where semiochemicals, such as pheromones and kairomones, can elicit many direct and indirect effects on development and survival within and among species. These effects can cascade up to higher levels of ecological organization. For example, predator kairomones can trigger cascading effects on the structure and functioning of aquatic food webs (Marino et al., 2015), or pheromones in insect pest outbreaks affecting primary production across large geographic areas. This information science has advanced to application with the development of databases of semiochemicals (http://www.pherobase.com/) for arthropod pest management, among other uses. Other approaches are used to assess the presence and importance of semiotic information as it mediates behavior, demography, and evolution (Dall et al., 2005; Schmidt et al., 2010). For example, the empirical studies of the role semiotic information plays in evolutionary or ecological processes have measured the consequences of information processing for the state of a receiving organism (Schmidt et al., 2010) such as its fitness at individual (Mery and Kawecki, 2005; Donaldson-Matasci et al., 2010) and population levels (Clobert et al., 2009; Fronhofer et al., 2017; Jacob et al., 2017).
Bridging Syntactic and Semiotic Information
Despite proliferation of information theory in several areas in ecology, an empirical synthesis of information stocks and flows is still elusive because different uses of information definitions and reference states makes it impossible to compare many estimates of entropy across systems. One issue is that the term p(xi) can take a variety of values that impart distinct meanings or interpretations on the resulting estimates of information. For example, when applying Shannon information to estimate the information contained in a genome or gene, the possible elements (nucleic acids) are finite and known (now), and their relative probabilities can be estimated (Jiang and Xu, 2010; Sherwin et al., 2017). This allows for the definition of a standard reference state for estimation of information in genetic systems for which these probabilities are already known. For a given species pool or sample, information theoretic methods now allow comparison and quantification of diversity across levels of biological organization (Gaggiotti et al., 2018). However, even with these advances, the values of these estimates do not constitute comparable estimates of information contained in biodiversity, in the sense of syntactic information that we are using here; a meta-analysis or synthetic assessment of information contained in would be un-interpretable diverse systems by comparing Shannon or Simpson measures. The reason for this incomparability is that entropy measured using the Shannon index depends on the estimate set of possible species, and this depends on the completeness of sampling or knowledge of the possibilities (e.g., species) in a system. Usually, this information is lacking; the choice of possible species and their relative probabilities is arbitrary because species pools are difficult to establish and are scale dependent (Jost, 2006; Gaggiotti et al., 2018). Approaches to estimating the full species pool are rarely reported or standardized. Thus, it is rarely possible to meaningfully compare reported values of Shannon diversity among different communities (Jost, 2006).
Bridging the gap between modes of studying and measuring syntactic and semiotic information also remains a major challenge (Adams, 2003). We still lack the ecological theory to relate the different measures and concepts of information to each other; we cannot track or account for information stocks and fluxes across studies or systems. For example, estimates of Shannon diversity using Hill numbers are not clearly comparable, in terms of information theories, to absolute references states that might allow a test of their relationship to energetics (Ulanowicz et al., 2009; Harte, 2011; Wagner, 2017; Hansen et al., 2018). The two entropy concepts that underlie thermodynamic and information theoretic definitions of information are not fully and directly interchangeable (Wicken, 1987). We should not view this gap to be a dead end; a similar situation occurs for energy, which also takes a variety of forms (e.g., radiant, thermal, chemical) that are difficult to quantify exactly and interconvert in living systems. Yet, we use energy and energetic models throughout ecological disciplines. As we have done with energetic models, we must explore relationships between different forms of information in nature. By attempting to understand the multiple information processes in ecological systems, we may overcome come of the challenges associated with diverse concepts and connect information use and its consequences in living systems.
Ecological systems integrate structural and syntactic information (Figure 1), just as they comprise multiple forms of energy and material. In fact, the way we understand ecological and evolutionary processes begins to bridge the gap between semiotic and syntactic information concepts. Two of the principles we propose for ecological knowledge explicitly recognize information of both kinds as fundamental to ecological systems (Box 1). We can continue to use thermodynamic, information theoretic and biosemiotic theories to gain insight about nature, and consistent with these principles (Box 4), do so in research that allows us to explore relationships between these information concepts. We believe such an approach may lead to deeper understanding of the extent and nature of relationships among the ways we observe and measure information in different contexts for different reasons. To help integrate information concepts with how we understand ecological systems, we can consider how information flows and accumulates in ecological systems. We refer to the flowing and accummulation of information as “information processing.”
How is Information a Dynamic Part of Living Systems?
What Is Information Processing?
Information is a dynamic feature of living systems because it is stored, transmitted, received, and used (Gatenby and Frieden, 2006; Erill, 2012) (Figure 2). In other words, living systems process information by combining semiotic and syntactic facets of information that we have reviewed so far (Gatlin, 1972; Maynard Smith, 2000; Farnsworth, 2013; Davies and Walker, 2016). Storage occurs in material or energy structures that reflect the events that created those structures. Structure stores information, thereby providing memory, about past events. Neural networks, social structures, morphologies, and learned behaviors are all examples of information storage, and therefore subject to general properties and constraints of information systems. Transmission occurs when stored information influences some informational, energetic or material process. Reception is the decoding and integration of the transmitted information in the receiving system or observer; reception of semiotic information involves a decoding process in which the state and previous information of the decoder is relevant. Reception is the physical receipt of information interacting with the state of the recipient. Use is the consequence in terms of what is done with the information received. Examples of information use include conversion of received information to stored information (e.g., observation to memory), and conversion of received information to work or function (observation to use) (Gatenby and Frieden, 2006) (refs in Figure 2). Transmission and reception are subjects of much of information theory and the literature on their role in communication and biology is vast (Dall et al., 2005; Chaine et al., 2013; Tkačik and Bialek, 2016). In this view, information transmission occurs through “channels,” and the information transmitted depends on the ability of the channel to transmit, and the receiver to receive, a signal relative to noise. Information transmission and reception can be modeled quantitatively using equations relating the probability of information received relative to what was possible based on a reference state (e.g., the properties of the transmitting channel, the expectation of the receiver, the context, etc.) (Figure 1).
When we consider information processing in living systems, we can quantify syntactic information stored, transmitted and received in objectively quantifiable units (Box 4). For example, the potential information stored in the action potentials that constitute neuronal communication (Tkačik and Bialek, 2016) (Figure 1) and the amount transmitted by neurons in flies (de Ruyter van Steveninck et al., 1997) has been estimated in bits, and even compared to the amount of information encoded by someone typing on a keyboard (Bergstrom and Rosvall, 2009). The amount of information associated with a particular ATP-binding phenotype is quantifiable (36.6 bits), and comparable to the amount of information needed for any single amino acid sequence [estimated at 345.8 bits; (Wagner, 2017)]. However, these quantitative measures of information processing fail to capture the content of information or the consequences of information processing, which are more often measured in terms of changes in the state or behavior of the user of the information (Figure 2).
Information processing systems confer general attributes and properties upon biological systems. These attributes include plasticity, memory, self-regulation, amplification, anticipation, cross-scale connections, and dependence upon energy and materials. The same information content can be stored in different structures and processed in different ways at different stages of an information processing system (Figure 2A). This property allows transfer of information among entirely different material or energetic systems, allowing the structure and materials of information processing systems to be plastic without necessarily compromising information flow. Information processing systems are typically characterized by indeterminacy in channels, and plasticity in the particular flows of information confer stability on the processing network (Patten and Odum, 1981; Flack et al., 2006; Moses et al., 2016), much as MacArthur showed in his idealized food web (MacArthur, 1955). Information processing systems can adapt to changing conditions, often reversing structural patterns in ecological networks (MacArthur, 1955; Ulanowicz, 2001; Flack et al., 2006; Ulanowicz et al., 2009; Valdovinos et al., 2016). For example, organismal processing systems such as visual or olfactory systems allow organisms to sense their environment, and based on a comparison of detected information with a reference state, act on that information (Figure 2).
Information processing systems relate past experiences and current conditions to anticipate likely future conditions so that the processing system (e.g., an organism or physiological system) can act accordingly (Rosen, 1985; Helm et al., 2013; Kinmonth-Schultz et al., 2013). Plasticity allows information processing systems to update, taking in new information from the environment or about the state of the processing system itself (Valone, 2006). For example, Fronhofer et al. (2017) and Jacob et al. (2017) have recently demonstrated experimentally that microbial organisms' abilities to sense their environment and select habitats where they are likely to perform well affects movement of individuals in ways that facilitates species' range expansion dynamics and local adaptation, thereby enhancing persistence in a changing environment. Many diverse information processing systems have evolved to allow organisms to perceive their environment and act upon that information. These evolved systems allow organisms or groups of organisms to use predictable environmental temporal patterns in day length or temperature to anticipate changing environmental conditions and to trigger developmental processes or other life history events. The genetic underpinnings—though diverse—of phenology and circadian rhythms constitute a memory of past successes associated with regularly occurring environmental conditions (Edgar et al., 2012; Kinmonth-Schultz et al., 2013). Another example of anticipation is the case when trees release and exchange infochemicals, often via mycorrhizal networks in the soil, and used by other individuals to infer, for example, the occurrence elsewhere of plant-insect interactions (especially pest insects) to change physiologically to minimize a likely future pest or disease attack (Barto et al., 2012).
The study of control and communication is the focus of the field of cybernetics. Self-regulation of a system's state through feedbacks is another attribute characteristic of the many information processing systems in ecology (Wiener, 1948; Patten and Odum, 1981; Farnsworth, 2013; Krakauer et al., 2014). For example, a self-regulating system is the physiological maintenance of thermal homeostasis in mammals (Woods and Wilson, 2013). Relevant to this review is the distinction between first- and second-order cybernetics as, respectively, the cybernetics of observed systems and the cybernetics of observing systems (von Foerster, 1974; Scott, 2004). Self-regulation in observing systems (e.g., organisms and their aggregates) requires information processing and an internal model of the world and of self to relate outputs to inputs and maintain feedbacks among sets of distinct steps; cybernetic systems also allow amplification of information as it is processed. Low or trace elements of chemicals (e.g., kairomones produced by predators) can elicit large behavioral, evolutionary and demographic responses in prey as that information is processed through physiological, neurological and genetic systems. Then, indirectly, population dynamics and even trophic cascades may be affected.
Information and the dynamics it elicits in ecological systems result in its inter-dependence on energy and material systems. The relationships between information and energy take a variety of forms. Information is carried by variations in the spectra of electromagnetic fields (e.g., light and magnetism) that are directly controlled by energy demanding organs of communicating organisms. Information processing requires energy and materials and is thereby subjected to physical and chemical constraints (Odum, 1988; Laughlin et al., 1998; Parrondo et al., 2015; Tkačik and Bialek, 2016; Kempes et al., 2017). Energy is required to create, maintain, and operate infrastructure to process information. This need for energy and materials has been considered as a “cost” of information. Information storage infrastructure, such as a brain or a gene, can be metabolically and materially expensive to build and maintain. The energetic and material requirements to maintain an information processing system may lead to changes in the network structure and its information processing over time, particularly as the external environment changes. Cybernetic models of information highlight relationships between information processing and system stability and energy fluxes in ways that are grounded in first principles of physics (O'Neill et al., 1986; Parrondo et al., 2015; Davies and Walker, 2016). In another approach, emphasizing semiotic information, behavioral ecologists have developed theoretical frameworks that relate use of private and social information (Danchin et al., 2004; Gil et al., 2018) to increased success in resource foraging and acquisition, suggesting that energetic and material costs of information processing systems may be balanced by resource gains. These examples illustrate how information-energy relationships have emerged as important in ecological studies; yet, as with other questions concerning information and energy, fully understanding how these different relationships fit together remains an important challenge for research, both in ecology and in physics.
Information processing systems occur within traditional levels of the ecological hierarchy (cells, organisms, populations), but also across levels, thereby linking ecological and evolutionary dynamics in what Koestler (1967) called a holarchy (Box 5). Holarchic systems are hierarchies where there is not a strict top-down flow of cause and effect; information flows up and down across levels mediating the dynamics of a system (Kay, 1999). The holarchic nature of information processing networks allows information to be stored and accessed at different levels of organization than the level at which it used. For example, genetic information in interaction with the environment may mediate phenotypic variation in physiology, communication, and species' interactions, with the emergent outcome that in turn affects patterns of selection and evolution. Eco-evolutionary processes are the direction expression of different modes of information flow in holarchic structured ecosystems.
Box 5. Eco-evolutionary model of information use processing.
Here we outline a model taking into account the fitness consequences of the information acquisition, storage, communication, and use. We first introduce the maximal information in an ecosystem accounting for the fitness of information traits of all phenotypes. In the second part of the box, we connect our maximal information criteria into a quantitative trait dynamics model. From first principles of thermodynamics an important reference point of interest is the maximum entropy, and around this point is a distribution of possible thermodynamic states (Table 1). For a living system defined by phenotypes with fitness, an analogously important reference point is that all phenotypes have equal frequency and equal fitness, and we assume this holds maximal information in the ecosystem. Around this point, there is a distribution of possible configurations of the ecosystem, with units of fitness. These distributions are intimately connected (Principle 2), but the former is abstracted in the following model derivation of information processing.
Information processing ecosystems are comprised of traits that influence a component of fitness variation, particularly those involved in information acquisition, storage, communication, and use (i.e., decision making) (Figure 2A). These we refer to as information processing traits, and as with other evolving traits, they are involved in tradeoffs among one another and with traits associated with energy (e.g., metabolic traits) and materials (e.g., resource acquisition traits) (Principle 3). The relationships among information traits, including the covariance matrix and the trait hierarchy taking into account processes across biological levels (Melián et al., 2018), define the occurrence and nature of these tradeoffs (Jablonka and Lamb, 2005). Information processing traits have cost functions. For example, acquiring and storing information about past environmental conditions and trait distributions could be adaptive during decision making (e.g., information usage), but there are likely significant energy costs associated with gathering social, habitat or species interactions information (e.g. movement across a landscape) and storing it for later use (e.g., metabolic costs of memory) (Giraldeau and Caraco, 2000).
Consider a population characterized by individuals each containing four information traits (acquisition, za, storage, zs, communication, zc, and use, zu), where the mean trait value of the information traits contained in each individual zi at time t in site x is given by:
where ca, cs, cc, and cu represent the information cost of acquisition, storage, communication and use, respectively, and T, Ψ, Ω, and Υ represent information from past environmental conditions, storage energy cost, the cost of gathering social and habitat information, and information usage cost, respectively.
In this example, perhaps the metabolic cost of memory increases exponentially with the total amount of information stored over the lifetime of an organism, including information acquired via movement through space and stored over time. These cost functions vary among traits, organisms types, individuals and development. Most individuals will use information from the most recent and spatially restricted state, with the variance depending on the cost of each information trait. In the canonical model of evolution, individuals use no information from previous states (i.e., strong costs), thus excluding adaptive strategies that involve information processing from prior states.
We consider the fitness function of each individual as the sum of information processing traits and other traits that underlie fitness variation. We can build a fitness functions based on these two components. For the non-information traits, fitness is often calculated from how well it matches a fitness optimum determined by the environment, specifically an environment that is determined by trade-offs associated with energy and material traits. For the information traits there is also an information environment, and we propose that its relationship between information processing traits, can also be a significant component of fitness variation (Principle 4). For example, individuals acquire information from their surroundings, and the canonical model does not incorporate how that information might be shared among individuals in a way that could influence both individual and population mean fitness. The adaptive use of information might build over time in a population and become a significant component of the fitness function (Principle 4). The fitness of individual i accounting for these two components can be expressed as:
where is the information trait value of individual i in time t and site x (Equation 1), is a measure of the optimal information content obtained from the population at time t, , is the non-information trait value of individual i in site x and θEx is the optimum determined by the environment. α and γ determine the interaction sensitivity to deviations from the information content optimum and from the environmental optimum, respectively. The effects of information and non-information trait correlations on fitness of each phenotype can be included by adding the covariance matrix with a multivariate fitness optimum (Melo and Marroig, 2015).
Modeling these two fitness components is a useful distinction and expansion of the canonical model. First, it adds more realism because information traits are now explicit in the evolutionary process alongside other evolving traits (Principle 1). Second, it allows us to contrast models of evolution with and without evolving information traits. This could also include models where the feedback between trait and the environment vary among traits. Information traits might have a more explicit feedback or feedforward relationship with the environment than other traits. Third, it is possible that information traits are evolving differently from other traits and experience different tradeoffs, such that modeling them separate from other traits might be a useful starting point. For example, information processing traits likely arise from different hierarchical levels of biological organization (Principle 5). In a plant- pollinator system, for example, the interactions in a typical evolutionary model do not incorporate the abilities of pollinator to acquire, access, and use prior foraging experience to make local decisions about which flowers to interact with. As such, there is no variation in the population with respect to decision making based on individual memory and learning potential (Watson et al., 2016). We posit that including such semiotic information into models of evolution will reveal new insights about natural populations, species interactions and the stability of ecological communities. The model outlined above can be run for many generations and for each time step, the phenotypes after selection and changes driven by structural modifications or plasticity in the information and the non-information traits can be used to update the fitness values (Melo and Marroig, 2015; DeLong and Gibert, 2016; de Andreazzi et al., 2018). Fitness will then determine the ecological dynamics and the total information content in the ecosystem. This total information content for each scenario can be compared with our reference point with all types having both equal frequency and equal fitness (i.e., maximal information in the system).
Information processing networks also have the capacity to perform computations at the local level that have far-reaching consequences at the macroscopic level. For example, insect societies are able to perform complex behaviors, including possibly computations, even though individual behaviors are simple, and these society-level behaviors produce and store information with emergence properties of long-range order (Solé et al., 2016). Though many apparent examples of information processing center on organisms and how they receive external signals and act on them, information processing also occurs within genetic, physiological, and neurological systems process information using analogous processing systems (Laughlin et al., 1998; Maynard Smith, 2000; Woods and Wilson, 2013; Tkačik and Bialek, 2016; Wagner, 2017) (Figure 2). Information is also processed at levels of organization above individuals (Frank, 2008) such as within social networks (Flack et al., 2006; Aplin et al., 2015), and even in non-Darwinian units such as communities (Gerlach et al., 2007; Farina, 2008; Gordon et al., 2018) and ecosystems (Patten, 1959; Odum, 1988) (Figure 1B). A cybernetic perspective, in particular a second-order perspective, challenges the heavily reductionist, mechanistic view of ecology because it demonstrates that flows of information among levels of organization mediate a living system's dynamics (Ulanowicz, 1997; Jablonka and Szathmary, 2004; Ulanowicz et al., 2006; Davies and Walker, 2016).
Are Organisms Causes or Consequences of Information Processing?
Taking a synthetic perspective on information in ecological systems reveals an additional duality: individual organisms are drivers of information processing, but organisms and other biological structures can be seen as emergent to the process of information processing (Davies and Walker, 2016). Much of mainstream ecological theory (e.g., Appendix 1) is predicated on the view that organisms are the fundamental agent of information processing and the consequences of information processing are behavioral, fitness, and population outcomes (Dall et al., 2005; Schmidt et al., 2010; Burns et al., 2011; Gil et al., 2018). However, another theme in how information theory has been used in biology, specifically to better understand the origin of life, suggests that organisms, like other levels of organization, may be emergent properties of information processing systems (Schrodinger, 1944; Schneider and Kay, 1994; Kay, 1999; Krakauer et al., 2014; Marshall et al., 2017). The information processing systems that allow organisms (or agents) to emerge and persist are not restricted to any particular biological scale. Therefore, there may be no reason to think that information processing and the stability and evolvability that comes with it are restricted to what we traditionally perceive as an “organism,” based on physical attributes of organisms. Indeed, recent discoveries on the holobiont (host-symbiont, host-parasite relationships) nature of the organism blurs our notions of individuality. There is substantial evidence for information processing to have cross-scale consequences and connections, and we will review these in the next section.
Together, the perspective that organisms are fundamental units in ecological systems, combined with the view that organisms are emergent properties of ecological information processing systems, suggests that there are feedbacks between information use and processing across scales of ecological organization (Ulanowicz, 1997). This dual relationship between information processing and the individual has been argued to underlie major evolutionary transitions from unicellular organisms to multicellular organisms as evolutionary units (Szathmáry and Smith, 2002; Jablonka and Szathmary, 2004; Jablonka and Lamb, 2005). In this way, information dynamics and the informational, material and energetic efficiency and stability they confer appear to have shifted the way evolutionary biology recognizes elements of living systems as “organisms” in recent decades. Some have extended the concepts of fundamental units of selection even further, based on information processing dynamics, to include kin, social groups, symbioses and even some species associations (Ehrlich and Raven, 1964; Szathmáry and Smith, 2002; Guimarães et al., 2011).
An information theory perspective suggests that Darwinian evolution is one fundamental form of information processing that produces structure and persistence in living systems (Gatlin, 1972; Maynard Smith, 2000; Frank, 2012; Wagner, 2017). But, evolution is not the only form of information processing prevalent or necessary for ecological systems—evolution at levels of organization other than the genetic population would be consistent with information processing, by cellular structure and contents, by mixed species communities and social systems, social learning, and thermodynamic information conversion to energy (Margalef, 1957; Wicken, 1987; Odum, 1988; Ulanowicz, 1997; Farnsworth, 2013; Kempes et al., 2017). From the perspective of life as non-equilibrium thermodynamic systems, ecosystems are considered holarchic systems that grow and accumulate information and organization over time (Schneider and Kay, 1994; Kay, 1999; Jorgensen et al., 2000); evolution is one mode of information accumulation, but not the only mode. This fact has profound implications for how we approach understanding ecology at this time because mainstream ecological theory is predominantly based on Darwinian models, assumptions and concepts, resulting in a strong emphasis in ecological theories on evolvable “Darwinian” units—individuals, symbioses, etc. (Szathmáry and Smith, 2002; Jablonka and Szathmary, 2004; Jablonka and Lamb, 2005). Taking the perspective that information and information processing is fundamental, and evolution is one manifestation of it, opens up additional perspectives on how living systems grow, change, and persist (Wicken, 1987; Ulanowicz, 1997; Frank, 2008; Farnsworth, 2013).
Advancing the Role of Information in Ecological Understanding: Challenges and Opportunities
We have outlined five fundamental principles for ecological science that integrate information and its role in living systems (Box 1). The five principles provide a foundation upon which new theories may be developed (or existing concepts and theories rooted). To build a theory for how ecological systems process information, conceptual and theoretical models need to be articulated (we offer one such approach in Box 5), and experiments will be needed to test their assumptions and derived hypotheses and predictions. Building this theory is a major challenge for ecological and evolutionary science. It will require synthesis of existing frameworks in molecular ecology and evolution, (semio)chemical ecology, behavioral ecology, physiology, systems ecology, and thermodynamics (to name a few). To inform this new direction, we can begin by drawing upon the rich theoretical and empirical literature on the role of information in evolution, behavioral ecology, and molecular biology (Dall et al., 2005; Ulanowicz et al., 2009; Schmidt et al., 2010; Farnsworth, 2013; Gil et al., 2018). We highlight two immediate challenges here.
Develop and Employ Empirical Approaches for Quantifying Information and Its Flow in Ecological Systems at Multiple Scales
The five principles capture the importance of information and its relationships with energy and materials. Yet, studying information empirically has been extremely difficult historically. Estimates of information content in cells, organisms, communities, and ecosystems were in the past severely constrained by infrastructure for measuring or observing structural information or behaviors associated with communication (Patten and Odum, 1981). Now, existing approaches allow information to be estimated in quantifiable and comparable terms (e.g., Jiang and Xu, 2010; Kempes et al., 2017) (Figure 1). Using physics concepts and models (Kempes et al., 2017), have recently estimated the thermodynamic information content of the biosphere based on estimates of the information content in cells. These approaches could be further developed and adapted to quantify information in communities and ecosystems, allowing tests of hypotheses related to principle 2. Other approaches we now have that were not available until recently were the computational and technological infrastructure to sample sounds, colors, and chemicals in the environment, and to analyze their possibilities and probabilities to then estimate information. Expanding the use of these approaches to generate estimates of information processing in living systems is an essential next step to understanding how information processing is driven by and changes energy and material processes as outlined by principles 3 and 4. These can be used to quantify information in ecological systems under different conditions and to further develop empirical knowledge of where and when information is processed.
An empirical, data-rich body of knowledge is essential to produce a more general and testable theory of ecology. Theory (Develop and Apply New Ecological Theory of Information) is also essential, because even though it is possible to assemble more and new kinds of data, we still face basic challenges comparing these measurements. In some ways the capacity to measure information (e.g., eDNA or remotely sensed observations) has outpaced our ecological theory for how to use these observations of information to understand ecological and evolutionary dynamics. For example, for a freshwater planktonic food web, it is possible estimate information content of the genomes of Daphnia and its phytoplankton prey, of the kairomone chemical indicator of a predator, of the interaction network and diversity of the community (Figure 1). However, it is not yet clear how to compare these to track information in the system over time or in response to environmental change. Yet another challenge arises when we consider the question, if information is defined by the sender and receiver, how is a third party to find meaning in it? This was precisely the problem faced by code breakers in World War II. It was from this challenge that a solution was found to objectively quantify information—this is was Claude Shannon's contribution, published in 1948. To build a more complete set of observations of information and information processing in ecological systems, we require new methods and theories to guide and interpret those methods.
Develop and Apply New Ecological Theory of Information
Calls for the integration of information theories with ecological theories have drawn on information concepts with distinct historical roots: semiotic information (Barbieri, 2008; Schmidt et al., 2010; Farina, 2011), structural information (Gatenby and Frieden, 2006; Tkačik and Bialek, 2016) and genetic information (Maynard Smith, 2000; Frank, 2008), and information criteria to support inference (Harte, 2011). This duality of syntactic vs. semiotic is a basic dichotomy in the literature that needs resolving if we are to develop an integrated theory of information for ecological systems. More explicit consideration of the interpretant—the scientist as the user of information, the relational roles of living systems as they use information—may bridge these information concepts (Atkin, 2006). Theories that admit semiotic information, syntactic information and the role of interpretants will integrate information approaches based on entropy measures of the structure and flows in communities and ecosystems (MacArthur, 1955; Margalef, 1957, 1985; Patten and Odum, 1981; Ulanowicz, 1997), with approaches in behavioral ecology and evolution (Maynard Smith, 2000; Dall et al., 2005; Farina, 2008; Frank, 2008; Schmidt et al., 2010; Pijanowski et al., 2011) that emphasize how organisms gather and use information to mediate their development, and their interactions with the environment that mediate their fitness (Holt, 2007). This joint description of ecological information dynamics will capture the principles outlined above and allow exploration of their consequences for the ecological patterns and processes we study. Resolving these gaps in information theory would then allow other theoretical advances to model dynamics of information in ecological systems (Gatenby and Frieden, 2006). Ultimately, this theory will allow us to interpret information flows across scales and how they map to fluxes of energy and materials and in so doing connect behavioral and cognitive ecology with community ecology and biogeography.
Conclusion
Ecological systems integrate information in multiple forms—syntactic and semiotic—with diverse relationships to energy and materials. We reviewed fundamental concepts in information theories, distinguishing between syntactic information and semiotic information. Information processing links these two forms in living systems, connecting processes across scales in holarchies. Taking this perspective on ecological systems may advance efforts to unify understanding across scales of life by drawing on knowledge systems related to different scientific disciplines. This unification brings challenges—understanding the relationships between different forms of information and energy, and developing methods for observing, quantifying and tracking information remain the focus of research efforts across disciplines.
Understanding ecology in terms of information and its links with energetic and material processes may help to bridge complex ideas and literatures in ecology and information sciences. To encourage such synthesis, we have articulated five principles for ecological systems that integrate diverse concepts of information in living systems (Box 1). Relating ecological ideas and questions to these principles to build a more coherent understanding of life is possible, and these principles lead to operational statements and empirical testable hypotheses.
Scientific and philosophical thinking and work has now placed information firmly “as one of three elemental components of existence (along with space/time and energy/matter)” (Atmar, 2001) in living and non-living systems, though this perspective is only just re-emerging in ecological thinking. A synthesis of information concepts in ecology is not that farfetched. It is well underway and operational in molecular biology, physiology and physics. Ecology has the opportunity to draw from these recent advances, and see our world from a new perspective.
A more robust and complete understanding of nature that is not restricted to a single research paradigm or scale of nature is not only an essential goal of science, but also critical to understanding how our world is changing. Harries-Jones (2009), drawing on the work of Douglas Bateson (Bateson, 1972), argued that the collapse of ecosystems follows the more subtle collapse of communicative order. This is a plausible hypothesis. Yet, modern ecological science is simply not equipped—theoretically or methodologically—to even test this hypothesis, nevermind to dismiss it. But we can remedy that, with rapid growth in an integrated science of the dynamics of information, energy, and materials in ecological systems. A synthesis in ecology could begin with explorations and tests of existing hypotheses about the role of information in living systems. A more unified and multi-scale ecological science that integrates information dynamics is not only possible but essential to pursue.
Author Contributions
MO, AG, and MP conceived of the study, reviewed literature and wrote manuscript. CM and BM developed model (Box 5). MO, MP, FA, BM, CM, and AG contributed to the development of the 5 principles and editing.
Funding
Funding is from the Swiss National Science Foundation Grants No PP00P3_179089 and 31003A_173074 and the University of Zurich Research Priority Programme URPP Global Change and Biodiversity (to FA). Funding from the EAWAG Socio-Economic-Technological Synthesis Center (SETS) to MO.
Conflict of Interest Statement
The authors declare that the research was conducted in the absence of any commercial or financial relationships that could be construed as a potential conflict of interest.
References
Adams, F. (2003). The informational turn in philosophy. Minds Machines 13, 471–501. doi: 10.1023/A:1026244616112
Akaike, H. (1974). A new look at the statistical model identification. IEEE Trans. Automat. Contr. 19, 716–723. doi: 10.1109/TAC.1974.1100705
Allen, A. P., and Gillooly, J. F. (2009). Towards an integration of ecological stoichiometry and the metabolic theory of ecology to better understand nutrient cycling. Ecol. Lett. 12, 369–384. doi: 10.1111/j.1461-0248.2009.01302.x
Allen, D. C., Cardinale, B. J., and Wynn-Thompson, T. (2014). Toward a better integration of ecological principles into ecogeoscience research. Bioscience 64, 444–454. doi: 10.1093/biosci/biu046
Altermatt, F., and Ebert, D. (2016). Reduced flight-to-light behaviour of moth populations exposed to long-term urban light pollution. Biol. Lett. 12, 20160111–20160114. doi: 10.1098/rsbl.2016.0111
Aplin, L. M., Farine, D. R., Morand-Ferron, J., Cockburn, A., Thornton, A., and Sheldon, B. C. (2015). Experimentally induced innovations lead to persistent culture via conformity in wild birds. Nature 518, 538–541. doi: 10.1038/nature13998
Atkin, A. (2006). Peirce's Theory of Signs. Available online at: https://plato.stanford.edu/archives/sum2013/entries/peirce-semiotics/
Atmar (2001). A profoundly repeated pattern. Bull. Ecol. Soc. Am. 82, 208–211. Available online at: www.jstor.org/stable/20168572
Babikova, Z., Gilbert, L., Bruce, T. J. A., Birkett, M., Caulfield, J. C., Woodcock, C., et al. (2013). Underground signals carried through common mycelial networks warn neighboring plants of aphid attack. Ecol. Lett. 16, 835–843. doi: 10.1111/ele.12115
Barbieri, M. (2008). Biosemiotics: a new understanding of life. Naturwissenschaften 95, 577–599. doi: 10.1007/s00114-008-0368-x
Barto, E. K., Weidenhamer, J. D., Cipollini, D., and Rillig, M. C. (2012). Fungal superhighways: do common mycorrhizal networks enhance below ground communication? Trends Plant Sci. 17, 633–637. doi: 10.1016/j.tplants.2012.06.007
Bateson, G. (1972). “Form, substance and difference,” in Steps to an Ecology of Mind (Chicago: University of Chicago Press).
Battesti, M., Pasquaretta, C., Moreno, C., Teseo, S., Joly, D., Klensch, E., et al. (2015). Ecology of information: social transmission dynamics within groups of non-social insects. Proc. R. Soc. B 282:20142480. doi: 10.1098/rspb.2014.2480
Bergstrom, C. T., and Rosvall, M. (2009). The transmission sense of information. Biol. Philos. 26, 159–176. doi: 10.1007/s10539-009-9180-z
Brooks, D. R., and Wiley, E. O. (1988). Evolution as Entropy: Toward a Unified Theory of Biology, 2nd Edn. Chicago, IL: University of Chicago Press.
Burnham, K. P., and Anderson, D. R. (2002). Model Selection and Multi-Model Inference: A Practical Information-Theoretic Approach. New York, NY: Springer Science and Business Media.
Burns, J. G., Foucaud, J., and Mery, F. (2011). Costs of memory: lessons from “mini” brains. Proc. R. Soc. Lond. B Biol. Sci. 278, 923–929. doi: 10.1098/rspb.2010.2488
Chaine, A. S., Legendre, S., and Clobert, J. (2013). The co-evolution of multiply-informed dispersal: information transfer across landscapes from neighbors and immigrants. PeerJ. 1, e44–e17. doi: 10.7717/peerj.44
Clobert, J., Le Galliard, J.-F., Cote, J., Meylan, S., and Massot, M. (2009). Informed dispersal, heterogeneity in animal dispersal syndromes and the dynamics of spatially structured populations. Ecol. Lett. 12, 197–209. doi: 10.1111/j.1461-0248.2008.01267.x
Coscieme, L., Pulselli, F. M., Jørgensen, S. E., Bastianoni, S., and Marchettini, N. (2013). Thermodynamics-based categorization of ecosystems in a socio-ecological context. Ecol. Modell. 258, 1–8. doi: 10.1016/j.ecolmodel.2013.02.031
Dall, S. R., Giraldeau, L. A., Olsson, O., McNamara, J. M., and Stephens, D. W. (2005). Information and its use by animals in evolutionary ecology. Trends Ecol. Evol. 20, 187–193. doi: 10.1016/j.tree.2005.01.010
Danchin, E., Giraldeau, L., Valone, T. J., and Wagner, R. H. (2004). Public information: from noisy neighbors to cultural evolution. Science 305, 487–491. doi: 10.1126/science.1098254
Davies, P., Imari Walker, S., and Ellis, G. F. R. (eds) (2017). From Matter to Life: Information and Causality. Cambridge University Press.
Davies, P. C., and Walker, S. I. (2016). The hidden simplicity of biology. Rep. Prog. Phys. 79, 1–19. doi: 10.1088/0034-4885/79/10/102601
Davy, S. K., Allemand, D., and Weis, V. M. (2012). Cell biology of cnidarian-dinoflagellate symbiosis. Microbiol. Mol. Biol. Rev. 76, 229–261. doi: 10.1128/MMBR.05014-11
de Andreazzi, C. S., Guimarães, P. R. Jr., and Melián, C. J. (2018). Eco-evolutionary feedbacks promote fluctuating selection and long-term stability of antagonistic networks. Proc. R. Soc. B 285:20172596. doi: 10.1098/rspb.2017.2596
de Ruyter van Steveninck, R. R., Lewen, G. D., Strong, S. P., Koberle, R., and Bialek, W. (1997). Reproducibility and variability in neural spike trains. Science 275, 1805–1809. doi: 10.1126/science.275.5307.1805
DeLong, J. P., and Gibert, J. P. (2016). Gillespie eco-evolutionary models (GEMs) reveal the role of heritable trait variation in eco-evolutionary dynamics. Ecol. Evol. 6, 935–945. doi: 10.1002/ece3.1959
Donaldson-Matasci, M. C., Bergstrom, C. T., and Lachmann, M. (2010). The fitness value of information. Oikos 119, 219–230. doi: 10.1111/j.1600-0706.2009.17781.x
Edgar, R. S., Green, E. W., Zhao, Y., van Ooijen, G., Olmedo, M., Qin, X., et al. (2012). Peroxiredoxins are conserved markers of circadian rhythms. Nature 485, 459–464. doi: 10.1038/nature11088
Ehrlich, P. R., and Raven, P. H. (1964). Butterflies and plants: a study in coevolution. Evolution 18, 586–608. doi: 10.1111/j.1558-5646.1964.tb01674.x
Erill, I. (2012). “Information theory and biological sequences: Insights from an evolutionary perspective,” in Information Theory New Research, eds. P. Deloumeaux and J. D. Gorzalka (Hauppauge, NY: Nova Science Publishers, Inc, 1–28.
Farina, A. (2008). The landscape as a semiotic interface between organisms and resources. Biosemiotics 1, 75–83. doi: 10.1007/s12304-008-9006-4
Farina, A. (2011). A biosemiotic perspective of the resource criterion: toward a general theory of resources. Biosemiotics 5, 17–32. doi: 10.1007/s12304-011-9119-z
Farina, A., and Belgrano, A. (2006). The eco-field hypothesis: toward a cognitive landscape. Landscape Ecol. 21, 5–17. doi: 10.1007/s10980-005-7755-x
Farina, A., and Pieretti, N. (2013). From umwelt to soundtope: an epistemological essay on cognitive ecology. Biosemiotics 7, 1–10. doi: 10.1007/s12304-013-9191-7
Farnsworth (2013). Living is information processing: from molecules to global systems. Acta Biotheor. 61, 203–222. doi: 10.1007/s10441-013-9179-3
Farnsworth, K. D., Lyashevska, O., and Fung, T. (2012). Functional complexity: the source of value in biodiversity. Ecol. Complex. 11, 46–52. doi: 10.1016/j.ecocom.2012.02.001
Fisher, R. A. (1925). The theory of statistical estimation. Proc. Camb. Philol. Soc. 22, 700–725. doi: 10.1017/S0305004100009580
Flack, J. C., Girvan, M., de Waal, F. B., and Krakauer, D. C. (2006). Policing stabilizes construction of social niches in primates. Nature 439, 426–429. doi: 10.1038/nature04326
Frank, S. A. (2008). Natural selection maximizes Fisher information. J. Evol. Biol. 22, 231–244. doi: 10.1111/j.1420-9101.2008.01647.x
Frank, S. A. (2012). Natural selection. V. How to read the fundamental equations of evolutionary change in terms of information theory. J. Evol. Biol. 25, 2377–2396. doi: 10.1111/jeb.12010
Fronhofer, E. A., Nitsche, N., and Altermatt, F. (2017). Information use shapes the dynamics of range expansions into environmental gradients. Global Ecol. Biogeogr. 26, 400–411. doi: 10.1111/geb.12547
Gaggiotti, O. E., Chao, A., Peres-Neto, P., Chiu, C.-H., Edwards, C., Fortin, M.-J., et al. (2018). Diversity from genes to ecosystems: a unifying framework to study variation across biological metrics and scales. Evol. Appl. 11, 1176–1193. doi: 10.1111/eva.12593
Gatenby, R. A., and Frieden, B. R. (2006). Information theory in living systems, methods, applications, and challenges. Bull. Math. Biol. 69, 635–657. doi: 10.1007/s11538-006-9141-5
Gatlin, L. L. (1972). Information Theory and the Living System. New York, NY: Columbia University Press.
Gerlach, G., Atema, J., Kingsford, M. J., Black, K. P., and Miller-Sims, V. (2007). Smelling home can prevent dispersal of reef fish larvae. Proc. Natl. Acad. Sci. U.S.A. 104, 858–863. doi: 10.1073/pnas.0606777104
Gil, M. A., Hein, A. M., Spiegel, O., Baskett, M. L., and Sih, A. (2018). Social information links individual behavior to population and community dynamics. Trends Ecol. Evol. 33, 535–548. doi: 10.1016/j.tree.2018.04.010
Giraldeau, L., and Caraco, T. (2000). Social Foraging Theory. Princeton, NJ: Princeton University Press. doi: 10.1515/9780691188348
Goldstein, D. S., and Kopin, I. J. (2017). Homeostatic systems, biocybernetics, and autonomic neuroscience. Auton. Neurosci. Basic Clin. 208, 15–28. doi: 10.1016/j.autneu.2017.09.001
Gordon, T. A. C., Harding, H. R., Wong, K. E., Merchant, N. D., Meekan, M. G., McCormick, M. I., et al. (2018). Habitat degradation negatively affects auditory settlement behavior of coral reef fishes. Proc. Natl. Acad. Sci. U.S.A. 115, 5193–5198. doi: 10.1073/pnas.1719291115
Groom, M. J., Meffe, G. K., and Carroll, C. R. (2005). Principles of Conservation Biology, 3rd Edn. eds.M. J. Groom, G. K. Meffe, and C. R. Carroll (Sunderland, Mass, MI: Sinauer Press).
Guimarães, P. R. Jr., Jordano, P., and Thompson, J. N. (2011). Evolution and coevolution in mutualistic networks. Ecol. Lett. 14, 877–885. doi: 10.1111/j.1461-0248.2011.01649.x
Gurevitch, J., Fox, G. A., Wardle, G. M., and Inderjit Taub, D. (2011). Emergent insights from the synthesis of conceptual frameworks for biological invasions. Ecol. Lett. 14, 407–418. doi: 10.1111/j.1461-0248.2011.01594.x
Hansen, L. D., Popovic, M., Tolley, H. D., and Woodfield, B. (2018). Laws of evolution parallel the laws of thermodynamics. J. Chem. Thermodyn. 124, 141–148. doi: 10.1016/j.jct.2018.05.005
Harries-Jones, P. (2009). Honeybees, communicative order, and the collapse of ecosystems. Biosemiotics 2, 193–204. doi: 10.1007/s12304-009-9044-6
Harte, J., Zillio, T., Conlisk, E., and Smith, A. B. (2008). Maximum entropy and the state variable approach to macroecology. Ecology, 89, 2700–2711. doi: 10.1890/07-1369.1
Helm, B., Ben-Shlomo, R., Sheriff, M. J., Hut, R. A., Foster, R., Barnes, B. M., et al. (2013). Annual rhythms that underlie phenology: biological time-keeping meets environmental change. Proc. R. Soc. B 280, 20130016. doi: 10.1098/rspb.2013.0016
Holt, R. (2007). The unraveling of Nature's information webs: the next depressing frontier in conservation? Israeli J. Ecol. Evol. 53, 229–236. doi: 10.1560/IJEE.53.3.229
Jablonka, E., and Lamb, M. J. (2005). Evolution in Four Dimensions: Genetic, Epigenetic, Behaviorial and Symbolic Variation in the History of Life. Cambridge, MA: The MIT Press.
Jablonka, E., and Szathmary, E. (2004). The evolution of information storage and heredity, Trends Ecol. Evol. 10, 206–211. doi: 10.1016/S0169-5347(00)89060-6
Jacob, S., Legrand, D., Chaine, A. S., Bonte, D., Schtickzelle, N., Huet, M., et al. (2017). Gene flow favours local adaptation under habitat choice in ciliate microcosms. Nat. Ecol. Evol. 1, 1–4. doi: 10.1038/s41559-017-0269-5
Jaynes, E. T. (1957). Information theory and statistical mechanics. Phys. Rev. 106:620. doi: 10.1103/PhysRev.106.620
Jiang, Y., and Xu, C. (2010). The calculation of information and organismal complexity. Biol. Direct 5:59. doi: 10.1186/1745-6150-5-59
Johnson, H. A. (1970). Information theory in biology after 18 years. Science 168, 1545–1550. doi: 10.1126/science.168.3939.1545
Jorgensen, S. E., Patten, B. C., and Straskraba, M. (2000). Ecosystems emerging: 4. growth. Ecol. Modell. 126, 249–284. doi: 10.1016/S0304-3800(00)00268-4
Kay, J. J. (1999). “Ecosystems as self-organizing holarchic open systems: narratives and the second law of thermodynamics,” in Handbook of Ecosystem Theories and Management eds S. E. Jorgensen and F. Muller (Boca Raton, FL: CRC Press - Lewis, 135–160.
Kempes, C. P., Wolpert, D., Cohen, Z., and Pérez-Mercader, J. (2017). The thermodynamic efficiency of computations made in cells across the range of life. Philos. Trans. A Math. Phys. Eng. Sci. 375, 20160343–20160326. doi: 10.1098/rsta.2016.0343
Kinmonth-Schultz, H. A., Golembeski, G. S., and Imaizumi, T. (2013). Circadian clock-regulated physiological outputs: dynamic responses in nature. Semin. Cell Dev. Biol. 24, 407–413. doi: 10.1016/j.semcdb.2013.02.006
Krakauer, D. C., Bertschinger, N., Olbrich, E., Ay, N., and Flack, J. C. (2014). The information theory of individuality. arXiv 1412.2447.v1.
Kull, K. (1999). Biosemiotics in the twentieth century: a view from biology. Semiotica 127, 385–414. doi: 10.1515/semi.1999.127.1-4.385
Laughlin, S. B., de Ruyter van Steveninck, R. R., and Anderson, J. C. (1998). The metabolic cost of neural information. Nat. Neurosci. 1, 36–41. doi: 10.1038/236
Lee, A. E. G., Ounsley, J. P., Coulson, T., Rowcliffe, J. M., and Cowlishaw, G. (2016). Information use and resource competition: an integrative framework. Proc. R. Soc. B. 283:1825. doi: 10.1098/rspb.2015.2550
Loreau, M. (2010). From Populations to Ecosystems: Theoretical Foundations for a New Ecological Synthesis. Princeton, NJ: Princeton University Press.
MacArthur, R. H. (1955). Fluctuations of animal populations and a measure of community stability. Ecology 36, 533–536. doi: 10.2307/1929601
Margalef, R. (1957). Information theory in ecology. Mem. real Acad. Cience. Art. Barcelona 32, 373–449. doi: 10.1017/S0031819100052062
Margalef, R. (1961). Communication of structure in planktonic populations. Limnol. Oceanogr. 6, 124–128.
Margalef, R. (1963). On certain unifying principles in ecology. Am. Nat. 97, 357–374. doi: 10.1086/282286
Margalef, R. (1985). From hydrodynamic processes to structure (information) and from information to process. Can. Bull. Fish. Aqual. Sci. 213, 200–220.
Marino, N. A. C., Srivastava, D. S., and Farjalla, V. F. (2015). Predator kairomones change food web structure and function, regardless of cues from consumed prey. Oikos 125, 1017–1026. doi: 10.1111/oik.02664
Marquet, P. A, Allen, A. P., Brown, J. H., Dunne, J. A., Enquist, B. J., Gillooly, J. F., et al. (2014). On theory in ecology. Bioscience 64, 701–710. doi: 10.1093/biosci/biu098
Marshall, W., Kim, H., Walker, S. I., Tononi, G., and Albantakis, L. (2017). How causal analysis can reveal autonomy in models of biological systems. Philos. Trans. Ser. A. 375:2109. doi: 10.1098/rsta.2016.0358
Martín, J., and López, P. (2013). Effects of global warming on sensory ecology of rock lizards: increased temperatures alter the efficacy of sexual chemical signals. Funct. Ecol. 27, 1332–1340. doi: 10.1111/1365-2435.12128
Maynard Smith, J. (2000). The concept of information in biology*. Philos. Sci. 67, 177–194. doi: 10.1086/392768
Melián, C. J., Matthews, B., de Andreazzi, C. S., Rodríguez, J. P., Harmon, L. J., and Fortuna, M. A. (2018). Deciphering the interdependence between ecological and evolutionary networks. Trends Ecol. Evol. 33, 504–512. doi: 10.1016/j.tree.2018.04.009
Melo, D., and Marroig, G. (2015). Directional selection can drive the evolution of modularity in complex traits. Proc. Natl. Acad. Sci. U.S.A. 112, 470–475. doi: 10.1073/pnas.1322632112
Mery, F., and Kawecki, T. J. (2005). A cost of long-term memory in Drosophila. Science 308:1148. doi: 10.1126/science.1111331
Morowitz, H. J. (1955). Some order-disorder considerations in living systems. Bull. Math. Biophys. 17, 81–86. doi: 10.1007/BF02477985
Moses, M., Bezerra, G., Edwards, B., Brown, J., and Forrest, S. (2016). Energy and time determine scaling in biological and computer designs. Philos. Trans. R. Soc. B Biol. Sci. 371, 20150446–20150411. doi: 10.1098/rstb.2015.0446
Munday, P. L., Dixson, D. L., Donelson, J. M., Jones, G. P., Pratchett, M. S., Devitsina, G. V., et al. (2009). Ocean acidification impairs olfactory discrimination and homing ability of a marine fish. Proc. Natl. Acad. Sci. U.S.A. 106, 1848–1852. doi: 10.1073/pnas.0809996106
Norton, B. G., and Ulanowicz, R. (2017). Scale and biodiversity policy: a hierarchical approach. Ambio 21, 244–249. doi: 10.1007/978-1-4612-4018-1_31
Odum, H. T. (1988). Self-organization, transformity, and information. Science 242, 1132–1139. doi: 10.1126/science.242.4882.1132
O'Neill, R. V., Deangelis, D. L., Waide, J. B., and Allen, T. F. H. (1986). A Hierarchical Concept of Ecosystems. Princeton, NJ: Princeton University Press.
Parrondo, J. M. R., Horowitz, J. M., and Sagawa, T. (2015). Thermodynamics of information. Nat. Phys. 11, 131–139. doi: 10.1038/nphys3230
Patten, B. C. (1959). An introduction to the cybernetics of the ecosystem: the trophic-dynamic aspect. Ecology 40, 221–231. doi: 10.2307/1930032
Patten, B. C. (2014). Systems ecology and environmentalism: Getting the science right. Part I: Facets for a more holistic Nature Book of Ecology. Ecol. Model. 293, 4–21. doi: 10.1016/j.ecolmodel.2014.04.010
Patten, B. C., and Odum, E. P. (1981). The cybernetic nature of ecosystems. Am. Nat. 118, 886–895. doi: 10.1086/283881
Patten, B. C., Straskraba, M., and Jorgensen, S. E. (2011). Ecosystem emerging. 5: constraints. Ecol. Model. 222, 2945–2972. doi: 10.1016/j.ecolmodel.2011.04.019
Phillips, S. J., Anderson, R. P., and Schapire, R. E. (2006). Maximum entropy modeling of species geographic distributions. Ecol. Modell. 190, 231–259. doi: 10.1016/j.ecolmodel.2005.03.026
Phillips, S. J., and Dudik, M. (2008). Modeling of species distributions with Maxent: new extensions and a comprehensive evaluation. Ecography 31, 161–175. doi: 10.1111/j.0906-7590.2008.5203.x
Pielou, E. (1967). “The use of information theory in the study of the diversity of biological populations,” in Fifth Berkeley Symposium (Berkeley, CA), 1–15. doi: 10.1016/0022-5193(67)90048-3
Pijanowski, B. C., Farina, A., Gage, S. H., Dumyahn, S. L., and Krause, B. L. (2011). What is soundscape ecology? An introduction and overview of an emerging new science. Landscape Ecol. 26, 1213–1232. doi: 10.1007/s10980-011-9600-8
Rosen, R. (1985). Anticipatory Systems. Philosophical, Mathematical and Methodological Foundations. New York, NY: Pergamon Press.
Rossi-Santos, M. R. (2015). Oil industry and noise pollution in the humpback whale (Megaptera novaeangliae) soundscape ecology of the Southwestern Atlantic Breeding Ground. J. Coast. Res. 31, 184–195. doi: 10.2112/JCOASTRES-D-13-00195.1
Sánchez-García, F. J., Machado, V., Galián, J., and Gallego, D. (2017). Application of the eco-field and general theory of resources to bark beetles: beyond the niche construction theory. Biosemiotics 10, 1–17. doi: 10.1007/s12304-016-9283-2
Scheiner, S. M., and Willig, M. R. (2008). A general theory of ecology. Theor. Ecol. 1, 21–28. doi: 10.1007/s12080-007-0002-0
Schmidt, K. A., Dall, S. R. X., and van Gils, J. A. (2010). The ecology of information: an overview on the ecological significance of making informed decisions. Oikos 119, 304–316. doi: 10.1111/j.1600-0706.2009.17573.x
Schneider, E. D., and Kay, J. J. (1994). Life as a manifestation of the second law of thermodynamics*. Math. Comput. Modell. 19, 25–48. doi: 10.1016/0895-7177(94)90188-0
Schneider, T. D. (2010). A brief review of molecular information theory. Nano Commun. Netw. 1, 173–180. doi: 10.1016/j.nancom.2010.09.002
Schrodinger, E. (1944). What is life? the physical aspects of the living cell. Cambridge: Cambridge University Press.
Schwarz, G. (1978). Estimating the dimension of a model. Ann. Statist. 6, 461–464. doi: 10.1214/aos/1176344136
Scott, B. (2004). Second-order cybernetics: an historical introduction. Kybernetes 33, 1365–1378. doi: 10.1108/03684920410556007
Sebeok, T. A., and Umiker-Sebeok, J. (1992). “Biosemiotics: the semiotic web,” eds T. A. Sebeok and J. Umiker-Sebeok (Berlin; New York, NY: Mouton de Gruyter).
Sentis, A., Ramon-Portugal, F., Brodeur, J., and Hemptinne, J.-L. (2015). The smell of change: warming affects species interactions mediated by chemical information. Global Change Biol. 21, 3586–3594. doi: 10.1111/gcb.12932
Seoane, L. F., and Solé, R. V. (2018). Information theory, predictability and the emergence of complex life. R. Soc. Open Sci. 5, 172221–172217. doi: 10.1098/rsos.172221
Shannon, C. E. (1948). A mathematical theory of communication. Bell Syst. Tech. J. 27, 379–423. doi: 10.1002/j.1538-7305.1948.tb01338.x
Sherwin, W. B., Chao, A., Jost, L., and Smouse, P. E. (2017). Information theory broadens the spectrum of molecular ecology and evolution. Trends Ecol. Evol. 32, 948–963. doi: 10.1016/j.tree.2017.09.012
Solé, R., Amor, D. R., Duran-Nebreda, S., Conde-Pueyo, N., Carbonell-Ballestro, M., and Montañez, R. (2016). Synthetic collective intelligence. BioSystems. 148, 47–61. doi: 10.1016/j.biosystems.2016.01.002
Sole, R. V., Manrubia, S. C., Luque, B., Delgado, J., and Bascompte, J. (1996). Phase transitions and complex systems: Simple, nonlinear models capture systems at the edge of chaos. Complexity 1, 13–36. doi: 10.1002/cplx.6130010405
Stevens, M., and Ruxton, G. D. (2011). Linking the evolution and form of warning coloration in nature. Proc. R. Soc. B 279, 417–426. doi: 10.1098/rspb.2011.1932
Szathmáry, E., and Smith, J. M. (2002). The major evolutionary transitions. Nature 374, 227–232. doi: 10.1038/374227a0
Szilard, L. (1929). Entropieverminderung in einem thermodynamischen system bei eingriffen intelligenter wesen. Zeitschrift fur Physik 53, 840–856. doi: 10.1007/BF01341281
Tkačik, G., and Bialek, W. (2016). Information processing in living systems. Annu. Rev. Condens. Matter Phys. 7, 89–117. doi: 10.1146/annurev-conmatphys-031214-014803
Ulanowicz, R. (2001). The balance between adaptability and adaptation. BioSystems. (New York, NY), 64, 13–22. doi: 10.1016/S0303-2647(01)00170-8
Ulanowicz, R. E. (2002). Information theory in ecology. Comput. Chem. 25, 393–399. doi: 10.1016/S0097-8485(01)00073-0
Ulanowicz, R. E., Goerner, S. J., Lietaer, B., and Gomez, R. (2009). Quantifying sustainability: resilience, efficiency and the return of information theory. Ecol. Complex. 6, 27–36. doi: 10.1016/j.ecocom.2008.10.005
Ulanowicz, R. E., Jørgensen, S. E., and Fath, B. D. (2006). Exergy, information and aggradation: an ecosystems reconciliation. Ecol. Modell. 198, 520–524. doi: 10.1016/j.ecolmodel.2006.06.004
Valdovinos, F. S., Brosi, B. J., Briggs, H. M., Moisset de Espanés, P., Ramos-Jiliberto, R., and Martinez, N. D. (2016). Niche partitioning due to adaptive foraging reverses effects of nestedness and connectance on pollination network stability. Ecol. Lett. 19, 1277–1286. doi: 10.1111/ele.12664
Valone, T. J. (2006). Are animals capable of Bayesian updating? An empirical review. Oikos 112, 252–259. doi: 10.1111/j.0030-1299.2006.13465.x
van der Sluijs, I., Gray, S. M., Amorim, M. C. P., Barber, I., Candolin, U., Hendry, A. P., et al. (2010). Communication in troubled waters: responses of fish communication systems to changing environments. Evol. Ecol. 25, 623–640. doi: 10.1007/s10682-010-9450-x
von Uexkull, J. (1992). A stroll through the worlds of animals and men: a picture book of invisible worlds. Semiotica 89, 319–391. doi: 10.1515/semi.1992.89.4.319
Wagner, A. (2017). Information theory, evolutionary innovations and evolvability. Philos. Trans. R. Soc. B Biol. Sci. 372, 20160416–20160418. doi: 10.1098/rstb.2016.0416
Walker, S. I., Kim, H., and Davies, P. C. (2016). The informational architecture of the cell. Phil. Trans. R. Soc. A 374, 20150057–20150020. doi: 10.1098/rsta.2015.0057
Watson, R. A., Mills, R., Buckley, C. L., Kouvaris, K., Jackson, A., Powers, S. T., et al. (2016). Evolutionary connectionism: algorithmic principles underlying the evolution of biological organisation in evo-devo, evo-eco and evolutionary transitions. Evol. Biol. 43, 553–581. doi: 10.1007/s11692-015-9358-z
Wicken, J. S. (1987). Entropy and Information: suggestions for common language. Philos. Sci. 54, 176–193. doi: 10.1086/289369
Wiener, N. (1948). “Cybernetics: or control and communication in the animal and the machine,” eds. HermannCie (Cambridge, MA: MIT Press), 194.
Woods, H. A., and Wilson, J. K. (2013). An information hypothesis for the evolution of homeostasis. Trends Ecol. Evol. 28, 283–289. doi: 10.1016/j.tree.2012.10.021
Appendix 1. Existing Ecological Principles
Fundamental principles are broad statements about empirical patterns and the processes that operate to create patterns (Scheiner and Willig, 2008) that are central to any scientific understanding expressed by theory. Fundamental principles are not novel statements but instead are true and foundational for modern accepted theories of ecology, often invoked to understand new problems or to organize or synthesize diverse ideas (Margalef, 1963; Allen and Gillooly, 2009; Gurevitch et al., 2011). Here we present a few examples of recently published principles of ecological science. These sets of principles are taken to be uncontroversial and consistent with any basic ecological text or training. Scheiner and Willig offer these 7 principles as central to a general and broad theory of ecology. The other two sets illustrate how ecological principles are typically referenced as starting points to understand a more specific, novel or applied subject. We find these are not foundational for an ecological science for the future, changing world, because they do not adequately reflect information processes that define living systems and the relationships they mediate between information, and energy and material flows.
7 Principles of the Theory of Ecology (Scheiner and Willig, 2008), articulated after an extensive review of recent progress in ecological understanding:
1. Organisms are distributed in space and time in a heterogeneous matter
2. Organisms interact with their abiotic and biotic environment environments
3. The distributions of organisms and their interactions depend on contingencies
4. Environmental conditions are heterogeneous in space and time
5. Resources are finite and heterogeneous in space and time
6. All organisms are mortal
7. The ecological properties of species are the result of evolution
Principles of Conservation Biology cites these as the shared principles with ecology (Groom et al., 2005).
1. Evolution is the basic axiom that unites all biology
2. The ecological world is dynamic and largely non-equilibrial
3. Human presence must be included in conservation planning
Three basic principles of ecology invoked to understand ecogeoscience research (Allen et al., 2014)
1. Biological traits exist in a distribution due to species diversity
2. Biological traits are adaptable and dynamic through time
3. Dynamically coupled relationships between species and their environments create biotic-abiotic feedback cycles.
Keywords: information theory, semiotic, entropy, organization, first principles, ecology, evolution
Citation: O'Connor MI, Pennell MW, Altermatt F, Matthews B, Melián CJ and Gonzalez A (2019) Principles of Ecology Revisited: Integrating Information and Ecological Theories for a More Unified Science. Front. Ecol. Evol. 7:219. doi: 10.3389/fevo.2019.00219
Received: 31 December 2018; Accepted: 24 May 2019;
Published: 18 June 2019.
Edited by:
Luciano Bosso, Department of Agriculture, University of Naples Federico II, ItalyReviewed by:
Melanie E. Moses, University of New Mexico, United StatesBrian Gregory Eddy, Natural Resources Canada, Canada
Copyright © 2019 O'Connor, Pennell, Altermatt, Matthews, Melián and Gonzalez. This is an open-access article distributed under the terms of the Creative Commons Attribution License (CC BY). The use, distribution or reproduction in other forums is permitted, provided the original author(s) and the copyright owner(s) are credited and that the original publication in this journal is cited, in accordance with accepted academic practice. No use, distribution or reproduction is permitted which does not comply with these terms.
*Correspondence: Mary I. O'Connor, oconnor@zoology.ubc.ca