- 1Department of Food and Resource Economics, University of Copenhagen, Frederiksberg, Denmark
- 2School of Economics, College of Business and Economics, University of Gondar, Gondar, Ethiopia
- 3Center for Microecology, Evolution and Climate, University of Copenhagen, Copenhagen, Denmark
Rural households across the tropics rely on bushmeat hunting to fulfill their subsistence and cash income needs. As human populations grow, and urban market demand drives commercial trade, hunting is often unsustainable, compromising community long-term food security and wildlife conservation objectives. Scarce information about the effectiveness of different intervention options hampers design of informed management strategies to reduce bushmeat hunting while simultaneously safeguarding community's food security. Here we examine the potential of interventions aimed at reducing bushmeat demand by evaluating the own- and cross-price elasticities, i.e., how consumers respond to changes in the price of bushmeat and the price of five substitutes—beef, chicken, lamb, goat, and fish. We conducted stated preference surveys, complemented by a socio-economic survey using the Poverty Environment Network protocol in 452 households in 21 villages in the Greater Serengeti Ecosystem in Tanzania. Using random intercept Poisson regression models, we find significant and elastic negative own-price elasticities of bushmeat demand and significant positive cross-price elasticities except for goat and fish. The significant (all at the 0.01 level) own-price elasticities ranges from −1.099 when bushmeat is paired with beef to −0.718 when bushmeat is paired with fish while the significant cross-price elasticities ranges from 0.128 when bushmeat is paired with beef to 0.590 when bushmeat is paired with lamb suggesting that most cross-price relations were inelastic. Variation between districts was considerable and depended on substitutes included in the model. Estimated elasticities were modified by socio-economic covariates including ethnicity, household size, household income, household Tropical Livestock Units ownership, household land ownership and distance to nearest protected area boundary, Lake Victoria and nearest road. Overall, we find mixed support for the hypothesis that interventions increasing the price of bushmeat and decreasing that of its substitutes will reduce bushmeat demand. The effectiveness of demand reducing interventions should increase if complemented by other policy interventions, e.g., interventions that increase the opportunity cost of hunting, by providing alternative income generation opportunities for hunters.
Introduction
Rural households across the tropics and sub-tropics rely on bushmeat hunting for subsistence and to generate cash income (Nielsen et al., 2017, 2018). However, bushmeat hunting is in many locations unsustainable (Dirzo et al., 2014; Ripple et al., 2016a; Benítez-López et al., 2017). Human population growth, technological advancement of hunting equipment and improved access to transport is driving a commercial bushmeat trade supplying urban centers of demand (Bennett and Robinson, 1999; Cawthorn and Hoffman, 2015). The resulting depletion of wildlife populations threatens both local food security and biodiversity conservation across the tropics (Harrison, 2011; Lindsey et al., 2013; Cawthorn and Hoffman, 2015; Ripple et al., 2016b). Hunting is often illegal, unregulated and unreported and most protected areas in the tropics are affected to some extent by bushmeat hunting (Schipper et al., 2008; Jones et al., 2018; Schulze et al., 2018). Hence, appropriate interventions are necessary to reduce illegal bushmeat hunting while safeguarding rural communities food security.
Interventions aiming to reduce illegal bushmeat hunting can target the supply side (i.e., hunters and other actors in the bushmeat market value chain), by increasing law enforcement or providing alternative livelihood opportunities for hunters (Moro et al., 2013; Nielsen et al., 2014). Interventions can alternatively target the demand side (i.e., consumers), by changing the purchasing habits of consumers by affecting the price of bushmeat and its substitutes to reduce demand (Rentsch and Damon, 2013). Evidence on the effect of demand-side interventions is scarce and inconclusive (Wilkie et al., 2005; van Velden et al., 2018; Veríssimo et al., 2018). Existing empirical evidence on price effects is ambiguous and appears highly context-dependent but tend to show that a price increase of bushmeat leads to decreased household bushmeat consumption while a price increase of substitute meat products leads to increased bushmeat consumption (Wilkie and Godoy, 2001; Wilkie et al., 2005; Fa and Brown, 2009; Foerster et al., 2012; Rentsch and Damon, 2013; Moro et al., 2015).
A considerable number of studies have examined bushmeat hunting in the Greater Serengeti Ecosystem (GSE) and found detrimental effects on wildlife populations including in Serengeti National Park (SNP) (Setsaas et al., 2007; Marealle et al., 2010; Strauss et al., 2015). Various interventions have been implemented to reduce bushmeat hunting in the SNP. These include strengthening law enforcement capacity and enhancing wildlife conservation awareness, promoting alternative protein and income sources (e.g., wage-earning activities), and providing veterinary care for domestic animals as well as community-level benefits including schools and health dispensaries (Moro et al., 2013, 2015; Rentsch and Damon, 2013; Kaaya and Chapman, 2017). Despite these interventions, bushmeat hunting persists and is expected to increase in the future (Rentsch and Packer, 2014) due to population growth and infrastructure development increasing market access (Dobson et al., 2010). In general, interventions aim to reduce bushmeat consumption by (i) raising local household income (i.e., assuming that bushmeat is an inferior good), (ii) increasing the price of bushmeat relative to its substitutes (i.e., other meat products), and (iii) increasing the opportunity cost of hunting. These interventions all affect the real price of bushmeat and its substitutes in one way or another. However, interventions have often been designed with a limited understanding of the effect of the price of bushmeat and its substitutes on bushmeat demand, which may explain the limited impact of interventions. Existing studies of the elasticity of bushmeat demand in the GSE have been geographically restricted to Western Serengeti and focused on a few substitute meat products (beef, fish, and daaga or chicken and fish) (Rentsch and Damon, 2013; Moro et al., 2015). However, determining how bushmeat demand responds to price changes and change in the price of a broader range of its substitutes across the wider GSE is essential to evaluate the heterogeneity in the likely effect on bushmeat hunting (Moro et al., 2015). Spatial heterogeneity may occur for example due to distance-induced differences in the availability of bushmeat and its substitutes and location-specific culturally determined differences in the acceptability of substitutes.
Economic theory suggests that change in the price of bushmeat can affect bushmeat consumption in two different ways: (i) increased bushmeat price reduce bushmeat demand and vice versa (the law of demand), and (ii) increased bushmeat price increase bushmeat demand and vice versa (Giffen goods hypothesis) (Varian, 2010). The latter is more hypothetical but could occur because bushmeat remains cheaper (relative to other meat types) even if its price increases and people consume more bushmeat at the expense of more expensive substitutes to compensate for lost income due to the increased price of bushmeat (i.e., the income effect outweighs the substitution effect). The relationship between bushmeat demand and its price is measured by own-price elasticity indicating the responsiveness of bushmeat demand to change in its price. In addition, change in the price of substitute protein sources can affect bushmeat demand in two ways: (i) increased substitute price increase bushmeat demand and vice versa (substitute good hypothesis) and (ii) increased substitute price reduce bushmeat demand and vice versa (complementary goods hypothesis) (Varian, 2010). The latter would occur if the substitute meat types were consumed together with bushmeat, e.g., for cultural or culinary reasons which is not the case here. The relationship between bushmeat demand and the price of its substitutes (or complements) are measured through the cross-price elasticity of bushmeat demand indicating the responsiveness of bushmeat demand to changes in prices of other (meat) products.
Evaluating household level price elasticities of bushmeat demand requires as a minimum information about bushmeat demand and price. This data can be obtained through revealed preferences in conventional household surveys—i.e., observing actual consumption over time (e.g., Rentsch and Damon, 2013). However, the revealed preference approach has limitations in its application to bushmeat research for at least two reasons: (i) the illegal nature of bushmeat supply and resulting fear of repercussions, may cause households to withhold or provide incorrect information (Nuno et al., 2013) and (ii) observed prices might not change markedly during the survey period and hence not include sufficient variation to support policy development (Moro et al., 2015). Stated preference methods have shown potential to overcome problems affecting data quality when asking sensitive questions in conservation research and are thus increasingly used (e.g., Moro et al., 2013; Nielsen et al., 2014). A stated preference approach also enables generation of bushmeat demand and price data beyond the current market situation enabling predictions about the likely response of bushmeat consumers if prices change significantly in the near future. Such predictions can provide relevant insights about bushmeat market development for informed policymaking. We, therefore, used a stated preference survey to generate data about bushmeat demand in response to price. The generated data hence represents stated rather than actual market demand.
Only one study in the GSE by Moro et al. (2015) has employed a stated preference survey to assess the price elasticity of bushmeat demand. Their study was geographically limited to Western Serengeti and assessed the price effects of two potential substitutes (chicken and fish) making results less representative across the ecosystem and for all available meat types. However, a broader insight into the effects of price change is needed to support conservation intervention across the GSE that encompasses considerable cultural and livelihood strategy diversity. Here we aim to bridge this information gap by assessing the own- and cross-price elasticity of bushmeat demand in five districts bordering protected areas around the GSE (452 households in 21 communities) including the effect of five available meat substitutes (fish, lamb, beef, chicken, and goat) and considering the effect of socio-economic (e.g., income, livestock ownership) and location (district) covariates. We also test the effect of spatial covariates including minimum distance to protected area boundaries, Lake Victoria and the nearest road as reflections of access to bushmeat and availability of substitutes.
Methods
Study Area
The GSE is dominated by plains hosting the last remaining great wildlife migration consisting of wildebeests (Connochaetes), zebras (Equus quagga) and Thomson's gazelles (Eudorcas thomsonii) tracking the availability of grazing across the Ecosystem. Both the national economy and local households in the GSE depend on tourism revenue and direct use of natural resources in the GSE. Over two million people live in the nearest seven districts (Kaltenborn et al., 2011) originating from more than 27 different ethnic groups. The most prevalent tribes are Maasai, Sukuma, and Kurya. Poverty levels are high and economic development is constrained by low agricultural productivity and market access and restrictions on land utilization imposed by the protected areas (Fyumagwa et al., 2017). The human population is growing at an alarming pace increasing pressure on the ecosystem to meet the demand for food, fuelwood, construction material, water, and land (Dybas, 2011; Estes et al., 2012). Protected areas in the GSE with different level of protection includes Serengeti National Park, Ikorongo, Maswa, and Grumeti Game Reserves, Ngorongoro Conservation Area and Loilondo Game Controlled Area (see Figure 1). Consumptive activities (e.g., settlement, agriculture, livestock grazing, environmental goods collection, and bushmeat hunting) are not allowed in the first four areas while regulated pastoralism is permitted in the latter two areas. However, illegal bushmeat hunting is widespread across the GSE. Numerous studies have attempted to estimate the prevalence of bushmeat hunting but are constrained by the secretive nature of this trade. Hence, estimates vary considerably across studies ranging over 32% (Loibooki et al., 2002), 9% (Knapp et al., 2010) and 18% (Nuno et al., 2013) of households in Western Serengeti—the latter estimate based on the unmatched-count technique. Rentsch and Packer (2014) estimate an annual offtake of 97,796–140,615 wildebeests threatening conservation objectives.
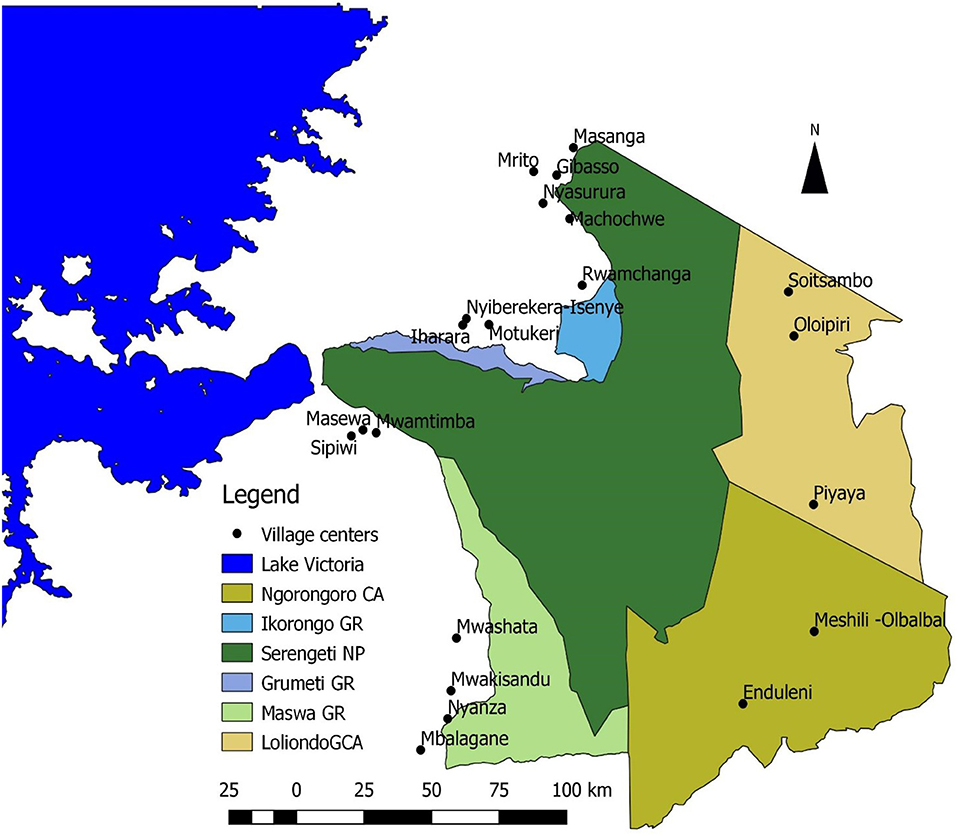
Figure 1. Villages surveyed in the Greater Serengeti Ecosystem. CA, Conservation area; GR, Game Reserve; NP, National Park; GCA, Game Controlled Area; only part of Lake Victoria shown.
Data Collection
The sampling unit is the household defined as a group of people (both family and non-family members) living under the same roof sharing labor and income (PEN, 2007). Households were selected using a three-stage stratified sampling strategy. In the first stage, 21 villages in the five districts, Meatu, Bariadi, Serengeti, Tarime, and Ngorongoro districts (see Figure 1) were purposively selected to encompass the variation in biophysical, socio-economic and administrative characteristics of the GSE. The districts differed markedly in precipitation, soil characteristics, human population density, ethnic composition and level of development (Table 1).
Communities were selected in clusters of three villages within each district at an increasing distance from the nearest protected area boundary. In the second stage, forty households were selected in each community (a total of 840 households) using stratified random sampling based on participatory wealth ranking (Grandin, 1988) of all households residing in the community according to an updated village register. Wealth ranking was conducted by a focus group consisting of 6–8 community members knowledgeable about local affairs grouping all households into three wealth categories (rich, intermediate, and poor) based on locally relevant and agreed criteria. From this sample 10, 20, and 10 households were randomly selected from the rich, intermediate and poor group, respectively, along with a contingency sample of three wealthy, four intermediate, and three poor households, which was used as a replacement in case of attrition. All households in the sample were subjected to four quarterly household surveys each producing detailed records of all cash and subsistence income the past 1 or 3 months depending on income source following the Poverty Environment Network questionnaire protocol (see Jiao et al., 2018). In the third stage, a sub-sample of approximately 21 households per village was presented with the stated preference survey. The choice of a sub-sample was based on cost, time and respondent fatigue implications in relation to other survey objectives (Walelign et al., 2019). The final sample in the analysis of own and cross-price elasticities consists of 452 households. Sample weights reflecting the inverse of the probability that a household was included based on the sampling strategy and the wealth rank distribution in the village was used in the analysis.
Designing the Choice Experiments
Development of the experimental design followed a three-step process. First, Focus Group Discussions (FGDs) were conducted in one village in each of four districts representative of the diversity of the GSE to understand meat consumption behavior, identify substitute protein sources, and determine price variations of bushmeat and the substitutes. The four villages are Mwamtimba, Gibaso, Oloipiri and Nyiberekera-Isenye in Bariadi, Tarime, Ngorongoro and Serengeti districts, respectively. Households in the GSE consume a wide variety of protein sources including various bushmeat species, beef, goat, lamb, chicken, larger fish that typically are tilapia, dagaa (small dried fish), milk, and eggs as well as vegetable-based protein sources. Milk, egg and vegetables are typically from own production or can be sourced locally very cheap (Lowassa et al., 2012). Dagaa is similarly, frequently consumed and very cheap. These goods are therefore not seen as substitutes for meat or larger fish, and policy intervention aiming to change the price of these goods may thus not significantly affect bushmeat consumption. We, therefore, focused on five meat types namely beef, lamb, goat, chicken, and larger fish the price of which is considered more likely to influence bushmeat demand and therefore constitutes better targets for policy interventions. FGDs aimed to establish common units of measurement across meat types in the level of processing (raw or sundried, with or without bones) and part often traded. Visual aids were developed consisting of pictures showing selected meat types, units and their size to ensure respondents common frame of reference. Three prices were obtained for each meat types: (1) the prices at which the household would consume the meat type as the main protein source (hereafter the minimum price) keeping the price of other meat sources at the current level, (2) the prevailing current market price (hereafter current price), and (3) the expected price 10 years from now assuming prevailing inflation rates due to population growth and resulting resource scarcity and as indicated by the focus groups (hereafter maximum price; Table 2).
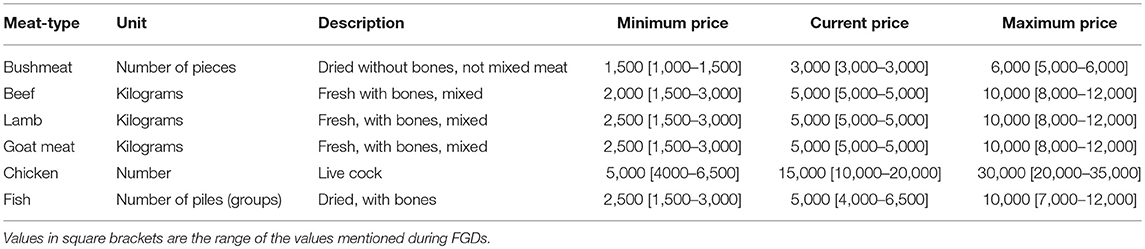
Table 2. List of substitute meat types and characteristics i.e. measurement unit, description, minimum, current, and maximum prices in TSH.
Secondly, the price of bushmeat was paired with the price of one substitute at a time to develop a total of five experiments: bushmeat vs. beef, bushmeat vs. chicken, bushmeat vs. lamb, bushmeat vs. goat meat and bushmeat vs. fish. This design was selected to reduce the cognitive burden on respondents as a design combining all meat types proved challenging to comprehend for the respondents during pre-testing. Each household was subjected to a stated preference questionnaire with two randomly selected meat types. A full factorial design (using the three prices; the minimum, current and maximum prices) was generated for each experiment resulting in nine combinations (32). The nine combinations were divided into three random blocks, and each respondent was randomly presented with two blocks representing two different meat types—i.e., each respondent was presented with twelve choice tasks (six from each experiment; see Figure 2 for an example of a choice card).

Figure 2. Example of a choice card with the associated question—how many piles/Kgs/pieces/number of the two types of meat would you buy at the indicated prices?.
Thirdly, the questionnaire was pilot tested in October 2016 in 64 households not part of the final sample to improve the clarity of questionnaires and update the description of scenarios with further information relevant for households demand decision and to make scenarios as credible as possible to respondents in accordance with Johnston et al. (2017). The generated choice-sets were posed as an open-ended choice experiment allowing respondents to provide an answer about the quantity of meat demanded at a given combination of prices. Thus, while the approach resembles designs from the discrete choice experiment literature, the data generated are continuous in the form of a count. The questions were asked in an interview-based questionnaire survey preceded by an introductory explanation given by enumerators. The explanation included a cheap talk script and a budget reminder to minimize bias arising from the hypothetical nature of the experiments (Tonsor and Shupp, 2011; Ladenburg and Olsen, 2014). Follow-up questions were included to determine whether the respondents attend to all meat type prices presented to them (SM1 in Supplementary Materials).
The data was collected between November 2016 and February 2017 using an ODK tablet interface, which enabled real-time data entry, and facilitated showing respondents pictures of meat types, units and their magnitudes to ensure common frame of reference (cf. above). The tablets also enabled presenting videos introducing the choice experiment. Interviews targeted the household head along with the wife (if the head was a man) whenever possible. In the rare cases where the household head was absent (estimated to be 1% of the households), the wife (if the head was a man) or the senior female household member (if the head was a woman) were interviewed alone as we believe that these individuals are in the best position to know and make decisions about meat demand on behalf of the household.
Data Analysis
As the demand was measured as the number of pieces purchased at a given price, the nature of the outcome variable is a count. We, therefore, employed Poisson models to examine the effect of price and other covariates on bushmeat demand. The basic Poisson regression model involves an equidispersion assumption requiring that the mean and the variance are equal (Wooldridge, 2002; Cameron and Trivedi, 2005). However, this assumption is often violated, and researchers, therefore, use alternative specifications of the general model including the negative binomial count model (Greene, 2008) and mixed effect Poisson regression models (Rabe-Hesketh and Skrondal, 2012). Mixed effect models have the advantage of being able to accommodate within-group covariance originating from the nested structure of the sampling strategy, sampling at the district and village level and each household performing several choice tasks. However, (i) the intracluster correlation (ICC) for the district level random intercept was <10%—a commonly used threshold for including random intercepts and slopes in mixed effect models (Rabe-Hesketh and Skrondal, 2012) and (ii) for the village level random intercept, either ICC was <10%, or its inclusion did not improve model performance (See SM2 in Supplementary Materials). We, therefore, used mixed effect Poisson models with household ID as the only random intercept. Following Rabe-Hesketh and Skrondal (2012), the expected number of pieces of bushmeat demanded, λij for the i′th choice situation in the j′th household can be estimated by:
where, γj is the household level intercept with a random distribution with zero mean and constant variance. Pij is a vector of the logarithmic transformed price of meat types, which implies that the average marginal effects can be interpreted as elasticities reflecting the proportional change in bushmeat demand as a result of a 1% change in the price of bushmeat or its substitute, keeping other covariates constant. PijXij is an interaction between the vector of meat type prices and a vector of socio-economic covariates. PijDij is an interaction between the vector of meat type prices and a vector of spatial variables including effect coded variables representing district. The covariates enter the model through interaction with prices, as the covariates were not included as attributes in the stated preference design. Hence, the coefficient of the interaction terms (i.e., βpx or βpd) reflects differences in responsiveness to price between socio-economic groups or locations. The average marginal effects were estimated as the average of the marginal effects for each observation which in turn were estimated as the product of the predicted values based on the model in Equation 1 and βp.
We considered different specifications of Equation 1: simple models and extended models with socio-economic and spatial covariates. This was guided by the aim of estimating the price elasticities from the simple model and determine how different covariates modify the estimated elasticities. Due to multicollinearity between the co-variates, we tested sets of covariates in different models. This implies that where multicollinearity is high (we used Variance Inflation Factor (VIF) above 10 as a threshold), we may end up with a less efficient model and exagerate or underestimate the effect of a given parameter (depending on sign). We therefore started with simple models where bushmeat demand was modeled as a function of the logarithmically transformed own-price and substitute price for individual meat types. We then extend the simple model by including socio-economic covariates. The socio-economic covariates were selected based on general theory about determinants of demand and previous empirical findings (see SM3 in Supplementary Materials for a description of the covariates). Selected covariates include: livestock possession measured in Tropical Livestock Units (TLUs), land ownership in acre, ethnic group affiliation, household size measured in Adult Equivalent Units (AEUs), total household income in PPP converted USD, and household preference for the relevant meat type measured as stated attendance to the prices in the experiments1. To assess differences between districts, we also extend the simple model by including an effect coded district variable (in place of socio-economic covariates) so that the effect of each district can be interpreted by comparison to all other districts and not just a single reference district (Gupta, 2008). Since respondents were presented with two meat type experiments, we controlled for the order in which the experiment under consideration was presented. Control for the order was included in all three versions of the model (i.e., simple model, model with socioeconomic covariates, and the model with district variables). We did not include the demand for substitute products as independent variables in the models for two main reasons. This includes the assumption that prices are the main determinants of demand for products rather than the demand for its substitutes. And the fact that because the demand for bushmeat and its substitutes are determined simultaneously, inclusion of demand for the substitutes could entail endogeneity bias.
Spatial variables reflecting the minimum distance to the protected area boundary, to Lake Victoria and a road, were not included in models mentioned above due to multicollinearity (i.e., mean VIF = 2902.26 and mean VIF = 2.68, with and without the distance variables, respectively, in the model extended with district variables). The effect of these variables on the elasticities was instead estimated through extracting the average marginal effect for significant elasticities for each household from the models with socio-economic covariates averaged across choice cards and regressed against the spatial variables using Ordinary Least Squares (OLS) regression. We furthermore explored non-linearity by including the level and squared terms of distance variables in the OLS models. The order in which the meat experiment under consideration was presented, was also controlled for in these models. Distances were determined as the minimum Euclidian distance. We preferred distance as a proxy for access to and availability of bushmeat and its substitutes over other spatial variables (e.g., forest cover), for two main reasons. First, the GSE is dominated by grasslands and the use of forest cover does not represent wildlife densities. Second, closeness to the source of meat and fish determines access to and availability by reducing transport costs and risk of being caught while transporting the meat in the case of bushmeat. In addition, the empirical literature on the availability of bushmeat in the GSE and elsewhere suggests that distance to protected areas is an appropriate measure of the availability of bushmeat (e.g., Brashares et al., 2011; Nuno et al., 2013).
Results
The results of the simple models, including the price of bushmeat and its substitute only, are presented in Table 3 reflecting the sign and statistical significance of the own- and cross-price elasticities for bushmeat and individual substitutes separately. The coefficients reflect the sign and statistical significance of the attributes and the average marginal effects can be interpreted as the magnitude of elasticities reflecting the proportional change in bushmeat demand as a result of a 1% change in the price of bushmeat or its substitute, keeping other things constant. As expected, the coefficient of bushmeat price is negative and significant indicating that the desired amount of bushmeat decreases as the price increases (i.e., it has a negative own-price elasticity). The own-price elasticities represented by the average marginal effects for the statistically significant coefficients range from −1.099, when bushmeat is paired with beef, to −0.718 when bushmeat is paired with fish. The beta coefficient for the price of substitutes is as expected positive and significant (beef at the 0.1 level) indicating positive cross-price elasticities except for goat and fish where it is insignificant. The estimated average marginal effects reflecting the magnitude of cross-price elasticities range from 0.128 when bushmeat is paired with beef to 0.590 when bushmeat is paired with lamb.
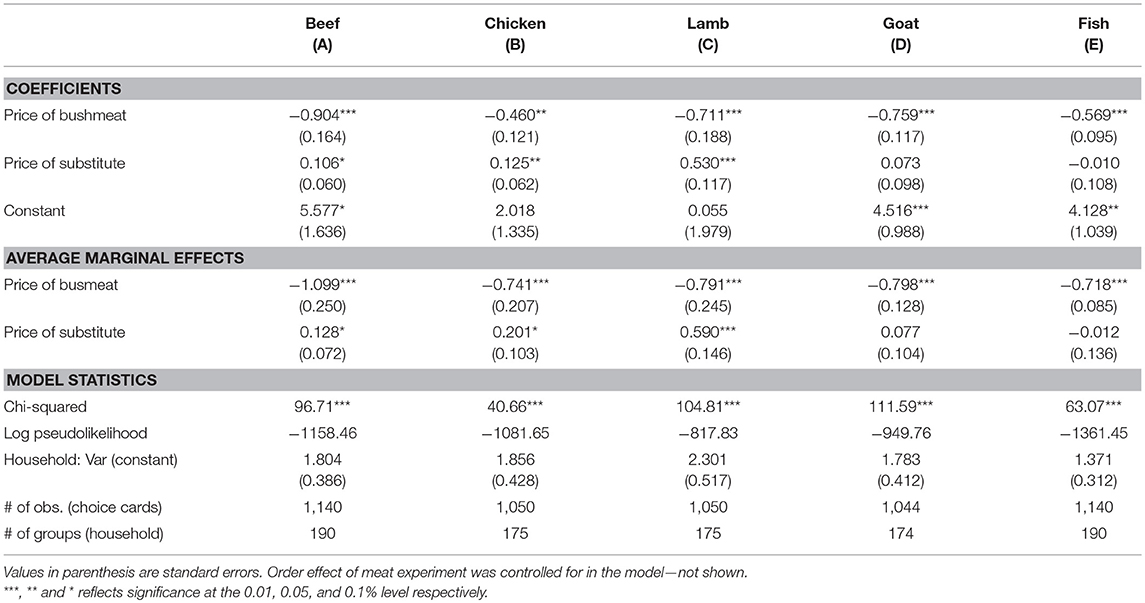
Table 3. Coefficients of the simple mixed effect Poisson models representing the own and cross-price elasticities of bushmeat demand for individual substitute meat types.
In summary, bushmeat demand is largely inelastic with respect to both its own price and the price of its substitutes in the simple model meaning that 1 percent increase in the price of bushmeat or its substitutes leads to <1 percent decrease and increase in bushmeat demand, respectively. The only exception is beef where one percent increase in the price of bushmeat leads to slightly above one percent decrease in bushmeat demand.
The models controlling for the effect of socioeconomic covariates are presented in Table 4. The own-price elasticity of bushmeat demand increase (numerically) when controlling for socioeconomic characteristics to the extent that average marginal effects become elastic for all meat types except goat. This implies that if bushmeat price increases by 1% it leads to more than 1% decrease in bushmeat demand when the substitute is beef, chicken, lamb and fish. However, cross-price elasticities were statistically insignificant except for fish where bushmeat demand was inelastic to change in fish price. These results indicate that the inelastic feature from Table 3, may be caused by heterogeneity in socioeconomic groups as it applies to cross-price elasticity.
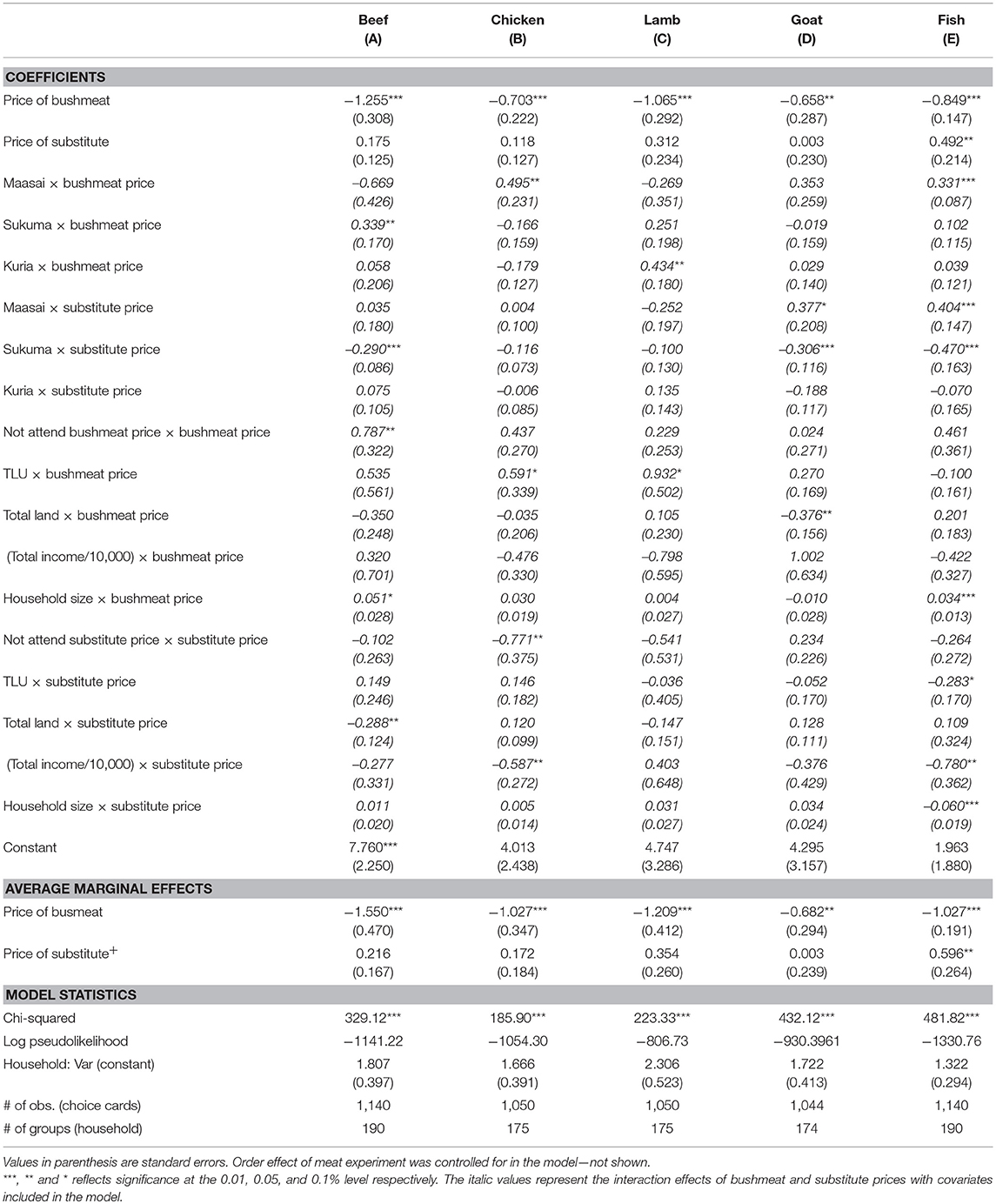
Table 4. Coefficients of the extended mixed effect Poisson models representing the own and cross-price elasticities of bushmeat demand for individual substitute meat types contingent on socioeconomic covariates.
Few socioeconomic covariates had significant effects and these varied between models depending on substitutes. Bushmeat demand by higher income households was significantly less responsive to the price of substitutes when the substitutes were chicken and fish only. In other word bushmeat demand by higher-income households increased less than by poorer households as the price of substitutes increased. Households with high TLUs were significantly (at the 0.1 level) less responsive to bushmeat price when the substitutes were chicken and lamb and less responsive to substitute price when the substitute was fish. Land rich households were more responsive to bushmeat price when the substitute was goat and less responsive to substitute price when the substitute was beef. Finally, larger households were less responsive to bushmeat price when the substitutes were beef (at the 0.1 level) and fish and less responsive to substitute price when the substitute was fish. Overall, the results reveal that larger households are less responsive to own-price whereas wealthier households measured in TLU and land are less and more responsive, respectively. Larger households, more income rich households and households wealthier in TLUs, and land were less responsive to substitute price.
Maasai household's bushmeat demand was less responsive to bushmeat price when the substitutes were chicken and fish and more responsive to substitute price when the substitutes were goat (at the 0.1 level) and fish (Table 4). Bushmeat demand by the Sukuma was less responsive to bushmeat price when the substitute was beef and less responsive to substitute price when the substitutes were beef, goat, and fish. Bushmeat demand by the Kuria was less responsive to the price of bushmeat when the substitute was lamb, and it was irresponsive to the price of any of the substitutes. Attendance to the price of meat types in making the demand decision differed between ethnic groups. Maasai households had the largest proportion of any tribe stating not attending to the price for all meat types (SM4 in Supplementary Materials). The model presented in Table 4 included a variable controlling for attendance to bushmeat price and the price of the relevant substitute. Households who do not attend to bushmeat and substitute price are less responsive to change in both bushmeat price when the substitute was beef and price of substitutes when the substitute was chicken.
The results of models exploring differences between districts are presented in Table 5. The own-price elasticity of bushmeat demand was higher in Serengeti district compared to other districts when the substitute was chicken while it was lower in Bariadi and Tarime districts when the substitute was fish. Cross-price elasticities were higher in Serengeti district for beef, chicken and fish and in Bariadi district it was lower for beef, goat and fish. In Meatu district the cross-price elasticity was lower for beef and fish. In these models, eastern GSE districts function as a baseline. However, if setting all other districts as a baseline, we find low own-price elasticities in Loliondo Game Controlled Area (LGCA) when the substitute is chicken and goat and in Ngorongoro Conservation Area (NCA) when the substitute is chicken (at the 0.1 level) and lamb (SM5 in Supplementary Materials). Cross-price elasticities were lower in NCA for chicken (at the 0.1 level) and higher for goat compared to other districts. These results indicate that demand responsiveness to price varies considerably between districts depending on substitutes.
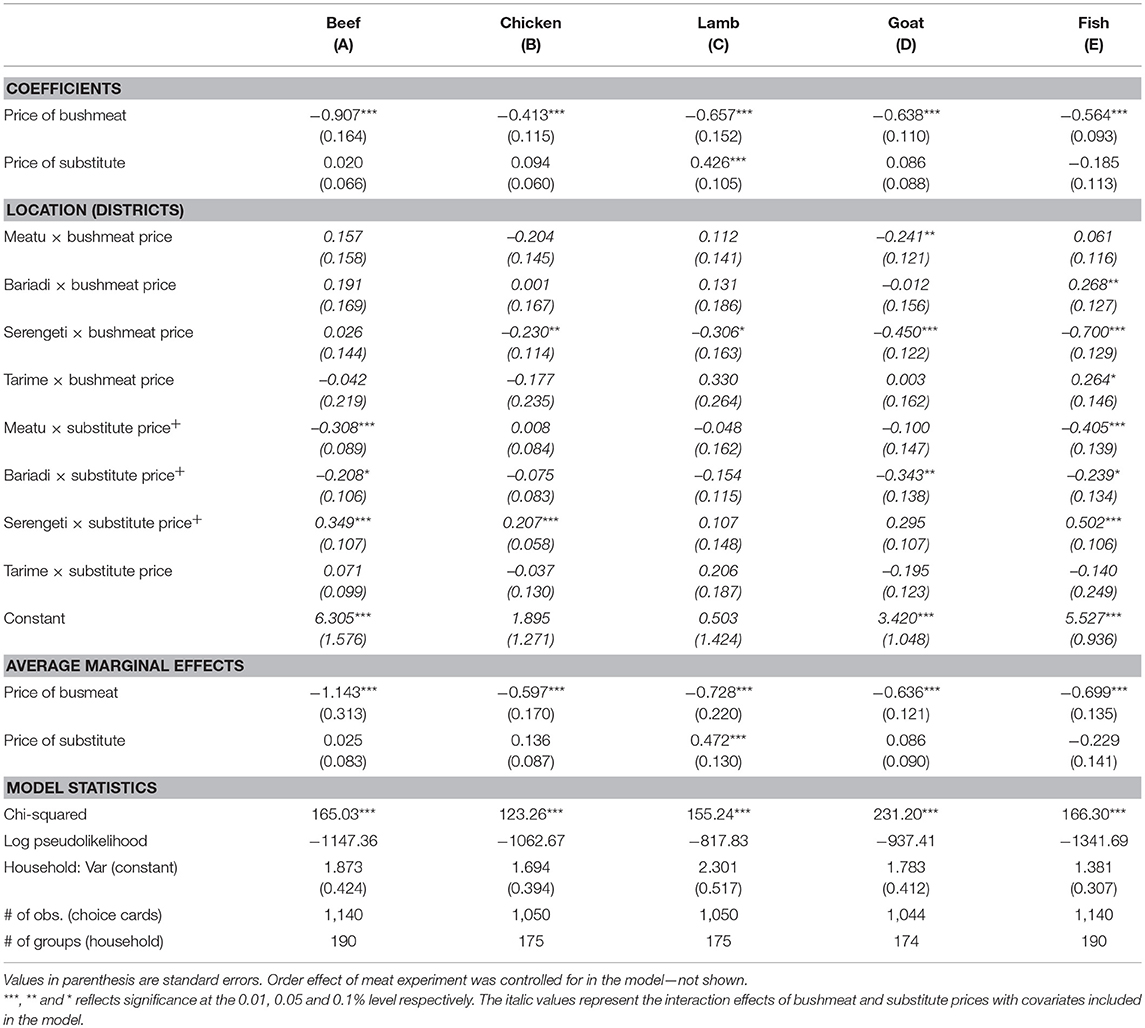
Table 5. Coefficients of the mixed effects Poisson models representing the own and cross-price elasticities of bushmeat demand for individual substitute meat types contingent on district effects.
We included distance to protected area boundaries and to Lake Victoria as variables in an ex-post OLS regression of elasticities as indicators of access to bushmeat and availability of substitutes, mainly fish. This approach was selected over including distance variables directly in the estimation due to multicollinearity. The results presented in Table 6 reveal that the own-price elasticity of bushmeat demand is positively associated with distance to the nearest protected area boundary and negatively associated with the distance to Lake Victoria when the substitute was beef (based on predictions of elasticities presented in Table 4 controlling for socioeconomic covariates). Hence responsiveness to bushmeat price is lower further from protected areas but higher further from Lake Victoria and it appears that distance to Lake Victoria exerts a higher impact on the own-price elasticity. The squared terms of these distances were also significantly positive and negative, respectively, meaning that the observed effects increase at an increasing rate as distance increase. When the substitute was chicken, the own-price elasticity of bushmeat demand was significantly positively associated with both the level and squared terms of distance to Lake Victoria indicating that the own-price elasticity of bushmeat demand decrease with distance to Lake Victoria at an increasing rate. The own-price elasticity of bushmeat demand decreased significantly and linearly with distances to the nearest road and Lake Victoria when the substitutes were lamb and fish, respectively. Fish was the only substitute for which bushmeat demand had significant cross-price elasticity when controlling for socioeconomic covariates (i.e., Table 4). The fish cross-price elasticity was significantly negatively associated with distance to Lake Victoria. This relationship means that the responsiveness of bushmeat demand to fish price decrease linearly as the distance to Lake Victoria increase.
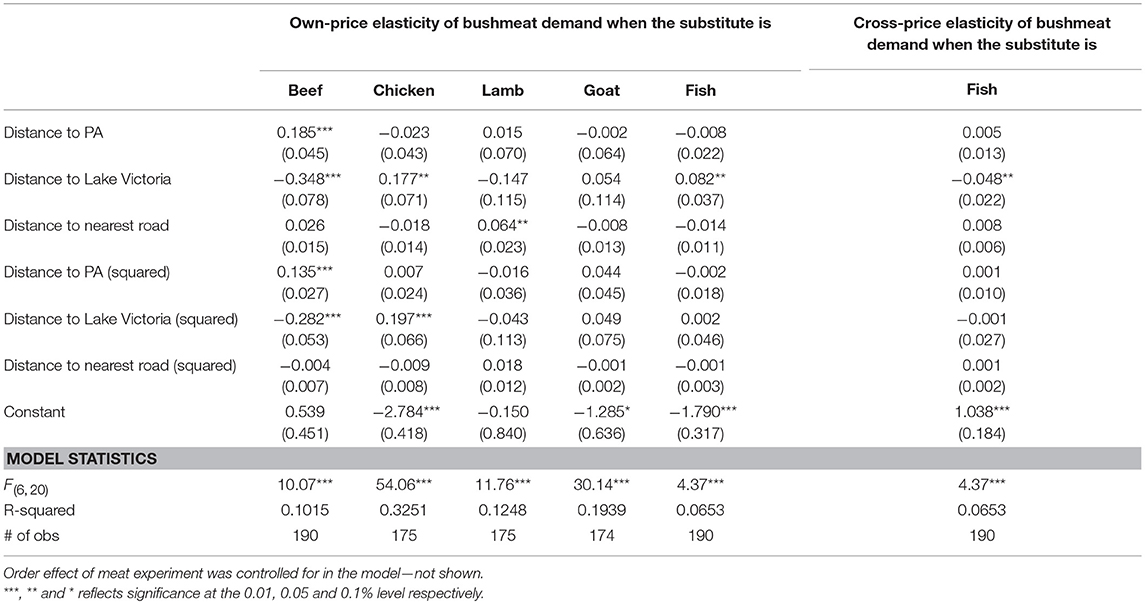
Table 6. OLS regression of estimated elasticities for individual meat types contingent on distance variables. Values in parenthesis are clustered standard errors at the village level.
Discussion
This study has investigated the own- and cross-price elasticity of bushmeat demand to provide information for informed decisions about interventions and policies to reduce hunting by affecting the bushmeat trade that currently exerts considerable pressure on wildlife populations threatening conservation objectives in the GSE as well as in other biodiversity-rich tropical regions. Compared to studies using observed preferences, we can evaluate the implications of larger price changes because we rely on stated preferences. Including a wider geographical area of the GSE furthermore, allow us to make more general conclusions including about geographical differences.
Responsiveness of Demand
We find that bushmeat demand responds negatively to changes in own-price in all five simple models without covariates and in all five extended models with socioeconomic covariates implying that bushmeat price increase will decrease demand across the GSE. This is consistent with the law of demand. Bushmeat demand is inelastic to price in four of the simple models indicating that one percent price increase leads to <1 percent decrease in bushmeat demand. However, controlling for socioeconomic covariates, four of the five extended models reveal elastic responses to bushmeat price increase. This suggests that socioeconomic covariates (i.e., household income, livestock ownership/TLU, land owned and household size) are important determinants of the responsiveness of bushmeat demand in GSE. Rentsch and Damon (2013) used an Almost Ideal Demand System analysis on revealed meat expenditure data from 131 households collected over 34 months in eight communities in Serengeti and Bunda districts implementing Seemingly Unrelated Regression models accounting for cross-equation correlations in evaluating elasticities. In their study Rentsch and Damon also found elastic uncompensated (Marshallian) own-price elasticities (−1.122) but contrary to us found inelastic income-compensated (Hicksian) elasticities (−0.696) in an analysis including beef, fish and dagaa as substitutes. Moro et al. (2015) using a stated preference approach with separate models for each substitute (very similar to ours) on data from 200 households in six villages in Western Serengeti found inelastic own-price elasticities in both simple models (−0.657 and −0.703) and in a model controlling for socioeconomic covariates (−0.138 and −0.551) with chicken and fish as substitutes.
We also find that bushmeat demand responds positively to a price increase for three out of five substitutes (consistent with the substitute good hypothesis) but only to fish when controlling for socioeconomic covariates. Consistent with other studies in the GSE none of these cross-price effects were elastic (Rentsch and Damon, 2013; Moro et al., 2015). Moro et al. (2015) found significant cross-price elasticities when the substitute was chicken only in the simple model (+0.286) whereas the cross-price elasticities for fish were significant in both the simple model (+0.371) and in models with socioeconomic covariates (+0.734 to +0.974), albeit not elastic. Rentsch and Damon (2013) found that beef, dagaa and fish all were substitutes for bushmeat and more so in the income-compensated model but also not elastically.
Overall this indicates that initiatives targeting poachers to increase the supply–costs thereby increasing the price of bushmeat, through enhanced enforcement and severer sanctions, are more likely to effectively reduce bushmeat demand than policies aiming to make substitutes cheaper (e.g., through subsidies and extension programs). Similar conclusions were reached by the two previous studies in the GSE (Rentsch and Damon, 2013; Moro et al., 2015). However, our findings suggests that the effectiveness of supply side interventions can be optimized by designing policies to target the different substitutes across the various social, economic and spatial contexts in the ecosystem as the results shows differential effect of availability of different meat types across socio-economic groups, districts and with distance to the source of the meat type (see below for details)
A number of revealed preference studies on the role of price on meat consumption has been conducted among Amerindian communities in Bolivia. Wilkie and Godoy (2001) found more elastic own-price elasticities, particularly in the top half of the income distribution (−5.852) but similarly weak cross-price elasticities using a sample of 443 households in 42 communities (Wilkie and Godoy, 2001). Apaza et al. (2002) found less elastic own-price elasticities (-1.145) but elastic cross-price elasticities for fish (+1.464) and particularly livestock (+7.446) expanding the same sample to 510 households in 59 communities. Similar results to ours but with inelastic own-price as well as cross-price elasticities were also observed in a study in 1,208 rural and urban households in six locations across Gabon (Wilkie et al., 2005). The considerable differences in the magnitude of elasticities between the Latin American and African studies may originate from differences in purchasing power and the availability of different meat types.
As expected demand differed depending on the substitute to which it was compared. Bushmeat demand was more responsive to own-price when the substitute was beef and least responsive when the substitute was fish or goat depending on control for socioeconomic covariates (i.e., with and without). Bushmeat demand was more responsive to substitute price when the substitute was lamb and fish, with and without control for socioeconomic covariates, respectively. Hence, attempting to reduce bushmeat demand by increasing its price is theoretically likely more effective when beef is available as a substitute, while strategies working through reduced substitute price will likely be more effective when substitutes are lamb or fish. However, increasing the availability of substitutes sufficiently to reduce the price and affect bushmeat demand is complicated by the currently large price differences between bushmeat and its substitutes (Ndibalema and Songorwa, 2008; Nielsen and Meilby, 2015), the ability of Lake Victoria fish stocks to sustainably support demand (Rentsch and Damon, 2013) and environmental impacts of even higher demand for grazing land for livestock production in the GSE (Veldhuis et al., 2019). Change in the price of one meat type will likely have ecological consequences through the system by affecting demand for other meat types that are intrinsically linked and may produce negative externalities (Brashares et al., 2004; Rentsch and Damon, 2013).
Effects of Socioeconomic Characteristics
Including socioeconomic variables in the models revealed that household income and wealth measured in livestock ownership (i.e., TLUs) and area of land owned reduced responsiveness to substitute price whereas effects on responsiveness to bushmeat price were mixed revealing lower and higher responsiveness of TLU and land-rich households, respectively. This indicates that wealthier households less readily shift to substitutes when the price of these decrease but that only land-rich households reduce bushmeat demand more than land-poor households when its price increase whereas the demand of TLU-rich households are less affected than TLU-poor households. The effect of TLU may initially seem counterintuitive but may be explained by the dominance of cattle in the TLU measure (about 74%) used mainly for milk production rather than to satisfy household meat demand, and that cattle in the GSE constitutes a source of saving and prestige more than meat (Knapp et al., 2015).
The mixed finding in relation to income and asset variables is comparable with the previous studies in the GSE that do not provide a uniform conclusion. For instance, evaluating expenditure elasticities as a measure of wealth assuming a high relation between income and expenditure due to generally low savings, Rentsch and Damon (2013) found elastic positive expenditure elasticities for bushmeat (+1.322) as well as for beef (+1.184) and fish (+1.006) indicating that consumption of these goods will increase as income (expenditure) increases. Moro et al. (2015) found that household wealth and number of household members with a job surprisingly had no significant effect on demand response to bushmeat price in Western Serengeti. Nyahongo et al. (2009) investigating bushmeat consumption frequencies in five villages in Western Serengeti found no relation with household income except in a village 80 km from the SNP.
Similar inconclusive findings are observed in other ecosystems. For instance, in Bolivia, no significant relationships were observed between bushmeat consumption and income or wealth in Amerindian households (Wilkie and Godoy, 2001; Apaza et al., 2002) although the elasticity varied from a necessity in the bottom half (+0.056) to an inferior good in the top half of the income distribution (−0.137) (Wilkie and Godoy, 2001). However, an extension of these surveys using a five-wave panel dataset from 324 households found a significant positive association between bushmeat consumption and wealth but not income and attributed this to a high degree of self-sufficiency and wealth being associated with investment in hunting technology (Godoy et al., 2010). Studies in Sub-Saharan Africa have found both negative (Albrechtsen et al., 2006), and positive relationships between bushmeat demand or consumption and income or wealth (East et al., 2005; Wilkie et al., 2005; Brashares et al., 2011; Foerster et al., 2012). Wilkie et al. (2005) for instance found increasing consumption of bushmeat (+0.169) as well as fish (+0.266), chicken (+0.262), and livestock (+0.144) with income and largest effect at the low end of the income distribution (due to the curvilinear relationship of log-transformed variables). National-wide surveys in Liberia found a considerable decrease of bushmeat consumption during the Ebola outbreak, but that wealthier households reduced their bushmeat consumption less than poorer households, that bushmeat prices remained stable and that peoples preferences for bushmeat remained the same despite its possible role as a disease vector (Ordaz-Németh et al., 2017). The stable price of bushmeat was likely explained by decreased hunting countering the lower demand. Households were, furthermore more likely to decrease bushmeat consumption if believing that Ebola could be contracted from bushmeat consumption.
In summary, our findings show that the relationship between bushmeat demand and wealth depends on the type of meat available, which is consistent with findings in the literature that show that bushmeat demand varies depending on the context, including whether it is rural or urban (Fa and Brown, 2009; Brashares et al., 2011; Luiselli et al., 2019) and likely also depending on food state and bushmeat species (East et al., 2005; Schenck et al., 2006; Ndibalema and Songorwa, 2008; Mwakatobe et al., 2012). Our results only partially support the concerns of other studies in the GSE indicating that efforts to increase household income and wealth will also increase bushmeat demand as well as demand for other protein sources. In general, there is a need for a much better understanding of what poverty is and how it relates to motivations for hunting and consuming bushmeat (Duffy et al., 2016).
Household size was also negatively associated with bushmeat demand responsiveness to own price (beef) and substitute price (beef and fish). The opposite results were observed by Moro et al. (2015) who found that household size increased own and cross-price elasticity in models where the substitute was chicken and fish, respectively. This difference may be explained by the lower variation in household size in their sample from Western Serengeti (mainly in Serengeti district), largely excluding households in Meatu and Bariadi district that tend to be significantly larger [mean AEU = 7.93, 7.72 and 5.80 for Meatu, Bariadi and Serengeti districts, respectively, P < 0.01 (ANOVA with Bonferroni multiple comparison test)]. Other studies have found negative relations between the quantity of bushmeat demanded or consumed per individual and household size (Wilkie et al., 2005; Albrechtsen et al., 2006; Godoy et al., 2010; Foerster et al., 2012), which contradicts general theory predicting higher efficiency of larger households (see Foerster et al., 2012). In this case, we expect that higher protein demands of larger households marginally override budget constraints also because demand is not measured per AEU.
The responsiveness of bushmeat demand also varied depending on household ethnicity and substitute considered. The own-price elasticity decreased when the respondent was from a Maasai household, and the substitute was chicken or fish; when the respondent was Sukuma, and the substitute was beef; and Kuria and the substitute was lamb. The cross-price elasticity for goat and fish declined when the respondent was Massai. It also declined for beef, goat and fish when the respondent was Sukuma, and for lamb when the respondent was Kuria. Moro et al. (2015) also found differences between ethnic groups in Western Serengeti in the effect of substitutes on elasticities. Culturally determined consumption preferences are likely to be important determinants of bushmeat consumption (Fa et al., 2002; East et al., 2005; Kaltenborn et al., 2005; Schenck et al., 2006; Kiffner et al., 2015). A survey of 600 households in five districts in Bunda, Meatu, Bariadi, and Tarime districts in GSE found high variability in bushmeat consumption and that the Ikoma and other inhabitants in Bunda district consumed more bushmeat than members of the Sukuma and Kurya ethnic groups (Ndibalema and Songorwa, 2008). Ceppi and Nielsen (2014) also found differences in prevalence and diversity of bushmeat consumption across a sample of 300 households from 10 ethnic groups across Tanzania. However, information about cultural and taste preferences for specific domestic as wells as wildlife species are currently insufficient to rigorously interpret these results.
Spatial Effects on Bushmeat Demand
The responsiveness of demand to price varied between districts and in relation to distance to spatial features in the landscape. Demand was more responsive to bushmeat price in Serengeti district compared to other districts while it was less responsive in Bariadi and Tarime districts but inconsistently and depending on the substitute. Responsiveness to beef price was high in Serengeti and Meatu and low in Bariadi while responsiveness to fish price was high in both Serengeti and Bariadi. Responsiveness to goat price was low in Bariadi but high in the NCA. Previous studies have found high consumption of bushmeat in Serengeti district followed closely by Meatu and Bariadi compared to Bunda and Tarime (Ndibalema and Songorwa, 2008). By including particularly the LGCA and NCA but also other districts not considered in previous elasticity studies (Rentsch and Damon, 2013; Moro et al., 2015) our results provide new insights to the design of policies aiming to reduce bushmeat demand through interventions manipulating prices by enabling optimization of design to the population's preferences in each district adjacent to the GSE.
Preferences were also influenced by households location in relation to spatial features irrespective of districts, but the direction of influence depends on the substitute. The responsiveness to bushmeat price was lower further away from the protected areas when the substitute was beef and decreased at an increasing rate with distance to the boundary perhaps indicating a tendency to becoming a luxury good with households further from the boundary willing to pay higher prices for bushmeat. Responsiveness to bushmeat price was also higher further away from Lake Victoria and increased at an increasing rate when the substitute was beef while it was lower and decreased at an increasing rate when the substitute was chicken. Responsiveness to the price of fish also decreased linearly with distance to Lake Victoria. These trends are likely associated with culturally determined preferences of the Massai in the eastern part of the GSE who may find chicken and fish unacceptable substitutes although bushmeat consumption by the Massai is also a relatively recent development (Ceppi and Nielsen, 2014; Kiffner et al., 2015). Finally, the responsiveness of demand to bushmeat price decreased with increasing distance to a road indicating that more remote households have a higher preference for bushmeat. A number of studies have investigated the influence of roads and distance to protected areas on bushmeat consumption, trade and game depletion (Macdonald et al., 2012; Fa et al., 2015; Mavah et al., 2018). Macdonald et al. (2011) surveyed bushmeat trading points in 87 villages in Nigeria and Cameroon through 150 days and found that prices increased with distance from protected area boundaries and were also higher closer to road networks. In Gabon, a study covering 928 households in 56 villages adjacent to three newly established national parks found that bushmeat consumption decreased as distance to protected area boundaries increased (Foerster et al., 2012). In Western Serengeti, the study by Nyahongo et al. (2009) found that bushmeat consumption declined significantly with distance to the protected area boundary.
Overall our results suggest that policies aiming to reduce bushmeat consumption through manipulation of prices are likely to be most effective by targeting areas close to the boundary in more remote areas where also evidence from other studies indicates that the amount of bushmeat consumed is likely to be higher. On a larger scale, such initiatives are either more or less likely to work further away from Lake Victoria depending on local culture and acceptability of substitutes.
Assessment of the Empirical Approach
We asked people to state the amount of meat they would purchase at different combinations of prices. As this is a hypothetical question rather than actual market transaction, it involves uncertainty about the amounts, familiarity with substitutes and own demand and is subject to hypothetical market bias. Hence, our results do not predict elasticities of actual demand but instead, reflect elasticities of stated demand. Furthermore, since bushmeat trade is illegal in the GSE, respondents may have incentives to provide strategic answers to influence policy decision in their favor. It is not clear which direction such motivations would have—i.e., whether they would increase or lower elasticities. Furthermore, previous studies using stated preference experiments in the context of bushmeat trade with actors actively involved in hunting and trading bushmeat suggests a large potential to provide information about such sensitive activities (Nielsen et al., 2014). Our design furthermore framed the experiment as a legal trade where all meat types were sold by a vendor coming to respondents household. Therefore, we do not expect the strategic element to be driving the results.
We found different elasticity estimates depending on the substitutes considered in each model. This is likely due to heterogeneity in our sample as a result of the large geographical extent and cultural diversity of the population in our study area causing different preferences, availability and familiarity with different meat types. Such differences were also observed in interviews of 2453 individuals in 27 communities across Nigeria, Togo, Niger and Burkina Faso (Luiselli et al., 2019) and may explain why most cross-price elasticities were insignificant. However, as we have tried to capture this heterogeneity by the inclusion of relevant covariates, we can discern general trends in bushmeat demand elasticities and identify differences in elasticities across socio-economic groups, locations and depending on spatial variables.
The estimated models only consider bushmeat demand while the demand for its substitutes was not included, contrary to the approach used by Rentsch and Damon (2013). However, if a household has no preference for consuming bushmeat or its substitutes at the given prices, this could have implications for estimation, reducing, the elasticity of bushmeat demand. We included a variable in each model reflecting whether respondents attended to bushmeat and substitute price in making their demand decisions to ensure that the results are not driven by such differences. However, we found that respondents not attending to the price of bushmeat in the model with beef as the substitute, indicating that they do not consume bushmeat, have significantly lower elasticity for bushmeat (cf. Table 4) and we, therefore, cannot exclude such effects in all models.
Conclusion
We assessed the own- and cross-price elasticity of bushmeat demand for more substitutes and across a wider geographical area of the GSE than previous studies and evaluated the implications of socioeconomic differences, distances to protected area boundaries, Lake Victoria and roads and compared districts using a stated preference approach. Bushmeat demand was negatively correlated with the price of bushmeat (i.e., negative own-price elasticity) and positively correlated with the price of substitutes (i.e., positive cross-price elasticity) (particularly for fish). Demand responded elastically to the price of bushmeat indicating that a 1% increase in the price of bushmeat leads to more than 1% decrease in bushmeat demand in most models controlling for socioeconomic covariates. However, demand responded inelastically to substitute price. These results suggest that increasing the price of bushmeat by targeting poachers to increase the supply–costs likely makes policies and initiatives aiming to reduce bushmeat hunting more effective than subsidies and extension programs aiming to make substitutes cheaper. Observed differences between ethnic groups and districts provide important insights enabling optimization of program design to the population's preferences in each district adjacent to the GSE. Household income and wealth measured in TLU and land mainly reduced the cross-price elasticity of bushmeat demand but also reduced the own-price elasticity of bushmeat demand for more land-rich households. This only partially support previous findings that efforts to improve household welfare across the GSE will increase protein demand increasing the pressure on wildlife populations. Demand responsiveness to bushmeat price furthermore declined with distance to protected area boundary but increased with distance to Lake Victoria. However, most effects differed between models depending on substitute considered, in a pattern that is difficult to explain due to limited information about cultural and taste preferences for specific domestic as wells as wildlife species.
Overall our results reveal that interventions aiming to reduce bushmeat demand by affecting prices while maintaining communities food security may not meaningfully reduce demand within the realistic price range shifts in the GSE context. However, the effectiveness of demand-reducing interventions should increase if complemented by other policy interventions. These interventions should ideally provide intrinsic motivations, that can be developed into long-lasting cultures of conservation (Cetas and Yasué, 2017) by appropriately acknowledging local value orientations in relation to wildlife and bushmeat (van Vliet, 2018). Options for engendering change in consumer preferences as well as hunter behavior may include edutainment interventions if appropriately designed to achieve sufficient audience penetration (Veríssimo et al., 2018), social marketing in the form of community engagement and information campaigns (Chaves et al., 2018; Green et al., 2019; Greenfield and Veríssimo, 2019; Veríssimo, 2019), social learning (Roux et al., 2011) and environmental education (Salazar et al., 2018). Simultaneously providing alternative income generation opportunities for hunters, that ideally should be incompatible with poaching or contingent on wildlife increase, may further increase the opportunity cost of hunting but may require substantial conceptual rethinking as well as improvement in funding design, monitoring and evaluation and the use of adaptive management strategies (Wright et al., 2016; Wicander and Coad, 2018). Furthermore, given sufficient time and prevalent urbanization, cultural norms and preferences toward bushmeat consumption are likely to change and reduce the acceptability of bushmeat consumption (Luiselli et al., 2019). The question is in what state the GSE and its wildlife populations will be at that time.
Ethics Statement
This study passed an EU Horizon 2020 ethics review procedure before commencement of the project (proposal number 641918). The ethics advisory group of the Department of Food and Resource Economics at the University of Copenhagen evaluated and approved the ethics guidelines, free, prior informed consent form (ID: GA641918). The Tanzanian Commission for Science and Technology granted permission for implementation of the study (research permit No 2017-299-NA2011-21). Finally, procedures for collecting information from human subjects were approved by the Tanzanian National Health Research Ethics Committee (ID: NIMR/HQ/R.8a/Vol. IX/2609).
Author Contributions
All the authors were involved in designing the study and choice experiment as well as writing the manuscript. SW conducted the focus group discussions and undertook data collection, management and analysis.
Conflict of Interest Statement
The authors declare that the research was conducted in the absence of any commercial or financial relationships that could be construed as a potential conflict of interest.
Acknowledgments
We are grateful for the efforts of TAWIRI enumerators and field supervisors for assisting with data collection. Permission for this study was granted through COSTECH research clearance No 2017-299-NA2011-21. This study was financed by the European Union's Horizon 2020 research and innovation program under grant agreement No. 641918 through the AfricanBioServices project. JBJ also acknowledges support from the Danish National Research Foundation to the Center for Macroecology, Evolution, and Climate.
Supplementary Material
The Supplementary Material for this article can be found online at: https://www.frontiersin.org/articles/10.3389/fevo.2019.00162/full#supplementary-material
Footnotes
1. ^Using a wealth index was not considered as it would not allow us to assess the importance of different asset types. Furthermore, including income may potentially impose an endogeneity issue. Although we cannot rule out an effect of income, we tried to avoid it by explicitly telling respondents to consider income constraints in their choices.
References
Albrechtsen, L., Fa, J. E., Barry, B., and Macdonald, D. W. (2006). Contrasts in availability and consumption of animal protein in Bioko Island, West Africa: the role of bushmeat. Environ. Conserv. 32, 340–348. doi: 10.1017/S0376892906002694
Apaza, L., Wilkie, D., Byron, E., Huanca, T., Leonard, W., Pérez, E., et al. (2002). Meat prices influence the consumption of wildlife by the Tsimane' Amerindians of Bolivia. Oryx 36, 382–388. doi: 10.1017/S003060530200073X
Benítez-López, A., Alkemade, R., Schipper, A. M., Ingram, D. J., Verweij, P. A., Eikelboom, J. A. J., et al. (2017). The impact of hunting on tropical mammal and bird populations. Science 356, 180–183. doi: 10.1126/science.aaj1891
Bennett, E. L., and Robinson, J. G. (1999). “Hunting for sustainability: the start of a synthesis,” in Hunting for Sustainability in Tropical Forests, eds E. L. Bennett and J. G. Robinson (New York, NY: Columbia University Press), 499–520.
Brashares, J. S., Arcese, P., Sam, M. K., Coppolillo, P. B., Sinclair, A. R. E., and Balmford, A. (2004). Bushmeat hunting, wildlife declines, and fish supply in West Africa. Science 306, 1180–1183. doi: 10.1126/science.1102425
Brashares, J. S., Golden, C. D., Weinbaum, K. Z., Barrett, C. B., and Okello, G. V. (2011). Economic and geographic drivers of wildlife consumption in rural Africa. Proc. Natl. Acad. Sci. U.S.A. 108, 13931–13936. doi: 10.1073/pnas.1011526108
Cameron, A. C., and Trivedi, P. K. (2005). Microeconometrics: Methods and Applications. New York, NY: Cambridge University Press.
Cawthorn, D. M., and Hoffman, L. C. (2015). The bushmeat and food security nexus: a global account of the contributions, conundrums and ethical collisions. Food Res. Int. 76, 906–925. doi: 10.1016/j.foodres.2015.03.025
Ceppi, S. L., and Nielsen, M. R. (2014). A comparative study on bushmeat consumption patterns in ten tribes in Tanzania. Tropic. Conserv. Sci. 7, 272–287. doi: 10.1177/194008291400700208
Cetas, E. R., and Yasué, M. (2017). A systematic review of motivational values and conservation success in and around protected areas. Conserv. Biol. 31, 203–212. doi: 10.1111/cobi.12770
Chaves, W. A., Valle, D. R., Monroe, M. C., Wilkie, D. S., Sieving, K. E., and Sadowsky, B. (2018). Changing wild meat consumption: an experiment in the central Amazon, Brazil: changing wild meat consumption in the Amazon. Conserv. Lett. 11:e12391. doi: 10.1111/conl.12391
Dirzo, R., Young, H. S., Galetti, M., Ceballos, G., Isaac, N. J. B., and Collen, B. (2014). Defaunation in the anthropocene. Science 345, 401–406. doi: 10.1126/science.1251817
Dobson, A. P., Borner, M., Sinclair, A. R. E., Hudson, P. J., Anderson, T. M., Bigurube, G., et al. (2010). Road will ruin Serengeti. Nature 467, 272–273. doi: 10.1038/467272a
Duffy, R., St John, F. A. V., Büscher, B., and Brockington, D. (2016). Toward a new understanding of the links between poverty and illegal wildlife hunting: poverty and illegal wildlife hunting. Conserv. Biol. 30, 14–22. doi: 10.1111/cobi.12622
Dybas, C. L. (2011). Saving the serengeti_madai mara. Bioscience 61, 850–855 doi: 10.1525/bio.2011.61.11.4
East, T., Kümpel, N. F., Milner-Gulland, E. J., and Rowcliffe, J. M. (2005). Determinants of urban bushmeat consumption in Río Muni, Equatorial Guinea. Biol. Conserv. 126, 206–215. doi: 10.1016/j.biocon.2005.05.012
Estes, A. B., Kuemmerle, T., Kushnir, H., Radeloff, V. C., and Shugart, H. H. (2012), Land-cover change human population trends in the Greater Serengeti ecosystem from 1984-2003. Biol. Conserv. 147, 255–263. doi: 10.1016/j.biocon.2012.01.010
Fa, J. E., and Brown, D. (2009). Impacts of hunting on mammals in African tropical moist forests: a review and synthesis. Mamm. Rev. 39, 231–264. doi: 10.1111/j.1365-2907.2009.00149.x
Fa, J. E., Olivero, J., Real, R., Farfán, M. A., Márquez, A. L., Vargas, J. M., et al. (2015). Disentangling the relative effects of bushmeat availability on human nutrition in central Africa. Sci. Rep, 5:8168. doi: 10.1038/srep08168
Fa, J. E., Peres, C. A., and Meeuwig, J. (2002). Bushmeat exploitation in tropical forests: an intercontinental comparison. Conserv. Biol. 16, 232–237. doi: 10.1046/j.1523-1739.2002.00275.x
Foerster, S., Wilkie, D. S., Morelli, G. A., Demmer, J., Starkey, M., Telfer, P., et al. (2012). Correlates of bushmeat hunting among remote rural households in Gabon, Central Africa. Conserv. Biol. 26, 335–344. doi: 10.1111/j.1523-1739.2011.01802.x
Fyumagwa, R. D., Mfunda, J., Ntalwila, J., and Jackson, C. R. (2017). “The serengeti ecosystem,” in Northern Serengeti Road Ecology, eds R. D. Fyumagwa, I. M. Mfunda, J. Ntalwila, and E. Røskaft (Bergen: Vigmostad and bjørke AS), 20–45.
Godoy, R., Undurraga, E. A., Wilkie, D., Reyes-García, V., Huanca, T., Leonard, W. R., et al. (2010). The effect of wealth and real income on wildlife consumption among native Amazonians in Bolivia: estimates of annual trends with longitudinal household data (2002-2006): Wildlife consumption, wealth and income. Anim. Conserv. 13, 265–274. doi: 10.1111/j.1469-1795.2009.00330.x
Grandin, B. (1988). Wealth Ranking in Smallholder Communities: A Field Manual. London: Intermediate Technology Publications.
Green, K. M., Crawford, B. A., Williamson, K. A., and DeWan, A. A. (2019). A meta-analysis of social marketing campaigns to improve global conservation outcomes. Soc. Market. Q. 25, 69–87. doi: 10.1177/1524500418824258
Greene, W. (2008). Functional forms for the negative binomial model for count data. Econ. Lett. 99, 585–590. doi: 10.1016/j.econlet.2007.10.015
Greenfield, S., and Veríssimo, D. (2019). To what extent is social marketing used in demand reduction campaigns for illegal wildlife products? insights from elephant ivory and rhino horn. Soc. Mar. Q. 25, 40–54. doi: 10.1177/1524500418813543
Gupta, R. (2008). Coding Categorical Variables in Regression Models: Dummy and Effect Coding. StatNews #72; Cornell Statistical Consulting Unit; Ithaca, NY: Cornell University.
Harrison, R. D. (2011). Emptying the forest: hunting and the extirpation of wildlife from tropical nature reserves. Bioscience 61, 919–924. doi: 10.1525/bio.2011.61.11.11
Jiao, X., Walelign, S. Z., Nielsen, M. R., and Smith-Hall, C. (2018). Environmental Reliance in the Greater Serengeti-Mara Ecosystem. Manuscript. Department of Food and Resource Economics; Copenhagen: University of Copenhagen.
Johnston, R. J., Boyle, K. J., Adamowicz, W., Bennett, J., Brouwer, R., Cameron, T. A., et al. (2017). Contemporary guidance for stated preference studies. J. Assoc. Environ. Res. Economists 4, 319–405. doi: 10.1086/691697
Jones, K. R., Venter, O., Fuller, R. A., Allan, J. R., Maxwell, S. L., Negret, P. J., et al. (2018). One-third of global protected land is under intense human pressure. Science 360, 788–791. doi: 10.1126/science.aap9565
Kaaya, E., and Chapman, M. (2017). Micro-credit and community wildlife management: complementary strategies to improve conservation outcomes in Serengeti National Park, Tanzania. Environ. Manage. 60, 464–475. doi: 10.1007/s00267-017-0856-x
Kaltenborn, B. P., Nyahongo, J. W., and Kideghesho, J. R. (2011). The attitudes of tourists towards the environmental, social and managerial attributes of Serengeti National Park, Tanzania. Tropic. Conserv. Sci. 4, 132–148. doi: 10.1177/194008291100400204
Kaltenborn, B. P., Nyahongo, J. W., and Tingstad, K. M. (2005). The nature of hunting around the western corridor of Serengeti National Park, Tanzania. Eur. J. Wildl. Res. 51, 213–222. doi: 10.1007/s10344-005-0109-9
Kiffner, C., Peters, L., Stroming, A., and Kioko, J. (2015). Bushmeat consumption in the Tarangire-Manyara ecosystem, Tanzania. Tropic. Conserv. Sci. 8, 318–332. doi: 10.1177/194008291500800204
Knapp, E. J., Rentsch, D., Schmitt, J., Lewis, C., and Polasky, S. (2010). A tale of three villages: choosing an effective method for assessing poaching levels in western Serengeti, Tanzania. Oryx 44, 178–184. doi: 10.1017/S0030605309990895
Knapp, L. M., Knapp, E. J., Metzger, K. L., Rentsch, D., Beyers, R., Hampson, K., et al. (2015). “Human health in the Greater Serengeti ecosystem,” in Serengeti IV : Sustaining Biodiversity in a Coupled Human-Natural System, eds A. R. E. Sinclair, R. B. Boone, K. L. Metzger, S. A. R. Mduma, and J. M. Fryxell (Chicago: University of Chicago Press), 451–482.
Ladenburg, J., and Olsen, S. B. (2014). Augmenting short cheap talk scripts with a repeated opt-out reminder in choice experiment surveys. Res. Energy Econ. 37, 39–63. doi: 10.1016/j.reseneeco.2014.05.002
Lindsey, P. A., Balme, G., Becker, M., Begg, C., Bento, C., Bocchino, C., et al. (2013). The bushmeat trade in African savannas: impacts, drivers, and possible solutions. Biol. Conserv. 160, 80–96. doi: 10.1016/j.biocon.2012.12.020
Loibooki, M., Hofer, H., Campbell, K. L. I., and East, M. L. (2002). Bushmeat hunting by communities adjacent to the Serengeti National Park, Tanzania: the importance of livestock ownership and alternative sources of protein and income. Environ. Conserv. 29, 391–398 doi: 10.1017/S0376892902000279
Lowassa, A., Tadie, D., and Fischer, A. (2012). On the role of women in bushmeat hunting – Insights from Tanzania and Ethiopia. J. Rural Stud. 28, 622–630. doi: 10.1016/j.jrurstud.2012.06.002
Luiselli, L., Hema, E. M., Segniagbeto, G. H., Ouattara, V., Eniang, E. A., Di Vittorio, M., et al. (2019). Understanding the influence of non-wealth factors in determining bushmeat consumption: results from four West African countries. Acta Oecol. 94, 47–56. doi: 10.1016/j.actao.2017.10.002
Macdonald, D. W., Johnson, P. J., Albrechtsen, L., Dutton, A., Seymour, S., Dupain, J., et al. (2011). Association of body mass with price of bushmeat in Nigeria and Cameroon: association of body mass with price of bushmeat. Conserv. Biol. 25, 1220–1228. doi: 10.1111/j.1523-1739.2011.01741.x
Macdonald, D. W., Johnson, P. J., Albrechtsen, L., Seymour, S., Dupain, J., Hall, A., et al. (2012). Bushmeat trade in the Cross-Sanaga rivers region: evidence for the importance of protected areas. Biol. Conserv. 147, 107–114. doi: 10.1016/j.biocon.2011.12.018
Marealle, W. N., Fossøy, F., Holmern, T., Stokke, B. G., and Røskaft, E. (2010). Does illegal hunting skew Serengeti wildlife sex ratios? Wildlife Biol. 16, 419–429. doi: 10.2981/10-035
Mavah, G. A., Funk, S. M., Child, B., Swisher, M. E., Nasi, R., and Fa, J. E. (2018). Food and livelihoods in park-adjacent communities: the case of the Odzala Kokoua National Park. Biol. Conserv. 222, 44–51. doi: 10.1016/j.biocon.2018.03.036
Moro, M., Fischer, A., Czajkowski, M., Brennan, D., Lowassa, A., Naiman, L. C., et al. (2013). An investigation using the choice experiment method into options for reducing illegal bushmeat hunting in western Serengeti. Conserv. Lett. 6, 37–45. doi: 10.1111/j.1755-263X.2012.00284.x
Moro, M., Fischer, A., Milner-Gulland, E. J., Lowassa, A., Naiman, L. C., and Hanley, N. (2015). A stated preference investigation of household demand for illegally hunted bushmeat in the Serengeti, Tanzania. Anim. Conserv. 18, 377–386. doi: 10.1111/acv.12184
Mwakatobe, A., Røskaft, E., and Nyahongo, J. (2012). Bushmeat and food security: species preference of sundried bushmeat in communities in the Serengeti - Mara ecosystem, Tanzania. Int. J. Biodivers. Conserv. 4, 548–559.
Ndibalema, V. G., and Songorwa, A. N. (2008). Illegal meat hunting in serengeti: dynamics in consumption and preferences. Afr. J. Ecol. 46, 311–319. doi: 10.1111/j.1365-2028.2007.00836.x
Nielsen, M. R., Jacobsen, J. B., and Thorsen, B. J. (2014). Factors determining the choice of hunting and trading bushmeat in the Kilombero Valley, Tanzania: choice of hunting and trading bushmeat. Conserv. Biol. 28, 382–391. doi: 10.1111/cobi.12197
Nielsen, M. R., and Meilby, H. (2015). Hunting and trading bushmeat in the Kilombero Valley, Tanzania: motivations, cost-benefit ratios and meat prices. Environ. Conserv. 42, 61–72. doi: 10.1017/S0376892914000198
Nielsen, M. R., Meilby, H., Smith-Hall, C., Pouliot, M., and Treue, T. (2018). The importance of wild meat in the global south. Ecol. Econ. 146, 696–705. doi: 10.1016/j.ecolecon.2017.12.018
Nielsen, M. R., Pouliot, M., Meilby, H., Smith-Hall, C., and Angelsen, A. (2017). Global patterns and determinants of the economic importance of bushmeat. Biol. Conserv. 215, 277–287. doi: 10.1016/j.biocon.2017.08.036
Nuno, A., Bunnefeld, N., Naiman, L. C., and Milner-Gulland, E. J. (2013). A novel approach to assessing the prevalence and drivers of illegal bushmeat hunting in the Serengeti. Conserv. Biol. 27, 1355–1365. doi: 10.1111/cobi.12124
Nyahongo, J. W., Holmern, T., Kaltenborn, B. P., and Røskaft, E. (2009). Spatial and temporal variation in meat and fish consumption among people in the western Serengeti, Tanzania: the importance of migratory herbivores. Oryx 43, 258–266. doi: 10.1017/S0030605307991127
Ordaz-Németh, I., Arandjelovic, M., Boesch, L., Gatiso, T., Grimes, T., Kuehl, H. S., et al. (2017). The socio-economic drivers of bushmeat consumption during the West African Ebola crisis. PLoS Negl. Trop. Dis. 11:e0005450. doi: 10.1371/journal.pntd.0005450
PEN (2007). PEN Technical Guidelines. Retrieved from: https://www.cifor.org/pen/the-pen-technical-guidelines/ (accessed May 6, 2019).
Rabe-Hesketh, S., and Skrondal, A. (2012). Multilevel and Longitudinal Modeling Using Stata: Categorical Responses, Counts, and Survival, Vol. II, 3rd Edn. College Station, TX: Stata Press.
Rentsch, D., and Damon, A. (2013). Prices, poaching, and protein alternatives: an analysis of bushmeat consumption around Serengeti National Park, Tanzania. Ecol. Econ. 91, 1–9. doi: 10.1016/j.ecolecon.2013.03.021
Rentsch, D., and Packer, C. (2014). The effect of bushmeat consumption on migratory wildlife in the Serengeti ecosystem, Tanzania. Oryx 49, 287–294. doi: 10.1017/S0030605313001038
Ripple, W. J., Abernethy, K., Betts, M. G., Chapron, G., Dirzo, R., Galetti, M., et al. (2016b). Bushmeat hunting and extinction risk to the world 's mammals. R. Soc. Open Sci. 3:160498. doi: 10.1098/rsos.160498
Ripple, W. J., Chapron, G., López-bao, J. V., Durant, S. M., Macdonald, D. W., Corlett, R. T., et al. (2016a). Saving the world's terrestrial megafauna. Bioscience 66, 807–812. doi: 10.1093/biosci/biw092
Roux, D. J., Murray, K., Nel, J. L., Hill, L., Roux, H., and Driver, A. (2011). From scorecard to social learning: a reflective coassessment approach for promoting multiagency cooperation in natural resource management. Ecol. Soc. 16:24. doi: 10.5751/ES-03888-160124
Salazar, G., Mills, M., and Veríssimo, D. (2018). Qualitative impact evaluation of a social marketing campaign for conservation. Conserv. Biol. 33, 634–644. doi: 10.1111/cobi.13218
Schenck, M., Nsame Effa, E., Starkey, M., Wilkie, D., Abernethy, K., Telfer, P, et al. (2006). Why people eat bushmeat: results from two-choice, taste tests in Gabon, Central Africa. Hum. Ecol. 34, 433–445. doi: 10.1007/s10745-006-9025-1
Schipper, J., Chanson, J. S., Chiozza, F., Cox, N. A., Hoffmann, M., Katariya, V., et al. (2008). The status of the world's land and marine mammals: diversity, threat, and knowledge. Science 322, 225–230. doi: 10.1126/science.1165115
Schulze, K., Knights, K., Coad, L., Geldmann, J., Leverington, F., Eassom, A., et al. (2018). An assessment of threats to terrestrial protected areas. Conserv. Lett. 11:e12435 doi: 10.1111/conl.12435
Setsaas, T. H., Holmern, T., Mwakalebe, G., Stokke, S., and Røskaft, E. (2007). How does human exploitation affect impala populations in protected and partially protected areas? - a case study from the Serengeti Ecosystem, Tanzania. Biol. Conserv. 136, 563–570 doi: 10.1016/j.biocon.2007.01.001
Strauss, M. K. L., Kilewo, M., Rentsch, D., and Packer, C. (2015). Food supply and poaching limit giraffe abundance in the Serengeti. Popul. Ecol. 57, 505–516. doi: 10.1007/s10144-015-0499-9
Tonsor, G. T., and Shupp, R. S. (2011). Cheap talk scripts and online choice experiments: “looking beyond the mean. Am. J. Agric. Econ. 93, 1015–1031. doi: 10.1093/ajae/aar036
van Velden, J., Wilson, K., and Biggs, D. (2018). The evidence for the bushmeat crisis in African savannas: a systematic quantitative literature review. Biol. Conserv. 221, 345–356. doi: 10.1016/j.biocon.2018.03.022
van Vliet, N. (2018). “Bushmeat crisis” and “cultural imperialism” in wildlife management? Taking Value orientations into account for a more sustainable and culturally acceptable wildmeat sector. Front. Ecol. Evol. 6. doi: 10.3389/fevo.2018.00112
Varian, H. R. (2010). Intermediate Microeconomics: A Modern Approach, 8th Edn. New York, NY: W. W. Norton and Co.
Veldhuis, M. P., Ritchie, M. E., Ogutu, J. O., Morrison, T. A., Beale, C. M., Estes, A. B., et al. (2019). Cross-boundary human impacts compromise the Serengeti-Mara ecosystem. Science 363, 1424–1428. doi: 10.1126/science.aav0564
Veríssimo, D. (2019). The past, present, and future of using social marketing to conserve biodiversity. Soc. Mar. Q. 25, 3–8 doi: 10.1177/1524500419825545
Veríssimo, D., Schmid, C., Kimario, F. F., and Eves, H. E. (2018). Measuring the impact of an entertainment-education intervention to reduce demand for bushmeat. Anim. Conserv. 21, 324–331. doi: 10.1111/acv.12396
Walelign, S. Z., Nielsen, M. R., and Jacobsen, J. B. (2019). Roads and livelihood activity choices in the Greater Serengeti Ecosystem, Tanzania. PLoS ONE 14:e0213089. doi: 10.1371/journal.pone.0213089
Wicander, S., and Coad, L. (2018). Can the provision of alternative livelihoods reduce the impact of wild meat hunting in west and Central Africa? Conserv. Soc. 16, 441–458 doi: 10.4103/cs.cs_17_56
Wilkie, D. S., and Godoy, R. A. (2001). Income and price elasticities of bushmeat demand in lowland Amerindian Societies\relasticidades de insumo y preciosde demanda en sociedades Amerindias de Tierras Bajas. Conserv. Biol. 15, 761–769. doi: 10.1046/j.1523-1739.2001.015003761.x
Wilkie, D. S., Starkey, M., Abernethy, K., Effa, E. N., Telfer, P., and Godoy, R. (2005). Role of prices and wealth in consumer demand for bushmeat in Gabon, Central Africa. Conserv. Biol. 19, 268–274. doi: 10.1111/j.1523-1739.2005.00372.x
Wooldridge, J. M. (2002). Economic Analysis of Cross Section and Panel Data. Cambridge, MA: MIT Press.
Keywords: bushmeat demand, preference experiment, price elasticities, Greater Serengeti Ecosystem, demand side policy
Citation: Walelign SZ, Nielsen MR and Jakobsen JB (2019) Price Elasticity of Bushmeat Demand in the Greater Serengeti Ecosystem: Insights for Managing the Bushmeat Trade. Front. Ecol. Evol. 7:162. doi: 10.3389/fevo.2019.00162
Received: 31 October 2018; Accepted: 24 April 2019;
Published: 15 May 2019.
Edited by:
Nathalie Van Vliet, Center for International Forestry Research, IndonesiaReviewed by:
Laura Vang Rasmussen, University of British Columbia, CanadaWillandia Chaves, Woodrow Wilson School of Public and International Affairs, Princeton University, United States
Copyright © 2019 Walelign, Nielsen and Jakobsen. This is an open-access article distributed under the terms of the Creative Commons Attribution License (CC BY). The use, distribution or reproduction in other forums is permitted, provided the original author(s) and the copyright owner(s) are credited and that the original publication in this journal is cited, in accordance with accepted academic practice. No use, distribution or reproduction is permitted which does not comply with these terms.
*Correspondence: Solomon Zena Walelign, szw@ifro.ku.dk