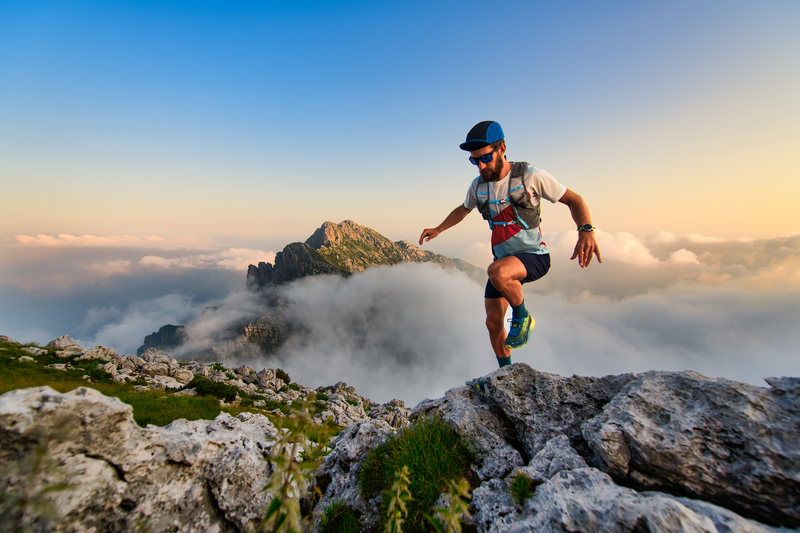
94% of researchers rate our articles as excellent or good
Learn more about the work of our research integrity team to safeguard the quality of each article we publish.
Find out more
ORIGINAL RESEARCH article
Front. Ecol. Evol. , 03 May 2019
Sec. Evolutionary and Population Genetics
Volume 7 - 2019 | https://doi.org/10.3389/fevo.2019.00152
Circadian rhythms are ubiquitous among taxa and are essential for coping with recurrent daily events, leading to selection on the properties of the clock underlying these rhythms. To quantify this selection in the wild, we need, however, to phenotype wild individuals, which is difficult using the standard laboratory approach for which individuals need to be kept under constant conditions. To overcome this problem, we explored the possibility to link the variation in a key clock property, circadian period (Tau), to genetic variation. We measured Tau in 152 captive great tits (Parus major). We further linked Tau to two circadian phase markers, the onset of activity in the Light:Dark cycle, and the first onset in constant conditions (Dim:Dim), directly after entrainment. We did a genome-wide association study using a 650 k SNP chip, and we linked genetic polymorphisms of a set of 12 candidate genes, to Tau and the two circadian phase markers. In line with earlier studies, Tau was heritable (h2 = 0.48 ± 0.22). Despite this genetic variation, we did not find any significant associations at the genome-wide level with the measured traits and only one candidate gene showed association with onset of activity in the Light:Dark cycle. Identifying the genetic base of circadian timing for wild species thus remains challenging. Including alternative molecular methods such as epigenetics or transcriptomics could help to unravel the molecular basis of the biological clock in great tits.
Circadian rhythms are ubiquitous to both uni- and multi-cellular organisms, and govern numerous physiological and behavioral functions such as cell division, gene expression, hormone secretion, and rest-activity cycles (Takahashi et al., 2001). Given the importance of these rhythms, it is assumed that there will be strong selection on properties of the circadian clock underlying these rhythms (Johnson, 2005). Indeed, the circadian pacemaker appears to be crucial for individual fitness, for example for the regulation of physiology and behavior in advance of changes in environmental conditions (Daan, 1981; Pittendrigh, 1993; DeCoursey et al., 2000; Merrow et al., 2005). In the presence of a natural light-dark cycle, individuals will synchronize their endogenous circadian pacemaker to the external day-night rhythm (a process called entrainment). In the absence of time cues, organisms continue to express activity in a regular pattern. The duration of this free running rhythm is determined by the endogenous circadian period (Tau), a key property of the clock underlying this rhythm (Aschoff, 1989). Tau is around 24 h, as it is evolutionary adaptive to have a Tau close to the natural light-dark cycle, and varies between individuals. Individuals living in an environment with a light-dark cycle that differs from their circadian period are compromised in their longevity and reproduction (Pittendrigh and Minis, 1972; Hurd and Ralph, 1998; Spoelstra et al., 2016). Variation in Tau was found to be heritable in the great tit (Parus major; Helm and Visser, 2010).
The current knowledge on how circadian clocks function in the wild (Schwartz et al., 2017) is however strongly hampered by measuring clock phenotypes, which, when obtained, could then be related to fitness of individuals under natural conditions. This will then open the possibility to document variation in selection on clocks between for instance males and females, or along a latitudinal transect. However, unmasking clock phenotypes by keeping individuals in the absence of time cues by keeping them for weeks in constant conditions [e.g., constant darkness or dim light; (Aschoff, 1960)] as is often used in the laboratory, is not feasible for wild individuals. Such conditions may render fitness measurements inaccurate by changes in the phase of the circadian pacemaker and thus disrupting the animals when returned to the field. Also, when removed from the field for weeks, animals may for instance lose their territory, also affecting fitness measurements. One way to assess Tau in wild animals is the measurement of the circadian period in cultured fibroblasts (Yagita et al., 2001), which has been explored in humans (Pagani et al., 2010; Hida et al., 2017). Another alternative way to phenotype wild individuals is to link the variation in clock properties to genetic variation, and then to genotype wild individuals using a small blood sample and subsequently associate this genetic variation with fitness under natural conditions.
Over the last two decades, knowledge on the molecular mechanisms underlying circadian rhythms has strongly increased. The physical location, and the extent of concentration and hierarchy of these mechanisms varies between organisms (Bell-Pedersen et al., 2005), but these mechanisms are generally composed of multiple, interacting molecular feedback loops (Brown et al., 2012). An important factor why the knowledge on these molecular feedback loops driving cellular circadian rhythms has advanced so much is because many genes coding for the proteins involved in molecular feedback loops have been discovered (Shearman et al., 2000; Lowrey and Takahashi, 2011). Early examples of such findings are the shortening of Tau by mutant casein kinase I epsilon (CSNK1E) in hamsters (Ralph and Menaker, 1988), the changes in Tau by mutant Cryptochrome 1 and 2 proteins (Horst et al., 1999) and the loss of rhythmicity by the mutant Clock protein (CLOCK) in mice (Vitaterna et al., 1994).
Here, to predict the circadian phenotype from wild animals, we aimed to link genetic variation to these phenotypes by genotyping a number of wild great tits with already measured Tau and the phase of activity (i.e., the moment of activity onset relative to the moment the light went on), using both genome-wide association and candidate gene studies. We obtained Tau as well as two circadian parameters that are dependent on Tau: the onset of activity in the Light:Dark cycle and the first onset in constant conditions (Dim:Dim) directly after entrainment (Aschoff and Wever, 1966; Dominoni et al., 2013).
In our experiments, we use the great tit, which is a model species in ecology and evolution and for which a comprehensive molecular toolbox is available, including a well annotated reference genome (Laine et al., 2016), whole transcriptomes and methylomes from several tissues (Santure et al., 2011; Derks et al., 2016; Laine et al., 2016) and two SNP chips, 10 and 650 k (Van Bers et al., 2012; Kim et al., 2018). Tau was a trait found to be heritable in great tits (Helm and Visser, 2010), and contrary to many lab model species, ecological and physiological traits can be studied in both the lab and the field relatively easily in this species (Schaper et al., 2012). Moreover, there have been a large number of wild individuals genotyped (Gienapp et al., 2019) for which also fitness data are available so when we can associate SNP variation to variation in Tau we can directly estimate the selection on Tau in a long-term wild population.
In this study, the great tits used originated from a large-scale artificial selection experiment in which selection on seasonal timing is studied by creating selection lines for early and late breeding (Verhagen et al. under review). In total, 186 Dutch great tits from three generations (F1 = 66, F2 = 101, and F3 = 19) went through a procedure to estimate Tau (see “Measurement of circadian rhythms” below). The birds were tested in five batches due to space limitations (Supplementary Table 1).
The birds were individually housed in light-tight cages (85 × 40 × 48 cm) that were distributed over four walls in three rooms. Every cage contained two perches of which one rested on micro-switches that were connected to a computer to measure perch-hopping activity (software developed by T&M Automation, Leidschendam, The Netherlands). For all calculations, we used 2 min intervals with binary information indicating whether birds were active or inactive (i.e., birds had landed or left a micro switch equipped perch). Using a 1,000 lux light (18 W Havells Sylvana Activa 172, East Sussex, UK) at perch level birds were entrained to a Light:Dark schedule (LD) (Supplementary Table 1) for 6 days. Light was turned off subsequently to initiate Dim:Dim (DD), i.e., the phase in which the birds received constant green dim light (Alecto ANV-17, 0.5 lux at perch level, see Helm and Visser, 2010; Spoelstra et al., 2018) for 14 days during which Tau was measured. We masked neighboring vocalizations and activity by playing white noise to prevent birds from influencing each other. Testing for neighboring effects using a Monte Carlo test showed that the birds did not influence each other (Supplementary Table 2). Food was available ad libitum and provided at random times between 08:00 AM and 14:00 PM when birds were in Dim:Dim to avoid entrainment on the feeding regime. All birds went through this experiment once and as such we obtained a single measurement per individual.
Tau was measured using the Sokolove and Bushell (1978) periodogram analysis using the software program Chronoshop v1.1. We calculated the onset phase of activity relative to lights on in entrained conditions according to the routine implemented in (Spoelstra et al., 2004) (onset phase from now on). Briefly, the onset phase is defined as the time difference between the start of activity which is detected as soon as the activity level in 2 min bins exceeds the average activity per 2 min bin. We subsequently assessed the phase of entrainment, which is defined by the moment the endogenous circadian system triggers activity in absence of time cues. We did this by measuring the time of activity onset directly after switching to DD conditions by backwards extrapolating onsets of activity of consecutive activity cycles in DD, using the Aschoff type II protocol (DD phase shift from now on) (Aschoff, 1965; Spoelstra et al., 2018).
Trait heritability of Tau, onset phase and DD phase shift was determined using two approaches, (i) using pedigree information and (ii) using the genomic marker relatedness between individuals. In approach (i) we calculated the heritability of the three traits for our dataset with an “animal model” (Henderson, 1986; Kruuk, 2004) based on the social pedigree, using a restricted estimated maximum likelihood approach in ASReml-R (Butler et al., 2009; Gilmour et al., 2009). In approach (ii) we replaced the pedigree with the genomic relatedness matrix (GRM) based on genomic markers using the relatedness matrix generated in Gienapp et al. (2019) to estimate heritability. The difference between the GRM and the relatedness matrix estimated from the pedigree is that the GRM is much less sparse; that is, it contains estimates of relatedness for any pair of individuals even if they are not directly related. The advantage, therefore, is that estimates of heritability can be more accurate than those obtained from pedigree-derived relatedness matrices, although at too small sample sizes of phenotyped individuals, sampling effects may exacerbate biases in estimates (Gienapp et al., 2017a).
We fitted an animal model for 152 individuals with values for Tau, onset phase, and DD phase shift. These values could not be assessed for 34 birds with insufficient activity in constant conditions (DD), and hence these birds were excluded. All traits were variance-standardized before analysis, to make variance components directly interpretable as proportion of variance explained. Fixed predictors initially included all two-way interactions between sex, selection line (early vs. late) and generation (F1, F2, or F3), as well as the observed light levels (Lux). Conditional Wald F tests (p < 0.05) were used to remove non-significant fixed effects. As random effects we included the inverse of the GRM to estimate additive genetic variation, as well as the identity of the mother to estimate maternal effects. The statistical significance of the random effects was tested using likelihood-ratio tests with 1 degree of freedom.
Since Tau is a trait with little (phenotypic) variation, heritability may not be the best measure for “evolvability” (Houle, 1992). We therefore also calculated a mean-standardized evolvability of Tau, rather than the usual variance-standardized measure that is heritability (Hansen et al., 2011). Following Houle (1992), the coefficient of additive genetic variation (CVA) is defined as CVA = , where X is the mean value of the trait. As a measure of evolvability, Hansen et al. (2011) recommend IA, i.e., the mean standardized additive genetic variance, which is equivalent to (CVA)2. IA can be interpreted as the percent expected change in the trait under a unit strength of selection. We calculated IA for the additive genetic variance components of both models (GRM- vs. pedigree based).
From the Tau measured birds, 156 individuals were genotyped with the Great Tit Affymetrix 650 k SNP chip (Kim et al., 2018). The GenABEL package v1.8-0 (Aulchenko et al., 2007) implemented in R v3.2.3 (R Development Core Team, 2015) was used to perform a quality control on the dataset. We first discarded 41 670 SNPs and six individuals, because of having a low call rate (< 95%). Furthermore, we discarded 60 210 SNPs having low minor allele frequency (MAF-threshold of 0.1). We did not exclude SNPs that deviated from Hardy-Weinberg equilibrium (HWE), because the circadian traits in our study populations might be under directional selection and causal loci may therefore deviate from HWE and low number of individuals from a selection line experiment might affect the HWE calculations. Because we use the same SNPs as in the study performed by Gienapp et al. (2017b), in which the genome-wide association between SNPs and timing of reproduction was assessed in wild Dutch great tits, we extracted the allele frequencies and HWE p-values from this study for further analysis. The final set of SNPs and individuals was 436, 113, and 150, respectively. We also calculated genomic relatedness, based on IBS, for all individuals across all SNPs and a genetic distance from this using GenABEL. This genetic distance matrix was then used, for multi-dimensional scaling (MDS) to identify whether there was clustering of genetically similar individuals. This showed substructures caused by the two breeding lines of late and early birds (Supplementary Figure 2) and thus we used line as a covariate in the GWAS model below.
SNPs were tested for allelic association with each circadian trait using the “polygenic” and “GRAMMAR-gamma” functions in GENABEL, adjusting for kinship due to the presence of related individuals by fitting the internally calculated genome-wide kinship matrix as a random effect (Amin et al., 2007). We also added “selection line” (as mentioned above), batch and sex as covariates. A Bonferroni adjustment was applied to correct for multiple comparisons, and the threshold for genome-wide significance was set at a P-value < 1.15 × 10−7 (= 0.05/436 113 variants).
The identification of candidate genes was done using a literature search for genes involved in the circadian rhythm, sleep disruption and the free-running period across humans, mice, and birds. We selected 12 genes of interest: CLOCK, PER2, PER3, CRY1, CRY2, ARNTL, CSNK1E, NPAS2, ADCYAP1, RORa, AANAT, and NR1D1 (Supplementary Table 3).
These 12 genes were scanned for variation in indels and sequence repeats using 20 re-sequenced great tits from Europe (excluding birds from Oxford) extracted from Laine et al. (2016) with Geneious v9.1.5 (Kearse et al., 2012). From these sequences, 57 variation sites were selected for further screening, based on occurrence of variation in 20 birds, location in genes (coding/non-coding) and length of indel/repetitive sequence (Supplementary Table 3).
To test whether the variation sites in the candidate genes were linked to Tau, we sequenced 46 out of 57 variation sites using two sets of four birds with extreme Tau measurements (Supplementary Table 4). Set 1 was used at the start of the project. However, the amount of DNA and the DNA quality became too low so set 1 was replaced by set 2 for further testing (see which set was used for each primer pair in Supplementary Table 5). Eleven sites were eliminated using the criteria mentioned above or no primers could be designed within those regions. In total, 47 primer pairs (for one variation site two primer pairs) were designed using Geneious v9.1.5 (Supplementary Table 5). Primers were selected using the following criteria: fragment size of approximately 500 bp, GC content below 60%, melting temperature (Tm) of ~60°C and primer length of 20–26 bp. All primers were located by performing a NCBI BLAST search of the Parus major reference genome build 1.1 (https://www.ncbi.nlm.nih.gov/assembly/GCF_001522545.2).
PCR reactions were performed with a C1000 Touch Thermal Cycler (Bio-Rad, United States) in a 10 μl reaction volume using 1x PCR Master Mix (Promega, The Netherlands), MgCl2 25 mM, 3.3 pmol of each primer and 10–20 ng of genomic DNA. After initial denaturation at 94°C for 1.30 min, 35 cycles of 30 s at 94°C for denaturation, 45 s annealing at 62°C and 45 s extension at 72°C were completed, followed by a final extension of 1 min at 62°C and 5 min at 72°C. The size of the products and specificity of the primers were determined by gel electrophoresis for 1 h at 90 V in a 1.5% agarose gel, washed with ethidium bromide and compared with a size standard (O'Gene ruler, DNA ladder mix). The PCR products were cleaned for sequencing with the QIAquick PCR purification Kit (Qiagen, The Netherlands). The samples (18 μl; approximately 10–20 ng DNA) and primers (20 μl; stock solution 100 μM) were sent for Sanger-sequencing (LGC Genomics, Berlin, Germany) using both the forward and reverse direction. The obtained sequences were checked with Geneious v9.1.5.
The variation in length polymorphism in ARNTL and CLOCK were based on sequence data. From the 46 sequenced variation sites, the gene ARNTL showed indel variation of four base pairs (ACAA) linking with Tau in the four sequenced individuals. To study this link further we sequenced for another 96 birds with Tau measurements to test the association. A 96-wells plate was sent for Sanger-sequencing (LGC Genomics, Berlin, Germany) containing 18 μl PCR product per well (cleaned by the company) and 20 μl stock solution of the reverse primer. This yielded in total of 100 birds with an ARNTL genotype length polymorphism and phenotypic trait measurements for circadian rhythm. We also sequenced 94 birds for the well-known polyglutamine repeat variation (poly-Q) in CLOCK (Darlington et al., 1998; Liedvogel and Sheldon, 2010). A 96-wells plate was sent for Sanger-sequencing (LGC Genomics, Berlin, Germany) containing 18 μl PCR product per well (cleaned by the company) and 20 μl stock solution of the reverse primer.
With capillary electrophoresis we further analyzed three candidate genes: RORa, CRY2, and PER3 which showed indel polymorphism for a minimum of three base pairs in the sequencing analysis (Supplementary Table 8). The analyses for an association with phenotypic traits were done in 100 individuals. Primers for length polymorphism were designed as described above, with labeling forward primer with fluorescent label, and also the reaction mix used was the same (Supplementary Table 5). The PCR reaction was performed in a C1000 Touch Thermal Cycler (Bio-Rad, United States). After initial denaturation at 94°C for 1.30 min, 40 cycles of 45 s annealing at 55°C, 45 s at 72°C and 30 s at 94°C were completed, followed by a final extension of 1 min at 55°C and 5 min at 72°C. To check specificity of the primers the PCR product was subjected to gel electrophoresis for 1 h at 5 V/cm in a 1.5% agarose gel, washed with ethidium bromide and compared with a size standard (O'Gene ruler, DNA ladder mix). The PCR products were diluted by adding 190 μl water to the PCR wells of RORa and 90 μl water to CRY2 and PER3. Fragment sizes were determined in an ABI3130 Genetic Analyzer (Applied Biosystems, Foster City, CA) with GeneScan 500 LIZ Size Standard (Applied Biosystems, Foster City, CA). Genotypes were quantified using the software GeneMapper 5.0 (Applied Biosystems, Foster City, CA). Statistical analyses were done with a general mixed model including the factors genotype (3 levels: deletion, heterozygote, insertion), line and sex and batch as a random effect. The primers designed to analyse length polymorphisms in RORa, CRY2, and PER3 showed the two peaks of the expected size based on the DNA sequence in the four sequenced birds (Supplementary Table 8).
After careful assessment of the data, we could use activity measurements of 152 birds for final data analysis on activity parameters (Supplementary Table 1, Supplementary Figure 1). Tau was significantly shorter than 24 h (mean ± SD:23.70 ± 0.16; range: 23.40–24.27 h; t154 = 1,856.3, p < 0.001). Tau was, on average, significantly shorter in females (mean: 23.65) than males (mean: 23.74) [difference 0.09 h, F(1, 148) = 11.680, p = 0.001] and the DD phase shift was also earlier in males (mean: −1.238 h) compared to females (mean: −1.068 h) [difference 0.17 h, F(1, 148) = 6.319, p = 0.013, Table 1]. Batch also significantly affected the DD phase shift where the earliest shift is found in batch 3 (mean: −1.768 h) and the latest found in batch 5 (mean: −0.0131 h) (Table 1). No significant effect was found for batch or selection line on Tau and selection line did not have a significant effect on onset phase or DD phase shift either. No sex or batch effect could be found on onset phase. Tau positively correlated with DD phase shift but no other trait correlation was found (Supplementary Table 6).
The heritabilities of the measured circadian traits are summarized in Table 2 and in more details in Supplementary Table 7. Using an “animal” model approach based on pedigree information the heritability of Tau was estimated to be h2 = 0.54 (±0.23; χ2 = 5.73, df = 1, p = 0.017). Variation associated with maternal effects was estimated at 0.23 (±0.12; χ2 = 5.81, df = 1, p = 0.016). In comparison, using the GRM approach, we found a heritability of 0.48 (±0.22; χ2 = 5.73, df = 1, p = 0.017) in Tau. Variation associated with maternal effects was estimated at 0.27 (±0.13; χ2 = 7.6, df = 1, p = 0.006). In onset phase the heritability was about the half of the Tau's heritability but not significant (Table 2). The DD phase shift was not heritable.
Table 2. Estimates and standard errors for the variance components explaining variation in Tau, onset phase, and DD phase shift.
Despite being moderately strongly heritable, evolvability of Tau was only marginal (for the pedigree and GRM analysis, respectively: IA = 2.52*10−5 and 2.20*10−5).
None of the SNPs were associated with any of the measured traits at the genome-wide significance level (Table 3, Supplementary Figure 3) and there was no indication of inflation (Supplementary Figure 4). Of the most significant SNPs (n = 15 in total for the three traits) nine were located within a gene other than the candidate genes (Table 3).
Table 3. The five most significant SNPs per trait for genome-wide association analyses testing for an interaction between SNP effects and activity traits, ordered by significance.
For seven variation sites in five genes, the sequences across the four sequenced birds were identical. Altogether 34 variation sites in nine genes did show variation across the four sequenced birds, these were not linked to the measured phenotypes since the variation occurred in either one, three, or all of the reference birds (Supplementary Table 3). However, variation in length of five sequenced sites in the genes ARNTL, CRY2, PER3, and RORa, did correlate with long or short Tau and were therefore further tested (Supplementary Table 3).
We found a significant relation between the onset phase and variation in ARNTL [F(2, 91.6) = 4.69; P = 0.012] (Figure 1, Supplementary Table 9). The great tits in our population were monomorphic for CLOCK poly-Q in all of the sequenced individuals. There were no significant associations between the phenotypic traits and sequence length polymorphisms in rest of the genes of interest (RORa, CRY2, PER3) when looking at all the genotyped birds (Figure 1, Supplementary Table 9). Note that differences between genotype groups are small, as the axis for Tau for example spans approximately 10 min.
Figure 1. Three phenotypic traits of rhythmicity plotted against the three genotypes (deletion, heterozygote, insertion) of the four candidate genes. Plotted values are estimated means ± standard error from the statistical model. N indicates the number of individuals.
Our results demonstrate that Tau is significantly heritable in our natural bird population, albeit (non-significantly) lower than in a previous study done with great tits (where the h2 was 0.86 ± 0.24; Helm and Visser, 2010) and with low evolvability (IA). Furthermore, onset phase and DD phase shift were not heritable in our birds although showing slight positive correlation with Tau. Given this heritable variation in Tau it is well possible that there are evolutionary forces acting on variation in Tau in the wild, but to assess this we need to obtain Tau phenotypes of wild birds and associate these with fitness. The aim of our study was to determine this via genetic associations between genotypic and phenotypic variation. However, we found no significant associations, neither on the genome-wide or candidate gene level between genetic variation and our Tau phenotypes. Overall, our results suggest that the molecular mechanism underlying differences in circadian timing in great tits lies somewhere else than in the genetic polymorphisms we studied. Furthermore, the measured traits could be also highly polygenic where they are controlled by many loci of small effect throughout the genome making the studying of the genomic background difficult. Thus, predicting phenotypes from these genotypes might not be feasible in this species with the current datasets available.
There are a number of potential reasons why we did not find a clear genomic signal underlying the variation in circadian traits. We only had a limited number of individuals with a known phenotype (152), but especially for the GWAS an additional problem is that these birds were from a selective breeding scheme and thus had a high relatedness. Moreover, the variation in phenotypes was relatively small. Combined, this limits the power of the analysis. However, there was ample genetic variation as is shown in the heritability analysis. So, it remains puzzling why typical clock genes did not associate with the trait, in either the GWAS or the candidate gene approach. The genes that came out strongest in the GWAS—albeit not significantly—were not related to known internal clock functions. Among the candidate genes, ARNTL was found to be associated only with one trait, onset phase and this association could be studied more in the future. However, candidate gene studies are known to have limitations especially when working with a small sample size and possible biases can occur for example through population structuring and these studies are not able to find novel gene associations or regulatory effects with the trait. Thus, future candidate gene studies should have bigger sample size and preferable birds from several populations.
One of our candidate genes was CLOCK, which has a glutamine-rich region that is highly conserved and of variable length in different species (Darlington et al., 1998; Saleem et al., 2001) but highly homogeneous within different bird species (Fidler and Gwinner, 2003). In blue tits (Cyanistes caeruleus) there is a latitudinal cline in mean poly-Q CLOCK repeat length with longer alleles associated with higher latitudes but not in bluethroats (Luscinia svecica) (Johnsen et al., 2007). Similar pattern was also found in Chinook salmon (Oncorhynchus tshawytscha) (O'Malley and Banks, 2008). However, low variability at the poly-Q locus has been found in great tits in Wytham woods and the spatial distribution of CLOCK alleles across Wytham was highly homogenous. Furthermore, there was no evidence for a connection between CLOCK genotype and reproductive timing phenotype in female great tits and no effect of CLOCK genotype on reproductive success (Liedvogel and Sheldon, 2010). We also observed a low variability of the same poly-Q region in our samples and could not link it to any of our measured circadian traits. These results from both UK and Dutch great tit populations are thus in contrast to the pattern found for the blue tit population, suggesting that phenotypic effects of CLOCK are not general in passerine birds.
As there is significant genetic variation in clock properties, we would expect some representation at the genomic level. However, post-transcriptional modification processes may play an important role. The importance of alternative splicing in circadian processes has been shown both in animals and plants (Sanchez et al., 2010; Petrillo et al., 2011). Furthermore, epigenetic modifications are also important in controlling circadian rhythmicity. There is strong evidence for histone oscillations in the circadian clock feedback loop (Katada and Sassone-Corsi, 2010; Koike et al., 2012). However, the role of cytosine modification in the circadian clock is unclear but recently it has been shown in mice that epigenetically variable cytosines show a circadian pattern (Oh et al., 2018). Combining transcriptomics and epigenetics would possibly reveal interesting patterns in the great tits as well and is an interesting path to explore in future studies.
In summary, we showed moderate heritability for Tau but not for the other traits. Among the genotyped samples there was no clear association between phenotype and genetic polymorphism. Our data suggest that in great tits the genetic background is more complex or lies somewhere else not measured here or our dataset was too small. This thus prevents the phenotyping of wild individuals from their genome, and thereby hampers the studies on “wild clocks” (Kronfeld-Schor et al., 2013). Other techniques, like the fibroblast methodology (Yagita et al., 2001), may help overcome this as studying how clocks function in the natural environment, including the selection operating on them, is essential for our understanding of how circadian rhythms can adapt to a changing world.
This study was carried out in accordance with the recommendations of Animal Experimentation Committee (DEC), Amsterdam, The Netherlands. The protocol was approved by the DEC, protocol NIOO 14.10 addendum 1.
MV and KvO designed the study. IV performed the experiments, with the assistance of PV. VL conducted the GWAS analysis and supervised with CM the candidate gene approach done by EA. JR calculated the heritability of the traits. VL, MV, and KS wrote the manuscript with the help of the other co-authors. All co-authors commented on the manuscript.
This study was supported by an ERC Advanced Grant (339092—E-response to MV). Funding agency played no role in the design, execution, and analysis of the study.
The authors declare that the research was conducted in the absence of any commercial or financial relationships that could be construed as a potential conflict of interest.
We thank Marylou Aaldering, Coretta Jongeling, Franca Kropman, Anouk de Plaa, and Ruben de Wit for taking care of the birds and facilities. Also, Amrit Knoppers and Bart van Lith for building the experimental setup and Gilles Wijlhuizen and Jeroen Laurens for technical assistance prior and during the experiments.
The Supplementary Material for this article can be found online at: https://www.frontiersin.org/articles/10.3389/fevo.2019.00152/full#supplementary-material
Amin, N., van Duijn, C. M., and Aulchenko, Y. S. (2007). A genomic background based method for association analysis in related individuals. PLoS ONE 2:e1274. doi: 10.1371/journal.pone.0001274
Aschoff, J. (1960). Exogenous and endogenous components in circadian rhythms. Cold Spring Harb. Symp. Quant. Biol. 25, 11–28.
Aschoff, J. (1965). “Response curves in circadian periodicity,” in Circadian Clocks, ed Aschoff, J. (Amsterdam: North-Holland Publishing), 95–111.
Aschoff, J. (1989). Temporal orientation: circadian clocks in animals and humans. Anim. Behav. 37, 881–896. doi: 10.1016/0003-3472(89)90132-2
Aschoff, J., and Wever, R. (1966). Circadian period and phase-angle difference in chaffinches (Fringilla coelebs L.). Comp. Biochem. Physiol. 18, 397–404. doi: 10.1016/0010-406X(66)90197-6
Aulchenko, Y. S., Ripke, S., Isaacs, A., and van Duijn, C. M. (2007). GenABEL: an R library for genome-wide association analysis. Bioinformatics 23, 1294–1296. doi: 10.1093/bioinformatics/btm108
Bell-Pedersen, D., Cassone, V. M., Earnest, D. J., Golden, S. S., Hardin, P. E., Thomas, T. L., et al. (2005). Circadian rhythms from multiple oscillators: lessons from diverse organisms. Nat. Rev. Genet. 6, 544–556. doi: 10.1038/nrg1633
Brown, S. A., Kowalska, E., and Dallmann, R. (2012). (Re)inventing the circadian feedback loop. Dev. Cell 22, 477–487. doi: 10.1016/j.devcel.2012.02.007
Butler, D., Cullis, B. R., Gilmour, A. R., and Gogel, D. J. (2009). ASReml-R Reference Manual, Release 3.0. Department of Primary Industries and Fisheries.
Daan, S. (1981). “Adaptive Daily Strategies in Behavior,” in Handbook of Behavioral Neurobiology; Biological Rhythms, ed Aschoff, J. (New York, NY: Plenum Press), 275–298.
Darlington, T. K., Wager-Smith, K., Ceriani, M. F., Staknis, D., Gekakis, N., Steeves, T. D., et al. (1998). Closing the circadian loop: CLOCK-induced transcription of its own inhibitors per and tim. Science 280, 1599–1603.
DeCoursey, P. J., Walker, J. K., and Smith, S. A. (2000). A circadian pacemaker in free-living chipmunks: essential for survival? J. Comp. Physiol. A 186, 169–180. doi: 10.1007/s003590050017
Derks, M. F. L., Schachtschneider, K. M., Madsen, O., Schijlen, E., Verhoeven, K. J. F., and van Oers, K. (2016). Gene and transposable element methylation in great tit (Parus major) brain and blood. BMC Genomics 17:332. doi: 10.1186/s12864-016-2653-y
Dominoni, D. M., Goymann, W., Helm, B., and Partecke, J. (2013). Urban-like night illumination reduces melatonin release in European blackbirds (Turdus merula): implications of city life for biological time-keeping of songbirds. Front. Zool. 10:60. doi: 10.1186/1742-9994-10-60
Fidler, A. E., and Gwinner, E. (2003). Comparative analysis of Avian BMAL1 and CLOCK protein sequences: a search for features associated with owl nocturnal behaviour. Comp. Biochem. Physiol. B Biochem. Mol. Biol. 136, 861–874. doi: 10.1016/S1096-4959(03)00276-8
Gienapp, P., Calus, M. P. L., Laine, V. N., and Visser, M. E. (2019). Genomic selection on breeding time in a wild bird population. Evol. Lett. 3, 142–151. doi: 10.1002/evl3.103
Gienapp, P., Fior, S., Guillaume, F., Lasky, J. R., Sork, V. L., and Csilléry, K. (2017a). Genomic quantitative genetics to study evolution in the wild. Trends Ecol. Evol. 32, 897–908. doi: 10.1016/j.tree.2017.09.004
Gienapp, P., Laine, V. N., Mateman, A. C., van Oers, K., and Visser, M. E. (2017b). Environment-dependent genotype-phenotype associations in avian breeding time. Front. Genet. 8:102. doi: 10.3389/fgene.2017.00102
Gilmour, A. R., Gogel, B. J., Cullis, B. R., and Thompson, R. (2009). ASReml User Guide Release 3.0. VSN International.
Hansen, T. F., Pélabon, C., and Houle, D. (2011). Heritability is not evolvability. Evol. Biol. 38, 258–277. doi: 10.1007/s11692-011-9127-6
Helm, B., and Visser, M. E. (2010). Heritable circadian period length in a wild bird population. Proc. R. Soc. B Biol. Sci. 277, 3335–3342. doi: 10.1098/rspb.2010.0871
Henderson, C. R. (1986). Estimation of variances in animal model and reduced animal model for single traits and single records. J. Dairy Sci. 69, 1394–1402. doi: 10.3168/jds.S0022-0302(86)80546-X
Hida, A., Ohsawa, Y., Kitamura, S., Nakazaki, K., Ayabe, N., Motomura, Y., et al. (2017). Evaluation of circadian phenotypes utilizing fibroblasts from patients with circadian rhythm sleep disorders. Transl. Psychiatry 7, 1–5. doi: 10.1038/tp.2017.75
Horst, G. T. J., Van, D., Muijtjens, M., Kobayashi, K., Takano, R., Kanno, S., Takao, M., et al. (1999). Mammalian Cry1 and Cry2 are essential for maintenance of circadian rhythms. Nature 398, 627–630. doi: 10.1038/19323
Houle, D. (1992). Comparing evolvability and variability of quantitative traits. Genetics 130, 195–204.
Hurd, M. W., and Ralph, M. R. (1998). The significance of circadian organization for longevity in the golden hamster. J. Biol. Rhythms 13, 430–436.
Johnsen, A., Fidler, A. E., Kuhn, S., Carter, K. L., Hoffmann, A., Barr, I. R., et al. (2007). Avian Clock gene polymorphism: evidence for a latitudinal cline in allele frequencies. Mol. Ecol. 16, 4867–4880. doi: 10.1111/j.1365-294X.2007.03552.x
Johnson, C. H. (2005). Testing the adaptive value of circadian systems. Methods Enzymol. 393, 818–837. doi: 10.1016/S0076-6879(05)93043-7
Katada, S., and Sassone-Corsi, P. (2010). The histone methyltransferase MLL1 permits the oscillation of circadian gene expression. Nat. Struct. Mol. Biol. 17, 1414–1421. doi: 10.1038/nsmb.1961
Kearse, M., Moir, R., Wilson, A., Stones-Havas, S., Cheung, M., Sturrock, S., et al. (2012). Geneious Basic: an integrated and extendable desktop software platform for the organization and analysis of sequence data. Bioinformatics 28, 1647–1649. doi: 10.1093/bioinformatics/bts199
Kim, J.-M., Santure, A. W., Barton, H. J., Quinn, J. L., Cole, E. F., Great Tit HapMap Consortium, et al. (2018). A high-density SNP chip for genotyping great tit (Parus major) populations and its application to studying the genetic architecture of exploration behaviour. Mol. Ecol. Resour. 18, 877–891. doi: 10.1111/1755-0998.12778
Koike, N., Yoo, S.-H., Huang, H.-C., Kumar, V., Lee, C., Kim, T.-K., et al. (2012). Transcriptional architecture and chromatin landscape of the core circadian clock in mammals. Science 338, 349–354. doi: 10.1126/science.1226339
Kronfeld-Schor, N., Bloch, G., and Schwartz, W. J. (2013). Animal clocks: when science meets nature. Proc. R. Soc. B Biol. Sci. 280, 3–6. doi: 10.1098/rspb.2013.1354
Kruuk, L. E. B. (2004). Estimating genetic parameters in natural populations using the “animal model.” Philos. Trans. R. Soc. B Biol. Sci. 359, 873–890. doi: 10.1098/rstb.2003.1437
Laine, V. N., Gossmann, T. I., Schachtschneider, K. M., Garroway, C. J., Madsen, O., Verhoeven, K. J. F., et al. (2016). Evolutionary signals of selection on cognition from the great tit genome and methylome. Nat. Commun. 7:10474. doi: 10.1038/ncomms10474
Liedvogel, M., and Sheldon, B. C. (2010). Low variability and absence of phenotypic correlates of Clock gene variation in a great tit Parus major population. J. Avian Biol. 41, 543–550. doi: 10.1111/j.1600-048X.2010.05055.x
Lowrey, P. L., and Takahashi, J. S. (2011). Genetics of circadian rhythms in mammalian model organisms. Adv. Genet. 74, 175–230. doi: 10.1016/B978-0-12-387690-4.00006-4
Merrow, M., Spoelstra, K., and Roenneberg, T. (2005). The circadian cycle: daily rhythms from behaviour to genes. EMBO Rep. 6, 930–935. doi: 10.1038/sj.embor.7400541
Oh, G., Ebrahimi, S., Carlucci, M., Zhang, A., Nair, A., Groot, D. E., et al. (2018). Cytosine modifications exhibit circadian oscillations that are involved in epigenetic diversity and aging. Nat. Commun. 9:644. doi: 10.1038/s41467-018-03073-7
O'Malley, K. G., and Banks, M. A. (2008). A latitudinal cline in the Chinook salmon (Oncorhynchus tshawytscha) Clock gene: evidence for selection on PolyQ length variants. Proc. R. Soc. B Biol. Sci. 275, 2813–2821. doi: 10.1098/rspb.2008.0524
Pagani, L., Semenova, E. A., Moriggi, E., Revell, V. L., Hack, L. M., Lockley, S. W., et al. (2010). The physiological period length of the human circadian clock in vivo is directly proportional to period in human fibroblasts. PLoS ONE 5:e13376. doi: 10.1371/journal.pone.0013376
Petrillo, E., Sanchez, S. E., Kornblihtt, A. R., and Yanovsky, M. J. (2011). Alternative splicing adds a new loop to the circadian clock. Commun. Integr. Biol. 4, 284–286. doi: 10.4161/cib.4.3.14777
Pittendrigh, C. S. (1993). Temporal organization: reflections of a Darwinian Clock-Watcher. Annu. Rev. Physiol. 55, 17–54.
Pittendrigh, C. S., and Minis, D. H. (1972). Circadian systems: longevity as a function of circadian resonance in Drosophila melanogaster. Proc. Natl. Acad. Sci. U S A. 69, 1537–1539.
R Development Core Team. (2015). R: A Language and Environment for Statistical Computing. Vienna: R Foundation for Statistical Computing.
Ralph, M., and Menaker, M. (1988). A mutation of the circadian system in golden hamsters. Science 241, 1225–1227.
Saleem, Q., Anand, A., Jain, S., and Brahmachari, S. K. (2001). The polyglutamine motif is highly conserved at the Clock locus in various organisms and is not polymorphic in humans. Hum. Genet. 109, 136–142. doi: 10.1007/s004390100550
Sanchez, S. E., Petrillo, E., Beckwith, E. J., Zhang, X., Rugnone, M. L., Hernando, C. E., et al. (2010). A methyl transferase links the circadian clock to the regulation of alternative splicing. Nature 468, 112–116. doi: 10.1038/nature09470
Santure, A. W., Gratten, J., Mossman, J. A., Sheldon, B. C., and Slate, J. (2011). Characterisation of the transcriptome of a wild great tit Parus major population by next generation sequencing. BMC Genomics 12:283. doi: 10.1186/1471-2164-12-283
Schaper, S. V., Dawson, A., Sharp, P. J., Caro, S. P., and Visser, M. E. (2012). Individual variation in avian reproductive physiology does not reliably predict variation in laying date. Gen. Comp. Endocrinol. 179, 53–62. doi: 10.1016/j.ygcen.2012.07.021
Schwartz, W. J., Helm, B., and Gerkema, M. P. (2017). Wild clocks: preface and glossary. Philos. Trans. R. Soc. B Biol. Sci. 372:20170211. doi: 10.1098/rstb.2017.0211
Shearman, L. P., Sriram, S., Weaver, D., Maywood, E., Chaves, I., and Zhang, B. (2000). Interacting molecular loops in the mammalian circadian clock. Science 288, 1013–1019. doi: 10.1126/science.288.5468.1013
Sokolove, P. G., and Bushell, W. N. (1978). The chi square periodogram: its utility for analysis of circadian rhythms. J. Theor. Biol. 72, 131–160.
Spoelstra, K., Albrecht, U., Van Der Horst, G. T. J., Brauer, V., and Daan, S. (2004). Phase responses to light pulses in mice lacking functional per or cry genes. J. Biol. Rhythms 19, 518–529. doi: 10.1177/0748730404268122
Spoelstra, K., Verhagen, I., Meijer, D., and Visser, M. E. (2018). Artificial light at night shifts daily activity patterns but not the internal clock in the great tit (Parus major). Proc. R. Soc. B Biol. Sci. 285:20172751. doi: 10.1098/rspb.2017.2751
Spoelstra, K., Wikelski, M., Daan, S., Loudon, A. S. I., and Hau, M. (2016). Natural selection against a circadian clock gene mutation in mice. Proc. Natl. Acad. Sci. U S A. 113, 686–691. doi: 10.1073/pnas.1516442113
Takahashi, J. S., Turek, F. W., and Moore, R. Y. (2001). “Circadian clocks,” in Handbook of Behavioral Neurobiology (Boston, MA: Springer US).
Van Bers, N. E. M., Santure, A. W., Van Oers, K., De Cauwer, I., Dibbits, B. W., Mateman, C., et al. (2012). The design and cross-population application of a genome-wide SNP chip for the great tit Parus major. Mol. Ecol. Resour. 12, 753–770. doi: 10.1111/j.1755-0998.2012.03141.x
Vitaterna, M. H., King, D. P., Chang, A. M., Kernhauser, J. M., Lowrey, P. L., McDonald, J. D., et al. (1994). Mutagenesis and mapping of a mouse gene, clock, essential for circadian behavior. Science 264, 719–725. doi: 10.1126/science.8171325
Keywords: circadian rhythm, clock, GWAS, heritability, Parus major
Citation: Laine VN, Atema E, Vlaming P, Verhagen I, Mateman C, Ramakers JJC, van Oers K, Spoelstra K and Visser ME (2019) The Genomics of Circadian Timing in a Wild Bird, the Great Tit (Parus major). Front. Ecol. Evol. 7:152. doi: 10.3389/fevo.2019.00152
Received: 10 January 2019; Accepted: 18 April 2019;
Published: 03 May 2019.
Edited by:
Guo-Bo Chen, Zhejiang Provincial People's Hospital, ChinaReviewed by:
Marta Barluenga, Spanish National Research Council (CSIC), SpainCopyright © 2019 Laine, Atema, Vlaming, Verhagen, Mateman, Ramakers, van Oers, Spoelstra and Visser. This is an open-access article distributed under the terms of the Creative Commons Attribution License (CC BY). The use, distribution or reproduction in other forums is permitted, provided the original author(s) and the copyright owner(s) are credited and that the original publication in this journal is cited, in accordance with accepted academic practice. No use, distribution or reproduction is permitted which does not comply with these terms.
*Correspondence: Veronika N. Laine, Vi5MYWluZUBuaW9vLmtuYXcubmw=
Disclaimer: All claims expressed in this article are solely those of the authors and do not necessarily represent those of their affiliated organizations, or those of the publisher, the editors and the reviewers. Any product that may be evaluated in this article or claim that may be made by its manufacturer is not guaranteed or endorsed by the publisher.
Research integrity at Frontiers
Learn more about the work of our research integrity team to safeguard the quality of each article we publish.