- 1Department of Aquatic Sciences and Assessment, Swedish University of Agricultural Sciences, Uppsala, Sweden
- 2School of Natural Resources, University of Nebraska - Lincoln, Lincoln, NE, United States
- 3U.S. Geological Survey - Nebraska Cooperative Fish & Wildlife Research Unit, University of Nebraska, Lincoln, NE, United States
- 4Department of Agronomy and Horticulture, University of Nebraska - Lincoln, Lincoln, NE, United States
- 5Department of Ecology, Evolution and Plant Sciences, Stockholm University, Stockholm, Sweden
It is well-recognized in plankton ecology that phytoplankton development can lead to distinct peaks (i.e., blooms) during spring and summer. We used a 5-year (2007–2011) phytoplankton data set and utilized discontinuity analysis to assess resilience attributes of spring and summer blooms based on the cross-scale resilience model. Using the size structure (i.e., cross-scale structure as an indicator of resilience) in the sampled plankton data, we assessed whether spring and summer blooms differ substantially between but not within blooms; that is, whether they comprise alternative community regimes. Our exploratory study supported this expectation and more broadly resilience theory, which posits that ecological systems can manifest in and change between alternative regimes. The dynamics of regimes receives increased attention because rapid environmental change potentially irreversibly alters ecosystems. Model organisms are needed that allow revealing patterns and processes of various aspects of regime dynamics at tractable time scales. Our preliminary findings suggest that phytoplankton can be suitable models for assessing the intricacies of regimes and regime changes.
Introduction
Patterns of discrete size structures of organisms in communities are related to a number of abiotic and biotic factors that operate across distinct scales of space and time; that is, they reflect the hierarchical organization of ecosystems (Nash et al., 2014; Sundstrom et al., 2018). This is due to competitive interactions and behavioral, life-history, and morphological adaptations to resources (e.g., food and shelter) that prevail at each scale (Holling, 1992; Segura et al., 2013). For instance, an elephant and an ant in a savannah interact with and exploit very different scales in the system. The discrete size structure within communities, also referred to as cross-scale structure (Sundstrom et al., 2018), has been suggested to serve as a surrogate of resilience in ecosystems (Angeler et al., 2016), and other complex systems (Sundstrom et al., 2014). This is due to the ability of one scale (discrete size structure) in the system being able to buffer against disturbances at other scales as a function of their functional trait characteristics and diversity (Angeler and Allen, 2016).
Resilience theory posits that ecological systems undergo substantial reorganization when they shift between alternative regimes (Angeler and Allen, 2016). Spanbauer et al. (2016) showed that the discrete size structure of benthic diatoms in Foy lake (USA) substantially changed as a result of a regime shift. This is consistent with predictions that cross-scale structure as an indicator of resilience can identify regimes and regime changes (Baho et al., 2017). Phytoplankton communities are both discontinuously structured (Segura et al., 2013; Downing et al., 2014) and they can undergo substantial seasonal reorganization (e.g., Sommer et al., 2012; Behrenfeld and Boss, 2014). For instance, in the Baltic Sea phytoplankton communities show multiple seasonal peaks (“blooms”). These blooms have been shown to undergo repeated cycles of reorganization and collapse, which from a resilience perspective suggest community-level regime shifts (Angeler et al., 2015). However, it is still unclear if these dynamics reflect the manifestation of and change between alternative regimes posited by resilience theory. Therefore, research is needed to address this question, especially to shed light on potential similarities and differences between resilience-based approaches and taxonomic studies that have dominated research agendas in ecology in the past.
Phytoplankton is potentially a useful model for studying alternative community regimes because they have fast turnover, communities have high species diversity, and they show complex system dynamics (Leibold and Norberg, 2004). This behavior is influenced by feedbacks that emanate from interacting biotic (trophic cascades and competition) and abiotic (stratification, nutrients, temperature) variables (Sommer et al., 1986; Leibold and Norberg, 2004). These dynamics are well-documented in ecology, particularly ecological succession, and plankton ecology (Clements, 1916; Sommer et al., 2012). These paradigms are therefore valuable for assessing alternative community regimes, based on the cross-scale structure present in the study system. Phytoplantkon communities develop into spring and summer blooms and in relation to a spring clear-water phase (Sommer et al., 1986; Hajdu, 2002), although these patterns can vary among ecosystems (Jaanus et al., 2011). In our study system, the Baltic Sea, spring blooms develop from March to May and summer blooms occur between June and August (Angeler et al., 2015). These blooming periods are stable and recur annually, although there is annual variation in bloom timing. This timing provides an opportunity to assess whether spring and summer blooms comprise alternative phytoplankton community regimes.
In the Baltic Sea, spring blooms are the result of several factors. Among those are seasonal increases in temperature and solar radiation. Temperature and saline gradients that lead to stratification after winter mixing can also be important. At the beginning, spring blooms, which are characterized by high biomass, are dominated by diatoms that grow fast and later replaced by dinoflagellates with slower growth. With increasing temperature increase and nutrient depletion, spring blooms collapse, and reorganize in species-rich summer blooms with many inedible flagellates and cyanobacteria (Angeler et al., 2015). This reorganization is partly the result of changing food webs that alter biotic feedbacks; specifically, top-down effects, including zooplankton grazing that shapes the dynamics of phytoplankton (Sommer et al., 1986, 2012). In addition, Baltic Sea phytoplankton shows variability in taxonomic structure and the phenology of blooms (Jaanus et al., 2011; Klais et al., 2011; Suikkanen et al., 2013), which results from the interaction of anthropogenic (eutrophication, overfishing), climatic, and biotic factors (Elmgren, 1989; Wasmund and Uhlig, 2003; Österblom et al., 2007). Complex interactions between winter temperature and nutrient dynamics further affect community responses during spring and summer (Janssen et al., 2004).
The seasonally recurring spring and summer blooms that reflect phytoplankton dynamics allow for assessing assumptions regarding community dynamics during different successional stages. In this exploratory study, we assess cross-scale structure in spring and summer phytoplankton blooms to test the following expectations:
Phytoplankton blooms across seasons represent alternative regimes, reflected in different cross-scale structure, because of differing abiotic and biotic conditions between both bloom seasons.
During the duration of individual blooms, cross-scale structure between sampling events should not be significantly different as a result of the intrinsic regime properties that organize community dynamics within each bloom.
Materials and Methods
Ethics Statement
All field and laboratory work is approved by the Swedish Agency for Marine and Water Management (HaV) and are part of the Baltic Sea Monitoring Program. Data used in this study are available through the Swedish Meteorological and Hydrological Institute (SMHI) and the Dryad Digital Repository (http://doi.org/10.5061/dryad.8hj8t). No endangered or protected species were involved in this study.
Sites and Sampling
Phytoplankton communities were assessed at the 40 m deep B1 station near the coast of Askö (58°48′ N, 17°38′ E) and at the 459 m deep BY31 offshore station at Landsort Deep (58°35.90′ N, 18°14.21′ E). These sites are located in the southern area of the Baltic Sea, specifically in the NW Baltic Proper. Data were collected from both stations in weekly to fortnightly intervals in spring (March–May) and summer (June–August) between 2007 and 2011. Sampling and analysis adhered to standardized protocols. Phytoplankton samples were taken as integrated samples with a sampling hose (inner diameter 19 mm) from 0 to 20 m and preserved with acid Lugol's solution (Willén, 1962). In this way we could integrate abiotic and biotic heterogeneity in the water column and more accurately capture bloom aspects over the spring and summer. Taxonomic experts carried out the evaluation of phytoplankton adhering to standard protocols. Briefly, an inverted microscope with phase contrast was used to count phytoplankton (>2 um) after sedimentation in 10- or 25-mL chambers. Cells were measured and their sizes classified following HELCOM (2008). Carbon content was calculated from the biovolume of all individuals of a species, including colonial taxa. These evaluations were carried out following Olenina et al. (2006) and standardized volumes (http://www.ices.dk/marine-data/vocabularies/Documents/PEG_BVOL.zip).
Statistical Analyses
We used discontinuity analysis for assessing the cross-scale structure present in the phytoplankton communities (Barichievy et al., 2018). The evaluation of cross-scale structure has been originally based on animal body sizes (Holling, 1992). More recently it has been extended to a broader discontinuity framework that accounts for abundances in ecological studies (Angeler et al., 2014; Sundstrom et al., 2018) and that accommodates metrics from non-ecological systems (e.g., city size: Garmestani et al., 2008). There is also evidence that plankton studies based on body mass of single species and biovolume of populations give similar results (Baho et al., 2015). In this study we used volumetric data and assessed cross-scale structure in the carbon content of phytoplankton using Bayesian Classification and Regression Trees (BCART) (Chipman et al., 1998). Phytoplankton carbon content was rank ordered based on ascending log-transformed measures of individual populations in the communities. Rank-ordered matrices were created for each phytoplankton community at each sampling date during the study period. BCART was conducted individually on these matrices to identify biomass groups in the phytoplankton community data for each sampling date by assessing within-group homogeneity (Stow et al., 2007). The analysis was based on a million iterations repeated 25 times. From this universe of trees the best 20 were displayed and the tree with the best (highest) log-likelihood ratio was selected for further analysis. The trees branch into distal nodes that comprise groups with highest homogeneity. In our study, these homogeneity groups are composed of phytoplankton populations that differ between groups in terms of their homogeneities in carbon content, and they likely emerge from the patterns-process relationships present within each bloom regime (Holling, 1992; Allen et al., 2005; Angeler et al., 2012). That is, the homogeneity groups identified by the BCART trees were used for classifying the phytoplankton populations into aggregation groups, thereby determining the cross-scale structure in the community. These homogeneity or aggregation groups were used to calculate nine diagnostics of phytoplankton cross-scale structure in carbon content for further analysis. These diagnostics are rooted in and therefore represent individually and collectively the cross-scale resilience model (Peterson et al., 1998), a commonly applied tool for quantifying resilience (Angeler and Allen, 2016). These diagnostics are: (1) total number of aggregation groups for the phytoplankton community at each sampling date, (2) the average of their aggregation group lengths (each aggregation length was measured as the difference between the lowest and highest log-transformed carbon content of a specific aggregation group), (3) averages of gap lengths (each gap length was measured as the difference between the log-biomass value of edges between adjacent aggregation groups), (4) standard deviation of aggregation lengths within a community as a variability measure of aggregation lengths in the community, (5) standard deviations of gap lengths, (6) average number of phytoplankton species composing carbon content aggregation groups of each analysis, (7) standard deviation of species composing aggregation groups, (8) lowest carbon content, and (9) highest carbon content values for each community were used for bounding the other diagnostics according to carbon content ranges of the phytoplankton communities.
Permutational multivariate ANOVA (PERMANOVA) (Anderson, 2005) was used to contrast the diagnostics of phytoplankton biomass cross-scale structure following the design of Angeler et al. (2015). The PERMANOVA model had three main terms. Factor 1 (Blooms) was fixed and contrasted blooms between spring and summer based on average cross-scale structure of phytoplankton. Factor 2 (Years) was random and categorical and comprised the study years from 2007 to 2011. Factor three (Months nested in years) was also random and comprised within-bloom dynamics during spring (March, April, May) and summer (June, July, August). Interaction terms between these factors were also tested. Three terms allowed assessing whether phytoplankton communities organize in distinct spring and summer community regimes in the Baltic Sea under our study period: (1) The term “Blooms” tests if phytoplankton cross-scale structure differs between spring and summer. This term is expected to be significant if spring and summer blooms comprise alternative community regimes. (2) The interaction term “Blooms × Months (Years)” assesses dynamic change of cross-scale structure within blooms. Because individual blooms are considered alternative community regimes, cross-scale structure between sampling events should not be significantly different due to regime-inherent properties that steer community dynamics within each bloom (Angeler et al., 2015). (3) The interaction term “Blooms × Years” tests if diagnostics of cross-scale structure are stable during spring and summer over the study years. If phytoplankton organizes in different spring and summer alternative community regimes consistently over time this term is not expected to be significant. PERMANOVA was calculated on Euclidean distance matrix of standardized diagnostics of phytoplankton biomass cross-scale structure using the coastal and offshore sites as replicates. Nine thousand nine hundred and ninety-nine unrestricted permutations of raw data were used and calculations were carried out with PERMANOVA v1.6 (Anderson, 2005). Significance testing was based on Monte Carlo asymptotic P-values.
Results
Phytoplankton community cross-scale structure showed substantial variation between and within blooms between 2007 and 2011 at the coastal and offshore site in the Baltic Sea (Figure 1). Examining visually individual resilience metrics used for analysis revealed subtle differences between spring and summer blooms, with the exception of the variation (standard deviation) in the length of aggregations (i.e., the difference between the lowest and highest log-transformed biomass of a specific aggregation group), which was higher in summer compared to spring blooms (Figure 2). Despite the apparent similarities observed in individual metric comparisons and the variability in the data set, PERMANOVA detected a significant “Blooms” effect (Table 1). This suggests that phytoplankton cross-scale structure may reorganize in alternative community regimes between spring and summer. The effect of “Years” (study period between 2007 and 2011) was not significant, highlighting that the phytoplankton cross-scale structure of blooms is not changing during the study period. The insignificant interaction term “Blooms × Years” highlights that phytoplankton cross-scale structure present during spring and summer blooms remains differentiated over the study period. Finally, the term “Blooms × Months (Years)” was not significant. This suggests similar cross-scale structure between sampling events within each regime.
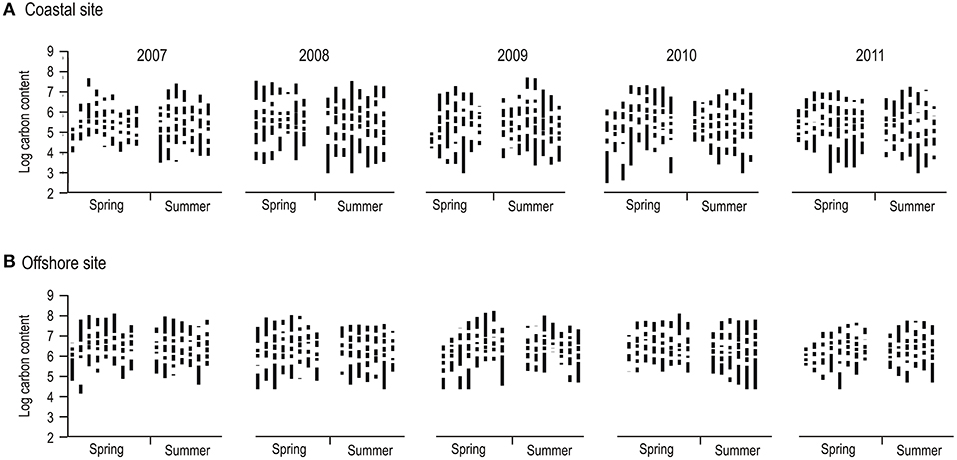
Figure 1. Phytoplankton cross-scale structure during spring and summer blooms at a coastal (A) and offshore (B) site between 2007 and 2011 in the Baltic Sea. Shown are stacked biomass aggregation groups (black bars; ranges from lowest to highest biomass within a group) separated by gaps (white bars; no biomass present between aggregation groups) during repeated (8–9) sampling events within blooms.
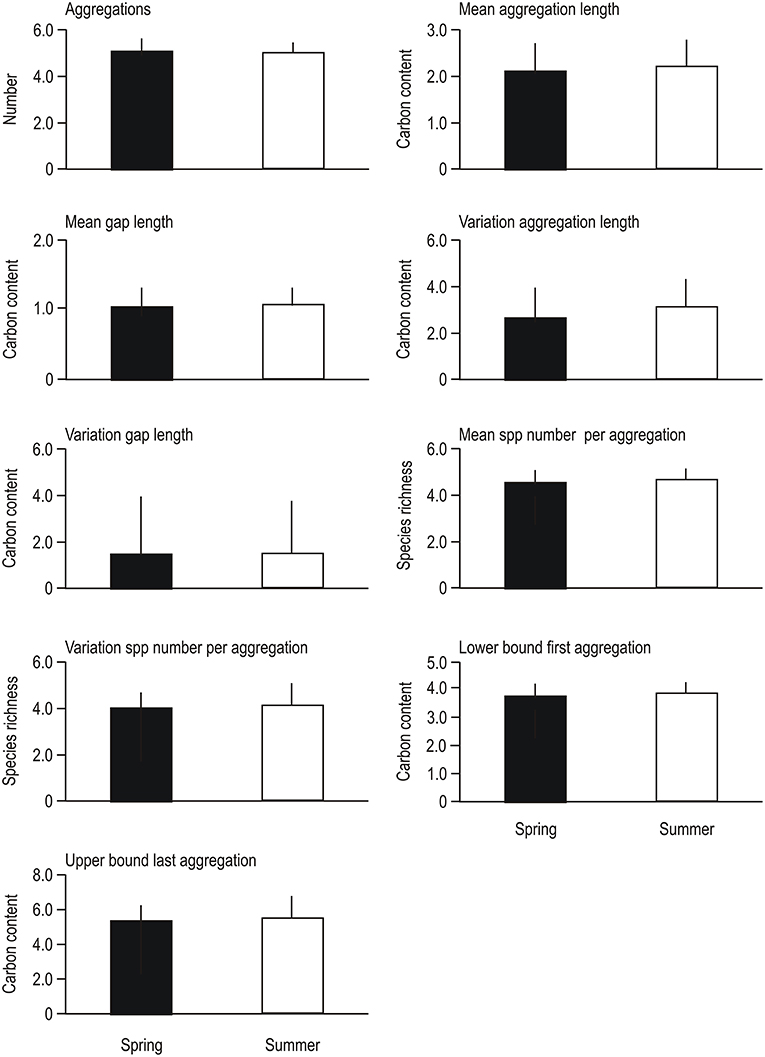
Figure 2. Comparison of resilience metrics based on the cross-scale resilience model used for analysis. Shown are the means and standard deviations from 3 yearly measurements for each site (coastal and offshore) for the study period 2007–2011 (n = 36). See section Materials and Methods for descriptions and calculations of these metrics.
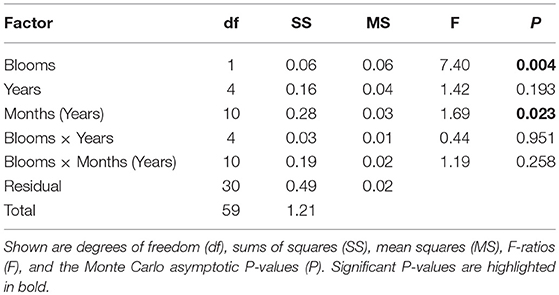
Table 1. Results of PERMANOVA analysis contrasting multivariate cross-scale structure across blooms (averaged spring and summer blooms of phytoplankton), years (2007–2011), months nested in years (3 months comprising each bloom), and their interactions.
Discussion
The results of our exploratory study show that phytoplankton seasonal development reflects the organization into alternative regimes, consistent with ecological resilience theory. This finding aligns with a previous study that assessed patterns of bloom collapse and reorganization following the adaptive cycle (Angeler et al., 2015), a heuristic of complex system change (Holling, 1986; Gunderson and Holling, 2002). The results are also in agreement with a plethora of taxonomic studies in marine (Edwards and Richardson, 2004; Lindemann and St John, 2014; Vidal et al., 2017) and freshwater (Sommer, 1985; Munawar and Munawar, 1986; Reynolds, 2006) environments that have documented substantial community changes in terms of phytoplankton species composition and biomass as a result of abiotic variability.
Although our results need to be interpreted with caution due to low sample size and heterogeneity of sites, the significant “blooms” term in the PERMANOVA model preliminarily supports the interpretation that spring and summer blooms generally comprise alternative phytoplankton community regimes. Alternative regimes are usually associated with ecosystem dynamics (e.g., Beisner et al., 2003); however, alternative regimes at the community level, which are often transient, have also been documented (Fukami and Nakajima, 2011; Jiang et al., 2011). Such changes can occur when variability at the ecosystem level creates abiotic and biotic conditions that entail a substantial restructuring at lower hierarchical levels in the system; i.e., at the scale of ecological communities (Allen et al., 2014). Such reorganization was the case in our study. Although the Baltic Sea is considered to operate in a stable eutrophic regime (Yletyinen et al., 2017), the substantial seasonal changes in the abiotic and biotic environment can lead to a transitioning of phytoplankton communities between alternative regimes. In the Baltic Sea, abiotic changes are related to temperature, nutrients, stratification, and salinity (Angeler et al., 2015). Biotic changes are manifested in alterations of food webs; i.e., trophic cascades including zooplankton grazing affect the dynamics of phytoplankton assemblages (Winder and Cloern, 2010; Sommer et al., 2012; Behrenfeld and Boss, 2014).
Most phytoplankton successional studies are based on taxonomic analyses. These studies show high community turnover between spring and summer blooms and also a high replacement of species and major taxonomic groups from the onset to the end of blooms (Reynolds, 2006). For instance, in the Baltic Sea dinoflagellates replace initially dominant diatoms toward the end of spring blooms. Similarly, summer blooms are characterized by a dynamic replacement between cyanobacteria, cryptophytes, and dinoflagellates (Angeler et al., 2015). These changes are due to functional attributes of phytoplankton species with some species (diatoms) being r-strategists; that is, they grow fast when nutrient availability is high (Sommer, 1981). On the other hand there are some species that are K-strategists, which characterize slow growing species (dinoflagellates) that are competitively weaker compared to diatoms, and that become abundant during periods of low nutrient availability (Sommer, 1981; Lembi and Waaland, 2007).
Using cross-scale structure in the carbon content of phytoplankton populations in the discontinuity analysis rather than taxonomic information, our results show an important difference between both approaches. A previous taxonomy based study using the same data set, which thus allows for direct comparisons, found significant within-bloom variability in phytoplankton community composition (Angeler et al., 2015). However, such an effect was not detected using the cross-scale structure of carbon content in this study. Both findings are not mutually exclusive. Taxonomic studies capture the pronounced abiotic and biotic variability within blooms while cross-scale structure as a measure of system resilience provides a more conservative, systemic measure of relative stability, and persistence of a regime. In this context stability and persistence are defined as the regime dynamics that are bound within a basin of attraction (Angeler and Allen, 2016). Specifically, alternative regimes are characterized by patterns-process relationships and feedbacks that are relatively stable (Beisner et al., 2003), although variability occurs within a regime when they adapt and cope with disturbances (Gunderson, 2000; Angeler et al., 2019). Such variability is clearly evident in the dynamics of cross-scale patterns within spring and summer regimes (Figure 1), which may reflect adaptive community dynamics within the basins of attraction of spring and summer blooms.
We conclude with acknowledging that we could only use two sites for this study, which prevents us from drawing firm conclusions about phytoplankton dynamics in the Baltic Sea. However, the exploratory results allow us to highlight the potential to study plankton seasonality from a resilience perspective. Accounting for the complexity inherent in resilience might potentially contribute to a better understanding of ecosystem dynamics and potentially management (Angeler et al., 2014). Specifically, our preliminary findings broadly supported resilience theory, which posits that ecological systems can manifest and change between alternative regimes. The dynamics of regimes receive increased attention by scientists and managers because rapid environmental change potentially irreversibly alters ecosystems. Model organisms are needed that allow revealing patterns and processes of various aspects of regime dynamics at tractable time scales. Our findings suggest that phytoplankton can be suitable models for assessing the intricacies of regimes and regime changes repeatedly over relatively short time spans.
Author Contributions
DA conceived and designed study, carried out analysis, and wrote the paper. CA, DT, and MW contributed to idea development and the writing. All authors provided funding and approved submission.
Funding
Any use of trade, firm, or product names is for descriptive purposes only and does not imply endorsement by the U.S. Government. The Nebraska Cooperative Fish and Wildlife Research Unit is jointly supported by a cooperative agreement among the U.S. Geological Survey, the Nebraska Game, and Parks Commission, the University of Nebraska, the U.S. Fish and Wildlife Service, and the Wildlife Management Institute. This work was carried out while DA was contracted at Stockholm University. Additional support was provided through a visiting professorship awarded to DA at the University of Nebraska—Lincoln. Financial support was provided by research grants from SERDP (RC-2510), the Swedish Research Councils Formas (2014-1193), and VR (2014-5828). This work results from the Workshop on Complex Systems: Patterns and Processes held in June 2017 in Granada, Spain financed by the University of Nebraska—Lincoln. All funding agencies covered parts of DA's salary.
Conflict of Interest Statement
The authors declare that the research was conducted in the absence of any commercial or financial relationships that could be construed as a potential conflict of interest.
Acknowledgments
We thank two reviewers for helpful comments that substantially improved the paper. We acknowledge U. Larsson, M. Tirén, S. Haidu, and S. Nyberg who provided the data for this study.
References
Allen, C. R., Angeler, D. G., Garmestani, A. S., Gunderson, L. H., and Holling, C. S. (2014). Panarchy: theory and application. Ecosystems 17, 578–589. doi: 10.1007/s10021-013-9744-2
Allen, C. R., Gunderson, L., and Johnson, A. R. (2005). The use of discontinuities and functional groups to assess relative resilience in complex systems. Ecosystems 8, 958–966. doi: 10.1007/s10021-005-0147-x
Anderson, M. J. (2005). PERMANOVA: A FORTRAN Computer Program for Permutational Multivariate Analysis of Variance. New Zealand: Department of Statistics, University of Auckland.
Angeler, D. G., and Allen, C. R. (2016). Quantifying resilience. J. Appl. Ecol. 53, 617–624. doi: 10.1111/1365-2664.12649
Angeler, D. G., Allen, C. R., Barichievy, C., Eason, T., Garmestani, A. S., Graham, N. A. J., et al. (2016). Management applications of discontinuity theory. J. Appl. Ecol. 53, 688–698. doi: 10.1111/1365-2664.12494
Angeler, D. G., Allen, C. R., Birgé, H. E., Drakare, S., McKie, B. G., and Johnson, R. K. (2014). Assessing and managing freshwater ecosystems vulnerable to environmental change. AMBIO 43, 13–125. doi: 10.1007/s13280-014-0566-z
Angeler, D. G., Allen, C. R., Garmestani, A. S., Gunderson, L. H., Hjerne, O., and Winder, M. (2015). Quantifying the adaptive cycle. PLoS ONE 10:e0146053. doi: 10.1371/journal.pone.0146053
Angeler, D. G., Allen, C. R., and Johnson, R. K. (2012). Insight on invasions and resilience derived from spatiotemporal discontinuities of biomass at local and regional scales. Ecol. Soc. 17:32. doi: 10.5751/ES-04928-170232
Angeler, D. G., Fried-Petersen, H., Allen, C. R., Garmestani, A., Twidwell, D., Chuang, W., et al. (2019). Adaptive capacity in ecosystems. Adv. Ecol. Res. 60, 1–24. doi: 10.1016/bs.aecr.2019.02.001
Baho, D. L., Allen, C. R., Garmestani, A., Fried-Petersen, H. B., Renes, S. E., Gunderson, L. H., et al. (2017). A quantitative framework for assessing ecological resilience. Ecol. Soc. 22:17. doi: 10.5751/ES-09427-220317
Baho, D. L., Tavşanoglu, Ü. N., Šorf, M., Stefanidis, K., Drakare, S., Scharfenberger, U., et al. (2015). Macroecological patterns of resilience revealed through a multinational, synchronized experiment. Sustainability 7, 1142–1160. doi: 10.3390/su7021142
Barichievy, C., Angeler, D. G., Eason, T., Garmestani, A. S., Nash, K. L., Stow, C. A., et al. (2018). A method to detect discontinuities in census data. Ecol. Evol. 8, 9614–9623. doi: 10.1002/ece3.4297
Behrenfeld, M. J., and Boss, E. S. (2014). Resurrecting the ecological underpinnings of ocean plankton blooms. Annu. Rev. Mar. Sci. 6, 167–194. doi: 10.1146/annurev-marine-052913-021325
Beisner, B. E., Haydon, D. T., and Cuddington, K. (2003). Alternative stable states in ecology. Front. Ecol. Environ. 1, 376–382. doi: 10.1890/1540-9295(2003)001[0376:ASSIE]2.0.CO;2
Chipman, H. A., George, E. I., and McCulloch, R. E. (1998). Bayesian cart model search. J. Am. Stat. Assoc. 93, 935–948. doi: 10.1080/01621459.1998.10473750
Clements, F. E. (1916). Plant Succession: An Analysis of the Development of Vegetation. Washington, DC: Carnegie Institute Publication 242. doi: 10.5962/bhl.title.56234
Downing, A. S., Hajdu, S., Hjerne, O., Otto, S. A., Blenckner, T., Larsson, U., et al. (2014). Zooming in on size distribution patterns underlying species coexistence in Baltic Sea phytoplankton. Ecol. Lett. 17, 1219–1227. doi: 10.1111/ele.12327
Edwards, M., and Richardson, A. J. (2004). Impact of climate change on marine pelagic phenology and trophic mismatch. Nature 430, 881–884. doi: 10.1038/nature02808
Elmgren, R. (1989). Man's impact on the ecosystem of the Baltic Sea: energy flows today and at the turn of the century. AMBIO 18, 326–332.
Fukami, T., and Nakajima, M. (2011). Community assembly: alternative stable states or alternative transient states? Ecol. Lett. 14, 973–984. doi: 10.1111/j.1461-0248.2011.01663.x
Garmestani, A. S., Allen, C. R., and Gallagher, C. M. (2008). Power laws, discontinuities and regional city size distributions. J. Econ. Behav. Org. 68, 209–216. doi: 10.1016/j.jebo.2008.03.011
Gunderson, L. (2000). Ecological resilience-in theory and application. Annu. Rev. Syst. Ecol. 31, 425-439. doi: 10.1146/annurev.ecolsys.31.1.425
Gunderson, L. H., and Holling, C. S. (2002). Panarchy: Understanding Transformations in Human and Natural Systems. Washington, DC: Island Press.
Hajdu, S. (2002). Phytoplankton of Baltic Environmental Gradients: Observations on Potentially Toxic Species. PhD Thesis. Stockholm University, Stockholm.
HELCOM (2008). Manual for Marine Monitoring in the COMBINE Programme of HELCOM. HELCOM. Available online at: http://www.helcom.fi/action-areas/monitoring-and-assessment/manuals-and-guidelines/combine-manual (accessed March 20, 2019).
Holling, C. S. (1986). “The resilience of terrestrial ecosystems: local surprise and global change,” in Sustainable Development of the Biosphere, eds Clark, W. C. and Munn, R. E. (Cambridge:Cambridge University Press), 292–320.
Holling, C. S. (1992). Cross-scale morphology, geometry, and dynamics of ecosystems. Ecol. Monogr. 62, 447–502. doi: 10.2307/2937313
Jaanus, A., Andersson, A., Olenina, I., Toming, K., and Kaljurand, K. (2011). Changes in phytoplankton communities along a north-south gradient in the Baltic Sea between 1990 and 2008. Boreal Environ. Res. 16 (Suppl. A), 191–208.
Janssen, F., Neumann, T., and Schmidt, M. (2004). Inter-annual variability in cyanobacteria blooms in the Baltic Sea controlled by wintertime hydrographic conditions. Mar. Ecol. Prog. Ser. 275, 59–68. doi: 10.3354/meps275059
Jiang, L., Joshi, H., Flakes, S. K., and Jung, Y. (2011). Alternative community compositional and dynamical states: the dual consequences of assembly history. J. Animal Ecol. 80, 577–585. doi: 10.1111/j.1365-2656.2010.01799.x
Klais, R., Tamminen, T., Kremp, A., Spilling, K., and Olli, K. (2011). Decadal-scale changes of dinoflagellates and diatoms in the anomalous Baltic Sea spring bloom. PLoS ONE 6:e21567. doi: 10.1371/journal.pone.0021567
Leibold, M. A., and Norberg, J. (2004). Biodiversity in metacommunities: plankton as complex adaptive systems? Limnol. Oceanogr. 49, 1278–1289. doi: 10.4319/lo.2004.49.4_part_2.1278
Lembi, C. A., and Waaland, J. R. (2007). Algae and Human Affairs. Cambridge, NY: Cambridge University Press.
Lindemann, C., and St John, M. A. (2014). A seasonal diary of phytoplankton in the North Atlantic. Front. Mar. Sci. 1:37. doi: 10.3389/fmars.2014.00037
Munawar, M., and Munawar, I. F. (1986). “The seasonality of phytoplankton in the North American Great Lakes, a comparative synthesis,” in: Seasonality of Freshwater Phytoplankton, eds Munawar, M. and Talling, J. F. (Dordrecht: Springer), 85–115. doi: 10.1007/978-94-009-4818-1_7
Nash, K. L., Allen, C. R., Angeler, D. G., Barichievy, C., Eason, T., Garmestani, A. S., et al. (2014). Discontinuities, cross-scale patterns and the organization of ecosystems. Ecology 95, 654–667. doi: 10.1890/13-1315.1
Olenina, I., Hajdu, S., Edler, L., Andersson, A., Wasmund, N., Busch, S., et al. (2006). Biovolumes and size-classes of phytoplankton in the Baltic Sea. Baltic Sea Environ. Proc. 106, 1–144.
Österblom, H., Hansson, S., Larsson, U., Hjerne, O., Wulff, F., Elmgren, R., et al. (2007). Human-induced trophic cascades and ecological regime shifts in the Baltic Sea. Ecosystems 10, 877–889. doi: 10.1007/s10021-007-9069-0
Peterson, G. D., Allen, C. R., and Holling, C. S. (1998). Ecological resilience, biodiversity, and scale. Ecosystems 1, 6–18. doi: 10.1007/s100219900002
Reynolds, C. S. (2006). The Ecology of Phytoplankton, Cambridge: Cambridge University Press. doi: 10.1017/CBO9780511542145
Segura, A. M., Kruk, C., Calliari, D., García-Rodriguez, F., Conde, D., Widdicombe, C. E., et al. (2013). Competition drives clumpy species coexistence in estuarine phytoplankton. Sci. Rep. 3:1037. doi: 10.1038/srep01037
Sommer, U. (1981). The role of r- and K- selection in the succession of phytoplankton in Lake Constance. Acta Oecol. 2, 327–342.
Sommer, U. (1985). Seasonal succession of phytoplankton in Lake Constance. Bioscience 35, 351–357. doi: 10.2307/1309903
Sommer, U., Adrian, R., De Senerpont Domis, L., Elser, J. J., Gaedke, U., Ibelings, B., et al. (2012). Beyond the Plankton Ecology Group (PEG) Model: Mechanisms driving plankton succession. Annu Rev. Ecol. Evo. Syst. 43, 429–448. doi: 10.1146/annurev-ecolsys-110411-160251
Sommer, U., Gliwicz, Z. M., Lampert, W., and Duncan, A. (1986). The PEG-model of seasonal succession of planktonic events in fresh waters. Arch. Hydrobiol. 106, 433–471.
Spanbauer, T. L., Allen, C. R., Angeler, D. G., Eason, T., Fritz, S. C., Garmestani, A. S., et al. (2016). Body size distributions signal a regime shift in a lake ecosystem. Proc. R. Soc. B 283:20160249. doi: 10.1098/rspb.2016.0249
Stow, C., Allen, C. R., and Garmestani, A. S. (2007). Evaluating discontinuities in complex systems: Toward quantitative measures of resilience. Ecol. Soc. 12:26. doi: 10.5751/ES-02055-120126
Suikkanen, S., Pulina, S., Engström-Öst, J., Lehtiniemi, M., Lehtinen, S., and Brutemark, A. (2013). Climate change and eutrophication induced shifts in Northern summer plankton communities. PLoS ONE 8:e66475. doi: 10.1371/journal.pone.0066475
Sundstrom, S. M., Angeler, D. G., Barichievy, C., Eason, T., Garmestani, A., Gunderson, L., et al. (2018). The distribution and role of functional abundance in cross-scale resilience. Ecology 99, 2421–2432. doi: 10.1002/ecy.2508
Sundstrom, S. M., Angeler, D. G., Garmestani, A. S., García, J. H., and Allen, C. R. (2014). Transdisciplinary application of cross-scale resilience. Sustainability 6, 6925–6948. doi: 10.3390/su6106925
Vidal, T., Calado, A. J., Moita, M. T., and Cunha, M. R. (2017). Phytoplankton dynamics in relation to seasonal variability and upwelling and relaxation patterns at the mouth of Ria de Aveiro (West Iberian Margin) over a four-year period. PLOS ONE 12:e0177237. doi: 10.1371/journal.pone.0177237
Wasmund, N., and Uhlig, S. (2003). Phytoplankton trends in the Baltic Sea. ICES J. Mar. Sci. 60, 177–186. doi: 10.1016/S1054-3139(02)00280-1
Willén, T. (1962). Studies on the phytoplankton of some lakes connected with or recently isolated from the Baltic. Oikos 13, 169–199. doi: 10.2307/3565084
Winder, M., and Cloern, J. E. (2010). The annual cycles of phytoplankton biomass. Phil. Trans. Royal. Soc. B 365, 3215–3226. doi: 10.1098/rstb.2010.0125
Yletyinen, J., Blenckner, T., and Peterson, G. (2017). “Baltic Sea – eutrophication,” in: Regime Shifts Database, (Stockholm: Stockholm Resilience Center). Available online at: www.regimeshifts.org
Keywords: Baltic Sea, resilience, cross-scale structure, phytoplankton, blooms, alternative regimes, discontinuities, community succession
Citation: Angeler DG, Allen CR, Twidwell D and Winder M (2019) Discontinuity Analysis Reveals Alternative Community Regimes During Phytoplankton Succession. Front. Ecol. Evol. 7:139. doi: 10.3389/fevo.2019.00139
Received: 07 December 2018; Accepted: 07 April 2019;
Published: 01 May 2019.
Edited by:
Agostino Merico, Leibniz Centre for Tropical Marine Research (LG), GermanyReviewed by:
Lance Gunderson, Emory University, United StatesFrancesco Pomati, Swiss Federal Institute of Aquatic Science and Technology, Switzerland
Copyright © 2019 Angeler, Allen, Twidwell and Winder. This is an open-access article distributed under the terms of the Creative Commons Attribution License (CC BY). The use, distribution or reproduction in other forums is permitted, provided the original author(s) and the copyright owner(s) are credited and that the original publication in this journal is cited, in accordance with accepted academic practice. No use, distribution or reproduction is permitted which does not comply with these terms.
*Correspondence: David G. Angeler, ZGF2aWQuYW5nZWxlckBzbHUuc2U=