- 1Federated Department of Biological Sciences, New Jersey Institute of Technology, Newark, NJ, United States
- 2Federated Department of Biological Sciences, Rutgers University-Newark, Newark, NJ, United States
- 3School of Earth and Space Exploration, Arizona State University, Tempe, AZ, United States
- 4Department of Biological Sciences, Macquarie University, Sydney, NSW, Australia
Throughout evolution, living systems have developed mechanisms to make adaptive decisions in the face of complex and changing environmental conditions. Most organisms make such decisions despite lacking a neural architecture. This is the case of the acellular slime mold Physarum polycephalum that has demonstrated remarkable information processing and problem-solving abilities. Previous studies suggest that the membrane of P. polycephalum plays an important role in integrating and processing information leading to the selection of a resource to exploit. The cyclical contraction-relaxation pattern of the membrane changes with the local quality of the environment, and individual contractile regions within a P. polycephalum can entrain neighboring regions, providing a potential mechanism for information processing and propagation. In this study, we use an information-theoretic tool, transfer entropy, to study the flow of information in single tubule segments of P. polycephalum in a binary choice between two food sources. We test P. polycephalum tubules in two food choice conditions, where the two available options are either symmetric in their nutrient concentrations or with one more concentrated in nutrients than the other (i.e., asymmetric). We measure the contractile pattern of the P. polycephalum membrane and use these data to explore the direction and amount of information transfer along the tubule as a function of the cell's final decision. We find that the direction of information transfer is different in the two experimental conditions, and the amount of information transferred is inversely proportional to the distance between different contractile regions. Our results show that regions playing a leading role in information transfer changes with the decision-making challenges faced by P. polycephalum.
Introduction
In order to develop, survive and reproduce, all living organisms have to make decisions regarding what resource to exploit, which microclimate to inhabit, when to forage in an environment, etc. Therefore, natural selection has led to the evolution of information processing capabilities in living systems that help them make adaptive choices in the face of complex and changing environmental conditions. The majority of the work on decision-making has focused on organisms with a neuron-based information processing system, and it is only recently that researchers have started investigating non-neuronal organisms in the context of decision-making (see Reid et al., 2015; Baluška and Levin, 2016 for full review). Non-neuronal organisms—like plants, bacteria, fungi, and protist—constitute a majority of living species on Earth and do not possess cells and organs dedicated to integrating information from their multiple sensory systems. These organisms have existed long before the evolution of neuronal organisms (Baluška and Levin, 2016) and, despite lacking neurons, have the capability to process information in order to exploit their environment in a non-random, adaptive fashion. For instance, Escherichia coli bacteria have been shown to select the best of multiple resources of varying quality (Kim and Kim, 2010). Rhodospirillum and Rhodobacter bacteria (Sackett et al., 1997), Dictyostelium discoideum protists (Keating and Bonner, 1977), Phytophthora capsici oomycetes (Katsura and Miyata, 1971) actively move toward locations with microclimates more favorable to their development. Moreover, Paenibacillus dendritiformis bacteria (Harshey, 2003; Ben-Jacob and Levine, 2006), Abutilon theophrasti plants (Cahill et al., 2010), Phanerochaete velutina fungi (Bebber et al., 2007), Dictyostelium discoideum protists (Martínez-García and Tarnita, 2017) make compensatory decisions when faced with multiple conflicting sources of environmental information. However, little is known about the mechanisms by which non-neuronal organisms integrate multiple, sometimes conflicting sources of information when navigating their environment in search for better living conditions.
The acellular slime mold Physarum polycephalum has recently emerged as a model system for studying information processing and problem-solving in non-neuronal organisms (Beekman and Latty, 2015; Oettmeier et al., 2017). P. polycephalum is a unicellular, multi-nucleated protist that can cover an area of over 900 cm2 (Kessler, 1982) and move up to a speed of 5 cm/h (Aldrich and Daniel, 1982). Despite lacking neurons, P. polycephalum shows complex decision-making behaviors. For instance, it can solve labyrinth mazes (Nakagaki et al., 2000); form adaptive networks balancing efficiency, cost and fault tolerance, similar to those found in man-made structures (Tero et al., 2010); solve complex optimization problems (Reid and Beekman, 2013); anticipate periodic events (Saigusa et al., 2008); avoid previously exploited areas by using its extracellular slime trail as an externalized memory (Reid et al., 2012); habituate to repeated irrelevant stimuli (Boisseau et al., 2016); and even make economically irrational decisions that were previously deemed a by-product of neuronal decision-making only (Latty and Beekman, 2011). Combined with its macroscopic scale and its experimental tractability, the cognitive-like abilities of P. polycephalum make it a unique model organism to investigate the mechanisms of problem-solving in non-neuronal systems.
Previous studies have suggested that the problem-solving abilities of the slime mold P. polycephalum are driven by a coupled-oscillator based sensorimotor system present in its membrane (Matsumoto et al., 1986, 1988). Indeed, P. polycephalum's membrane is composed of multiple rhythmically contractile regions that lead to the emergence of a complex pattern of contraction-relaxation cycles at the organism level. The contractions occur about once every 60–120 s (Wohlfarth-Bottermann, 1977) and result from the activity of the actomyosin protein networks that comprise the cell cytoskeleton (Isenberg and Wohlfarth-Bottermann, 1976). The membrane contraction-relaxation cycles are coordinated at the organismal level such that they cause the protoplasm1 to flow rhythmically back and forth throughout the cell (Alim et al., 2013), a phenomenon called shuttle streaming. The individual contractile regions change their contraction intensity in response to both the quality of the local environment (Tso and Mansour, 1975; Durham and Ridgway, 1976; Wohlfarth-Bottermann and Block, 1981; Miyake et al., 1994) and the contraction intensities of the neighboring regions (i.e., the coupling between the neighboring contractile regions) (Durham and Ridgway, 1976; Matsumoto et al., 1986). Previous studies have found that the contraction intensities in the slime mold P. polycephalum can change both in frequency (Durham and Ridgway, 1976; Matsumoto et al., 1986) and amplitude (Alim et al., 2017). When a region of a P. polycephalum encounters an attractive (e.g., a food source) or a repulsive (e.g., bright areas) stimulus in the local environment, the contraction intensity of the region increases or decreases, respectively. The coupling between the neighboring regions triggers a change in the pattern of membrane contractions throughout the cell, followed by the movement of the cell toward attractive and away from repulsive stimuli (Durham and Ridgway, 1976; Matsumoto et al., 1986; Miyake et al., 1994). Therefore, this coupling is a potential mechanism for transferring information about the quality of the local environment to distant regions of the cell.
This information processing mechanism in P. polycephalum is part of the broader class of distributed decision-making mechanisms present in numerous biological systems (Miyake et al., 1996). Decision-making in these systems is decentralized (i.e., without a leader or a pre-designed blueprint), with different parts of the system changing their behavior based on information extracted from the local environment and/or transferred from neighboring parts (Camazine et al., 2003). Information transfer between the parts is achieved via repeated interactions and enables the system to collectively integrate information about the environment and generate a response at the level of the group. For instance (Strandburg-Peshkin et al., 2013), showed that local visual interactions in schools of golden shiner fish (Notemigonus crysoleucas) allow individuals informed about the location of a resource to steer the entire group toward it. Non-neuronal organisms have also been observed to process information and solve problems in a distributed fashion reviewed in Reid et al. (2015). For instance, in Arabidopsis thaliana plants, information about a herbivore attack on a leaf is transferred to the undamaged parts through the transfer of molecular Ca2+ signals (Toyota et al., 2018). This information is subsequently used by the undamaged parts to activate their defense responses against herbivory.
Our objective in this study is to investigate the distributed information transfer between different contractile regions in P. polycephalum, in the presence of an attractive stimulus (i.e., a food source). To this effect, we recorded the contraction-relaxation pattern of a straight tubule-shaped cell of P. polycephalum connected on both ends to two food sources. We tested two experimental conditions: a symmetric condition in which both food sources were identical in their nutrient concentration, and an asymmetric condition in which one food source was more concentrated with nutrients than the other one. We measured the contractile behavior of different parts on the tubule while the cell was integrating and processing information about the food sources. Finally, we used a model-free, information-theoretic tool known as transfer entropy to measure the relative influence of the contraction-relaxation pattern of each tubule regions on the others.
The concept of transfer entropy is built upon the theoretical concept of Shannon entropy (Shannon, 1948). Shannon entropy (hereafter simply referred to as entropy) is a measure that quantifies the degree of uncertainty in predicting the value of a random variable. For instance, consider the thickness of a region of a P. polycephalum as a random variable determined by the cyclical pattern of contractile behavior of that region. In a condition with complete lack of contractions, i.e., constant thickness over time and no cycle, entropy is precisely zero, independent of the particular thickness value. When the cycles are more variable, i.e., changing in either frequency or amplitude over time, the thickness of the P. polycephalum region is characterized by high uncertainty and therefore high entropy. We can take a step further and look at how the entropy of different regions of a P. polycephalum are related to each other. Transfer entropy is a tool that measures the reduction in uncertainty in the future values of a focal random variable when we take into consideration its past values as well as the current values of another random variable (Schreiber, 2000). It is the entropy in common between the present of one variable and the future of the other. Due to this directionality in time, it can be interpreted as the directed transfer of information between these two variables. In our case, it measures the reduction in uncertainty of the future thickness values of a focal region given knowledge of the current thickness of a different region. Conceptually, transfer entropy quantifies the direction and amount of predictive information between two dynamical processes (Lizier and Prokopenko, 2010). Although it is not a measure of causality, as it can lead to false positives, it can identify causal interactions when present. For this reason, transfer entropy has been used to infer leader-follower relationships in bats (Orange and Abaid, 2015) and zebrafishes (Butail et al., 2016), to study animal-robot interactions (Butail et al., 2014), neural connections in the brain (Dimitrov et al., 2011; Timme et al., 2016), decision-making in agent collectives (Valentini et al., 2018) as well as information transfer between financial time-series (Kwon and Yang, 2008).
Several attempts to characterize information transfer in P. polycephalum have been made before (Durham and Ridgway, 1976; Matsumoto et al., 1986; Miyake et al., 1996; Alim et al., 2017), but none of them relied on a formal definition of information and formal tools to characterize its dynamics. Information theory provides us with a toolbox that we use here to quantify the direction and amount of information transferred between different contractile regions of P. polycephalum when choosing between two food sources. We find that the direction of information transfer in a P. polycephalum tubule differs with the food choice condition presented and the amount of information transferred is inversely proportional to the distance between the contractile regions along a P. polycephalum tubule. Our results provide a characterization of information transfer in P. polycephalum, which can inform and guide the design of future experiments for understanding the functioning of this organism, and contribute to a more general understanding of the role of information and its fundamental processes—storage, transfer, and modification (Crutchfield, 1994)—in other distributed computing systems.
Materials and Methods
Biological Material
The acellular slime mold P. polycephalum is a protist (phylum Amoebozoa, class Myxogastria) that inhabits shady, cool and moist areas of temperate forests. In its vegetative or “plasmodium” stage, it exists as a macroscopic cell with a large number of nuclei freely floating within the protoplasm, without any cellular plasma membrane separating them. The plasmodium moves through its environment by extending and retracting multiple tubular extensions (referred to as pseudopods) in an amoeba-like fashion (Figure 1A). As a consequence of this feature, it is referred to as the multi-headed (polycephalum in Latin) slime mold.
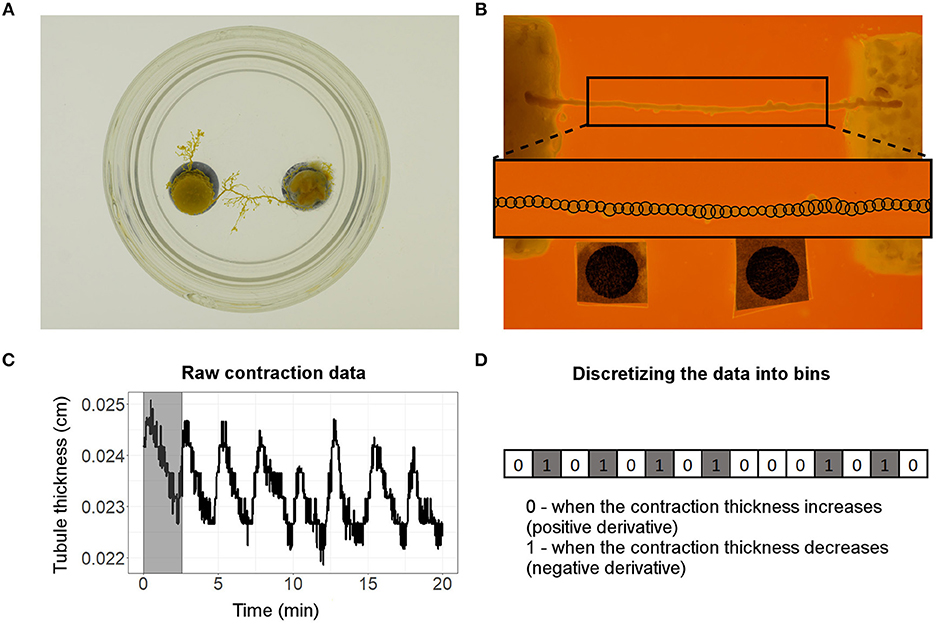
Figure 1. (A) Picture of the setup used to make the tubule-shaped cells of P. polycephalum. (B) Straightened tubule of P. polycephalum placed between 2 food sources (10% w/v oat-agar block on the left and 2% w/v oat-agar block on the right). The two black discs (Ø = 0.3 cm) at the bottom of the image were used as reference points and scale for the image analysis. Outset shows the 50 equidistant locations along the length of the tubule (shown by black circles along the tubule axis) at which the contractions were measured. (C) An example of a contractile behavior observed at the 25th contractile location on the tubule. The period of the first oscillation of the contractile behavior (shown by the shaded region) was found to be 2.58 min. (D) Discretized version of the raw data used in calculating the transfer entropy. A value of 0 or 1 is assigned when the contractions increase or decrease, respectively, in time. The derivatives shown are for the first 15 time steps of the raw data shown in (C).
For our experiments, P. polycephalum stocks were obtained from Carolina Biological Supply Company® and cultured in Petri plates (Ø = 10 cm, H = 1.5 cm) in a dark environment with a controlled temperature of 26°C. Each Petri plate was filled with a water solution with 1% w/v (weight/volume) non-nutrient agar to provide a gel-like, moist substrate, and 5% w/v blended oat flakes (Quaker Oats Company®) as food. Laboratory stocks were recultured on a weekly basis using new, 5% w/v oat-agar plates.
Experimental Setup
We studied information transfer during food choice in tubule-shaped cells of P. polycephalum. P. polycephalum tubules were obtained using the following procedure. Two agar blocks—an agar-only block and a 5% oat-agar block (or food agar)—were placed above the surface of a pool of water using a support (Figure 1A). The two agar blocks were separated by a distance of approximately 4 cm. A P. polycephalum biomass of ~0.5 g was placed on top of the agar-only block and allowed to grow tubular extensions on the water surface. After about 18–24 h, P. polycephalum formed a tubule network extending between the agar-only and food-agar blocks. Tubules of 2.5 cm in length were cut from the network, straightened, and placed between two food sources in the final experimental setup (Figure 1B). The final experimental setup was a Petri plate (Ø = 6 cm, H = 1.3 cm) with a substratum of 1% w/v non-nutrient agar that provided a gel-like, moist base. Two food blocks were embedded in the non-nutrient agar substrate separated by a distance of 2 cm (Figure 1B). The tubule was arranged on the two food blocks such that equal lengths of tubule interacted with food at each end. The P. polycephalum tubule was presented with two food choice treatments. In the first treatment, two identical food sources with 10% oat-agar were placed at each tubule end (hereafter referred to as the symmetric food choice condition). While in the second treatment, a 10 and 2% oat-agar food block were placed at the left and right tubule end, respectively (hereafter referred to as the asymmetric food choice condition).
The contractile behavior of the P. polycephalum tubule was recorded with high-resolution time-lapse photography using a Panasonic® Lumix GH4 camera fitted with an Olympus® M.Zuiko Digital ED 60 mm macro lens. Images were captured every second for a total of 9,999 s (or approximately 2 h and 45 min, the maximum authorized by the camera in this configuration). In order to capture high-resolution images of the tubule, we recorded only the portion of the experimental setup between the two food sources where the tubule in located. The experimental setup was illuminated from below using an LED panel (www.superbrightleds.com®) to allow the recording of high-definition tubule edges. As P. polycephalum is sensitive to and avoids UV and short wavelength visible light, we placed a 610 nm long-pass filter (Newport Corporation®) between the experimental setup and the LED panel. Previous studies have shown that P. polycephalum is not sensitive to wavelengths of light passing through this filter (Alim et al., 2013).
The use of 610 nm light filter made the slime mold growth on the food source appear indistinguishable from the food block background by our computer vision software. Therefore, we visually inspected the experimental setup at the end of the experiment to record the final food choice of the P. polycephalum tubule. When a distinguishable amount of biomass aggregation was visually observed on the left or right food source, the final choice was recorded as the left or right choice, respectively. In the case in which the relative P. polycephalum biomass growth on the two food sources was visually indistinguishable, the final outcome of the experiment was recorded as undecided.
The contractile behavior of the tubules was measured using a dedicated, computer vision script written in MATLAB®. For each time-lapse picture, the script detects the tubule edges, extracts it from the background, and measures the tubule thickness at 50 equally spaced locations along the length of the tubule (Figure 1B outset). The script positions a circle at each location and adjusts their diameters to match the thickness of the tubule location. Over the course of the experiment, changes in the circles' diameters at each tubule location captures the contractile behavior of the tubule. Figure 1C shows the typical form of raw data that we obtained and used for the analysis. The contractile behavior of the tubule was measured within a centrally located 1.6 cm long segment, i.e., we disregarded 0.2 cm of tubule section on each side as the overlapping of tubule with the food sources did not allow us to reliably track its contractions.
Data Preprocessing
As the tubule contracts over time, its thickness sampled at each location varies and produces a noisy, cyclical signal as shown in Figure 1C. To reduce the effects of noise, we filtered our raw data using a LOWESS smoothing algorithm (Cleveland, 1979). In our analysis, we varied the smoothing span, i.e., the fraction of data used to locally estimate the smoothed signal, between 0% (no smoothing) and 1% of the raw data. The smoothing span we use to filter raw data is given in Analysis of the parameter space and the rationale behind choosing a value is explained in the section below (Metrics). Finally, we discretize the filtered signals sampled at each of the 50 locations of the tubule and obtain a set of 50 binary time series (one for each location). For each signal, we compute the derivative in time and construct a time series X = {x1, …, xn} (see Figure 1D) by assigning a value of xi = 0 when the tubule thickness increases at time i (i.e., positive derivative) or a value of xi = 1 if the tubule thickness decreases at time i (i.e., negative derivative).
Metrics
Final Tubule Choice
A one-sample proportionality test with Yates' continuity correction (Wilson, 1927) was used to determine whether the tubules had a statistically significant preference for either of the two food sources. Under the null hypothesis (i.e., no preference for either of the two food sources) we expect the theoretical proportions of choosing either of the two food sources to be equal. However, the theoretical proportion of the undecided cases cannot be calculated as it would require knowing the exact decision-making mechanism. The undecided cases is nonetheless an essential piece of information indicating when P. polycephalum could not express a preference for either of the food sources, and therefore should not be discarded from the analysis. As a consequence, half of the undecided experimental trials were counted toward the left food source and the other half toward the right food source. This ensured that a lack of choice in one or more trials was represented in the final outcome of the analysis.
Transfer Entropy
Our analysis of information transfer underlying food choice by the slime mold P. polycephalum is based on the notion of transfer entropy introduced by Schreiber (Schreiber, 2000). Transfer entropy, TY → X, is an information-theoretic measure that quantifies the exchange of information originating from a process Y and directed toward a process X. In our analysis, process X and Y correspond to a pair of oscillatory signals generated by the contractile behavior of the tubule at two different locations and discretized as explained in Data preprocessing. Transfer entropy TY → X from a location Y on the tubule toward a location X is given by
In the above equation, p(·) represents the empirical probability of a certain event (e.g., xi = 1) estimated from the time series X and Y while represents the k-history of X at time i and is given by the k consecutive values of the time series preceding and including xi. In other words, transfer entropy provides a measure of the amount of information that we gain about the contractile behavior of the tubule at location X from knowledge of the same behavior at location Y given the history of X. All computations of transfer entropy were performed using package rinform-1.0.1 (Moore et al., 2018) in R version 3.4.3 (R Core Team, 2017).
Based on transfer entropy, we then define different aggregation measures to study the dynamics of information transfer. We consider the total transfer entropy TTE over all locations of the P. polycephalum tubule defined as
i.e., the sum of transfer entropy over all pairs of locations x and y on the tubule. We use TTE as a target measure to find the parameters that optimize and improve the quality of information transfer measurements in the food choice experiments. Specifically, we calculated TTE for different combinations of parameters, namely history length k and LOWESS smoothing span. The combination of k and LOWESS span that maximizes TTE is used in the computation of net transfer entropy NTE(x) values described in detail below.
We define the net transfer entropy NTE(x) as a measure for the study of information transfer as a function of the location x∈{1, …, 50} of the tubule
NTE(x) computes the difference between outgoing information and incoming information for a particular location of the P. polycephalum tubule. That is, the difference between total information transferred from location x toward any other location y ≠ x of the tubule (outgoing information) and the total information transferred to location x from any other location y ≠ x of the tubule (incoming information). Positive values of NTE(x) indicate that location x is a source of predictive information about the system. Negative values of NTE(x), indicate instead that location x is an information destination. NTE(x) as defined above is a variation of the standard, pairwise net transfer entropy
between a pair of processes X and Y [cf. (Butail et al., 2016)]. We use NTEX → Y between a pair of locations x and y of the tubule to determine and visualize the flow of information across the tubule as a network and gain insight into the orientation of the information flow. We do so by grouping locations on the tubule in 5 sets of ten (i.e., {1, 2, …, 10}, …, {41, 42, …, 50}). For each pair of groups, the total net transfer entropy was calculated as the sum of contributions of NTEX → Y between all pairs of locations belonging to the two groups.
We computed all measures defined above considering also an artificially created dataset that we used as the control to define a reference level of zero transfer of information. We do so by computing transfer entropy TY → X between pairs of locations x and y whose time series X and Y have been collected from two different experimental trials (i.e., two different tubules). As the experimental trials are independent of each other, there is no real transfer of information happening across the two tubules. The results computed in this way allow us to discriminate between information transfer generated by intrinsic noise in our experimental procedure and actual information transfer happening among the tubule regions during an experiment. Additionally, by pairing signals collected by two different experimental trials, we effectively augment the size of the control dataset with respect to the actual number of trials (e.g., 42·41 for the asymmetric treatment with 42 trials). All results discussed in Analysis of the parameter space and Information flow network are reported at the net of the same measures computed over the control dataset based on 252 random pairs of trials. In Information sources and destinations, the results computed using the control dataset are visualized separately.
Results
We performed two series of experiments (or treatments) where the quality of the food source placed on the left side of the tubule was kept constant with a 10% w/v oat-agar block and varied that of the food source placed on the right side of the tubule with either 10% w/v (i.e., the symmetric food choice condition) or 2% w/v oat-agar blocks (i.e., the asymmetric food choice condition). Figure 2 shows the distributions of the final decisions of the P. polycephalum tubules over the different experimental trials. In the symmetric treatment, P. polycephalum does not show a significant preference for either of the two food sources (proportionality test: p = 0.1696, 54 trials) with a large number (23 out of 54) of experimental trials concluding in an undecided state. However, in the asymmetric treatment, P. polycephalum shows a significant preference (proportionality test: p = 4.896e−05, 42 trials) for the left block composed of 10% w/v oat-agar over the right block with 2% w/v oat-agar. This result is consistent with the findings from a previous study by Latty and Beekman (Latty and Beekman, 2010), which showed that P. polycephalum has a significant preference for the more nutrient concentrated food option when given a choice between two food sources with different nutrient concentrations.
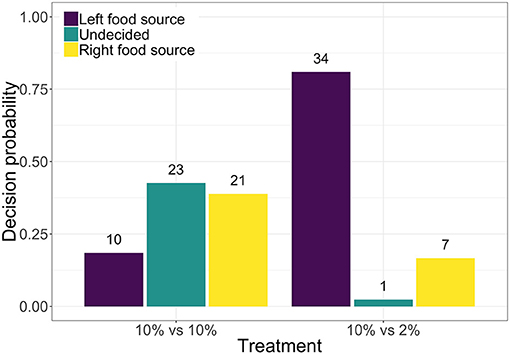
Figure 2. Distribution of final decisions (i.e., left food source, undecided, or right food source) taken by P. polycephalum tubules for the symmetric food choice condition with two 10% w/v oat-agar blocks (54 experimental trials with both left and right food sources being 10% w/v oat-agar food block) and the asymmetric food choice condition with 10% w/v and 2% w/v oat-agar blocks (42 experimental trials with left food source being 10% w/v oat-agar food block and right being 2% w/v oat-agar food block).
Analysis of the Parameter Space
For both treatments, we calculated the total transfer entropy averaged over all experimental trials as a function of the history length k and of the LOWESS smoothing span. We performed the same calculations for the control dataset and computed the difference between the real results and the control ones. Figure 3 shows the distribution of total transfer entropy (TTE; adjusted to that of the control dataset) over the parameter space. The landscapes of TTE in the two experimental treatments are similar to each other, however, the particular parameters that maximize TTE differ. In the symmetric food choice condition, TTE is maximized for history length k = 3 and smoothing span 0.001 (see Figure 3A). In the asymmetric food choice condition instead, TTE is maximized for history length k = 10 and smoothing span of 0.006 (see Figure 3B). While the accuracy of these parameter configurations is subject to the number of experimental trials, their values provide us with a good representation of information transfer occurring within the tubules.
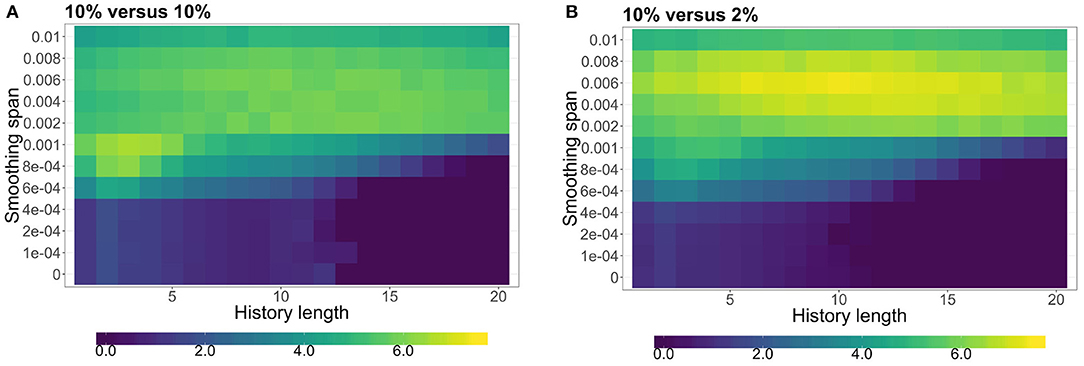
Figure 3. Total transfer entropy (TTE) as a function of the history length (k ∈ {1, …, 20}) and of the LOWESS smoothing span (smoothing span ∈ {0, 0.0001, …, 0.01}). (A) The symmetric food choice condition with two 10% w/v oat-agar blocks where TTE is maximized for history length k = 3 and smoothing span 0.001. (B) The asymmetric food choice condition with 10% w/v and 2% w/v oat-agar blocks where TTE is maximized for history length k = 10 and smoothing span of 0.006.
Information Sources and Destinations
To understand how P. polycephalum process information during the food choice experiments, it is imperative to investigate where information is generated or gathered from the environment (i.e., source) and to which locations of the system it is transferred (i.e., destination). We examined the net transfer entropy as a function of the location on the P. polycephalum tubule. Figure 4 shows the results of our analyses for both experimental treatments and their control datasets arranged by the final decision of the P. polycephalum tubule. In the symmetric food choice condition with two 10% w/v oat-agar blocks, the net transfer entropy is symmetrically distributed around the center of the tubule (i.e., locations 25-26) with the extremities of the tubule characterized by positive values of NTE and the middle section characterized by negative values independently from the final food choice (cf. Figures 4A,B). Additionally, for both final food choices, the values of net transfer entropy were similar along the length of the tubule (in the range between −0.025 and 0.05 bits). However, in the asymmetric food choice condition, NTE is positive for locations on the P. polycephalum tubule that is opposite to the chosen food source and negative for locations in the proximity of this source. Note that the scale of the y-axis in Figures 4A,B is different, with the NTE values being higher in the tubule regions near the chosen food source when the final choice was the 2% oat-agar food block (upper limit of the y-axis is approximately 0.4 bits) relative to the case when the tubule choice was the 10% oat-agar food block (upper limit of the y-axis is approximately 0.1 bits). Similarly, the regions near the rejected food source show lower NTE values when the tubule choice was 2% oat-agar food block (lower limit of the y-axis is approximately −0.3 bits) than the case when the choice was 10% oat-agar food block (lower limit of the y-axis is approximately −0.05 bits). In Figure 4C, we aggregated the results of all experimental trials by inverting the order of the tubule locations for all trials where the tubule chose the right food source and half of the trials recorded as undecided. This allows us to visualize the results of NTE for all trials independently from the nutrient concentration of the food source chosen by the P. polycephalum tubule. While in the symmetric food choice condition information originates at the extremities of the tubules (i.e., from the tubule regions near the two food sources) and flows toward the center without a noticeable correlation with the final food choice (i.e., left), whereas in the asymmetric food choice condition, information originates at the opposite side of the chosen food source and flows toward the tubule region in its proximity. That is, when a more nutrient-rich food option is available, the contractile dynamics of the tubule in the proximity of the chosen food source are anticipated by those at the opposite extremity.
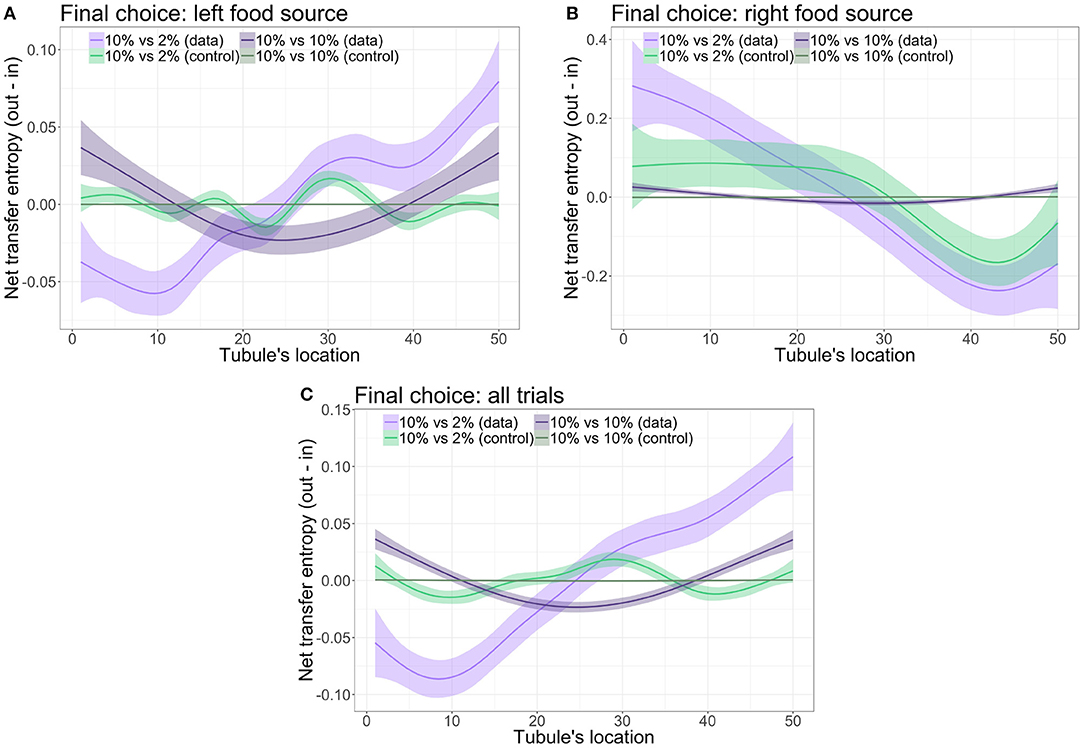
Figure 4. Net transfer entropy at the contractile locations along the length of the tubule ({1, …, 50}) for (A) trials with final choice being the left food source, (B) trials with final choice being the right food source, and (C) all trials such that the chosen food source is on the left and rejected food source on the right. Parameters: 10 vs. 10% w/v (k = 3, smoothing span 0.001), 10 vs. 2% w/v (k = 10, smoothing span 0.006).
Information Flow Network
Finally, we look at the direction and amount of information flow within the tubules of the slime mold P. polycephalum during the food choice experiment. Measurements were coarse-grained to obtain a simpler and clearer picture of information transfer. Adjacent locations on the P. polycephalum tubule were binned into five groups of ten locations each (i.e., we equally divided the tubule into 5 sub-segments or regions). Figure 5 shows the total net transfer entropy between these portions of the tubule averaged over all experimental trials and corrected with the results of the control dataset. As in the previous section, we reversed the orientation of the tubules in all experimental trials where the final choice was the right food source and in half of the trials recorded as undecided. As a consequence of this, the left side of the networks (i.e., vertex 1) represents the extremity of the tubule in the proximity of the chosen food source regardless of its nutrient concentration. Likewise, the right side of the networks represents the extremity that is next to the rejected food source. Both the symmetric and asymmetric treatments show well-defined networks of information flow. However, the resulting network topologies are significantly different between the two food choice conditions. The symmetric food choice condition, shown in the top panel of Figure 5, is characterized by a flow of information originating from both extremities (i.e., vertex 1 and vertex 5) of the tubule and converging toward its center (i.e., vertex 3). As also shown by the corresponding adjacency matrix, the topology defined by the edges is approximately symmetric with respect to the center of the tubule. In contrast, in the asymmetric food choice condition, shown in the bottom panel of Figure 5, is characterized by a flow of information originating at the extremity of the tubule in contact with the rejected food source and directed toward the opposite extremity. As shown by the corresponding adjacency matrix, for each group of locations 1 < i ≤ 5, there are edges i → j connecting the group to all groups j < i on its left. In both experimental conditions, the absolute values of net transfer entropy represented by the adjacency matrices in Figure 5 are inversely proportional to the distance between two regions of the tubule. Moreover, their amount differs between conditions: in the asymmetric food choice condition, the tubule transfers an amount of information (0.2092 ± 0.038 bits) that is approximately four times the amount transferred during the symmetric food choice condition (0.0537 ± 0.0297 bits). That is, more information is transferred across the tubule when there is a contrast in the concentration of nutrients between the two available food options.
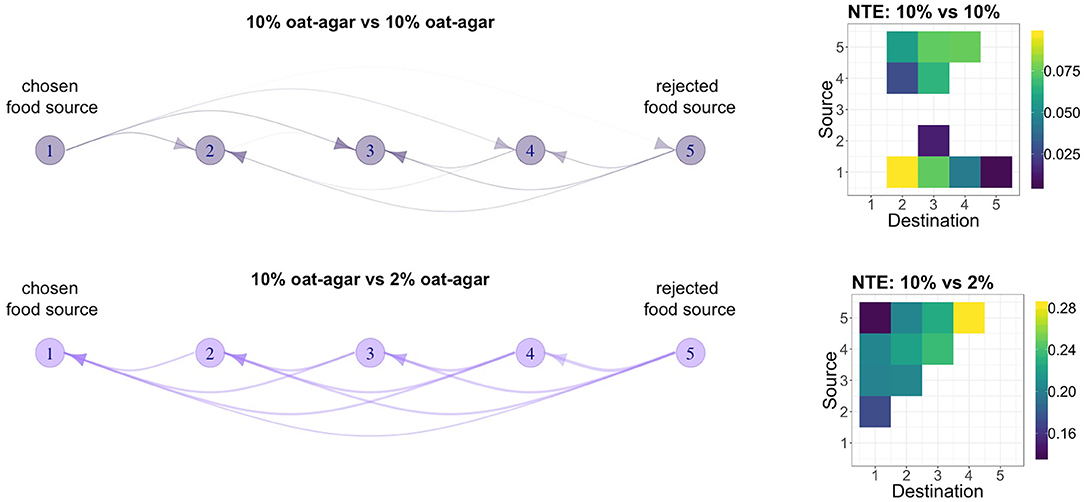
Figure 5. Networks and their adjacency matrix of information flow between groups of adjacent locations (i.e., 1 = {1, 2, …, 10}, …, 5 = {41, 42, …, 50}) on the P. polycephalum tubule constructed from net transfer entropy averaged over all experimental trials. The orientation of the tubule in each trial is adjusted so that the final choice of the tubule is always on the left side of the network (i.e., vertex 1); edge width is proportional to the net transfer entropy. Parameters: 10 vs. 10% w/v (k = 3, smoothing span 0.001), 10 vs. 2% w/v (k = 10, smoothing span 0.006).
Discussion
We studied the relative influence between the contractile regions of a P. polycephalum tubule while it was choosing between two food sources. The tubule was presented with two food choice conditions, which were either symmetric or asymmetric in nutrient concentrations. We studied information transfer across the tubule by measuring the change of thickness at 50 equidistant locations and computing the transfer entropy between each of them.
Our results show that P. polycephalum does not have a significant preference for either of the food options in the symmetric food choice condition, while it has significant preference for the more nutrient-rich option in the asymmetric food choice condition. We showed that the difference in food qualities affects information transfer between the contractile regions of P. polycephalum. In the symmetric food choice condition, the tubule locations near each of the two food sources act as the information sources while those near the center of the tubule act as information destinations. Information transfer is symmetrically distributed around the center of the tubule. These trends are similar regardless of the food source chosen by the tubule at the end of the experiment. Conversely, in the asymmetric food choice condition, the tubule locations near the chosen food source, regardless of the food source quality, act as the destinations of information while those in the proximity of the rejected food act as the information sources therefore, the tubule sub-regions near the rejected food source transfer information toward the sub-regions near the chosen food source. Additionally, the amount of information transfer was found to be inversely proportional to the distance between the sub-regions, similar to the results obtained in the symmetric food choice condition. Interestingly, the amount of information transferred between the tubule regions in the asymmetric food choice condition was four times higher than that of the symmetric condition.
Transfer entropy measures the amount of directed predictive information flow (or information transfer) between two processes, but establishing a causal relationship between the behaviors requires additional considerations (Lizier and Prokopenko, 2010). However, the P. polycephalum tubule is a continuous system and all the locations that we observed are physically connected to each other and constantly interacting with one another through physical forces and chemical exchanges. Therefore, we preliminarily conclude that our results about information transfer capture causal interactions between physically adjacent locations. The existence of a causal relationship between the locations along the tubule will be formally tested in future experiments by physically intervening or perturbing the contractile behavior at a given location.
While our work indicates the occurrence of information transfer between contractile regions of the tubule, the precise medium of the information being transferred remains unknown. In view of this, it is important to note that the organisms tested were reacting to external food stimuli. Although the information transfer that we observed in this study dominantly originates from the end of the P. polycephalum tubule in proximity to the lower nutrient concentrated food source, it would be naïve to neglect the apparent role that food has in stimulating and promoting the contractile behavior (Durham and Ridgway, 1976; Matsumoto et al., 1986; Miyake et al., 1994; Alim et al., 2017). Food-containing vesicles both stimulate local changes in intracellular signal transduction and are degraded to yield sugars and amino acids that fuel all cellular functions. For instance, the contraction-relaxation cycles of the membrane that generate protoplasmic flow involve dynamic rearrangements of actin cytoskeleton and signaling that require ATP (Korohoda et al., 1983) and Ca2+ (Yoshimoto et al., 1981), both of which are derived from food (Matveeva et al., 2010, 2012). By this reasoning, information flow should originate from nutrient-rich food sources. While local contraction intensity may be increased by food stimuli, we suggest that the long-range coordination of the contractile behavior across the P. polycephalum (Alim et al., 2013) is influenced by the contractile behavior at the tubule end with lower nutrient concentrated food source. Information transfer in the form of molecular stimuli originating from the food source and related signal transduction events were not measured in the current work. Yet, this stimulus clearly drives the observed changes in contractile behavior patterns and is likely to represent the primary medium of information acquisition. Future work will be directed toward determining the precise physicochemical signaling that is initiated by foods of differing quality and their impact to oscillatory regulation and ultimately decision-making in P. polycephalum.
Previous studies have shown that when a P. polycephalum cell interacts with an attractive substance, the cell regions located in the vicinity of the substance acts as the information source and the regions located away from the attractive substance act as the information destination (Durham and Ridgway, 1976; Matsumoto et al., 1986; Alim et al., 2017). Moreover, these studies show that the information transfer occurs via changes in contraction intensities that can be in the form of changes in frequency or amplitude. Our results do not indicate whether the information transfer occurs by increasing or decreasing the contraction frequency and/or amplitude. However, the results show that the tubule regions acting as information sources and destinations varied with respect to the food choice conditions presented to the P. polycephalum tubule. Moreover, this imbalance in the localized nature of predictive information with certain regions of the organism covering a more prominent role than the others is reminiscent of the control kernel in yeast-cell regulatory networks (Kim et al., 2015) or of informed individuals playing leadership roles in a school of fishes (Strandburg-Peshkin et al., 2013).
The two food choice conditions used in our study presented the P. polycephalum tubule with two different decision-making challenges. As the direction and amount of information transfer differed in the two treatments, this opens the question of whether the information transfer in P. polycephalum changes relative to the decision-making problem faced by the cell. The experiment we suggest to test this behavior in detail is to progressively increase the quality of the lower concentrated food source and compare the effects on the direction and amount of information transfer.
Finally, our analysis does not provide details about the dynamics of information transfer during the course of the experiment. A refined examination during various stages of decision-making may find information transfer relationships more specifically pertaining to food detection, nutrient transfer, and/or final decision execution. Experiments should be conducted for a longer period of time which would permit to repeat a similar analysis but at different phases of the experiment.
Data Availability
The datasets generated for this study are available on request to the corresponding author.
Author Contributions
SR, GV, CR, and SG conceived the study. SR, AH, and CR devised the experimental setup and protocol. SR and PS conducted the experiments and collected data. SR and SG prepared the computer vision scripts for data extraction. GV performed the statistical and transfer entropy analysis. SR and GV led the writing of the manuscript, with feedback and contributions from CR, GW, and SG.
Funding
This material was based upon work partially supported by the National Science Foundation (NSF) under Grant DMS-1638521 to the Statistical and Applied Mathematical Sciences Institute. Any opinions, findings, and conclusions or recommendations expressed in this material are those of the author(s) and do not necessarily reflect the views of the National Science Foundation. SR and SG are supported by NSF grant No. IOS-1557610. GV is supported by NSF grant No. PHY-1505048. GW and AH are supported by NSF grant No. IOS-1557651. Supplement funding for this project to AH was provided by the Rutgers University—Newark Chancellor's Research Office.
Conflict of Interest Statement
The authors declare that the research was conducted in the absence of any commercial or financial relationships that could be construed as a potential conflict of interest.
Acknowledgments
This study is a direct result of a workshop on Data-Driven Modeling of Collective Behavior and Emergent Phenomena in Biology (DDM-Bio), organized by the Statistical and Applied Mathematical Institute (SAMSI), Durham, NC, USA. We thank the organizers of DDM-Bio, in particular Dr. Jason Graham, for facilitating the collaboration for this project. We thank SAMSI for providing us with the funding to participate in the workshop.
Footnotes
1. ^Protoplasm: is the sol-like substance flowing within a P. polycephalum cell. The protoplasm contains the cell cytoplasm, organelles, nutrients and signaling molecules.
References
Aldrich, H. C., and Daniel, J. W. (1982). Cell Biology of Physarum and Didymium Volume 1: Organisms, Nucleus, and Cell Cycle. New York, NY: Academic Press Inc. Available online at: https://www.elsevier.com/books/cell-biology-of-physarum-and-didymium-v1/aldrich/978-0-12-049601-3
Alim, K., Amselem, G., Peaudecerf, F., Brenner, M. P., and Pringle, A. (2013). Random network peristalsis in Physarum polycephalum organizes fluid flows across an individual. Proc. Natl. Acad. Sci. U.S.A. 110, 13306–13311. doi: 10.1073/pnas.1305049110
Alim, K., Andrew, N., Pringle, A., and Brenner, M. P. (2017). Mechanism of signal propagation in Physarum polycephalum. Proc. Natl. Acad. Sci. U.S.A. 20:1618114. doi: 10.1073/pnas.1618114114
Baluška, F., and Levin, M. (2016). On having no head: cognition throughout biological systems. Front. Psychol. 7:902. doi: 10.3389/fpsyg.2016.00902
Bebber, D. P., Hynes, J., Darrah, P. R., Boddy, L., and Fricker, M. D. (2007). Biological solutions to transport network design. Proc. Biol. Sci. 274, 2307–2315. doi: 10.1098/rspb.2007.0459
Beekman, M., and Latty, T. (2015). Brainless but multi-headed: decision making by the acellular slime mould Physarum polycephalum. J. Mol. Biol. 427, 3734–3743. doi: 10.1016/j.jmb.2015.07.007
Ben-Jacob, E., and Levine, H. (2006). Self-engineering capabilities of bacteria. J. R. Soc. Interface 3, 197–214. doi: 10.1098/rsif.2005.0089
Boisseau, R. P., Vogel, D., and Dussutour, A. (2016). Habituation in non-neural organisms: evidence from slime moulds. Proc. Biol. Sci. 283:20160446. doi: 10.1098/rspb.2016.0446
Butail, S., Ladu, F., Spinello, D., and Porfiri, M. (2014). Information flow in animal-robot interactions. Entropy 16, 1315–1330. doi: 10.3390/e16031315
Butail, S., Mwaffo, V., and Porfiri, M. (2016). Model-free information-theoretic approach to infer leadership in pairs of zebrafish. Phys Rev E 93:042411. doi: 10.1103/PhysRevE.93.042411
Cahill, J. F. Jr, McNickle, G. G., Haag, J. J., Lamb, E. G., Nyanumba, S. M., and St Clair, C. C. (2010). Plants integrate information about nutrients and neighbors. Science 328:1657. doi: 10.1126/science.1189736
Camazine, S., Deneubourg, J.-L., Franks, N. R., Sneyd, J., Bonabeau, E., and Theraulaz, G. (2003). Self-organization in Biological Systems. Princeton University Press Available online at: https://market.android.com/details?id=book-zMgyNN6Ufj0C
Cleveland, W. S. (1979). Robust locally weighted regression and smoothing scatterplots. J. Am. Stat. Assoc. 74, 829–836. doi: 10.1080/01621459.1979.10481038
Crutchfield, J. P. (1994). The calculi of emergence. Physica D 75, 11–54. doi: 10.1016/0167-2789(94)90273-9
Dimitrov, A. G., Lazar, A. A., and Victor, J. D. (2011). Information theory in neuroscience. J. Comput. Neurosci. 30, 1–5. doi: 10.1007/s10827-011-0314-3
Durham, A. C., and Ridgway, E. B. (1976). Control of chemotaxis in Physarum polycephalum. J. Cell Biol. 69, 218–223. doi: 10.1083/jcb.69.1.218
Harshey, R. M. (2003). Bacterial motility on a surface: many ways to a common goal. Annu. Rev. Microbiol. 57, 249–273. doi: 10.1146/annurev.micro.57.030502.091014
Isenberg, G., and Wohlfarth-Bottermann, K. E. (1976). Transformation of cytoplasmic actin importance for the organization of the contractile gel reticulnm and the contraction–relaxation cycle of cytoplasmic actomyosin. Cell Tissue Res. 173, 495–528. doi: 10.1007/BF00224311
Katsura, K., and Miyata, Y. (1971). Swimming behavior of Phytophthora capsici zoospores. Morphol & Biochem Events in Plant parasite Interaction. Available online at: http://agris.fao.org/agris-search/search.do?recordID=US201302389449
Keating, M. T., and Bonner, J. T. (1977). Negative chemotaxis in cellular slime molds. J. Bacteriol. 130, 144–147.
Kessler, D. (1982). “Plasmodial structure and motility,” in Cell biology of Physarum and Didymium, eds H. C. Aldrich, and J. W. Daniel Available online at: http://agris.fao.org/agris-search/search.do?recordID=US201302576850
Kim, H., Davies, P., and Walker, S. I. (2015). New scaling relation for information transfer in biological networks. J. R. Soc. Interface 12:20150944. doi: 10.1098/rsif.2015.0944
Kim, M., and Kim, T. (2010). Diffusion-based and long-range concentration gradients of multiple chemicals for bacterial chemotaxis assays. Anal. Chem. 82, 9401–9409. doi: 10.1021/ac102022q
Korohoda, W., Shraideh, Z., Baranowski, Z., and Wohlfarth-Bottermann, K. E. (1983). Energy metabolic regulation of oscillatory contraction activity in Physarum polycephalum. Cell Tissue Res. 231, 675–691. doi: 10.1007/BF00218125
Kwon, O., and Yang, J. -S. (2008). Information flow between stock indices. EPL 82:68003. doi: 10.1209/0295-5075/82/68003
Latty, T., and Beekman, M. (2010). Food quality and the risk of light exposure affect patch-choice decisions in the slime mold Physarum polycephalum. Ecology 91, 22–27. doi: 10.1890/09-0358.1
Latty, T., and Beekman, M. (2011). Irrational decision-making in an amoeboid organism: transitivity and context-dependent preferences. Proc. Biol. Sci. 278, 307–312. doi: 10.1098/rspb.2010.1045
Lizier, J. T., and Prokopenko, M. (2010). Differentiating information transfer and causal effect. Eur. Phys. J. B 73, 605–615. doi: 10.1140/epjb/e2010-00034-5
Martínez-García, R., and Tarnita, C. E. (2017). Seasonality can induce coexistence of multiple bet-hedging strategies in Dictyostelium discoideum via storage effect. J. Theor. Biol. 426, 104–116. doi: 10.1016/j.jtbi.2017.05.019
Matsumoto, K., Ueda, T., and Kobatake, Y. (1986). Propagation of phase wave in relation to tactic responses by the plasmodium of Physarum polycephalum. J. Theor. Biol. 122, 339–345. doi: 10.1016/S0022-5193(86)80125-4
Matsumoto, K., Ueda, T., and Kobatake, Y. (1988). Reversal of thermotaxis with oscillatory stimulation in the plasmodium of Physarum polycephalum. J. Theor. Biol. 131, 175–182. doi: 10.1016/S0022-5193(88)80235-2
Matveeva, N. B., Teplov, V. A., and Beylina, S. I. (2010). Suppression of the autooscillatory contractile activity of Physarum polycephalum plasmodium by the inhibitor of the IP3-Induced Ca2+ release, 2-aminoethoxydiphenyl borate. Biochem. (Moscow) Suppl. Series A Membr. Cell Biol. 4, 70–76. doi: 10.1134/s1990747810010113
Matveeva, N. B., Teplov, V. A., and Beylina, S. I. (2012). Coupling of phospholipase C and PI3K/PTEN signaling pathways in Physarum polycephalum: the action of U73122 on motile and autooscillatory activity of plasmodium. Biochem. (Moscow) Suppl. Series A Membr. Cell Biol. 6, 255–264. doi: 10.1134/s1990747812030142
Miyake, Y., Tabata, S., Murakami, H., Yano, M., and Shimizu, H. (1996). Environment-dependent self-organization of positional information field in chemotaxis of Physarum Plasmodium. J. Theor. Biol. 178, 341–353. doi: 10.1006/jtbi.1996.0030
Miyake, Y., Tada, H., Yano, M., and Shimizu, H. (1994). Relationship between intracellular period modulation and external environment change in Physarum plasmodium. Cell Struct. Funct. 19, 363–370. doi: 10.1247/csf.19.363
Moore, D. G., Valentini, G., Walker, S. I., and Levin, M. (2018). Inform: efficient information-theoretic analysis of collective behaviors. Front. Robotics and AI 5:60. doi: 10.3389/frobt.2018.00060
Nakagaki, T., Yamada, H., and Tóth, A. (2000). Maze-solving by an amoeboid organism. Nature 407:470. doi: 10.1038/35035159.
Oettmeier, C., Brix, K., and Döbereiner, H. G. (2017). Physarum polycephalum-a new take on a classic model system. J. Phys. D. Appl. Phys. 50:413001. doi: 10.1088/1361-6463/aa8699
Orange, N., and Abaid, N. (2015). A transfer entropy analysis of leader-follower interactions in flying bats. Eur. Phys. J. Spec. Top. 224, 3279–3293. doi: 10.1140/epjst/e2015-50235-9
R Core Team (2017). R: A Language and Environment for Statistical Computing. Vienna: R Foundation for Statistical Computing. Available online at: https://www.R-project.org/
Reid, C. R., and Beekman, M. (2013). Solving the Towers of Hanoi - how an amoeboid organism efficiently constructs transport networks. J. Exp. Biol. 216, 1546–1551. doi: 10.1242/jeb.081158
Reid, C. R., Garnier, S., Beekman, M., and Latty, T. (2015). Information integration and multiattribute decision making in non-neuronal organisms. Anim. Behav. 100, 44–50. doi: 10.1016/j.anbehav.2014.11.010
Reid, C. R., Latty, T., Dussutour, A., and Beekman, M. (2012). Slime mold uses an externalized spatial “memory” to navigate in complex environments. Proc. Natl. Acad. Sci. U.S.A. 109, 17490–17494. doi: 10.1073/pnas.1215037109
Sackett, M. J., Armitage, J. P., Sherwood, E. E., and Pitta, T. P. (1997). Photoresponses of the purple nonsulfur bacteria Rhodospirillum centenum and Rhodobacter sphaeroides. J. Bacteriol. 179, 6764–6768. doi: 10.1128/jb.179.21.6764-6768.1997
Saigusa, T., Tero, A., Nakagaki, T., and Kuramoto, Y. (2008). Amoebae anticipate periodic events. Phys. Rev. Lett. 100:018101. doi: 10.1103/PhysRevLett.100.018101
Schreiber, T. (2000). Measuring information transfer. Phys. Rev. Lett. 85, 461–464. doi: 10.1103/PhysRevLett.85.461
Shannon, C. E. (1948). A mathematical theory of communication. Bell Syst. Tech. J. 27, 379–423. doi: 10.1002/j.1538-7305.1948.tb01338.x
Strandburg-Peshkin, A., Twomey, C. R., Bode, N. W. F., Kao, A. B., Katz, Y., Ioannou, C. C., et al. (2013). Visual sensory networks and effective information transfer in animal groups. Curr. Biol. 23, R709–R711. doi: 10.1016/j.cub.2013.07.059
Tero, A., Takagi, S., Saigusa, T., Ito, K., Bebber, D. P., Fricker, M. D., et al. (2010). Rules for biologically inspired adaptive network design. Science 327, 439–442. doi: 10.1126/science.1177894
Timme, N. M., Ito, S., Myroshnychenko, M., Nigam, S., Shimono, M., Yeh, F.-C., et al. (2016). High-degree neurons feed cortical computations. PLoS Comput. Biol. 12:e1004858. doi: 10.1371/journal.pcbi.1004858
Toyota, M., Spencer, D., Sawai-Toyota, S., Jiaqi, W., Zhang, T., Koo, A. J., et al. (2018). Glutamate triggers long-distance, calcium-based plant defense signaling. Science 361, 1112–1115. doi: 10.1126/science.aat7744
Tso, W. W., and Mansour, T. E. (1975). Thermotaxis in a slime mold, Physarum polycephalum. Behav. Biol. 14, 499–504. doi: 10.1016/S0091-6773(75)90672-0
Valentini, G., Moore, D. G., Hanson, J. R., Pavlic, T. P., Pratt, S. C., and Walker, S. I. (2018). “Transfer of information in collective decisions by artificial agents,” in 2018 Conference on Artificial Life: A Hybrid of the European Conference on Artificial Life (ECAL) and the International Conference on the Synthesis and Simulation of Living Systems (ALIFE) (Cambridge, MA: MIT Press), NO. 30, 641–648. doi: 10.1162/isal_a_00117
Wilson, E. B. (1927). Probable inference, the law of succession, and statistical inference. J. Am. Stat. Assoc. 22, 209–212. doi: 10.2307/2276774
Wohlfarth-Bottermann, K. E. (1977). Oscillating contractions in protoplasmic strands of Physarum: simultaneous tensiometry of longitudinal and radial rhythms, periodicity analysis and temperature dependence. J. Exp. Biol. 67, 49–59.
Wohlfarth-Bottermann, K. E., and Block, I. (1981). The pathway of photosensory transduction in Physarum polycephalum. Cell Biol. Int. Rep. 5, 365–373. doi: 10.1016/0309-1651(81)90006-0
Keywords: slime mold, protist, Physarum polycephalum, non-neuronal, decision-making, food choice, information theory, transfer entropy
Citation: Ray SK, Valentini G, Shah P, Haque A, Reid CR, Weber GF and Garnier S (2019) Information Transfer During Food Choice in the Slime Mold Physarum polycephalum. Front. Ecol. Evol. 7:67. doi: 10.3389/fevo.2019.00067
Received: 12 October 2018; Accepted: 22 February 2019;
Published: 19 March 2019.
Edited by:
Sasha Raoul Xola Dall, University of Exeter, United KingdomReviewed by:
Ximena J. Nelson, University of Canterbury, New ZealandAnindita Bhadra, Indian Institute of Science Education and Research Kolkata, India
Copyright © 2019 Ray, Valentini, Shah, Haque, Reid, Weber and Garnier. This is an open-access article distributed under the terms of the Creative Commons Attribution License (CC BY). The use, distribution or reproduction in other forums is permitted, provided the original author(s) and the copyright owner(s) are credited and that the original publication in this journal is cited, in accordance with accepted academic practice. No use, distribution or reproduction is permitted which does not comply with these terms.
*Correspondence: Subash K. Ray, c3I1MjNAbmppdC5lZHU=
Gabriele Valentini, Z3ZhbGVudGluaUBhc3UuZWR1