- 1Warnell School of Forestry and Natural Resources, University of Georgia, Athens, GA, United States
- 2Department of Biology, Trinity University, San Antonio, TX, United States
Developing rigorous ecological models is a fundamental goal of conservation biologists seeking to forecast biotic responses to climate change. A limitation of many models is they are amechanistic and lack integration of behavior, which is fundamental to animal biology. We integrated biophysical and agent-based models (ABM) to examine how behavior could affect the sensitivity of Plethodontid salamander activity time to climate. Specifically, our model used a temperature differential to stimulate plant climbing, a widely observed behavior among salamanders, which would allow salamanders to reduce body temperatures and associated dehydration rates. Consistent with expectations, predicted activity time was positively correlated with precipitation. The model showed that climbing plants increased activity time in drier conditions, particularly for smaller salamanders. The predicted importance of climbing behavior, a form of behavioral plasticity, was highly sensitive to assumptions about the threshold of water loss an individual was willing to tolerate. Because activity time is associated with fitness, increased activity time as a consequence of climbing behavior could moderate salamander sensitivity to shifts in weather patterns. Our results demonstrate the potential and importance of integrating behaviors into ecophysiological models when evaluating a species' potential sensitivity to climate.
Introduction
Models are important tools for understanding ecological systems and predicting how those systems may change across spatial and temporal gradients. The development of rigorous distribution, population, and performance models has reemerged as a major focus of conservation biologists attempting to forecast population responses to environmental change (Guisan et al., 2006; Araújo and New, 2007; Jackson et al., 2009; Urban et al., 2016). Due to relative ease of data accessibility, distribution models based on correlations between species presence data and environmental attributes are commonly used to make predictions about how species distributions may change under future environmental conditions (Pearson and Dawson, 2003; Araújo et al., 2005; Buckley et al., 2010). Criticisms of these bioclimatic models include the assumption that key mechanisms are captured within the correlations, missing other key parameters such as dispersal, migration, or biotic interactions, and concerns about extrapolation to future novel environments (Pearson and Dawson, 2003; Hampe et al., 2004; Araújo et al., 2005; Soberon and Peterson, 2005; Peterson et al., 2015). Mechanistic models address the assumptions outlined above and are potentially more robust than correlative bioclimatic models. For example, ecophysiological models are a type of mechanistic model that use mathematical models and fundamental principles of physics to predict how an organism's performance (e.g., metabolic rate, energy acquisition, activity, survival) varies in response to environmental variation (e.g., Peterman and Gade, 2017). Assuming that variation in individual performance correlates with spatial or temporal variation in abundance, ecophysiological models can be particularly useful for modeling species responses to environmental change (Kearney and Porter, 2009). The development of mechanistic distribution models remains inaccessible for many species because key relationships between organisms and environmental factors are poorly understood (Kearney and Porter, 2009; Buckley et al., 2010). Even for well-studied species, mechanistic models may not be robust for predicting changes in species distribution or abundance if those models do not account for other key processes such as physiological plasticity, biotic interactions, or compensatory behaviors (Buckley et al., 2010).
Behavior is a key process that—when missing—may limit the predictive capacity of ecophysiological models (Beever et al., 2017; Riddell et al., 2018). Behavior permits flexibility and is the proximate means by which most animals—particularly ectotherms—remain relatively homeostatic despite environmental heterogeneity (i.e., Hertz and Huey, 1981; Bauwens et al., 1996; Belasen et al., 2016; Muñoz and Losos, 2018). Importantly, behaviors interact with an animals' physiology to determine performance. For example, animals engage in thermoregulatory behaviors in response to extreme temperatures such as seeking warmer microhabitats when conditions are cool, and cooler microhabitats when conditions are hot. These compensatory behaviors allow animals to occupy a wider range of climates at higher abundances than might be predicted by physiological tolerances alone (Sears et al., 2011; Riddell et al., 2018). Of course, while behaviors may allow animals to occupy a wider range of climates, there are likely performance costs associated with behavioral compensation. For example, choosing to bask in the sun to increase motor function consequently requires increased caloric intake to compensate for a higher metabolic rate. Identifying how behaviors interact with physiological processes to allow animals to occupy a broader range of environments while potentially constraining performance within some environments is particularly relevant to understanding how animals may respond to future novel environments. Novel environments provide additional complexity for predicative models (Williams and Jackson, 2007), and using mechanistic models that address behavioral and physiological plasticity can yield more realistic projections about species performances in those novel environments. A few recent studies have integrated behavioral plasticity into predictive, bioclimatic models in an effort to provide more realistic projections of species' distributions and performance under current and future climates (Gifford and Kozak, 2012; Sears and Angilletta, 2015; Peterman and Gade, 2017; Riddell et al., 2018).
Individual or agent-based models (hereafter, ABMs) provide a useful platform for integration of behavior into ecophysiological models. This use of ABMs was first applied to animal systems to model recruitment in fish populations and has been subsequently used for a variety of animal species, primarily focused on movement or migration behavior (summarized in DeAngelis and Grimm, 2014). Sears and Angilletta (2015) and Sears et al. (2011) integrated behavior and physiology into an ABM to model how local thermal heterogeneity might affect lizard performance. Those studies highlighted the importance of behavioral plasticity and local environmental heterogeneity to determine lizard energetics. Beyond these examples, ABMs remain an underutilized tool in ecological research specifically seeking to understand how behavior may act as a mechanism influencing animal responses to environmental change. That is not to suggest the relationship has not been considered in other contexts or using other methods.
We integrated ecophysiological models into an agent-based modeling framework to explore the influence of two behaviors—surfacing and plant climbing—on the sensitivity of Plethodontid salamander activity to variation in air temperature, soil temperature, and relative humidity. Because Plethodontid salamanders are lungless, they are dependent on moist skin for gas exchange and vulnerable to water loss. Consequently, Plethodontid activity and performance are strongly linked to moisture and limiting water loss (Feder, 1983). The Southern Appalachian Mountains are a global hotspot for Plethodontid diversity, and within the topographically complex region, species occupy landscapes with steep natural gradients in rainfall and temperature. The region is expected to experience increased temperature and more variable precipitation over the coming century, prompting a growing number of efforts to forecast how plethodontids will respond to future climate scenarios (Milanovich et al., 2010; Gifford and Kozak, 2012; Riddell et al., 2018). We simulated weather and environmental conditions based on field measurements and estimated the effects of the threshold of water loss on surfacing activity and climbing behavior on nightly and seasonal activity times. Plethodontid salamanders are known to retreat from the surface to below ground refugia to avoid water loss, though this comes at the expense of foraging time (Fraser, 1976). Other recent models of salamander activity or energetics have included surfacing and retreating behaviors (Gifford and Kozak, 2012; Peterman et al., 2013; Caruso et al., 2014; Peterman and Gade, 2017; Riddell et al., 2018), but no prior models have considered other compensatory behaviors like plant climbing. When active above ground, plethodontids will routinely move between the ground and climbing on vegetation, which may allow animals to alter dehydration rates by altering their body temperature (McEntire, 2016). We also included multiple size classes of animal in our model, which has only been included in one prior model (Riddell et al., 2018).
Materials and Methods
Model Overview
The model estimated activity time using salamander dehydration rates. Although increased surface activity could make salamanders more vulnerable to predators, surface activity is directly correlated with foraging activity (Jaeger, 1972, 1980; Fraser, 1976), which serves a as a good proxy for fitness (Adolph and Porter, 1993). Correlations between higher predicted activity time and higher estimated density in the field (Peterman and Semlitsch, 2013, 2014), supports activity time as a good proxy for fitness despite any altered predation risk. We based our model on prior models of salamander activity and dehydration rates (Feder, 1983; Gifford and Kozak, 2012; Caruso et al., 2014; Peterman and Gade, 2017). As an additional compensatory behavior, we modeled plant climbing effects on activity, through its relationship to thermal-dehydration regulation. We varied the probability of rainfall to examine how surfacing and climbing behavior affected the sensitivity of salamander activity time to variation in precipitation patterns.
Model Landscape Development
Detailed methods and explanations of model structure are presented in the form of an “ODD” (Overview, Design concepts, and Details) protocol (Grimm et al., 2006, 2010) in the Supplementary Materials. We used NetLogo (Version 6.0.2, U. Wilensky, 1999) to simulate salamander activity on a 50 × 50 tile landscape, with each tile representing one square meter of forest habitat. Daily probability of rainfall ranged from 0.3 to 0.9 and was consistent for all cells in the landscape to create a gradient from relatively wet to dry climates among simulations. The simulations ran 20 times for each set of parameters and a single simulation lasted for one active season (April through October) with alternating day and night time steps (428 total steps). “Night” time steps varied in absolute length in terms of minutes, dependent on the monthly hours of darkness (see below), and dehydration rate was calculated on this minute-by-minute scale. The code is available from the authors upon request.
We simulated weather events, including rainfall events and nightly temperature, once every “24 h” (two time steps) for the entire virtual landscape, where all cells maintained the same values. Rainfall events occurred based on a probability ranging from 0.3 to 0.9 (in 0.05 increments). These events were assumed to be large enough to saturate the ground surface, but rainfall amounts were not directly modeled. Because rainfall events were used to determine relative humidity, the amount of rainfall would not be as important as frequency of events in this simulation. During rainfall events the relative humidity was set at 100%. Relative humidity is the result of a large number of interacting factors including cloud cover, temperature, air pressure, and rainfall events. For simplicity, when not raining, the humidity decreased by 10% each day it did not rain. Though a simplification of how relative humidity fluctuates, it created variability over time within the virtual environment, and comparable values to those observed during field studies (Howard, 2018). The model simulated seasonal changes in air temperature by generating temperatures from a random-normal distribution using the monthly average and standard deviation for nightly temperature data recorded at the Coweeta Hydrologic Laboratory at 5 stations spread over an area of 25 square kilometers from 2013 to 2014 (Miniat et al., 2017). Soil temperature was set to the average monthly soil temperature at 5 cm (Miniat et al., 2017). We used temperature data collected during this time period, because it was all that was available at the time of model development. Directly modeling vapor pressure deficit rather than calculating it may have been more efficient, but it is more challenging to model over time as it depends on relative humidity which is also difficult to simulate. Although a simplification, because air temperatures are typically measured at a height above the plants, plant temperatures are generally intermediate between measured soil and air temperatures (Geiger, 1965); therefore, we set vegetation surface temperature to an average between the soil and air temperature. Hours of daylight were set for each month to the monthly average for the latitude of the Coweeta Hydrologic Laboratory (35° 3′35.70″N, http://aa.usno.navy.mil/data/docs/Dur_OneYear.php).
Agent Simulation
The agents were modeled as salamanders in the genus Plethodon, specifically the Plethodon jordani species complex. We included three size classes of individuals: hatchlings [snout-vent-length (SVL) < 32 mm], juveniles (SVL 32–42 mm), and adults (SVL 43–72 mm). We simulated 1,000 agents of each size class for a total of 3,000 agents randomly distributed across the uniform landscape, and we did not include any density-dependent affects.
Resistance to water loss is a critical value for estimating activity time using biophysical models. Some studies found amphibians' skin acts as a free water surface (Spotila and Berman, 1976), meaning their resistance value is very close to zero. However, other studies have found species-specific differences and variable resistance values based on environmental conditions with values greater than zero (Littleford et al., 1947; Cohen, 1952; Ray, 1958; Spotila, 1972; Riddell and Sears, 2015; Riddell et al., 2017). We used data for P. jordani reported in Spotila (1972) to create a function of predicted resistance to water loss based on soil temperature Equation (1). We used soil temperature as the primary temperature because salamanders regularly experience soil temperatures for extended periods compared to brief episodic climbing events. Therefore, we expect salamanders to be physiologically acclimated to ambient soil temperatures. There is little data on acclimation rates of salamander physiological processes, but available studies suggest processes such as water loss rate are unlikely to occur within the time frame of a single night of foraging (e.g., Riddell et al., 2017).
Where R is the resistance to water loss in seconds per centimeter and Ts is the soil temperature in Celsius. While recent physiological models suggested the importance of including calculations of boundary layer resistance as part of the estimate of salamander's resistance to water loss (Riddell et al., 2017), we used literature values for skin resistance based on field-recorded water loss, which effectively included the boundary layer resistance in the value of skin resistance to water loss. Riddell et al. (2018) recently demonstrated seasonal plasticity in skin resistance, suggesting the monthly changes in water loss resistance in our model were appropriate.
We assumed body temperature was equivalent to the surface the salamander was sitting on. This deviates from previous studies that estimated operative body temperature using mathematical equations (Gifford and Kozak, 2012; Peterman and Gade, 2017). Body temperatures measured in the field were equivalent to the temperature of the substrate upon which they were found (McEntire, 2018) and were on average 2.38 ± 1.04°C cooler than the air temperature (McEntire, 2018). These relationships, along with previous studies using the temperature of the substrate beneath the salamander as the body temperature (Feder and Lynch, 1982), suggest it was reasonable to assume modeled soil temperature as the agents' initial body temperature. If a salamander remained on the ground, they maintained the soil temperature as their body temperature; when a salamander climbed on vegetation, they adopted the temperature of the plant as their body temperature. Salamanders on the ground maintained consistent conditions; we did not include the possible differences in microclimate above or below leaf litter. We assumed consistent relative humidity and still (no wind) conditions for both agents on the ground and climbing.
When air temperatures were cooler than soil temperatures, agents had a 0.50 probability of climbing once they were surface active. This value was not chosen to represent natural climbing rates, which remain unknown. By setting a fixed probability of climbing, this resulted in ~50% of the agents climbing on any given evening, which allowed us to compare differences in activity time between those agents that climbed and those that did not. We did not allow salamanders to climb when soil temperatures were cooler than air temperatures because we assumed that this would create conditions where dehydration was always faster for climbing animals. This was not to imply that real animals would not climb under such conditions in the field (see McEntire, 2018).
Model Process
We constrained surface activity based on field observations and other published studies to times when: (1) the relative humidity was above 45% and (2) the animal's water deficit was <4% (Feder and Londos, 1984). Although salamanders sometimes retreated before reaching 4% water loss (the 3% water loss threshold simulations), this threshold offered the possibility of losing water while in the soil or failing to fully rehydrate. Simulated body temperatures did not fall out of the range of active temperatures used in previous studies (Peterman and Gade, 2017; Riddell et al., 2018), so this restriction was not included. The salamanders had one opportunity to surface at the beginning of the night and remained active until they reached their water loss threshold. Nightly foraging time in minutes was calculated based on dehydration rate Equation (2)
where EWL is evaporative water loss (g cm−2 sec−1); R is the resistance value of the salamander to dehydration (sec cm−1); ρsal is the vapor pressure density at the surface of the salamander and is assumed to be equal to the saturation vapor pressure density (Tracy, 1976); ρair is the vapor pressure density of the air given the relatively humidity. We estimated these values based on standard equations related to temperature (Supplementary Materials). We multiplied the evaporative water loss rate by an agent's surface area (cm2) to estimate grams of water lost per minute. The agents remained active until they lost 3–10% of their body mass, which is the threshold range observed in dehydration studies and used in other models (Ray, 1958; Feder and Londos, 1984; Gifford and Kozak, 2012; Caruso et al., 2014; Peterman and Gade, 2017; Riddell et al., 2018). For each simulation, the percentage of water lost was held constant and consistent among all individuals, but we compared 3, 5, 7, and 10% water loss thresholds because previous models suggested high sensitivity to this value (Peterman and Gade, 2017).
When simulated salamanders were inactive, either during the day, at night when not surfacing, or after retreating for the night, they rehydrated on a minute-by-minute basis as determined by soil temperature and soil moisture Equation (3) (Spotila et al., 1992):
Where rehydration is measured in grams per cm2 per minute, φsal is the water potential of the salamander in pascals, φsoil is the soil moisture tension in pascals, and K is the hydraulic conductance, set at 0.00000013 g cm−2 min−1 pa−1 as calculated for Leopard frogs, Rana pipiens, (Tracy, 1976). The water flux of the salamanders was estimated using the following Equation (4) established for Leopard frogs (Tracy, 1976).
Where θ is the hydration level of the salamander (1—percent of mass lost to water loss). These parameters are currently unavailable for salamanders; however, the leopard frog values produce reasonable rehydration rates reflective of those found in previous studies with salamanders (Cohen, 1952; Spight, 1967a,b; Spotila, 1972; Feder, 1983). Soil moisture was modeled simply and remained well above the minimum water potential for salamanders to rehydrate (−2 atm, Spight, 1967a,b; Spotila, 1972) as modeling soil moisture dynamics went beyond the scope of this study.
Agents recorded their nightly foraging time in minutes, which allowed for comparison of nightly activity with simulated environmental factors. At each “night” time step, we recorded the average nightly activity time of each size class. When climbing occurred, we divided the agents into “climbers” and “non-climbers,” then recorded the average nightly time for each size class in both categories.
Sensitivity Analysis
We conducted a sensitivity analyses for the assigned threshold for water loss. We did not conduct sensitivity analyses on other model values that have been validated by previous studies or were based on the best available published values (Table 1; Whitford and Hutchison, 1967; Spotila, 1972; Tracy, 1976; Peterman and Gade, 2017). There were 52 possible simulations combining possible rainfall probabilities and thresholds for water loss.
Data Analysis
We limited comparison of nightly activity between climbing and non-climbing salamanders to simulated nights when air temperature was cooler than soil temperature. We subtracted the recorded average activity time of non-climbing agents from the time for climbing agents to determine any difference in nightly activity time associated with the behavior. We visually examined the effects of weather, body size class, and threshold for water loss on differences in nightly activity time between climbing and not climbing salamanders. We used general additive models with smoothing to make patterns easier to visualize. To evaluate the effects of climbing on sensitivity of salamanders to “climate,” we summed the nightly activity times for each size class and rainfall probability per simulation across the rainfall probability gradient. We assumed animals were active every night of the simulation, so the absolute values do not reflect true estimates of seasonal activity for salamanders.
Results
As part of the model structure, climbing only occurred on nights when simulated air temperature was lower than soil temperature. This resulted in ~85 nights when climbing could occur out of 214 nights per active season simulation. The maximum potential difference in nightly activity time between climbing and non-climbing animals ranged from 6.1 to 7.0 h. There were a few instances when climbing resulted in lower activity times compared to remaining on the ground, with those differences not exceeding 22 min. During rainy nights with only slight differences between soil and air temperature, there was little to no difference in activity time between climbing and non-climbing salamanders (Figure 1). As the temperature difference between the ground and air increased, the benefits of climbing increased for all size classes of salamander and all thresholds of water loss (Figure 1). Hatchlings were the most responsive to very small temperature differences regardless of water loss threshold or time since last rainfall (Figure 1C). Importantly, as conditions dried [days since rain increased], the advantages of climbing manifest with a smaller temperature difference between the ground and air, though days since rain also constrained the realized activity time because of the negative relationship to relative humidity. The general patterns of increased activity among climbing animals with increasing temperature difference did not vary with the threshold of water loss; however, the benefits of climbing manifest at a lower temperature difference as the threshold for water loss declined (Figure 1). The unimodal patterns of differences in activity time as a function of temperature differences during rainy nights reflected other constraining conditions. Specifically, in our observed weather data used to simulate weather in the model, we observed air temperatures ~5+ °C cooler than soil temperatures when cold fronts moved in, creating near freezing air temperatures while soils remained relatively warm (Figure 2). These cold conditions constrained salamander activity independent of factors affecting dehydration rates. Across our rainfall probability gradient, total seasonal activity time increased with increasing probability of rainfall (wetter climate), and the benefit of climbing was consistent across the rainfall probability gradient (Figure 3). Overall, climbing had the largest benefit for hatchlings across the rainfall probability gradient (Figure 3). The total seasonal activity time was sensitive to the water loss threshold, with increasing activity time in relation to increased thresholds (Figure 3).
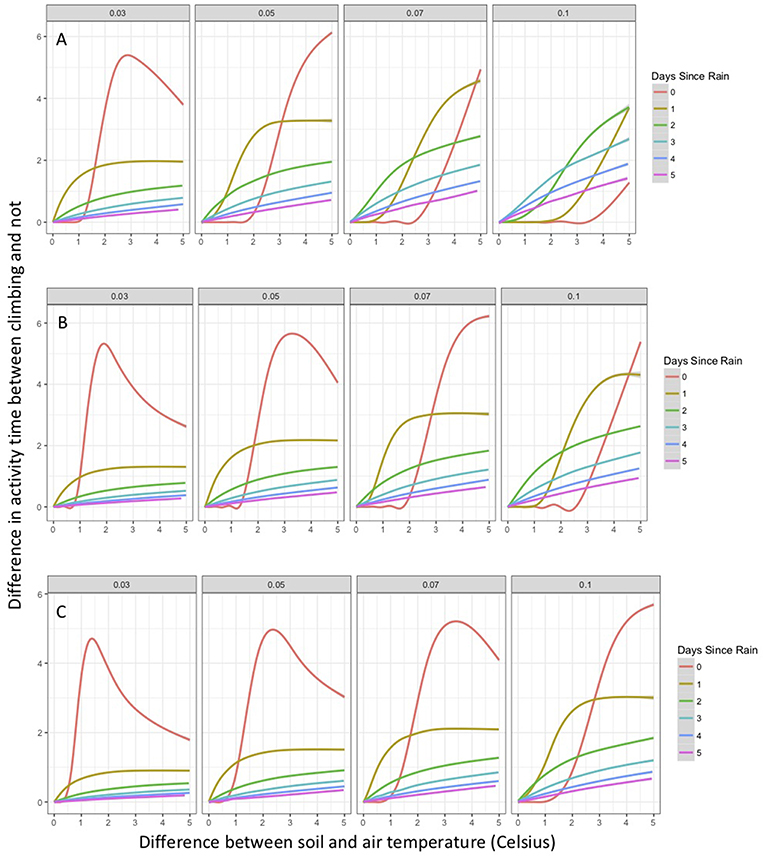
Figure 1. Percent change in activity time of climbing compared to non-climbing salamanders as a function of the difference between air and ground temperature (limited to most common values), days since last rain, water loss threshold, and body size class. Shown is the generalized additive model of the percent increase in mean activity time for non-climbing salamanders compared to the mean activity time of climbing salamanders on the same simulated night. Positive value indicates greater activity time among climbing salamanders. The gray area around each line represents a 95% confidence interval. (A) adult salamanders, (B) juvenile salamanders, and (C) hatchling salamanders.
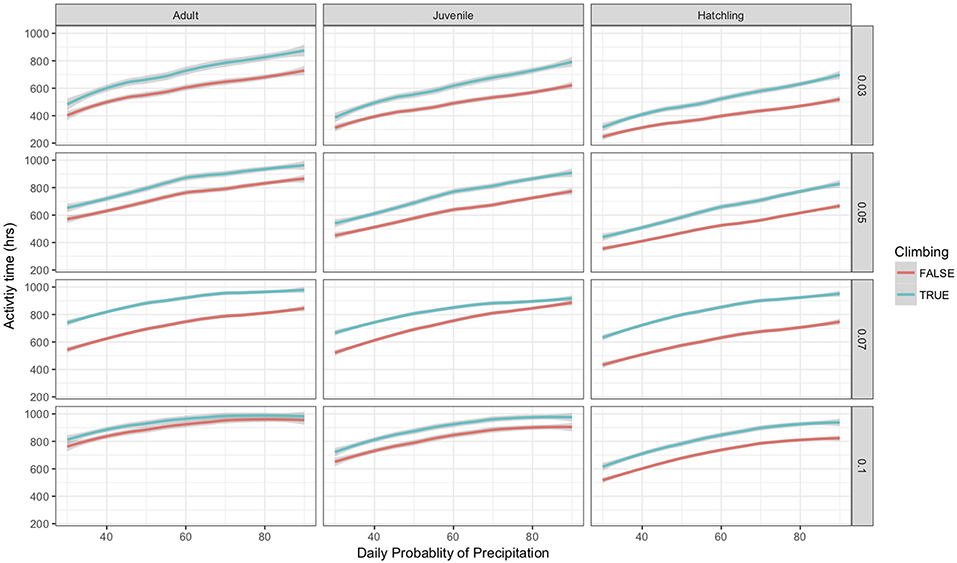
Figure 3. Estimated mean total seasonal activity time, restricted to nights when climbing occurred, for climbing and non-climbing adult, juvenile, and hatchling salamanders as a function of the probability of precipitation and water loss threshold in the model simulation. The lines were smoothed using a logarithmic linear model fit to the data, gray bands surrounding each line indicate a 95% confidence interval. Each water-loss threshold is shown individually as indicated by the far right axis.
Discussion
Inclusion of climbing behavior increased activity time of salamanders under the specified conditions and acted as a compensatory behavior when interacting with both time since last rain and differences in air and soil temperatures. As a compensatory behavior, climbing may decrease the predicted sensitivity of salamanders to climate change compared to models that do not including climbing. This is broadly consistent with other recent models that show the inclusion of behavioral and physiological plasticity reduces the predicted impact of climate warming on the performance and distribution of salamanders (Riddell et al., 2018) and other taxa (Sears et al., 2011; Sears and Angilletta, 2015). In laboratory studies, Plethodon cinereus and P. metcalfi have demonstrated physiological plasticity in response to temperature (Riddell and Sears, 2015; Novarro et al., 2018). An interesting outcome of our model results is the demonstration that the addition of climbing behavior creates a mechanism by which salamanders that occupy areas with lower rainfall probabilities can realize comparable amounts of activity time to salamanders that occupy areas with a higher rainfall probability. We caution that these inferences do not account for any costs that may be associated with climbing such as increased predation pressure or altered temperature-dependent metabolic rate. In addition, variation in rainfall likely affects productivity and food availability independent of activity time, and we are assuming that equivalent activity time translates to equivalent food intake rates regardless of whether the salamander is climbing or not. This assumption has not been validated in the field. Jaeger (1978) found that eastern red-backed salamanders (P. cinereus) had larger, higher-quality prey in their stomachs compared to animals found on the ground; and Mitchell and Taylor (1986) reported arboreal invertebrates in the diets of red-legged salamanders (P. shermani), suggesting climbing salamanders can access specific prey. However, a more recent study of P. shermani found similar diets and volume of prey between individuals collected on plants and those collected on the ground (Lewis et al., 2014), and Roberts and Liebgold (2008) estimated that potential prey of P. cinereus were actually less abundant on plants compared to the ground. Therefore, it remains to be determined whether salamanders that climb are able to translate increased activity time to equal or greater intake of prey or some other fitness benefit.
Assuming that climbing is adaptive, particularly in areas with less frequent rainfall, our model results would suggest a shallower relationship between rainfall gradients and salamander abundance than would be predicted by models that exclude climbing or other plastic traits. Because salamander activity is tied to rainfall, this may suggest climbing offers a mechanism for salamanders to increase their activity time in drier areas. Our predictions are consistent with other studies that find hatchling salamanders should be most sensitive to drier conditions (created by either declining precipitation or increased temperature, Riddell et al., 2018). We currently lack empirical data that can be used to test this prediction. Our model predictions are generally consistent with two studies that demonstrate both reduced spatial abundance of salamanders in relation to mean annual precipitation (Howard, 2018) or soil moisture (Peterman and Semlitsch, 2013). In both studies, the largest contributor to declining abundance was the decline in hatchling or juvenile abundance, which is also consistent with model predictions. Howard (2018) estimated salamander abundance and population structure across the Coweeta basin, which served as the context for our simulation model and was the source of our weather conditions in simulations. Her results demonstrate a rather shallow decline in abundance over a large range of mean annual precipitation until condition become very dry. Qualitatively, the shape of this relationships is similar to our modeled estimates of activity time across the rainfall probability gradient.
One powerful use of models is to examine and estimate latent processes that cannot be observed. It is currently not practical (or likely even possible) to directly measure salamander activity time in the field, yet it is widely accepted as a critical currency in salamander fitness. One emergent pattern in our model was that the benefits of climbing increase as time since rain decreases (decreased relative humidity). This contradicts earlier characterizations of this behavior. Jaeger (1978) hypothesized that dehydration during plant climbing would restrict the behavior to rainy nights. Our model results, across a range of weather conditions and thresholds for water loss, suggest the benefits of climbing to activity time increase with time since rain and are instead likely to be determined by differences in temperature between the ground and air rather than rain events. In fact, at higher water loss thresholds, which have been demonstrated for our model Plethodon species (Riddell et al., 2018), our model predicts little to no advantage in terms of activity time for climbing during rainy nights unless extreme temperature differences occur. Although the model is constrained by the coded rules, this interaction between temperature and relative humidity (a function of days since rain) was not directly coded and was an emergent result. Whether this predicted relationship is true requires field validation.
There are some important limitations to our model in terms of predicting the advantages of climbing or when we expect to see increases of climbing in the field. First, as mentioned previously, our model does not incorporate potential costs that may be associated with climbing. Second, in addition to regulating water loss, salamanders may be selecting among thermal microhabitats to optimize metabolic rate and may be balancing increases in activity time with metabolic efficiency (Riddell et al., 2018). Though our model can integrate metabolic rates and efficiencies, we did not evaluate that parameter in these simulations. In our model, we strictly evaluate the benefits of climbing on activity time as a function of water loss, because any potential costs are unknown. Our results suggest that—generally—the benefits of climbing increase as the air becomes progressively cooler compared to the ground. In reality, weather data indicate that this occurs most frequently during cooler seasons when the ground is warmer but the air is particularly cold. Under these conditions, it is reasonable that a salamander would remain on the ground where temperatures are more metabolically optimal even though their dehydration rate would be greater and their activity time shorter. Ultimately, field studies that can directly or indirectly relate activity time to behavior as a function of weather are needed to validate our and other models and the importance of behaviors in determining salamander fitness and demography.
Another outstanding issue in ecophysiological models of salamanders and other amphibians is the threshold for evaporative water loss before an animal will cease activity and retreat into moist refugia. An early laboratory study on Desmognathus ochrophaeus suggested salamanders will “give up” activity at much lower levels of water loss than they can tolerate before becoming impaired, and that water loss thresholds may be plastic depending on the dehydration potential of the environment (Feder and Londos, 1984). A model by Peterman and Gade (2017) estimated similar high sensitivity to the threshold for water loss for Plethodon. However, field measures of Plethodon metcalfi water deficits recorded by Riddell et al. (2018), suggested the species may tolerate higher water loss thresholds than has been demonstrated in the lab or through other models. The sensitivity of our and other model estimates to an assumed water loss threshold (e.g., Gifford and Kozak, 2012; Peterman and Gade, 2017; Riddell et al., 2018) illustrates the need to better understand this parameter, particularly for models that will be used in climate change projections. Moreover, differences in water loss resistance and threshold water loss tolerances among size classes and species would aid in understanding behavioral differences in the field and comparing the relative sensitivity of species to spatial and temporal climate change. For example, our model predicts that size classes or species with reduced water loss resistance or lower water loss thresholds or greater surface area to volume ratios would realize a greater benefit from climbing at a lower temperature differential. We would expect these size classes or species to show a greater propensity to climb compared to larger size classes or species with greater skin resistance to water loss.
A final limitation of our model, and all models to date, was our inability to deal with natural variation in soil moisture and soil tension. Our model does include some variability in soil moisture, but we were unable to provide additional insight about this poorly understood relationship. During model development, when soil moisture dropped below −0.2 atm, the majority of animals would “die” from an inability to rehydrate in the soil. However, Spotila (1972) and Spight (1967a) demonstrated that Plethodon could rehydrate up to a soil moisture of −2 atm. Salamanders are also known to create a “wetting front” to slow water loss in dry soils (Spotila, 1972), but the mechanism and the conditions under which this front is formed along with the hydraulic conductance or water flux rates for salamanders remains undescribed. This indicates that current rehydration models for salamanders [and other amphibians] are incomplete. This also illustrates that while salamanders spend a large portion of their time underground or in retreats, all current models of salamander interactions with climate have focused only on their time above ground (i.e., Walls, 2009; Milanovich et al., 2010; Gifford and Kozak, 2012; Ficetola et al., 2016; Peterman and Gade, 2017; Riddell et al., 2018). Until our knowledge gap about salamander below ground relationships to soil conditions is filled, our models of these organisms will remain incomplete and prone to error. This illustrates a wider problem with the development of mechanistic models. Plethodontid salamanders are a group that are well-studied and for which there is a relatively rich physiological literature, and yet our current knowledge is still insufficient to construct a full working physiological model of how these animals interact with their environment (Gifford, 2016). Knowledge of most other animal species is far more deficient, which will limit the ability to develop and apply mechanistic models to understanding the performance of those species in current or future novel environments.
Because of the heterogeneous nature of most environments, most animals use behaviors to compensate for less suitable conditions that might otherwise limit their performance and ability to occupy a site. Behavioral and physiological plasticity and acclimation are one of the most proximate phenotypic responses of animals to environmental change, and compensatory behaviors and plasticity in physiology act as key mechanisms for acclimatization to seasonal climates or short-term changes in weather, food availability, predation (Muñoz et al., 2016; Beever et al., 2017). These compensatory traits, therefore, shape the potential sensitivity of animals to longer-term environmental changes (Beever et al., 2017). After field validation, by including multiple behaviors and physiological processes into mechanistic models, we can make more robust predictions about how organisms might respond to future novel environments. Although recent models are incomplete, the demonstration by several recent studies that the integration of behavioral and physiological plasticity dramatically reduces the projected impacts of climate change on some species is heartening and should have important ramifications for how we think about threats and conservation actions.
Data Availability Statement
The raw data supporting the conclusions of this manuscript will be made available by the authors, without undue reservation, to any qualified researcher.
Author Contributions
JM contributed to idea generation, designing sensitivity analyses, and writing. KM was primarily responsible for model development and contributed to idea generation and writing.
Conflict of Interest Statement
The authors declare that the research was conducted in the absence of any commercial or financial relationships that could be construed as a potential conflict of interest.
Acknowledgments
We thank Dr. Jeffrey Hepinstall-Cymerman, Heather Abernathy, Jenny Asper, Jillian Howard, and Elizabeth Hunter for assistance with model development. This research was supported by two National Science Foundation awards from the Long Term Ecological Research Program to the Coweeta LTER Program at the University of Georgia (DEB-0823293 and DEB-1637522). Additional support was provided by the Daniel B. Warnell School of Forestry and Natural Resources at the University of Georgia and the Graduate School at the University of Georgia. Precipitation data was provided by the Coweeta LTER and the USDA Forest Service. We thank Dr. Chelcy Miniat for logistical support and for permission to use Forest Service data when appropriate.
Supplementary Material
The Supplementary Material for this article can be found online at: https://www.frontiersin.org/articles/10.3389/fevo.2019.00022/full#supplementary-material
References
Adolph, S. C., and Porter, W. P. (1993). Temperature, activity, and lizard life histories. Am Nat. 142, 273–295. doi: 10.1086/285538
Araújo, M. B., and New, M. (2007). Ensemble forecasting of species distributions. Trends Ecol. Evol. 22, 42–47. doi: 10.1016/j.tree.2006.09.010
Araújo, M. B., Whittaker, R. J., Ladle, R. J., and Erhard, M. (2005). Reducing uncertainty in projections of extinction risk from climate change. Glob. Ecol. Biogeogr. 14, 529–538. doi: 10.1111/j.1466-822X.2005.00182.x
Bauwens, D., Hertz, P. E., and Castilla, A. M. (1996). Thermoregulation in a lacertid lizard: the relative contributions of distinct behavioral mechanisms. Ecology 77, 1818–1830.
Beever, E. A., Hall, L. E., Varner, J., Loosen, A. E., Dunham, J. B., Gahl, M. K., et al. (2017). Behavioral flexibility as a mechanism for coping with climate change. Front. Ecol. Environ. 1–10. doi: 10.1002/fee.1502
Belasen, A., Brock, K., Li, B., Chremou, D., Valakos, E., Pafilis, P., et al. (2016). Fine with heat, problems with water: microclimate alters water loss in a thermally adapted insular lizard. Oikos 126, 447–457 doi: 10.1111/oik.03712
Buckley, L. B., Urban, M. C., Angilletta, M. J., Crozier, L. G., Rissler, L. J., and Sears, M. W. (2010). Can mechanism inform species' distribution models? Ecol. Lett. 13, 1041–1054. doi: 10.1111/j.1461-0248.2010.01479.x
Caruso, N. M., Sears, M. W., Adams, D. C., and Lips, K. R. (2014). Widespread rapid reductions in body size of adult salamanders in response to climate change. Glob. Chang. Biol. 20, 1751–9. doi: 10.1111/gcb.12550
Cohen, N. (1952). Comparative rates of dehydration and hydration in some california salamanders. Ecology 33, 462–479. doi: 10.2307/1931521
DeAngelis, D. L., and Grimm, V. (2014). Individual-based models in ecology after four decades. F1000Prime Rep. 6:39. doi: 10.12703/P6-39
Feder, M. (1983). Integrating the ecology and physiology of plethodontid salamanders. Herpetologica 39, 291–310.
Feder, M., and Londos, P. (1984). Hydric constraints upon foraging in a terrestrial salamander, Desmognathus ochrophaeus (Amphibia : Plethodontidae). Oecologia 64, 413–418. doi: 10.1007/BF00379141
Feder, M., and Lynch, J. (1982). Effects of latitude, season, elevation, and microhabitat on field body temperatures of neotropical and temperate zone salamanders. Ecology 63, 1657–1664. doi: 10.2307/1940107
Ficetola, G. F., Colleoni, E., Renaud, J., Scali, S., Padoa-Schioppa, E., and Thuiller, W. (2016). Morphological variation in salamanders and their potential response to climate change. Glob. Chang. Biol. 20, 2013–2024. doi: 10.1111/gcb.13255
Fraser, D. (1976). Empirical evaluation of the hypothesis of food competition in salamanders of the genus plethodon. Ecology 57, 459–471. doi: 10.2307/1936431
Gifford, M. E. (2016). Physiology of Plethodontid salamanders: a call for increased efforts. Copeia 2016, 42–51. doi: 10.1643/OT-14-223
Gifford, M. E., and Kozak, K. H. (2012). Islands in the sky or squeezed at the top? Ecological causes of elevational range limits in montane salamanders. Ecography (Cop.). 35, 193–203. doi: 10.1111/j.1600-0587.2011.06866.x
Grimm, V., Berger, U., Bastiansen, F., Eliassen, S., Ginot, V., Giske, J., et al. (2006). A standard protocol for describing individual-based and agent-based models. Ecol. Modell. 198, 115–126. doi: 10.1016/j.ecolmodel.2006.04.023
Grimm, V., Berger, U., DeAngelis, D. L., Polhill, J. G., Giske, J., and Railsback, S. F. (2010). The ODD protocol: A review and first update. Ecol. Modell. 221, 2760–2768. doi: 10.1016/j.ecolmodel.2010.08.019
Guisan, A., Lehmann, A., Ferrier, S., Austin, M., Overton, J. M. C., Aspinall, R., et al. (2006). Making better biogeographical predictions of species' distributions. J. Appl. Ecol. 43, 386–392. doi: 10.1111/j.1365-2664.2006.01164.x
Hampe, A., Pearson, R. G., and Dawson, T. P. (2004). Bioclimate envelope models: what they detect and what they hide. Glob. Ecol. Biogeogr. 13, 469–471. doi: 10.1111/j.1466-822X.2004.00090.x
Hertz, P. E., and Huey, R. B. (1981). Compensation for altitudinal changes in the thermal environment by some anolis lizards on hispaniola. Ecology 62, 515–521. doi: 10.2307/1937714
Howard, J. (2018). Modeling the Effects of Precipitation on Salamander Demography for Conservation Planning. Dissertation, University of Georgia, Athens, GA.
Jackson, S. T., Betancourt, J. L., Booth, R. K., and Gray, S. T. (2009). Ecology and the ratchet of events : climate variability, niche dimensions, and species distributions. Proc. Natl. Acad. Sci. U.S.A. 106, 19685–19692. doi: 10.1073/pnas.0901644106
Jaeger, R. (1972). Food as a limited resource in competition between two species of Terrestrial salamanders. Ecology 53, 535–546. doi: 10.2307/1934249
Jaeger, R. (1978). Plant climbing by salamanders : periodic availability of plant-dwelling prey. Copeia 1978, 686–691. doi: 10.2307/1443697
Jaeger, R. G. (1980). Fluctuations in prey availability and food limitation for a terrestrial salamander. Oecologia 44, 335–341. doi: 10.1007/BF00545237
Kearney, M., and Porter, W. (2009). Mechanistic niche modelling: combining physiological and spatial data to predict species' ranges. Ecol. Lett. 12, 334–350. doi: 10.1111/j.1461-0248.2008.01277.x
Lewis, J. D., Connette, G. M., Deyrup, M. A., Carrel, J. E., and Semlitsch, R. D. (2014). Relationship between diet and microhabitat use of red-legged salamanders (Plethodon shermani) in Southwestern North Carolina. Copeia 2014, 201–205. doi: 10.1643/CE-13-089
Littleford, R., Keller, W., and Phillips, N. (1947). Studies on the vital limits of water loss in the plethodont salamanders. Ecology 28, 440–447. doi: 10.2307/1931231
McEntire, K. D. (2016). Arboreal ecology of plethodontidae: a review. Copeia 2016, 124–131. doi: 10.1643/OT-14-214
McEntire, K. D. (2018). Integration of Behavior Into Biophysical Models to Estimate Salamander Sensitivity to Climate and Midstory Forest Management. Dissertation, University of Georgia, Athens, GA.
Milanovich, J. R., Peterman, W. E., Nibbelink, N. P., and Maerz, J. C. (2010). Projected loss of a salamander diversity hotspot as a consequence of projected global climate change. PLoS ONE 5:e12189. doi: 10.1371/journal.pone.0012189
Miniat, C. F., Laseter, S. H., Swank, W. T., and Swift, L. W. Jr. (2017). Daily Precipitation Data From Recording Rain Gages (RRG) at Coweeta Hydrologic Lab, North Carolina. Fort Collins, CO: Forest Service Research Data Archive. (Accessed May 31, 2018).
Mitchell, J., and Taylor, J. (1986). Predator-prey size relationships in a north carolina population of Plethodon jordani. J. Herpetol. 20, 562–566. doi: 10.2307/1564254
Muñoz, D. J., Hesed, K. M., Grant, E. H. C., and Miller, D. A. W. (2016). Evaluating within-population variability in behavior and fitness for the climate adaptive potential of a dispersal-limited species, Plethodon cinereus. Ecol. Evol. 6, 8740–8755. doi: 10.1002/ece3.2573
Muñoz, M. M., and Losos, J. B. (2018). Thermoregulatory behavior simultaneously promotes and forestalls evolution in a tropical lizard. Am. Nat. 191, E15–E26. doi: 10.1086/694779
Novarro, A. J., Gabor, C. R., Goff, C. B., Mezebish, T. D., Thompson, L. M., and Grayson, K. L. (2018). Physiological responses to elevated temperature across the geographic range of a terrestrial salamander. J. Exp. Biol. 221:jeb178236. doi: 10.1242/jeb.178236
Pearson, R. G., and Dawson, T. P. (2003). Predicting the impacts of climate change on the distribution of species: are bioclimate envelope models useful? Glob. Ecol. Biogeogr. 12, 361–371. doi: 10.1046/j.1466-822X.2003.00042.x
Peterman, W., Locke, J., and Semlitsch, R. (2013). Spatial and temporal patterns of water loss in heterogeneous landscapes: using plaster models as amphibian analogues. Can. J. Zool. 140, 135–140. doi: 10.1139/cjz-2012-0229
Peterman, W. E., and Gade, M. (2017). The importance of assessing parameter sensitivity when using biophysical models : a case study with a Plethodontid salamander. Popul. Ecol. 29, 275–286. doi: 10.1007/s10144-017-0591-4
Peterman, W. E., and Semlitsch, R. D. (2013). Fine-scale habitat associations of a terrestrial salamander: the role of environmental gradients and implications for population dynamics. PLoS ONE 8:e62184. doi: 10.1371/journal.pone.0062184
Peterman, W. E., and Semlitsch, R. D. (2014). Spatial variation in water loss predicts terrestrial salamander distribution and population dynamics. Oecologia 176, 357–369. doi: 10.1007/s00442-014-3041-4
Peterson, A. T., Papeş, M., and Soberón, J. (2015). Mechanistic and correlative models of ecological niches. Eur. J. Ecol. 1, 28–38. doi: 10.1515/eje-2015-0014
Ray, C. (1958). Vital limits and rates of desiccation in salamanders. Ecology 39, 75–83. doi: 10.2307/1929968
Riddell, E. A., Apanovitch, E. K., Odom, J. P., and Sears, M. W. (2017). Physical calculation of resistance to water loss improve predictions of species range models. Ecol. Monogr. 87, 1–50. doi: 10.1002/ecm.1240
Riddell, E. A., Odom, J. P., Damm, J. D., and Sears, M. W. (2018). Plasticity reveals hidden resistance to extinction under climate change in the global hotspot of salamander diversity. Sci. Adv. 4:eaar5471. doi: 10.1126/sciadv.aar5471
Riddell, E. A., and Sears, M. W. (2015). Geographic variation of resistance to water loss within two species of lungless salamanders : implications for activity. Ecosphere 6:art86. doi: 10.1890/ES14-00360.1
Roberts, A. M., and Liebgold, E. B. (2008). The effects of perceived mortality risk on habitat selection in a terrestrial salamander. Behav. Ecol. 19, 621–626. doi: 10.1093/beheco/arn012
Sears, M. W., and Angilletta, M. J. (2015). Costs and benefits of thermoregulation revisited: both the heterogeneity and spatial structure of temperature drive energetic costs. Am. Nat. 185, E94–E102. doi: 10.1086/680008
Sears, M. W., Raskin, E., and Angilletta, M. J. (2011). The world is not flat: defining relevant thermal landscapes in the context of climate change. Integr. Comp. Biol. 51, 666–675. doi: 10.1093/icb/icr111
Soberon, J., and Peterson, A. T. (2005). Interpretation of models of fundamental ecologicial niches and species' distributional areas. Biodivers. Informatics 2, 1–10. doi: 10.17161/bi.v2i0.4
Spight, T. M. (1967a). The water economy of salamanders : exchange of water with the soil. Biol. Bull. 132, 126–132. doi: 10.2307/1539882
Spight, T. M. (1967b). The water economy of salamanders: water uptake after dehydration. Comp. Biochem. Physiol. 20, 767–771. doi: 10.1016/0010-406X(67)90050-3
Spotila, J. (1972). Role of temperature and water in the ecology of lungless salamanders. Ecol. Monogr. 42, 95–125. doi: 10.2307/1942232
Spotila, J. R., and Berman, E. N. (1976). Determination of skin resistance and the role of the skin in controlling water loss in amphibians and reptiles. Comp. Biochem. Physiol. Part A Physiol. 55, 407–411. doi: 10.1016/0300-9629(76)90069-4
Spotila, J. R., O'Connor, M. P., and Bakken, G. S. (1992). “Biophysics of Heat and Mass Transfer,” in Environmental Physiology of the Amphibians, eds M. E. Feder and W. W. Burggren (Chicago, IL: University of Chicago Press), 59–80.
Tracy, C. (1976). A model of the dynamic exchanges of water and energy between a terrestrial amphibian and its environment. Ecol. Monogr. 46, 293–326. doi: 10.2307/1942256
Urban, M. C., Bocedi, G., Hendry, A. P., Mihoub, J. B., Pe'er, G., Singer, A., et al. (2016). Improving the forecast for biodiversity under climate change. Science 353:aad8466. doi: 10.1126/science.aad8466
Walls, S. C. (2009). The role of climate in the dynamics of a hybrid zone in Appalachian salamanders. Glob. Chang. Biol. 15, 1903–1910. doi: 10.1111/j.1365-2486.2009.01867.x
Whitford, W. G., and Hutchison, V. H. (1967). Body size and metabolic rate in salamanders. Physiol. Zool. 40, 127–133. doi: 10.1086/physzool.40.2.30152447
Wilensky, U. (1999). NetLogo. Evanston, IL: Center for Connected Learning and Computer-Based Modeling, Northwestern University. Available online at: http://ccl.northwestern.edu/netlogo/
Keywords: individual-based models, amphibian, conservation, ecology, management
Citation: McEntire KD and Maerz JC (2019) Integrating Ecophysiological and Agent-Based Models to Simulate How Behavior Moderates Salamander Sensitivity to Climate. Front. Ecol. Evol. 7:22. doi: 10.3389/fevo.2019.00022
Received: 14 November 2018; Accepted: 22 January 2019;
Published: 08 February 2019.
Edited by:
Caitlin R. Gabor, Texas State University System, United StatesReviewed by:
Brittany Ousterhout, Lewis and Clark Community College, United StatesVincent R. Farallo, Virginia Tech, United States
Copyright © 2019 McEntire and Maerz. This is an open-access article distributed under the terms of the Creative Commons Attribution License (CC BY). The use, distribution or reproduction in other forums is permitted, provided the original author(s) and the copyright owner(s) are credited and that the original publication in this journal is cited, in accordance with accepted academic practice. No use, distribution or reproduction is permitted which does not comply with these terms.
*Correspondence: Kira D. McEntire, a21jZW50aXJAdHJpbml0eS5lZHU=