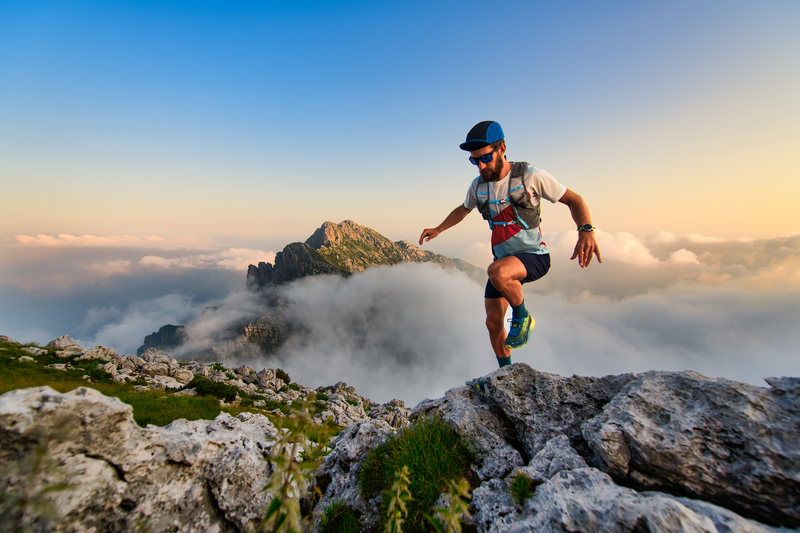
95% of researchers rate our articles as excellent or good
Learn more about the work of our research integrity team to safeguard the quality of each article we publish.
Find out more
ORIGINAL RESEARCH article
Front. Ecol. Evol. , 28 September 2018
Sec. Biogeography and Macroecology
Volume 6 - 2018 | https://doi.org/10.3389/fevo.2018.00151
This article is part of the Research Topic Antarctic Biology: Scale Matters View all 21 articles
Antarctic specimens collected during various research expeditions are preserved in natural history collections around the world potentially offering a cornucopia of morphological and molecular data. Historical samples of marine species are, however, often preserved in formaldehyde which may render them useless for genetic analysis. We sampled stomachs and hindguts from 225 Trematomus specimens from the Natural History Museum London. These samples were initially collected between 20 and 100 years ago and fixed in either formaldehyde or ethanol. A 313 bp fragment of the cytochrome c oxidase subunit I (COI) was amplified and sequenced for prey item identification in the stomach and a 450 bp region of the 16S rRNA gene to investigate microbiome composition in the gut system. Both data sets were characterized by large dropout rates during extensive quality controls. Eventually, no unambiguous results regarding stomach content (COI) were retained, possibly due to degraded DNA, inefficient primers and contamination. In contrast, reliable microbiome composition data (16S rRNA) was obtained from 26 samples. These data showed a correlation in change of microbiome composition with fish size as well as year of the catch, indicating a microbiome shift throughout ontogeny and between samples from different decades. A comparison with contemporary samples indicated that the intestinal microbiome of Trematomus may have drastically changed within the last century. Further extensive studies are needed to confirm these patterns with higher sample numbers. Molecular analyses of museum stored fish can provide novel micro evolutionary insights that may benefit current efforts to prioritize conservation units in the Southern Ocean.
The Antarctic continent and the surrounding Southern Ocean contain fragile and unique ecosystems, molded by cold climate, seasonal photoperiod, and remoteness. Despite its distance to congested areas, human influences on the ecosystems include both direct impacts (such as commercial fishing, tourism, and research) and indirect impacts (such as global warming or ocean acidification). These impacts increased considerably during the last 100 years. For example, Antarctica is among the areas most affected by climate change (Solomon et al., 2009), which is expected to have negative effects on marine Antarctic ecosystems (Griffiths et al., 2017). All over the world, climatic changes have already had severe effects on fish populations (Roessig et al., 2004). These effects are expected to be especially grave in the Southern Ocean, as it is home to a unique fish fauna adapted to the prevailing stenothermal conditions (Eastman, 1993). This fragility is due to thermal limitations of the fish species itself, but also to temperature restraints and preferences of complex associated prey, symbiont, and microbiome communities. The wellbeing of fish stocks is often dependent on high abundance of their main prey items (Roessig et al., 2004). Key prey species may be forced to change their distribution range, driving the fish to either prey on other taxa or migrate and follow the initial prey. Baseline data studying these shifts in associated fauna is often lacking, especially for remote areas such as the Southern Ocean. Therefore, describing the associated fauna of fish species in the present and in the past may be a yet underappreciated way to understand eco-evolutionary processes and follow environmental changes.
Natural history museums worldwide house an immense number of preserved specimens. Many of these samples were collected before or during the period of rapid increase in anthropogenic impact on natural populations, and could therefore yield valuable information regarding recent eco-evolutionary processes (Ceballos and Ehrlich, 2002; Wandeler et al., 2007). While such museum samples have been used extensively for morphological studies in the past (e.g., taxonomy, morphometrics, and meristic counts), they often pose challenges for genetic and genomic analysis due to nucleic acid degeneration (Chakraborty et al., 2006; Bi et al., 2012, 2013). Amongst the first reports of successful DNA extraction from archived samples was the study of Higuchi et al. (1984) targeting the extinct quagga, a member of the horse family. Molecular analysis of museum samples, sometimes also referred to as “ancient DNA” studies, have gained popularity since (e.g., Lambert et al., 2002; Nielsen et al., 2017). Advances of high-throughput sequencing techniques applied to museum samples have changed the possible scale and effort needed for archived DNA investigations and shown how powerful and valuable these can be (Bi et al., 2012, 2013; Nielsen et al., 2017). To date, studies using museum samples have mainly focused on the host (e.g., extracting DNA from bones, otoliths, teeth), whereas organisms that lived on or within the host, as well as ingested prey organisms are mostly ignored. Museum samples can be used to reveal the genetic variation of a host species in space and time. In addition, these samples may be useful to study associated symbionts, parasites, prey items, or microbiome composition, with enormous potential to unveil eco-evolutionary processes over large time scales.
Metabarcoding has the potential to simultaneously assess the presence and to some extent also abundance of hundreds of species, which makes it attractive for diversity assessment studies. Knowledge of prey items or the microbiome composition within species can often highlight spatial differences in diet, behavior, or environmental pressure. Recently, studies assessed the biodiversity of ancient samples by metabarcoding permafrost samples (Jørgensen et al., 2012; Bellemain et al., 2013; Willerslev et al., 2014) and dental calculus (Eisenhofer et al., 2017), however, such studies are few. Using ancient samples gives a unique opportunity to compare community structures through time and space.
The gastrointestinal microbiome, the community of protozoans, bacteria, and viruses inhabiting the digestive system of a fish host, may display fundamental interspecific as well as intraspecific variation (Ghanbari et al., 2015; Egerton et al., 2018). It is affected by the physical properties of a habitat, such as water temperature, salinity or pollution, as well as biological factors, such as preferred prey, interactions with other species, and the ambient microbiome of the water column (Tarnecki et al., 2017; Bagi et al., 2018; Chen et al., 2018). In turn, the gastrointestinal bacterial community can influence the nutrition (Bäckhed et al., 2004; Turnbaugh et al., 2006) and therefore growth and reproduction, general behavior (Cryan and Dinan, 2012), and vulnerability to diseases (Kau et al., 2011) of the host itself. The microbiome composition varies and reflects both host-specific changes as well as environmental changes. Understanding past and present diversity patterns of diet and gastrointestinal microbiome composition can help pinpoint ecological implications of centennial-scale change in the Southern Ocean. Such insights may be useful in the context of current conservation planning, especially when integrated with other information sources and disciplines (e.g., Dawson et al., 2011). With the present proof-of-concept study, we aim to advance the development of metabarcoding techniques applied to museum samples originating from the Southern Ocean. We hope that such innovative methods lead to a better understanding of eco-evolutionary dynamics between host, prey, and microbiome species in times of rapid change.
Notothenioidei include the dominant fishes in the High-Antarctic and feature prominent adaptations such as antifreeze glycoproteins (Chen et al., 1997), reduced ossification (Eastman and Devries, 1981), and loss of heat-shock response (e.g., Hofmann et al., 2000). This makes them valuable model species for evolutionary and developmental biology (Rutschmann et al., 2011; Postlethwait et al., 2016). The genus Trematomus includes 13 species (sensu Duhamel et al., 2014) of medium-sized high-Antarctic shelf fishes that display ecological diversification (Lannoo and Eastman, 2000; Janko et al., 2011; Duhamel et al., 2014). They show differing levels of habitat preference in terms of depth and bottom association (inshore—deep-sea; cryopelagic—benthic), population genetic structure, and feeding habits (Dewitt et al., 1990; Van De Putte et al., 2012; Mcmullin et al., 2017). Morphological stomach content identification has shown that Trematomus species feed mainly on a variety of small crustaceans (amphipods, copepods, euphaussiids, isopods, mysids), but also molluscs, polychaetes, algae, and fish (Vacchi and La Mesa, 1995; La Mesa et al., 1997, 2004, 2015; Moreira et al., 2014; Jurajda et al., 2016). Precise, species level prey identification necessary for high-resolution intra- and interspecific comparisons, however, can be challenging due to degradation and lost taxonomic characters. The diversification of Trematomus is part of the adaptive radiation of the Notothenioidei, believed to coincide with extinction and recolonization cycles in the Antarctic (Near et al., 2012). Ancient climate change has likely played a major role in facilitating the evolution of these fishes (Matschiner et al., 2011; Near et al., 2012). However, contemporary rates of environmental change and additional stressors such as pollution and new colonizing species are unprecedented and may fundamentally alter future evolution or even cause extinction of high-Antarctic fish species (Dornburg et al., 2017).
The aims of this study are threefold: (1) to test the applicability of metabarcoding techniques to determine the prey and microbiome composition of museum samples (2) to compare results from museum samples to those of contemporary samples (2017–2018), and (3) to explore potential driving factors of microbiome variability found in museum samples.
Stomach and hindgut samples of 225 specimens of the genus Trematomus were obtained from the Natural History Museum, London. Fish were carefully dissected to minimize damage to the specimens. Stomachs were opened to remove stomach content and a small portion of the hindgut (1 cm) was removed. Stomach content and hindgut were stored separately in 70% ethanol. Sampling dates ranged from 1901 to 1988. Standard length (SL), year of catch, location of catch, and species identity (based on the morphological identification of the initial identifier) were recorded from the ledgers of the museum for all samples as far as available. Contemporary samples (n = 15) were caught with hook and line in the vicinity of the Gerlache Strait, Antarctic Peninsula in the season of 2017–2018. They were morphologically identified and frozen at −20°C until being processed.
Eight protocols were tested for DNA purification, including two commercial kits specialized for formalin fixed and paraffin embedded (FFPE) tissue (Supplementary Material; Sato et al., 2001; Shi et al., 2002, 2004; Joshi et al., 2013). The method described below is strongly based on Shi et al. (2002) and Shi et al. (2004) and showed the most promising results. During molecular laboratory work special care was taken to prevent (cross-) contamination of samples. A large piece of stomach content (0.5 × 0.5 cm) or the entire piece of hindgut (1 cm) was placed into screwcap microtubes (fitted with rubber seals) with 500 μl of Phosphate Buffered Saline (PBS) at pH 9. Tissue was fragmented thoroughly in each tube to ameliorate efficiency. Samples were heated to 100°C for 10 min, left to cool on ice for 5 min and then spun down with 20,000 × g for 5 min. PBS was carefully removed without taking any tissue and replaced by 500 μl of PBS at pH 7.2 and again heated to 100°C for 10 min. PBS was again carefully removed and further purification steps were conducted using the commercial Nucleospin® Tissue (Macherey-Nagel, Accession number: 740952) DNA extraction kit following the manufacturer's protocol. Since more tissue was used than the manufacturer anticipated, multiple (2×, 3×, or 4×) amounts of the manufacturer's recommended chemicals were used depending on the amount of initial tissue. Furthermore, digestion was extended from 2 to 48 h. Final elution of DNA from the columns was also extended to 1 h. Workbench wipes (workbench contamination), human saliva wipes (human contamination) and no-template extractions (blanks) were included as contamination controls for amplification and sequencing.
For prey identification a 313 bp region of the COI gene was amplified from the stomach content using the tailed primers NGSmlCOIint and NGSjgHCO2198 according to Leray et al. (2013). The V3 and V4 region (460 bp) of the 16S rRNA gene was amplified using the tailed primers 16s-IllumTS-F and (4) 16s-IllumTS-R to assess the microbiome composition (Klindworth et al., 2013). The reaction mix for the amplicon PCR for COI contained 12.5 μl of MytaqTM 2x Mix (Bioline, Accession number: BIO-25041), 0.5 μl of each primer (20 μM), 10.5 μl of molecular grade water and 1 μl of DNA template with a PCR profile of 10 s of denaturation at 95°C, 30 s of annealing at 62°C and 60 s elongation at 72°C for 16 cycles with the annealing temperature dropping every cycle by 1°C, followed by 25 cycles with an annealing temperature at 46°C. The reaction mix for the amplicon PCR for 16S contained 12.5 μl of MytaqTM 2x Mix, 2.5 μl of each primer (1 μM), 2.5 μl of DNA template (5 ng ul−1) and 5 μl of molecular grade water with a PCR profile of 60 s of initial denaturation at 95°C followed by 25 cycles of 15 s denaturation at 95°C, 15 s of annealing at 55°C and 10 s elongation at 72°C, finishing with a final extension of 72°C for 300 s. PCR products were cleaned up using Agencourt AMPure XP beads (Beckman Coulter, Accession number: A63882) following the manufacturer's instructions with a bead to template ratio of 0.8 to 1. Thereafter followed an indexing PCR, which binds a unique primer barcode to each respective sample following Lange et al. (2014) with a PCR mix of 10 μl of MytaqTM 2x Mix, 0.5 μl of each forward and reverse indexing-primer (to form a unique identifiable primer combination for each sample; 20 μM) and 9 μl of DNA template with a PCR profile of an initial denaturation of 1 min at 95°C followed by 15 cycles of denaturation for 15 s at 95°C, 15 s of annealing at 51°C and 10 s of extension at 72°C finishing with a final extension of 5 min at 72°C. The PCR product was cleaned up again, then quantified using the commercial Quant-iTTM Picogreen® kit (Thermo Fisher) and pooled, if sufficient template (20 ng) was available. Sequencing took place on an Illumina MiSeq PE 3000 (Genomics Core, KU Leuven, Belgium).
After the generation of the raw reads samples were demultiplexed using the bcl2fastq v2.16 tool integrated in the Illumina platform. Barcode mismatch was set to 0 to avoid index cross ambiguity errors. 16S rRNA data was analyzed through QIIME v.1.9.1 (Caporaso et al., 2010) to OTU level via NEPHELE (NEPHELE, 2016) for FASTQ paired-end reads: All reads with ambiguous base calls or a Phred score below 20 were removed from the dataset. Forward and reverse reads were joined with a minimum overlap of 30 bp and a maximum of 25% difference in base calls in the overlapping regions. Reads were classified by similarity based on Operational Taxonomic Units (OTUs) using an open reference approach. Chimeras were identified and removed using uchime (Edgar et al., 2011). OTUs were identified by alignment to the SILVA 128 (Quast et al., 2012) reference database based on 99% similarity. The final output for both 16s rRNA and COI data resulted in an OTU table with number of reads per OTU for each sample. Analysis of COI data followed the protocol of Aylagas and Rodríguez-Ezpeleta (2016). The quality of the reads were checked using FASTQC v0.11.5 (Andrews, 2010) and merged using FLASH v1.2.11 (Magoč and Salzberg, 2011) with a minimum and maximum overlap of 217 bp and 257 bp, respectively. Reads with a Phred score below 25 were removed using Trimmomatic v0.36 (Bolger et al., 2014). Reads were classified into OTUs using an open reference approach with mothur v.35.1 (Schloss et al., 2009). Taxonomic assignment of OTUs was conducted using the Barcode of Life Datasystem (BOLD, www.boldsystems.com). Rarefaction curves were created in Nephele to assess the number of identified OTUs over the number of reads per sample and per group. Data was analyzed using Calypso (Zakrzewski et al., 2016). Principal Coordinate Analysis (PCoA) was applied in R Studio with R v.3.3.2 (R Core Team, 2016). Linear models (LM; R base package “stats” v3.5.0) were utilized to assess the relationship between the microbiome composition as reflected in the second PCoA axis and standard length (SL) and the year of catch. Species identity and location were found to be non-significant, possibly due to small sample size and therefore not further considered. Both significant variables (SL, year of catch) are not independent of each other and cannot be disentangled due to limited sample number because of large dropout rates (Figure 1). Therefore, both variables were used separately to create LMs, which were tested for significance (ANOVA) and adjusted for multiple testing using the Bonferroni correction. The diversity was assessed using Calypso and significance was tested using Tukey's (HSD) post-hoc test.
Figure 1. Vertical bar chart showing the remaining sample size for museum gut (16S gene, gray) and museum stomach (COI gene, black) content analysis after every DNA metabarcoding pipeline step.
All data are available online under http://dx.doi.org/10.17632/8cr8yzvsj2.9: containing metadata for all museum fish (including dorsal and lateral photos) as well as demultiplexed raw MiSeq reads from museum and contemporary samples. Metadata for contemporary samples are available under http://dx.doi.org/10.17632/gk94xj8ydg.1.
The complete workflow was characterized by high dropout rates of museum samples at every stage (Figure 1). Initially, 400 specimens of the genus Trematomus were identified in the museum's catalogs. Of these only 225 were suitable for stomach and gut analysis, either due to fish size or to the fact that the intestines had already been removed previously. After extraction and amplification, sufficient DNA (at least 20 ng for sequencing) was available in 84 gut and 67 stomach samples. Only 44 gut and 49 stomach samples were sequenced with at least 1,000 reads per sample. Quality filtering reduced the sample size further to 35 gut and 27 stomach samples. In the final step samples were compared to blank, human contamination, and workbench contamination samples. The microbiome composition (16S rRNA) of most museum samples clustered distinctly apart from the majority of the control samples (Figure 2A). Samples with a negative value on PCoA 1 axis (n = 5) clustered close to the control samples. They were therefore removed from the dataset as being contaminated by either the environment (indicated by proximity to workbench samples), the researcher (proximity to human contamination samples), or a combination of both. One blank control (positive value on the PCoA 1 axis) clustered close to the museum samples. This blank control was most likely contaminated by museum samples. The PCoA of the prey item composition (COI) shows that museum samples and control samples were evenly distributed (Figure 2B). Here, blank as well as workbench contamination samples cluster within the museum samples, indicating great homogeneity between them. All prey items from museum samples were manually assessed, and the taxonomic classification evaluated (data not shown). Samples were dominated by reads linked to environmental (other species used in the same laboratory) and human contamination. Few reads (<3% in all samples) were of species that actually occur in the Southern Ocean.
Figure 2. Principal Coordinate Analysis (PCoA) of (A) microbiome composition in the gut and (B) prey item composition in the stomach of Antarctic Trematomus fish from natural history museum (gray) and control samples (black).
Microbial data (16S rRNA) was reanalyzed for the remaining museum (M) samples together with contemporary (C) Trematomus samples to evaluate a temporal contrast with 23 museum samples, one blank extraction, three human contamination, three workbench contamination controls, and 15 contemporary samples (Table 1). In total 2,331,397 reads were obtained for all samples with an average of 51,809 (± SE 10,266) reads per sample. Contemporary samples produced more than twice as many reads as museum samples with averages of 88,578 (± SE 8,399) and 38,899 (± SE 17,774) reads per sample, respectively. The blank sample had in total 14 reads. For most samples rarefaction curves of the number of observed species over number of sequences per sample leveled out at about 1000 sequences per sample, indicating that the sampling effort was more than sufficient, and a majority of the species present were recorded (Figure 3A). Rarefaction curves for the samples clustered by treatment groups (museum samples, contemporary samples, workbench controls, and human controls) show that the curve for museum samples evened out at only about 4,000 reads, indicating more rare species in the samples compared to the contemporary samples (Figure 3B). Overall, this indicates that sufficient reads per sample and per treatment were obtained in order to sequence a majority of the bacterial species present. For further analysis all OTUs that counted less than two reads were removed from the dataset.
Figure 3. Rarefaction plots of the Alpha diversity of (A) all samples and (B) average number of observed species for each treatment group (Museum samples, contemporary samples, workbench control and human control). Error bars indicate the standard deviation.
Principal component analysis (PCoA) showed that in both the microbiome (16S rRNA) as well as prey item composition (COI) the contemporary and museum samples cluster distinctly away from each other (data not shown), with little overlap between contemporary and recent samples. Contemporary samples did not cluster within the control samples for both datasets.
The microbiome composition of Trematomus museum samples proved to be highly diverse (a full abundance list of all taxa can be found online (http://dx.doi.org/10.17632/8cr8yzvsj2.9) under bacterial_taxa_summary.html). Here, the focus lays on a comparison between species, where museum as well as contemporary samples were available (T. hansoni, T. newnesi, T. loennbergii). Bacterial composition is compared at the phylum and family level (Figure 4). The most abundant phylum in all sample groups was Proteobacteria. However, abundance of both phyla and family varied greatly between museum and contemporary samples of the same species. Trematomus hansoni showed the most similar microbiome between museum and contemporary samples when analyzed at the phylum level. However, family level analyses presented large differences as well. In total, museum and contemporary samples shared only 32, 49, and 33 OTUs for T. hansoni (total number of OTUs M: 131, C: 110), T. newnesi (M: 131, C: 134), and T. loennbergii (M: 80, C: 96), respectively (Figure 5). In T. hansoni the composition of phyla was most similar with Proteobacteria (M:75%, C: 79%), Deionococcus-Thermus (M:14%, C: 2%), Bacteroidetes (M: 2%, C: 9%), and Actinobacteria (M: 0.5%, C: 2%) present in notable (≥1%) abundance in both museum and contemporary samples. Museum and contemporary samples of T. newnesi differed most with only Proteobacteria (M: 79%, C: 22%), Cyanobacteria (M: 1%, C: 60%), Bacteroidetes (M: 2%, C: 1%), and Actinobacteria (M: 1%, C: 1%) occurring in notable abundances. Similarly, T. loennbergii showed overlaps in the phyla Proteobacteria (M: 94%, C: 80%) and Bacteroidetes (M: 1%, C: 5%). A visual comparison at the family level (Figure 4) points to greater overlap between different species within the same time frame (museum vs. contemporary) rather than within species (T. hansoni vs. T. newnesi vs. T. loennbergii). Furthermore, museum samples showed much more similarity to each other when compared to contemporary samples, as is reflected in the number of OTUs shared between all species in museum (65 OTUs) and contemporary (33 OTUs) samples (Figure 5).
Figure 4. Taxonomic information of the microbiome of Trematomus hansoni, T. newnesi and T. loennbergii. The abundance of each microbial taxonomic group is represented in bar plots on phylum (P) as well as family (F) level for museum and contemporary samples of each fish species. Phyla are separated by black lines drawn through both P and F barplots. The taxa listed in the family level plots are positioned as in the phylum level plots (i.e., they correspond to the same phyla). Within the bar plots the phylum of the family plots correspond to the same position as of the one from the phylum plots. Diversity plots for species evenness, Shannon index and species richness of museum and contemporary samples are presented for each species below the taxonomic plots.
Figure 5. Six-way Venn diagram of the microbial OTU composition in the guts of members of the genus Trematomus (T. hansoni, T. newnesi, T. loennbergii) from museum and contemporary samples.
An overall comparison between museum and contemporary samples shows that there was no significant difference between average Shannon indices, although museum samples exhibited much less variability than contemporary samples [TukeyHSD: F(1, 21) = 1.988, p > 0.05, Figure 6A]. Species richness, on the other hand, was higher and exhibited less variation in museum samples [TukeyHSD: F(1, 21) = 16.85, p < 0.001, Figure 6B]. There were no statistically significant differences between evenness of museum and recent samples [TukeyHSD: F(1, 21) = 1.5518, p > 0.05, Figure 6C].
Figure 6. Diversity graphs for all museum and contemporary samples: (A) Shannon index, (B) species richness and (C) evenness. Error bars indicate standard deviation.
There were no statistically significant differences [TukeyHSD: F(3, 19) = 6.798, p > 0.05] between average species richness between museum and recent samples of T. hansoni (M: 134.4 ± 13; C: 85 ± 38.4), T. newnesi (M: 112, 5 ± 4.4; C: 50.8 ± 10.4), or T. loennbergii (M: 98.4 ± 14.3; C: 105.2 ± 8.3). However, there was a significant difference between average species richness between museum T. hansoni and contemporary T. newnesi samples (p = 0.0054). The average Shannon index [TukeyHSD: F(3, 19) = 1.039, p > 0.05] and evenness [TukeyHSD: F(3, 19) = 0.832, p > 0.05] were similar with no significant differences (p > 0.05).
Non-contaminated samples of the microbiome analysis differ mainly along the second PCoA axis (Figure 2A). PCoA values were extracted and tested for correlations with metadata. There was no correlation of PCoA 2 values of the microbiome composition with neither species identity nor location. However, there was a correlation between the PCoA 2 values and the standard length of the fish [Bonferroni corrected p = 0.0053, F(1, 21) = 11.6, R2 = 0.3556, Figure 7A] as well as a correlation between the PCoA 2 values of the microbiome composition and year of catch [Bonferroni corrected p = 0.0345, F(1, 21) = 6.685, R2 = 0.2415, Figure 7B]. The linear models explain 35.5 and 24.2% of the variability of the data, respectively. In contemporary fish the microbiome composition differed mainly along the first PCoA 1 axis and these values were therefore used for further analysis. However, the PCoA 1 values did not correlate to any of the potential explanatory variables (Figure 7A). Noteworthy is also the distribution of the year of catch of samples from which meaningful results were obtained (Figure 7B). A majority of these samples were caught between 1901 and 1913 (n = 14), with another small cluster (n = 4) from 1938/39, another cluster (n = 4) from 1988, and one single sample from 2006.
Figure 7. Scatter plots of PCoA values for contemporary and museum samples of the microbiome composition plotted against (A) the standard length (SL) in cm of the fish. Triangles (Δ) represent contemporary samples and are plotted on the PCoA 1 axis, whereas dots (∙) represent museum samples and are plotted on the PCoA 2 axis. Linear models (LM) were fitted to both data with significance levels of p = 0.0053 and p = 0.0685, respectively. (B) PCoA 2 values of the microbiome composition of museum samples plotted against the year of catch. A LM was fitted to the data with a significance level of p = 0.0345.
In this study, we targeted the stomach content and internal microbiome of fish that have been stored in museum collections for a prolonged amount of time. Extraction of DNA, amplification of a gene fragment (COI/16S rRNA), and successful sequencing has proven extremely difficult and characterized by high dropout rates. We established an intensive control system in order to ensure that results do not merely reflect contamination of ambient bacterial communities, which has been problematic in many metabarcoding studies (Ficetola et al., 2016). The COI data, targeting prey items in the stomach, showed particularly high contamination rates and even the removal of all OTUs from the dataset that were present in contamination controls yielded no usable results. We therefore conclude that this method as presented and applied here is not suitable to amplify COI fragments from the stomach of museum stored fish specimens.
In contrast, reliable results of the microbiome composition (16S rRNA) for 23 fish was obtained. Even with high dropout rates, this is a promising finding, because it opens up enormous possibilities for future studies to assess the intestinal microbiome of fish from museum collections. The 16S rRNA data of museum samples, targeting the bacterial community within the hindgut, was distinctly different from control samples, indicating no contamination issues. One confounding explanation might be that the bacterial communities found here were remnants of bacterial communities from the ethanol used to preserve the fish in the museum. However, if that was the case, we would expect greater homogeneity in the contamination of the samples, i.e., bacterial communities would be more homogenous between samples indicated by nearly all OTUs shared and also more samples would likely have been contaminated. In addition, fish were not previously opened (access to gut) before this study, which should limit contamination within the intestine. Interestingly, the few samples that passed all pipeline and quality control steps, were all collected at few time points (year of catch). This possibly reflects a strong influence of the initial (and long-term) preservation method. Formalin (formaldehyde) gained increasing popularity for specimens after the first quarter of the twentieth century in all fields of biological sciences (Herbin, 2013). Formalin replaced the more expensive ethanol as standard preservative, also providing better preservation and higher efficiency, especially for larger specimens. However, even if buffered properly, formalin causes crosslinking among DNA molecules, between DNA molecules and nucleoproteins, and between nucleoproteins alone (Koshiba et al., 1993). This complicates/hampers DNA extraction and marker amplification. If not buffered correctly, formic acid forms with time in the formalin preservative. Depending on the pH, the effects of this process can range from structural modifications, over denaturation to complete depolymerization of the DNA (Thomas and Doty, 1956; Geiduschek, 1958). Furthermore, if conditions remain acidic, DNA hydrolyzes resulting in further structural changes (Koshiba et al., 1993). In museum collections, the initial preservative (formalin/ethanol fixation) is generally not documented. Formalin, as a preservative, was first introduced in 1891 and gained attention between the years of 1896 to 1937 (Herbin, 2013). Between 1960 and 1980 it was used almost exclusively. Due to the health risks of formalin, starting around 2000 samples were over time gradually transferred to ethanol, without keeping a record of the initial preservative. Most likely the successful metabarcoding sequences of this study are from specimens that have never been stored in formalin. That might explain why more ancient samples (anterior to 1913) have better amplification success and come in batches (same time and same expedition, so preserved in a similar way). Unfortunately, preservation data is not available, so this hypothesis cannot be tested here. We recommend that future museum metabarcoding studies carefully evaluate from which time periods samples are available and whether there is any information on preservation techniques. It would be interesting to specifically test sequencing success in relation to formalin/ethanol use, although many confounding factors (e.g., time between capture and transfer to preservative, organism size, tissue permeability) may be present. In cases with no information available, researchers have to critically weigh their options as many samples may fail as shown here, which can drastically increase the cost of such a project. Unfortunately, most surveys and collections from the Southern Ocean were collected in the second half of the twentieth century, after formalin became the preservative of choice for fish, but before the potential of DNA was taken into account. The use of these samples for molecular studies is therefore probably limited.
It is also unclear why we were able to obtain data for the microbiome composition (16S rRNA; both historical and contemporary) and unable for the prey item composition of the historical samples (COI; data for the prey item composition of contemporary samples were generated but not shown, available under http://dx.doi.org/10.17632/gk94xj8ydg.1). It might be that bacterial communities based on their cellular structure and properties are more resistant and cope better with long term storage in the environment of the stomach and intestines, which can include aggressive digestive acids. Prey items may not withstand such an environment that well with the consequence of genetic material degrading in the course of the many years of storage. As there is no evidence for this suggestion in the literature, it remains speculation at this point in time. Another possible hypothesis would be that 16S rRNA primers used in this study are generally more efficient than the ones used for the COI fragment. In combination with low quality template DNA, this might result in a successful amplification for the 16S rRNA fragment, but not the COI fragment. Further technical tests or replication studies are needed to clarify the cause of the problem here and/or to find alternative ways to successfully obtain COI data from historical stomach contents.
DNA metabarcoding studies in general can suffer from poor taxonomic resolution due to primer bias (Deagle et al., 2014). Regarding museum samples short target fragments are often the only option due to fragmented and/or degraded DNA. If well-preserved samples yielding high-quality DNA is available, metagenomics (i.e., using genomewide markers or whole-genome data) offer a taxonomically more representative alternative (Porter and Hajibabaei, 2018). Natural history museums may contain samples amenable to such approaches as well (e.g., long-term ethanol preserved specimens, that were not exposed to formalin). Despite these limitations and trade-offs, museum metabarcoding may offer unique insights into the temporal dynamics and driving factors of fish microbiome variation. Such data can be especially valuable to understand the responses to decadal to centennial scale environmental changes. In the following we explore temporal and biological signals with our—-admittedly small—-data set after quality control to showcase potential trends in Antarctic fish microbiome composition.
So far only one study has investigated the microbial gut fauna of Antarctic fishes (Notothenia coriiceps and Chaenocephalus aceratus), using Sanger sequencing (Ward et al., 2009). We found much greater microbial diversity in both museum and contemporary samples of Trematomus spp., which can be expected given that our data are based on high-throughput sequencing. In general, the number of OTUs found in the intestinal microbiome is very variable in marine fish (Sullam et al., 2012). Two of the OTUs found in Ward et al. (2009) were also present in our contemporary samples (AF206298-Ehrlichia sp. “trout isolate,” FM178379.1-Aliivibrio salmonicida LFI1238). In nine cases the same genus (Fusobacterium, Photobacterium, Aliivibrio, Desulfovibrio, Mycoplasma, Desulfovibrio, Shewanella, Moritella, Sphingomonas) was present in our contemporary samples. The museum sample microbiomes from our study included three genera (Fusobacterium, Shewanella, Sphingomonas), that were found by Ward et al. (2009) as well. Differences between the two studies are probably largely driven by the different sequencing techniques and possibly to a lesser extent by the different target species. Interestingly, there is less overlap between our museum samples and the results of Ward et al. (2009) than between our contemporary samples and Ward et al. (2009)
We also directly compared microbiome composition between contemporary and museum samples of three Trematomus species and found little overlap. Temporal comparisons of the same species show more variation than between species comparisons from the same sample type. Such results, albeit based on small sample size, could indicate that the microbiome of all fishes has undergone a drastic community shift. While there is large overlap in the functional groups (phylum level) in terms of presence and absence, the actual proportions of each group vary greatly between museum and contemporary samples. At higher resolution of the microbial community (family level), there is a clear change in composition, with few OTUs present in both museum and contemporary samples. This supports the idea that the microbiome may have fundamentally changed in these three species within the last century. We need to stress, however, that due to the large amounts of dropout during quality control our findings are based on very small sample sizes. The observed differences could therefore be driven by an insufficient community representation (especially in T. hansoni and T. loennbergii). Further studies ideally selecting individuals from similar locations and with known preservation history are needed to confirm these patterns. It is also unclear how such a drastic change could have occurred. The microbiome composition can rapidly and drastically be affected by change in behavior (David et al., 2014a), changes in diet (David et al., 2014b) or due to environmental influences such as pollution (Bagi et al., 2018; Chen et al., 2018). Ancient intestinal microbiome studies in humans show great resemblance of coprolite samples (between 8,000 and 1,400 years B.P.) with that of contemporary traditional rural communities, but drastic differences with samples associated with a cosmopolitan lifestyle (Tito et al., 2012). This shows that strong community shifts in the intestinal microbiome do occur across populations, but are generally thought to be associated with environmental pressures or changes in lifestyle (Schnorr et al., 2016). In our case, it is unclear what could have caused the indicated shift. One suggestion might be that humans have an increasing impact on the Antarctic environment. Despite the distance to congested areas, impacts on the Antarctic ecosystem have increased dramatically in the last 100 years. They include direct impacts such as pollution, tourism, and research (Clarke and Harris (2003), as well as indirect impacts such as the emission of greenhouse gases (Trathan and Agnew, 2010). While the consequences of direct impacts are relatively minor compared to other areas in the world (Halpern et al., 2008; Trathan and Agnew, 2010), indirect impacts have already and are expected to greatly affect and permanently alter the ecosystems of the Southern Ocean and Antarctica in the near future (Clarke and Harris, 2003; Schofield et al., 2010; Mintenbeck et al., 2012; Griffiths et al., 2017). Changing microbiome composition may be among these alterations. However, only a larger dataset with more environmental information and samples from multiple museum collections will be able to validate this hypothesis. Our results for contemporary samples also represent an important baseline that will be useful for the study of future changes in the microbiome composition of fish.
A correlation between the microbiome composition and the size of the fish, and therefore also its age (White, 1991), was found for museum samples and indicated as well in contemporary samples. The contemporary samples were used as a control in this study with low sample size. The hinted trend here remains to be validated. There are many cases where the intestinal microbiome evolves throughout the development of an individual, as it changes its lifestyle or diet. There is a clear change in the microbiome composition of children compared to adults (Kau et al., 2011). In fish, body size (and therefore age) plays a crucial role in the interaction between predators and prey (Lundvall et al., 1999). With increasing size bigger prey items can be ingested and smaller ones might become less important. This shift might be reflected in the changing composition of the intestinal microbiome composition with size. Notothenioid fish have diversified ecologically and therefore feature a variety of life styles and feeding habits. Members of the genus Trematomus also show diversification in habitat use and diet (e.g., Brenner et al., 2001). At the same time they attain a similar range of maximum sizes and undergo ontogenetic shifts in in life style (Dewitt et al., 1990). It seems therefore plausible that microbiome composition of Trematomus fishes is dependent on fish size. This hypothesis would be supported by studies concerning the development of the intestinal microbiome of Coho salmon (Oncorhynchus kisutch) where the authors conclude that an early life microbiome is unstable (Romero and Navarrete, 2006). Initial colonization of the gut occurs after first feeding by microbes derived from the water column and the prey items. Over time, initial microbes are outcompeted by strains that are adapted to the intestinal environment. This is in line with other studies of humans (e.g., Cho and Blaser, 2012) and mice (El Aidy et al., 2012, 2013), that show that the initial intestinal microbiome is highly unstable and subject to change during development. In contrast, some studies support a more vertical transmission (from parent to progeny) of bacteria and highlight its importance (Funkhouser and Bordenstein, 2013). It seems that in Trematomus size, development, and age play a predominant role in the composition of the intestinal microbiome. Species and location appear to be of lesser importance. Larger sample sizes, a better understanding of the life history and more information of feeding habits are needed in order to better comprehend this relationship.
Here we show how to obtain information about the intestinal microbiome of century old fish from museum collections through metabarcoding. The feasibility of this provides an excellent opportunity to go back in time and learn about centennial scale microbiome community shifts. Our results indicate that a drastic shift may have occurred in recent years, coinciding with increasing environmental pressure from global change. Furthermore, microbiome composition of Trematomus fishes seems linked to ontogeny, rather than species identity or locality. Due to many samples failing at quality control steps, these findings are based on very small sample sizes and more extensive studies are needed to confirm such patterns. Future studies could try to select specimens with known preservation history and avoid formalin fixated samples, as these might be responsible for the high dropout rates we experienced. Overall, metabarcoding studies of museum fish harbor great potential for understanding eco-evolutionary processes that lead to adaptation within relatively short time scales.
All generated data (raw reads) and metadata for this study can be found in the Mendeley Data repository (http://dx.doi.org/10.17632/8cr8yzvsj2.9) under the title “Historical DNA metabarcoding of the prey and microbiome of trematomid fishes using museum samples.” Metadata and COI data for contemporary fish can be found in the Mendeley Data repository (http://dx.doi.org/10.17632/gk94xj8ydg.1) under the title “Trophic assessment of Antarctic fish.”
FH conceived the study with input from HC, BF, AD, GL, GM, AV, and FV. FH collected data, performed molecular laboratory work, and analyzed the data. FH and HC led the writing of the manuscript.
This research received support from the SYNTHESYS Project (http://www.synthesys.info/), which is financed by European Community Research Infrastructure Action under the FP7 Capacities Program. It furthermore received funds through the Brilliant Marine Research Idea Philanthropy Award 2017 issued by the Vlaams Instituut voor de Zee (VLIZ), Belgium. Research was funded by the Refugia and Ecosystem Tolerance in the Southern Ocean project (RECTO; BR/154/A1/RECTO) as well as the Ecosystem Responses to global change—a multiscale approach in the Southern Ocean project (vERSO; BR/132/A1/vERSO) (http://rectoversoprojects.be), both funded by the Belgian Science Policy Office (BELSPO). This is contribution number 003 of the RECTO project and contribution number 029 of the vERSO project. HC was supported by a grant from the former Flemish agency for Innovation by Science and Technology (IWT), now managed through Flanders Innovation & Entrepreneurship (VLAIO, Grant No. 141328).
The authors declare that the research was conducted in the absence of any commercial or financial relationships that could be construed as a potential conflict of interest.
We thank the Natural History Museum, London, for museum samples and especially J. Maclaine for assistance during sampling. Furthermore, we thank B. Wallis and the crew of Ocean Expeditions as well as Thomas Desvignes for the acquisition of contemporary samples. We also thank Bart Hellemans for assistance during molecular laboratory work. Furthermore, we thank the reviewers for their constructive and detailed comments and suggestions.
The Supplementary Material for this article can be found online at: https://www.frontiersin.org/articles/10.3389/fevo.2018.00151/full#supplementary-material
Andrews, S. (2010). FastQC: A Quality Control Tool for High Thoughout Sequence Data [Online]. Available online at: http://www.bioinformatics.babraham.ac.uk/projects/fastqc (Accessed 10.6.2017).
Aylagas, E., and Rodríguez-Ezpeleta, N. (2016). “Analysis of illumina MiSeq metabarcoding data: application to benthic indices for environmental monitoring,” in Marine Genomics: Methods and Protocols, ed S.J. Bourlat. (New York, NY: Springer New York), 237–249.
Bäckhed, F., Ding, H., Wang, T., Hooper, L. V., Koh, G. Y., Nagy, A., et al. (2004). The gut microbiota as an environmental factor that regulates fat storage. Proc. Natl. Acad. Sci. U. S. A. 101, 15718–15723. doi: 10.1073/pnas.0407076101
Bagi, A., Riiser, E. S., Molland, H. S., Star, B., Haverkamp, T. H., Sydnes, M. O., et al. (2018). Gastrointestinal microbial community changes in Atlantic cod (Gadus morhua) exposed to crude oil. BMC Microbiol. 18:25. doi: 10.1186/s12866-018-1171-2
Bellemain, E., Davey, M. L., Kauserud, H., Epp, L. S., Boessenkool, S., Coissac, E., et al. (2013). Fungal palaeodiversity revealed using high-throughput metabarcoding of ancient DNA from arctic permafrost. Environ. Microbiol. 15, 1176–1189. doi: 10.1111/1462-2920.12020
Bi, K., Linderoth, T., Vanderpool, D., Good, J. M., Nielsen, R., and Moritz, C. (2013). Unlocking the vault: next-generation museum population genomics. Mol. Ecol. 22, 6018–6032. doi: 10.1111/mec.12516
Bi, K., Vanderpool, D., Singhal, S., Linderoth, T., Moritz, C., and Good, J. M. (2012). Transcriptome-based exon capture enables highly cost-effective comparative genomic data collection at moderate evolutionary scales. BMC Genomics 13:403. doi: 10.1186/1471-2164-13-403
Bolger, A. M., Lohse, M., and Usadel, B. (2014). Trimmomatic: a flexible trimmer for Illumina sequence data. Bioinformatics 2014, 2114–2120. doi: 10.1093/bioinformatics/btu170
Brenner, M., Buck, B. H., Cordes, S., Dietrich, L., Jacob, U., Mintenbeck, K., et al. (2001). The role of iceberg scours in niche separation within the antarctic fish genus trematomus. Polar Biol. 24, 502–507. doi: 10.1007/s003000100246
Caporaso, J. G., Kuczynski, J., Stombaugh, J., Bittinger, K., Bushman, F. D., Costello, E. K., et al. (2010). QIIME allows analysis of high-throughput community sequencing data. Nat. Methods 7, 335–336. doi: 10.1038/nmeth.f.303
Ceballos, G., and Ehrlich, P. R. (2002). Mammal population losses and the extinction crisis. Science 296, 904–907. doi: 10.1126/science.1069349
Chakraborty, A., Sakai, M., and Iwatsuki, Y. (2006). Museum fish specimens and molecular taxonomy: a comparative study on DNA extraction protocols and preservation techniques. J. Appl. Ichthyol. 22, 160–166. doi: 10.1111/j.1439-0426.2006.00718.x
Chen, L., Devries, A. L., and Cheng, C.-H. C. (1997). Evolution of antifreeze glycoprotein gene from a trypsinogen gene in Antarctic notothenioid fish. Proc. Natl. Acad. Sci. 94, 3811–3816. doi: 10.1073/pnas.94.8.3811
Chen, L., Hu, C., Lai, N. L.-S., Zhang, W., Hua, J., Lam, P. K., et al. (2018). Acute exposure to PBDEs at an environmentally realistic concentration causes abrupt changes in the gut microbiota and host health of zebrafish. Environ. Poll. 240, 17–26. doi: 10.1016/j.envpol.2018.04.062
Cho, I., and Blaser, M. J. (2012). The human microbiome: at the interface of health and disease. Nat. Rev. Genet. 13:260. doi: 10.1038/nrg3182
Clarke, A., and Harris, C. M. (2003). Polar marine ecosystems: major threats and future change. Environ. Conserv. 30, 1–25. doi: 10.1017/S0376892903000018
Cryan, J. F., and Dinan, T. G. (2012). Mind-altering microorganisms: the impact of the gut microbiota on brain and behaviour. Nat. Rev. Neurosci. 13, 701–712. doi: 10.1038/nrn3346
David, L. A., Materna, A. C., Friedman, J., Campos-Baptista, M. I., Blackburn, M. C., Perrotta, A., et al. (2014a). Host lifestyle affects human microbiota on daily timescales. Genome Biol. 15:R89. doi: 10.1186/gb-2014-15-7-r89
David, L. A., Maurice, C. F., Carmody, R. N., Gootenberg, D. B., Button, J. E., Wolfe, B. E., et al. (2014b). Diet rapidly and reproducibly alters the human gut microbiome. Nature 505:559. doi: 10.1038/nature12820
Dawson, T. P., Jackson, S. T., House, J. I., Prentice, I. C., and Mace, G. M. (2011). Beyond predictions: biodiversity conservation in a changing climate. science 332, 53–58. doi: 10.1126/science.1200303
Deagle, B. E., Jarman, S. N., Coissac, E., Pompanon, F., and Taberlet, P. (2014). DNA metabarcoding and the cytochrome c oxidase subunit I marker: not a perfect match. Biol. Lett. 10:20140562. doi: 10.1098/rsbl.2014.0562
Dewitt, H. H., Heemstra, P. C., and Gon, O. (1990). Nototheniidae: In Fishes of the Southern Ocean, eds O. Gon and P. C. Heemstra. Grahamstown: J.L.B. Smith Institute of Ichthyology.
Dornburg, A., Federman, S., Lamb, A. D., Jones, C. D., and Near, T. J. (2017). Cradles and museums of Antarctic teleost biodiversity. Nat. Ecol. Evol. 1:1379. doi: 10.1038/s41559-017-0239-y
Duhamel, G., Hulley, P.-A., Causse, R., Koubbi, P., Vacchi, M., Pruvost, P., et al. (2014). Biogeographic Patterns of Fish: in Biographic Atlas of the Southern Ocean. Cambridge: Scientific Committee on Antarctic Research.
Eastman, J. T. (1993). Antarctic Fish Biology: Evolution in a Unique Environment. San Diego, CA: Academic Press.
Eastman, J. T., and Devries, A. L. (1981). Buoyancy adaptations in a swim-bladderless Antarctic fish. J. Morphol. 167, 91–102. doi: 10.1002/jmor.1051670108
Edgar, R. C., Haas, B. J., Clemente, J. C., Quince, C., and Knight, R. (2011). UCHIME improves sensitivity and speed of chimera detection. Bioinformatics 27, 2194–2200. doi: 10.1093/bioinformatics/btr381
Egerton, S., Culloty, S., Whooley, J., Stanton, C., and Ross, R. P. (2018). The gut microbiota of marine fish. Front. Microbiol. 9:00873. doi: 10.3389/fmicb.2018.00873
Eisenhofer, R., Anderson, A., Dobney, K., Cooper, A., and Weyrich, L. S. (2017). Ancient microbial DNA in dental calculus: a new method for studying rapid human migration events. J. Island Coast. Archaeol. 2017, 1–14. doi: 10.1080/15564894.2017.1382620
El Aidy, S., Van Baarlen, P., Derrien, M., Lindenbergh-Kortleve, D. J., Hooiveld, G., Levenez, F., et al. (2012). Temporal and spatial interplay of microbiota and intestinal mucosa drive establishment of immune homeostasis in conventionalized mice. Mucosal Immunol. 5:567. doi: 10.1038/mi.2012.32
El Aidy, S., Van Den Abbeele, P., Van De Wiele, T., Louis, P., and Kleerebezem, M. (2013). Intestinal colonization: how key microbial players become established in this dynamic process. Bioessays 35, 913–923. doi: 10.1002/bies.201300073
Ficetola, G. F., Taberlet, P., and Coissac, E. (2016). How to limit false positives in environmental DNA and metabarcoding? Mol. Ecol. Resour. 16, 604–607. doi: 10.1111/1755-0998.12508
Funkhouser, L. J., and Bordenstein, S. R. (2013). Mom knows best: the universality of maternal microbial transmission. PLoS Biol. 11:e1001631. doi: 10.1371/journal.pbio.1001631
Geiduschek, E. P. (1958). On the reversibility of the acid denaturation of sodium desoxyribose nucleate. J. Polymer Sci. Part A 31, 67–75.
Ghanbari, M., Kneifel, W., and Domig, K. J. (2015). A new view of the fish gut microbiome: advances from next-generation sequencing. Aquaculture 448, 464–475. doi: 10.1016/j.aquaculture.2015.06.033
Griffiths, H. J., Meijers, A. J., and Bracegirdle, T. J. (2017). More losers than winners in a century of future Southern Ocean seafloor warming. Nat. Clim. Chang. 7, 749. doi: 10.1038/nclimate3377
Halpern, B. S., Walbridge, S., Selkoe, K. A., Kappel, C. V., Micheli, F., D'agrosa, C., et al. (2008). A global map of human impact on marine ecosystems. Science 319, 948–952. doi: 10.1126/science.1149345
Herbin, M. (2013). La conservation des collections en fluide. CeROArt 259. Available Online at: http://journals.openedition.org/ceroart/3432
Higuchi, R., Bowman, B., Freiberger, M., Ryder, O. A., and Wilson, A. C. (1984). DNA sequences from the quagga, an extinct member of the horse family. Nature 312, 282–284. doi: 10.1038/312282a0
Hofmann, G. E., Buckley, B. A., Airaksinen, S., Keen, J. E., and Somero, G. N. (2000). Heat-shock protein expression is absent in the antarctic fish Trematomus bernacchii (family Nototheniidae). J. Exp. Biol. 203, 2331–2339.
Janko, K., Marshall, C., Musilov,á, Z., Van Houdt, J., Couloux, A., Cruaud, C., et al. (2011). Multilocus analyses of an Antarctic fish species flock (Teleostei, Notothenioidei, Trematominae): phylogenetic approach and test of the early-radiation event. Mol. Phylogenet. Evol. 60, 305–316. doi: 10.1016/j.ympev.2011.03.008
Jørgensen, T., Kjær, K. H., Haile, J., Rasmussen, M., Boessenkool, S., Andersen, K., et al. (2012). Islands in the ice: detecting past vegetation on Greenlandic nunataks using historical records and sedimentary ancient DNA Meta-barcoding. Mol. Ecol. 21, 1980–1988. doi: 10.1111/j.1365-294X.2011.05278.x
Joshi, B. D., Mishra, S., Singh, S. K., and Goyal, S. (2013). An effective method for extraction and polymerase chain reaction (PCR) amplification of DNA from formalin preserved tissue samples of snow leopard. Afr. J. Biotechnol. 12, 3399–3404. doi: 10.5897/AJB12.2759
Jurajda, P., Roche, K., Sedláček, I., and Všetičkov,á, L. (2016). Assemblage characteristics and diet of fish in the shallow coastal waters of James Ross Island, Antarctica. Polar Biol. 39, 1–11. doi: 10.1007/s00300-016-1896-z
Kau, A. L., Ahern, P. P., Griffin, N. W., Goodman, A. L., and Gordon, J. I. (2011). Human nutrition, the gut microbiome and the immune system. Nature 474, 327–336. doi: 10.1038/nature10213
Klindworth, A., Pruesse, E., Schweer, T., Peplies, J., Quast, C., Horn, M., et al. (2013). Evaluation of general 16S ribosomal RNA gene PCR primers for classical and next-generation sequencing-based diversity studies. Nucleic Acids Res. 41:e1. doi: 10.1093/nar/gks808
Koshiba, M., Ogawa, K., Hamazaki, S., Sugiyama, T., Ogawa, O., and Kitajima, T. (1993). The effect of formalin fixation on DNA and the extraction of high-molecular-weight DNA from fixed and embedded tissues. Pathol. Res. Pract. 189, 66–72. doi: 10.1016/S0344-0338(11)80118-4
La Mesa, M., Catalano, B., and Jones, C. D. (2015). Early life history traits of Trematomus scotti in the Bransfield Strait. Antarctic Sci. 27, 535–542. doi: 10.1017/S0954102015000280
La Mesa, M., Dal,ú, M., and Vacchi, M. (2004). Trophic ecology of the emerald notothen Trematomus bernacchii (pisces, nototheniidae) from Terra Nova Bay, Ross Sea, Antarctica. Polar Biol. 27, 721–728. doi: 10.1007/s00300-004-0645-x
La Mesa, M., Vacchi, M., Castelli, A., and Diviacco, G. (1997). Feeding ecology of two nototheniid fishes, Trematomus hansoni and Trematomus loennbergii, from Terra Nova Bay, Ross Sea. Polar Biol. 17, 62–68. doi: 10.1007/s003000050105
Lambert, D., Ritchie, P., Millar, C., Holland, B., Drummond, A., and Baroni, C. (2002). Rates of evolution in ancient DNA from Adélie penguins. Science 295, 2270–2273. doi: 10.1126/science.1068105
Lange, V., Böhme, I., Hofmann, J., Lang, K., Sauter, J., Schöne, B., et al. (2014). Cost-efficient high-throughput HLA typing by MiSeq amplicon sequencing. BMC Genom. 15:63. doi: 10.1186/1471-2164-15-63
Lannoo, M. J., and Eastman, J. T. (2000). Nervous and sensory system correlates of an epibenthic evolutionary radiation in Antarctic notothenioid fishes, genus Trematomus (Perciformes; Nototheniidae). J. Morphol. 245, 67–79. doi: 10.0.3.234/1097-4687(200007)245:1%3C67::AID-JMOR5%3E3.0.CO;2-W
Leray, M., Yang, J. Y., Meyer, C. P., Mills, S. C., Agudelo, N., Ranwez, V., et al. (2013). A new versatile primer set targeting a short fragment of the mitochondrial COI region for metabarcoding metazoan diversity: application for characterizing coral reef fish gut contents. Front. Zool. 10:34. doi: 10.1186/1742-9994-10-34
Lundvall, D., Svanbäck, R., Persson, L., and Byström, P. (1999). Size-dependent predation in piscivores: interactions between predator foraging and prey avoidance abilities. Canad. J. Fish. Aquat. Sci. 56, 1285–1292. doi: 10.1139/f99-058
Magoč, T., and Salzberg, S. L. (2011). FLASH: fast length adjustment of short reads to improve genome assemblies. Bioinformatics 27, 2957–2963. doi: 10.1093/bioinformatics/btr507
Matschiner, M., Hanel, R., and Salzburger, W. (2011). On the origin and trigger of the notothenioid adaptive radiation. PLoS ONE 6:e18911. doi: 10.1371/journal.pone.0018911
Mcmullin, R. M., Wing, S. R., Wing, L. C., and Shatova, O. A. (2017). Trophic position of Antarctic ice fishes reflects food web structure along a gradient in sea ice persistence. Mar. Ecol. Prog. Ser. 564, 87–98. doi: 10.3354/meps12031
Mintenbeck, K., Barrera-Oro, E. R., Brey, T., Jacob, U., Knust, R., Mark, F. C., et al. (2012). Impact of climate change on fishes in complex antarctic ecosystems. Adv. Ecol. Res. 46, 351–426. doi: 10.1016/B978-0-12-396992-7.00006-X
Moreira, E., Juáres, M., and Barrera-Oro, E. (2014). Dietary overlap among early juvenile stages in an Antarctic notothenioid fish assemblage at Potter Cove, South Shetland Islands. Polar Biol. 37, 1507–1515. doi: 10.1007/s00300-014-1545-3
Near, T. J., Dornburg, A., Kuhn, K. L., Eastman, J. T., Pennington, J. N., Patarnello, T., et al. (2012). Ancient climate change, antifreeze, and the evolutionary diversification of Antarctic fishes. Proc. Natl. Acad. Sci. 109, 3434–3439. doi: 10.1073/pnas.1115169109
NEPHELE (2016). Office of Cyber Infrastructure and Computational Biology (OCICB), National Institute of Allergy and Infectious Diseases (NIAID). Nephele. [Online]. Available online at: http://nephele.niaid.nih.gov (Accessed 01-04 2018).
Nielsen, E. E., Morgan, J., Maher, S., Edson, J., Gauthier, M., Pepperell, J., et al. (2017). Extracting DNA from ‘jaws': high yield and quality from archived tiger shark (Galeocerdo cuvier) skeletal material. Mol. Ecol. Resour. 17, 431–442. doi: 10.1111/1755-0998.12580
Porter, T. M., and Hajibabaei, M. (2018). Scaling up: a guide to high-throughput genomic approaches for biodiversity analysis. Mol. Ecol. 27, 313–338. doi: 10.1111/mec.14478
Postlethwait, J. H., Yan, Y. L., Desvignes, T., Allard, C., Titus, T., Le François, N. R., et al. (2016). Embryogenesis and early skeletogenesis in the antarctic bullhead notothen, Notothenia coriiceps. Dev. Dyn. 245, 1066–1080. doi: 10.1002/dvdy.24437
Quast, C., Pruesse, E., Yilmaz, P., Gerken, J., Schweer, T., Yarza, P., et al. (2012). The SILVA ribosomal RNA gene database project: improved data processing and web-based tools. Nucleic Acids Res. 41, D590–D596. doi: 10.1093/nar/gks,1219
R Core Team (2016). R: A Language and Environment for Statistical Computing. R Foundation for Statistical Computing, Vienna. Available online at: https://www.R-project.org/
Roessig, J. M., Woodley, C. M., Cech, J. J., and Hansen, L. J. (2004). Effects of global climate change on marine and estuarine fishes and fisheries. Rev. Fish Biol. Fish 14, 251–275. doi: 10.1007/s11160-004-6749-0
Romero, J., and Navarrete, P. (2006). 16S rDNA-based analysis of dominant bacterial populations associated with early life stages of coho salmon (Oncorhynchus kisutch). Microb. Ecol. 51, 422–430. doi: 10.1007/s00248-006-9037-9
Rutschmann, S., Matschiner, M., Damerau, M., Muschick, M., Lehmann, M. F., Hanel, R., et al. (2011). Parallel ecological diversification in Antarctic notothenioid fishes as evidence for adaptive radiation. Mol. Ecol. 20, 4707–4721. doi: 10.1111/j.1365-294X.2011.05279.x
Sato, Y., Sugie, R., Tsuchiya, B., Kameya, T., Natori, M., and Mukai, K. (2001). Comparison of the DNA extraction methods for polymerase chain reaction amplification from formalin-fixed and paraffin-embedded tissues. Diagn. Mol. Pathol. 10, 265–271. doi: 10.1097/00019606-200112000-00009
Schloss, P. D., Westcott, S. L., Ryabin, T., Hall, J. R., Hartmann, M., Hollister, E. B., et al. (2009). Introducing mothur: open-source, platform-independent, community-supported software for describing and comparing microbial communities. Appl. Environ. Microbiol. 75, 7537–7541. doi: 10.1128/AEM.01541-09
Schnorr, S. L., Sankaranarayanan, K., Lewis, C. M., and Warinner, C. (2016). Insights into human evolution from ancient and contemporary microbiome studies. Curr. Opin. Genet. Dev. 41, 14–26. doi: 10.1016/j.gde.2016.07.003
Schofield, O., Ducklow, H. W., Martinson, D. G., Meredith, M. P., Moline, M. A., and Fraser, W. R. (2010). How do polar marine ecosystems respond to rapid climate change? Science 328, 1520–1523. doi: 10.1126/science.1185779
Shi, S.-R., Cote, R. J., Wu, L., Liu, C., Datar, R., Shi, Y., et al. (2002). DNA extraction from archival formalin-fixed, paraffin-embedded tissue sections based on the antigen retrieval principle: heating under the influence of pH. J. Histochem. Cytochem. 50, 1005–1011. doi: 10.1177/002215540205000802
Shi, S.-R., Datar, R., Liu, C., Wu, L., Zhang, Z., Cote, R. J., et al. (2004). DNA extraction from archival formalin-fixed, paraffin-embedded tissues: heat-induced retrieval in alkaline solution. Histochem. Cell Biol. 122, 211–218. doi: 10.1007/s00418-004-0693-x
Solomon, S., Plattner, G.-K., Knutti, R., and Friedlingstein, P. (2009). Irreversible climate change due to carbon dioxide emissions. Proc. Natl. Acad. Sci. 106, 1704–1709. doi: 10.1073/pnas.0812721106
Sullam, K. E., Essinger, S. D., Lozupone, C. A., O'connor, M. P., Rosen, G. L., Knight, R., et al. (2012). Environmental and ecological factors that shape the gut bacterial communities of fish: a meta-analysis. Mol. Ecol. 21, 3363–3378. doi: 10.1111/j.1365-294X.2012.05552.x
Tarnecki, A. M., Burgos, F. A., Ray, C. L., and Arias, C. R. (2017). Fish intestinal microbiome: diversity and symbiosis unraveled by metagenomics. J. Appl. Microbiol. 7, 2–17. doi: 10.1111/jam.13415
Thomas, C. A., and Doty, P. (1956). The mild acidic degradation of desoxyribose nucleic acid. J. Am. Chem. Soc. 78, 1854–1860. doi: 10.1021/ja01590a023
Tito, R. Y., Knights, D., Metcalf, J., Obregon-Tito, A. J., Cleeland, L., Najar, F., et al. (2012). Insights from characterizing extinct human gut microbiomes. PLoS ONE 7, e51146. doi: 10.1371/journal.pone.0051146
Trathan, P. N., and Agnew, D. (2010). Climate change and the Antarctic marine ecosystem: an essay on management implications. Antarctic Sci. 22, 387–398. doi: 10.1017/S0954102010000222
Turnbaugh, P. J., Ley, R. E., Mahowald, M. A., Magrini, V., Mardis, E. R., and Gordon, J. I. (2006). An obesity-associated gut microbiome with increased capacity for energy harvest. Nature 444, 1027–1131. doi: 10.1038/nature05414
Vacchi, M., and La Mesa, M. (1995). The diet of the Antarctic fish Trematomus newnesi Boulenger, 1902 (Nototheniidae) from Terra Nova Bay, Ross Sea. Antarctic Sci. 7, 37–38. doi: 10.1017/S0954102095000071
Van De Putte, A. P., Janko, K., Kasparova, E., Maes, G. E., Rock, J., Koubbi, P., et al. (2012). Comparative phylogeography of three trematomid fishes reveals contrasting genetic structure patterns in benthic and pelagic species. Mar. Genom. 8, 23–34. doi: 10.1016/j.margen.2012.05.002
Wandeler, P., Hoeck, P. E., and Keller, L. F. (2007). Back to the future: museum specimens in population genetics. Trends Ecol. Evol. 22, 634–642. doi: 10.1016/j.tree.2007.08.017
Ward, N. L., Steven, B., Penn, K., Meth,é, B. A., and Detrich, W. H. (2009). Characterization of the intestinal microbiota of two Antarctic notothenioid fish species. Extremophiles 13, 679–685. doi: 10.1007/s00792-009-0252-4
White, M. (1991). “Age determination in Antarctic fish,” in Biology of Antarctic fish. eds. G. di Prisco, B. Maresca, and B. Tota (Berlin: Springer), 87–100.
Willerslev, E., Davison, J., Moora, M., Zobel, M., Coissac, E., Edwards, M. E., et al. (2014). Fifty thousand years of Arctic vegetation and megafaunal diet. Nature 506:47. doi: 10.1038/nature12921
Keywords: natural history museum, notothenioidei, 16S rRNA, COI, Southern Ocean, Antarctica
Citation: Heindler FM, Christiansen H, Frédérich B, Dettaï A, Lepoint G, Maes GE, Van de Putte AP and Volckaert FAM (2018) Historical DNA Metabarcoding of the Prey and Microbiome of Trematomid Fishes Using Museum Samples. Front. Ecol. Evol. 6:151. doi: 10.3389/fevo.2018.00151
Received: 11 June 2018; Accepted: 10 September 2018;
Published: 28 September 2018.
Edited by:
Oana Moldovan, Emil Racovita Institute of Speleology, RomaniaReviewed by:
Katy Morgan, University of Bath, United KingdomCopyright © 2018 Heindler, Christiansen, Frédérich, Dettaï, Lepoint, Maes, Van de Putte and Volckaert. This is an open-access article distributed under the terms of the Creative Commons Attribution License (CC BY). The use, distribution or reproduction in other forums is permitted, provided the original author(s) and the copyright owner(s) are credited and that the original publication in this journal is cited, in accordance with accepted academic practice. No use, distribution or reproduction is permitted which does not comply with these terms.
*Correspondence: Franz M. Heindler, ZnJhbnptYXhpbWlsaWFuLmhlaW5kbGVyQGt1bGV1dmVuLmJl
orcid.org/0000-0003-4305-7296
Disclaimer: All claims expressed in this article are solely those of the authors and do not necessarily represent those of their affiliated organizations, or those of the publisher, the editors and the reviewers. Any product that may be evaluated in this article or claim that may be made by its manufacturer is not guaranteed or endorsed by the publisher.
Research integrity at Frontiers
Learn more about the work of our research integrity team to safeguard the quality of each article we publish.