- 1Institute of Biodiversity, Animal Health & Comparative Medicine, University of Glasgow, Glasgow, United Kingdom
- 2Centre for Ecological and Evolutionary Synthesis, Department of Biosciences, University of Oslo, Oslo, Norway
- 3Department of Biological and Environmental Sciences, University of Stirling, Stirling, United Kingdom
- 4Centre for Organismal Studies Heidelberg, Department of Biodiversity and Plant Systematics, Botanic Garden and Herbarium Heidelberg, University of Heidelberg, Heidelberg, Germany
- 5Department of Bioinformatics, University of Oslo, Oslo, Norway
Comparative genomics of non-model organisms has resurrected whole genome duplication (WGD) from being viewed as a somewhat obscure process that happens in plants to a primary driver of eukaryotic diversification. The shadow of past ploidy increases has left a strong signature of duplicated genes organized into gene families, even in small genomes that have undergone effectively complete rediploidization. Nevertheless, despite continually advancing technologies and bioinformatics pipelines, resolving the fate of duplicate genes remains a substantial challenge. For example, many important recognition processes are driven not only by allelic expansion through retention of duplicates but also by diversification and copy number variation. This creates technical difficulties with assembly to reference genomes and accurate interpretation of homology. Thus, relatively little is known about the impacts of recent polyploidization and hybridization on the evolution of gene families under selective forces that maintain diversity, such as balancing selection. Here we use a complex of species and ploidy levels in the genus Arabidopsis (A. lyrata and A. arenosa) as a model to investigate the evolutionary dynamics of a large and complicated gene family known to be under strong balancing selection: the receptor-like kinases, which include the female component of genetically controlled self-incompatibility. Specifically, we question: (1) How does diversity of S-receptor kinase (SRK) alleles in tetraploids compare to that in their close diploid relatives? (2) Is there increased trans-specific polymorphism (i.e., sharing of alleles that transcend speciation, characteristic of balancing selection) in tetraploids compared to diploids due to the higher number of copies they carry? (3) Do these highly variable loci show evidence of introgression among extant species/ploidy levels within or outside known zones of hybridization? (4) Is there evidence for copy number variation among paralogs? We use this example to highlight specific issues to consider when interpreting gene family evolution, particularly in relation to polyploids but also more generally in diploids. We conclude with recommendations for strategies to address the challenges of resolving such complex loci in the future, using advances in deep sequencing approaches.
Introduction
Background and Aims
The sequencing of the human genome in 2001 (Lander et al., 2001) promised to revolutionize modern medicine and lead to a new era in understanding the complexity of genetic control of complex phenotypes. While this has certainly been true, it is really the comparative genomics of non-model organisms that has led to a complete revolution in understanding (e.g., Seeb et al., 2011; da Fonseca et al., 2016). One unexpected finding was that whole genome duplication (WGD) has been an important process contributing to the genomic history of all eukaryotes, including those with relatively small genomes, such as the yeast Saccharomyces cerevisiae (Wolfe and Shields, 1997) and the model plant Arabidopsis thaliana (Blanc and Wolfe, 2004). Although Susumu Ohno in the late 1960s had emphasized the central role of gene duplication in the evolutionary history of vertebrates (Ohno, 1970), it wasn't until after his death in 2000 that comparative genomic studies confirmed that fish had undergone multiple rounds of WGD (e.g., Meyer and Van de Peer, 2005), as he had predicted. He also had predicted that effective rediploidization following duplication was inevitable but that some duplicates would be retained to perform new or specialized functions, leaving a footprint of past duplications and organization of genes into gene families. His ideas about the fates of duplicate genes to include specialization of function (now known as “subfunctionalization”; Force et al., 1999) also have been resurrected and form the basis for understanding the history of complex genomes such as salmonids, which underwent an independent WGD after the last teleost specific duplication (Hermansen et al., 2016; Lien et al., 2016). Comparative studies of vertebrates have thus been critical for establishing polyploidization as a creative evolutionary force shaping the genomes of all eukaryotes (Van de Peer et al., 2017), as had long been recognized for plants (e.g., Soltis et al., 1992; Adams, 2007).
Nevertheless, despite recognition that duplicated genes are critical for understanding genome structure and function (Van de Peer et al., 2017), the practicalities of assembling duplicates in genomic resequencing studies, resolving orthology, and interpreting their potentially redundant effects on phenotypes remains a substantial challenge (da Fonseca et al., 2016). Retention of duplicate genes following genomic or tandem duplication is non-random (Adams, 2007) and is both constrained and promoted by achieving appropriate levels of expression (e.g., Gout and Lynch, 2015; Mattenberger et al., 2017; Rodrigo and Fares, 2018). The “gene balance” hypothesis, for example, predicts that loci involved in regulating levels of expression of integrated genetic pathways (such as transcription factors or members of signal transduction pathways) should show increased retention of duplicates to maintain coordinated function (Birchler and Veitia, 2010). Genes for which high expression is advantageous might be expected to retain expression in duplicated copies whereas divergence in patterns of expression could be advantageous for others. Genes that are retained in duplicate through one round of WGD also have been found to be preserved through later rounds (Seoighe and Gehring, 2004). Thus, not considering the role of gene copies retained in duplicate could alter interpretation of regulatory processes associated with adaptation.
One type of adaptive process often associated with large and complex gene families is recognition of self vs. non-self, where high polymorphism is favored by continually changing selection pressures, and retention of duplicate copies could be beneficial for increasing allelic repertoire. For example, the “big bang” theory of the emergence of the adaptive immune systems in vertebrates invokes multiple rounds of WGD as the major source of this potential (Flajnik and Kasahara, 2010). Similarly, investigation of the genomic repertoire of pathogen-associated genes (R genes) in several crop plants through targeted sequence capture (Jupe et al., 2012, 2013; Giolai et al., 2016; Van Weymers et al., 2016) has revealed much more extensive gene families than was previously predicted based on whole genome resequencing studies. R genes have also been demonstrated to show signatures of adaptive introgression between closely related species of Arabidopsis, with extensive trans-specific sharing of alleles across species (Bechsgaard et al., 2017). An added complication for these types of gene families is that copy number can be variable even among individuals within a species (e.g., Mable et al., 2015), meaning that genome references will not always include the full complement of copies. Copy number variation has been linked to disease severity in humans (Beckmann et al., 2007; Wheeler et al., 2008) and adaptive processes in other organisms (Saintenac et al., 2011; Zmienko et al., 2014; Duvaux et al., 2015; Hull et al., 2017) but methods that can reliably distinguish between lack of coverage and variation in presence of a particular gene copy are required to fully evaluate the evolutionary significance of presence/absence polymorphisms following gene duplication.
The high polymorphism expected for recognition genes means that they are prime candidates to be “lost” in genomic resequencing studies, even in diploids. For example, genes controlling sporophytically controlled self-incompatibility (SI) in plants have been found to be missing from resequencing assemblies because they are too divergent from the reference genome and so trawling in the unassembled reads is necessary to characterize these highly polymorphic genes (Mable et al., 2017). Both male and female components are members of large gene families that show extensive trans-specific polymorphism, with highly similar alleles shared across species and even genera but high divergence between functional specificities (Schierup et al., 1998; Paetsch et al., 2006; Castric and Vekemans, 2007; Busch et al., 2008; Guo et al., 2011; Tedder et al., 2011; Leducq et al., 2014). The gene controlling female specificity (S-receptor kinase, SRK) is part of a large family of receptor kinases, which evolved through a complex history of gene duplication and loss, followed by gene fission and fusion (Xing et al., 2013). Gene conversion between SRK and other members of the gene family is also thought to have contributed to expansion of functional allelic diversity (Prigoda et al., 2005; Guo et al., 2011). This creates additional challenges with interpreting which variants are parts of the functional locus regulating the SI response and which are functionally unlinked but show high sequence similarity. For sporophytic SI, the phenotype of the pollen is determined by the genotype of the diploid (or tetraploid) parent, so there can be dominance in both pollen and stigma. Dominance is known to be complex, with non-linear interactions that can differ between pollen and stigma (Lewis, 1947; Stevens and Kay, 1989; Hatakeyama et al., 1998; Shiba et al., 2002; Mable et al., 2003; Llaurens et al., 2009; Schoen and Busch, 2009). Trans-specific polymorphism (i.e., sharing of alleles that transcends speciation) of SRK alleles has been well established for diploids (Charlesworth et al., 2006; Boggs et al., 2009; Castric et al., 2010), and is thought to be a key indicator of the action of balancing selection (Takahata, 1990). However, the strength of balancing selection on tetraploids has not been assessed specifically. Since tetraploid individuals can carry up to four different SRK alleles, there is potential for increased sharing across species, at least of recessive alleles. They can also carry multiple copies of recessive alleles (Mable et al., 2004), which could result in the maintenance of more variants within specificities than for diploids. While previous work has demonstrated that linkage and dominance works similarly in tetraploid compared to diploid Arabidopsis lyrata (Mable et al., 2004), the evolutionary dynamics of S-alleles in tetraploids has not been studied.
In addition, interpreting the fate of duplicate genes in polyploids is complicated by the fact that hybridization is often associated with WGD and so it can be difficult to disentangle the effects of combining and duplicating genomes on patterns of duplicate gene expression or dynamics of gene families (e.g., Evans, 2007; Guggisberg et al., 2009; Mable, 2013). Fortunately, rapid advances in sequencing technology and bioinformatic processing mean that the toolbox available to resolve such challenges continues to improve. Targeted sequence capture, for example, has been used effectively to investigate genomic changes in polyploids (Salmon et al., 2012; Gardiner et al., 2016; Krasileva et al., 2017). However, even with these advances in technology there are important issues to consider when resolving and interpreting evolutionary dynamics of gene families, particularly for systems in which recent polyploidization and hybridization could complicate accurate assembly into orthologs and subsequent genotyping within and between copies.
The purpose of this paper is to discuss these issues in the context of understanding the evolutionary dynamics of the SRK gene family in a species complex (A. lyrata and A. arenosa) that includes both diploids and tetraploids, with tetraploids showing extensive introgression in a hybrid zone in central Europe (Schmickl et al., 2010; Jørgensen et al., 2011; Schmickl and Koch, 2011; Hohmann et al., 2014; Muir et al., 2015; Novikova et al., 2016; Hohmann and Koch, 2017). In A. arenosa, tetraploids have been predicted to have arisen through autopolyploidisation (Arnold et al., 2015); secondary contact with A. lyrata during interglacial and postglacial range contractions and expansions has subsequently led to introgression between tetraploids in the two species. Our intent was to use investigation of S-receptor kinase evolution in this species complex as a model for understanding how balancing selection operates in polyploid genomes and to determine whether these highly polymorphic gene families could be useful indicators of hybridization and introgression. Specifically, our objectives were to question: (1) How does diversity of SRK-related alleles in tetraploids compare to that in their close diploid relatives? (2) Is there increased trans-specific polymorphism of SRK alleles in tetraploids compared to diploids because of the increased number of copies they can carry? (3) Do these highly variable loci show evidence of introgression among extant species/ploidy levels within or outside known zones of hybridization? (4) Is there evidence for copy number variation among paralogs?
We use these questions to highlight the challenges for interpreting gene family evolution, particularly in polyploids, but also relevant to diploids. We conclude with recommendations for how some of these challenges might be overcome using deep sequencing approaches. We reiterate the recommendation from others (Salmon et al., 2012; Jupe et al., 2013; Gardiner et al., 2016; Van Weymers et al., 2016; Krasileva et al., 2017) that non-amplicon based targeted sequence capture (e.g., whole genome exon capture or targeting of particular gene families) is the most promising method for tackling the full complexity of gene family evolution in complex genomes but suggest cautionary strategies that should be considered when interpreting evolutionary patterns.
Notes on Terminology and Known Challenges Associated With the SRK Gene Family
A complication with understanding the evolution of complex gene families is distinguishing what is meant by an “allele.” For SRK, there can be sequence variation within “specificities,” which are SRK types that confer a specific SI phenotype (i.e., a protein expressed on the surface of the stigma that is recognized as self by the comparable protein expressed on the surface of the pollen grain). These specificities (which we will refer to as “alleles”) can be as divergent from one another as they are from other genes (which we will refer to as “loci”) in the same gene family. Moreover, phylogenetic clustering alone is not sufficient to predict which sequence variants represent SRK alleles because gene conversion with unlinked loci has resulted in higher similarity between paralogs than among SRK alleles (Prigoda et al., 2005). Diploid individuals should contain only two functional SRK alleles but could contain varying numbers of loci in the gene family that are not linked to the SI phenotype; since tetraploids can contain multiple copies of the same allele without altering the specificity or dominance (Mable et al., 2004), the number of SRK alleles expected in a polyploid cannot be predicted. Thus, assigning “sequence variants” to gene family loci or SRK alleles is even more complicated in polyploids than for diploids. SRK alleles have been grouped into four different dominance classes (A1, A2, A3, B; Prigoda et al., 2005). Polymorphisms within specificities/alleles (which we will refer to as “haplotypes”) are more apparent for recessive than dominant alleles because the former are expected to occur at higher frequency and show more sharing between populations (Bechsgaard et al., 2006; Castric and Vekemans, 2007; Castric et al., 2008, 2010; Stoeckel et al., 2008; Llaurens et al., 2009; Goubet et al., 2012). There is a single most recessive allele (S1, Class A1; Prigoda et al., 2005) that is found globally and in multiple species in the genus Arabidopsis (Mable et al., 2003; Dart et al., 2004; Prigoda et al., 2005; Mable and Adam, 2007; Castric et al., 2010; Foxe et al., 2010). Alleles in Class B are recessive to all other classes except S1 but are more similar to unlinked loci (Aly13-2 and Aly13-7) than to the other classes (Prigoda et al., 2005) and show more intra-allele polymorphisms than dominant alleles (Classes A2 and A3; Prigoda et al., 2005; Castric et al., 2010). The high trans-specific polymorphism also means that naming of alleles can be confusing because a variant found in certain species is often provided a specific number before discovering that it potentially represents the same specificity as an already named allele in another species (Castric et al., 2010). Thus, alleles are named with the species in which they were originally described as a prefix (e.g., Aly refers to A. lyrata, Aha refers to A. halleri, Ath refers to A. thaliana, Aar refers to A. arenosa). Finally, since the SI phenotype is determined by a combination of variants at the female SRK and male SCR genes, phenotypic specificities are labeled only “S#” (e.g., S1) for segregation analyses.
From our previous studies on the evolutionary dynamics of SRK alleles in diploids, we have already described challenges in generating robust data for interpreting these complex gene families in diploids, relevant for the sequencing strategies we apply here: (1) Primers designed to be general enough to recognize all SRK alleles also amplify the rest of the gene family, so a major challenge is assigning sequence variants to loci (Schierup et al., 2001; Charlesworth et al., 2003b; Mable et al., 2003, 2017; Mable and Adam, 2007). (2) This is complicated by the fact that, due to the extensive polymorphism in SRK and evidence that gene conversion has contributed to allelic repertoire, paralogs that are not linked to the SI phenotype can be more similar to “real” alleles than “real” alleles are to one another, so similarity can't always be used to assign functionality (Schierup et al., 2001; Mable et al., 2003; Prigoda et al., 2005). (3) Amplicon-based approaches are inherently at risk of generating PCR recombinants between copies, making it difficult to distinguish errors from actual recombination, introgression in hybrids, or gene conversion between sequences. (4) It is also difficult to distinguish presence/absence of paralogs from amplification biases during PCR (Mable et al., 2017). (5) There is extensive length heterogeneity within and between members of the gene family, so it can be difficult to establish the positional homology necessary to interpret patterns of selection (Charlesworth et al., 2003a). (6) The highly polymorphic nature of SRK alleles means that they are sometimes too divergent from the reference genome to be assembled using standard filtering strategies; this means that these types of alleles might frequently be found in the unassembled reads for resequencing projects (Mable et al., 2017).
Materials and Methods
Sampling and Overview of Methods
Samples were obtained from both diploid and tetraploid populations of A. lyrata and A. arenosa sampled from Central Europe (Table 1). Although current systematics suggests separation of diploid and tetraploid A. arenosa into distinct species taxonomically (Koch et al., 2008), for simplicity, we will refer to both as A. arenosa here. We sampled individuals from 3-5 populations of each “type”: A2x refers to diploid A. arenosa, A4x to tetraploid A. arenosa, L2x to diploid A. lyrata and L4x to tetraploid A. lyrata. Tetraploid populations occurring in a hybrid zone between the two species (Schmickl, 2009; Schmickl and Koch, 2011; Hohmann et al., 2014; Muir et al., 2015; Novikova et al., 2016) were included to test for patterns of introgression. Diploids have not been found to hybridize (Jørgensen et al., 2011) and so were considered “pure” populations. To test patterns of linkage of sequence variants with the SI phenotype, we also included 104 individuals from crosses between A. lyrata tetraploid parents whose genotypes had been partially resolved by cloning and Sanger sequencing; we performed di-allele crosses within these families to establish SI phenotypes that could be compared to the 454 genotypes.
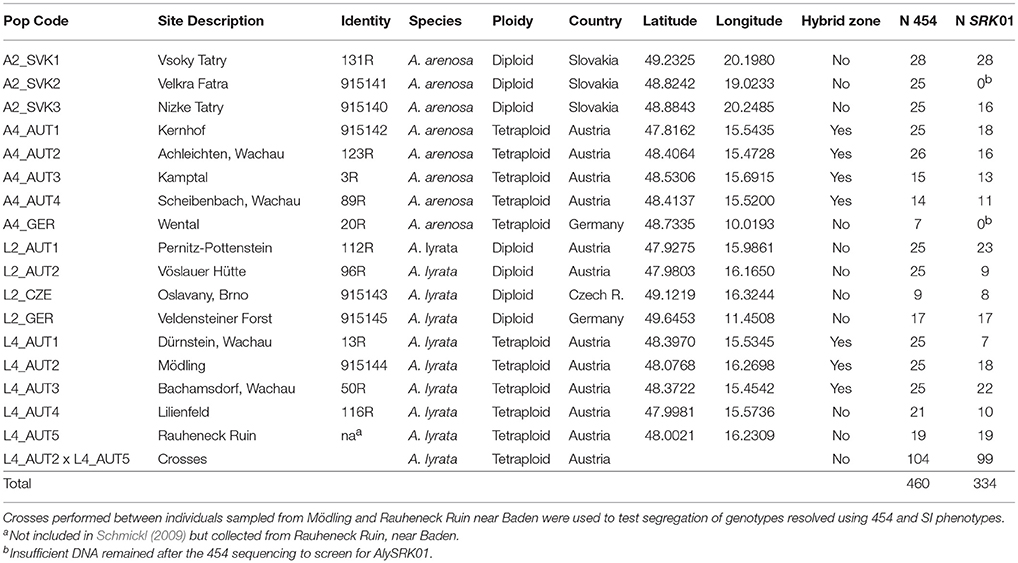
Table 1. Populations sampled, indicating the code (Pop Code) used to identify populations in our study, the population identifier (identity) from Schmickl (2009), site description, ploidy, species, country of origin, GPS coordinates (latitude and longitude), and whether the population is in the known hybrid zone in Austria, as well as sample sizes for the 454 pyrosequencing (N 454) and targeted amplicon sequencing of SRK01 (N SRK01).
We used a combination of approaches to address the main research questions: (1) 454 pyrosequencing using degenerate primers (Supplementary Table 1) targeting the SRK gene family (Jørgensen et al., 2012) to characterize diversity and patterns of allele sharing in diploids and polyploids; (2) direct Sanger sequencing to investigate signatures of introgression in shared haplotypes and for segregation analyses to test linkage to the SI phenotype; (3) cloning and Sanger sequencing using degenerate primers (Supplementary Table 1) to obtain longer products than possible with 454 pyrosequencing to further characterize potentially new alleles; and (4) using data from a recent genomic resequencing study (Novikova et al., 2016) to search for the SRK gene family using novel assembly approaches, to test whether copy number variation and patterns of introgression can be mined using existing genomic data. We focused on variation in exon 1 (the S-domain) because it contains the sites used for recognition of self vs. non-self (Schierup et al., 2001; Charlesworth et al., 2003a). However, we also used the genome mining approach to determine whether we could pull out full-length sequences that include the functional kinase domain (exons 3-7).
While 454 pyrosequencing has largely been replaced by methods demonstrated to show higher accuracy such as Illumina (Schirmer et al., 2015, 2016; D'Amore et al., 2016), we use results from this study as a platform to highlight considerations for working with gene families that should apply across methods. We thus haven't focused on attempting to resolve 454 specific problems but instead on general issues with clustering and assigning sequence variants to loci and designating allelic specificities for interpretation of gene family evolution. We include these as “challenges” in relation to the methods used to address each objective.
Detailed Methodology
Clustering and SRK Genotyping Strategies
To increase the probability of amplifying all variants of SRK present in the populations sampled, we used 454 pyrosequencing of pooled amplicons from four sets of degenerate primers but sharing a common reverse sequence, SLGR (Supplementary Table 1; Schierup et al., 2001). Detailed methods for the 454 analyses are described in Jørgensen et al. (2012), including estimation of error rates and the use of segregation within known families to test the reliability of genotyping. The initial paper described the strategies used for clustering reads into contigs and filtering to reduce errors. We recommended that optimal clustering was obtained with a 90% sequence similarity criterion and excluding sequences present at a frequency of <7% of the total reads for an individual; these conclusions were based on a subset of the original data that included repeated runs involving the same individuals. We also recommended that clustering should be conducted after reads were trimmed to 200 bp from the “common primer” end (SLGR in this case).
Although the crosses between tetraploid A. lyrata individuals confirmed presence of the expected SRK alleles known to be present in the parents, they also indicated some inaccuracy in allele calls in relation to barcodes; a number of alleles that were not in the parents were assigned to individuals from the crosses, sometimes at high read numbers (see Jørgensen et al., 2012). We concluded that this was due to tag switching between barcodes, as had been suggested from other studies (van Orsouw et al., 2007; Carlsen et al., 2012). Blank lanes (negative controls) also sometimes contained sequences matching known SRK alleles, again often at high read numbers. We thus modified our filtering and clustering strategies in the analysis of the full dataset.
Reads were initially assembled into contigs based on clustering to sequences from a reference database of known SRK alleles and known members of the gene family that have been characterized in other studies and from our unpublished data from Sanger sequencing. A second iteration then used newly sequenced reads as seeds for clustering, in order to identify putatively new alleles (generating “read-only” contigs). BLAST analyses of “read only” contigs indicated that some known alleles (both SRK and paralogs) had been fragmented into multiple contigs. In such cases, contigs for a particular allele were combined, sequences sorted by barcode, and read numbers counted for each individual that contained a particular sequence type. Remaining “read only” contigs that did not show at least 80% similarity to S-related kinases from Genbank were not considered further. Final contigs were then sorted into putative “types”: known SRK alleles, putatively new SRK-like variants, or known paralogs. Contigs assigned to SRK alleles whose dominance had been established previously (Prigoda et al., 2005; Goubet et al., 2012) were further sorted into the following classes: (1) A1, consisting of a single most recessive allelic specificity that has been found globally in Arabidopsis species (SRK01); (2) A2, dominant to all other classes; (3) A3, recessive only to class A2; and (4) B, recessive to all except A1 and showing high similarity to unlinked loci (Aly13-2 and Aly13-7). Contigs were also inspected for clustering of more than one named SRK allele from the database.
The next step was to subdivide variants within contigs into individual haplotypes, in order to test patterns of trans-specific polymorphism and to assess evidence for introgression between species. In our pilot study (Jørgensen et al., 2012) we recommended that only sequence variants present in at least 7% of the reads for an individual should be “counted” as true variants. However, in the full analysis, inspection of the contigs associated with particular alleles revealed very uneven read numbers both between individuals (ranging from a minimum of a single read to a maximum of 1,126 reads in the 465 individuals screened; average 344 ± 156) and across loci (i.e., SRK alleles and paralogs) within individuals. Low read numbers of particular alleles were also not directly proportional to the overall read numbers in the individual. The strict 7% threshold would have excluded some alleles that amplified in multiple individuals but were only present at low read numbers within individuals. A striking example was SRK01: it was fragmented across multiple contigs but when reassembled, it tended to be found at very low read numbers within individuals but was found across a wide range of individuals and showed population- and species-specific variants, as expected for a recessive allele (Billiard et al., 2007; Goubet et al., 2012). Many individuals showed <20 reads but the individuals that showed high read numbers (>100) tended not to show amplification of any other alleles, suggesting competition in the PCR when other alleles were present.
For haplotype calling, we thus also considered genotype calls at thresholds of at least 4% of reads and between 0 and 4% of reads. A problem with assessing such optimization strategies when including tetraploids is that there is not a robust basis for excluding individuals based on numbers of expected haplotypes. Although we could use diploids to determine thresholds of read numbers that minimized calling of more than two SRK alleles per individual and predicting homozygosity only for recessive alleles, this was confounded by the difficulties of predicting linkage of newly identified alleles (Charlesworth et al., 2003b; Prigoda et al., 2005). Tetraploids are expected to have up to four copies of SRK per individual but they can also contain multiple copies of recessive alleles (Mable et al., 2004), precluding extrapolating “confidence thresholds” based on diploids. We thus decided on a conservative threshold of at least 20 reads for a given haplotype to make relative comparisons among populations and species in the frequency of presence of particular variants. For reconstruction of evolutionary relationships among alleles, haplotypes present in <20 reads in a single individual and individuals with <200 total reads were excluded.
Statistical Analyses
To investigate whether there were differences in sequencing quality, detection biases, or real differences in frequency of sequence variants found we used generalized linear models to test whether the variation was significantly explained by ploidy, species or their interaction. Since multiple 454 runs were used for genotyping, we included barcoding tag number and lane as random effects, to account for any variation they explained. Analyses were conducted using JMP version 10.0 (SAS Institute, Incorporated).
Reconstructing Evolutionary Relationships Among Alleles
To establish phylogenetic relationships of newly identified alleles and to predict their dominance, we aligned the 454 sequences to the reference set (Supplementary Data Sheet 1) and reconstructed phylogenetic trees, using MEGA 7.0 (Kumar et al., 2016). We extracted consensus sequences for each haplotype of the SRK-like alleles identified and initially performed multiple alignments using the online version of Clustal Omega (Sievers et al., 2011) and then optimized by eye to establish positional homology and to set the correct reading frame to minimize stop codons, using Se-al version 2.0 (Rambaut, 1996) and McClade version 4.0 (Maddison and Maddison, 2000). To assess patterns of trans-specific polymorphism, if there was an exact match of a sequence to the reference database used for clustering, we named the haplotype “REF_HAP1” but if there was no exact match we retained the database allele (just named “REF”). We also added homologs from A. lyrata, A. arenosa, A. halleri and A. thaliana from Genbank for each specificity identified among the 454 samples (e.g., AHASRK04 and ATH-haplogroup A have been identified as homologs of AlySRK37; Bechsgaard et al., 2006). As implemented in MEGA, the best fitting substitution model was identified using ModelTest and then Maximum Likelihood was used to cluster sequences, using 1,000 bootstrap replicates. Due to the reticulate nature of evolution in this gene family, a strictly bifurcating evolutionary history is not expected but a tree-like representation is useful for identifying clusters of similar sequences. In previous studies, we have found that phylogenetic clustering is informative about dominance for Class A3 and B alleles but that Class A2 are paraphyletic based on alignments of approximately 900 bp of sequences in exon 1 of SRK (Prigoda et al., 2005). We thus used phylogenetic clustering to predict dominance of new specificities identified or known specificities for which dominance had not been established. We calculated genetic distances within and between dominance classes using both the best fitting substitution model and raw % similarity, using MEGA. We then mapped relative frequency of each haplotype in the four types of populations onto the tree, using Evolview in the Evolgenius package (He et al., 2016).
Testing the Accuracy of 454 Genotyping Using Segregation Analyses
We used the 454 pyrosequencing to genotype SRK from 11 families raised from crosses between tetraploid A. lyrata individuals whose grandparents had at least partially resolved SRK genotypes, in order to test segregation of alleles and as an additional test of reliability of the clustering thresholds set. Given the low read numbers found for SRK01, we established genotypes by a combination of allele-specific Sanger sequencing for this allele with the 454 sequencing for other alleles to compare segregation of alleles within families and to aid in excluding spurious allele calls. For a subset of these crosses, we performed controlled pollinations among all pairwise combinations of individuals, in order to test linkage of the variants identified to the SI phenotype and to predict dominance relationships (as in Mable et al., 2004).
Direct Sanger Sequencing of SRK01
To complement the 454 sequencing, we used targeted direct Sanger sequencing to resolve SRK01 genotypes to be able to investigate signatures of introgression of this recessive allele. We screened all individuals raised from the crosses between tetraploid A. lyrata individuals to aid in segregation analyses and a subset of individuals from the population survey to confirm haplotype calls and obtain more accurate frequencies of variants within and between individuals (Table 1).
We amplified products using an allele-specific primer (qtAlSRK01F: TCCTACATCATCGCAG) with the general reverse primer (SLGR: ATCTGACATAAAGATCTTGACC) that had been used for 454 sequencing. The 20 μL PCR reactions (using reagents from Invitrogen, Inc., Paisley, UK) consisted of 1 μL template, 2 μL 10x PCR buffer (Invitrogen Incorporated, Paisley, UK), 2 μL 10 mM dNTPs, 1 μL 50 mM MgCL2x, 0.2 μL 10 μM of each primer, and 0.2 μL Taq polymerase. The PCRs were run in MJ research thermocyclers using the following program: initial denaturing phase of 3 min at 94°C, 1 min annealing at 54°C, 2 min extension at 72°C; followed by 34 cycles of 30 s at 94°C, 30 s at 54°C, 2 min at 72°C; and a final extension step of 6 min at 72°C.
Individuals that showed amplification of products of the expected size (~500 bp) were sent for sequencing to The GenePool in Edinburgh, using the reverse primer SLGR. Chromatograms were checked for base-calling errors using Sequencher 4.7 (Gene Codes Corporation, Ann Arbor, MI) and BLAST was used to confirm sequence identity.
Sequences were aligned using Sequencher, version 4.7 and heterozygous positions were recorded using IUPAC (International Union of Pure and Applied Chemistry) ambiguity codes. The phase of heterozygous positions was resolved by matching to variants found in the 454 sequencing and to homozygous sequences found in the Sanger sequencing. Genotypes predicted based on this process were then aligned to the specific 454 sequences for each individual. Species-specific variants were identified in diploids based on private haplotypes for the two species. We used the datamonkey server (www.datamonkey.org; Delport et al., 2010), which implements statistical tests associated with the programme HyPhy (Pond et al., 2005), to test for evidence of recombination using GARD (Genetic Algorithm for Recombination Detection; Pond et al., 2006). In addition, we manually inspected alignments for evidence of potential breakpoints and in such cases, aligned each “section” independently to the other haplotypes identified for a particular specificity. Where a putatively recombinant type showed similarity to two or more species-specific haplotypes in different regions of the sequence, they were classified as potentially introgressed. A minimum spanning network (Bandelt et al., 1999) was drawn using PopArt (Leigh and Bryant, 2015) to resolve the relationships among the SRK01 haplotypes.
Cloning and Sanger Sequencing of Longer SRK Alleles
As the 454 sequences were too short to be informative for future population genetics analyses and tests for selection, we used degenerate primers (Supplementary Table 1) to amplify longer products from tetraploid A. lyrata and A. arenosa sampled from the hybrid zone in the Wachau region of Austria (~600 bp, also described in Ruiz-Duarte, 2012). We then used these products as seeds for the genome mining (see section Mining SRK Alleles From Genome Resequencing Data) to determine whether we could determine the genomic location of the “new” alleles found, as an indication of linkage to the S-locus.
Genomic DNA was extracted from three to four leaves from plants of tetraploid A. lyrata and A. arenosa individuals using a modified CTAB protocol (Doyle and Doyle, 1987). Degenerate primers known to amplify a number of different gene family copies and SRK alleles (Schierup et al., 2001) in A. lyrata and A. halleri (Forward: 13SeqF1, 5′-ccgacggtaaccttgtcatcctc-3′ and Reverse: SLGR, 5′-atctgacataaagatcttgacc-3′) were used (Charlesworth et al., 2000). Genomic DNA was mixed with a pair of primers, 10 μmol each, 4 μl of 5x buffer (ready-made), 50 mM MgCl2, 0.4 μl of 10 mM dNTP mixtures, 0.1 μl Taq DNA Polymerase (Mango Taq, Bioline). PCR amplification conditions were as follows: denaturation at 94°C for 2 min followed by 34 cycles of 94°C for 30 s, 50°C for 30 s, and 72°C for 30 s; a final extension at 72°C for 5 min.
PCR products were cloned into pGEM®-T Vector Systems (Promega Inc.). Colony PCR (20–30 colonies per individual) was conducted to test for inserts using SP6 and T7 primers, followed by AluI digestion to identify clones carrying different putative SRK alleles. To avoid errors that might occur during PCR amplification and sequencing, a minimum of three independent clones with the same restriction profile were sequenced at the GATC BIOTECH facility. SeqMan software (DNASTAR, Inc) was used to clean and create consensus sequences.
We created separate alignments for each allele that was found both in the 454 and the Sanger sequencing by aligning the new sequences to references from Genbank and to the 454 sequences, in order to confirm shared specificity (Supplementary Data Sheet 2).
Mining SRK Alleles From Genome Resequencing Data
The 454 pyrosequencing data was not appropriate for determining presence and absence of paralogs because of: (1) the difficulty of distinguishing gene copies from new alleles at the SRK locus; and (2) amplification biases that made it difficult to set thresholds for reliability. Several known paralogs (Aly8, Aly9, Aly13-2/13-7) were expected to amplify with the primer set used. Polymorphic regions like the S-locus are known to be difficult to assemble in genome resequencing studies due to divergence from the reference genome (Mable et al., 2017) but we tested whether de novo assemblies from a genome resequencing study (Novikova et al., 2016) could be used to assess copy number of the SRK–related kinase gene family. We also attempted to pull out full-length sequences that spanned the S-domain (exon 1), transmembrane (exon 2) and kinase domains (exons 3-7) (Charlesworth et al., 2003a).
There are currently 28 fully resequenced genomes available from diploid and tetraploid A. lyrata and A. arenosa, from which we selected three or four individuals from each species and ploidy level to test whether we could obtain useful information on copy number and complete gene sequences. We used our paired end read data (Genbank SRR2040821, SRR2040822, SRR2040825, SRS945917, SRS1256176, SRS1256175, SRR2020827, SRR2040828, SRR2040829, SRR2040830, SRR2040791, SRR3111440, SRR3111441) and trimmed the reads for adapter contamination using cutadapt (Martin, 2011) and the respective adapter sequences. To obtain SRK alleles from these data we attempted two different approaches: mapping based and de novo assembly, on average we used ~110 million paired end reads for the tetraploid accessions and ~60 million reads for the diploid accessions corresponding to an average coverage of 20x.
In the initial mapping strategy we used as reference the S-locus region of the SRK locus on scaffold 7 of the MN74 reference genome (which was originally sampled from a North American outcrossing populations and has the S13 allele of the genes AL7G32720 = SCR, AL7G32730 = SRK, AL7G32710 = ARK3; Mable et al., 2017). Upon mapping we intended to extract reads that mapped to SRK and adjacent sequences in pairs and to perform a de novo assembly of these sequences only. In a first attempt we mapped reads using bwa (Li and Durbin, 2009). However, this approach did not yield any or an extremely low number of reads mapping to SRK, while adjacent regions were covered by the expected number of sequencing reads. Since bwa expects reads to have an identity of 90% or more to the reference and SRK alleles show much lower similarity (as little as 70% identity), we were not successful in mapping SRK reads to the reference. In a second attempt we used Next Gen Mapper (Sedlazeck et al., 2013), which only requires 65% of identity between read and reference. By this approach we were able to map reads to the S-locus including SRK but nevertheless a de novo assembly of these reads into complete or partial copies of the SRK locus failed.
We used CLC genomics workbench (https://www.qiagenbioinformatics.com/) to perform de novo assemblies using standard settings (automatic word and bubble size, minimum contig length 500 bp, reads were mapped back to contigs setting mismatch costs, insertion costs and deletion costs to 3 and length fraction as well as similarity fraction were set to 0.9) and the scaffolding option. Resulting scaffolds/contigs were indexed as BLAST libraries. We initially used FJ867321 (the S-domain from AlySRK30) to BLAST against these libraries to pull out sequences predicted to be SRK based on more than 50% coverage of the query sequence (filtered for low complexity, expect set to 10, word size to 11, match to 2, mismatch to −3, gap existence to 5, gap extension to 2). These hits were aligned to the first exon of AL7G32730 (AlySRK13 from the MN47 reference genome) to identify intron/exon boundaries and then trimmed if necessary. This approach yielded in total 66 sequences in the 13 accessions analyzed (Supplementary Table 10). Therefore, our BLAST search also must have identified other S-domain encoding genes besides SRK.
In order to obtain an overview on the presence of S-domain encoding genes we performed another BLAST search using the first exon of the MN47 SRK against the MN47 reference genome. This search revealed five genes encoding proteins that have an S-domain (AL7G32730 = SRK, AL7G32710 = Aly8, AL6G48380 = Aly3, AL3G23610 = Aly9, AL2G23090 = Aly10.2). From this result we expected that our contigs identified in the 13 resequenced accessions should have their best BLAST hit with one of these five loci. So, we aligned the 66 contig sequences to the first exon of the MN47 SRK and trimmed them in length to the first exon. Then we performed a blast search of the 66 trimmed sequences against the MN47 reference genome. All of the 66 sequences had their best blast hit with one of the five loci we had identified beforehand. Typically hits for AL7G32710, AL6G48380, AL3G23610, AL2G23090 showed a very small E-value and a high score while AL7G32730 hits were characterized by a lower score and E-value due to the lower conservation for alleles of this locus.
We initially used the BLAST results to predict similarity to known SRK alleles and related receptor kinase gene family members available in Genbank for each of the contigs. However, since we had identified potentially new variants in this study, we also aligned sequences pulled out from the resequenced genomes to our reference database and to the sequences found using 454 and the longer Sanger sequences to confirm sequence identity (Supplementary Data Sheet 2; Supplementary Table 12). We used clustering in phylogenetic trees (reconstructed using Maximum Likelihood in MEGA 7.0) to predict SRK specificity and to determine presence/absence of other members of the gene family.
One of the paralogs (Aly9) is known to amplify in all A. lyrata individuals that have been tested using PCR-based screening (Mable, personal observation). We thus used identification of this locus as a control for whether it was likely that the genome-mining approach could be reliably used to detect copy number variation in highly polymorphic gene families. The approach described initially only identified this locus in three of 12 genomes so we trialed another approach, using the sequences in Supplementary Data Sheet 2, along with the 66 contigs originally identified to BLAST the de novo assemblies for each genome. This resulted in an additional 102 contigs, which then were aligned back to the reference database and identities confirmed using cluster analysis. In this analysis Aly9 was resolved for all individuals and more complete genotypes were obtained for SRK and the other paralogs screened, so only the results from this final analysis are presented.
Results and Discussion
Objectives 1 and 2: Diversity and Allele Sharing of SRK in Diploids and Tetraploids
After filtering and assigning variants to alleles based on sequence similarity and predicting dominance classes and linkage to the SI phenotype based on phylogenetic clustering, we identified 107 haplotypes (unique sequence variants) that could be grouped into 63 potential alleles (specificities) that were at least 80% similar to SRK (Figure 1; Supplementary Table 2). Seventeen were potentially new specificities that were <90% similar to the A. lyrata, A. halleri or A. arenosa reference sequences included (Supplementary Table 3). However, seven of these new variants were predicted not to be linked to SI based on phylogenetic clustering and so could represent other members of the gene family. All of the new potentially unlinked alleles were found in diploid and/or tetraploid A. arenosa, with two of them also occurring at high frequency in L4x populations but only a single L2x individual sharing one of the new unlinked alleles with A4x individuals. The new alleles predicted to be linked to SI were distributed more evenly among the two species.
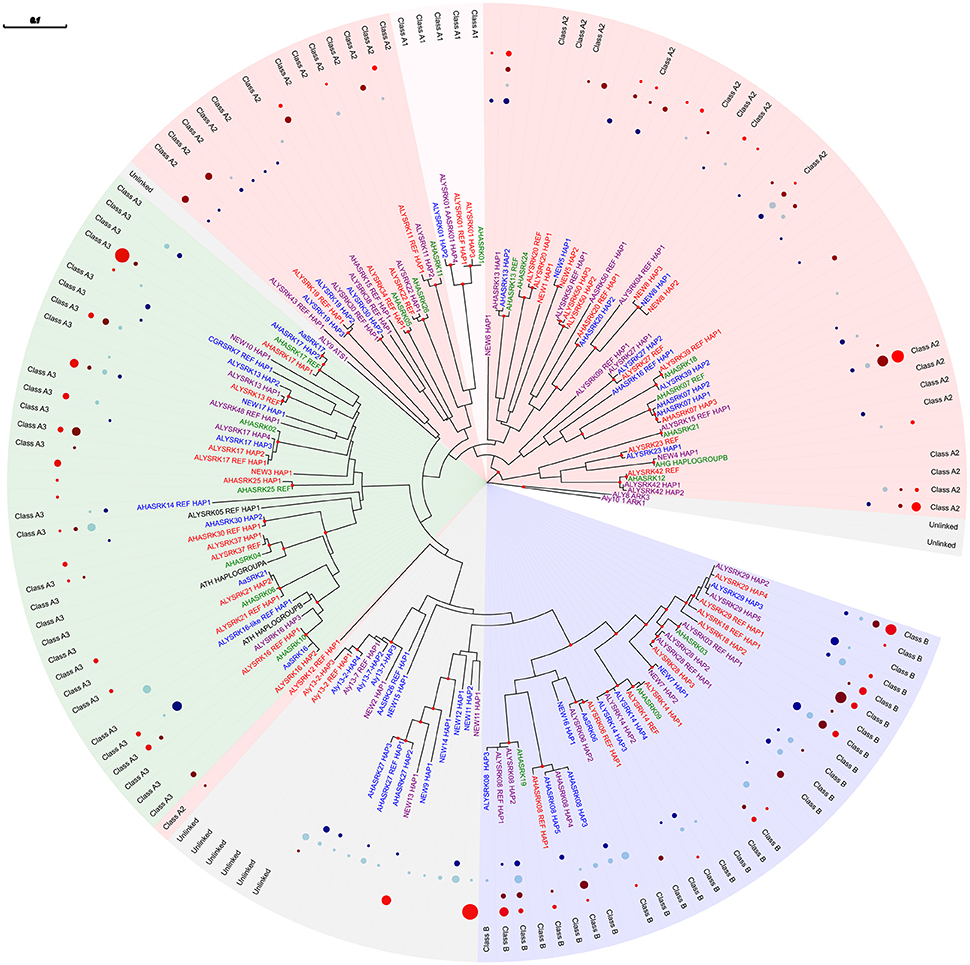
Figure 1. Maximum likelihood tree based on SRK-like sequences resolved through 454 pyrosequencing, reconstructed using MEGA 7 under an HKY85 model of evolution with rate heterogeneity modeled under a gamma distribution and with proportion of invariant sites estimated. Bootstrap proportions above 70% are indicated as filled circles on nodes. The tree was rooted with the unlinked paralogs Aly8 (Ark3 in A. thaliana) and Aly10.1 (Ark1 in A. thaliana). Alleles for each SRK specificity are assigned to a dominance class based on previous studies of A. lyrata (Prigoda et al., 2005) and A. halleri (A1 = yellow; A2 = red; A3 = green; B = blue; unlinked = gray); new alleles or previously identified alleles where dominance has not been confirmed are colored according to the class predicted by their position in the tree. Tip labels are colored according to the species in which they were found in the 454 sequences (lyrata = red; lyrata+arenosa = purple; arenosa = blue) or the origin of the reference allele in cases where there was no exact match (halleri = green; thaliana = black). Also shown is the frequency of a particular haplotype in each of the four groups compared (diploid arenosa, A2x = dark blue; tetraploid arenosa, A4x = light blue; diploid lyrata, L2x = dark red; tetraploid lyrata, L4x = light red). Due to the high number of haplotypes but low read numbers for AlySRK01 and the unlinked loci Aly13-2 and Aly13-7, only a subset of haplotypes are included and frequencies are not indicated.
When accounting for variation due to lane and tag as random effects using generalized linear models, we found no evidence for significant differences between species or ploidy levels or their interactions in terms of number of reads, total number of contigs resolved (indicative of the wider gene family), the number of SRK-like alleles (i.e., variants showing at least 80% similarity to known SRK sequences, so including unlinked alleles), or the number of alleles or haplotypes per individual predicted to be linked to SRK (Supplementary Table 4). There was a significant interaction between ploidy and species in the proportion of contigs resolved that were at least 80% similar to SRK (i.e., more reads were SRK-like than similar to other members of the gene family), with a significantly higher proportion in tetraploids compared to A. arenosa diploids but no significant difference compared to A. lyrata diploids. Since the primers used were developed based on variation within A. lyrata (Schierup et al., 2001; Charlesworth et al., 2003a, 2006), this could be an indication that not all SRK-like alleles were amplified for A. arenosa due to variation in the primer regions, resulting in resolution of more spurious contigs due to non-specific amplification. However, overall, there was very little evidence that tetraploids were fundamentally different to diploids in terms of sequence quality or the ability to resolve variants.
The 200 bp sequences produced similar resolution in phylogenetic clustering as previous studies using 600 bp (Tedder et al., 2011) and resulted in consistent patterns of polymorphism expected for dominant and recessive alleles at SRK. Examination of relative frequency distributions also generally met theoretical expectations but indicated no obvious differences in diversity between ploidy levels. There was extensive variability in relative frequencies of each haplotype, with some being restricted to certain species or populations and some being found across both species and ploidy levels (Figure 1; Supplementary Table 2). We predicted that there should be highest interspecific sharing of individual haplotypes among tetraploids due to their known introgression (Schmickl, 2009; Schmickl et al., 2010; Jørgensen et al., 2011; Schmickl and Koch, 2011) but also because they can maintain more allelic copies within individuals. We found that 23 haplotypes were shared between A4x and L4x compared to 12 between A2x and L2x, including seven that were shared among all four population types (Supplementary Table 2). Sharing between the two types of tetraploids was similar to that among ploidy levels within species (24 among A. lyrata and 22 among A. arenosa). The highest number of private haplotypes was also found for diploids: 19 for A2x and 15 for L2x, compared to 12 for A4x and 8 for L4x. These results are consistent with predicted patterns of introgression among the tetraploids in northeastern Austria (Wachau region and Forealps; Schmickl et al., 2010; Jørgensen et al., 2011; Schmickl and Koch, 2011).
Although it is difficult to separate increased transpecific polymorphism from this introgression, we found some evidence that there might be more differences in selection pressure or demographic history between species than between ploidy levels. Plotting allele frequency distributions for each ploidy and species combination demonstrated an excess of intermediate frequency alleles in both diploid and tetraploid A. arenosa (Figure 2), as expected for a locus under balancing selection (Mable and Adam, 2007). However, the pattern was more skewed toward low frequency alleles in A. lyrata, particularly in tetraploids. In North American populations of A. lyrata, a difference in allele frequency spectrum for SRK was found between inbreeding and outcrossing populations (Mable and Adam, 2007) but the latter showed more similar patterns as those observed for A. arenosa in this study. Since shifts toward intermediate frequencies are also expected for population bottlenecks (Luikart et al., 1998), it is possible that in particular diploid A. arenosa experienced a larger decline in population numbers since the past glaciation. What was striking in the current study was that tetraploids did not have a dramatically higher number of alleles or haplotypes within populations or alleles or haplotypes per individual than diploids, regardless of dominance class (Table 2). Furthermore, for neutral genes, there is a steep gradient of increasing genomic contribution of A4x found within introgressed A. lyrata along a transect in the hybrid zone (Schmickl, 2009; Schmickl et al., 2010; Jørgensen et al., 2011; Schmickl and Koch, 2011; Hohmann et al., 2014; Muir et al., 2015) but this is not reflected in the SRK distribution; i.e., SRK are more mixed than would be predicted based on neutral patterns, as might be expected under balancing selection. This suggests that tetraploids are not fundamentally different from diploids in their capacity for maintaining diversity of SRK, as suggested previously from segregation analyses within tetraploid families based on crosses involving one of the same tetraploid populations studied here (L4_AUT2) and a tetraploid population from Aggsbach, Austria (Mable et al., 2004).
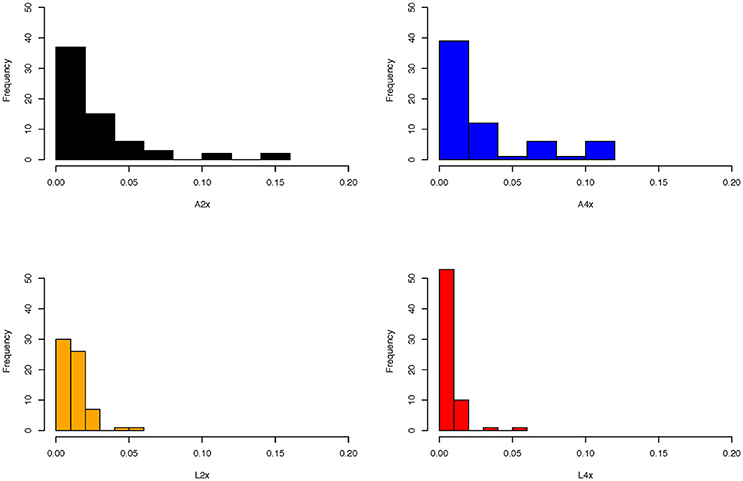
Figure 2. Allele frequency distributions of SRK alleles identified in diploid and tetraploid populations of A. lyrata and A. arenosa using 454 pyrosequencing. Note that there appears to be an excess of intermediate frequencies in A. arenosa (A2x = diploids; A4x = tetraploids), with more of a skew toward low frequency alleles in A. lyrata, particularly in tetraploids.
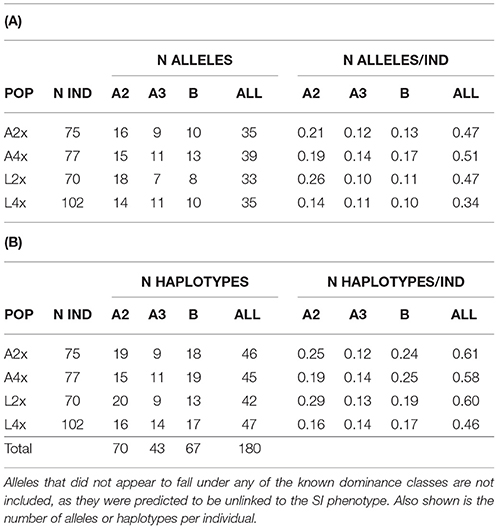
Table 2. Distribution of alleles (A) and haplotypes (B) across diploid (A2x, L2x) and tetraploid (A4x, L4x) populations (POP) for different predicted dominance classes (A2 and A3 are dominant to B), excluding Class A1, which is represented only by SRK01; read numbers were too low to be certain about presence or absence for that allele.
Consistent with theory (Billiard et al., 2007), recessive alleles in diploids have been demonstrated to occur at higher frequency, to show shallower branch lengths in phylogenetic analyses, and more extensive polymorphism within specificities than dominant alleles (Llaurens et al., 2008, 2009; Castric et al., 2010; Vekemans et al., 2011; Goubet et al., 2012). In our study, Class B alleles (recessive to A2 and A3 classes) showed lower intraclass polymorphism (13% average pairwise sequence divergence, compared to 25% for Class A2 and 15% for Class A3) but more haplotypes per allele than the two dominant classes (2.56 ± 1.33 compared to 1.59 ± 0.75 in Class A2 and 1.56 ± 0.89 in Class A3, Table 2) and there was high divergence between classes (26–29%; Table 3). The paralogous locus identified in previous studies that is similar to class B alleles (Aly13-2) showed similar within locus variation (13%) as for class B alleles and lower divergence from class B than the other dominance classes (16% compared to at least 27% to the others). There was a higher proportion of alleles restricted to only one of the species among the dominant (29% for Class A2 and 50% for Class A3) than recessive (20% for Class B) alleles but a majority of the unlinked alleles (67%) were only found in A. arenosa (Supplementary Table 2). Thirteen alleles were found only in tetraploids, but none were Class B and only four (three Class A2 and one Class A3) were shared between the two species. Thus, results were consistent with the increased trans-specific polymorphism expected for recessive alleles at a locus under balancing selection (Billiard et al., 2007; Llaurens et al., 2008; Castric et al., 2010; Goubet et al., 2012).
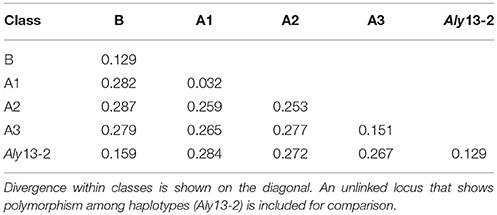
Table 3. Percent sequence divergence within and between dominance classes, for alleles identified using 454 sequencing.
Overall, these results suggest that tetraploids do not show increased mate availability due to an increase in S-locus repertoire but instead might be constrained by the potential mate limitation caused by having “too many” S-alleles. This is similar in theory to expectations for immune genes in animals, where an optimal number of alleles has been suggested as conferring higher fitness than maximizing allelic diversity (Reusch et al., 2001; Aeschlimann et al., 2003; Wegner et al., 2003; Kalbe et al., 2009). The high allele sharing among ploidy levels precluded testing of whether there is relaxed balancing selection acting in tetraploids but this was not suggested by the site frequency distributions, which suggested a stronger species than ploidy effect. Nevertheless, there are some important caveats to consider in the interpretation of these results, due to particular challenges when working with this type of gene families (see Challenges below).
In the crosses between tetraploid A. lyrata individuals, we found the same three SRK01 haplotypes using both 454 and targeted Sanger sequencing (haplotypes 1, 2, and 3). This allowed us to test the accuracy of the 454 genotyping despite the low read numbers for SRK01 and provide more complete data for segregation analyses. For 50% of the individuals identical genotypes were predicted using the two approaches, with 14% testing negative for the allele-specific PCR but positive using 454, compared to 10% showing the opposite pattern (Table 4). Different haplotypes were predicted by the two methods only for a single individual. However, the direct sequencing was more sensitive, resolving heterozygotes in 24% of the individuals that were predicted to be homozygous based on the 454 sequencing (compared to only 2% showing the opposite pattern). Segregation of SRK01 genotypes in the crosses confirmed previous predictions (Mable et al., 2004) that tetraploids could harbor multiple copies of haplotypes for this recessive specificity (Table 5). These data were then combined with segregation of the haplotypes resolved using 454 pyrosequencing (Supplementary Table 5). After excluding 454 alleles not present in the parents, the majority of individuals showed four or fewer expected haplotypes. Comparison of segregation of predicted genotypes with self-incompatibility phenotypes (Figure 3; Supplementary Tables 6, 7), confirmed linkage of two alleles previously tested in other crosses (SRK16 and SRK29) and one that had been identified in the grandparents but had not been deposited to Genbank (SRK48). However, the segregation analyses suggested that not all alleles were detected by 454 and suggested that the stringent filtering in some cases omitted alleles that must have been present based on the incompatibility phenotypes.
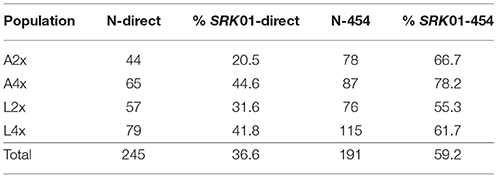
Table 4. Proportion of individuals that tested positive for SRK01 specificity using direct Sanger and 454 sequencing, indicating the population (A2x = diploid A. arenosa; A4x = tetraploid A. arenosa; L2x = diploid A. lyrata; L4x = tetraploid A. lyrata), sample sizes (N-direct, N-454) and % of individuals that tested positive for SRK01 in each.
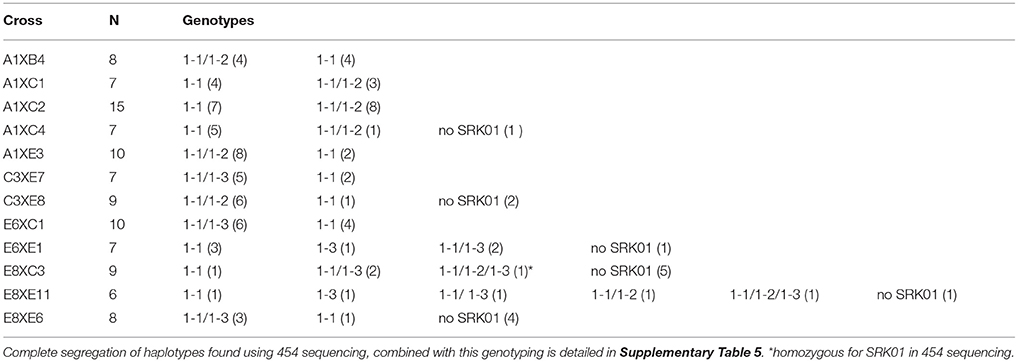
Table 5. Segregation of SRK01 genotypes within families raised from crosses between tetraploid A. lyrata individuals, as determined by direct Sanger sequencing; the number of individuals where a particular genotype was found is indicated in parentheses.
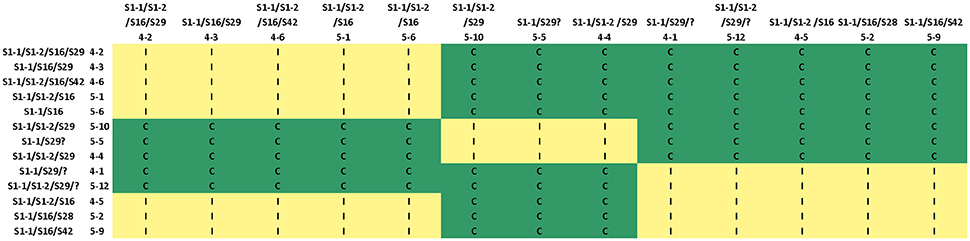
Figure 3. Segregation analysis for the cross A1 × C2, based on combined genotypes from the direct sequencing of SRK01 and from 454 pyrosequencing based on genotyping of other alleles found in the crosses. The cross was between two different tetraploid A. lyrata populations in Austria that were thought to be outside of the hybrid zone with A. arenosa. The predicted genotype of the donor is indicated along the top row and that of the recipient in the column to the left. Incompatible cross combinations are indicated with an I (and shaded yellow), compatible combinations with C (and shaded green). Comparison of segregation of SRK haplotypes with the phenotype suggests that: S16 is expressed with all other haplotypes; it appears to be codominant with S42 in stigmas but recessive in pollen. S29 is recessive to S16. Individuals 4-1 and 5-12 must have an allele that has not been identified because they show different patterns of compatibility than 5-10, 5-5, and 4-4, which also only have S1-1 and S29. S28 was only found in three individuals and only one (5-2) was included in the analyses shown here; based on the 454 genotyping it can be difficult to distinguish S18, S28, and S29 so this could be an error in assignment. Individual 5-5 must have S29 but it was not detected in the 454 analyses.
Challenge: Filtering Decisions for Clustering
Despite recommendations from our pilot study that a threshold of 90% similarity would be appropriate for clustering (Jørgensen et al., 2012), our analyses of the full dataset suggested that a single threshold may not be appropriate for gene families that include different levels of divergence among classes or copies; for example, in relation to dominance (Prigoda et al., 2005). In our study, BLAST analysis of “read only” contigs demonstrated that some known alleles were fragmented across multiple contigs. For recessive alleles (Class B, SRK01) and unlinked loci (Aly9, 13-2 and 13-7), combining contigs resulted in mixtures of haplotypes from different alleles (specificities), making it challenging to assign sequence variants to alleles. While several dominant alleles (Aly16, Aly30, and Aly42) also showed fragmentation, there was no ambiguity in assigning sequence variants to alleles. Resolving recessive alleles into unique contigs thus required more manual manipulation and sorting of variants into haplotypes. Since recessive alleles also had on average more haplotypes per allele (2.44 ± 1.42) than dominant alleles (1.57 ± 0.74 for A2; 1.56 ± 0.89 for A3) (Supplementary Table 2), read numbers per haplotype were often lower, which made setting a single threshold for reducing spurious genotyping difficult.
Challenge: Amplicon Based Errors and Biases
From previous studies we anticipated that the single most recessive allele, SRK01, would be present at high frequency and would show a higher number of haplotypes than other specificities (Billiard et al., 2007; Castric and Vekemans, 2007; Llaurens et al., 2008; Castric et al., 2010; Goubet et al., 2012; Vekemans et al., 2014). In our 454 data, SRK01 was present in all populations surveyed and we identified 15 unique variants that were present in more than one individual; however, read numbers tended to be very low (often with <10 reads per individual) and fell well below the thresholds set for considering “real” presence of a given haplotype used for other loci for most individuals. Although multiple haplotypes differing by a single or few bp are expected for recessive alleles (Castric and Vekemans, 2007), the low read numbers made it difficult to distinguish PCR errors from actual polymorphism. High read numbers were found for some individuals, but they tended to show the presence of few other sequence variants. In addition, several known paralogs that should be present in all individuals (Aly8, Aly9; Charlesworth et al., 2003b) were expected to amplify with the primer set used but this was very inconsistent. Aly9 was present in the majority of individuals but read numbers varied dramatically from 0.5 to 92% of the total reads in an individual. There was a significant difference in the proportion of reads that were Aly9, with A. arenosa tetraploids showing a higher proportion than both diploids, which showed a significantly higher proportion than A. lyrata tetraploids (Supplementary Table 4). Whether this is due to an amplification bias or expansion of the gene family is difficult to distinguish. For Aly8, only 41/460 sequenced individuals showed any amplification and most were present at only low read numbers (maximum 15%). We thus could not assess presence or absence of other members of the gene family based on the 454 sequencing or use the paralogs to make inferences about introgression in the tetraploids to avoid the confounding effects of balancing selection. Even after correcting for chimeras, there was some evidence for recombination in some of the specificities showing polymorphism among populations (e.g., SRK01, some of the class B alleles) but this was difficult to distinguish from PCR recombinants, particularly with only 200 bp of sequence.
Challenge: Assessing the Accuracy of Genotyping
Although arguably more problematic for 454 pyrosequencing than for more recently developed approaches due to tag switching of barcodes, which we previously found could occur for up to 7% of samples (Jørgensen et al., 2012) and has been reported in other studies (Carlsen et al., 2012), the biggest challenge was deciding on thresholds and criteria for assessing accuracy of genotyping and efficiency of filtering strategies. The 200 bp sequences resolved were useful for assessing haplotype diversity within alleles, identifying putatively new alleles, predicting dominance based on phylogenetic clustering, and the distribution of allele and haplotype frequencies among populations. The results also generally fit with theoretical predictions. However, there was less certainty for determining individual genotypes; the crosses, for example, included more alleles than should have been present in some individuals, including alleles that were not identified in the parents (Supplementary Table 5). The haplotype frequencies indicated in Figure 1 and Supplementary Table 2 are thus based on a conservative threshold of at least 20 reads per individual but this likely underestimates patterns of haplotype sharing across populations and species. Nevertheless, an advantage of studying gene family evolution in SI genes over comparable systems like the MHC in vertebrates is that linkage of each new variant could be tested by segregation analyses to a known phenotype (Schierup et al., 2001; Mable et al., 2003, 2004; Prigoda et al., 2005). In our study, the low amplification of SRK01, which we otherwise knew from Sanger sequencing based genotyping of the parents should have multiple variants within families, precluded confidence in segregation analyses based only on the 454 data. However, targeted Sanger sequencing for this allele aided in interpretation of the segregation analyses. Unfortunately, as we performed crosses before the 454 sequencing, we could not test linkage of all new variants found to the SI phenotype. It was also not feasible to determine when unlinked alleles were amplified based on the presence of “too many” haplotypes.
Objective 3: Introgression of SRK Alleles
For the population survey, the 454 genotyping identified 22 SRK01 variants. Using targeted amplifications and Sanger sequencing we identified 24 haplotypes. All of these but seven had been found using the 454 pyrosequencing, but including five that matched the 454 sequences but had additional polymorphisms outside of the shared sequence region (indicated by distinct letters after the haplotype name; Supplementary Tables 8, 9). However, only 11 of the 22 variants found by 454 sequencing were confirmed by direct sequencing and there was a higher proportion of PCR positive results among the 454 than the Sanger sequences (Table 4).
Using the diploids as a guide, we identified “arenosa” and “lyrata” specific haplotypes, as well as three that appeared to be recombinants between species-specific variants (haps 7, 8, and 10; Supplementary Data Sheet 3), two of which were identified from a single A4x population that was predicted to be introgressed (A4X_AUT1, from Kerhnoff; Schmickl, 2009). Although analyses using GARD in the HYPHY package did not find statistical evidence for recombination breakpoints, this might have been because of the short tracts of introgression. The minimum spanning network indicated that haps 7 and 8 did in fact fall between species-specific clusters whereas hap10 was on a tip in the A. arenosa part of the network (Figure 4). What is striking is that reticulation in the network involved primarily A. arenosa tetraploids and that diploids had a lower diversity of SRK01 haplotypes compared to tetraploids. There was also some haplotype sharing among tetraploids but not between the diploids. Since the crosses established that individual tetraploids could harbor up to three different SRK01 haplotypes and many were heterozygous for two, this higher diversity among tetraploids could be because SRK01 is effectively neutral and so could accumulate more mutations in tetraploids because of the higher copy number maintained (Mable et al., 2004). Crossing data suggest that SRK is functional in individuals sampled from the hybrid zone (Ruiz-Duarte, 2012), but it is also possible that selection pressure to maintain restricted recombination in the S-locus region (Charlesworth et al., 2006) would be relaxed with the increased copy number in tetraploids. Moreover, introgression of recessive alleles between A. lyrata and A. halleri has been found in diploids (Castric et al., 2010), suggesting that hybridization might disrupt linkage. Although the crosses we performed only included tetraploid A. lyrata from outside of the known hybrid zone, two individuals in one family were self-compatible (Supplementary Table 6). It is thus also possible that increased recombination at the S-locus occurs with spontaneous loss of SI in some individuals.
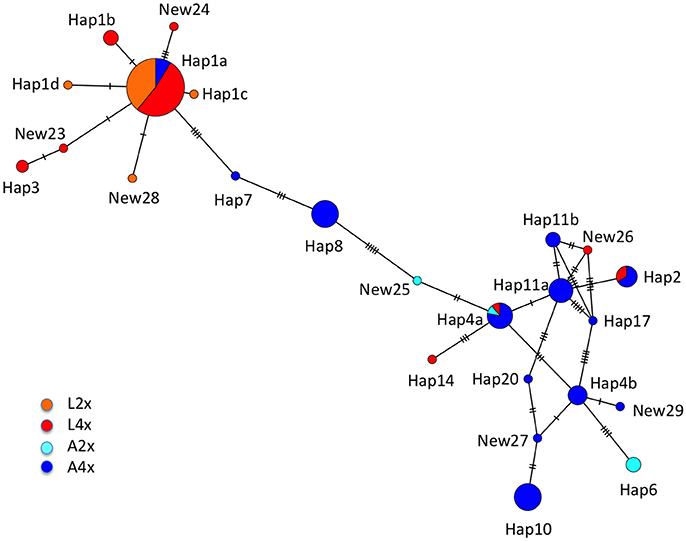
Figure 4. Minimum spanning network for SRK01 haplotypes resolved using direct Sanger sequencing. Circles are drawn proportionately to the frequency of the haplotype and colored by relative frequency in each population type: A2x = light blue; A4x = dark blue; L2x = orange; L4x = red. Vertical bars on the connecting branches indicate the number of nucleotide substitutions separating haplotypes. Haplotypes 7, 8, and 10 were predicted to be recombinants between “arenosa” and “lyrata” specific sequences; haps 7 and 8 appear intermediate between the two clusters whereas hap10 is on a tip in the “arenosa” part of the network. NEW25 also appears intermediate and so could be another introgressed haplotype but it was only found in a single individual. Note that extensive reticulation was found predominantly among sequences found in A4x populations and that there is less variation among haplotypes restricted to diploids than those found in tetraploids.
The presence of A. arenosa like haplotypes in two of the A. lyrata tetraploid populations and the most frequent A. lyrata haplotype (hap1) in most A. arenosa populations from the hybrid zone (Table 4, Supplementary Table 9) could suggest more recent and secondary hybridization while the introgressed haplotypes (i.e., those that appeared to be recombinants between the species-specific variants) could reflect older events. One A. arenosa-like haplotype (hap2) was found in an A. lyrata tetraploid population in the Northeastern Austrian Forealps (L4_AUT4 from Lilienfeld), and in the crosses, which involved individuals from two peripheral A. lyrata populations (L4_AUT2 from Mödling and L4_AUT5 from Rauheneck Ruin, near Baden). This could suggest undetected hybridization within these “pure” populations, as also suggested by whole-genome data (Hohmann and Koch, 2017). While these results fit with expectations based on predicted patterns of hybridization in tetraploid populations from Austria (Schmickl, 2009; Schmickl et al., 2010; Schmickl and Koch, 2011; Muir et al., 2015), there are similar caveats about the use of PCR-based genotyping as raised for the 454 sequences, as described below.
Challenge: PCR Based Approaches to Genotyping
Overall, there was not much consensus between the SRK01 genotypes resolved using 454 and direct sequencing. While the crosses demonstrated that the latter was more sensitive to detect heterozygotes when products were amplified, the population survey revealed a potential bias against amplifying variants found in A2x populations. A much lower proportion of individuals from these populations tested positive than from other populations, and many of the haplotypes found using 454, but not direct sequencing, were from A2x populations. This potential bias reduced the sample sizes that could be used to classify haplotypes showing species-specific presence. In the segregation analyses (Table 5), two individuals had all three SRK01 haplotypes segregating in the parents: one individual didn't show presence of other alleles expected in the parents based on the 454 sequencing but showed some unexpected alleles; the other individual showed more than four expected haplotypes (Supplementary Table 5). Thus, we cannot rule out contamination. Moreover, interpretation of introgressed haplotypes could have been confounded by PCR-based recombination but they were found only in a stabilized hybrid population. Moreover, some haplotypes were only resolved from direct sequences of heterozygotes; in those cases cloning would be required to absolutely confirm the full range of haplotypes present. We had originally intended to also test the utility of other polymorphic members of the gene family (e.g., Aly9); however, since there were even more haplotypes predicted by the 454 sequencing but separated by fewer variants (data not shown), there would have been too much reliance on accurately identifying singletons.
Objective 4: Copy Number Variation in the SRK-Related Gene Family
Clustering of contigs resolved from the de novo assembly approach to genome mining of our database of SRK and its paralogs (i.e., all unique variants found using the 454 pyrosequencing, targeted sequencing of SRK01, cloning of longer products using degenerate primers, and additional sequences available in Genbank) was used to uncover receptor-like kinases from published diploid and tetraploid genome sequences (Supplementary Table 10). This resulted in identification of 1-2 predicted SRK alleles in the diploid and 1-4 in the tetraploid accessions for both species among the 13 short read sets screened (Table 6; Supplementary Table 11). In total 29/177 contigs were assigned as SRK, but 12 of these would have been mis-assigned based only on BLAST (Supplementary Table 10). Aly13-2-like sequences were pulled out in seven accessions, but would have been classified as SRK based only on BLAST (Table 6; Supplementary Table 10). This locus is not present in all individuals, so copy number variation is expected (Mable et al., 2017). Other alleles predicted to be unlinked to the SI phenotype were also resolved by the clustering analysis but none of these would have been assigned as SRK-like based on BLAST (Table 6; Supplementary Table 10). One published allele (AlySRK32) whose phylogenetic position and dominance have not been resolved in previous studies was pulled out from six accessions; based on the length of its branch to other SRK sequences, it has been predicted to be unlinked to the SI phenotype (Tedder et al., 2011). AlySRK47 (found in four of the accessions) is also predicted to be unlinked, based on its phylogenetic position relative to linked sequences. The other four paralogs tested were present in all accessions, except for one diploid A. lyrata that lacked ARK3 (Aly8). Since this latter locus is tightly linked to SRK in some specificities and shows high polymorphism (Kusaba et al., 2001; Charlesworth et al., 2003b; Guo et al., 2011; Vekemans et al., 2014), this could be due to divergence from the reference sequence. AL2G2623090 included sequences similar to both Aly10.1 (ARK1 in A. thaliana) and Aly10.2 (ARK2 in A. thaliana), which were detected in all individuals. Aly10.2 is a suspected pseudogene in A. lyrata due to a large deletion and does not amplify in all individuals (Charlesworth et al., 2003b), whereas Aly10.1 is predicted to be functional and amplifies in more individuals. Clustering suggested that only four individuals had both genes but not all contigs could be resolved due to missing parts of the sequence. AL6G484380 (Aly3) was found in all individuals. Fourteen of the contigs clustered into two distinct clades that did not show similarity to any known paralogs (contig-only clusters; Supplementary Table 10). One was found in 10/13 accessions while the other was only found in four; these could represent previously uncharacterized members of the S-receptor kinase gene family.
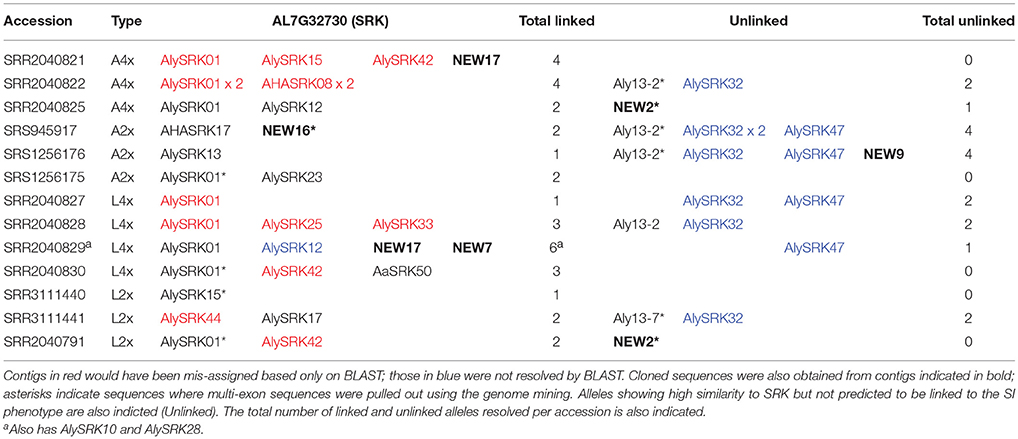
Table 6. Identity of SRK-like (AL7G32730) contigs pulled out by genome mining and confirmed by phylogenetic clustering.
For the SRK sequences, five of the putatively new alleles found by 454 pyrosequencing were pulled out, all of which also were detected by cloning and sequencing using degenerate primers; multi-exon sequences were mined from the genomes for two of them (NEW2, NEW16; Table 6). Multi-exon sequences were also pulled out for AlySRK01, AlySRK15, Aly13-2, and Aly13-7. While the genome mining approach seems promising, the presence of homozygotes for SRK for three individuals suggests that not all SRK alleles were identified within individual genomes: one L2x and one A2x individual had a single dominant allele each (AlySRK15, in dominance Class A2 and AlySRK13 in dominance Class A3, respectively). One L4x individual was homozygous for AlySRK01, which is plausible, as homozygotes for this recessive allele have been found in previous segregation-based analyses of tetraploid A. lyrata from Austria (Mable et al., 2004).
Challenge: Extracting Full-Length Sequences of Polymorphic Genes From Short Read Data
While the genome mining holds promise for investigating copy number variation and obtaining full-length sequences from new alleles, the approach that worked best required a detailed reference database of alleles in order to accurately assign sequences to loci. BLAST analyses alone resulted in mis-assignment of SRK alleles to other paralogs and other paralogs were sometimes assigned as SRK alleles. While part of this was because not all sequences were available in Genbank for BLAST analysis, the gene conversion with unlinked loci that makes similarity alone unreliable (Prigoda et al., 2005) remained problematic in these analyses. For example, Aly13-2/13-7 sequences (which are not linked but are highly similar to Class B SRK alleles) were assigned as SRK in the initial analyses using only the five genes extracted from the MN47 genome. Manual alignments and phylogenetic clustering were required to determine allelic identities and to assign sequences to paralogous loci. However, there were clues in the BLAST analyses that suggested mis-assignment of SRK-like alleles; a signature of high E-value and low score in all cases predicted clustering to SRK-like sequences (although including unlinked loci such as Aly13-2). Nevertheless, the presence of only a single dominant allele in some accessions suggested that the genome mining did not pull out all SRK sequences that should have been present (since homozygotes should only be possible for recessive alleles).
While we had hoped also to be able to use this approach to map the potentially new alleles found using 454 sequencing to genomic regions to predict linkage to the S-locus, the failure of the mapping approach meant that this was not possible. However, when amplifying longer sequences using degenerate primers, we were able to obtain full-length sequences for some of the potentially new specificities predicted from the 454 analyses that we could use to BLAST the de novo assemblies (Table 6).
Conclusions and Recommendations
The results presented here suggest that the highly polymorphic SRK alleles could be useful for interpreting evolutionary patterns of gene flow among populations, species and ploidy levels. We have demonstrated that tetraploids show no apparent advantage in terms of allelic or haplotypic repertoire due to more relaxed selection than diploids but that there is increased evidence for introgression (at least based on the most recessive SRK allele) among tetraploids from suspected hybrid populations. We also demonstrated that following up high throughput genotyping with targeted PCR can help to increase accuracy and completeness. We also identified new alleles not previously characterized and predicted dominance based on phylogenetic clustering.
Nevertheless, there are some important caveats from the analyses, which highlight considerations for future studies based on more robust approaches to high throughput genotyping. We make the following recommendations for future investigations of gene family evolution, in diploids as well as polyploids: (1) applying a hierarchical strategy to filtering decisions for cluster analyses could improve assignment of sequence variants to allelic variants, similar to suggestions for hierarchical AMOVA or STRUCTURE analyses (Holsinger and Mason-Gamer, 1996; Herdegen et al., 2014); (2) amplicon-based approaches for genotyping using deep sequencing should be avoided if there are other options available, as differential amplification and the difficulty of distinguishing PCR errors from real biological processes are difficult to overcome by any current sequencing technology; (3) due to the difficulty of assigning variants to gene copies, interpretation of gene family evolution should always be accompanied by co-segregation of sequence variants with the phenotype, whenever possible; (4) genome mining of resequenced genomes has the potential to investigate copy number variation and obtain full-length sequences that would be useful for population genetics analyses and tests for selection but lack of assembly of highly polymorphic genes to references means that this might only be practical for genes where there is already extensive knowledge about the components of the gene family.
While our results have demonstrated some useful insights into the dynamics of a complex gene family in polyploids and hybrids, we recommend that non-PCR-based sequence capture approaches hold the most promise for assessing patterns of selection on genes under balancing selection, where trans-specific polymorphism, reduced differentiation among alleles, and intermediate frequency alleles are predicted. Such approaches, for example, have been successfully applied to investigating R-gene variation in crop plants (Jupe et al., 2012, 2013; Andolfo et al., 2014; Giolai et al., 2016; Russell et al., 2016; Van Weymers et al., 2016). Whole genome resequencing approaches could be useful for setting the genomic context and fate of duplications, but there are still substantial challenges to resolve in distinguishing loss of copies from lack of coverage or lack of assembly to the reference due to high sequence divergence. A hierarchical approach to filtering or assembly to multiple references (e.g., multiple individuals or multiple alleles or gene family members) could help to overcome such difficulties but resolving fine-scale variation among variants from errors (e.g., haplotypes within specificities) and resolving complete heterozygous genotypes (particularly in polyploids) will require some creative bioinformatic solutions.
Data Availability Statement
The 200 bp fragments generated by 454 sequencing are too short for submission to Genbank but a full alignment of the sequences identified has been provided as Supplementary Data Sheet 1 (including only the 454 sequences and references), 2 (including all unique alleles found across analyses), and 3 (SRK01 sequences). All new Sanger sequences have been deposited to Genbank (Accession numbers: MH507371-MH507400). Accession numbers and details for all unique sequences identified, along with those for reference sequences already available in Genbank are provided in Supplementary Table 12.
Author Contributions
BM wrote the paper and performed the bulk of the sequence analyses described. MJ, AC, and PR-D generated sequence data for objectives 1, 2, and 3, respectively. MJ also performed the crosses for segregation analyses. KL and CK developed bespoke bioinformatics pipelines for objectives 1 and 4, respectively. AB contributed conceptually and financially to objectives 1 and 2. MK contributed conceptually to all aspects of the project and provided samples and advice on sampling locations and interpretation of the hybrid zones. AB, MK, and CK contributed to writing the manuscript.
Funding
This project was funded by a NERC Advanced Research Fellowship (NE/B50094X/1) to BM, and support from the Centre for Ecology and Evolutionary Synthesis (RCN/179569) to AB. Further support for genome resequencing was granted through a DFG grant (DFG SPP 1529; KO2302-14) to MK.
Conflict of Interest Statement
The authors declare that the research was conducted in the absence of any commercial or financial relationships that could be construed as a potential conflict of interest.
Acknowledgments
We thank Aileen Adam and Elizabeth Kilbride for technical assistance.
Supplementary Material
The Supplementary Material for this article can be found online at: https://www.frontiersin.org/articles/10.3389/fevo.2018.00114/full#supplementary-material
References
Adams, K. L. (2007). Evolution of duplicate gene expression in polyploid and hybrid plants. J. Hered. 98, 136–141. doi: 10.1093/jhered/esl061
Aeschlimann, P. B., Häberli, M. A., Reusch, T. B. H., Boehm, T., and Milinski, M. (2003). Female sticklebacks Gasterosteus aculeatus use self-reference to optimize MHC allele number during mate selection. Behav. Ecol. Sociobiol. 54, 119–126. doi: 10.1007/s00265-003-0611-6
Andolfo, G., Jupe, F., Witek, K., Etherington, G. J., Ercolano, M. R., and Jones, J. D. G. (2014). Defining the full tomato NB-LRR resistance gene repertoire using genomic and cDNA RenSeq. BMC Plant Biol. 14:120. doi: 10.1186/1471-2229-14-120
Arnold, B., Kim, S. T., and Bomblies, K. (2015). Single geographic origin of a widespread autotetraploid arabidopsis arenosa lineage followed by interploidy admixture. Mol. Biol. Evol. 32, 1382–1395. doi: 10.1093/molbev/msv089
Bandelt, H. J., Forster, P., and Rohl, A. (1999). Median-joining networks for inferring intraspecific phylogenies. Mol. Biol. Evol. 16, 37–48. doi: 10.1093/oxfordjournals.molbev.a026036
Bechsgaard, J., Hedegaard Jorgensen, T., and Schierup, M. (2017). Evidence for adaptive introgression of disease resistance genes among closely related arabidopsis species. G3 7, 2677–2683. doi: 10.1534/g3.117.043984
Bechsgaard, J. S., Castric, V., Charlesworth, D., Vekemans, X., and Schierup, M. H. (2006). The transition to self-compatibility in Arabidopsis thaliana and evolution within S-haplotypes over 10 Myr. Mol. Biol. Evol. 23, 1741–1750. doi: 10.1093/molbev/msl042
Beckmann, J. S., Estivill, X., and Antonarakis, S. E. (2007). Copy number variants and genetic traits: closer to the resolution of phenotypic to genotypic variability. Nat. Rev. Genet. 8, 639–646. doi: 10.1038/nrg2149
Billiard, S., Castric, V., and Vekemans, X. (2007). A general model to explore complex dominance patterns in plant sporophytic self-incompatibility systems. Genetics 175, 1351–1369. doi: 10.1534/genetics.105.055095
Birchler, J. A., and Veitia, R. A. (2010). The gene balance hypothesis: implications for gene regulation, quantitative traits and evolution. New. Phyt. 186, 54–62. doi: 10.1111/j.1469-8137.2009.03087.x
Blanc, G., and Wolfe, K. H. (2004). Widespread paleopolyploidy in model plant species inferred from age distributions of duplicate genes. Plant Cell 16, 1667–1678. doi: 10.1105/tpc.021345
Boggs, N. A., Dwyer, K. G., Shah, P., McCuuoch, A. A., Bechsgaard, J., Schierup, M. H., et al. (2009). Expression of distinct self-incompatibility specificities in Arabidopsis thaliana. Genetics 182, 1313–1321. doi: 10.1534/genetics.109.102442
Busch, J. W., Sharma, J., and Schoen, D. J. (2008). Molecular characterization of Lal2, an SRK-like gene linked to the S-locus in the wild mustard Leavenworthia alabamica. Genetics 178, 2055–2067. doi: 10.1534/genetics.107.083204
Carlsen, T., Aas, A. B., Lindner, D., Vrålstad, T., Schumacher, T., and Kauserud, H. (2012). Don't make a mista(g)ke: is tag switching an overlooked source of error in amplicon pyrosequencing studies? Fungal Ecol. 5, 747–749. doi: 10.1016/j.funeco.2012.06.003
Castric, V., Bechsgaard, J., Schierup, M. H., and Vekemans, X. (2008). Repeated adaptive introgression at a gene under multiallelic balancing selection. PLoS Genet. 4:e1000168. doi: 10.1371/journal.pgen.1000168
Castric, V., Bechsgaard, J. S., Grenier, S., Noureddine, R., Schierup, M. H., and Vekemans, X. (2010). Molecular evolution within and between self-incompatibility specificities. Mol. Biol. Evol. 27, 11–20. doi: 10.1093/molbev/msp224
Castric, V., and Vekemans, X. (2007). Evolution under strong balancing selection: how many codons determine specificity at the female self-incompatibility gene SRK in Brassicaceae? BMC Evol. Biol. 7:132. doi: 10.1186/1471-2148-7-132
Charlesworth, D., Awadalla, P., Mable, B. K., and Schierup, M. H. (2000). Population-level studies of multiallelic self-incompatibility loci, with particular reference to Brassicaceae. Ann. Bot. 85, 227–239. doi: 10.1006/anbo.1999.1015
Charlesworth, D., Bartolome, C., Schierup, M. H., and Mable, B. K. (2003a). Haplotype structure of the stigmatic self-incompatibility gene in natural populations of Arabidopsis lyrata. Mol. Biol. Evol. 20, 1741–1753. doi: 10.1093/molbev/msg170
Charlesworth, D., Kamau, E., Hagenblad, J., and Tang, C. L. (2006). Trans-specificity at loci near the self-incompatibility loci in Arabidopsis. Genetics 172, 2699–2704. doi: 10.1534/genetics.105.051938
Charlesworth, D., Mable, B. K., Schierup, M. H., Bartolome, C., and Awadalla, P. (2003b). Diversity and linkage of genes in the self-incompatibility gene family in Arabidopsis lyrata. Genetics 164, 1519–1535.
da Fonseca, R. R., Albrechtsen, A., Themudo, G. E., Ramos-Madrigal, J., Sibbesen, J. A., Maretty, L., et al. (2016). Next-generation biology: Sequencing and data analysis approaches for non-model organisms. Mar. Genomics 30, 3–13. doi: 10.1016/j.margen.2016.04.012
D'Amore, R., Ijaz, U. Z., Schirmer, M., Kenny, J. G., Gregory, R., Darby, A. C., et al. (2016). A comprehensive benchmarking study of protocols and sequencing platforms for 16S rRNA community profiling. BMC Gen. 17:55. doi: 10.1186/s12864-015-2194-9
Dart, S., Kron, P., and Mable, B. K. (2004). Characterizing polyploidy in Arabidopsis lyrata using chromosome counts and flow cytometry. Can. J. Bot. 82, 185–197. doi: 10.1139/b03-134
Delport, W., Poon, A. F., Frost, S. D., and Kosakovsky Pond, S. L. (2010). Datamonkey 2010: a suite of phylogenetic analysis tools for evolutionary biology. Bioinformatics 26, 2455–2457. doi: 10.1093/bioinformatics/btq429
Doyle, J. J., and Doyle, J. L. (1987). A rapid DNA isolation procedure for small quantities of fresh leaf tissue. Phytochem.Bull. 19, 11–15.
Duvaux, L., Geissmann, Q., Gharbi, K., Zhou, J. J., Ferrari, J., Smadja, C. M., et al. (2015). Dynamics of copy number variation in host races of the pea aphid. Mol. Biol. Evol. 32, 63–80. doi: 10.1093/molbev/msu266
Evans, B. J. (2007). Ancestry influences the fate of duplicated genes millions of years after polyploidization of clawed frogs (Xenopus). Genetics 176, 1119–1130. doi: 10.1534/genetics.106.069690
Flajnik, M. F., and Kasahara, M. (2010). Origin and evolution of the adaptive immune system: genetic events and selective pressures. Nat. Rev. Genet. 11, 47–59. doi: 10.1038/nrg2703
Force, A., Lynch, M., Pickett, F. B., Amores, A., Yan, Y. L., and Postlethwait, J. (1999). Preservation of duplicate genes by complementary, degenerative mutations. Genetics 151, 1531–1545.
Foxe, J. P., Stift, M., Tedder, A., Haudry, A., Wright, S. I., and Mable, B. K. (2010). Reconstructing origins of loss of self-incompatibility and selfing in North American Arabidopsis lyrata: a population genetic context. Evolution 64, 3495–3510. doi: 10.1111/j.1558-5646.2010.01094.x
Gardiner, L. J., Bansept-Basler, P., Olohan, L., Joynson, R., Brenchley, R., Hall, N., et al. (2016). Mapping-by-sequencing in complex polyploid genomes using genic sequence capture: a case study to map yellow rust resistance in hexaploid wheat. Plant J. 87, 403–419. doi: 10.1111/tpj.13204
Giolai, M., Paajanen, P., Verweij, W., Percival-Alwyn, L., Baker, D., Witek, K., et al. (2016). Targeted capture and sequencing of gene-sized DNA molecules. Biotechniques 61, 315–322. doi: 10.2144/000114484
Goubet, P. M., Berges, H., Bellec, A., Prat, E., Helmstetter, N., Mangenot, S., et al. (2012). Contrasted patterns of molecular evolution in dominant and recessive self-incompatibility haplotypes in Arabidopsis. PLoS Genet. 8:e1002495. doi: 10.1371/journal.pgen.1002495
Gout, J. F., and Lynch, M. (2015). Maintenance and loss of duplicated genes by dosage subfunctionalization. Mol. Biol. Evol. 32, 2141–2148. doi: 10.1093/molbev/msv095
Guggisberg, A., Mansion, G., and Conti, E. (2009). Disentangling reticulate evolution in an arctic-alpine polyploid complex. Syst. Biol. 58, 55–73. doi: 10.1093/sysbio/syp010
Guo, Y. L., Zhao, X., Lanz, C., and Weigel, D. (2011). Evolution of the S-locus region in Arabidopsis relatives. Plant Physiol. 157, 937–946. doi: 10.1104/pp.111.174912
Hatakeyama, K., Watanabe, M., Takasaki, T., Ojima, K., and Hinata, K. (1998). Dominance relationships between S-alleles in self-incompatible Brassica campestris L. Heredity 80, 241–247. doi: 10.1046/j.1365-2540.1998.00295.x
He, Z., Zhang, H., Gao, S., Lercher, M. J., Chen, W. H., and Hu, S. (2016). Evolview v2: an online visualization and management tool for customized and annotated phylogenetic trees. Nucleic Acids Res. 44, W236–W241. doi: 10.1093/nar/gkw370
Herdegen, M., Babik, W., and Radwan, J. (2014). Selective pressures on MHC class II genes in the guppy (Poecilia reticulata) as inferred by hierarchical analysis of population structure. J. Evol. Biol. 27, 2347–2359. doi: 10.1111/jeb.12476
Hermansen, R. A., Hvidsten, T. R., Sandve, S. R., and Liberles, D. A. (2016). Extracting functional trends from whole genome duplication events using comparative genomics. Biol. Proc. Online 18:11. doi: 10.1186/s12575-016-0041-2
Hohmann, N., and Koch, M. A. (2017). An Arabidopsis introgression zone studied at high spatio-temporal resolution: interglacial and multiple genetic contact exemplified using whole nuclear and plastid genomes. BMC Gen. 18:6. doi: 10.1186/s12864-017-4220-6
Hohmann, N., Schmickl, R., Chiang, T. Y., Lu Anova, M., Kola, F., Marhold, K., et al. (2014). Taming the wild: resolving the gene pools of non-model Arabidopsis lineages. BMC Evol. Biol. 14:224. doi: 10.1186/s12862-014-0224-x
Holsinger, K., and Mason-Gamer, R. J. (1996). Hierarchical analysis of nucleotide diversity in geographically structured populations. Genetics 142, 629–639.
Hull, R. M., Cruz, C., Jack, C. V., and Houseley, J. (2017). Environmental change drives accelerated adaptation through stimulated copy number variation. PLoS Biol. 15:e2001333. doi: 10.1371/journal.pbio.2001333
Jørgensen, M. H., Ehrich, D., Schmickl, R., Koch, M. A., and Brysting, A. K. (2011). Interspecific and interploidal gene flow in Central European Arabidopsis (Brassicaceae). BMC Evol. Biol. 11:346. doi: 10.1186/1471-2148-11-346
Jørgensen, M. H., Lagesen, K., Mable, B. K., and Brysting, A. K. (2012). Using high-throughput sequencing to investigate the evolution of self-incompatibility genes in the Brassicaceae: strategies and challenges. Plant Ecol. Divers 5, 473–484. doi: 10.1080/17550874.2012.748098
Jupe, F., Pritchard, L., Etherington, G. J., Mackenzie, K., Cock, P. J., Wright, F., et al. (2012). Identification and localisation of the NB-LRR gene family within the potato genome. BMC Gen. 13:75. doi: 10.1186/1471-2164-13-75
Jupe, F., Witek, K., Verweij, W., Sliwka, J., Pritchard, L., Etherington, G. J., et al. (2013). Resistance gene enrichment sequencing (RenSeq) enables reannotation of the NB-LRR gene family from sequenced plant genomes and rapid mapping of resistance loci in segregating populations. Plant J. 76, 530–544. doi: 10.1111/tpj.12307
Kalbe, M., Eizaguirre, C., Dankert, I., Reusch, T. B. H., Sommerfeld, R. D., and Wegner, K. M. (2009). Lifetime reproductive success is maximized with optimal major histocompatibility complex diversity. Proc. R. Soc. B. 276, 925–934. doi: 10.1098/rspb.2008.1466
Koch, M. A., Wernisch, M., and Schmickl, R. (2008). Arabidopsis thaliana's wild relatives: an updated overview on systematics, taxonomy and evolution. Taxon 57, 933–943.
Krasileva, K. V., Vasquez-Gross, H. A., Howell, T., Bailey, P., Paraiso, F., Clissold, L., et al. (2017). Uncovering hidden variation in polyploid wheat. Proc. Natl. Acad. Sci. U.S.A. 114, E913–E921. doi: 10.1073/pnas.1619268114
Kumar, S., Stecher, G., and Tamura, K. (2016). MEGA7: Molecular evolutionary genetics analysis version 7.0 for bigger datasets. Mol. Biol. Evol. 33, 1870–1874. doi: 10.1093/molbev/msw054
Kusaba, M., Dwyer, K., Hendershot, J., Vrebalov, J., Nasrallah, J. B., and Nasrallah, M. E. (2001). Self-incompatibility in the genus Arabidopsis: characterization of the S locus in the outcrossing A. lyrata and its autogamous relative A. thaliana. Plant Cell 13, 627–643. doi: 10.1105/tpc.13.3.627
Lander, E. S., Linton, L. M., Birren, B., Nusbaum, C., Zody, M. C., Baldwin, J., et al. (2001). Initial sequencing and analysis of the human genome. Nature 409, 860–921. doi: 10.1038/35057062
Leducq, J. B., Gosset, C. C., Gries, R., Calin, K., Schmitt, E., Castric, V., et al. (2014). Self-incompatibility in Brassicaceae: identification and characterization of SRK-like sequences linked to the S-locus in the tribe Biscutelleae. Genes Genom. Genet. 4, 983–992. doi: 10.1534/g3.114.010843
Leigh, J. W., and Bryant, D. (2015). POPART: full-feature software for haplotype network construction. Meth. Ecol. Evol. 6, 1110–1116. doi: 10.1111/2041-210X.12410
Lewis, D. (1947). Competition and dominance of incompatibility alleles in diploid pollen. Heredity 1, 85–108. doi: 10.1038/hdy.1947.5
Li, H., and Durbin, R. (2009). Fast and accurate short read alignment with Burrows-Wheeler transform. Bioinformatics 25, 1754–1760. doi: 10.1093/bioinformatics/btp324
Llaurens, V., Billiard, S., Castric, V., and Vekemans, X. (2009). Evolution of dominance in sporophytic self-incompatibility systems: I. Genetic load and coevolution of levels of dominance in pollen and pistil. Evolution 63, 2427–2437. doi: 10.1111/j.1558-5646.2009.00709.x
Llaurens, V., Billiard, S., Leducq, J. B., Castric, V., Klein, E. K., and Vekemans, X. (2008). Does frequency-dependent selection with complex dominance interactions accurately predict allelic frequencies at the self-incompatibility locus in Arabidopsis halleri? Evolution 62, 2545–2557. doi: 10.1111/j.1558-5646.2008.00469.x
Luikart, G., Allendorf, F. W., Cornuet, J. M., and Sherwin, W. B. (1998). Distortion of allele frequency distributions provides a test for recent population bottlenecks. J. Hered. 89, 238–247. doi: 10.1093/jhered/89.3.238
Mable, B. K. (2013). Polyploids and hybrids in changing environments: winners or losers in the struggle for adaptation? Heredity 110, 95–96. doi: 10.1038/hdy.2012.105
Mable, B. K., and Adam, A. (2007). Patterns of genetic diversity in outcrossing and selfing populations of Arabidopsis lyrata. Mol. Ecol. 16, 3565–3580. doi: 10.1111/j.1365-294X.2007.03416.x
Mable, B. K., Beland, J., and Di Berardo, C. (2004). Inheritance and dominance of self-incompatibility alleles in polyploid Arabidopsis lyrata. Heredity 93, 476–486. doi: 10.1038/sj.hdy.6800526
Mable, B. K., Hagmann, J., Kim, S. T., Adam, A., Kilbride, E., Weigel, D., et al. (2017). What causes mating system shifts in plants? Arabidopsis lyrata as a case study. Heredity 118, 52–63. doi: 10.1038/hdy.2016.99
Mable, B. K., Kilbride, E., Viney, M. E., and Tinsley, R. C. (2015). Copy number variation and genetic diversity of MHC Class IIb alleles in an alien population of Xenopus laevis. Immunogenetics 67, 591–603. doi: 10.1007/s00251-015-0860-3
Mable, B. K., Schierup, M. H., and Charlesworth, D. (2003). Estimating the number, frequency, and dominance of S-alleles in a natural population of Arabidopsis lyrata (Brassicaceae) with sporophytic control of self-incompatibility. Heredity 90, 422–431. doi: 10.1038/sj.hdy.6800261
Maddison, D. R., and Maddison, W. P. (2000). Macclade 4: Analysis Of Phylogeny And Character Evolution, Version 4.0 edn. Sunderland, MA: Sinauer Associates.
Martin, M. (2011). Cutadapt removes adapter sequences from high-throughput sequencing reads. EMBnet J. 2011:17. doi: 10.14806/ej.17.1.200
Mattenberger, F., Sabater-Munoz, B., Toft, C., Sablok, G., and Fares, M. A. (2017). Expression properties exhibit correlated patterns with the fate of duplicated genes, their divergence, and transcriptional plasticity in Saccharomycotina. DNA Res 24, 559–570. doi: 10.1093/dnares/dsx025
Meyer, A., and Van de Peer, Y. (2005). From 2R to 3R: evidence for a fish-specific genome duplication (FSGD). Bioessays 27, 937–945. doi: 10.1002/bies.20293
Muir, G., Ruiz-Duarte, P., Hohmann, N., Mable, B. K., Novikova, P., Schmickl, R., et al. (2015). Exogenous selection rather than cytonuclear incompatibilities shapes asymmetrical fitness of reciprocal Arabidopsis hybrids. Ecol. Evol. 5, 1734–1745. doi: 10.1002/ece3.1474
Novikova, P. Y., Hohmann, N., Nizhynska, V., Tsuchimatsu, T., Ali, J., Muir, G., et al. (2016). Sequencing of the genus Arabidopsis identifies a complex history of nonbifurcating speciation and abundant trans-specific polymorphism. Nat. Genet. 48, 1077–1082. doi: 10.1038/ng.3617
Paetsch, M., Mayland-Quellhorst, S., and Neuffer, B. (2006). Evolution of the self-incompatibility system in the Brassicaceae: identification of S-locus receptor kinase (SRK) in self-incompatible Capsella grandiflora. Heredity 97, 283–290. doi: 10.1038/sj.hdy.6800854
Pond, K. S. L., Frost, S. D. W., and Muse, S. V. (2005). HyPhy: hypothesis testing using phylogenies. Bioinformatics 21, 676–679. doi: 10.1093/bioinformatics/bti079
Pond, S. L. K., Posada, D., Gravenor, M. B., Woelk, C. H., and Frost, S. D. W. (2006). GARD: a genetic algorithm for recombination detection. Bioinformatics 22, 3096–3098. doi: 10.1093/bioinformatics/btl474
Prigoda, N. L., Nassuth, A., and Mable, B. K (2005). Phenotypic and genotypic expression of self-incompatibility haplotypes in Arabidopsis lyrata suggests unique origin of alleles in different dominance classes. Mol. Biol. Evol. 22, 1609–1620. doi: 10.1093/molbev/msi153
Rambaut, A. (1996). Se-Al: Sequence Alignment Editor, Version 1.0 Alpha1. Distributed Over the World Wide Web. Available online at: http://tree.bio.ed.ac.uk/software/seal/
Reusch, T. B. H., Haberli, M. A., Aeschlimann, P. B., and Milinski, M. (2001). Female sticklebacks count alleles in a strategy of sexual selection explaining MHC polymorphism. Nature 414, 300–302. doi: 10.1038/35104547
Rodrigo, G., and Fares, M. A. (2018). Intrinsic adaptive value and early fate of gene duplication revealed by a bottom-up approach. Elife 7:29739. doi: 10.7554/eLife.29739
Ruiz-Duarte, P. (2012). Self Incompatibility Alleles In Wild Relatives Of Arabidopsis Thaliana. PhD thesis, University of Heidelberg, Heidelberg.
Russell, J., Mascher, M., Dawson, I. K., Kyriakidis, S., Calixto, C., Freund, F., et al. (2016). Exome sequencing of geographically diverse barley landraces and wild relatives gives insights into environmental adaptation. Nat. Genet. 48, 1024–1030. doi: 10.1038/ng.3612
Saintenac, C., Jiang, D. Y., and Akhunov, E. D. (2011). Targeted analysis of nucleotide and copy number variation by exon capture in allotetraploid wheat genome. Genome Biol. 12:r88. doi: 10.1186/gb-2011-12-9-r88
Salmon, A., Udall, J. A., Jeddeloh, J. A., and Wendel, J. (2012). Targeted capture of homoeologous coding and noncoding sequence in polyploid cotton. Genes Genom. Genet. 2, 921–930. doi: 10.1534/g3.112.003392
Schierup, M. H., Mable, B. K., Awadalla, P., and Charlesworth, D. (2001). Identification and characterization of a polymorphic receptor kinase gene linked to the self-incompatibility locus of Arabidopsis lyrata. Genetics 158, 387–399.
Schierup, M. H., Vekemans, X., and Christiansen, F. B. (1998). Allelic geneologies in sporophytic self-incompatibility systems in plants. Genetics 150, 1187–1198.
Schirmer, M., D'Amore, R., Ijaz, U. Z., Hall, N., and Quince, C. (2016). Illumina error profiles: resolving fine-scale variation in metagenomic sequencing data. BMC Bioinformatics 17:976. doi: 10.1186/s12859-016-0976-y
Schirmer, M., Ijaz, U. Z., D'Amore, R., Hall, N., Sloan, W. T., and Quince, C. (2015). Insight into biases and sequencing errors for amplicon sequencing with the Illumina MiSeq platform. Nucleic Acids Res. 43:1341. doi: 10.1093/nar/gku1341
Schmickl, R. E. (2009). Reticulate Evolution in Glacial Refuge Areas-the Genus Arabidopsis in the Eastern Austrian Danube Valley (Wachau). PhD thesis, Ruperto-Carolo University of Heidelberg.
Schmickl, R., Jørgensen, M. H., Brysting, A. K., and Koch, M. A. (2010). The evolutionary history of the Arabidopsis lyrata complex: a hybrid in the amphi-Beringian area closes a large distribution gap and builds up a genetic barrier. BMC Evol. Biol. 10:98. doi: 10.1186/1471-2148-10-98
Schmickl, R., and Koch, M. A. (2011). Arabidopsis hybrid speciation processes. Proc. Natl. Acad. Sci. U.S.A. 108, 14192–14197. doi: 10.1073/pnas.1104212108
Schoen, D. J., and Busch, J. W. (2009). The evolution of dominance in sporophytic self-incompatibility systems. II. Mate availability and recombination. Evolution 63, 2099–2113. doi: 10.1111/j.1558-5646.2009.00686.x
Sedlazeck, F. J., Rescheneder, P., and von Haeseler, A. (2013). NextGenMap: fast and accurate read mapping in highly polymorphic genomes. Bioinformatics 29, 2790–2791. doi: 10.1093/bioinformatics/btt468
Seeb, J. E., Carvalho, G., Hauser, L., Naish, K., Roberts, S., and Seeb, L. W. (2011). Single-nucleotide polymorphism (SNP) discovery and applications of SNP genotyping in nonmodel organisms. Mol. Ecol. Resour. 11, 1–8. doi: 10.1111/j.1755-0998.2010.02979.x
Seoighe, C., and Gehring, C. (2004). Genome duplication led to highly selective expansion of the Arabidopsis thaliana proteome. Trends Genet. 20, 461–464. doi: 10.1016/j.tig.2004.07.008
Shiba, H., Iwano, M., Entani, T., Ishimoto, K., Shimosato, H., Che, F. S., et al. (2002). The dominance of alleles controlling self-incompatibility in Brassica pollen in regulated at the RNA level. Plant Cell 14, 491–504. doi: 10.1105/tpc.010378
Sievers, F., Wilm, A., Dineen, D., Gibson, T. J., Karplus, K., Li, W., et al. (2011). Fast, scalable generation of high-quality protein multiple sequence alignments using Clustal Omega. Mol. Sys. Biol. 7:539. doi: 10.1038/msb.2011.75
Lien, S., Koop, B. F., Sandve, S. R., Miller, J. R., Kent, M. P., Nome, T., et al. (2016). The Atlantic salmon genome provides insights into rediploidization. Nature 533, 200–205. doi: 10.1038/nature17164
Soltis, P. S., Doyle, J., and Soltis, D. E. (eds.). (1992). “Molecular data and polyploid evolution in plants,” in Molecular Systematics of Plants (New York, NY: Chapman and Hall), 177–201.
Stevens, J. P., and Kay, Q. O. N. (1989). The number, dominance relationships and frequencies of self-incompatibility alleles in a natural population of Sinapis arvensis L. in South Wales. Heredity 62, 199–205. doi: 10.1038/hdy.1989.29
Stoeckel, S., Castric, V., Mariette, S., and Vekemans, X. (2008). Unequal allelic frequencies at the self-incompatibility locus within local populations of Prunus avium L.: an effect of population structure? J. Evol. Biol. 21, 889–900. doi: 10.1111/j.1420-9101.2008.01504.x
Takahata, N. (1990). A simple genealogical structure of strongly balanced allelic lines and trans-specific evolution of polymorphism. Proc. Natl. Acad. Sci. U.S.A. 87, 2419–2423.
Tedder, A., Ansell, S. W., Lao, X., Vogel, J. C., and Mable, B. K. (2011). Sporophytic self-incompatibility genes and mating system variation in Arabis alpina. Ann. Bot. 108, 699–713. doi: 10.1093/aob/mcr157
Van de Peer, Y., Mizrachi, E., and Marchal, K. (2017). The evolutionary significance of polyploidy. Nat. Rev. Genet. 18, 411–424. doi: 10.1038/nrg.2017.26
van Orsouw, N. J., Hogers, R. C. J., Janssen, A., Yalcin, F., Snoeijers, S., Verstege, E., et al. (2007). Complexity reduction of polymorphic sequences (CRoPS™): A novel approach for large-scale polymorphism discovery in complex genomes. PLoS ONE 2:e1172. doi: 10.1371/journal.pone.0001172
Van Weymers, P. S. M., Baker, K., Chen, X., Harrower, B., Cooke, D. E. L., Gilroy, E. M., et al. (2016). Utilizing “omic” yechnologies to identify and prioritize novel sources of resistance to the oomycete pathogen Phytophthora infestans in potato germplasm collections. Front. Plant Sci. 7:672. doi: 10.3389/fpls.2016.00672
Vekemans, X., Leducq, J. B., Llaurens, V., Castric, V., Saumitou-Laprade, P., and Hardy, O. J. (2011). Effect of balancing selection on spatial genetic structure within populations: theoretical investigations on the self-incompatibility locus and empirical studies in Arabidopsis halleri. Heredity 106, 319–329. doi: 10.1038/hdy.2010.68
Vekemans, X., Poux, C., Goubet, P. M., and Castric, V. (2014). The evolution of selfing from outcrossing ancestors in Brassicaceae: what have we learned from variation at the S-locus? J. Evol. Biol. 27, 1372–1385. doi: 10.1111/jeb.12372
Wegner, K. M., Kalbe, M., Kurtz, J., Reusch, T. B. H., and Milinski, M. (2003). Parasite selection for immunogenic optimality. Science 301:1343. doi: 10.1126/science.1088293
Wheeler, D. A., Srinivasan, M., Egholm, M., Shen, Y., Chen, L., McGuire, A., et al. (2008). The complete genome of an individual by massively parallel DNA sequencing. Nature 452, 872–U875. doi: 10.1038/nature06884
Wolfe, K. H., and Shields, D. C (1997). Molecular evidence for an ancient duplication of the entire yeast genome. Nature 387, 708–713. doi: 10.1038/42711
Xing, S. L., Li, M. Y., and Liu, P. (2013). Evolution of S-domain receptor-like kinases in land plants and origination of S-locus receptor kinases in Brassicaceae. BMC Evol. Biol. 13:69. doi: 10.1186/1471-2148-13-69
Keywords: polyploidy, gene family evolution, self-incompatibility, copy number variation, trans-specific polymorphism, balancing selection, introgression
Citation: Mable BK, Brysting AK, Jørgensen MH, Carbonell AKZ, Kiefer C, Ruiz-Duarte P, Lagesen K and Koch MA (2018) Adding Complexity to Complexity: Gene Family Evolution in Polyploids. Front. Ecol. Evol. 6:114. doi: 10.3389/fevo.2018.00114
Received: 29 March 2018; Accepted: 17 July 2018;
Published: 07 August 2018.
Edited by:
Richard John Abbott, University of St Andrews, United KingdomReviewed by:
Céline Poux, Université de Lille, FranceBaocheng Guo, Institute of Zoology (CAS), China
Copyright © 2018 Mable, Brysting, Jørgensen, Carbonell, Kiefer, Ruiz-Duarte, Lagesen and Koch. This is an open-access article distributed under the terms of the Creative Commons Attribution License (CC BY). The use, distribution or reproduction in other forums is permitted, provided the original author(s) and the copyright owner(s) are credited and that the original publication in this journal is cited, in accordance with accepted academic practice. No use, distribution or reproduction is permitted which does not comply with these terms.
*Correspondence: Barbara K. Mable, YmFyYmFyYS5tYWJsZUBnbGFzZ293LmFjLnVr