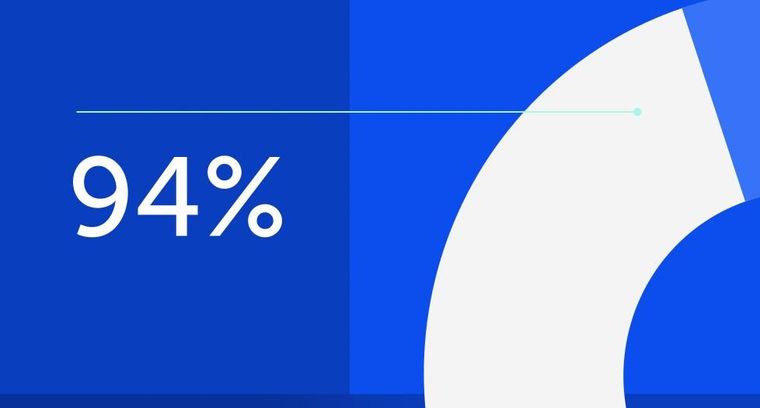
94% of researchers rate our articles as excellent or good
Learn more about the work of our research integrity team to safeguard the quality of each article we publish.
Find out more
METHODS article
Front. Environ. Sci., 15 April 2020
Sec. Soil Processes
Volume 8 - 2020 | https://doi.org/10.3389/fenvs.2020.00042
Soil is a heterogeneous mixture of various organic and inorganic parent materials. Major soil functions are driven by their quality, quantity and spatial arrangement, resulting in soil structure. Physical protection of organic matter (OM) in this soil structure is considered as a vital mechanism for stabilizing organic carbon turnover, an important soil function in times of climate change. Herein, we present a technique for the correlative analysis of 2D imaging visible light near-infrared spectroscopy and 3D X-ray computed microtomography (μCT) to investigate the interplay of biogeochemical properties and soil structure in undisturbed soil samples. Samples from the same substrate but different soil management and depth (no-tilled topsoil, tilled topsoil and subsoil) were compared in order to evaluate this method in a diversely structured soil. Imaging spectroscopy is generally used to qualitatively and quantitatively identify OM with high spatial resolution, whereas 3D X-ray μCT provides high-resolution information on pore characteristics. The unique combination of these techniques revealed that, in undisturbed samples, OM can be found mainly at greater distances from macropores and close to biopores. However, alterations were observed because of disturbances by tillage. The correlative application of imaging infrared spectroscopic and X-ray μCT analysis provided new insights into the biochemical processes affected by soil structural changes.
Soils are heterogeneous and complex mixtures of organic matter (OM), mineral particles and pore space. Their inherent functionality emerges from the spatial arrangement of these constituents on various spatial scales (Ritz et al., 2004; Portell et al., 2018; Wanzek et al., 2018). Important soil physical properties and processes such as conductivity, water retention, gas exchange and root penetration are defined by soil structure, i.e., the spatial arrangement of solids and voids (Bronick and Lal, 2005; Rabot et al., 2018). The interplay of soil chemical and biological properties through physical protection has been considered a key factor in stabilizing soil carbon (Dungait et al., 2012; Mueller et al., 2012; Stockmann et al., 2013; Wiesmeier et al., 2019). This, in turn, is influenced by soil structure, which defines the microenvironmental conditions for microbial carbon turnover. OM is not evenly distributed in soils. For instance, the walls of biopores have been often reported as OM rich compared with bulk soil (Banfield et al., 2017; Hoang et al., 2017; Hobley et al., 2018). It is assumed that the heterogeneous location of OM in soil may be a significant factor controlling the extent of OM mineralization (Dungait et al., 2012; Steffens et al., 2017). Therefore, to understand the relationship between soil structure, carbon dynamics and biogeochemical processes, it is crucial to characterize undisturbed soil samples. However, so far this relationship is often investigated by techniques based on soil disturbance, e.g., by extracting aggregate fractions (Young et al., 2008) from the bulk soil, which indeed provides insights into microscale processes, but the complete image of the soil architecture is lost (Baveye et al., 2018).
Visible light near-infrared spectroscopy (Vis-NIR) is an established method for the simultaneous analysis of various soil properties (Stenberg et al., 2010; Soriano-Disla et al., 2014). However, soil samples have to be ground and homogenized for optimum performance. Imaging Vis-NIR (imVNIR) is an emerging technique that allows the spatial analyses of intact soil samples (Buddenbaum and Steffens, 2011; Steffens and Buddenbaum, 2013). Steffens and Buddenbaum (2013), Steffens et al. (2014) and Hobley et al. (2018) demonstrated the potential of imVNIR by improving our understanding in soil classification, chemical composition and carbon storage on the micrometer scale for whole pedons.
The combinatory application of 2D and 3D imaging of chemical composition and soil structure, respectively, may provide new insights for the physical properties and the spatial extent of soil structures along with their chemical information. X-ray computed microtomography (X-ray μCT) is nowadays considered as a commonly used technique for describing soil structure with physical parameters such as pore size distribution and connectivity (Rabot et al., 2018). As 2D imVNIR and 3D X-ray μCT give different but corroborating information on the same material, the combination of the two techniques can therefore be used to uncover and quantify biogeochemical processes in intact soil samples. The combination of different biogeochemical imaging methods, designated as correlative microscopy or correlative imaging, is increasingly used in life sciences (Caplan et al., 2011; Handschuh et al., 2013; Guyader et al., 2018). A few recent applications have demonstrated that there is a great potential for the application of correlative imaging in soil sciences (Hapca et al., 2011; Juyal et al., 2019; Kravchenko et al., 2019; Schlüter et al., 2019). Schlüter et al. (2019) highlighted that a combination of different 2D techniques for chemical and microbial imaging, such as scanning electron microscope-energy dispersive using X-ray (SEM-EDX) analysis and nanoscale secondary ion mass spectrometry (nanoSIMS) or fluorescence microscopy and X-ray μCT, can be used to reveal specific soil microenvironments and thus biogeochemical and physical processes in structured soil. Compared with these 2D techniques, the spatial resolution of Vis-NIR imaging spectroscopy is lower (several μm instead of nm) but allows the investigation of larger samples up to a complete pedon. Therefore, adding imVNIR to the toolbox of correlative analyses of 2D imaging techniques and 3D X-ray μCT for fast imaging of several samples may reveal patterns of soil biochemical processes at the pedon level of intact soils.
In this study, we developed a procedure for the correlative image analysis of 2D imVNIR and 3D X-ray CT on intact soil cores. Our approach consisted of X-ray μCT scanning, followed by impregnation with resin, slicing, imVNIR and classification of the same undisturbed soil core sample. After image registration, the classified imVNIR images and the X-ray μCT images were analyzed with correlative image analysis, e.g., by analyzing the OM distribution as a function of distance to pores and biopores. We used cores sampled from a space-for-time reclaimed chronosequence. These displayed both structural and chemical differences within the same substrate (Lucas et al., 2019; Pihlap et al., 2019) and thus provided perfect conditions for validating the correlation analysis method on undisturbed field samples.
Intact soil cores were obtained from reclaimed soils in the Garzweiler open pit mine area, 40 km west of Cologne (Germany). A detailed site characterization together with a description of the reclamation procedure and crop rotation of the space-for-time chronosequence is described in the study of Pihlap et al. (2019). Briefly, an approx. 20-meter-thick layer of loess, including approx. 2.2 m of developed Luvisol, was excavated during coal mining, mixed to afford a Luvisol/loess ratio of 1:10 and deposited. This mixture was used for reclamation with alfalfa (Medicago sativa) in a pioneering phase for 3 years. Between the 4th and 6th year, the reclamation was based on a crop rotation including Triticum aestivum L. (wheat) and Hordeum vulgare L. (barley). In the years after, a variation of T. aestivum L. (winter wheat and summer wheat), H. vulgare L. (winter barley), Brassica napus L. (rapeseed) and Zea mays L. (maize) were grown. About 30 t/ha of organic fertilizer (compost or manure) were added in the 4th and 7th year of the reclamation procedure.
Three cores with different soil structures were selected on the basis of the study of Lucas et al. (2019) for correlative analysis with imVNIR, representing different soil development levels (3, 6, and 12 years after reclamation) and management stages (no tillage/tillage) (Table 1). The selected undisturbed soil cores (height 20 cm and diameter 10 cm) were obtained from fields with different ages, in depths of 0–20 and 40–60 cm, using a custom-made drill for undisturbed sampling of cylindrical soil cores (UGT GmbH, Germany). To overcome the trade-off between resolution and sample size, three subsamples of 3 cm diameter and 3 cm height were collected per core.
The no-tilled topsoil is an immature soil with low OM content and dense soil structure that was not affected by soil tillage. After 6 years of reclamation, the tilled topsoil was characterized by high macroporosity due to plowing and high OM content owing to the organic fertilizer amendment. The subsoil represents a sample with many macropores due to high biological activity, namely, high biopore density, which developed for 12 years under a tilled topsoil.
The cylindrical subsamples with a diameter of 3 cm were scanned using an X-ray microtomograph (X-TEk XCT 225, Nikon 162 Metrology). Reconstruction was achieved with a spatial resolution of 19 μm, and the images were processed and segmented into solids and pores, as described in detail by Lucas et al. (2019). Specifically, all biopores were segmented in the binary images using the Tubeness plugin in Fiji to separate all tubular objects. All biopores, including filled biopores with roots or earthworm cast, were separated from the remaining, irregularly shaped, pore network in the binary images. Biopores in the resulting images were therefore empty, and the rough pore wall was excluded. These biopores were dilated five times in all 3D directions (kernel diameter of 3 voxels), and then the image of the segmented pores was subtracted in Fiji. This allowed us to include the walls of the biopores and solids, like earthworm cast within the biopores, to the resulting image.
After X-ray μCT analysis, the same intact soil cylinders were dehydrated gradually with acetone [series from 30–100% (v/v)], and subsequently, the soil cores were impregnated with polyester resin (PALATAL P 6-01, BÜFA, Germany). After 5 weeks of polymerization, the cores were placed in an oven at 40°C for 48 h to cure the surface of the impregnated samples. All cores were cut in two slices of 1 cm thickness, polished and scanned with a hyperspectral camera (VNIR-1800, Norsk Elektro Optikk Ås, Norway). The camera lens distance from the sample surface was set to approx. 30 cm, and the sample was illuminated with two light sources in front of and behind the camera (angle about 45°). An image of 53 × 53 μm2/pixel (1800 pixel/line) and spectral range with 196 bands between 400 and 990 nm was thus obtained. A certified reflectance standard (reflectance 50%) was adopted and used to normalize the reflectance on the target image in order to adjust the differences in illumination:
where Lobj is the radiance of the recorded sample, Lref the radiance of the certified reflectance standard and ρref the reflectance of the certified reflectance standard (Peddle et al., 2001; Steffens and Buddenbaum, 2013)
Hyperspectral images were processed in ENVI Classic (Version 5.2, Exelis Visual Information Solutions, Boulder, Colorado, United States) following the workflow in Figure 1. In particular, we used a principal component analysis (PCA) to concentrate the spectral information in images and remove the correlation between neighboring bands (Rodarmel and Shan, 2002; Kavzoglu et al., 2018). PCA images show spectral spatial variability of different components on the soil surface and helps us to define number of endmembers (classes) (Steffens et al., 2014). Each endmember represents pure spectra from a selected component (class) and it is a basis for determining the composition of each pixel with linear spectral unmixing (LSU). The first 10 principle components were used to separate polyester resin and pores filled with polyester from the soil matrix and to identify pure spectral endmembers of observed components for the subsequent image processing steps. The main criteria for the endmember collection were to select the most abundant features (from PCA analyze) and to cover pixels from different spatial segments in the image. According to the PCA analyze in total we selected six different components as endmembers, such as loess parent material, OM particles, Fe-oxide (FeOx) dominated pixels and iron-manganese concretions. For the tilled topsoil and subsoil, two additional endmembers were selected because of their specific features. The tilled topsoil was affected by plowing, which created voids on top of the loess. As a polyester resin is a transparent material, in tilled topsoil we captured also a reflectance “noise” from the loess that was underneath the shallow tillage void. This gave different reflectance than the voids created by roots and earthworms extending vertically through the entire sample. Thus, additional endmembers for tillage voids were necessary. An additional endmember was also selected from the subsoil to describe fresh, OM-rich pixels, which displayed the most defined OM spectrum compared with that of other OM-dominated areas. The statistical significance of the selected endmembers was tested with the Jeffries–Matusita distance test (Dabboor et al., 2014). For detecting and mapping the abundance of each spectral endmember, LSU was conducted using the manually selected endmembers (Supplementary Figure 1). LSU generates abundance maps, where the relative contribution of the selected endmembers to each pixel is depicted (Ravel et al., 2018). The obtained abundance maps (Supplementary Figures 2–4) were subsequently used for a supervised classification using maximum likelihood classifier (Borra et al., 2019). For the supervised classification, new additional ROIs were selected along with the Jeffries–Matusita distance evaluation. The subsequent area coverage of the classes was calculated in the classified images. Maximum likelihood classification is created by results of the abundancy maps (LSU), of which we know every class contribution to every pixel in 2D space. This means that for example a pixel classified as Fe is dominated by iron, but may also contain fractions of other endmembers. The accuracy and the kappa coefficient of the classification were tested with a confusion matrix processed in ENVI Classic software. For the confusion matrix we selected random pixels that were not part of the ROIs used for the classification itself and compared them with the classification results. The kappa coefficient is a basis to estimate the agreement of classification and it is computed in the ENVI Classic software as follows (L3Harris Geospatial Solutions, 2020):
Figure 1. Workflow of imVNIR image processing. After X-ray μCT analysis, the intact soil cylinders impregnated with polyester resin were cut in two slices of 1 cm thickness. These slices were scanned with a hyperspectral camera. In the first image processing step, scanned images were used to compute a principal component analysis (PCA), which concentrates the spectral information on images and removes correlations between neighboring bands. Principle components were used to separate polyester resin, pores filled with polyester from the soil matrix, and to identify pure spectral endmembers of observed components. In the example of the workflow, we selected five different classes as endmembers (ROI-s) and they were used to calculate relative abundance in each pixel with linear spectral unmixing (LSU). With LSU, abundance maps of each endmember is created, where the most intensive color refers to the highest contribution of selected endmember to the pixel. Obtained abundance maps were subsequently used for a supervised classification using maximum likelihood, and the area coverage of each class can be calculated.
where i is the number of classes, N is the total number of classified values compared to truth values, mi,i is the number of values that belongs to the truth class i and have also been classified as class i, Ci is the total number of predicted values belonging to class i, Gi is the total number of truth values belonging to class i.
The images obtained from X-ray μCT and imVNIR had different resolution and orientation. To overcome these constraints, an image registration was needed prior to correlative analysis. The aim of the registration is to find a geometric transformation to map the pixels of the first (moving) image into the second (target) image. Therefore, 2D/3D image registration was performed using the elastix software (Klein et al., 2010; Shamonin et al., 2013) along with a protocol similar to that described by Schlüter et al. (2019). In the current study, the moving image was always the filtered X-ray CT image and the imVNIR image was the target image. Both images were used as gray scale images, however, the information transported by a certain gray scale value differs with the source. We also used a combination of two metrics, the mean of the Euclidean distance between the corresponding landmark points and the mutual information criterion (Mattes et al., 2001). The mutual information is a statistical measure of the degree of dependences of two random variables A and B. It is closely related to the entropy, i.e., the amount of uncertainty, of the two variables and hence also to the joint entropy. More precisely, this means it quantifies the “amount of information” given by the random variable A about the variable B and vice versa (Maes et al., 1997). Here, it defines the objective function that has to be optimized and such allows the entropy to be matched with the corresponding image pairs For example, homogenous features, such as macropores in μCT images, should be matched with homogenous features visible in imVNIR (Figure 2). A second metric, which uses landmarks (at least three manually selected points), was also added. This ensured that the optimization of the moving image did not result in local minima. A pyramid schedule was used to achieve a fast registration, starting with a coarse resolution and moving toward the next finer scale.
Figure 2. Principle of the image registration. (A) 2D section of the 3D μCT image (gray) and a 2D imVNIR slice were chosen as a registration example with the software elastix. They were not aligned on each other in the beginning of the registration [(A), left]. Through different iterations, the μCT image was transformed so that both images match at each position [(A), right]. For this, the two metrics Euclidean distance and mutual information were used for optimization. The Euclidean distance metric aims to minimize the distance between the manually set points (red crosses). In this example, it is minimized from a mean of 237 to 4 pixel. The second metric represents the extent of mutual information between the registered μCT image and the imVNIR image, i.e., it measures the “amount of information” given by the μCT image about the imVNIR and vice versa. The scatter plots of gray values from imVNIR and CT (B) at the beginning of the registration reveals two clusters, which are formed by the μCT image (pores and matrix) but are not shared by the corresponding imVNIR pixel values. This results in a low mutual information of 0.19. After the final iteration, pore and matrix clusters were formed by both variables, which is also reflected by the isolines showing high kernel densities. Thus at the end of the registration mutual information is high (0.86).
The best results were obtained by using a similarity transform, defined firstly by the objective function of the two metrics. Afterwards, the resulting parameters were used as the initial transform for affine transformation, for which only one metric was used to define the objective function. A first rough registration was thus performed and was mainly guided by the landmark points, and a second step, using only the mutual information metric, resulted in a more accurate result that did not rely on manually set points. Additionally, the similarity transformation involved rotation, translation and scaling and therefore had 7 degrees of freedom in 3D. Instead, the affine transformation added aspect ratio and shear to the allowed transformation, resulting in 12 degrees of freedom in 3D (Klein et al., 2010). Corresponding elastix script and example images can be found in the Supplementary Material.
X-ray μCT analysis allowed the characterization and the localization of pores >38 μm (2 pixel), whereas imVNIR enabled the determination of the soil’s chemical composition. From imVNIR we derived OM and FeOx abundance maps obtained with LSU, whereas from CT images we used the binary (pore) images and the images of Euclidean distances to pores and biopores. The Euclidean distance transformation (EDT) in Fiji was computed to obtain the straight-line distance of each pixel to the next pore/biopore in 3D. In order to compare the data from the two sources (correlative image analyses) the CT images were transformed with transformix, a subroutine of elastix, using the transformation parameters of the original CT image to assure that the images match the corresponding imVNIR image (see section “Image Registration”). Thereafter, multichannel images of the Euclidean distances, pores and results of LSU were created in Fiji, from which we could calculate the relative contribution of OM-rich pixels (obtained with LSU) with distance to pores or biopores (obtained with EDT). In addition, the solid and pore volume was also measured with distance to biopores. This made possible to take into account density shifts around biopores by normalizing the relative contribution of OM-rich pixels by the solid volume fraction. Only distances with a certain number of pixels (100), equal to an area of about 0.3 cm2, were taken into account, to avoid high standard deviation for smaller quantities. It should be noted that correlative imaging does not necessarily include correlation in a statistical sense.
Figure 3 displays the maximum likelihood classification images for the three soil cores and the respective coverage of the five endmembers. The maximum likelihood classification was conducted through relative abundance of each component obtained from LSU. The overall classification accuracy was 92.0% (k = 0.89) in the no-tilled topsoil, 91.6% (k = 0.90) in the tilled topsoil and 88.3% (k = 0.85) in the subsoil. Our technique enabled the clear separation of voids and soil matrix in all samples. The soil matrix was characterized irregular distribution of loess and Luvisol that were mixed during the soil reclamation procedure. Images recorded at later reclamation stages (tilled topsoil and subsoil images) also showed soil management effects on the soil chemical structural composition. Specifically, the no-tilled topsoil was characterized by a dense soil matrix with a higher area coverage of loess soil and spherical aggregates, as e.g., shown in the areas E5,F, L1–2, and M3 in Figure 3. In two tilled topsoil slices, we observed an increase in OM content and aggregate shape, due to the organic fertilizer amendment (Figure 3, B,C11 and B12, K11–12, L,M10, and L,M11). The area coverage of OM in the slices of the subsoil was comparable with that of the tilled topsoil, although the total organic carbon of the topsoil was rather low (Table 1). Although Fe/Mn concretions could only be found in the no-tilled topsoil, all samples contained FeOx-rich areas. The most distinguishable was slice one from the no-tilled topsoil sample, which had exceptionally high percentages of FeOx-dominated areas.
Figure 3. Classification images and relative coverage of each class. Classification was computed on images of linear spectral unmixing (LSU). LSU images contain information about each endmember contribution to each pixel in the 2D space. When we classify images according to their abundancy, classes are calculated based on the highest domination of selected component. It means that for example a pixel classified as Fe is dominated by iron, but may also contain fractions of other endmembers.
Only 4–5 common points were sufficient to align the μCT images with the imVNIR images by a similarity transformation. This initial registration was suitable to ensure a good result of the affine transformation, which relied only on mutual information. Figure 4 depicts all 2D slice cuts corresponding to original μCT images, which were mapped according to the imVNIR images. The similarity could be distinguished especially in large pores, such as the central biopore in the subsoil sample or in large cracks. An example for a large crack can be found in A–E14,15 and G–J14,15, whereas a large biopore is visible in N–P15,16 and S–U15,16 (Figure 4). These characteristics were less pronounced in other denser or more mixed samples. However, other features such as aggregates also indicated correct registrations. For example, in the tilled topsoil sample (e.g., Figure 4, areas G10,11 and U9), the identified aggregates were rich in OM and therefore had lower reflectance in the imVNIR images. The corresponding regions in the μCT image were also darker because of the low electron density of the OM compared with that of the other solid substances. However, in some areas, slight differences could be observed because of the pre-treatment of the intact soil cores during resin impregnation. It should be noted that some pores in the imVNIR images are not black. In shallow pores, solid material from deeper layers was visible through the transparent resin (Figure 4, areas I9, H10, I10, H12, and T9), hence the reflectance was affected by the signal from the material below.
Figure 4. Image pairs of registered 2D slices of μCT (gray) and imVNIR images (visible spectra). Gray values of μCT represent dense areas (white) and pores (black). The pores in the imVNIR images can be black or light colored, since the resin used is transparent.
The EDT was computed individually for segmented pores and biopores, and the corresponding images show the Euclidean distance from any non-pore/non-biopore voxel to the next pore/biopore. Figure 5 illustrates the results of the correlative analysis by observing the OM intensity distribution over the distance to the macropores and biopores. Correlative analysis with macropore distances in the no-tilled sample demonstrated a small increase in OM content with increasing distance. In contrast, the tilled topsoil sample revealed a decreasing trend of OM content with increasing distance to macropores. Furthermore, in the tilled topsoil sample, there were no soil matrix pixels more than 0.5 mm away from the next macropore. The subsoil sample (slice 1) had a relatively small abundance of OM-rich pixels near the pores, which then increased to about 100% at a distance of 0.75 mm. This relatively high distance was observed only in the subsoil sample.
Figure 5. Area based relative contribution of OM in linear spectral unmixing images with the 3D Euclidean distance to pores (upper graphs) and biopores (bottom graphs). The pictures on the right display the same cutout of macropores and biopores (gray) and the corresponding distances (small distance = purple, high distance = orange).
The density of biopores in samples was much lower compared with that of the total amount of macropores, leading to much longer distances (>4 mm). Compared with the distance to pores, the OM trends around the biopores were less pronounced (Figure 5). In both slices of the tilled topsoil sample and in one slice of the subsoil, a small increase in OM was observed with increasing distance. Moreover, the biopores (0 mm distance) of the tilled soil samples exhibited low OM content.
Figure 6A illustrates the macroporosity distribution relative to the distance to biopores. In all samples, macroporosity decreased with increasing distance (up to roughly 0.2 mm), but this gradient was predominant in the subsoil (Figure 6A). The relative abundance of OM in the vicinity of the subsoil biopores increased after normalizing the local OM contribution by the corresponding fraction of dense soil material, i.e., taking the shift in macroporosity into account (Figure 6B).
Figure 6. (A) Porosity and (B) spectral intensity of OM normalized by the solid volume fraction, as a function of the 3D Euclidean distance to biopores. The normalization made it possible to account for the density shifts around the biopores as visible in (A).
The contribution of FeOx (Supplementary Figure 5) did not significantly change in any sample with the distance to pores and biopores. Interestingly, the FeOx abundance next to biopores increased in the no-tilled topsoil sample. The deviation of the FeOx distribution directly in the pores from zero can be attributed to the high amount of pores, which are smaller than the spatial resolution of the hyperspectral camera (53 μm). In this case, smaller pores are not detectable in the imVNIR images, but they are visible in μCT (19 μm resolution), resulting in a mixed spectrum of pores and FeOx-rich solid volume. This can explain the strong signal of FeOx in the LSU images at locations that are classified as pores in the μCT image instead.
ImVNIR is a non-destructive analysis to characterize physicochemical properties in soil. However, when slicing field moist samples, several artifacts such as smearing soil can be created, thus destroying the macropore system and creating surface topography that leads to shadows. In our study, we successfully applied, for the first time, resin impregnation with polyester followed by cutting in combination with subsequent imVNIR. The developed impregnation procedure allowed us to sustain the integrity of the soil microstructure and avoid the destruction of the macropore system (Rasa et al., 2012; Jangorzo et al., 2014; Gutiérrez Castorena et al., 2016; Mueller et al., 2017; Schlüter et al., 2019). In rare instances, we observed small changes occurring especially in looser areas, due to sample preparation artifacts. In the acetone dehydration pre-treatment, a large pore collapsed in the tilled topsoil (Figure 4, areas C11 and H,I11) during the impregnation, whereas some cracks that were visible in the no-tilled topsoil (Figure 4, areas O6 and T6) diminished. Although there are different dehydration methods available (e.g., drying in the oven), they can create other specific artifacts, such as crack formation, that could complicate the registration and interpretation (Jongerius and Heintzberger, 1975). Despite the occurrence of small changes, the currently developed sample pre-treatment method allowed the horizontal cut of the soil in larger slices without smearing (Figure 4), and thus, a good registration of μCT and imVNIR images was achieved (Figure 4).
The impregnated soil slices provided a perfect basis for the segmentation of different compositional and structural features, such as OM- and FeOx-dominated areas (Figure 3), with imVNIR. The slopes in the spectrum (Supplementary Figure 1) of selected endmembers were in line with data reported by Steffens and Buddenbaum (2013), as their selected regions, such as Mn- and FeOx-dominated areas in a loess-derived stagnic Luvisol, exhibited comparable spectra. As soil cores originated from the same substrate, imVNIR image processing revealed that the chemical and structural features were distributed differently within the samples due to the soil development after reclamation. In the no-tilled topsoil and subsoil, we observed features that were influenced by the reclamation process. Moreover, μCT revealed structural pores inside the OM-rich aggregates of these samples. These aggregates, known as “rolled aggregates”, are typically formed on the conveyor belt during transportation of the reclamation soil in the area and can be rich in OM due to the admixture of old topsoil (Luvisol) (Pihlap et al., 2019). In contrast, the sample from the tilled topsoil was characterized by a tillage-induced structure and contained distinctively shaped, OM-rich aggregates (Figure 3, areas B,C 11,12 and K11,12). Segmentation and analysis of the soil composition in undisturbed soil cores defined their 2D spatial distribution and their heterogeneity in 53 μm resolution from samples of 3 cm diameter.
Correlative imaging allowed the enhancement of the information obtained by imVNIR with data provided by μCT. Hence, we were able to correlate the compositional information of the solid phase with the soil structure, such as the OM distribution as a function of the 3D Euclidean distance to pores and biopores (Figure 5). Nowadays, besides imVNIR, several techniques are available for chemical mapping of 2D slices, such as SEM-EDX, near edge X-ray absorption fine structure spectroscopy, nanoSIMS, or matrix-assisted laser desorption/ionization (Baveye et al., 2018). These techniques could be also applied for correlative analyses to obtain additional chemical information on the 3D pore structure derived from the μCT, as reported in the study of Schlüter et al. (2019) for SEM-EDX and nanoSIMS analysis. Most of these techniques can even have a resolution on the nm scale and thus exceed the resolution of both imVNIR and μCT. However, high-resolution results in small sample sizes, thus limiting these techniques to microaggregate analyses (Baveye et al., 2018). In contrast, the combination of imVNIR and μCT can be applied to scan soil cores up to 10 cm width and infinite length. Thus the linkage between soil structure and soil composition can be investigated in larger soil volumes up to a complete pedon. In addition, relative fast imaging (μCT ∼30 min, imVNIR ∼5min), the fast and parallelized elasitx registration method (∼5 min) and the possibility to embed all samples at the same time make it possible to use the method on multiple soil cores in a full field study, even if samples are sliced several times (every 0.5 cm).
The methods employed in the present correlative imaging had different spatial resolutions. The resolution of the 3 cm scanned samples in X-ray μCT images was higher (19 μm) than that of the Vis-NIR images (53 μm), thus making only the pores with >38 μm (2 pixel) detectable, whereas local differences within the micropores (diameter <38 μm) could not be addressed. Alternatively, sub-resolution features from greyscale μCT images could have been taken into account by using the gray values around macropores to normalize the OM contribution (Baveye et al., 2018), instead of using only visible pore and solid fraction (Figure 6). However, the normalization we used indicated a closer linkage between the soil composition and the soil structural features, visible in μCT, i.e., macropores >38 μm. Potentially, an even broader spectrum of pore sizes could be investigated using subsamples for μCT images and combining the information of pore size distribution from different sample sizes (Vogel et al., 2010). In the current study, we demonstrated that registering higher-resolution μCT images and combining them with imVNIR images expands the information level on the sub-resolution features in larger samples.
Correlative imaging with imVNIR and μCT revealed patterns in FeOx and OM distribution through the distance to pores and biopores (Figure 5). The different trends in OM, as a function of distance to macropores, demonstrated that OM accumulated with increasing distance to macropores, most probably due to physical protection, as the absence of macropores may lead to a deficient aeration but may also hinder microorganisms of reaching OM (Dungait et al., 2012). The comparison between the tilled topsoil and subsoil samples revealed how tillage affects the OM distribution, as shown by previously observed homogenization in OM distribution (Yang and Wander, 1999; Kay and VandenBygaart, 2002). In contrast to our expectations, we did not identify higher OM quantities in biopore walls; in some samples even lower intensities were detected (Figure 5), perhaps due to local differences in macroporosity that were higher close to biopores (Figure 6). When the OM contribution was normalized according to the density shift from μCT, the OM in the no-tilled topsoil and subsoil was higher next to the walls of biopores compared with that in bulk soil (Figure 6). Consequently, based on the OM quantity per gram soil, the OM content would be higher close to pore walls. This shows the possibility and strength of the new method to explain spatial OM distribution obtained by imVNIR image analysis and additionally explain possible contradictory results in OM distribution by incorporating the shifts in density revealed by μCT. Considering that the impact of soil management on carbon stabilization has been particularly investigated (Follett, 2001; Chivenge et al., 2007; Cárcer et al., 2019), correlative analysis with imVNIR and X-ray μCT may broaden our understanding of OM distribution, associated with physical protection due to its location in the porous soil.
In this study, we present an approach for the identification and correlation of soil chemical composition and pore structure in intact soil cores. To that end, we developed a novel combination of 2D imVNIR and 3D μCT through registration and correlative analysis. As a proof of principle, we analyzed three intact soil samples, each with two slices that differed in soil structure and OM content (no-tilled topsoil, tilled topsoil and subsoil). Correlative analysis along with 3D soil structural information about pores can give new insights into the linkage between the biopore network and the soil chemical composition including OM distribution. The analysis indicated that, when the soil is not disturbed by tillage, OM accumulates and is more protected as the distance to macropores increases. μCT data gave valuable input not only by locating pores and biopores but also through local shifts in bulk density. As both techniques give complementary and partly corroborating information on the same material, the development of the applied method improves our understanding on the spatial distribution of soil components and their correlation to soil structure.
The datasets generated for this study are available on request to the corresponding author.
All authors listed have made a substantial, direct and intellectual contribution to the work and approved it for publication. ML conducted image registration and correlative imaging; EP conducted imVNIR image analysis.
This study was funded by the Deutsche Forschungsgemeinschaft within the framework of the research unit DFG AOBJ: 628683. The budget of the UFZ library supports the open access publications fees.
The authors declare that the research was conducted in the absence of any commercial or financial relationships that could be construed as a potential conflict of interest.
We gratefully acknowledge Manuel Endenich (Department of Reclamation, RWE Power AG) for the access to the study fields and his support during sampling campaigns. We are thankful to Carsten W. Mueller and Gertraud Harrington for their valuable advice and assistance with sample preparation and to Steffen Schlüter for his advice on elastix software. We are grateful to reviewers CS and HZ, as their comments helped to improve the manuscript.
The Supplementary Material for this article can be found online at: https://www.frontiersin.org/articles/10.3389/fenvs.2020.00042/full#supplementary-material
Banfield, C. C., Dippold, M. A., Pausch, J., Hoang, D. T. T., and Kuzyakov, Y. (2017). Biopore history determines the microbial community composition in subsoil hotspots. Biol. Fertil. Soils 53, 573–588. doi: 10.1007/s00374-017-1201-5
Baveye, P. C., Otten, W., Kravchenko, A., Balseiro-Romero, M., Beckers, É, Chalhoub, M., et al. (2018). Emergent properties of microbial activity in heterogeneous soil microenvironments: different research approaches are slowly converging, yet major challenges remain. Front. Microbiol. 9:11054. doi: 10.3389/fmicb.2018.01929
Borra, S., Thanki, R., and Dey, N. (2019). Satellite Image Analysis: Clustering and Classification. Singapore: Springer Singapore.
Bronick, C. J., and Lal, R. (2005). Soil structure and management: a review. Geoderma 124, 3–22. doi: 10.1016/j.geoderma.2004.03.005
Buddenbaum, H., and Steffens, M. (2011). Short communication: laboratory imaging spectroscopy of soil profiles. J. Spectral Imaging 2:a2. doi: 10.1255/jsi.2011.a2
Caplan, J., Niethammer, M., Taylor, R. M., and Czymmek, K. J. (2011). The power of correlative microscopy: multi-modal, multi-scale, multi-dimensional. Curr. Opin. Struct. Biol. 21, 686–693. doi: 10.1016/j.sbi.2011.06.010
Cárcer, P. S., de Sinaj, S., Santonja, M., Fossati, D., and Jeangros, B. (2019). Long-term effects of crop succession, soil tillage and climate on wheat yield and soil properties. Soil Tillage Res. 190, 209–219. doi: 10.1016/j.still.2019.01.012
Chivenge, P., Murwira, H., Giller, K., Mapfumo, P., and Six, J. (2007). Long-term impact of reduced tillage and residue management on soil carbon stabilization: implications for conservation agriculture on contrasting soils. Soil Tillage Res. 94, 328–337. doi: 10.1016/j.still.2006.08.006
Dabboor, M., Howell, S., Shokr, M., and Yackel, J. (2014). The Jeffries–Matusita distance for the case of complex Wishart distribution as a separability criterion for fully polarimetric SAR data. Int. J. Remote Sens. 35, 6859–6873. doi: 10.1080/01431161.2014.960614
Dungait, J. A. J., Hopkins, D. W., Gregory, A. S., and Whitmore, A. P. (2012). Soil organic matter turnover is governed by accessibility not recalcitrance. Glob. Change Biol. 18, 1781–1796. doi: 10.1111/j.1365-2486.2012.02665.x
Follett, R. F. (2001). Soil management concepts and carbon sequestration in cropland soils. Soil Tillage Res. 61, 77–92. doi: 10.1016/S0167-1987(01)00180-5
Gutiérrez Castorena, E. V., Gutiérrez-Castorena, M. D. C., González Vargas, T., Cajuste Bontemps, L., Delgadillo Martínez, J., Suástegui Méndez, E., et al. (2016). Micromapping of microbial hotspots and biofilms from different crops using digital image mosaics of soil thin sections. Geoderma 279, 11–21. doi: 10.1016/j.geoderma.2016.05.017
Guyader, J.-M., Huizinga, W., Poot, D. H. J., van Kranenburg, M., Uitterdijk, A., Niessen, W. J., et al. (2018). Groupwise image registration based on a total correlation dissimilarity measure for quantitative MRI and dynamic imaging data. Sci. Rep. 8:137. doi: 10.1038/s41598-018-31474-7
Handschuh, S., Baeumler, N., Schwaha, T., and Ruthensteiner, B. (2013). A correlative approach for combining microCT, light and transmission electron microscopy in a single 3D scenario. Front. Zool. 10:44. doi: 10.1186/1742-9994-10-44
Hapca, S. M., Wang, Z. X., Otten, W., Wilson, C., and Baveye, P. C. (2011). Automated statistical method to align 2D chemical maps with 3D X-ray computed micro-tomographic images of soils. Geoderma 164, 146–154. doi: 10.1016/j.geoderma.2011.05.018
L3Harris Geospatial Solutions (2020). Calculate Confusion Matrices. Availabe at: https://www.harrisgeospatial.com/docs/CalculatingConfusionMatrices.html (accessed February 24, 2020).
Hoang, D. T. T., Bauke, S. L., Kuzyakov, Y., and Pausch, J. (2017). Rolling in the deep: priming effects in earthworm biopores in topsoil and subsoil. Soil Biol. Biochem. 114, 59–71. doi: 10.1016/j.soilbio.2017.06.021
Hobley, E., Steffens, M., Bauke, S. L., and Kögel-Knabner, I. (2018). Hotspots of soil organic carbon storage revealed by laboratory hyperspectral imaging. Sci. Rep. 8:151. doi: 10.1038/s41598-018-31776-w
Jangorzo, N. S., Schwartz, C., and Watteau, F. (2014). Image analysis of soil thin sections for a non-destructive quantification of aggregation in the early stages of pedogenesis. Eur. J. Soil Sci. 65, 485–498. doi: 10.1111/ejss.12110
Jongerius, A., and Heintzberger, G. (1975). Methods in Soil Micromorphology: a Technique for the Preparation of Large Thin Sections. London: Soil Survey Institute.
Juyal, A., Otten, W., Falconer, R., Hapca, S., Schmidt, H., Baveye, P. C., et al. (2019). Combination of techniques to quantify the distribution of bacteria in their soil microhabitats at different spatial scales. Geoderma 334, 165–174. doi: 10.1016/j.geoderma.2018.07.031
Kavzoglu, T., Tonbul, H., Yildiz Erdemir, M., and Colkesen, I. (2018). Dimensionality reduction and classification of hyperspectral images using object-based image analysis. J. Indian Soc. Remote Sens. 46, 1297–1306. doi: 10.1007/s12524-018-0803-1
Kay, B. D., and VandenBygaart, A. J. (2002). Conservation tillage and depth stratification of porosity and soil organic matter. Soil Tillage Res. 66, 107–118. doi: 10.1016/S0167-1987(02)00019-3
Klein, S., Staring, M., Murphy, K., Viergever, M. A., and Pluim, J. (2010). elastix: a toolbox for intensity-based medical image registration. IEEE Trans. Med. Imaging 29, 196–205. doi: 10.1109/TMI.2009.2035616
Kravchenko, A. N., Guber, A. K., Razavi, B. S., Koestel, J., Quigley, M. Y., Robertson, G. P., et al. (2019). Microbial spatial footprint as a driver of soil carbon stabilization. Nat. Commun. 10:9575. doi: 10.1038/s41467-019-11057-4
Lucas, M., Schlüter, S., Vogel, H.-J., and Vetterlein, D. (2019). Soil structure formation along an agricultural chronosequence. Geoderma 350, 61–72. doi: 10.1016/j.geoderma.2019.04.041
Maes, F., Collignon, A., Vandermeulen, D., Marchal, G., and Suetens, P. (1997). Multimodality image registration by maximization of mutual information. IEEE Trans. Med. Imaging 16, 187–198. doi: 10.1109/42.563664
Mattes, D., Haynor, D. R., Vesselle, H., Lewellyn, T. K., and Eubank, W. (2001). “Nonrigid multimodality image registration,” in Medical Imaging 2001: Image Processing, eds M. Sonka and K. M. Hanson (Bellingham: SPIE), 1609–1620.
Mueller, C. W., Hoeschen, C., Steffens, M., Buddenbaum, H., Hinkel, K., Bockheim, J. G., et al. (2017). Microscale soil structures foster organic matter stabilization in permafrost soils. Geoderma 293, 44–53. doi: 10.1016/j.geoderma.2017.01.028
Mueller, C. W., Schlund, S., Prietzel, J., Kögel-Knabner, I., and Gutsch, M. (2012). Soil aggregate destruction by ultrasonication increases soil organic matter mineralization and mobility. Soil Sci. Soc. Am. J. 76:1634. doi: 10.2136/sssaj2011.0186
Peddle, D. R., Peter White, H., Soffer, R. J., Miller, J. R., and LeDrew, E. F. (2001). Reflectance processing of remote sensing spectroradiometer data. Comput. Geosci. 27, 203–213. doi: 10.1016/S0098-3004(00)00096-0
Pihlap, E., Vuko, M., Lucas, M., Steffens, M., Schloter, M., Vetterlein, D., et al. (2019). Initial soil formation in an agriculturally reclaimed open-cast mining area - the role of management and loess parent material. Soil Tillage Res. 191, 224–237. doi: 10.1016/j.still.2019.03.023
Portell, X., Pot, V., Garnier, P., Otten, W., and Baveye, P. C. (2018). Microscale heterogeneity of the spatial distribution of organic matter can promote bacterial biodiversity in soils: insights from computer simulations. Front. Microbiol. 9:39. doi: 10.3389/fmicb.2018.01583
Rabot, E., Wiesmeier, M., Schlüter, S., and Vogel, H.-J. (2018). Soil structure as an indicator of soil functions: a review. Geoderma 314, 122–137. doi: 10.1016/j.geoderma.2017.11.009
Rasa, K., Eickhorst, T., Tippkötter, R., and Yli-Halla, M. (2012). Structure and pore system in differently managed clayey surface soil as described by micromorphology and image analysis. Geoderma 173-174, 10–18. doi: 10.1016/j.geoderma.2011.12.017
Ravel, S., Fossati, C., and Bourennane, S. (2018). Spectral unmixing of hyperspectral images in the presence of small targets. Rem. Sens. 7, 1–12. doi: 10.18282/rs.v7i1.460
Ritz, K., McNicol, J. W., Nunan, N., Grayston, S., Millard, P., Atkinson, D., et al. (2004). Spatial structure in soil chemical and microbiological properties in an upland grassland. FEMS Microbiol. Ecol. 49, 191–205. doi: 10.1016/j.femsec.2004.03.005
Rodarmel, C., and Shan, J. (2002). Principal component analysis for hyperspectral image classification. Surv. Land Inform. Syst. 62, 115–123.
Schlüter, S., Eickhorst, T., and Mueller, C. W. (2019). Correlative imaging reveals holistic view of soil microenvironments. Environ. Sci. Technol. 53, 829–837. doi: 10.1021/acs.est.8b05245
Shamonin, D. P., Bron, E. E., Lelieveldt, B. P. F., Smits, M., Klein, S., and Staring, M. (2013). Fast parallel image registration on CPU and GPU for diagnostic classification of Alzheimer’s disease. Front. Neuroinform. 7:50. doi: 10.3389/fninf.2013.00050
Soriano-Disla, J. M., Janik, L. J., Viscarra Rossel, R. A., Macdonald, L. M., and McLaughlin, M. J. (2014). The performance of visible, near-, and mid-infrared reflectance spectroscopy for prediction of soil physical, chemical, and biological properties. Appl. Spectrosc. Rev. 49, 139–186. doi: 10.1080/05704928.2013.811081
Steffens, M., and Buddenbaum, H. (2013). Laboratory imaging spectroscopy of a stagnic Luvisol profile — High resolution soil characterisation, classification and mapping of elemental concentrations. Geoderma 19, 122–132. doi: 10.1016/j.geoderma.2012.11.011
Steffens, M., Kohlpaintner, M., and Buddenbaum, H. (2014). Fine spatial resolution mapping of soil organic matter quality in a Histosol profile. Eur. J. Soil Sci. 65, 827–839. doi: 10.1111/ejss.12182
Steffens, M., Rogge, D. M., Mueller, C. W., Höschen, C., Lugmeier, J., Kölbl, A., et al. (2017). Identification of distinct functional microstructural domains controlling C storage in soil. Environ. Sci. Technol. 51, 12182–12189. doi: 10.1021/acs.est.7b03715
Stenberg, B., Viscarra Rossel, R. A., Mouazen, A. M., and Wetterlind, J. (2010). “Visible and near infrared spectroscopy,” in Soil Science, ed. D. L. Sparks (Amsterdem: Elsevier), 163–215.
Stockmann, U., Adams, M. A., Crawford, J. W., Field, D. J., Henakaarchchi, N., Jenkins, M., et al. (2013). The knowns, known unknowns and unknowns of sequestration of soil organic carbon. Agricult. Ecosyst. Environ. 164, 80–99. doi: 10.1016/j.agee.2012.10.001
Vogel, H.-J., Weller, U., and Schlüter, S. (2010). Quantification of soil structure based on Minkowski functions. Comput. Geosci. 36, 1236–1245. doi: 10.1016/j.cageo.2010.03.007
Wanzek, T., Keiluweit, M., Varga, T., Lindsley, A., Nico, P. S., Fendorf, S., et al. (2018). The ability of soil pore network metrics to predict redox dynamics is scale dependent. Soil Syst. 2:66. doi: 10.3390/soilsystems2040066
Wiesmeier, M., Urbanski, L., Hobley, E., Lang, B., Lützow, M., von Marin-Spiotta, E., et al. (2019). Soil organic carbon storage as a key function of soils - A review of drivers and indicators at various scales. Geoderma 333, 149–162. doi: 10.1016/j.geoderma.2018.07.026
Yang, X.-M., and Wander, M. M. (1999). Tillage effects on soil organic carbon distribution and storage in a silt loam soil in Illinois. Soil Tillage Res. 52, 1–9. doi: 10.1016/S0167-1987(99)00051-3
Keywords: correlative imaging, X-ray CT, imaging Vis-NIR, soil structure, OM
Citation: Lucas M, Pihlap E, Steffens M, Vetterlein D and Kögel-Knabner I (2020) Combination of Imaging Infrared Spectroscopy and X-ray Computed Microtomography for the Investigation of Bio- and Physicochemical Processes in Structured Soils. Front. Environ. Sci. 8:42. doi: 10.3389/fenvs.2020.00042
Received: 07 November 2019; Accepted: 23 March 2020;
Published: 15 April 2020.
Edited by:
Pascal Boivin, University of Applied Sciences Western Switzerland, SwitzerlandReviewed by:
Hu Zhou, Chinese Academy of Sciences, ChinaCopyright © 2020 Lucas, Pihlap, Steffens, Vetterlein and Kögel-Knabner. This is an open-access article distributed under the terms of the Creative Commons Attribution License (CC BY). The use, distribution or reproduction in other forums is permitted, provided the original author(s) and the copyright owner(s) are credited and that the original publication in this journal is cited, in accordance with accepted academic practice. No use, distribution or reproduction is permitted which does not comply with these terms.
*Correspondence: Maik Lucas, bWFpay5sdWNhc0B1ZnouZGU=
†These authors share first authorship
Disclaimer: All claims expressed in this article are solely those of the authors and do not necessarily represent those of their affiliated organizations, or those of the publisher, the editors and the reviewers. Any product that may be evaluated in this article or claim that may be made by its manufacturer is not guaranteed or endorsed by the publisher.
Research integrity at Frontiers
Learn more about the work of our research integrity team to safeguard the quality of each article we publish.