- 1Laboratory of Applied Microbiology, University of Applied Sciences and Arts of Southern Switzerland, Bellinzona, Switzerland
- 2Department of Arctic Ecology, Norwegian Institute for Nature Research (NINA), Framsenteret, Tromsø, Norway
- 3Faculty of Biosciences, Fisheries and Economics, UiT The Arctic University of Norway, Tromsø, Norway
- 4EcoLab, CNRS, INP, UPS, Université de Toulouse, Toulouse, France
Multiple stressors are increasingly affecting organisms and communities, thereby modifying ecosystems' state and functioning. Raising awareness about the threat from multiple stressors has increased the number of experimental and observational studies specifically addressing consequences of stressor interactions on biota. Most studies measure the direct effects of multiple stressors and their interactions on biological endpoints such as abundance, biomass, or diversity of target organisms. This yields invaluable information for the management and restoration of stressed ecosystems. However, as we argue in our perspective paper, this common approach ignores a fundamental characteristic of communities and ecosystems, i.e., that organisms in ecosystems are interlinked by biotic interactions in ecological networks. Examples from the literature show that biotic interactions can modify stressor effects, transfer stressor effects to distant groups of organisms, and create new stressor interactions. These examples also suggest that changes in biotic interactions can have effects of similar or greater magnitude than direct stressor effects. We provide a perspective on how to include network characteristics and biotic interactions into analyses of multiple-stressor effects on ecosystems. Our approach can also make use of biomonitoring data produced with established and intercalibrated methods, and can combine it with novel metrics used to describe the functioning of ecosystems, such as trait information or stable-isotope measurements. The insights on network-mediated effects gained via the approach we propose can substantially increase mechanistic understanding of multiple-stressor effects, and in turn, the efficiency of ecosystem management and restoration.
Introduction
Anthropogenic stressors affecting organisms and ecosystems rarely occur in isolation. Multiple stressors may interact in their effects on the biota, and complicate understanding and assessment of anthropogenic impacts on ecosystems (Folt et al., 1999; Vinebrooke et al., 2004), thus challenging ecosystem management and restoration (Côté et al., 2016). Global sources of stressors, e.g., climate change, may interact with local stressors such as habitat loss, invasive species, harvesting, and pollution, together affecting most ecosystems (e.g., Allan, 2004). Cumulative effects of multiple stressors on organisms, communities, and ecosystems are difficult to predict because exposure, sensitivity, and adaptability to a given environmental stressor are modified by the effects of other stressors (Vinebrooke et al., 2004). Awareness of these challenges has markedly raised interest and activity in this research field in the last two decades, thus slowly reducing the knowledge gap. Yet, one of the greatest challenges to assess, understand, and predict the impact of multiple stressors on communities and ecosystems arises from stressor effects mediated and modified by biotic interactions (Rosenblatt et al., 2017; Seibold et al., 2018).
Most multiple-stressor experiments and impact assessments focus on species or groups of species as independent endpoints of analyses and correlate metrics describing them with stressor intensities in univariate analyses. However, biotic interactions are relevant for multi-stressed ecosystems because they mediate indirect effects of a stressor which can interact with the direct or indirect effects of other stressors (Schuwirth and Reichert, 2013; Bruder et al., 2017). Assessing biotic interactions is also indispensable for investigating changes in ecosystem functioning in response to stressors (Gray et al., 2014), e.g., in the use as functional indicators (Woodward et al., 2012). The intensity of biotic interactions is the result of abundance, biomass, and phenotype of interacting species, which can all be affected by stressors. Studies focusing on trophic interactions have revealed food-web mediated effects of multiple stressors (see examples below), but non-trophic interactions, such as facilitation and competition (Brooks and Crowe, 2018), and pollinator-plant interactions (Raitif et al., 2019), are also affected by multiple stressors with consequences at the ecosystem level. Accounting for biotic interactions is also paramount to elucidate their role compared to that of environmental factors (including stressors), and the interdependencies between the two in shaping communities and ecosystems (Schuwirth et al., 2016; Cadotte and Tucker, 2017; Seibold et al., 2018).
Species in communities are connected via a network of biotic interactions—both trophic and non-trophic interactions (Figure 1)—which as a whole determine ecosystem functioning and vulnerability, with implications for management (Tylianakis et al., 2010; Harvey et al., 2017; Seibold et al., 2018). The impact of multiple stressors on communities and ecosystems depends on the structure of ecological networks, and on the positions and roles of affected species therein (Table 1; Montoya et al., 2006). Network characteristics such as connectance (i.e., the proportion of realized out of all possible interactions) and modularity (degree to which species form distinct clusters of tightly interacting nodes) are relevant for network stability, and the impacts and spread of stressor effects (Kortsch et al., 2015). Quantifying and testing these and other network characteristics (Gray et al., 2014; Lau et al., 2017) in addition to specific biotic interactions may thus yield crucial information about impact of and vulnerability to multiple stressors not only for the parts (e.g., species and biotic interactions), but for the ecosystem as a whole.
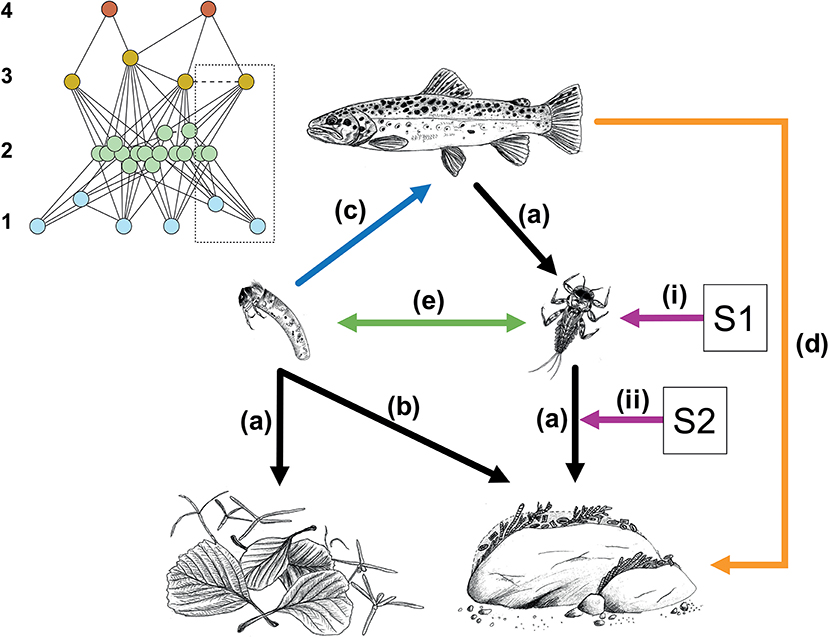
Figure 1. A simplified representation of biotic interactions in a stream food web based on leaf litter (left-hand pathway) and periphyton (right-hand pathway), and affected by multiple stressors (represented by stressors S1 and S2). Black arrows (a,b) represent trophic interactions with top-down effects on resources, with (b) showing the use of a secondary resource by a consumer with a certain degree of omnivory. The blue arrow (c) represents a trophic interaction with bottom-up effects on the consumer. The orange arrow (d) represents an indirect consequence of trophic interactions, e.g., cascading effects or trait-mediated effects, whereas the green arrow (e) represents non-trophic interactions, e.g., competition/facilitation among species in the same trophic level. Purple arrows represent multiple stressors (S1 and S2) with effects on organism groups (i) and on interactions (ii), e.g., due to changes in foraging activity. The inset shows the complete network of species at the site of which the large scheme is an extract or simplification as shown by the dotted box. Blue nodes (trophic level 1) in the inset represent basal resources including autotroph and heterotroph microorganisms (e.g., fungi), green nodes (trophic level 2) represent invertebrates, and orange (trophic level 3), and red nodes (trophic level 4) represent fish. Trophic interactions in the network are exemplified by continuous lines and non-trophic interactions by the dashed lines.
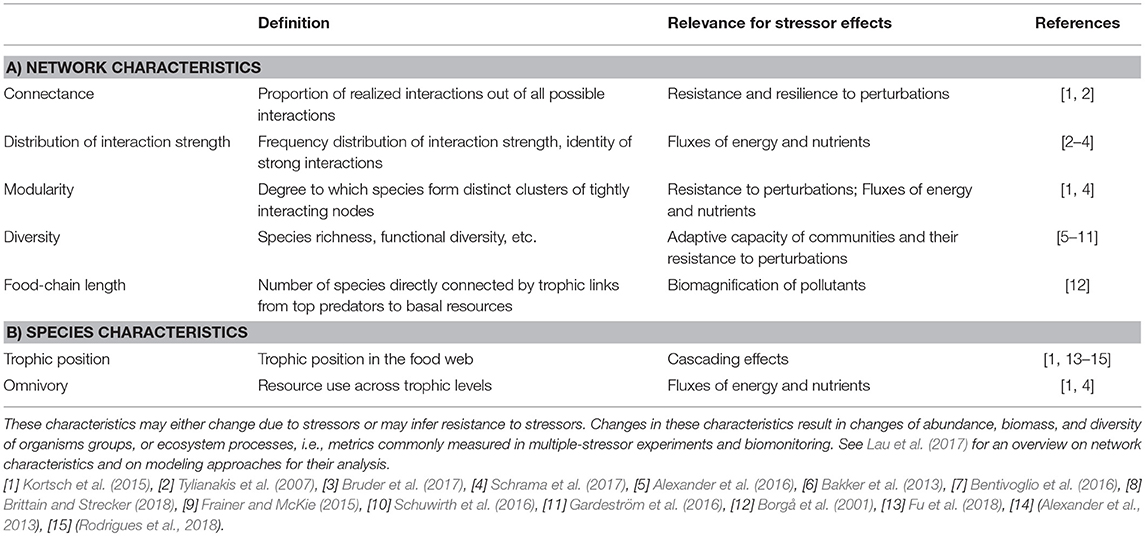
Table 1. Examples of network characteristics (A), and characteristics of species in networks (B), and their attributes relevant for stressor effects.
Statistical approaches to simultaneously quantify direct and indirect stressor effects as well as biotic interactions in complex communities and food webs have been developed and applied in multiple-stressor studies. Among them, structural equation modeling (SEM; Grace, 2006; Grace et al., 2010) is a powerful approach to investigate effects mediated by complex causal structures, and to test the direction and magnitude of the relationships specified by hypothesized causal links between multiple stressors and interacting species (see also Supplementary Material). SEM may thus yield valuable mechanistic understanding of multiple-stressor effects in ecological networks and communities (Grace et al., 2016). Other empirical modeling tools that may be used to address the combined effects of multiple stressors and biotic interactions include state-space models, appropriate when time-series data is available (Tett et al., 2013), and ecological network analyses (reviewed in Lau et al., 2017), which allow to address whole-ecosystem responses for highly resolved food webs (Fu et al., 2018). Mechanistic models have also proven useful in analyzing data from observational multiple-stressor studies (Schuwirth et al., 2016). We believe that embracing ecological network theory, and using statistical approaches that allow testing and quantifying effects mediated by biotic interactions and network characteristics would greatly benefit research and management of communities and ecosystems exposed to multiple stressors.
Applications of the network perspective in multiple-stressor research
Biotic interactions addressed in multiple-stressor studies may span from two-species systems to entire communities containing hundreds of species. System simplifications are often required in experimental studies (Figure 1, discussed below) and might lead to more robust analyses of direct and indirect causal pathways, whereas assessments of complete ecological networks might yield crucial understanding of ecosystem-level perturbations (Figure 1 inset). Here, we illustrate how biotic interactions might be included in multiple-stressors studies on two scales of complexity, (i) on simplifications of the network aiming at resolving causal pathways and measuring processes in detail, and (ii) on assessments of complex food webs aimed at analyzing energy pathways and network characteristics.
Experimental Studies of Food-Web Mediated Stressor Effects
Field experiments yield important insights into how multiple stressors may affect real ecosystems. Working on a land-use gradient in boreal streams, Frainer and McKie (2015) analyzed how land use affects the detritivorous invertebrate community and leaf litter decomposition rates. Their SEM analyses revealed indirect effects of agricultural stressors on decomposition rates of birch litter (Betula pendula) mediated by changes in the density, functional composition, and functional diversity of the invertebrate community. Their results also indicated that indirect stressor effects had similar magnitudes as direct effects. Schuwirth et al. (2016) tested the effects of agricultural and urban stressors on invertebrate communities using a mechanistic model based on Bayesian inference (Schuwirth and Reichert, 2013), and found that biotic interactions among invertebrate taxa (i.e., trophic interactions and competition) were more important in explaining their occurrence at a given site than their sensitivity to stressors.
The above examples are from field assessments, but experiments in mesocosms are increasingly used to test responses of communities to multiple stressors as they represent a useful compromise between experimental control and ecological realism (Stewart et al., 2013). Bruder et al. (2017) tested the effects of agricultural stressors on stream food webs in flow-through mesocosms. In their experiment, SEM revealed an indirect negative effect of reduced flow on the condition of juvenile brown trout (Salmo trutta) by lowering the abundance of invertebrate prey. This effect interacted synergistically with raising water temperatures (a direct stressor) in increasing fish mortality. In a similar experiment, Rodrigues et al. (2018) used SEM to quantify direct and indirect effects of an insecticide and of invasive species on a stream food web. In their study, indirect effects explained a positive effect of an invasive top-predator (Louisiana crayfish, Procambarus clarkii) on algal growth through cascading effects, and a positive effect of the insecticide on invertebrate prey survival due to behavioral changes, which reduced cues used by the predator.
Also in mesocosms, Alexander et al. (2013) tested the interactions between an insecticide mixture, nutrient levels, and predator identity on a stream food web. Using SEM, they found that insecticide stress resulted in a significant cascading effect on invertebrate abundance of lower trophic levels by alleviating the effect of a stonefly predator (Agnetina spp.). Interestingly, this top-down link was not detected in nutrient enriched treatments, possibly because it was masked by a positive effect of nutrients on biofilm-associated resources for the invertebrate community (i.e., a bottom-up link). In mesocosms mimicking agricultural ditches, Schrama et al. (2017) studied the effects of nutrients and insecticides on resource use by invertebrates using stable-isotope analysis. They found that grazers and detritivores preferentially fed on animal remains in stressed conditions, which became more available due to increased mortality, resulting in shifts in resource use, trophic position, and food-chain length.
Energy Pathway and Network Analyses
Studies may analyse how network characteristics of food webs change due to multiple stressors. For example, O'Gorman et al. (2012) analyzed how intertidal communities are affected by the addition of nitrogen and organic matter. Using generalized linear models (GLM) they found that the two stressors had additive effects on food-web connectance and mean food-chain length. Using a highly resolved food web with 180 trophospecies and 1,546 trophic links, Kortsch et al. (2015) analyzed how the addition of four generalist boreal fish species, which are moving into the Arctic due to climate-driven poleward migration, affect the structure of Arctic marine food webs. Their analyses revealed that the incoming species increased connectance and reduced modularity, resulting in a stronger benthic-pelagic link in the food web. Finally, Fu et al. (2018) utilized four different ecosystem models, including Ecopath with Ecosim (Christensen and Walters, 2004), and Atlantis (Fulton et al., 2004), to assess how fishing and primary productivity affect marine food webs in various regions around the globe. They found that taxa from lower trophic levels were more negatively affected by the combined pressure of fishing and low productivity (i.e., low phytoplankton biomass) than those from higher trophic levels.
Discussion
Integrating a network perspective and biotic interactions into the analysis of multiple-stressor experiments or impact assessments provides crucial information on stressor effects on organisms, communities, and ecosystem processes, and on their vulnerability to further environmental change. Testing biotic interactions explicitly may elucidate indirect stressor effects, which seem common in most ecosystem types given the complexity of their ecological network (Harvey et al., 2017). Accounting for biotic interactions and network characteristics may also yield important mechanistic information on the stressors' mode of action. The perspective we propose provides a foundation for simultaneous quantitative estimates of alterations in taxonomic and functional community composition due to multiple stressors, and their consequences on ecosystem functioning (Figure 1). Excluding these fundamental characteristics of biotic communities may thus limit the interpretation and applicability of findings from multiple-stressor analyses to research and management (see also Gray et al., 2014).
Indirect stressor effects mediated by biotic interactions are particularly relevant in a multiple-stressor context given the potential of stressors to ripple through communities and ecological networks, resulting in stressors interactions at distant groups of organisms (see examples above). Stressors change the availability of basal resources, consumer biomass, and behavioral or morphological traits, all affecting the intensity of consumption and energy flows (Brodin et al., 2014; Alexander et al., 2016; Rodrigues et al., 2018). Stressors may also increase susceptibility to infectious organisms (e.g., parasites; Studer and Poulin, 2013; Hofmann et al., 2016), with potential knock-on effects on host phenotype and ecological processes (Frainer et al., 2018). Indirect stressor effects mediated by biotic interactions may then interact with the effects of direct stressors and/or other indirect stressors near the top (Bruder et al., 2017), and the bottom of food webs (Alexander et al., 2016).
Biotic interactions also underlie masking effects that might occur when measuring species as independent endpoints of the analysis. Masking effects may be based on adaptation in resource use by consumers when measuring their biomass (Alexander et al., 2013), functional redundancy of consumers when measuring resource use (Rodrigues et al., 2018), or cascading effects across more than two trophic levels (Alexander et al., 2013; Rodrigues et al., 2018). Masking may erroneously be interpreted as organisms being insensitive or resistant toward the stressors. The above examples involve trophic interactions but indirect stressor effects may also be caused by non-trophic interactions. These may occur if stressors cause functional or density changes in organisms, thus triggering trait or density-mediated alterations of non-trophic interactions, e.g., competition and facilitation (Clements et al., 1989; Brooks and Crowe, 2018; Rodrigues et al., 2018). Specifically testing biotic interactions can reveal such indirect stressor effects, novel stressor combinations, and their ecosystem-level consequences.
Consequences of multiple stressors mediated by biotic interactions can alter network characteristics of communities due to changes in density, biomass, and/or traits of component species (Table 1; Lau et al., 2017). Stressor effects may manifest for instance as changes to network stability and its response to additional stressors, all potentially affecting overall resource use, fluxes of energy and nutrients, and other ecosystem processes (Kortsch et al., 2015; Fu et al., 2018). In many cases, the data available from experimental and observational studies, impact assessments, and ecosystem management is insufficient to test all network characteristics (Tylianakis et al., 2010). Knowledge on interactions among all taxa would be required (e.g., on trophic links established through gut-content or stable-isotope analyses; Gray et al., 2014; Schuwirth et al., 2016), which is challenging because the number of potential interactions and stressor impacts increases exponentially with taxon richness considered (Tylianakis et al., 2010; Lau et al., 2017). Interestingly, estimates of connectance seem to saturate more rapidly with sampling effort than most other network characteristics (Tylianakis et al., 2010), and may provide a good starting point.
Whichever the most appropriate approach for data analysis, reductions of the biological complexity of networks will often be required (Figure 1; Schuwirth and Reichert, 2013; Frainer et al., 2017). Possibilities include simplifying networks to few key taxa and species interactions that are carefully quantified (Gardeström et al., 2016; Bruder et al., 2017; Schrama et al., 2017), pooling species into higher-order taxa, feeding guilds or other functional attributes (Schuwirth et al., 2016; Schrama et al., 2017; Fu et al., 2018), or characterizing species in a multivariate space and utilizing one or few dimensions of that space as the variable(s) describing the community (Frainer and McKie, 2015). Obviously, such reductions have to be done with caution and sufficient knowledge of the study system in order to avoid oversimplification. Nevertheless, if done correctly, these simplifications represent attractive compromises between data availability and testing of relevant ecological questions.
Stressor effects may modify biotic interactions and network characteristics of communities in multi-stressed ecosystems and are thus relevant for biomonitoring (Gray et al., 2014) and ecosystem management (Carvalho et al., 2019), but also for ecotoxicology (Preston, 2002; Clements and Rohr, 2010; Segner et al., 2014; Nilsen et al., 2019). Although our examples are mainly derived from aquatic ecosystems, the findings and concepts we present are founded on ecological theory and are thus transferable to most other ecosystems, as exemplified by similar studies in grasslands (Grace et al., 2016) and urban soils (Tresch et al., 2019). The perspective and approaches we propose have the potential to yield ecologically more complete findings and in turn to increase the benefit from the resources invested in ecosystem assessment and management.
Author Contributions
AB and AF conceptualized and led the study. AB led the writing with contributions from AF, TR, and RP.
Funding
Funding for publication fees was granted by the publication fund of UiT The Arctic University of Norway.
Conflict of Interest Statement
The authors declare that the research was conducted in the absence of any commercial or financial relationships that could be construed as a potential conflict of interest.
Acknowledgments
We greatly appreciate the invitation from the Editors of the Frontiers special issue on Multiple stressors across ecosystem boundaries to contribute this perspective paper. We are also grateful for the comments by R. A. Paterson, K. Lange, and two reviewers on earlier versions of the manuscript.
Supplementary Material
The Supplementary Material for this article can be found online at: https://www.frontiersin.org/articles/10.3389/fenvs.2019.00059/full#supplementary-material
References
Alexander, A. C., Culp, J. M., Baird, D. J., and Cessna, A. J. (2016). Nutrient–insecticide interactions decouple density-dependent predation pressure in aquatic insects. Freshw. Biol. 61, 2090–2101. doi: 10.1111/fwb.12711
Alexander, A. C., Luis, A. T., Culp, J. M., Baird, D. J., and Cessna, A. J. (2013). Can nutrients mask community responses to insecticide mixtures? Ecotoxicology 22, 1085–1100. doi: 10.1007/s10646-013-1096-3
Allan, J. D. (2004). Landscapes and riverscapes: the influence of land use on stream ecosystems. Annu. Rev. Ecol. Evol. Syst. 35, 257–284. doi: 10.1146/annurev.ecolsys.35.120202.110122
Bakker, E. S., Dobrescu, I., Straile, D., and Holmgren, M. (2013). Testing the stress gradient hypothesis in herbivore communities: facilitation peaks at intermediate nutrient levels. Ecology 94, 1776–1784. doi: 10.1890/12-1175.1
Bentivoglio, F., Calizza, E., Rossi, D., Carlino, P., Careddu, G., Rossi, L., et al. (2016). Site-scale isotopic variations along a river course help localize drainage basin influence on river food webs. Hydrobiologia 770, 257–272. doi: 10.1007/s10750-015-2597-2
Borgå, K., Gabrielsen, G. W., and Skaare, J. U. (2001). Biomagnification of organochlorines along a Barents Sea food chain. Environ. Pollut. 113, 187–198. doi: 10.1016/S0269-7491(00)00171-8
Brittain, J. T., and Strecker, A. L. (2018). The interactive effect of multiple stressors on crustacean zooplankton communities in montane lakes. Water Resour. Res. 54, 939–954. doi: 10.1002/2017WR020605
Brodin, T., Piovano, S., Fick, J., Klaminder, J., Heynen, M., and Jonsson, M. (2014). Ecological effects of pharmaceuticals in aquatic systems—impacts through behavioural alterations. Philos. Trans. R. Soc. B Biol. Sci. 369:20130580. doi: 10.1098/rstb.2013.0580
Brooks, P. R., and Crowe, T. P. (2018). Density and biotic interactions modify the combined effects of global and local stressors. Oikos 127, 1746–1758. doi: 10.1111/oik.04459
Bruder, A., Salis, R. K., Jones, P. E., and Matthaei, C. D. (2017). Biotic interactions modify multiple-stressor effects on juvenile brown trout in an experimental stream food web. Glob. Chang. Biol. 23, 3882–3894. doi: 10.1111/gcb.13696
Côté, I. M., Darling, E. S., and Brown, C. J. (2016). Interactions among ecosystem stressors and their importance in conservation. Proc. R. Soc. B Biol. Sci. 283:20152592. doi: 10.1098/rspb.2015.2592
Cadotte, M. W., and Tucker, C. M. (2017). Should environmental filtering be abandoned? Trends Ecol. Evol. 32, 429–437. doi: 10.1016/j.tree.2017.03.004
Carvalho, L., Mackay, E. B., Cardoso, A. C., Baattrup-Pedersen, A., Birk, S., Blackstock, K. L., et al. (2019). Protecting and restoring Europe's waters: an analysis of the future development needs of the water framework directive. Sci. Total Environ. 658, 1228–1238. doi: 10.1016/j.scitotenv.2018.12.255
Christensen, V., and Walters, C. J. (2004). Ecopath with ecosim: methods, capabilities and limitations. Ecol. Modell. 172, 109–139. doi: 10.1016/j.ecolmodel.2003.09.003
Clements, W. H., Cherry, D. S., and Cairns, J. J. (1989). The influence of copper exposure on predator-prey interactions in aquatic insect communities. Freshw. Biol. 21, 483–488. doi: 10.1111/j.1365-2427.1989.tb01381.x
Clements, W. H., and Rohr, J. R. (2010). Community responses to contaminants: using basic ecological principles to predict ecotoxicological effects. Environ. Toxicol. Chem. 28, 1789–1800. doi: 10.1897/09-140.1
Folt, C. L., Chen, C. Y., Moore, M. V., and Burnaford, J. (1999). Synergism and antagonism among multiple stressors. Limnol. Oceanogr. 44, 864–877. doi: 10.4319/lo.1999.44.3_part_2.0864
Frainer, A., and McKie, B. G. (2015). Shifts in the diversity and composition of consumer traits constrain the effects of land use on stream ecosystem functioning. Adv. Ecol. Res. 52, 169–200. doi: 10.1016/bs.aecr.2015.03.002
Frainer, A., McKie, B. G., Amundsen, P.-A., Knudsen, R., and Lafferty, K. D. (2018). Parasitism and the biodiversity-functioning relationship. Trends. Ecol. Evol. 33, 260–268. doi: 10.1016/j.tree.2018.01.011
Frainer, A., Primicerio, R., Kortsch, S., Aune, M., Dolgov, A. V., Fossheim, M., et al. (2017). Climate-driven changes in functional biogeography of Arctic marine fish communities. Proc. Natl. Acad. Sci. 114, 12202–12207. doi: 10.1073/pnas.1706080114
Fu, C., Travers-Trolet, M., Velez, L., Grüss, A., Bundy, A., Shannon, L. J., et al. (2018). Risky business: the combined effects of fishing and changes in primary productivity on fish communities. Ecol. Modell. 368, 265–276. doi: 10.1016/j.ecolmodel.2017.12.003
Fulton, E. A., Parslow, J. S., Smith, A. D. M., and Johnson, C. R. (2004). Biogeochemical marine ecosystem models II: the effect of physiological detail on model performance. Ecol. Modell. 173, 371–406. doi: 10.1016/j.ecolmodel.2003.09.024
Gardeström, J., Ermold, M., Goedkoop, W., and McKie, B. G. (2016). Disturbance history influences stressor impacts: effects of a fungicide and nutrients on microbial diversity and litter decomposition. Freshw. Biol. 61, 2171–2184. doi: 10.1111/fwb.12698
Grace, J. (2006). Structural Equation Modeling and Natural Systems. New York, NY: Cambridge University Press.
Grace, J. B., Anderson, T. M., Olff, H., and Scheiner, S. M. (2010). On the specification of structural equation models for ecological systems. Ecol. Monogr. 80, 67–87. doi: 10.1890/09-0464.1
Grace, J. B., Anderson, T. M., Seabloom, E. W., Borer, E. T., Adler, P. B., Harpole, W. S., et al. (2016). Integrative modelling reveals mechanisms linking productivity and plant species richness. Nature 529:390. doi: 10.1038/nature16524
Gray, C., Baird, D. J., Baumgartner, S., Jacob, U., Jenkins, G. B., O'Gorman, E. J., et al. (2014). FORUM: ecological networks: the missing links in biomonitoring science. J. Appl. Ecol. 51, 1444–1449. doi: 10.1111/1365-2664.12300
Harvey, E., Gounand, I., Ward, C. L., and Altermatt, F. (2017). Bridging ecology and conservation: from ecological networks to ecosystem function. J. Appl. Ecol. 54, 371–379. doi: 10.1111/1365-2664.12769
Hofmann, H., Blasco-Costa, I., Knudsen, R., Matthaei, C. D., Valois, A., and Lange, K. (2016). Parasite prevalence in an intermediate snail host is subject to multiple anthropogenic stressors in a New Zealand river system. Ecol. Indic. 60, 845–852. doi: 10.1016/j.ecolind.2015.08.022
Kortsch, S., Primicerio, R., Fossheim, R., Dolgov, A. V., and Aschan, M. (2015). Climate change alters the structure of arctic marine food webs due to poleward shifts of boreal generalists. Proc. R. Soc. B Biol. Sci. 282:20151546. doi: 10.1098/rspb.2015.1546
Lau, M. K., Borrett, S. R., Baiser, B., Gotelli, N. J., and Ellison, A. M. (2017). Ecological network metrics: opportunities for synthesis. Ecosphere 8:e01900. doi: 10.1002/ecs2.1900
Montoya, J. M., Pimm, S. L., and Sol,é, R. V. (2006). Ecological networks and their fragility. Nature 442, 259–264. doi: 10.1038/nature04927
Nilsen, E., Smalling, K. L., Ahrens, L., Gros, M., Miglioranza, K. S. B., Pico, Y., et al. (2019). Critical review: grand challenges in assessing the adverse effects of contaminants of emerging concern on aquatic food webs. Environ. Toxicol. Chem. 38, 46–60. doi: 10.1002/etc.4290
O'Gorman, E. J., Fitch, J. E., and Crowe, T. P. (2012). Multiple anthropogenic stressors and the structural properties of food webs. Ecology 93, 441–448. doi: 10.1890/11-0982.1
Preston, B. L. (2002). Indirect effects in aquatic ecotoxicology: implications for ecological risk assessment. Environ. Manage. 29, 311–323. doi: 10.1007/s00267-001-0023-1
Raitif, J., Plantegenest, M., and Roussel, J.-M. (2019). From stream to land: ecosystem services provided by stream insects to agriculture. Agric. Ecosyst. Environ. 270–271, 32–40. doi: 10.1016/j.agee.2018.10.013
Rodrigues, A. C. M., Machado, A. L., Bordalo, M. D., Saro, L., Simão, F. C. P., Rocha, R. J. M., et al. (2018). Invasive species mediate insecticide effects on community and ecosystem functioning. Environ. Sci. Technol. 52, 4889–4900. doi: 10.1021/acs.est.8b00193
Rosenblatt, A. E., Smith-Ramesh, L. M., and Schmitz, O. J. (2017). Interactive effects of multiple climate change variables on food web dynamics: modeling the effects of changing temperature, CO2, and water availability on a tri-trophic food web. Food Webs 13, 98–108. doi: 10.1016/j.fooweb.2016.10.002
Schrama, M., Barmentlo, S. H., Hunting, E. R., van Logtestijn, R. S. P., Vijver, M. G., and van Bodegom, P. M. (2017). Pressure-induced shifts in trophic linkages in a simplified aquatic food web. Front. Environ. Sci. 5:75. doi: 10.3389/fenvs.2017.00075
Schuwirth, N., Dietzel, A., and Reichert, P. (2016). The importance of biotic interactions for the prediction of macroinvertebrate communities under multiple stressors. Funct. Ecol. 30, 974–984. doi: 10.1111/1365-2435.12605
Schuwirth, N., and Reichert, P. (2013). Bridging the gap between theoretical ecology and real ecosystems: modeling invertebrate community composition in streams. Ecology 94, 368–379. doi: 10.1890/12-0591.1
Segner, H., Schmitt-Jansen, M., and Sabater, S. (2014). Assessing the impact of multiple stressors on aquatic biota: the receptor's side matters. Environ. Sci. Technol. 48, 7690–7696. doi: 10.1021/es405082t
Seibold, S., Cadotte, M. W., MacIvor, J. S., Thorn, S., and Müller, J. (2018). The necessity of multitrophic approaches in community ecology. Trends Ecol. Evol. 33, 754–764. doi: 10.1016/j.tree.2018.07.001
Stewart, R. I. A., Dossena, M., Bohan, D. A., Jeppesen, E., Kordas, R. L., Ledger, M. E., et al. (2013). Mesocosm experiments as a tool for ecological climate-change research. Adv. Ecol. Res. 48, 71–181. doi: 10.1016/B978-0-12-417199-2.00002-1
Studer, A., and Poulin, R. (2013). Cercarial survival in an intertidal trematode: a multifactorial experiment with temperature, salinity and ultraviolet radiation. Parasitol. Res. 112, 243–249. doi: 10.1007/s00436-012-3131-3
Tett, P., Gowen, R., Painting, S., Elliott, M., Forster, R., Mills, D., et al. (2013). Framework for understanding marine ecosystem health. Mar. Ecol. Prog. Ser. 494, 1–27. doi: 10.3354/meps10539
Tresch, S., Frey, D., Le Bayon, R.-C., Zanetta, A., Rasche, F., Fliessbach, A., et al. (2019). Litter decomposition driven by soil fauna, plant diversity and soil management in urban gardens. Sci. Total Environ. 658, 1614–1629. doi: 10.1016/j.scitotenv.2018.12.235
Tylianakis, J. M., Lalibert,é, E., Nielsen, A., and Bascompte, J. (2010). Conservation of species interaction networks. Biol. Conserv. 143, 2270–2279. doi: 10.1016/j.biocon.2009.12.004
Tylianakis, J. M., Tscharntke, T., and Lewis, O. T. (2007). Habitat modification alters the structure of tropical host–parasitoid food webs. Nature 445, 202–205. doi: 10.1038/nature05429
Vinebrooke, R. D., Cottingham, K. L., Norberg, M. S., Dodson, S. I., Maberly, S. C., Sommer, U., et al. (2004). Impacts of multiple stressors on biodiversity and ecosystem functioning: the role of species co-tolerance. Oikos 104, 451–457. doi: 10.1111/j.0030-1299.2004.13255.x
Keywords: anthropogenic stressors, biotic communities, biotic interactions, ecosystem restoration, food webs, statistical modeling, structural equation modeling
Citation: Bruder A, Frainer A, Rota T and Primicerio R (2019) The Importance of Ecological Networks in Multiple-Stressor Research and Management. Front. Environ. Sci. 7:59. doi: 10.3389/fenvs.2019.00059
Received: 23 January 2019; Accepted: 17 April 2019;
Published: 07 May 2019.
Edited by:
Maarten Schrama, Leiden University, NetherlandsReviewed by:
James Alexander Orr, Trinity College Dublin, IrelandAlexa C. Alexander, Environment and Climate Change Canada, Canada
Copyright © 2019 Bruder, Frainer, Rota and Primicerio. This is an open-access article distributed under the terms of the Creative Commons Attribution License (CC BY). The use, distribution or reproduction in other forums is permitted, provided the original author(s) and the copyright owner(s) are credited and that the original publication in this journal is cited, in accordance with accepted academic practice. No use, distribution or reproduction is permitted which does not comply with these terms.
*Correspondence: André Frainer, andre.frainer@uit.no