- Faculty of Mechanical and Industrial Engineering, Warsaw University of Technology, Warsaw, Poland
Nowadays, it is still difficult to measure organizations’ performances due to the complexity of energy systems, their multiple functions, and the usage of unclear indicators. Organizations need to consider energy issues to contribute to sustainability. This paper aims to develop a methodology for measuring energy sustainability performance for a biogas plant, but the idea of the study is to establish to which degree the methodology may be substituted for the traditional methods based on multicriteria decision-making, which are used for sustainability assessment. Hence, the evaluation performance method combines fuzzy numbers, linguistic scale, and energy life-cycle indicators. The evaluation is carried out for the biodigester, presenting its results in terms of energy sustainability performance index for two scenarios (p and q) considering the three-dimensional sustainability structure. The results pictured that the value of energy sustainability performance index q (0.382) is relatively lower than the index achieved for scenario p (0.815). Biodigester for scenario q does not demonstrate a positive perspective on a transition toward energy sustainability. The presented methodology is a new simple method aimed at replacing the current energy performance assessments into a technology evaluation solution considering uncertainties, which is not supported by the software. The suggested energy life cycle analysis indicators could be used to evaluate similarly sized biogas plants across countries. They might be also used for prioritization of plants modeling various energy parameters or inspirational for energy decision-makers to deliver more flexible and reliable outcomes within an uncertain environment.
Introduction
Sustainable energy is a hot topic in recent years, which has been discussed throughout international energy programs (also Agenda 2030). The impact results from goals of sustainable development (called SDGs) and Renewable Energy in 2030 direct more efforts to improve energy efficiency to about 32.5% and raise renewable energy share to at least 32% (European Commission, 2016). This positive interaction between energy and SDGs in terms of impact and numbers was also stressed by McCollum et al. (2018). This connection between economy, society, and environment was also strengthened by EU Bioeconomy Strategy to ensure a reliable, circular bioeconomy for investments throughout cost-effectiveness, technological advancement, and bio-based resource availability across production processes (Scarlat et al., 2015). In the frame of circular, sustainable bioeconomy addresses to meet challenges of SDGs, one of them is to shift to a low carbon economy by 2050. Decisions related to the transition in terms of energy sustainability, increase in the share of renewables in the energy mix, and energy storage (Rogge and Reichardt, 2016) are often affected by rising energy demand, material prices (IEA, 2019), and sustainability-related indicators. Very often, these decisional problems are based on making choices under uncertainty. In this context, a biodigester plays a crucial role in the decarbonization of energy systems (Butnar et al., 2020), tackling it through low carbon production such as biogas from anaerobic digestion. Biogas, as one of the most common renewable energy resources, can be used to combine with environment-friendly systems such as heat and power (CHP)1 in cogeneration. Treating dairy manure involves several negative consequences, e.g., an essential increase in GHG emissions (especially CO2) and environmental pollution due to the large consumption of energy. These adverse effects require to be evaluated sustainably to make decisions about the implementation of bioenergy in achieving energy system transitions (Gielen et al., 2019). Anaerobic digestion of manure contributes to a decrease in GHG emissions emitted to the atmosphere by reduction of methane emissions (Scarlat et al., 2018). The sustainable decision-making process is now the key point in a strategy for industrial plants where decisions are based on effective performance assessment methods. Moreover, most evaluation approaches deal with impact assessment as evidence-based procedures2 instead of making decisions based on human behavior or predicting behavior across various domains. These methods lead to highly risky decisions due to unintended effects.
On one side, decisions supported by methods are focused on evidence that provides reliability; on the other hand, decision-makers have better knowledge about the energy situation in a company and make a decision based on their perception, sometimes without explanation of their rational decisions. In both scenarios, a decision-making process is focused on (energy) indicators or metrics. Unfortunately, traditional assessment approaches are still facing methodological problems due to the lack of universally accepted methods and associated metrics or indicators (Shortall and Davidsdottir, 2017), subjectivity in the assessment of different weighting of criteria, lack of data, or vagueness in data for sustainability evaluation (Onar et al., 2015; Wu et al., 2019). These approaches directed for energy sustainability assessment also handled uncertainties across various domains in the decision-making process.
Together with the opportunity to track energy metrics, it is necessary to put more attention to assessing energy from qualitative and quantitative perspectives due to EU legislative requirements and energy power needs. Although EU policies do not impede energy evaluation methods, it is still necessary to start with a simple method for assessing energy sustainability using energy-based indicators to develop standardized practices for energy policy development in consequence. It means that the implication of evaluation methods might be incorporated in future energy policies to measure their impact on industrial facilities (Krysiak and Kluczek, 2021). Although an initial attempt to evaluate renewable energy policy at a national level was performed but legislative requirements were critically reviewed proposing changes in the law (Marinescu, 2020), there are still missing assessments of the effect of energy efficiency in the sustainability context on the individual facility at local levels. For these reasons, evaluation of energy sustainability performance using key life cycle indicators is needed for the considered biogas production (Feiz et al., 2020).
An industrial practice prefers to use exact data, plant technical and operational parameters, or apply vague numbers based on linguistic evaluations. Classical methods (e.g., multicriteria decisional methods) used in sustainability assessments of various technologies and manufacturing processes are characterized by an imperfection in the applied indicators and metrics (Shortall and Davidsdottir, 2017), as well as subjectivity, uncertainty in the assessment of different weighting of criteria, unmeasurable objects, and vagueness in data (Onar et al., 2015; Wu et al., 2019). Unfortunately, the application of traditional assessment methods is sometimes not possible due to imprecise, or lack of, data and the usage of non-equal functional units for indicators for complex objects. Based on the discussed analysis, there is a lack of an integrative method for sustainability performance evaluation on a plant level combining fuzzy logic. In addition, no related papers were found on how industrial plants make energy technology–related decisions under uncertainty. Other assessment methods, like life cycle analysis (LCA), are based on multicriteria approaches (MDCA) that play an integrative role in their evaluation to assess energy systems (Baumann et al., 2019) or energy projects (Estévez et al., 2021; Javed et al., 2021). The robustness of the LCA from a methodical point of view allows supporting decision-making with a combination with relevant methods, involving uncertainty in estimating criteria weights and indicators of various functional units in sustainability assessment (Kluczek, 2019).
Although a recent study on sustainability provides linkages between sustainability considerations and energy-related matters (McCollum et al., 2018), a few of them represent the energy sustainability phenomenon or are intended to evaluate the development of energy sustainability evaluation method on a plant level (Iddrisu and Bhattacharyya, 2015; Shortall and Davidsdottir, 2017; Ninno Muniz et al., 2020). The disadvantages of available approaches for sustainability performance evaluation on the industrial plant level highlight a research gap. Therefore, it explains the need to develop a new method for energy sustainability performance evaluation, which might be mainstreamed into energy policy.
Considering the concept of sustainable bioeconomy in meeting the paper’s goal, a significant reduction of carbonization of energy systems can be achieved through the developed evaluation method for a biogas plant, contributing to meeting the EU energy goals simultaneously. In this context, manufacturers might be active consumers and make sustainable, “controlled” decisions.
The goal of the study is to provide a proposal of a method for energy sustainability performance evaluation dedicated to a biogas plant. The paper intends to establish to which degree the methodology might be replacing the classical methods based on multicriteria decision-making, which are used for sustainability assessment. The considered evaluation method will be based on the combination of fuzzy numbers, linguistic scale, and energy-related indicators based on LCA. Based on the case study for biodigester, two scenarios for calculation of energy sustainability performance index were examined. To achieve this goal, the paper tries to calculate a single aggregated index characterizing the energy sustainability performance considering the three dimensions of the triple bottom line, i.e., economic, environmental, and social. Triangle fuzzy numbers for representation of linguistic scale seem to be most appropriate for rational objective ranking. Fuzzy numbers as a model to deal with inconsistent, insufficient information are characterized by its membership function (l; m; u) and defining fuzzy values in the closed unit interval (0; 1), where 0 represents the lowest degree of membership and 1—the greatest degree of membership (Ross, 2016). Justification for using the linguistic numbers or preferences concentrates on incomplete expert’s knowledge to make decisions.
The uniqueness of the proposed method lies on
The use of energy LCA data passing the biodigester system boundary;
The combination of sustainability concept with fuzzy numbers for sustainability evaluation of a biodigester;
The verification of possibility to apply this new evaluation method in the practice;
The analysis on the micro-level of an industrial plant gives detailed insights into decisional problems and enables sustainability evaluation of the specific technology. Hence, to provide performance evaluation toward energy sustainability, the research is concentrating on a biodigester system in a biogas plant.
The study is focused on the sustainability evaluation of the biodigester system at the plant level. The presented approach will intend to compensate for the problem of subjective judgments (McCollum et al., 2018; Wu et al., 2019). It also enables decision-makers to access industrial energy-related data across multiple repositories with different data types and functional units.
Literature Review
Many researchers sought to contribute to the energy sustainability assessment debate by reviewing the underlining methodologies for the assessment methods. Different applications for technology assessment using linguistic scale were described, e.g., by Zadeh (1965) and Gladysz and Kluczek (2017). The emphasis in sustainability appraisals was put on integration because many approaches to sustainability assessment can be said to be exemplary of “integrated assessment”. In this integrated approach, environmental, social, and economic issues have been incorporated.
Most of the integrated sustainability assessments are based on life cycle method approaches, closely related to LCA applied to projects and assessment of energy systems (Goel et al., 2009). Goel et al. stated that environmental assessment contributed to sustainability by extending its scope to reflect the “three pillars” of sustainability or “triple bottom line” based on which scientific and feasible evaluation criteria were defined to achieve the optimal and effective assessment (Brundtland, 1987). This approach of extension of environmental assessment resulted in the model of TBL (triple bottom line) integrated assessment (Pope et al., 2017). Most suitable methods, like various multicriteria decision-making (MCDM) methods (including AHP, PROMETHEE, TOPSIS, VIKOR, and MAUT), are used for selecting suppliers’ (Luthra et al., 2017) (renewable) energy production (Nzila et al., 2012), assessing and outranking energy systems (Ghafghazi et al., 2010; Ren et al., 2010; Onar et al., 2015), energy planning (Kaya and Kahraman, 2010), and assessing and selecting energy projects (Büyüközkan and Karabulut, 2017) with a sustainability perspective. Various ranges of sustainability indicators have been incorporated within different sustainability assessment methods and assessed accordingly. A comparison of sustainability indicators in terms of environmental (techno-) economic and social criteria for various energy technologies was discussed in May and Brennan (2006), Santoyo-Castelazo and Azapagic (2014), Atilgan and Azapagic (2016), and Akber et al. (2017). Out of the considered studies, a small amount of research was used with energy indicators covering the energy life cycle of energy systems (Martin et al., 2018; Kluczek, 2019).
A comprehensive review of various assessment methods successfully used for solving issues related to energy systems or ranking alternative technology options and their classification was presented in Kumar et al. (2017) and Siksnelyte et al. (2019). A few multi-criteria-based sustainability assessments were combined with other methods (like fuzzy numbers or linguistic scale, or LCA, optimization model). Wu et al. (2019) employed a new decision assessment framework by combining LCA with the MDCM approach to select the best alternative energy system using multi-attribute criteria such as energy ratio, cost, and global warming potential (Wu et al., 2019). Various variations of MDCA in the field of renewable energy were examined in detail (Domínguez-Dafauce and Martín, 2015; Mardani et al., 2015).
Many authors have reviewed fuzzy-based approaches (e.g., fuzzy AHP, TOPSIS, VIKOR) designed for selecting sustainable suppliers of energy technology (Wu et al., 2019; Peng et al., 2020), assessing energy systems (Ren et al., 2010; Siksnelyte et al., 2019; Wang et al., 2020), or weighting sustainability criteria in selection energy resources (Taylan et al., 2020). Çelikbilek and Tüysüz (2016) established a methodology Analytic Network Process (ANP)-VIKOR method, while Kaya and Kahraman (2010) developed fuzzy VIKOR (VIsekriterijumska optimizacija i KOmpromisno Resenje) combined with AHP to make decisions for alternative energy technologies.
In the decision-making process, the three factors—cost and efficiency of energy systems as well as environmental awareness and protection—require to be considered (Zhang et al., 2019). In the assessment taking into consideration the context of sustainability, and environmental regulatory and technological circumstances, these drivers/indicators should be high on the priority list for assessing technologies or industrial facilities. Hence, selected economic and environmental indicators based on LCA were analyzed for energy sustainability performance. To overcome these problems, energy life cycle indicators (using fuzzy numbers) are developed for measuring the energy performance of combined heat and power (CHP) for manageability and quality.
The use of energy LCA encompasses data coming from energy audits, then converting them into energy LCA indicators and reporting in the energy Life Cycle Inventory. The indicators will gain new meaning or features to achieve sustainability goals. The current literature discussed presents that there is no clear evidence of how to evaluate the considered technology integrating the aforementioned components. Although similar combined methods within MDCA have been discussed (Section 2), sometimes critically, the presented integration of methods has not been used so far for energy technology. Through the research based on the literature review on assessment methods and related issues, as well as expert knowledge, the challenges faced by energy sustainability were identified as follows:
The lack of a true method for energy sustainability–based assessment as “a separate leg” to continuously move toward a sustainability assessment system;
Overcome limitations for unsuitable dynamic analysis of sustainability to be used for energy efficiency;
Inappropriate/no adequate metrics to use in the real assessment of technology considering the environmental impact categories, e.g., human health, ecotoxicity, etc. (Rebitzer et al., 2004; Zhang et al., 2016);
The incapability of existing sustainability assessment methods to evaluate energy sustainability;
Easiness of use of quantitative evaluation methods for decision-makers to analyze, assess, and draw conclusions.
The proposed method is intended to focus on sustainable energy criteria to measure which companies have the most favorable indicators associated with the negative effect on climate change. Expected results are to “judge” plants’ energy sustainable performance outcomes based on the defined criteria allowing to make a rational decision related to potential investment in technology. This concept of energy sustainability was not considered in Frontiers in Energy Research anymore, so it constitutes potential for discovering a novel methodology developed for the assessment of a biogas plant. In consequence, it will allow policy-makers, stakeholders, and investors to make rational decisions about “energy responsibility” providing transparency data for the whole manufacturers’ environment. In addition, the study aims to highlight a challenge in terms of the applicability of life cycle energy objective measures to evaluate energy sustainability for the biodigester system. So, the study examines whether proposed sustainable assessment indicators are suitable, applicable, and reliable for measuring the sustainability of energy performance. However, the central concern is focused on increasing the share of renewable energy access achieving to raise the economic effect of companies’ performance simultaneously (Balibrea-Iniesta, 2020). To avoid information distortion, fuzzy numbers can be applied in the real environment to present the fuzziness and hesitancy of expert knowledge (Wu et al., 2019).
From the analysis outlined earlier, all methods deal effectively with energy issues to rank the energy systems. The presented assessment methods seem to be complex and the results are often ineffective because statements or human thoughts are not easily quantifiable as well as the subjective nature of experts’ decisions (Marinescu, 2020). Another approach for assessing energy performance was used in Shortall and Davidsdottir (2017) in which the authors examined “weighted”, among others, aggregated environmental sustainability indicators describing the ratio of low carbon fuel sources in the energy mix and emission impact. Due to these indicators developed within the International Atomic Energy Agency’s Energy (EAPI) framework, the indicators allow assessing and comparing/benchmarking the current performance of national energy systems against a global energy environment. A few of the multi-criteria-based sustainability assessments with their indicators are promising to apply LCA-based criteria. A few of the multi-criteria methods for assessing sustainability provide a viable opportunity to incorporate/encompass LCA-based criteria.
Therefore, indicators based on LCA seem to be attractive for the evaluation of energy sustainability performance. The LCA method is more comprehensive in its coverage of energy efficiency; hence, it is considered as the most suitable indicator for measuring energy performance in the whole life cycle process while others concentrate on static values. If LCA is integrated with fuzzy numbers, shortcomings over the standalone one in energy sustainability–decision-making problems should be overtaken. The assessment of energy sustainability performance could be more feasible as the method is built based on the combination of LCA indicators with linguistic scale represented by fuzzy numbers. The advantage of the proposed method is to ignore value and decision-maker ranking, and subjectivity over fuzziness and “uncertain characteristics of the preference information” (Taylan et al., 2020). Fuzziness overcomes the difficulties with qualitative data (if appeared) (Taylan et al., 2020) or if some data are lacking (Çolak and Kaya, 2017). Although MDCA tools are very popular among researchers, they suffer from uncertainties in assessment (Cinelli et al., 2014; Troldborg et al., 2014) and different criteria leveling (weight deviation), and many scientists reviewed them in the context of suitability and adaptability for assessing energy in terms of sustainability. Also, most of them were reviewed to check their suitability and adaptability for assessing energy in terms of sustainability (Cinelli et al., 2014). Several selected research articles addressing sustainability assessment to energy impact are outlined in Table 1. For this reason, some papers were also analyzed sectorially and geographically. It was noted that most papers are focused on electricity generation, not contributing to any specific sector. The papers’ analysis concludes that the studies come from various countries across the world, with an emphasis on the US market.
Although the performance of biogas production was measured, the research has not considered energy sustainability yet. Only the environmental performance of household biogas production in terms of energy and environmental impact was assessed (Wang et al., 2018). Nonetheless, a small number of papers are dealing with energy LCA on technologies (Cabeza et al., 2014; Kluczek, 2018; Hosseini-Fashami et al., 2019) and do not always consider sustainability, but still attempt to integrate existing LCA-based assessment methods into the integrated sustainability methods. So, Life Cycle Sustainability Assessment (LCSA) definition adopted in practice and challenges identified in LCSA studies so far are overviewed (Zamagni, 2012; Onat et al., 2017). A combination of LCA and fuzzy numbers for assessing sustainability was developed by Mahmood et al. (2015). Hence, any other assessment approaches are still under consideration believing that sustainability is a rationale to build specific sustainability decision-making methods and optimization techniques (Wang et al., 2018). This was the reason why the author of the paper selected a simple fuzzy method as one of the compromises for optimization methods. Moreover, most of the investigated papers (Table 1) sketch that sustainability assessment was carried out based mostly on the national level, rarely on plant level. Therefore, the second rationale is to use an evaluation method in an individual plant to illustrate opportunities of using fuzzy numbers for sustainability assessment when judgments are uncertain.
By reviewing sustainability assessment methodologies, their potentials, and limitations (e.g., ignoring the social and economic aspects or concentrating on one of both topics; Choudhary, 2012), there is a need to apply to energy sustainability evaluation by the combination of fuzzy numbers. An evaluation for energy sustainability is incorporated using decision criteria that describe the sustainability dimensions (economic, environmental, and social) and their energy-related LCA indicators (Kluczek, 2019). The strength of the method is its ability to adapt to a new environment, arriving at a decision based on expert knowledge and involving risk (under uncertainties). In addition, the proposed method requires the use of basic mathematical knowledge of fuzzy algebra. To be in line with prospect theory, energy sustainability evaluation becomes more and more important to make decisions related to rational energy usage.
Hence, this research is aimed at making a rational decision on the selection of technological improvements based on the energy sustainability index.
Materials and Methods: Determining Simple Energy Sustainability Performance Evaluation
To evaluate the performance of biogas plants in terms of environmental sustainability, economic sustainability, and social sustainability, the evaluation index system from the combination of the methods is used. Figure 1 presents a methodology for energy sustainability evaluation incorporating fuzzy numbers.
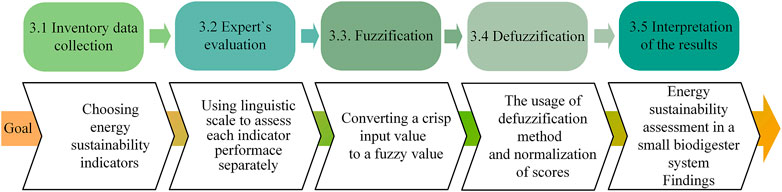
FIGURE 1. A schematic framework for energy sustainability (impact) assessment based on expert’s knowledge (own elaboration).
The methodology is based on expert judgments for different purposes. First, specific and physically measurable indicators are chosen for the assessed case. Then, each indicator is assessed by experts to evaluate its performance. Experts use linguistic scales to qualitatively assess performances across areas measured by indicators. Then, linguistic assessments are denoted using fuzzy numbers, which are later defuzzified, and aggregated for the total energy sustainability assessment.
For a transparent illustration of the sustainability assessment method, each dimension is described by environmental (IEENV), economic (IEECO), and social (IESOC) indicators, respectively. The energy-related sustainability index (IESUS) is considered as the area of these three aggregated sustainability indicators described as fuzzy numbers.
Inventory Data Collection
Key energy indicators for sustainability within Energy Life Cycle Inventory (eLCI) encompass the compilation and quantification of inputs and outputs passing the system boundary (biodigester installation). Data were gathered through energy audits from the plant treating dairy manure for a small biogas farm. The structure of indicators within the eLCI is pictured in Figure 2 including connections with the core PESUS node and the fixed relationship between sustainability dimensions. The eLCI was divided into three categories (Tier #2) represented by three indicators (Tier #3) as presented in the following subsections.
Environmental energy sustainability, IEENV, covers the following indicators:
The category for environmental energy sustainability collects indicators of CO2 emissions per kilowatt-hour of electrical energy produced, IEENV1;
Thermal fuel savings, IEENV2;
Energy intensity, IEENV3, creating positive economic and social effects. These indicators focus on maintaining and sustaining the energy quality through energy resource use and eco-friendly technology.
Economic energy sustainability, IEECO, covers the following indicators:
Costs related as life cycle cost (LCC), IEECO1;
Energy payback period, IEECO2;
Emission payback period, IECO3, which preserves positive economic business effects.
It combinates the reduction of energy consumption from non-renewable resources, an increase in energy use from renewable resources, and a rise in energy efficiency simultaneously.
Social energy sustainability, IESOC, covers the following indicators that involve wider social benefits offered for employees that can be derived from delivering energy-efficient investment:
Employment of provision, ISOC1;
The energy of plant labor, ISOC2;
A potential impact of risk allocation on discount rate development per investment, ISOC3.
A description of the nine categorized indicators in terms of the sustainability dimensions is framed in the hierarchical structure of the energy life cycle and outlined in Table 2.
The dependencies shown in the network structure between the aggregated aspects of sustainability and between them are considered and the global index, PESUS, is derived. Any changes in any sustainability dimension affect the other two pillars and have an impact on the value of energy sustainable performance index PESUS simultaneously (Tier #1). The aggregated global index is calculated based on fuzzy numbers representing linguistic scale. Therefore, with regards to Tier #2, indicators are aggregated and normalized for each sustainability aspect separately (environmental energy sustainability, IEENV; economic energy sustainability, IEECO; social energy sustainability, IESOC). Concerning the separate dimensions, indicators (represented by Tier #3) involve various biodigester-related indices like IEENV1 and IEENV2, ESOC1, which are interrelated with each other across the sustainability aspects. These indicators can be derived from delivering energy-efficient investment through the energy sustainability evaluation. Dashed lines symbolize the impacts of indicators among them. Solid lines symbolize the inclusion of indicators from all triple bottom line aspects into the final assessment. Rectangles symbolize those specific indicators that are aggregated on the level of sustainability dimension (economical, environmental, social) and then aggregated as PESUS. Indicators depicted in rectangles are collected within the eLCI framework.
Input data for the sustainability evaluation were calculated and depicted in a hierarchical structure of energy LCA-related indicators (Table 2).
In further analysis, the collection of variables (the energy inventory database) is converted into eLCIs (further also energy LCA-based indicators) by analyzing the contents of the energy audit report carried out by one of the paper`s authors. Then, the collection is aggregated to correspond to sustainability dimensions: three environmental, three economic, and three social indices. Nine indicators within the sustainability evaluation (regarding economic, environmental, and social sustainability) have been considered. These data (indicators) were classified into three criterion tiers (Tier #2): environmental energy sustainability IEENV, economic energy sustainability IEECO, and social energy sustainability IESOC.
Potential indicators like energy subsidies, which will be embodied within the economic dimension, may be economically costly to the potential end-user and taxpayers influencing a final value of economic energy sustainability, IEECO, thus affecting the energy sustainability performance index in the current study. Simultaneously, it can lead to increased GHG emissions into the atmosphere. A choice of indicators related to energy subsidies determinates the development level of economies and their engagement in energy subsidy reforms. Therefore, the report of Vagliasindi (2013) presents criteria and their dependencies (income per capita and net energy) as the key drivers in 20 developing countries. Access to subsidies may pose different challenges to the authors’ global energy sustainability performance index concerning the distributional impact of such reforms on economies (Li, 2018). For example, in Ecuador, small farmers have normally been installing biodigesters in the framework of projects with subsidies (Herrero et al., 2019).
Use of Linguistics Scales and Fuzzy Numbers for Expert’s Evaluation
Although the energy life cycle–objective led indicator values corresponding to the cogeneration technology are quantifiable, the decision-making cannot be clearly defined due to lack of comparability between other similar technologies. Due to this, fuzzy numbers are used as a representation of linguistic scales (Gladysz and Kluczek, 2017). There is no similarity in the definition of evaluation of indicators (variables) in the linguistic values with five terms or fuzzy numbers (very good, VG; good, G; average, A; bad, B; and very bad, VP). It means a 5-point linguistic scale (very) is used to evaluate the plant’s energy performance. Typical Likert scale from 1 to 5 was used as it is proved in some research that such scale allows operationalizing experts’ perceptions, when linguistically assessing options measured in different units, further not engaging to their minds when the items on the scale carry the statement of ideas near the truth for these experts from the field of biodigestion. A more dense scale is not easy to use and experts do not feel the difference between close values. On the other hand, there is no need to use the extended scale for a few indicators. So, the Likert scale of 1 to 5 seems to be reasonable allowing experts to easily operationalize their perceptions, and to understand the interpretation and analysis of data derived from this scale. Alternatively, decision-makers can choose between scales they feel reasonable for their specific case. For example, the scale 1–10 could be chosen because it allows better diversification of assessments if it is feasible from experts’ perspective to assess with more dense scales.
A panel of three trusted and independent experts (1 operational manager, 1 scientist, and a laborer associated with the energy generation) has been selected. In this context, a purposive random selection of experts has been used. Experts agreed on the scale used (Table 3).
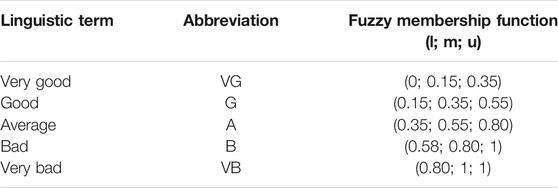
TABLE 3. Linguistic evaluation and triangular fuzzy number for defining fuzzy sustainability indicators in the CHP system assessment
Variables are determined based on the knowledge of the university`s energy experts. Linguistic terms and corresponding triangular fuzzy numbers for assessing the biodigester system are illustrated in Table 3.
To interpret any crisp input value of indicators considered in the previous step, “the determination of the membership values of each crisp input to all linguistic terms” (Mofarrah, 2010; Tulbure and Jischa, 2014). Due to the characteristics of the environmental dimension, not the same linguistic values are used, whereas the same linguistic values are adopted for indicators (Table 3) within the economic and social dimensions. In this stage, the numerical values will be converted into linguistic scale. After linguistic evaluation of the sustainability dimensions, fuzzy mean values are calculated separately for indicators corresponding to each dimension. In this paper, the fuzzy mean value of a fuzzy set is calculated separately according to Dubois and Prade (1987). Dubois and Prade defined a fuzzy mean as follows: “the mean value of a fuzzy interval Q is a closed interval bounded by the expectations calculated from its upper and lower distribution functions” (Dubois and Prade, 1987).
The output of the model from fuzzy numbers into non-fuzzy values (a single numerical measure) was transformed. Then, a crisp mean evaluation of the sustainability in terms of each dimension is calculated. Hence, the center of sums measure is considered as sustainable or unsustainable using the fuzzy numbers for the associated dimensions. Crisp values are obtained using the mathematical expressions (see Table 3, the last three columns) of defuzzification.
Using an expert evaluation system, Table 4 also contains the judgment decision-making matrix. The input values (energy indicators) corresponding to the environmental, economic, and social criteria are related to the expert evaluation and are given fuzzy mean values. In this case, the fuzzy sets for the description of the criteria IEENV, IEECO, and IESOC are derived from corresponding crisp partitions, leading to the determination of crisp values. Each criterion (from Tier #2) has been established by the three aggregated indicators, as shown in Table 3.
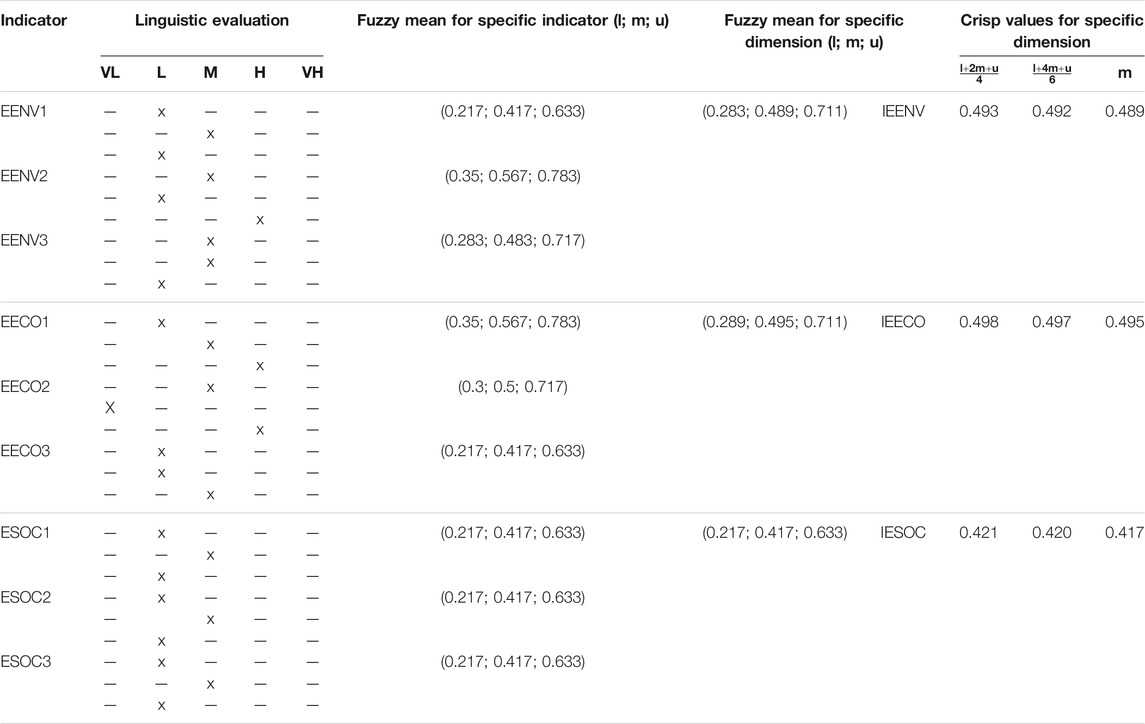
TABLE 4. Sustainability technology evaluation based on criteria for the considered biodigester using membership functions of variables
Results
Assessment is performed to calculate the sustainability performance evaluation index (p and q) for two scenarios. This framework was modeled based on a three-dimensional orthogonal Cartesian, where each axis is represented by the three sustainability dimensions. The value of q characterizes the area of triangle IESOC–IEENV–IEECO (Figure 4), which vertexes are (IEENV; 0; 0) and (0; IEECO; 0) and (0; 0; IESOC).
Energy sustainability performance evaluation is depicted as the field (q) within the triangle characterized by the crisp numbers representing environmental, economic, and social performance IEENV, IEECO, and IESOC, respectively (see Figure 4). The new proposed interpretation is the p indicator given by Eq. (1). It is the distance of point P (IEENV, IEECO, IESOC) from the coordinate system origin (Figure 3). Each cross-dimension described (IEENVIESOC, IEENVIEECO, IEECOIESOC) represents a combination of its fuzzy indicators, e.g., environmental performance is calculated as the length of vectors of indicators, expressed by Eqs 2–4. The measure of energy sustainable performance is visualized in the three-dimensional Cartesian orthogonal plot. To measure energy sustainability performance, crisp values are taken. Higher evaluation [01] of impact indicators relates to a greater energy sustainability performance. An analysis of results is depicted in Figure 3 (p) and Figure 4 (q), where a measure of energy sustainable performance is visualized on a Cartesian orthogonal plot. Each axis of sustainability is represented by an integrated indicator and is pictured by one of the three axes of the sustainability concept.
Performances in terms of specified characteristics were assessed linguistically by experts and represented as fuzzy numbers using the scale depicted in Table 2. There are no significant differences between values obtained using different defuzzification equations (Table 4). Therefore, for further computations, the most popular formula, i.e.,
The energy-related sustainable performance indices (p and q), both represented by the three aggregated sustainability dimensions, were calculated according to Eqs 1, 5. Those are crisp values from 0 to 1 with no physical units because they are derived from the defuzzification of fuzzy numbers calculated from experts’ linguistic judgments. Therefore, evaluation of energy sustainable performance for both scenarios (p and q) was expressed based on the crisp values, presented for each sustainability component. For the computation of crisp values for each dimension energy, life cycle indicators were used to embody a calculation of a value of p by evaluation of energy sustainable performance.
Equations 2–4 describe the distance between the points in the coordinate system XYZ (Eq. 2—length of the IEENVIESOC segment; Eq. 3—the length of the IESOCIEECO segment; Eq. 4—the length of the IEENVIEECO segment). Knowing the values of segments (IEENVIESOC, IESOCIEECO, IEENVIEECO), the value of q is calculated as a field of triangle IESOCIEENVIEECO where mentioned segments are its sides. A total value of p for energy sustainable performance is treated as the distance from the origin of the coordinate system. It means the length of the vector with the beginning (0; 0; 0) and the end (x; y; z). For the q, a triangle was calculated based on the values of points in the coordinate axes.
Discussion
The paper presents energy sustainability performance expressed as a function of the energy sustainability transition index to orient decision-making toward the evaluation of technology based on which rational/optimal decisions on energy-related investments are being made. The idea of the paper was not to provide the results for the specific industrial case, but an attempt to briefly depict the potential application of the energy sustainability evaluation framework modeling various parameters for biogas production. Therefore, the article constructed the evaluation method focusing on a variety of quantitative (actual data) and qualitative indicators based on the fuzzy decision-making process. The authors outlined two ways of calculating energy sustainability performance (p and q), each presenting a different way of calculation. Therefore, they are different in a way of their estimation, data collection, and finally analysis and interpretation.
The presented new approach for assessing energy sustainability performance has a significant advantage over the similar method discussed in the literature (Lucato et al., 2018). The author depicted and expressed the sustainability level of a manufacturing process in a single combined measure considering the three aspects of sustainability.
Such an approach to the calculation of q was adapted grounding on the previous research of Lucato et al. (2018). The limitation of the approach presented in Lucato et al. (2018) was the calculation of final measures that were based on indicators with different measurement units. This serious methodological drawback was eliminated in the authors’ approach by using experts’ linguistic evaluation based on quantified data for the considered indices. Therefore, the idea for estimation of q is enriched compared with the original work (Lucato et al., 2018) and eliminates the problem of inconsistent units of indices. Contrary to the aforementioned idea of q, this paper introduces a new approach based on the calculation of p as the distance of the point (IEENV; IEECO; IESOC) from the origin of the Cartesian coordinate system. Both approaches are based on the same data, but they differ in ease of analysis and interpretation.
In the initial analysis (Lucato et al., 2018), the indicator q seems to be difficult to understand causing intricacy and retaining a lot of ambiguity in its interpretation. So, an output achieved based on the value of p is more simplified, which can be an advantage for the authors’ study, thus overcoming the constraints of using different measurement units across the indices considered for all sustainability dimensions. A possibility of the implication of eLCIs based on fuzzy numbers, which are characterized by different functional (measurement) units, allows avoiding bias judgment providing the additional advantage. In addition, the advantage of both approaches presented in this paper (q and p) to the sustainability evaluation over the starting point (Lucato et al., 2018) is the possibility to use various measurement units for defining eLCIs based on fuzzy numbers. The inclusion of fuzzy numbers for the calculation of a general performance indicator p might make the decision-making process more feasible, whereas gathering energy data can be used for making deep energy flow analyses (minimization or maximization) to identify potential retrofitting technologies or improvements in processes across facilities. In this context, the energy data collection with the integration of LCA-based indicators might help decision-makers to assess the effect of facilities to increase the acceptance of investment in renewable energy technologies as was environmental impact analyses that were performed for the bioenergy systems (Paletto et al., 2019).
Results of analyses for p and q were discussed with experts involved in evaluations of indices (see Table 4). All of them concluded the advantage of p over q in terms of ease of its interpretation. The proposed evaluation method p seems to be promising when dealing with many “fuzzy” indicators (assessed with linguistic sales and corresponding fuzzy numbers) to make more accurate outputs. Interpreting p has also an advantage over q in terms of presenting and comparing the dynamics of values in time. For p, it is possible to make considerations of different values and compare them using a single chart, while for q, a single chart with more than one indicator pictured (as a triangle) easily becomes unreadable and hard to understand.
The LCA-based measures might be flexible with the use of indicators to change parameters depending on the profile of industrial plants. Hence, a reusage of the LCA-based measures constitutes the plant’s business circularity. Introducing energy LCA-based indicators based on fuzzy numbers as drivers for evaluation of sustainable energy performance may allow decision-makers to overcome human error, ambiguity, and uncertainties in calculations in the decision-making processes as stated in Onar et al. (2015) and Zhang et al. (2016). These indicators could be used for effective assessment due to the robustness of the LCA to meet Agenda 2030 goals (Firoiu et al., 2019; Siksnelyte et al., 2019) and they contribute to the present literature on energy indicators (Streimikiene et al., 2007; Feiz et al., 2020) as well as sustainability evaluation methods based on life cycles (Wulf et al., 2019; Bjørn et al., 2020) or dedicated for the energy sector (Stamford, 2020) at the same time.
A small number of judgments and LCA-based indicators involved in the energy sustainability performance evaluation generate limitations of the research and make the method no longer valid and justified. Nevertheless, the evaluation method could be applicable in any kind of organization providing flexibility in use and monitoring of performance progress at any time.
While the positive side of the method was to consider the relations between the indicators, the limitation of the assessment method is the application within a specific sector, in this case for assessing biogas plants only. This fact leads to a narrow list of industrial plants making it impossible to use on a larger scale. To overcome this drawback, a larger base of respondents and indicators of energy systems combined with sustainability assessment methods should be examined.
Regarding Analytical Hierarchy Process (AHP) method, according to Troldborg et al. (2014), results achieved depicted high uncertainty due to the application of vague input information. Abdelli et al. (2020) emphasized uncertainty in the data valuation when using AHP based on pairwise comparisons on a ratio scale. Therefore, more focus on MCDA studies addressing the uncertainties might avoid it in the input data when extending other methods within MCDA to operate with fuzzy numbers.
When analyzing other MCDA methods where an aggregate index value is calculated, some weak point was identified such as the lack of interactions between input data, which can affect the whole outcomes (Jasiulewicz-Kaczmarek et al., 2021). Compared with the current research, the authors ensured interaction between criteria and exclusive attributes for the developed energy sustainability performance index. Similar MCDA-based research was done for calculating energy performance indicator, called the base 100 index for the fertilizer plant processes (Valencia et al., 2017). For this case, the index was constructed on specified value constraints for energy consumption data (if the index is greater than 100%, then it translates into a good energy performance).
Historically, the economic dimension was always present in considerations. Then, environmental aspects become widely discussed considering climate change. Therefore, there is rich literature concerning those two sustainability dimensions, but the social dimension is still not widely considered. Most studies on the assessment of energy performance or energy projects deal with economic sustainability or environmental sustainability separately without social inclusion, which is the misleading use of the sustainability approach (Clark and Harley, 2020). Although a high value of social sustainability was obtained in the current study, incorporation of social sustainability might be sufficient for complex energy sustainability assessment. However, the social dimension (IESOC = 0.420) is still ranked lower than two others that are ranked closely (IEECO = 0.497 and IEENV = 0.492).
The empirical research suggests that the considered evaluation method for energy sustainable performance could be applied in energy-related wide scope for effective decision-making and selecting the best suitable energy technology from the sustainability perspective. On the other hand, it might give opportunities to embody the methods in different business models; one of them is a plant`s circular economy by using renewable energy, as presented in Figure 5. The model needs to be validated during the stakeholders’ workshop and by experts involved in this research to make sure of its robustness and accuracy of the model basis. Moreover, it is needed to check the degree to which the past data are an accurate representation of the real data. Considering the consistency and subjectivity of many decisions might cause this verification to be difficult. This business model will allow understanding relations between components within the plant’s circular economy and may lead to the plant’s circular success. In this perspective, the “fuzzy” energy LCA-based indicators also make it possible to carry out comparative evaluation or energy analysis of technologies or manufacturing processes in a user-friendly manner. By using the proposed evaluation method, an increase in competitive advantage in industrial plants could be achieved. Within energy circularity indicators (e.g., fossil energy use, total energy use, and CO2 emission), complementary impact indicators such as waste, materials, pollutants, and toxic emissions might be included. These indicators could be defined to use in effective assessment. More sustainable use of energy can lead to improvement of energy efficiency performance reducing energy consumption and production costs in consequence. The targeted effect is to minimize climate change compliant with SDGs.
Limitations could be twofold:
Limitations in the evaluation framework methods that concern
A wide range of different indicators of various measurement units covering environmental, economic, and social components;
Visualization of the achieved results in terms of the three-dimensional performance in graphical objects;
Judgment of individual energy sustainability side in terms of each sustainability criteria or overall one (based on a score with the worst/great energy performance);
The prevention of trade-off between the sustainability dimensions to provide equal importance, which was recognized in environmental evaluation (Gibson, 2013).
Limitations in the application of the energy sustainable performance method:
Overcoming “the boundaries between research and policy, due to inadequate design or implementation within institutions” (Shortall and Davidsdottir, 2017);
The lack of access to potential plant’s energy data being a beneficiary of the method.
Despite that, the study presents some limitations—the energy evaluation based on “fuzzy” energy LCA indicators might be inspirational for energy decision-makers and sustainability managers in many industrial plants giving a foundation toward energy sustainability. For the effective incorporation of energy sustainability, decision-makers should indicate and promote the best drivers to tackle existing barriers having their impact on other areas of industrial plants in terms of energy sustainability. Hence, the supportive role of the “fuzzy” evaluation method should be highlighted by decision-makers in informing employees about sustainability performance reached and tracking energy LCA-based parameters.
The presented algorithm using linguistic scales with corresponding fuzzy numbers may contribute to the current sustainability assessment framework for assessing sustainable energy, thus filling the gap stressed in the literature (Schrettle et al., 2014). In addition, the universality of the proposed method can be used somewhere where the parameters of different measurements units could not be integrated within the application of the traditional mathematical approach.
This method shows also the opposite side for the traditional assessment approaches (Ness et al., 2007; Fauré et al., 2017; Kumar et al., 2017) focusing on choosing suitable and sustainable indicators based on which evaluation was carried out (Luthra et al., 2017; Siksnelyte et al., 2018). Taking into consideration these literature findings, the presented methodological framework is an original solution aimed at enhancing energy performance assessment for technologies or industrial plants (Vilcekova and Kridlova Burdova, 2014).
Further research would be designed to mainstream into energy policy, which is still missing in the national energy and climate plan mandated by the European Union (Krysiak and Kluczek, 2021) to achieve Agenda 2030 (Firoiu et al., 2019). The implementation of this energy challenge requires governmental decisive actions based on various methods, as well as an adequate response from investors and society. The actions might have significant implications for the shape and condition of industrial plants and help plants’ decision-makers to increase ambition in the decarbonization of their energy systems.
Some possible complex lines of future agenda emerged are as follows:
Data set should be extended to analyze different numbers of criteria and alternatives, followed by the reproduction of the same assessment for biodigester.
The same kind of experiment should be applied using various MCDA methods to gain comparative analysis.
Considering MCDA studies in future research on energy sustainability evaluation, more focus should be put on these specific uncertainties that consider the various types of biodigesters or similar projects based on biomass/bio-resources to obtain more robust results and ensure better-informed decision-making.
Conclusion
The study demonstrated the sustainability evaluation for biodigester in the small-sized biogas plant using the fuzzy numbers, linguistic numbers, and energy LCA indicators under limited information within an uncertain environment. It provides the first evidence for a mechanism underpinning the gained results/outcomes that can be required to apply by the national government to scale up delivered action/interventions, and for contributing to the definition of sustainable bioeconomy based on a coherent assessment. The results achieved might allow suggesting simplification in outcomes, where a typical approach in terms of the problem to solution/outcome was used to produce intended outcomes. That is why the current research does not consider other assessment conditions and should be analyzed in future scale-up efforts.
This framework can be extended to other energy systems giving more flexibility in its application and reliability in results when the uncertainties are considered. It might be useful for the prioritization of plants modeling energy systems shaping a new attitude toward energy sustainability. Thanks to the valuable insight to the evaluation of energy sustainability performance of the biodigester, the calculated p allows making interventions or efforts to concentrate on the improvement of technologies or manufacturing processes. Therefore, it is also possible to compare or benchmark the present indicators as a basis versus improvement scenarios within the energy sustainability assessment. Hence, for further analysis, the study can be extended to similarly sized energy plants dealing with renewable energy sources to provide a comparative energy performance evaluation. On the other hand, the proposed method can be more accurate in its final evaluation if it is combined with other methods or techniques providing the objectivity of the primary assessment. This can help to allow enhancing the manager’s decision-making process by referring to a certain point of reference of the decisional problem. This confirms that the suggested evaluation method might be replacing the existing decision-making methods fostering a positive awareness of industrial practitioners and facilitating emerging bioeconomy enterprises. But before it happens, some specific requirements must be aligned with the condition of industrial plants to achieve a sustainable bioeconomy.
Data Availability Statement
The original contributions presented in the study are included in the article/Supplementary Materials, further inquiries can be directed to the corresponding author.
Author Contributions
AK organized the database. BG performed the numerical analysis. All authors contributed to conception and design of the study, first draft of the article, and article revision, and read and approved the submitted version.
Funding
This research was funded by the Polish National Agency for Academic Exchange under grant no. PPI/APM/2018/1/00047 entitled “Industry 4.0 in Production and Aeronautical Engineering” (International Academic Partnerships Programme). The APC was funded by the Polish National Agency for Academic Exchange.
Conflict of Interest
The authors declare that the research was conducted in the absence of any commercial or financial relationships that could be construed as a potential conflict of interest.
Publisher’s Note
All claims expressed in this article are solely those of the authors and do not necessarily represent those of their affiliated organizations, or those of the publisher, the editors, and the reviewers. Any product that may be evaluated in this article, or claim that may be made by its manufacturer, is not guaranteed or endorsed by the publisher.
Footnotes
1CHP provides economic benefits by reducing dependence on imported fuels being an alternative energy source for conventional power plants.
2In the context of energy consumption decisions when consumers behave according to prospect theory.
References
Abdelli, A., Mokdad, L., and Hammal, Y. (2020). Dealing with Value Constraints in Decision Making Using MCDM Methods. J. Comput. Sci. 44, 101154. doi:10.1016/j.jocs.2020.101154
Akber, M. Z., Thaheem, M. J., and Arshad, H. (2017). Life Cycle Sustainability Assessment of Electricity Generation in Pakistan: Policy Regime for a Sustainable Energy Mix. Energy Policy 111, 111–126. doi:10.1016/j.enpol.2017.09.022
Atilgan, B., and Azapagic, A. (2016). An Integrated Life Cycle Sustainability Assessment of Electricity Generation in Turkey. Energy Policy 93, 168–186. doi:10.1016/j.enpol.2016.02.055
Balibrea-Iniesta, J. (2020). Economic Analysis of Renewable Energy Regulation in France: A Case Study for Photovoltaic Plants Based on Real Options. Energies 13, 2760. doi:10.3390/en13112760
Baumann, M., Weil, M., Peters, J. F., Chibeles-Martins, N., and Moniz, A. B. (2019). A Review of Multi-Criteria Decision Making Approaches for Evaluating Energy Storage Systems for Grid Applications. Renew. Sust. Energ. Rev. 107, 516–534. doi:10.1016/j.rser.2019.02.016
Bhandari, R., Arce, B. E., Sessa, V., and Adamou, R. (2021). Sustainability Assessment of Electricity Generation in Niger Using a Weighted Multi-Criteria Decision Approach. Sustainability 13, 385. doi:10.3390/su13010385
Bjørn, A., Chandrakumar, C., Boulay, A.-M., Doka, G., Fang, K., Gondran, N., et al. (2020). Review of Life-Cycle Based Methods for Absolute Environmental Sustainability Assessment and Their Applications. Environ. Res. Lett. 15, 083001. doi:10.1088/1748-9326/ab89d7
Brundtland (1987). Our Common Future. The Brundtland Report to the World Commission on Environment and Development. Oxford, New York: Oxford University Press.
Butnar, I., Broad, O., Solano Rodriguez, B., and Dodds, P. E. (2020). The Role of Bioenergy for Global Deep Decarbonization: CO 2 Removal or Low-Carbon Energy? GCB Bioenergy 12, 198–212. doi:10.1111/gcbb.12666
Büyüközkan, G., and Karabulut, Y. (2017). Energy Project Performance Evaluation with Sustainability Perspective. Energy 119, 549–560. doi:10.1016/j.energy.2016.12.087
Cabeza, L. F., Rincón, L., Vilariño, V., Pérez, G., and Castell, A. (2014). Life Cycle Assessment (LCA) and Life Cycle Energy Analysis (LCEA) of Buildings and the Building Sector: A Review. Renew. Sust. Energ. Rev. 29, 394–416. doi:10.1016/j.rser.2013.08.037
Cavallaro, F., and Ciraolo, L. (2013). “Sustainability Assessment of Solar Technologies Based on Linguistic Information,” in Assessment and Simulation Tools for Sustainable Energy Systems: Theory and Applications Green Energy and Technology. Editor F. Cavallaro (London: Springer), 3–25. doi:10.1007/978-1-4471-5143-2_1
Çelikbilek, Y., and Tüysüz, F. (2016). An Integrated Grey Based Multi-Criteria Decision Making Approach for the Evaluation of Renewable Energy Sources. Energy 115, 1246–1258. doi:10.1016/j.energy.2016.09.091
Choudhary, D., and Shankar, R. (2012). An STEEP-Fuzzy AHP-TOPSIS Framework for Evaluation and Selection of thermal Power Plant Location: A Case Study from India. Energy 42, 510–521. doi:10.1016/j.energy.2012.03.010
Cinelli, M., Coles, S. R., and Kirwan, K. (2014). Analysis of the Potentials of Multi Criteria Decision Analysis Methods to Conduct Sustainability Assessment. Ecol. Indicators 46, 138–148. doi:10.1016/j.ecolind.2014.06.011
Clark, W. C., and Harley, A. G. (2020). Sustainability Science: Toward a Synthesis. Annu. Rev. Environ. Resour. 45, 331–386. doi:10.1146/annurev-environ-012420-043621
Çolak, M., and Kaya, İ. (2017). Prioritization of Renewable Energy Alternatives by Using an Integrated Fuzzy MCDM Model: A Real Case Application for Turkey. Renew. Sust. Energ. Rev. 80, 840–853. Available at: https://ideas.repec.org/a/eee/rensus/v80y2017icp840-853.html (Accessed December 11, 2020).
Data and statistics (2021). CO2 Emissions Statistics – Data Services IEA. Available at: https://www.iea.org/subscribe-to-data-services/co2-emissions-statistics (Accessed January 9, 2021).
Dhillon, B. S. (2009). Life Cycle Costing for Engineers. Boca Raton, FL: CRC Press. doi:10.1201/9781439816899
Domínguez-Dafauce, L. C., and Martín, F. M. (2015). Sustainable and Renewable Implementation Multi-Criteria Energy Model (SRIME)-case Study: Sri Lanka. Int. J. Energ. Environ Eng 6, 165–181. doi:10.1007/s40095-015-0164-2
Dubois, D., and Prade, H. (1987). The Mean Value of a Fuzzy Number. Fuzzy Sets Syst. 24, 279–300. doi:10.1016/0165-0114(87)90028-5
Estévez, R. A., Espinoza, V., Ponce Oliva, R. D., Vásquez-Lavín, F., and Gelcich, S. (2021). Multi-Criteria Decision Analysis for Renewable Energies: Research Trends, Gaps and the Challenge of Improving Participation. Sustainability 13, 3515. doi:10.3390/su13063515
European Commission (2016). Clean Energy for All Europeans. Communication from the Commission to the European Parliament. the Council, the European Economic and Social Committee, the Committee of the Regions and the European Investment Bank.
Fauré, E., Arushanyan, Y., Ekener, E., Miliutenko, S., and Finnveden, G. (2017). Methods for Assessing Future Scenarios from a Sustainability Perspective. Eur. J. Futures Res. 5. doi:10.1007/s40309-017-0121-9
Feiz, R., Johansson, M., Lindkvist, E., Moestedt, J., Påledal, S. N., and Svensson, N. (2020). Key Performance Indicators for Biogas Production-Methodological Insights on the Life-Cycle Analysis of Biogas Production from Source-Separated Food Waste. Energy 200, 117462. doi:10.1016/j.energy.2020.117462
Firoiu, D., Ionescu, G. H., Băndoi, A., Florea, N. M., and Jianu, E. (2019). Achieving Sustainable Development Goals (SDG): Implementation of the 2030 Agenda in Romania. Sustainability 11, 2156. doi:10.3390/su11072156
Ghafghazi, S., Sowlati, T., Sokhansanj, S., and Melin, S. (2010). A Multicriteria Approach to Evaluate District Heating System Options. Appl. Energ. 87, 1134–1140. doi:10.1016/j.apenergy.2009.06.021
Gibson, R. B. (2013). Avoiding Sustainability Trade-Offs in Environmental Assessment. Impact Assess. Project Appraisal 31, 2–12. doi:10.1080/14615517.2013.764633
Gielen, D., Boshell, F., Saygin, D., Bazilian, M. D., Wagner, N., and Gorini, R. (2019). The Role of Renewable Energy in the Global Energy Transformation. Energ. Strategy Rev. 24, 38–50. doi:10.1016/j.esr.2019.01.006
Gladysz, B., and Kluczek, A. (2017). A Framework for Strategic Assessment of Far-Reaching Technologies: A Case Study of Combined Heat and Power Technology. J. Clean. Prod. 167, 242–252. doi:10.1016/j.jclepro.2017.08.175
Herrero, J. M., Cuji, P., Ramírez, V., Rodríguez, L., Domínguez, D. L., and Cipriano, J. (2019). Towards a Sustainable Biodigester Sector in Ecuador: Inputs for a Biodigester Component of the PNBE. Online: CTCN-CIMNE-IIGE-INIAP Available at: https://www.ctc-n.org/system/files/dossier/3b/EN_R4.1-20191210_Programa%20nacional%20de%20biodigestores%20en%20Ecuador-CTCN.pdf (Accessed January 28, 2022).
Hosseini-Fashami, F., Motevali, A., Nabavi-Pelesaraei, A., Hashemi, S. J., and Chau, K.-w. (2019). Energy-Life Cycle Assessment on Applying Solar Technologies for Greenhouse Strawberry Production. Renew. Sust. Energ. Rev. 116, 109411. doi:10.1016/j.rser.2019.109411
Iddrisu, I., and Bhattacharyya, S. C. (2015). Sustainable Energy Development Index: A Multi-Dimensional Indicator for Measuring Sustainable Energy Development. Renew. Sust. Energ. Rev. 50, 513–530. doi:10.1016/j.rser.2015.05.032
IEA (2019). Perspectives for the Clean Energy Transition – Analysis. IEA Available at: https://www.iea.org/reports/the-critical-role-of-buildings (Accessed January 3, 2021).
Jasiulewicz-Kaczmarek, M., Żywica, P., and Gola, A. (2021). Fuzzy Set Theory Driven Maintenance Sustainability Performance Assessment Model: a Multiple Criteria Approach. J. Intell. Manuf 32, 1497–1515. doi:10.1007/s10845-020-01734-3
Javed, M. S., Ma, T., Jurasz, J., and Mikulik, J. (2021). A Hybrid Method for Scenario-Based Techno-Economic-Environmental Analysis of Off-Grid Renewable Energy Systems. Renew. Sust. Energ. Rev. 139, 110725. doi:10.1016/j.rser.2021.110725
Kannan, R., Leong, K. C., Osman, R., and Ho, H. K. (2007). Life Cycle Energy, Emissions and Cost Inventory of Power Generation Technologies in Singapore. Renew. Sust. Energ. Rev. 11, 702–715. doi:10.1016/j.rser.2005.05.004
Kaya, T., and Kahraman, C. (2010). Multicriteria Renewable Energy Planning Using an Integrated Fuzzy VIKOR & AHP Methodology: The Case of Istanbul. Energy 35, 2517–2527. doi:10.1016/j.energy.2010.02.051
Kluczek, A. (2019). An Energy-Led Sustainability Assessment of Production Systems - an Approach for Improving Energy Efficiency Performance. Int. J. Prod. Econ. 216, 190–203. doi:10.1016/j.ijpe.2019.04.016
Kluczek, A. (2018). Dynamic Energy LCA-Based Assessment Approach to Evaluate Energy Intensity and Related Impact for the Biogas CHP Plant as the Basis of the Environmental View of Sustainability. Proced. Manufacturing 21, 297–304. doi:10.1016/j.promfg.2018.02.124
Krysiak, M., and Kluczek, A. (2021). A Multifaceted Challenge to Enhance Multicriteria Decision Support for Energy Policy. Energies 14, 4128. doi:10.3390/en14144128
Kumar, A., Sah, B., Singh, A. R., Deng, Y., He, X., Kumar, P., et al. (2017). A Review of Multi Criteria Decision Making (MCDM) towards Sustainable Renewable Energy Development. Renew. Sust. Energ. Rev. 69, 596–609. doi:10.1016/j.rser.2016.11.191
Li, T., Roskilly, T., and Wang, Y. (2016). A Life Cycle Approach to Sustainability Assessment on Community Energy Projects in the UK.
Li, X. (2018). Efficiency Measures of the Chinese Biogas Project Subsidy Policy Using an Additive Two Stage DEA. J. Renew. Sust. Energ. 10, 015907. doi:10.1063/1.5008589
Lucato, W., Santos, J., and Pacchini, A. (2018). Measuring the Sustainability of a Manufacturing Process: A Conceptual Framework. Sustainability 10, 81. doi:10.3390/su10010081
Luthra, S., Govindan, K., Kannan, D., Mangla, S. K., and Garg, C. P. (2017). An Integrated Framework for Sustainable Supplier Selection and Evaluation in Supply Chains. J. Clean. Prod. 140, 1686–1698. doi:10.1016/j.jclepro.2016.09.078
Mahmood, S., Saman, M. Z. M., and Yusof, N. M. (2015). Proposed Framework for Assessing the Sustainability of Membrane Life Cycle. Proced. CIRP 26, 35–39. doi:10.1016/j.procir.2014.07.171
Mardani, A., Jusoh, A., Zavadskas, E., Cavallaro, F., and Khalifah, Z. (2015). Sustainable and Renewable Energy: An Overview of the Application of Multiple Criteria Decision Making Techniques and Approaches. Sustainability 7, 13947–13984. doi:10.3390/su71013947
Marinescu, N. (2020). Changes in Renewable Energy Policy and Their Implications: The Case of Romanian Producers. Energies 13, 6493. doi:10.3390/en13246493
Martin, M., Røyne, F., Ekvall, T., and Moberg, Å. (2018). Life Cycle Sustainability Evaluations of Bio-Based Value Chains: Reviewing the Indicators from a Swedish Perspective. Sustainability 10, 547. doi:10.3390/su10020547
Maxim, A. (2014). Sustainability Assessment of Electricity Generation Technologies Using Weighted Multi-Criteria Decision Analysis. Energy Policy 65, 284–297. doi:10.1016/j.enpol.2013.09.059
May, J. R., and Brennan, D. J. (2006). Sustainability Assessment of Australian Electricity Generation. Process Saf. Environ. Prot. 84, 131–142. doi:10.1205/psep.04265
McCollum, D. L., Echeverri, L. G., Busch, S., Pachauri, S., Parkinson, S., Rogelj, J., et al. (2018). Connecting the Sustainable Development Goals by Their Energy Inter-linkages. Environ. Res. Lett. 13, 033006. doi:10.1088/1748-9326/aaafe3
Mofarrah, A., and Husain, T. (2010). Methodology for Environmental Impact Assessment: A Fuzzy Logic Based Approach. doi:10.2316/P.2010.699-015
Moslehi, S., and Arababadi, R. (2016). Sustainability Assessment of Complex Energy Systems Using Life Cycle Approach-Case Study: Arizona State University Tempe Campus. Proced. Eng. 145, 1096–1103. doi:10.1016/j.proeng.2016.04.142
Ness, B., Urbel-Piirsalu, E., Anderberg, S., and Olsson, L. (2007). Categorising Tools for Sustainability Assessment. Ecol. Econ. 60, 498–508. Available at: https://econpapers.repec.org/article/eeeecolec/v_3a60_3ay_3a2007_3ai_3a3_3ap_3a498-508.htm (Accessed October 25, 2021). doi:10.1016/j.ecolecon.2006.07.023
Ninno Muniz, R., Frizzo Stefenon, S., Gouvêa Buratto, W., Nied, A., Meyer, L. H., Finardi, E. C., et al. (2020). Tools for Measuring Energy Sustainability: A Comparative Review. Energies 13, 2366. doi:10.3390/en13092366
Nzila, C., Dewulf, J., Spanjers, H., Tuigong, D., Kiriamiti, H., and van Langenhove, H. (2012). Multi Criteria Sustainability Assessment of Biogas Production in Kenya. Appl. Energ. 93, 496–506. doi:10.1016/j.apenergy.2011.12.020
Onar, S. C., Oztaysi, B., Otay, İ., and Kahraman, C. (2015). Multi-expert Wind Energy Technology Selection Using Interval-Valued Intuitionistic Fuzzy Sets. Energy 90, 274–285. doi:10.1016/j.energy.2015.06.086
Onat, N., Kucukvar, M., Halog, A., and Cloutier, S. (2017). Systems Thinking for Life Cycle Sustainability Assessment: A Review of Recent Developments, Applications, and Future Perspectives. Sustainability 9, 706. doi:10.3390/su9050706
Paletto, A., Bernardi, S., Pieratti, E., Teston, F., and Romagnoli, M. (2019). Assessment of Environmental Impact of Biomass Power Plants to Increase the Social Acceptance of Renewable Energy Technologies. Heliyon 5, e02070. doi:10.1016/j.heliyon.2019.e02070
Peng, J.-j., Tian, C., Zhang, W.-y., Zhang, S., and Wang, J.-q. (2020). An Integrated Multi-Criteria Decision-Making Framework for Sustainable Supplier Selection under Picture Fuzzy Environment. Technol. Econ. Dev. Economy 26, 573–598. doi:10.3846/tede.2020.12110
Pope, J., Bond, A., Hugé, J., and Morrison-Saunders, A. (2017). Reconceptualising Sustainability Assessment. Environ. Impact Assess. Rev. 62, 205–215. doi:10.1016/j.eiar.2016.11.002
Rebitzer, G., Ekvall, T., Frischknecht, R., Hunkeler, D., Norris, G., Rydberg, T., et al. (2004). Life Cycle Assessment. Environ. Int. 30, 701–720. doi:10.1016/j.envint.2003.11.005
Ren, H., Zhou, W., Nakagami, K. i., Gao, W., and Wu, Q. (2010). Multi-objective Optimization for the Operation of Distributed Energy Systems Considering Economic and Environmental Aspects. Appl. Energ. 87, 3642–3651. doi:10.1016/j.apenergy.2010.06.013
Richard Hern Daniel Radov Alon Carmel Marija Spasovska Jinzi Guo, S. (2015). Lot 1: Hurdle Rates Update for Generation Technologies, 109.Electricity Generation Costs and Hurdle Rates
Rogge, K. S., and Reichardt, K. (2016). Policy Mixes for Sustainability Transitions: An Extended Concept and Framework for Analysis. Res. Pol. 45, 1620–1635. doi:10.1016/j.respol.2016.04.004
Ross, T. (2016). Fuzzy Logic with Engineering Applications. 4th Edition. USA. Wiley Available at: https://www.wiley.com/en-us/Fuzzy+Logic+with+Engineering+Applications%2C+4th+Edition-p-9781119235866 (Accessed January 7, 2021).
Santoyo-Castelazo, E., and Azapagic, A. (2014). Sustainability Assessment of Energy Systems: Integrating Environmental, Economic and Social Aspects. J. Clean. Prod. 80, 119–138. doi:10.1016/j.jclepro.2014.05.061
Scarlat, N., Dallemand, J.-F., and Fahl, F. (2018). Biogas: Developments and Perspectives in Europe. Renew. Energ. 129, 457–472. doi:10.1016/j.renene.2018.03.006
Scarlat, N., Dallemand, J.-F., Monforti-Ferrario, F., and Nita, V. (2015). The Role of Biomass and Bioenergy in a Future Bioeconomy: Policies and Facts. Environ. Dev. 15, 3–34. doi:10.1016/j.envdev.2015.03.006
Schrettle, S., Hinz, A., Scherrer -Rathje, M., and Friedli, T. (2014). Turning Sustainability into Action: Explaining Firms' Sustainability Efforts and Their Impact on Firm Performance. Int. J. Prod. Econ. 147, 73–84. doi:10.1016/j.ijpe.2013.02.030
Shortall, R., and Davidsdottir, B. (2017). How to Measure National Energy Sustainability Performance: An Icelandic Case-Study. Energ. Sustain. Dev. 39, 29–47. doi:10.1016/j.esd.2017.03.005
Siksnelyte, I., Zavadskas, E. K., Bausys, R., and Streimikiene, D. (2019). Implementation of EU Energy Policy Priorities in the Baltic Sea Region Countries: Sustainability Assessment Based on Neutrosophic MULTIMOORA Method. Energy Policy 125, 90–102. doi:10.1016/j.enpol.2018.10.013
Siksnelyte, I., Zavadskas, E., Streimikiene, D., and Sharma, D. (2018). An Overview of Multi-Criteria Decision-Making Methods in Dealing with Sustainable Energy Development Issues. Energies 11, 2754. doi:10.3390/en11102754
Stamford, L. (2020). “Life Cycle Sustainability Assessment in the Energy Sector,” in Biofuels for a More Sustainable Future. Editors J. Ren, A. Scipioni, A. Manzardo, and H. Liang (Elsevier), 115–163. doi:10.1016/B978-0-12-815581-3.00005-1
Streimikiene, D., Ciegis, R., and Grundey, D. (2007). Energy Indicators for Sustainable Development in Baltic States. Renew. Sust. Energ. Rev. 11, 877–893. doi:10.1016/j.rser.2005.06.004
Taylan, O., Alamoudi, R., Kabli, M., AlJifri, A., Ramzi, F., and Herrera-Viedma, E. (2020). Assessment of Energy Systems Using Extended Fuzzy AHP, Fuzzy VIKOR, and TOPSIS Approaches to Manage Non-cooperative Opinions. Sustainability 12, 2745. doi:10.3390/su12072745
Troldborg, M., Heslop, S., and Hough, R. L. (2014). Assessing the Sustainability of Renewable Energy Technologies Using Multi-Criteria Analysis: Suitability of Approach for National-Scale Assessments and Associated Uncertainties. Renew. Sust. Energ. Rev. 39, 1173–1184. doi:10.1016/j.rser.2014.07.160
Tulbure, I., and Jischa, M. F. (2014). Fuzzy Logic Based Approach for Environmental Impact Assessment, 14.
Us Epa, O. (2015). Fuel and Carbon Dioxide Emissions Savings Calculation Methodology for Combined Heat and Power Systems. US EPA. Available at: https://www.epa.gov/chp/fuel-and-carbon-dioxide-emissions-savings-calculation-methodology-combined-heat-and-power (Accessed January 9, 2021).
Vagliasindi, M. (2013). Implementing Energy Subsidy Reforms : Evidence from Developing Countries. Available at: https://openknowledge.worldbank.org/handle/10986/11965?locale-attribute=en (Accessed January 27, 2022).
Valencia, G., Cardenas Escorcia, Y., Ramos, E., Morales, A., Campos, J., and Ochoa, V. (2017). Energy Saving in Industrial Process Based on the Equivalent Production Method to Calculate Energy Performance Indicators. Chem. Eng. Trans. 57, 713–714. doi:10.3303/CET1757119
Varun, V., Bhat, I. K., and Prakash, R. (2009). LCA of Renewable Energy for Electricity Generation Systems-A Review. Renew. Sust. Energ. Rev. 13, 1067–1073. doi:10.1016/j.rser.2008.08.004
Vilcekova, S., and Kridlova Burdova, E. (2014). Multi-criteria Analysis of Building Assessment Regarding Energy Performance Using a Life-Cycle Approach. Int. J. Energ. Environ Eng 5, 83. doi:10.1007/s40095-014-0083-7
Wang, R., Xiong, J., He, M.-f., Gao, L., and Wang, L. (2020). Multi-objective Optimal Design of Hybrid Renewable Energy System under Multiple Scenarios. Renew. Energ. 151, 226–237. doi:10.1016/j.renene.2019.11.015
Wang, Y., Wu, X., Tong, X., Li, T., and Wu, F. (2018). Life Cycle Assessment of Large-Scale and Household Biogas Plants in Northwest China. J. Clean. Prod. 192, 221–235. doi:10.1016/j.jclepro.2018.04.264
Wu, Y., Ke, Y., Xu, C., and Li, L. (2019). An Integrated Decision-Making Model for Sustainable Photovoltaic Module Supplier Selection Based on Combined Weight and Cumulative prospect Theory. Energy 181, 1235–1251. doi:10.1016/j.energy.2019.06.027
Wulf, C., Werker, J., Ball, C., Zapp, P., and Kuckshinrichs, W. (2019). Review of Sustainability Assessment Approaches Based on Life Cycles. Sustainability 11, 5717. doi:10.3390/su11205717
Zamagni, A. (2012). Life Cycle Sustainability Assessment. Int. J. Life Cycle Assess. 17, 373–376. doi:10.1007/s11367-012-0389-8
Zhang, H., Kou, G., and Peng, Y. (2019). Soft Consensus Cost Models for Group Decision Making and Economic Interpretations. Eur. J. Oper. Res. 277, 964–980. doi:10.1016/j.ejor.2019.03.009
Keywords: energy sustainability, energy evaluation, fuzzy numbers, life cycle assessment, indicators, energy-objective led indicators
Citation: Kluczek A and Gladysz B (2022) Energy Sustainability Performance Index of Biodigester Using Energy LCA-Based Indicators. Front. Energy Res. 10:848584. doi: 10.3389/fenrg.2022.848584
Received: 04 January 2022; Accepted: 03 February 2022;
Published: 16 March 2022.
Edited by:
Joni Jupesta, Research Institute of Innovative Technology for the Earth, JapanReviewed by:
Idiano D'Adamo, Sapienza University of Rome, ItalyGrigorios L. Kyriakopoulos, National Technical University of Athens, Greece
Copyright © 2022 Kluczek and Gladysz. This is an open-access article distributed under the terms of the Creative Commons Attribution License (CC BY). The use, distribution or reproduction in other forums is permitted, provided the original author(s) and the copyright owner(s) are credited and that the original publication in this journal is cited, in accordance with accepted academic practice. No use, distribution or reproduction is permitted which does not comply with these terms.
*Correspondence: Bartlomiej Gladysz, bartlomiej.gladysz@pw.edu.pl