- 1Chinese Center for Disease Control and Prevention, National Institute for Nutrition and Health, Beijing, China
- 2School of Behavioural and Health Sciences, Australian Catholic University, Fitzroy, VIC, Australia
- 3Faculty of Medicine, Dentistry and Health Sciences, University of Melbourne, Melbourne, VIC, Australia
- 4Department of Nutrition, Harvard T. H. Chan School of Public Health, Boston, MA, United States
- 5Institute of Food and Nutrition Development, Ministry of Agriculture and Rural Affairs, Beijing, China
- 6Department of Pediatrics, Chongqing Children's Hospital, Chongqing, China
- 7School of Public Health, Fudan University, Shanghai, China
- 8Department of Nutrition and Food Hygiene, Public Health College, Harbin Medical University, Harbin, China
- 9Department of Public Health, Shandong University, Jinan, China
- 10School Health Department, Guangzhou Center for Disease Control and Prevention, Guangzhou, China
- 11Institute of Child and Adolescent Health, School of Public Health, Peking University, Beijing, China
- 12Department of Nutrition and Food Hygiene, School of Public Health, Peking University, Beijing, China
Background: Findings for associations between cardiorespiratory fitness (CRF) and cardiometabolic risk (CMR) factors are inconsistent, and the interactive association between CRF and fatness with CMR factors is unclear in children. Our study aimed to examine whether CRF and fatness are independently and interactively associated with CMR factors.
Methods: We included 5,869 children aged 6–13 years in the analysis. Physical examinations, blood tests, and CRF were measured at baseline and 1 year later. Cardiometabolic risk score (CMRS) was computed by summing Z scores of waist circumference (WC), averaged systolic and diastolic blood pressure, glucose, high-density lipoprotein cholesterol (HDL-C, multiplied by −1), and triglycerides.
Results: There was a high correlation between fatness and CRF in both boys and girls. High baseline CRF was independently associated with favorable changes in CMRS, BMI, WC, percent body fat (PBF), total cholesterol, LDL-C, and HDL-C (all P < 0.025). Improved CRF was independently associated with favorable changes in CMRS, BMI, WC, PBF, total cholesterol, LDL-C, HDL-C, triglycerides, and fasting glucose (all P < 0.0321). Baseline BMI was positively associated with changes in CMRS, WC, blood pressure, triglycerides, insulin, and HOMA-IR (all P < 0.0462). Low PBF at baseline was associated with favorable changes in CMRS, BMI, WC, blood pressure, HDL-C, triglycerides, insulin, and HOMA-IR (all P < 0.0423). The percentage of the total effect of baseline CRF on changes in CMRS, triglycerides, HDL-C, PBF, and WC mediated by baseline BMI was 66.0, 61.6, 40.3, 20.7, and 9.2%, respectively. Baseline CRF was a significant mediator for the association between baseline BMI and changes in CMRS (mediated by 4.3%), triglycerides (5.1%), and HDL-C (12.0%). An inverse association was found between baseline CRF and CMRS in children with high baseline BMI/PBF only. Improved CRF was associated with decreased BMI and WC in children with low baseline CRF.
Conclusions: Fatness and CRF are each independently associated with changes in CMR factors. Fatness is a major mediator for the association between CRF and CMR factors, whereas the association between fatness and CMR factors is also mediated by CRF. The beneficial effect of high CRF on CMR factors was more evident in obese or unfit children.
Introduction
Cardiometabolic risk (CMR) factors including high levels of body mass index (BMI), glucose, cholesterol, and blood pressure accounted for more than 30.0% of global mortality in 2015 (1). There was a high prevalence of CMR factors in both children and adults in China (2), which may be largely due to the dramatic shift toward high energy-density diets and low physical activity lifestyle in the last two decades (3, 4). While childhood CMR factors are highly likely to persist into adulthood (5, 6), suggesting the importance of prevention of these risk factors at an early stage. Prevention of obesity and improvement in cardiorespiratory fitness (CRF) are thought to play an important role in CMR reduction (7–11).
An increasing body of evidence reveals an obesity paradox in cardiovascular disease (CVD) in adults that there is a better prognosis in overweight and mildly obese CVD patients than their leaner counterparts (12, 13). This obesity paradox has attracted increasing concerns over which of fitness and fatness is more important for the development of CVD (14). Some studies found BMI was a stronger predictor of CMR factors in children (15–18), whereas others showed CRF was an independent predictor for CMR factors regardless of BMI (19, 20). More recent research in adults suggests that CRF and adiposity interplayed on changes in CMR factors (12, 13); however, data from children are limited.
Several recent cross-sectional studies in children from Spain have shown that BMI was a mediator of the association between CRF and CMR factors (21, 22). Another cross-sectional study also from Spain performed moderation analysis and found the inverse association between CRF and CMR was more likely to be manifested in obese children (23). However, these studies are restricted by cross-sectional design or small sample sizes. A longitudinal analysis may provide more convincing evidence on the physiological pathways on the interactive and independent effects of CRF and fatness on CMR factors given its advantages in many aspects of a mediation and moderation model that are unavailable in cross-sectional data (24, 25).
We assumed that fatness than CRF was more predictive of CMR factors, and CRF and fatness were interactively associated with the changes in CMR factors in children. The present study examined whether CRF and fatness measured by BMI and percent body fat (PBF) are independently associated with changes in CMR factors in children. We also examined the association between CRF and changes in CMR factors that are moderated and mediated by fatness and whether the association between fatness and changes in CMR factors is mediated by CRF.
Materials and Methods
Study Design
The nutrition-based comprehensive intervention study on childhood obesity in China is a multicenter, randomized cluster controlled trial, and the design has been detailed elsewhere (26). The study was conducted in six capital or province capital cities: Beijing, Harbin, Jinan, Shanghai, Chongqing, and Guangzhou. Children in the intervention group received nutrition lectures (knowledge, attitudes, and dietary habits), as well as participated in two times of 10 min or one time of 20 min of Happy 10 program per day (involves various physical activities such as games, dance, and gymnastics, which were designed to stimulate children to enjoy physical activity). No interventions were conducted in the control group. Our previous work has shown the beneficial effects of the intervention on the changes in obesity makers including BMI and waist circumference (WC) (27). Children with serious diseases such as congenital heart disease and schools who participated or aimed to participate in any other research projects within 1 year were excluded. Data on 50-m × eight-shuttle run (50MESR) were not collected in Beijing such that data from the other five cities were included in our analysis. We excluded participants who were from Beijing (n = 2,150), those who were lost to follow-up (n = 829), and those who had missing values in CRF or BMI at baseline (n = 1,019). We included 5,869 children from 303 classes within 30 schools in the final analysis (Figure S1).
The study protocol was approved by the Ethical Review Committee of the National Institute for Nutrition and Food Safety, Chinese Center for Disease Control and Prevention. Oral assent was collected from children, whereas written informed consent was obtained from the next of kin, carers, or guardians of all participants.
Physical Examinations and Blood Tests
Physical examinations (fasting 10–14 h beforehand), and blood tests were performed at both baseline (May 2009) and follow-up (May 2010) following standardized procedure by trained staff. Blood samples were taken between 7:30 and 8:30 AM, and other examinations were then conducted between 8:45 and 9:30 in the morning.
Height was measured to the nearest 0.1 cm using a freestanding stadiometer (GMCS-I; Xindong Huateng Sports Equipment Co. Ltd., Beijing, China), and weight was measured to the nearest 0.1 kg using a double ruler scale (RGT-140; Wujin Hengqi Co. Ltd., Changzhou, China). Body mass index was computed as weight in kilograms divided by height in meters squared. Overweight was defined using BMI according to the International Obesity Task Force standard (28). Waist circumference was measured twice to the nearest 0.1 cm, and the average was used.
Impedance, phase, resistance, and reactance were measured using a single frequency (50 Hz) hand-to-foot bioelectrical impendence device (ImpDF50; Impedimed Pty Ltd., Queensland, Australia) to estimate the whole-body fat. Besides 10–14 h of fasting, children were required to (1) no water drinking in preceding 4 h, (2) no intensive physical activity in preceding 2 h, (3) urine emptying within 30 min, (4) no alcohol drinking in preceding 12 h, and (1) no period on the testing day for girls. Body fat mass was computed using the prediction formula developed by Deurenberg et al. (29), and PBF was then calculated.
Diastolic and systolic blood pressure (DBP and SBP) were measured in the seated position using a mercury sphygmomanometer by trained nurses. The first and fifth Korotkoff sounds were used to represent the SBP and DBP. Three measurements were taken to the nearest two mm Hg, and the average of the last two measurements was used. The mean arterial pressure (MAP) was calculated as DBP + (0.33 × [SBP – DBP]).
Fasting blood samples (5 mL) were collected in serum separator tubes in the morning after 10–14 h of overnight fast and then transported to be clotted for 20–30 min and centrifuged for 10 to 15 min at 3,200 rpm using a Multifuge 35R. Blood samples for glucose tests were transported to the clinical laboratory in a cooler with an ice block at 4°C. Other blood samples were stored in a deep freezer at −70°C until further testing. Fasting glucose was measured using the glucose-oxidized method (Daiichi Pharmaceutical Co., Ltd., Tokyo, Japan). Fasting insulin was measured using the Microparticle Enzyme Immunoassay method (the AxSYM insulin assay; Abbott Co., Ltd., Tokyo, Japan). The Homeostatic Model Assessment of Insulin Resistance (HOMA-IR) was computed as (fasting insulin [μU/L] × fasting glucose [mg/dL])/405. Conventional enzymatic assays were used to measure triglycerides (TGs), total cholesterol (TC), high-density lipoprotein cholesterol (HDL-C), and low-density lipoprotein cholesterol (LDL-C) with 7080 Automatic Analyzer (Daiichi Pharmaceutical Co., Ltd.). Values of TG, TG to HDL-C ratio, insulin, and HOMA-IR were log-transformed.
Cardiometabolic Risk Assessment
Cardiometabolic risk score (CMRS) was computed by summing age- and sex-specific Z scores of WC, the average of SBP and DBP, fasting glucose, HDL-C (multiplied by −1), and TG (30). Waist circumference was removed from this score in the mediation analysis for CRF and BMI with CMRS given the high correlation between WC and BMI.
Physical Fitness Measurements
50MESR as a traditional test in China was used to evaluate CRF among children (31). A physical education teacher and researcher were involved in the test. Before the test, they would check if the children were under good health conditions. The test would be terminated if the children were struggling. 50MESR test required children to run back and forth four times at their highest speed along a track between two poles set 50 m apart and to turn around the poles counterclockwise. The test was recorded to the nearest 0.1 s (HS-70W stopwatch; CASIO, Shenzhen, China). To motivate children to try their best in the test, the results would be accounted for as a component of the performance assessment of the physical education for the semester. We used the 50MSR speed (400 m divided by test time in seconds) as CRF with a higher value indicating better performance. Cardiorespiratory fitness was analyzed in quintiles as previous studies classified individuals as fit (≥20th centile) and unfit (<20th centile) using centiles of physical fitness (32, 33).
Covariates
Information about age, sex, class, and grade was provided by the selected schools and confirmed by the children using an administered questionnaire. Dietary intake was assessed using the 24-h diet recall for 3 consecutive days including 2 weekdays and 1 weekend day. Interviews were conducted by trained investigators. Physical activity was assessed using a validated questionnaire, from which metabolic equivalent (MET) was calculated (34). Puberty status was recorded by investigators during the interview when physical examinations were conducted. Children were considered among puberty if the onset of menstruation for girls and the first ejaculation for boys occurred. Birth weight, household income, parental education, and parental height and weight were reported by the parents using a self-administered questionnaire.
Statistical Analysis
Continuous variables were expressed as means ± standard deviation (SD) and categorical variables as frequency and percentage for baseline characteristics of participants. The t-test for continuous variables and the χ2 test for categorical variables were used to examine whether the description of baseline characteristics differed between boys and girls.
Body mass index, WC, PBF, SBP, DBP, MAP, TC, HDL-C, LDL-C, TG, fasting glucose, insulin, HOMA-IR, and 50MESR at baseline and follow-up were standardized (i.e., Z scores were calculated: Z = (value – mean)/SD using sex- and age-specific means and SDs). We performed the analysis in the whole population with sex and intervention as covariates given the interaction between BMI/PBF/CRF and intervention or sex for changes in most CMR factors was not significant (Tables S1, S2). Changes in CMR factors and CRF were calculated by subtracting the results at baseline from those at follow-up. We used generalized linear regression models (GLMs) to test the difference in changes of CRF across quintiles of baseline and changes in BMI and PBF. Generalized linear regression model was used to test the difference in changes in CMR factors between sex-specific quintiles of CRF/BMI/PBF. We tested two models: (1) adjusted for classes in school as clustering effects and characteristics of individuals including age and sex as fixed effects and (2) adjusted for Model 1 plus BMI, CRF, physical activity, energy intake at baseline, puberty, birth weight, grade, household income, mother's education, father's education, mother's BMI, and father's BMI. Benjamini–Hochberg's procedure was used to control the false discovery rate at level 5% for multiple comparisons (35). Bonferroni P-value adjustments were performed for all pairwise comparisons.
To examine whether the association between CRF and changes in CMR factors was modified by BMI or PBF, we performed interaction analysis using GLM adjusted for covariates in Model 3. Stratified analysis was conducted for those CMR factors with a significant interaction.
We examined the mediation effect of BMI/PBF on the association between baseline and changes in CRF and changes in CMR. The direct effects of the association between CRF and changes in CMR factors and the indirect effects of this association via BMI/PBF were obtained using GLM adjusted for covariates in Model 3 of Table 2. The percentage of the total effect of CRF on changes in CMR factors explained by BMI/PBF was then calculated (36, 37). We used the following criteria to establish mediation: (1) the mediator was significantly associated with the exposure; (2) the exposure was significantly associated with the outcome; (3) the mediator was significantly associated with the outcome; and (4) the association between the exposure and outcome was attenuated by the mediator (21). We also examined the mediation effect of CRF on the association between BMI/PBF and CMR factors.
Sensitivity analysis was performed to test the association between CRF/BMI/PBF and changes in CMR factors in children in the control group.
Analyses were performed using SAS version 9.4 (SAS Institute Inc., Cary, NC, USA), and all P-values were two-sided.
Results
Study Population
We included 5,869 children aged 9.1 ± 1.4 (6–13) years in the final analysis. Compared with dropouts, those included in the analysis had higher blood pressure, TC, insulin, and CMRS and similar age and BMI (Table S3). Boys had higher BMI, WC, SBP, MAP, fasting glucose and HDL-C and lower PBF, TC, TG, and LDL-C than girls (Table 1, all P < 0.05).
BMI, PBF, and CRF
The prevalence of overweight and obesity at baseline was 15.0 and 6.2%, respectively. The corresponding number at follow-up was 22.2 and 6.6%, respectively. The CRF in speed at baseline was 3.17 ± 0.42 m/s, and it increased by 0.08 ± 0.42 m/s during follow-up. The percentage of children being unfit (defined by Z score) was 26.7% at baseline and 27.4% at follow-up. Boys had a higher prevalence of obesity but a lower prevalence of unfitness than girls (P < 0.0001).
There was a high correlation between BMI and PBF and CRF at baseline in both boys and girls. Both BMI and PBF at baseline were inversely associated with the change in CRF in both boys and girls (all P < 0.0001). Change in BMI but not PBF was inversely associated with the change in CRF (Figure 1).
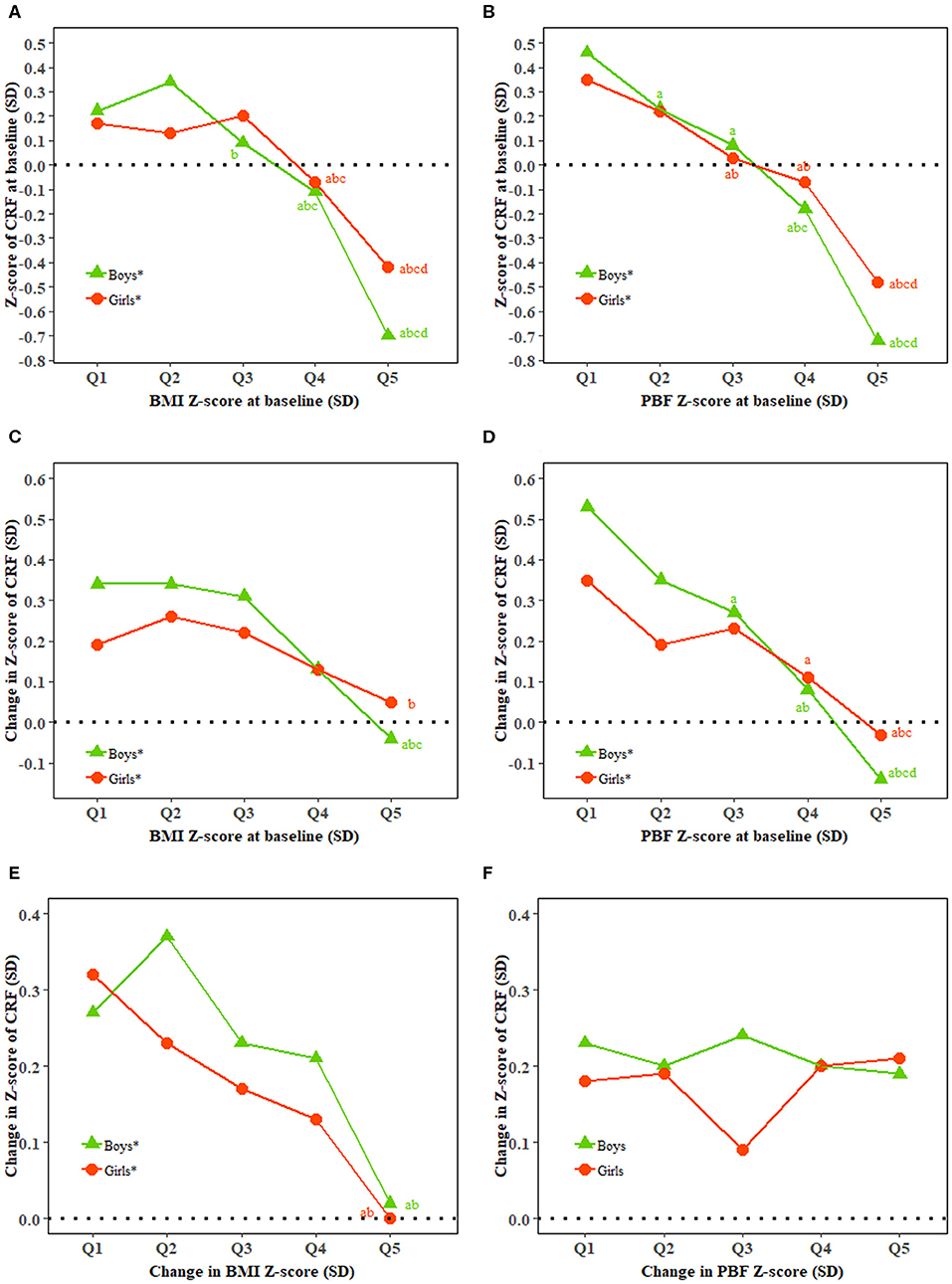
Figure 1. Relationship of BMI and percent body fat with cardiorespiratory fitness. BMI, body mass index; CRF, cardiorespiratory fitness; PBF, percent body fat; Q, quintile; SD, standard deviation. (A–F) Show baseline CRF and baseline BMI, baseline CRF and baseline PBF, change in CRF and baseline BMI, change in CRF and baseline PBF, change in CRF and change in BMI, and change in CRF and change in PBF, respectively. *Represents a significant linear trend of CRF associated with quintiles of BMI or PBF. Generalized linear regression model was used to estimate means and standard errors for each quintile of BMI and PBF adjusted for classes in school as clustering effects and characteristics of the individuals including age (additional adjustment for BMI and PBF for change in BMI and PBF and CRF at baseline for change in CRF) as fixed effects. abcdBonferroni post hoc test was used to examine the difference between every two quintiles of BMI and PBF with aindicating significance compared with Quintile 1, bindicating significance compared with Quintile 2, cindicating significance compared with Quintile 3, and dindicating significance compared with Quintile 4.
CRF and Changes in CMR Factors
High baseline CRF was associated with favorable changes in all CMR factors except TC and fasting glucose after adjustment for age and sex. These CMR factors were restricted to CMRS, BMI, WC, PBF, TC, LDL-C, and HDL-C after adjustment for full confounders (all P < 0.025). Improved CRF was associated with favorable changes in CMRS, BMI, WC, PBF, TC, LDL-C, HDL-C, TG, and fasting glucose in the multi-variable analysis (all P < 0.0321; Table 2).
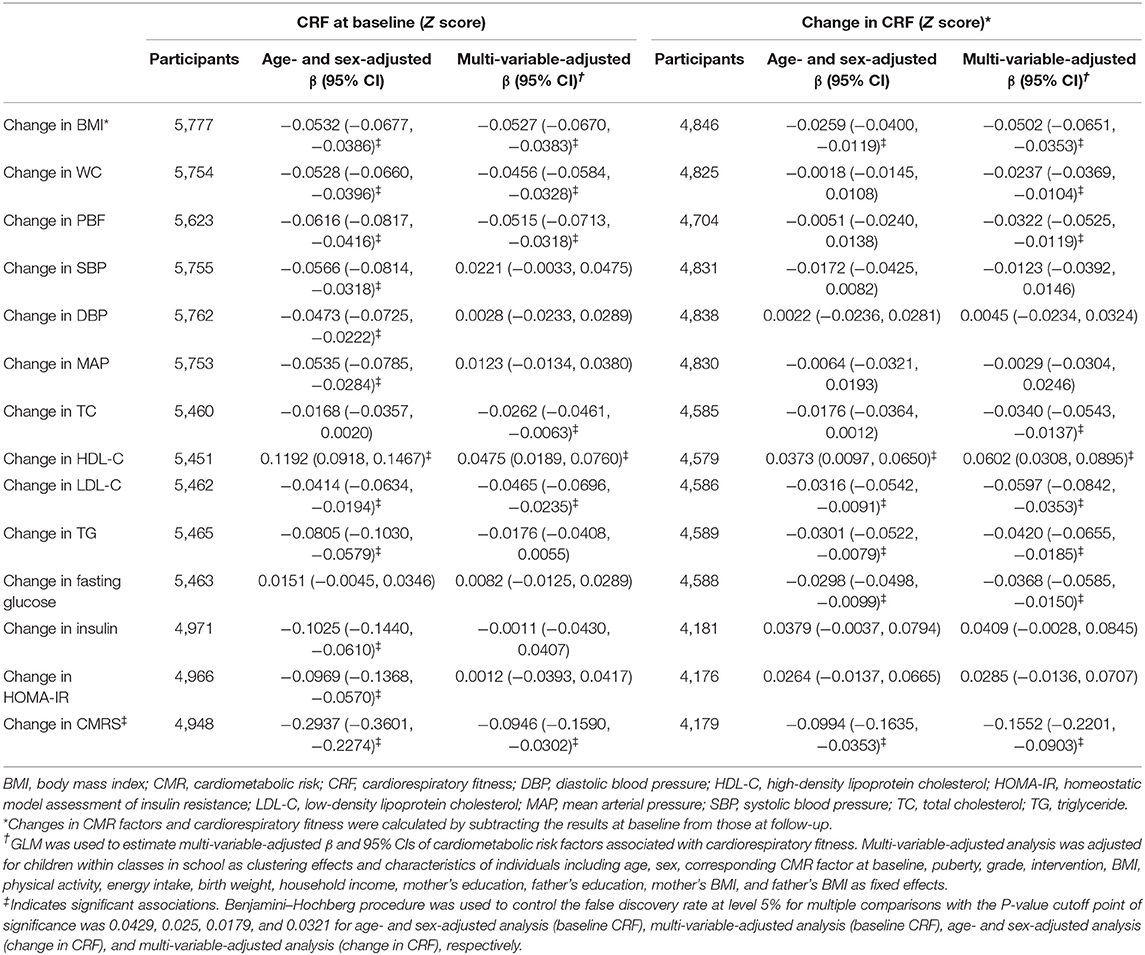
Table 2. Changes in cardiometabolic risk factors associated with baseline and change in cardiorespiratory fitness.
BMI and Changes in CMR Factors
Baseline BMI was positively associated with changes in CMRS, BMI, WC, PBF, SBP, DBP, MAP, TG, insulin, and HOMA-IR after adjustment for confounders (all P < 0.0462). Baseline BMI was inversely associated with changes in HDL-C, TC, and fasting glucose.
A low increase in BMI was associated with favorable changes in all CMR factors (all P < 0.05). Each SD increase in BMI was associated with a 1.2874 [95% confidence interval (CI), 1.1713, 1.4035] SD increase in CMRS after adjustment for confounders (Table 3).
PBF and Changes in CMR Factors
In the multi-variable analysis, low PBF at baseline was associated with favorable changes in CMRS, BMI, WC, PBF, SBP, DBP, MAP, HDL-C, TG, insulin, and HOMA-IR (all P < 0.0423). Percent body fat at baseline was inversely associated with the change in fasting glucose. A low increase in PBF was associated with favorable changes in all CMR factors except fasting glucose (all P < 0.0423, Table 4).
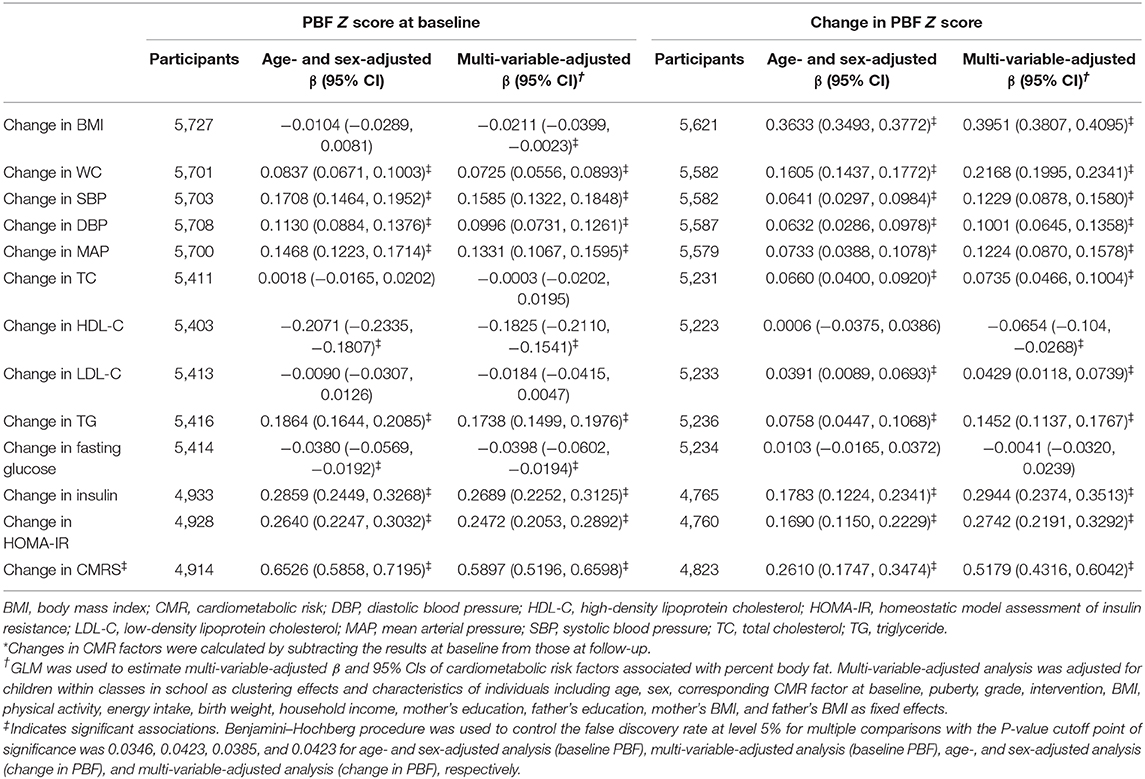
Table 4. Changes in cardiometabolic risk factors associated with baseline and changes in percent body fat.
WC and Changes in CMR Factors
A low increase in WC was associated with favorable changes in all CMR factors (all P < 0.05, Table S4).
Moderation Analysis
Stratified analysis showed an inverse association between baseline CRF and changes in CMRS in high but not low levels of BMI or PBF (Figure 2). An inverse association between baseline CRF and change in TG was observed in high levels of baseline BMI (Figure S2). High baseline BMI and high increase in BMI were associated with a higher increase in HOMA-IR. High baseline PBF and a greater increase in PBF were associated with a higher increase in CMRS (Figure S3). Improved CRF was associated with decreased BMI and WC in those with low but not high levels of baseline CRF (Figure S4). Improved CRF and a low increase in PBF were associated with a lower increase in fasting glucose (Figure S5).
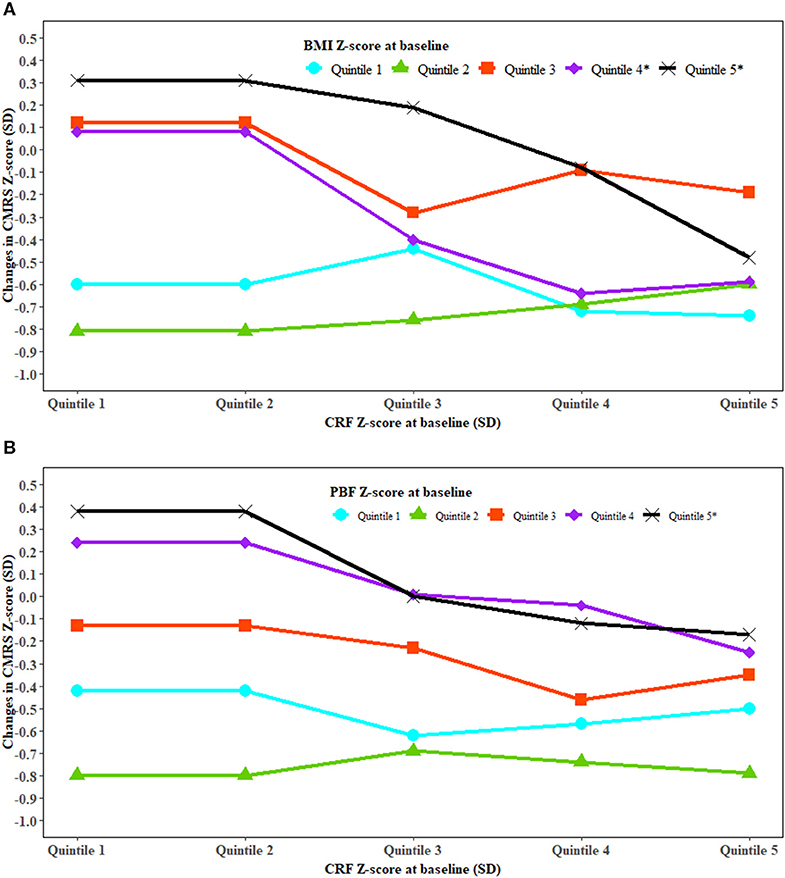
Figure 2. The association between cardiorespiratory fitness and change in cardiometabolic risk modified by fatness. BMI, body mass index; CRF, cardiorespiratory fitness; CMRS, cardiometabolic risk score; PBF, percent body fat; SD, standard deviation. Generalized linear regression was used to test whether the association between CRF and change in CMRS was modified by BMI (A) and PBF (B). The analysis was adjusted for covariates in Model 3 of Table 2. P interaction was 0.0002 for BMI and CRF at baseline with the change in CMRS and <0.0001 for PBF and CRF at baseline with the change in CMRS. *Significant trend for changes in CMRS with CRF at baseline in the specific quintiles of BMI and PBF at baseline.
Mediation Analysis
The percentage of the total effect of baseline CRF on changes in CMRS, TG, HDL-C, PBF, and WC mediated by baseline BMI was 66.0, 61.6, 40.3, 20.7, and 9.2%, respectively. Changes in BMI explained 71.4, 64.1, 26.3, 22.2, 22.0, 18.8, 6.6, and 3.4% of the association between changes in CRF and changes in SBP, WC, HDL-C, CMRS, TC, TG, LDL-C, and fasting glucose, respectively. Baseline PBF explained 51.9–68.2% of the association between baseline CRF and changes in HDL-C, CMRS, and TG.
Baseline CRF was a significant mediator for the association between baseline BMI and changes in CMRS (mediated by 4.3%), TG (5.1%), and HDL-C (12.0%). Changes in CRF were a significant mediator for the association between changes in BMI and changes in CMRS, TG, HDL-C, LDL-C, and fasting glucose with the mediation percentage ranging from 1.8 to 21.7% (Figure 3).
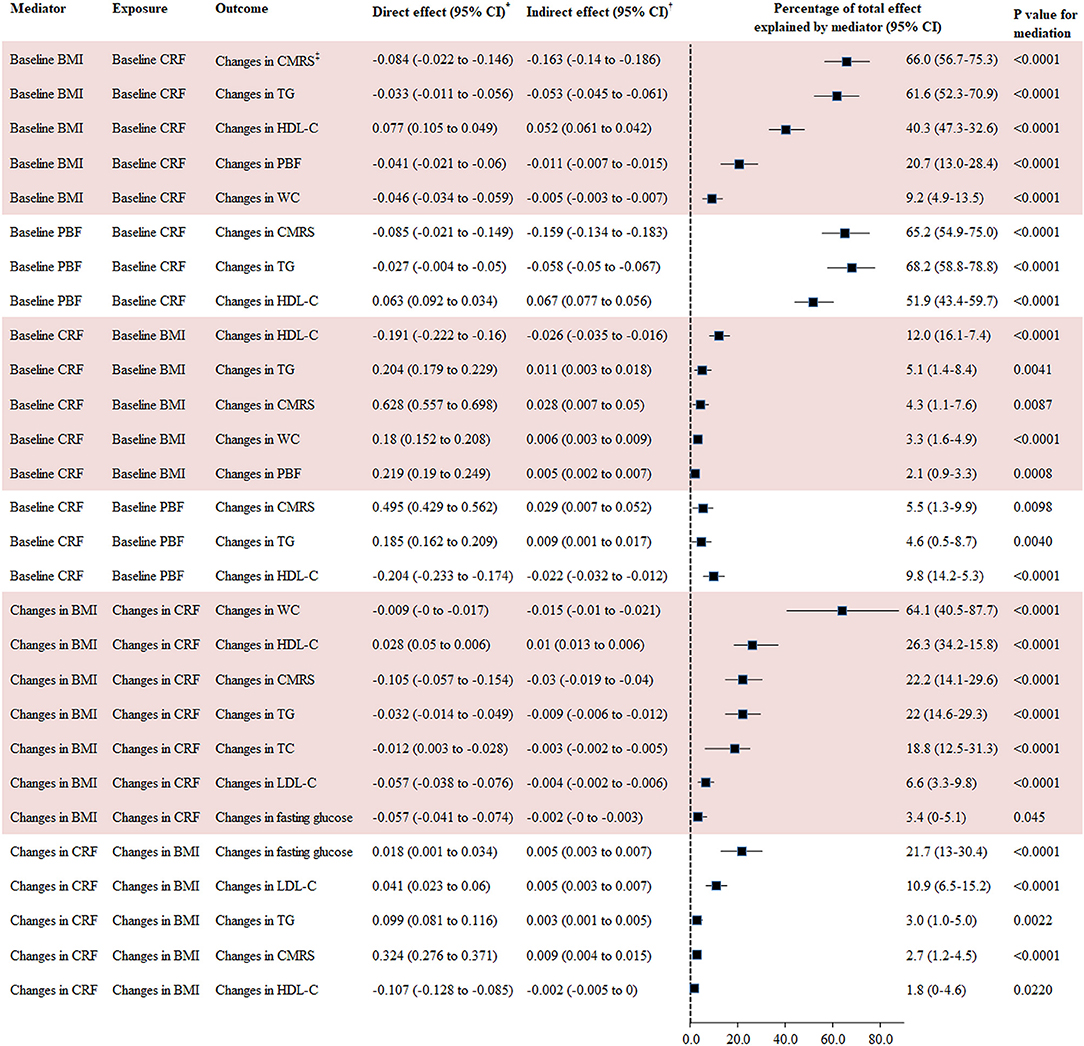
Figure 3. Mediation analysis for BMI, PBF, and CRF with changes in cardiometabolic risk factors. BMI, body mass index; CI, confidence interval; CMRS, cardiometabolic risk score; CRF, cardiorespiratory fitness; HDL-C, high-density lipoprotein cholesterol; LDL-C, low-density lipoprotein cholesterol; TC, total cholesterol; TG, triglyceride. *Direct effect represents the direct association between exposures and outcomes using GLM adjusted for covariates in Model 3 of Table 2. †Indirect effect represents the association between exposures and outcomes via mediators using GLM adjusted for covariates in Model 3 of Table 2. CMRS was calculated by summing age- and sex-specific Z scores of the average of SBP and DBP, fasting glucose, HDL-C (multiplied by −1), and TG. Waist circumference was not taken into consideration for calculating this score in the mediation analysis for CRF and BMI with CMRS given the high correlation between WC and BMI.
Sensitivity Analysis
In the multi-variable analysis among children in the control group, improved CRF was associated with favorable changes in CMRS, BMI, WC, TC, HDL-C, LDL-C, TG, and fasting glucose (Table S5). A low increase in BMI was associated with favorable changes in all CMR factors except fasting glucose (Table S6). The results for associations of PBF with changes in CMR factors were like those observed in the total population (Table S7).
Discussion
In this longitudinal analysis with large sample size, we found both fatness and CRF were independent predictors for changes in CMR factors in children. Baseline fatness was more predictive of changes in blood pressure, insulin, and HOMA-IR, and CRF was more predictive of changes in TC and LDL-C, suggesting CRF deserves scrutiny as a surrogate for fatness in predicting CMR factors. Fatness was a major mediator for the association between CRF and CMR, whereas CRF was a minor or a moderate mediator for the association between fatness and CMR. The inverse association between baseline CRF and change in CMRS and TG was more pronounced in obese children, and the inverse association between improved CRF and change in BMI and WC was more evident in unfit children.
We found boys had a lower prevalence of unfitness but a higher prevalence of obesity than girls. This is consistent with a systematic review of 1,142,026 children and youth from 50 countries showing that boys consistently had better performance in CRF than girls at each age group (32). Likely, a nationally representative survey of Chinese children reported that boys were two times more likely to be obese than girls (38). The increasing prevalence of obesity and the decreasing prevalence of healthy CRF in children worldwide as well as in China suggest it is imperative to intervene on lifestyle factors to address this problem (32, 39). In particular, resistance plus aerobic training may help result in substantial improvement in fatness and fitness in children (40, 41).
The inverse association between BMI and CRF in children has been well-established (42, 43). Likely, we found that both baseline and changes in BMI were inversely associated with changes in CRF. Some studies demonstrated that body fat mass might be more predictive of CRF compared with BMI in children (44, 45). We found the lower baseline PBF, the higher baseline CRF, and the greater increase in CRF during follow-up, but changes in PBF were not significantly associated with changes in CRF. Further study needs to examine whether changes in BMI than PBF are a better predictor for changes in CRF. Our study suggests a strong inverse association between BMI and PBF and CRF in children.
The beneficial effect of high CRF on fatness reduction in children has been well-known; however, there is less convincing evidence on other CMR factors (9). Most of the previous studies investigating the association between CRF and CMR factors were conducted in children from European countries, the United States, and Australia (9). Our study with a large sample size confirmed the beneficial effect of high baseline CRF on BMI, WC, and PBF, as well as CMRS, TC, HDL-C, LDL-C, and TG independent of corresponding CMR factor and BMI at baseline. A few longitudinal studies have investigated the association between change in CRF and changes in multiple CMR factors (9, 15, 17, 19). A 2-year longitudinal study of 1,199 European children aged 6–11 years demonstrated that low CRF was associated with higher CMRS, WC, blood lipids, and HOMA-IR independent of obesity (15). Data from another 2-year longitudinal study in 365 schoolchildren aged 7–11 years from Denmark found that change in CRF was inversely associated with the change in CMRS independent of obesity (19). Data from the HEALTHY Study of 3,514 children from the United States demonstrated that an increase in fitness was associated with favorable changes in TC, HDL-C, LDL-C, and CMRS among boys, and HDL-C among girls (17). However, these studies failed to control the corresponding CMR factor or CRF at baseline in their analysis, and no longitudinal studies from Asia have reported an association between CRF and multiple CMR factors in children. Our study showed that improved CRF was associated with favorable changes in adiposity, lipids, fasting glucose, and CMRS independent of BMI and corresponding CMR factor at baseline. One possible explanation for CRF being more predictive of changes in CMR factors in our study is the large sample size with large enough variation of baseline and change in CRF to test the significance. Furthermore, we used a test to estimate CRF different from that of previous studies. Although data from longitudinal studies in children are limited, previous studies in adults are supportive of our findings regarding the favorable effect of CRF improvement on CMR reduction (46, 47).
More studies from a larger range of countries have investigated the association between fatness and CMR factors in children compared with those studies associating CRF with CMR factors (48). There was strong evidence for the positive association between obesity and CMR factors (7, 15–17). In line with this, our study showed BMI, PBF, and WC at baseline were all positively associated with changes in CMRS, blood pressure, TG, insulin, and HOMA-IR and inversely associated with HDL-C. Although any of these obesity markers at baseline were not predictive of changes in TC or LDL-C in our study, a higher increase in each of them was associated with a higher increase in TC and LDL-C. Several longitudinal studies found baseline BMI was not significantly associated with the change in fasting glucose (7). However, we found an inverse association between baseline BMI and PBF and change in fasting glucose, even though the difference between the highest and lowest quintile was minimal (SD, 0.09). The significance of this minimal difference may be partly attributed to our large sample size. We also found that a decrease in BMI was associated with a favorable change in fasting glucose suggesting BMI reduction may be beneficial for glucose reduction. Our study agrees with previous studies showing that fatness measured in different ways was positively associated with changes in CMR factors (7).
Previous longitudinal studies from Europe and the United States have shown that BMI is a stronger predictor of changes in CMR factors than CRF (15–17). Although we found that baseline BMI/PBF/WC is predictive of changes in more CMR factors, baseline CRF is a better predictor for LDL-C and TC, suggesting CRF deserves concern as a surrogate for fatness in predicting CMR factors, whereas baseline CRF and BMI/PBF/WC are all strong predictors for TG, HDL-C, and CMRS, and changes in CRF and BMI/PBF/WC are each predictive of changes in SBP, TC, HDL-C, LDL-C, TG, fasting glucose, and CMRS, suggesting they may highly interact on CMR factors. Several cross-sectional studies from Europe have examined the extent to which the association between CRF and one or several CMR factors is explained by BMI (21, 22, 49). A cross-sectional study of 1,158 schoolchildren aged 8–11 years showed that BMI explained ~40% of the association between CRF and CMRS (21). Another cross-sectional study of 237 children and 260 adolescents demonstrated that 55.7–100% of the total effect of CRF on CMRS was mediated by BMI (22). Similarly, our mediation analysis showed that BMI and PBF were both a full or major mediator for the association between CRF and CMR factors. In contrast, CRF was a significant mediator for the association between BMI and PBF and CMR, even though the effect was relatively small. A cross-sectional analysis of 1,604 school children aged 4–7 years showed BMI acts as a full mediator in the association between CRF and MAP in boys at 62.3% and a partial mediator in girls at 35.2% (49). We found the inverse association between baseline CRF and changes in SBP was attenuated or even reversed after adjustment for BMI, suggesting the beneficial effect of high CRF may largely depend on BMI. Our mediation analysis based on longitudinal data with a large sample size suggests CRF and fatness highly interacted on the change in CMR factors. Given that these relevant studies were conducted in Europe and China (our study), more longitudinal studies from other countries are needed to examine the mediation effect of CRF on the association between fatness and CMR factors.
A few previous studies have investigated whether the association between CRF and CMR factors was modified by fatness. A pooled study of cross-sectional data from three projects with 1,247 children aged 8–11 years from Spain found an association between CRF and CMR factors was more evident in obese children (23). Similarly, a longitudinal analysis of 1,792 Australian participants aged 7–15 years reported that an increased risk of metabolic syndrome with lower CRF was only observed in the highest tertile of WC, although the interaction was not significant (P = 0.61) (20). Data from the HEALTHY Study of American children observed an additive effect of BMI and fitness on change in HDL-C (17). Consistently, we found the beneficial effect of high CRF on CMRS and TG reduction in the two highest quintiles of baseline BMI only. Our study also showed improved CRF was associated with a lower increase in BMI and WC in those with low baseline CRF only. This suggests encouraging children especially those with obesity or low CRF involved in exercise may significantly reduce the risk of CMR factors. However, for other CMR factors, the favorable change associated with high CRF was independent of BMI emphasizing the importance of supporting all children to take part in physical activity.
Strengths of the present study included its large sample size, multiple CMR factors examined, and various covariates adjusted for. Our study uniquely evaluated the percentage of the association between CRF and CMR explained by fatness and whether CRF was a mediator for the association between fatness and CMR in children based on longitudinal data.
The present study has several limitations. First, the relatively short time of follow-up (1 year) of our study may not be a long-enough duration to judge on the association between CRF and changes in CMR factors in children. However, we found both high baseline CRF and improvements in CRF were associated with favorable changes in CMR factors, suggesting the reliability of our findings. Second, our analysis as an observational design was based on a clinical trial, which might be biased even though the intervention was treated as a covariate in the multi-variable analysis. However, the repeated analysis in the control group showed similar results as those of the total population, suggesting that our findings are reliable. Although 50MESR has been strongly associated with CMR factors in our data and previous studies (31, 50, 51), it does not directly measure aerobic fitness.
In conclusion, the present longitudinal study demonstrates both CRF and fatness are independent predictors of changes in CMR factors in children. Although baseline fatness was predictive of changes in more CMR factors, CRF was more predictive of changes in TC and LDL-C, suggesting CRF deserves scrutiny as a surrogate for fatness in predicting CMR factors. Fatness largely mediated the association between CRF and changes in CMR factors, whereas CRF was a minor to a moderate mediator for the association between fatness and CMR factors. The favorable effect of improved CRF on changes in CMR factors was more likely to be manifested in obese or unfit children, emphasizing the importance of taking part in exercises in these subgroups.
Data Availability Statement
The datasets generated for this study are available on request to the corresponding author.
Ethics Statement
The studies involving human participants were reviewed and approved by Ethical Review Committee of the National Institute for Nutrition and Food Safety, Chinese Center for Disease Control and Prevention. Written informed consent to participate in this study was provided by the participants' legal guardian/next of kin.
Author Contributions
GM designed the research. GM, YiL, JM, GX, HG, TL, and WL conducted research. XS and YaL analyzed data. XS wrote the initial draft of the manuscript. XS, YaL, HX, and GM revised the manuscript. All authors read and approved the final manuscript.
Funding
This work was supported by the China Ministry of Science & Technology as Key Projects in the National Science & Technology Pillar Program during the Eleventh Five-Year Plan Period (Grant no. 2008BAI58B05).
Conflict of Interest
The authors declare that the research was conducted in the absence of any commercial or financial relationships that could be construed as a potential conflict of interest.
Acknowledgments
The authors would like to acknowledge the support of other team members and the participated students, teachers, parents, and local education and health staff members.
Supplementary Material
The Supplementary Material for this article can be found online at: https://www.frontiersin.org/articles/10.3389/fendo.2020.00342/full#supplementary-material
References
1. GBD 2015 Risk Factors Collaborators. Global, regional, and national comparative risk assessment of 79 behavioural, environmental and occupational, and metabolic risks or clusters of risks, 1990-2015: a systematic analysis for the Global Burden of Disease Study 2015. Lancet. (2016) 388:1659–724. doi: 10.1016/s0140-6736(16)31679-8
2. Adair LS, Gordon-Larsen P, Du SF, Zhang B, Popkin BM. The emergence of cardiometabolic disease risk in Chinese children and adults: consequences of changes in diet, physical activity and obesity. Obes Rev. (2014) 15(Suppl. 1):49–59. doi: 10.1111/obr.12123
3. Hu FB, Liu Y, Willett WC. Preventing chronic diseases by promoting healthy diet and lifestyle: public policy implications for China. Obes Rev. (2011) 12:552–9. doi: 10.1111/j.1467-789X.2011.00863.x
4. Cui Z, Hardy LL, Dibley MJ, Bauman A. Temporal trends and recent correlates in sedentary behaviours in Chinese children. Int J Behav Nutr Phys Act. (2011) 8:93. doi: 10.1186/1479-5868-8-93
5. Singh AS, Mulder C, Twisk JW, van Mechelen W, Chinapaw MJM. Tracking of childhood overweight into adulthood: a systematic review of the literature. Obes Rev. (2008) 9:474–88. doi: 10.1111/j.1467-789X.2008.00475.x
6. Laitinen TT, Pahkala K, Magnussen CG, Viikari J, Oikonen M, Taittonen L., et al. Ideal cardiovascular health in childhood and cardiometabolic outcomes in adulthood: the cardiovascular risk in young finns study. Circulation. (2012) 125:1971–8. doi: 10.1161/circulationaha.111.073585
7. Gishti O, Gaillard R, Durmus B, Abrahamse M, van der Beek EM, Hofman A, et al. BMI, total and abdominal fat distribution, and cardiovascular risk factors in school-age children. Pediatr Res. (2015) 77:710–8. doi: 10.1038/pr.2015.29
8. Friedemann C, Heneghan C, Mahtani K, Thompson M, Perera R, Ward AM. Cardiovascular disease risk in healthy children and its association with body mass index: systematic review and meta-analysis. BMJ. (2012) 345:e4759. doi: 10.1136/bmj.e4759
9. Mintjens S, Menting MD, Daams JG, van Poppel MNM, Roseboom JT, Gemke JBJR. Cardiorespiratory fitness in childhood and adolescence affects future cardiovascular risk factors: a systematic review of longitudinal studies. Sports Med. (2018) 48:2577–605. doi: 10.1007/s40279-018-0974-5
10. Jukarainen S, Holst R, Dalgard C, Piirila P, Lundbom J, Hakkarainen A, et al. Cardiorespiratory fitness and adiposity as determinants of metabolic health-pooled analysis of two twin cohorts. J Clin Endocrinol Metab. (2017) 102:1520–28. doi: 10.1210/jc.2016-3435
11. Valerio G, Licenziati MR, Tortorelli P, Calandriello LF, Alicante P, Scalfi L. Lower performance in the six-minute walk test in obese youth with cardiometabolic risk clustering. Front Endocrinol. (2018) 9:701. doi: 10.3389/fendo.2018.00701
12. Oktay AA, Lavie CJ, Kokkinos PF, Parto P, Pandey A, Ventura OH. The interaction of cardiorespiratory fitness with obesity and the obesity paradox in cardiovascular disease. Prog Cardiovasc Dis. (2017) 60:30–44. doi: 10.1016/j.pcad.2017.05.005
13. Elagizi A, Kachur S, Lavie CJ, Carbone S, Pandey A, Ortega BF, Milani VR. An overview and update on obesity and the obesity paradox in cardiovascular diseases. Prog Cardiovasc Dis. (2018) 61:142–50. doi: 10.1016/j.pcad.2018.07.003
14. Kennedy AB, Lavie CJ, Blair SN. Fitness or fatness: which is more important? JAMA. (2018) 319:231–2. doi: 10.1001/jama.2017.21649
15. Zaqout M, Michels N, Bammann K, Ahrens W, Sprengeler O, Molnar D, et al. Influence of physical fitness on cardio-metabolic risk factors in European children. The IDEFICS study. Int J Obes. (2016) 40:1119–25. doi: 10.1038/ijo.2016.22
16. Jago R, Drews KL, McMurray RG, Thompson D, Volpe LS, Moe EL, et al. Fatness, fitness, and cardiometabolic risk factors among sixth-grade youth. Med Sci Sports Exerc. (2010) 42:1502–10 doi: 10.1249/MSS.0b013e3181d322c4
17. Jago R, Drews KL, McMurray RG, Baranowski T, Halassetti P, Foster GD, et al. BMI change, fitness change and cardiometabolic risk factors among 8th grade youth. Pediatr Exerc Sci. (2013) 25:52–68. doi: 10.1123/pes.25.1.52
18. Wang PG, Gong J, Wang SQ, Talbott E, Zhang B, He QQ. Relationship of body fat and cardiorespiratory fitness with cardiovascular risk in Chinese children. PLoS ONE. (2011) 6:e27896. doi: 10.1371/journal.pone.0027896
19. Klakk H, Grontved A, Moller NC, Heidemann M, Andersen L, Wedderkopp N. Prospective association of adiposity and cardiorespiratory fitness with cardiovascular risk factors in healthy children. Scand J Med Sci Sports. (2014) 24:e275–82. doi: 10.1111/sms.12163
20. Schmidt MD, Magnussen CG, Rees E, Dwyer T, Venn AJ. Childhood fitness reduces the long-term cardiometabolic risks associated with childhood obesity. Int J Obes. (2016) 40:1134–40. doi: 10.1038/ijo.2016.61
21. Diez-Fernandez A, Sanchez-Lopez M, Mora-Rodriguez R, Notario-Pacheco B, Torrijos-Nino C V. Martinez-Vizcaino: obesity as a mediator of the influence of cardiorespiratory fitness on cardiometabolic risk: a mediation analysis. Diabetes Care. (2014) 37:855–62. doi: 10.2337/dc13-0416
22. Perez-Bey A, Segura-Jimenez V, Fernandez-Santos JD R, Esteban-Cornejo I, Gomez-Martinez S, Veiga O, et al. The influence of cardiorespiratory fitness on clustered cardiovascular disease risk factors and the mediator role of body mass index in youth: the UP&DOWN study. Pediatr Diabetes. (2019) 20:32–40. doi: 10.1111/pedi.12800
23. Nystrom CD, Henriksson P, Martinez-Vizcaino V, Medrano M, Cadenas-Sanchez C, et al. Does cardiorespiratory fitness attenuate the adverse effects of severe/morbid obesity on cardiometabolic risk and insulin resistance in children? A pooled analysis. Diabetes Care. (2017) 40:1580–7 doi: 10.2337/dc17-1334
24. MacKinnon DP, Fairchild AJ, Fritz MS. Mediation analysis. Annu Rev Psychol. (2007) 58:593–614. doi: 10.1146/annurev.psych.58.110405.085542
25. Hayes AF. Beyond baron and kenny: statistical mediation analysis in the New Millennium. Commun Monogr. (2009) 76:408–20. doi: 10.1080/03637750903310360
26. Li Y, Hu X, Zhang Q, Liu A, Fang H, Hao L, et al. The nutrition-based comprehensive intervention study on childhood obesity in China (NISCOC): a randomised cluster controlled trial. BMC Public Health. (2010) 10:229. doi: 10.1186/1471-2458-10-229
27. Xu H, Li Y, Zhang Q, Hu XL, Liu A, Du S, et al. Comprehensive school-based intervention to control overweight and obesity in China: a cluster randomized controlled trial. Asia Pac J Clin Nutr. (2017) 26:1139–51. doi: 10.6133/apjcn.112016.05
28. Cole TJ, Bellizzi MC, Flegal KM, Dietz WH. Establishing a standard definition for child overweight and obesity Worldwide: International survey. BMJ. (2000) 320:1240–3. doi: 10.1136/bmj.320.7244.1240
29. Deurenberg P, van der Kooy K, Leenen R, Weststrate JA, Seidell JC. Sex and age specific prediction formulas for estimating body composition from bioelectrical impedance: a cross-validation study. Int J Obes. (1991) 15:17–25.
30. Eisenmann JC. On the use of a continuous metabolic syndrome score in pediatric research. Cardiovasc Diabetol. (2008) 7:17. doi: 10.1186/1475-2840-7-17
31. Research Group on Chinese School Students Physical Fitness and Health: report on the physical fitness and health surveillance of Chinese school students in 2014. Higher Education Press, Beijing. (2016).
32. Tomkinson GR, Lang JJ, Tremblay MS, et al. International normative 20 m shuttle run values from 1 142 026 children and youth representing 50 countries. Br J Sports Med. (2017). 51:1545–54. doi: 10.1136/bjsports-2016-095987
33. Ortega FB, Lavie CJ, Blair SN. Obesity and cardiovascular disease. Circ Res. (2016) 118:1752–70. doi: 10.1161/circresaha.115.306883
34. Liu AL, Ma GS, Zhang Q, Ma WJ. Reliability and validity of a 7-day physical activity questionnaire for elementary students. Zhonghua Liu Xing Bing Xue Za Zhi. (2003) 24:901–4.
35. Benjamini Y, Hochberg Y. Controlling the false discovery rate: a practical and powerful approach to multiple testing. J R Stat Soc Ser. (1995) 57:289–300.
36. Lin DY, Fleming TR, de Gruttola V. Estimating the proportion of treatment effect explained by a surrogate marker. Stat Med. (1997) 16:1515–27.
37. Valeri L, Vanderweele TJ. Mediation analysis allowing for exposure-mediator interactions and causal interpretation: theoretical assumptions and implementation with SAS and SPSS macros. Psychol Methods. (2013) 18:137–50 doi: 10.1037/a0031034
38. Zhang J, Zhai Y, Feng XQ, Li WR, Lyu BY, Astell-Burt T, et al. Gender differences in the prevalence of overweight and obesity, associated behaviors, and weight-related perceptions in a National Survey of primary school children in China. Biomed Environ Sci. (2018) 31:1–11. doi: 10.3967/bes2018.001
39. Afshin A, Forouzanfar MH, Reitsma MB, Sur P, Estep K, Lee A, et al. Health effects of overweight and obesity in 195 countries over 25 years. N Engl J Med. (2017) 377:13–27 doi: 10.1056/NEJMoa1614362
40. Branco BH M, Valladares D, de Oliveira FM, Carvalho IZ, Marques CD, Coelho AA, et al. Effects of the order of physical exercises on body composition, physical fitness, and cardiometabolic risk in adolescents participating in an interdisciplinary program focusing on the treatment of obesity. Front Physiol. (2019) 10:1013 doi: 10.3389/fphys.2019.01013
41. Magnani Branco BH, Carvalho IZ, de Oliveira HG, Fanhani AP, Machado Dos Santos MC, de Oliveira LP, et al. Effects of 2 types of resistance training models on obese adolescents' body composition, cardiometabolic risk, and physical fitness. J Strength Cond Res. (2018). doi: 10.1519/jsc.0000000000002877. [Epub ahead of print].
42. Aires L, Andersen LB, Mendonca D, Martins C, Silva G, et al. A 3-year longitudinal analysis of changes in fitness, physical activity, fatness and screen time. Acta Paediatr. (2010) 99:140–4. doi: 10.1111/j.1651-2227.2009.01536.x
43. Zhang Y, Liu S, Li Y, Li X, Ren P, Luo F. The relationships between weight status and physical fitness among Chinese children and youth. Res Q Exerc Sport. (2019) 90:113–22. doi: 10.1080/02701367.2019.1603768
44. Tompuri T, Lintu N, Savonen K, Laitinen T, Laaksonen D, Jaaskelainen J, Lakka TA. Measures of cardiorespiratory fitness in relation to measures of body size and composition among children. Clin Physiol Funct Imaging. (2015) 35:469–77. doi: 10.1111/cpf.12185
45. Burns R, Hannon JC, Brusseau TA, Shultz B, Eisenman P. Indices of abdominal adiposity and cardiorespiratory fitness test performance in middle-school students. J Obes. (2013) 2013:912460. doi: 10.1155/2013/912460
46. Lee DC, Sui X, Church TS, Lavie C, Jackson AS, Blair SN. Changes in fitness and fatness on the development of cardiovascular disease risk factors hypertension, metabolic syndrome, and hypercholesterolemia. J Am Coll Cardiol. (2012) 59:665–72 doi: 10.1016/j.jacc.2011.11.013
47. Park YM, Sui X, Liu J, Zhou H, Kokkinos P, Lavie CJ, et al. The effect of cardiorespiratory fitness on age-related lipids and lipoproteins. J Am Coll Cardiol. (2015) 65:2091–100. doi: 10.1016/j.jacc.2015.03.517
48. Lo K, Wong M, Khalechelvam P, Tam W. Waist-to-height ratio, body mass index and waist circumference for screening paediatric cardio-metabolic risk factors: a meta-analysis. Obes Rev. (2016) 17:1258–75. doi: 10.1111/obr.12456
49. Pozuelo-Carrascosa DP, Sanchez-Lopez M, Cavero-Redondo I, Torres-Costoso A, Bermejo-Cantarero A, Martinez-Vizcaino V. Obesity as a mediator between cardiorespiratory fitness and blood pressure in preschoolers. J Pediatr. (2017) 182:114–9.e2. doi: 10.1016/j.jpeds.2016.11.005
50. Yang Y, Lau PW, Wang J, Dong B, Wu L, Quach B, et al. Associations among cardiorespiratory endurance, body mass index and blood pressure in Han Chinese children: results from the 2010 Chinese National survey on students' constitution and health. Hypertens Res. (2016) 39:799–804. doi: 10.1038/hr.2016.63
Keywords: cardiorespiratory fitness, fatness, cardiometabolic risk, mediation analysis, moderation analysis
Citation: Shang X, Li Y, Xu H, Zhang Q, Hu X, Liu A, Du S, Li T, Guo H, Li Y, Xu G, Liu W, Ma J and Ma G (2020) Independent and Interactive Associations of Fitness and Fatness With Changes in Cardiometabolic Risk in Children: A Longitudinal Analysis. Front. Endocrinol. 11:342. doi: 10.3389/fendo.2020.00342
Received: 03 March 2020; Accepted: 30 April 2020;
Published: 12 June 2020.
Edited by:
Lucia Pacifico, Sapienza University of Rome, ItalyReviewed by:
Braulio Henrique Magnani Branco, University Center of Maringá (UniCesumar), BrazilCaroline Brand, Universidade de Santa Cruz Do Sul, Brazil
Copyright © 2020 Shang, Li, Xu, Zhang, Hu, Liu, Du, Li, Guo, Li, Xu, Liu, Ma and Ma. This is an open-access article distributed under the terms of the Creative Commons Attribution License (CC BY). The use, distribution or reproduction in other forums is permitted, provided the original author(s) and the copyright owner(s) are credited and that the original publication in this journal is cited, in accordance with accepted academic practice. No use, distribution or reproduction is permitted which does not comply with these terms.
*Correspondence: Guansheng Ma, mags@bjmu.edu.cn
†These authors have contributed equally to this work